- 1Department of Pulmonary Medicine, Shanghai Chest Hospital, Shanghai Jiao Tong University, Shanghai, China
- 2Shanghai Institute of Thoracic Oncology, Shanghai Chest Hospital, Shanghai Jiao Tong University, Shanghai, China
- 3Translational Medical Research Platform for Thoracic Oncology, Shanghai Chest Hospital, Shanghai Jiao Tong University, Shanghai, China
Immunotherapy, a chemotherapy-free process, has emerged as a promising therapeutic strategy to prolong the overall survival (OS) of patients with non-small-cell lung cancer (NSCLC). However, effective stratification factors for immunotherapy remain unclear. The purpose of this study was to discuss the potential stratification factors of NSCLC immunotherapy using immune checkpoint inhibitors (ICIs) by integrating genomic profiling and tumor lesion–type information. In this study, 344 patients with NSCLC, whose clinical and tissue (including metastatic and primary lesions) mutation information was available, were included. The potential gene mutation status for predicting the outcomes of immunotherapy was screened by comparing the difference in mutation frequency between responders and non-responders. Our results indicated that the potential predictors of immunotherapy were significantly different, especially between patients with TP53(+) (including metastatic and primary lesions) and TP53(−) (including metastatic and primary lesions). According to this classification, patients with NSCLC who suggested immunotherapy had a higher OS than those who did not (25 months vs. 7 months, P < 0.0001, hazard ratio = 0.39). Collectively, this study provides a new perspective for screening immunotherapy predictors in NSCLC, suggesting that the TP53 mutation status and source of biopsy tissue should be considered during the development of immunotherapy biomarkers.
Introduction
Non-small-cell lung cancer (NSCLC) is one of the most malignant diseases, accounting for approximately 85% of lung cancer (1–3). Chemotherapy has played an important role in NSCLC treatment (4–6). Since 2009, targeting the tyrosine kinase inhibitors (TKIs) has changed the clinical course for NSCLC patients harboring epidermal growth factor receptor (EGFR) mutations and anaplastic lymphoma kinase (ALK) and proto-oncogene receptor tyrosine kinase (ROS1) rearrangements (7–10). However, for patients without driver gene mutations, the therapeutic regimen remains limited (6). Fortunately, recent advances in immunotherapy have provided new therapeutic targets for lung cancer (4, 11–13).
Immunity checkpoint inhibitors (ICIs), including programmed cell death protein 1 (PD1) and programmed cell death protein 1 ligand 1 (PD-L1) inhibitors, block the PD1/PD-L1 signaling pathway, relieve the immune escape of tumor cells, and kill tumor cells by activating cytotoxic T cells (14–16). Several clinical trials have reported that immunotherapy can significantly improve the overall survival (OS) of patients with NSCLC at first, second, and third lines (4, 11, 17–19). However, some patients in these trials received a long-term OS benefit, whereas others received a short-term OS benefit although all patients were characterized by similar pathological types and received the same ICI (20). These findings signify the urgent need to identify effective stratification factors for immunotherapy.
Several biomarkers, including PD-L1 expression, tumor mutation burden (TMB), and microsatellite instability (MSI), have been developed to distinguish responders to immunotherapy from non-responders in NSCLC (20–25). Among these biomarkers, PD-L1 expression and TMB have been included in National Comprehensive Cancer Network (NCCN) guideline for guiding immunotherapeutic clinical practice (6). However, the above biomarkers are associated with certain limitations (not all patients with a high PD-L1 expression/TMB/MSI responded well to immunotherapy), indicating that biomarker development needs to be explored further (26, 27). In the present study, we screened the immunotherapy stratifying factors through the classification of TP53 mutation status and biopsy lesion type in 344 patients with NSCLC who received immunotherapy.
Materials and Methods
Patients and Samples
This study enrolled 344 NSCLC patients who were approved by the institutional review board of the Memorial Sloan-Kettering Cancer Center (MSKCC) (26). All patients with NSCLC had received at least one cycle of immunotherapy (ICIs such as nivolumab, atezolizumab, ipilimumab, pembrolizumab, avelumab, tremelimumab, and durvalumab). All enrolled patients with NSCLC signed the informed consent for the companion study. Among the 344 patients with NSCLC, we obtained metastatic lesion samples from 176 patients and primary lesion samples from 168 patients. In addition, 217 patients harbored TP53 mutations, and 127 patients did not have this mutation.
Sequencing
The sequencing methods used in the study have been described in detail previously (28). Briefly, DNA was extracted from metastatic and primary lesions, end-repaired, adapter-ligated, and amplified. The quality control for amplified products was performed, following which they were sequenced. The MSK-IMPACT panel was used for targeted sequencing. Somatic tumor mutation calling was performed between the tissue sequencing and white blood cell (WBC) sequencing data. All somatic tumor mutation data and clinical information were downloaded from the cBioPortal for Cancer Genomics (www.cbioportal.org).
Mutation Frequency Analysis
The mutation frequency for the top 30 genes for all 344 patients with NSCLC was calculated. The most significant differences in mutation genes were screened by comparing the mutation frequency between patients with OS >12 months and those with OS ≤12 months. Here, the patients who received immunotherapy with OS >12 months were defined as “responder”; the patients who received immunotherapy with OS ≤12 months were defined as “non-responders”. Furthermore, the mutation frequency between different subgroups was analyzed using samples from metastatic and primary lesions; TP53(+) and TP53(−) patients; TP53(+) patients with metastatic lesions; TP53(+) patients with primary lesions; TP53(−) patients with metastatic lesions; and TP53(−) patients with primary lesions.
OS Analysis
The OS analysis was performed according to the methods described in our previous studies (5, 29, 30). We compared the mutation frequencies between different subgroups to select different predictors for stratification. GraphPad Prism 5 software was used to calculate the differences between different subgroups. The log-rank test was used to analyze significant differences (P values) between different cohorts. Hazard ratios (HRs) were calculated for OS.
Statistical Analysis
The log-rank (Mantel-Cox) test was used to test the difference of survival time between different cohorts. In addition, HRs and exact 95% confidence intervals (CIs) were reported. Differences were considered significant at *p < 0.05, **p < 0.01, and ***p < 0.001.
Results
The Mutational Differences Between Responders and Non-Responders Potentially Be Used as Predictor in NSCLC Immunotherapy
In this study, 344 patients with NSCLC (with clinical and mutation information) were screened to identify immunotherapy predictors, from an MSKCC cohort (1,661 cancer patients including NSCLC, melanoma, glioma, and colorectal cancer) (Figure 1). As shown in Figure 2A, in the present cohort, patients harboring TP53 mutations accounted for more than 60% of cases, followed by KRAS, STK11, and KEAP1. Next, the top 30 genes with mutation frequency were selected for further analysis. Our results indicated a significant difference in the mutation frequency between patients with OS ≤12 months and those with OS >12 months. Next, we calculated the ratios of mutation frequency for the top 30 genes and obtained an altered trend chart (Figure 2B). The top five altered genes, namely, ARID1A, ZFHX3, ATM, ARID2, and NTRK3, were named AZAAN. Therefore, we evaluated the effect of the predictor-AZAAN on responsive stratification in patients who had received immunotherapy. The results indicated that patients harboring AZAAN(+) received more OS benefits from immunotherapy than those patients harboring AZAAN(−) [AZAAN(+) vs. AZAAN(−): 22 months vs. 10 months, log-rank P value = 0.0006, HR = 0.59] (Figure 2C). TMB can be used as a predictor for immunotherapy response. As shown in Figure 2D, the log-rank P-value and HR of the predictor TMB (TMB ≥ 14) were superior to those of the predictor-AZAAN. However, either the predictor TMB or the predictor AZAAN just identified a small proportion of patients (no more than 28%) who were suggested to receive immunotherapy, indicating that immunotherapy predictors of NSCLC need to be further explored.
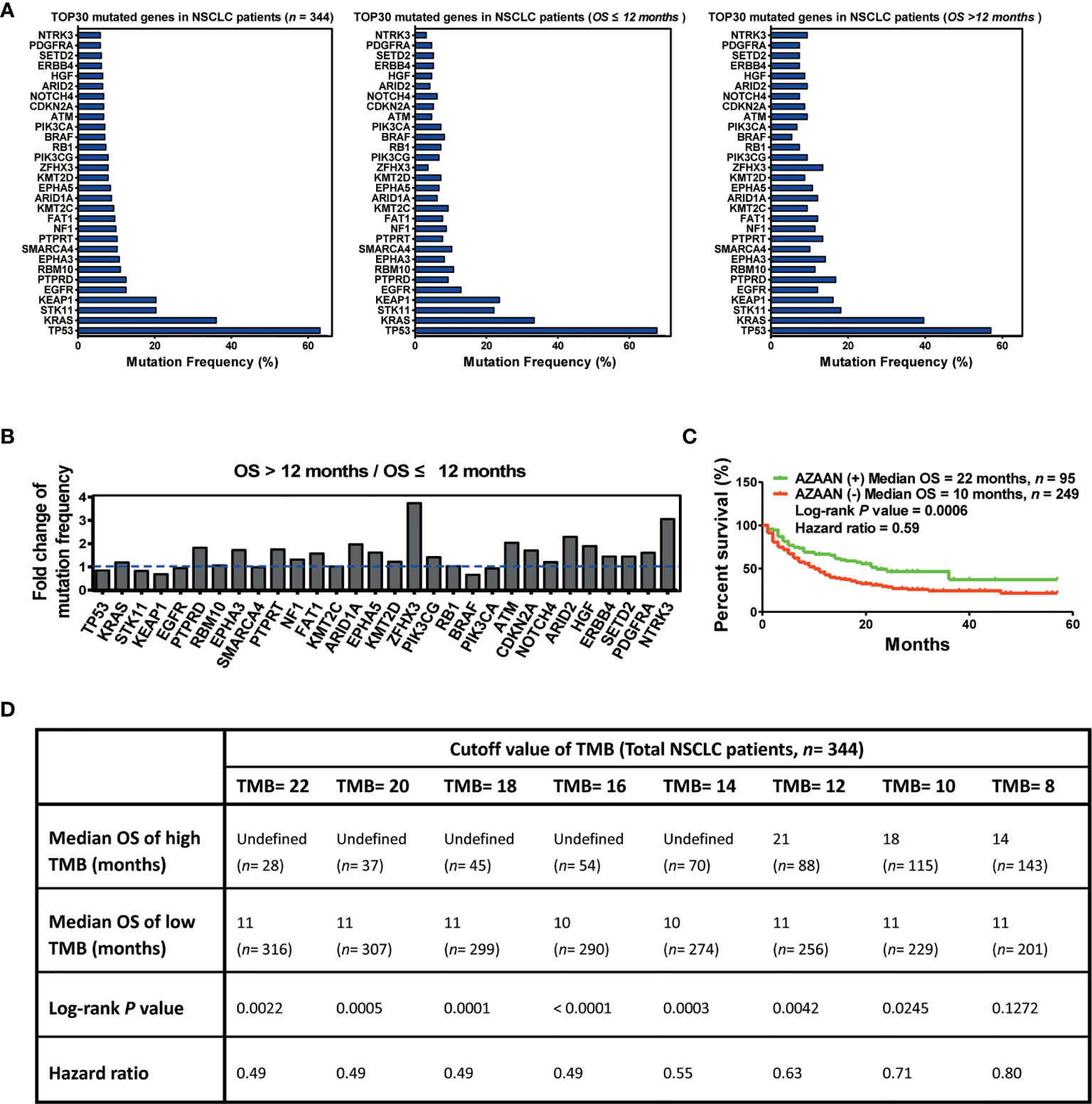
Figure 2 AZAAN mutation status as a stratifying predictor of immunotherapy in NSCLC. (A) Left: Mutation frequency of the top 30 genes. Middle: Mutation frequency of the top 30 genes for patients with NSCLC having OS ≤12 months. Right: Mutation frequency of the top 30 genes for patients with NSCLC having OS >12 months. (B) Fold change in mutation frequency with OS >12 months/OS ≤12 months for the top 30 genes. (C) Kaplan–Meier curve analysis of OS stratification using the AZAAN (ARID1A, ZFHX3, ATM, ARID2, and NTRK3) mutation status. (D) Different TMB cutoffs used as a predictor for immunotherapy.
Mutation Profiling From Different Biopsy Lesions Determine the Predictor Screening
To further understand the differences between primary and metastatic lesions, we divided 334 patients into two cohorts, namely, primary and metastatic sample cohorts. A comparison of two cohorts revealed that the mutation frequencies of the top 30 genes were significantly different between them. In addition, the mutation frequency of multiple genes changed remarkably between the OS >12 months cohort and the OS ≤12 months cohort in the metastatic sample cohort. The top five upregulated genes (AZACN: ARID1A, ZFHX3, ATM, CDKN2A, and NTRK3) and the bottom two downregulated genes (BRAF and PIK3CA) were selected as combined predictors for screening responders from non-responders. The results suggested that patients harboring AZACN(+) received more OS benefits from immunotherapy than those harboring AZACN(−) or harboring BRAF and PIK3CA (+) [AZACN(+) vs. AZACN(−) vs. BRAF and PIK3CA (+) = undefined vs. 9 months vs. 8 months] (Figure 3A). In the primary sample cohort, the top six upregulated genes (ZPAHPN: ZFHX3, PIK3CA, ARID2, HGF, PDGFRA, and NTRK3) and the bottom downregulated gene (KEAP1) were selected as combined predictors for screening responders from non-responders. The results indicated that patients harboring ZPAHPN(+) received more OS benefits than those harboring ZPAHAN(−) or KEAP1(+) [ZPAHPN(+) vs. ZPAHAN(−) vs. KEAP1(+) = 36 months vs. 13 months vs. 6 months] (Figure 3B). These results suggest that biopsy lesion type potentially affects biomarker screening for immunotherapy.
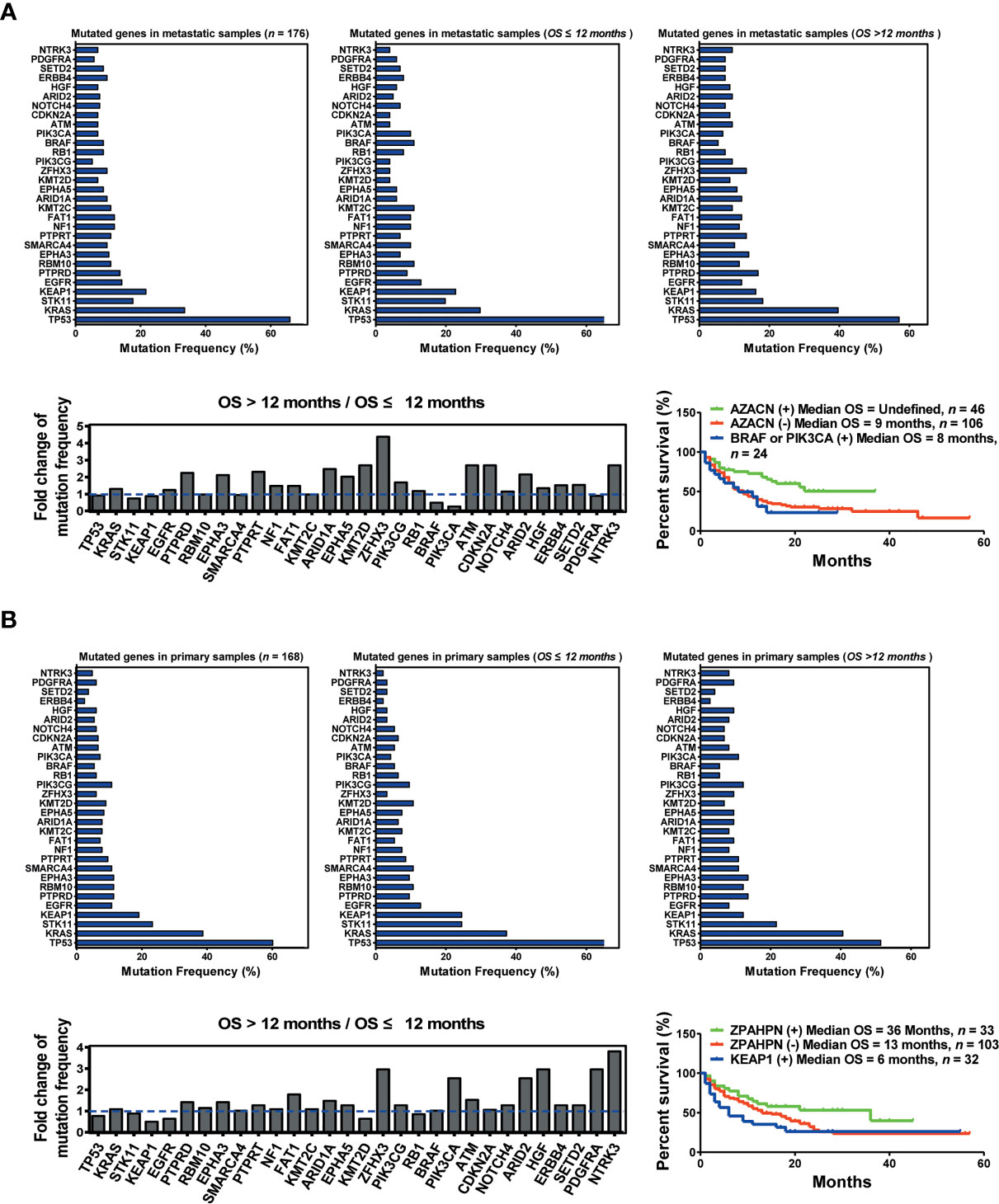
Figure 3 Biopsy lesion type affects the stratifying factors of immunotherapy. (A) Up left: Mutation frequency of the top 30 genes in the metastatic sample cohort. Upper middle: Mutation frequency of the top 30 genes in the metastatic sample cohort with OS ≤12 months. Upper right: Mutation frequency of the top 30 genes in the metastatic sample cohort with OS >12 months. Down left: Fold change in mutation frequency with OS >12 months/OS ≤12 months for the top 30 genes. Down right: Kaplan–Meier curve analysis of OS stratification using the AZACN (ARID1A, ZFHX3, ATM, CDKN2A, and NTRK3) mutation status. (B) Upper left: Mutation frequency of the top 30 genes in the primary sample cohort. Upper middle: Mutation frequency of the top 30 genes in the primary sample cohort with OS ≤12 months. Upper right: Mutation frequency of the top 30 genes in the primary sample cohort with OS >12 months. Down left: Fold change in mutation frequency with OS >12 months/OS ≤12 months for the top 30 genes. Down right: Kaplan–Meier curve analysis of OS stratification using the ZPAHPN (ZFHX3, PIK3CA, ARID2, HGF, PDGFRA, and NTRK3) mutation status.
Effect of Biopsy Lesion Types on Predictor Development in the TP53(+) Patients
To precisely screen the potential responders of immunotherapy via DNA profiling, we performed an integrated analysis based on TP53 mutation status as well as the biopsy lesion type. We found a significant difference in the mutation frequency of the top 30 genes between patients harboring TP53(+) and those harboring TP53(−). For patients harboring TP53(+), the top five upregulated genes (ZACNN: ZFHX3, ATM, CDKN2A, NOTCH4, and NTRK3) were selected as predictors for screening responders from non-responders. The results indicated that patients harboring ZACNN(+) received more OS benefits from immunotherapy than those harboring ZACNN(−) [ZACNN(+) vs. ZACNN(−) = undefined vs. 8 months, P < 0.0001] (Figure 4A). Using this stratification method, about 28.6% of TP53(+) patients were screened for immunotherapy recommendation. Furthermore, 217 patients harboring TP53(+) were divided into two cohorts according to the biopsy lesion type (metastatic sample and primary sample cohorts). In the metastatic sample cohort (116 patients), the top five upregulated genes (PKZAC: PTPRT, KMT2D, ZFHX3, ATM, and CDKN2A) were selected as predictors to screen the responders. Patients harboring PKZAC(+) received more OS benefits from immunotherapy than those harboring PKZAC(−) [PKZAC(+) vs. PKZAC(−) = 22 months vs. 7 months, P = 0.0008] (Figure 4B). In the primary sample cohort (101 patients), the top six upregulated genes (ZANHPN: ZFHX3, ATM, NOTCH4, HGF, PDGFRA, and NTRK3) were selected as predictors for screening responders from non-responders. The patients harboring ZANHPN(+) received more OS benefits from immunotherapy than those harboring ZANHPN(−) [ZANHPN(+) vs. ZANHPN(−) = 29 months vs. 8 months, P = 0.0005] (Figure 4C).
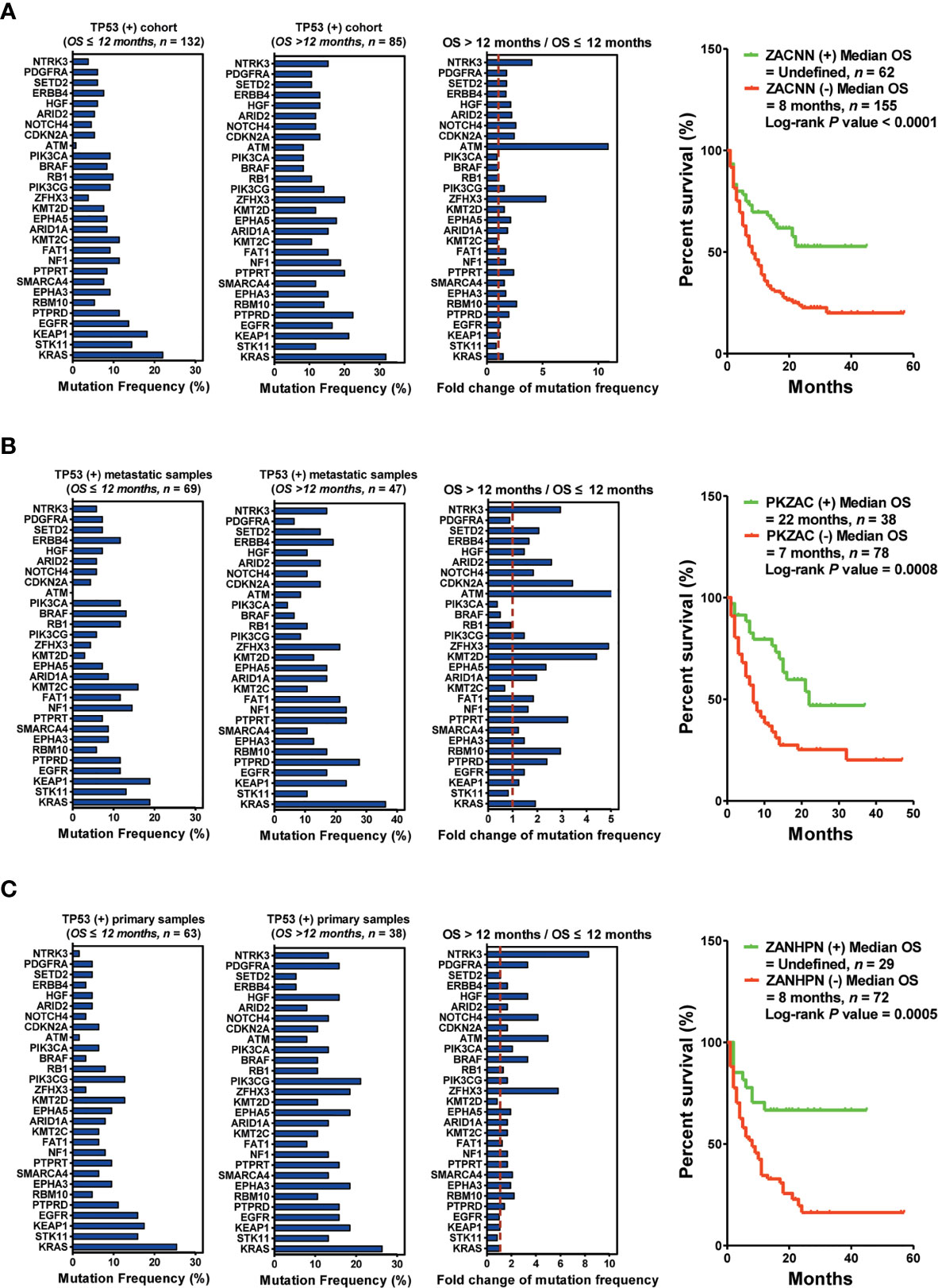
Figure 4 Biopsy lesion type affects the stratifying factors of immunotherapy in patients harboring TP53(+) mutation. (A) Left: Mutation frequency of the top 30 genes in the TP53(+) cohort with OS ≤12 months. Mutation frequency of the top 30 genes in the TP53(+) cohort with OS >12 months. Fold change in mutation frequency with OS >12 months/OS ≤12 months for the top 30 genes. Right: Kaplan–Meier curve analysis of OS stratification using the ZACNN (ZFHX3, ATM, CDKN2A, NOTCH4, and NTRK3) mutation status. (B) Left: Mutation frequency of the top 30 genes in the TP53(+) metastatic sample cohort with OS ≤12 months. Mutation frequency of the top 30 genes in the TP53(+) metastatic sample cohort with OS >12 months. Fold change in mutation frequency with OS >12 months/OS ≤12 months for the top 30 genes. Right: Kaplan–Meier curve analysis of OS stratification using the PKZAC (PTPRT, KMT2D, ZFHX3, ATM, and CDKN2A) mutation status. (C) Left: Mutation frequency of the top 30 genes in the TP53(+) primary sample cohort with OS ≤12 months. Mutation frequency of the top 30 genes in the TP53(+) primary sample cohort with OS >12 months. Fold change in mutation frequency with OS >12 months/OS ≤12 months for the top 30 genes. Right: Kaplan–Meier curve analysis of OS stratification using the ZANHPN (ZFHX3, ATM, NOTCH4, HGF, PDGFRA, and NTRK3) mutation status.
Effect of Biopsy Lesion Types on Predictor Development in the TP53(−) Patients
Next, 127 patients without TP53 mutations were subjected to another set of analyses. The bottom three downregulated genes (KBN: KEAP1, BRAF, and NOTCH4) were selected as predictors for screening responders from non-responders. The results indicated that the patients harboring KBN(−) received more OS benefits from immunotherapy than those harboring KBN(+) [KBN(−) vs. KBN(+) = 21 months vs. 6 months, P < 0.0001] (Figure 5A). In the metastatic sample cohort (60 patients), the bottom four downregulated genes (KRPN: KEAP1, RBM10, PIK3CA, and NOTCH4) were selected as predictors for screening responders from non-responders. Patients harboring KRPN(−) received more OS benefits from immunotherapy than those harboring KRPN(+) [KRPN(−) vs. KRPN(+) = 26 months vs. 6 months, P = 0.0064] (Figure 5B). In the primary sample cohort (67 patients), the bottom three downregulated genes (KEN: KEAP1, EGFR, and NOTCH4) were selected as predictors for screening responders from non-responders. Patients harboring KEN(−) received more OS benefits from immunotherapy than those harboring KEN(+) [KEN(−) vs. KEN(+) = 23 months vs. 6 months, P = 0.0003] (Figure 5C).
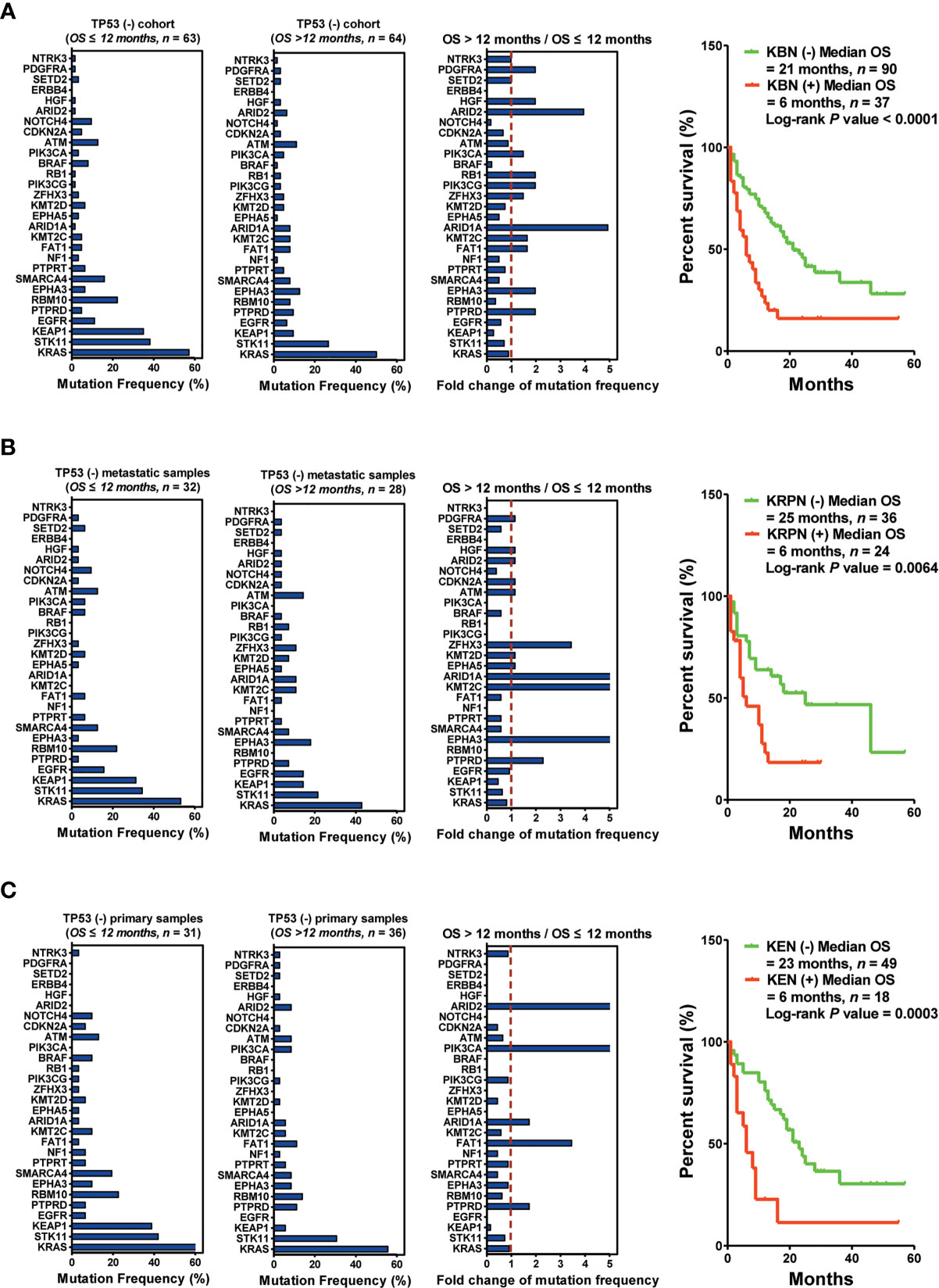
Figure 5 Biopsy lesion type affects the stratifying factors of immunotherapy in patients without TP53(+) mutation. (A) Left: Mutation frequency of the top 30 genes in the TP53(−) cohort with OS ≤12 months. Mutation frequency of the top 30 genes in the TP53(−) cohort with OS >12 months. Fold change in mutation frequency with OS >12 months/OS ≤12 months for the top 30 genes. Right: Kaplan–Meier curve analysis of OS stratification using the KBN (KEAP1, BRAF, and NOTCH4) mutation status. (B) Left: Mutation frequency of the top 30 genes in the TP53(−) metastatic sample cohort with OS ≤12 months. Mutation frequency of the top 30 genes in the TP53(−) metastatic sample cohort with OS >12 months. Fold change in mutation frequency with OS >12 months/OS ≤12 months for the top 30 genes. Right: Kaplan–Meier curve analysis of OS stratification using the KRPN (KEAP1, RBM10, PIK3CA, and NOTCH4) mutation status. (C) Left: Mutation frequency of the top 30 genes in the TP53(−) primary sample cohort with OS ≤12 months. Mutation frequency of the top 30 genes in the TP53(−) primary sample cohort with OS >12 months. Fold change in mutation frequency with OS >12 months/OS ≤12 months for the top 30 genes. Right: Kaplan–Meier curve analysis of OS stratification using the KEN (KEAP1, EGFR, and NOTCH4) mutation status.
Integration of TP53 Mutation Status and Biopsy Lesion Types for Predictor Development in Immunotherapy
Here, we observed an interesting phenomenon, that is, the predictors derived from TP53(+) patients were commonly used to screen responders, whereas those derived from TP53(−) patients were used to screen non-responders. Collectively, we performed a multiple classification analysis on 217 patients with TP53(−) and 127 patients with TP53(+), as well as the source of tissue, and identified four predictors (PKZAC, ZANHPN, KEN, and KRPN). Next, we provided stratifying management for patients receiving immunotherapy. Among the 344 patients with NSCLC, 152 patients were proposed to receive immunotherapy with a median OS of 25 months, and 192 patients were proposed not to receive immunotherapy with a median OS of 7 months (P < 0.0001, HR = 0.39) (Figure 6). Approximately 44.2% of patients were recommended to receive immunotherapy, with a reduced death risk of 61%. Collectively, the TP53 mutation status and biopsy lesion type potentially determined the stratifying pattern of immunotherapy.
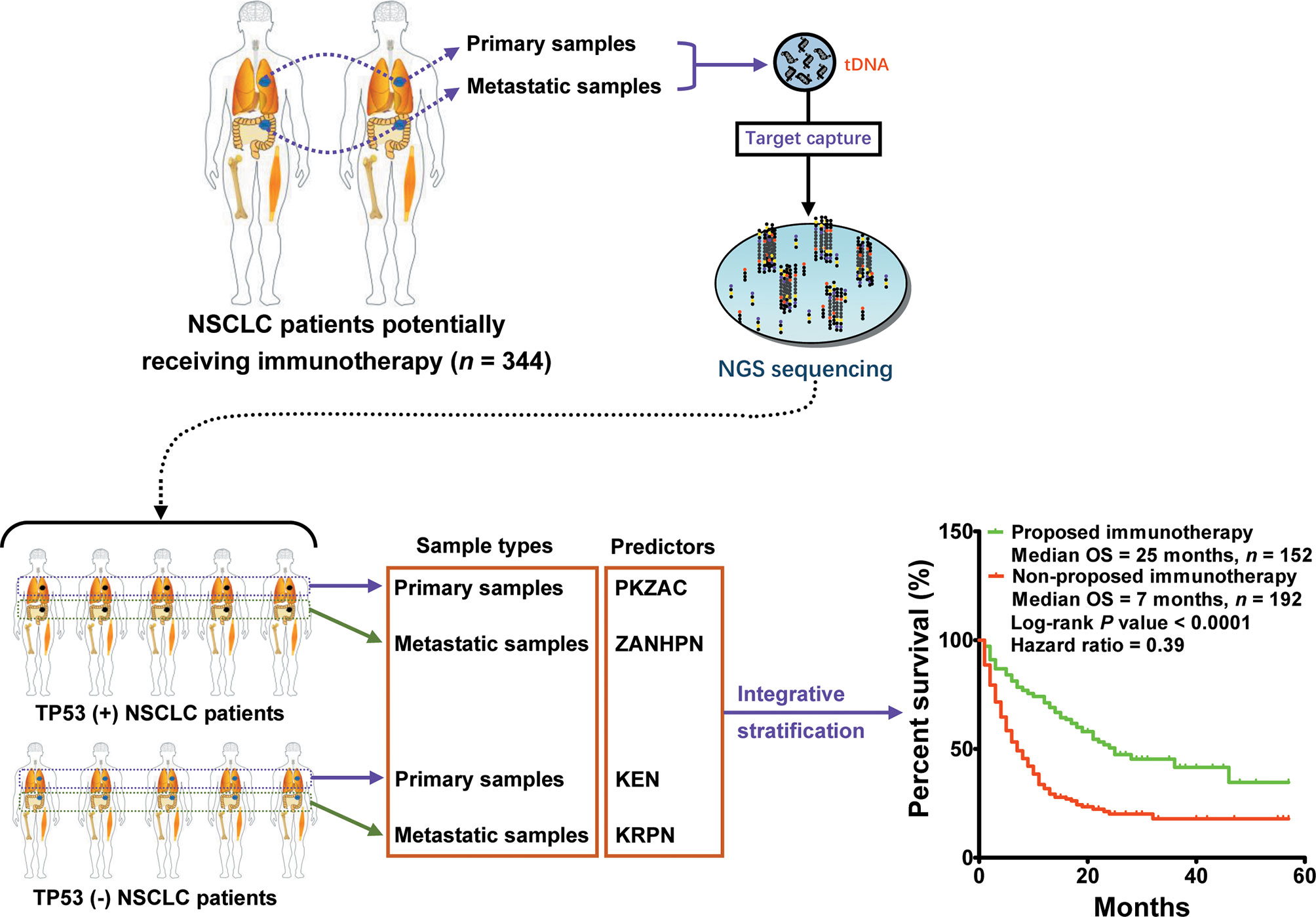
Figure 6 Integrative stratification using different TP53 mutation status and biopsy lesion types for immunotherapy in NSCLC. Samples from different lesions (metastatic and primary lesions) were subjected to standard high-throughput sequencing. The mutation profile of each patient was used for driver gene-based subtype analysis. In total, 344 patients with NSCLC were divided into two cohorts: [TP53(+) cohort and TP53(−) cohort]. According to biopsy lesion type, NSCLC patients were further divided into TP53(+) metastatic sample cohort, TP53(+) primary sample cohort, TP53(−) metastatic sample cohort, and TP53(−) primary sample cohort. Each cohort developed an independent optimal predictor. Patients who potentially received more OS benefits from immunotherapy were screened by integrative stratification.
Discussion
Immunotherapy is a novel therapeutic regimen that functions by blocking the PD1/PD-L1 signaling pathway, relieving the immune escape of tumor cells, and activating cytotoxic T cells. It has been demonstrated to play a critical role in NSCLC treatment (4, 6, 11–13). However, the effective stratifying factors for immunotherapy remain unclear. In the present study, 344 patients with NSCLC, whose clinical and mutation information was available, were enrolled to screen potential stratifying factors for immunotherapy.
Patients with a high PD-L1 expression in tumor tissue received more OS benefits from immunotherapy (22). This theory is beyond reproach because the immune escape of tumor cells is based on the activation of the PD1/PD-L1 signaling pathway (13, 14). The patients harboring higher expression of PD-L1 causes a greater response to ICIs. Based on the PD-L1 predictor, multiple important clinical trials of ICIs have achieved the OS endpoint (4, 11, 17). Therefore, PD-L1 plays a pioneering role in promoting the clinical practice of immunotherapy (22). Nevertheless, further studies found that not all patients with a high PD-L1 expression responded well to immunotherapy, and not all patients without PD-L1 expression not responded to immunotherapy (31–34). This phenomenon has motivated the researchers to screen new predictors that can be used for clinical stratification of immunotherapy. In 2015, Rizvi et al. first proposed that tumor mutation load could potentially be used for stratification of immunotherapy in NSCLC (24). They believed that numerous somatic mutations encoded multiple neoantigens, which determined the response of patients to ICIs (24). The predictor TMB was demonstrated to be effective in several subsequent studies (21, 31, 35). However, similar to PD-L1, not all patients with a high TMB showed a good response to immunotherapy or not all patients with low or moderate TMB responded to immunotherapy (31, 36, 37). These findings led the researchers to believe that TMB is not an enough effective predictor for immunotherapy (27). In addition, MSI can be regarded as a candidate predictor for stratification of immunotherapy (25). Altogether, the above predictors (PD-L1, TMB, and MSI) play an important role in the development of immunotherapy.
We found that the predictors (PD-L1, TMB, and MSI) were independent of TP53 mutation status and the source of biopsy tissue. Based on existing evidence, there may be great differences in tumor biology between patients with NSCLC harboring TP53 mutations and those without TP53 mutations, and the mutation profiling of metastatic lesions may differ from that of primary lesions (38–43). In the present study, we found that the predictor AZAAN potentially guided the stratification of immunotherapy, regardless of the tissue source and TP53 mutation status. These results suggest that a combination of mutated genes can potentially be used as a predictor for immunotherapy by comparing the mutation frequency between responders and non-responders. However, the mutation landscape of metastatic lesions is different from that of primary lesions. Whether these differences determine the response rate to immunotherapy remains unclear. Therefore, we subdivided the 344 patients’ cohort into two cohorts (metastatic sample cohort and primary sample cohort) according to the source of biopsy tissue and performed predictor screening analysis. Interestingly, the results demonstrated a significant difference in predictors between the metastatic and primary sample cohorts. These results indicate that the biopsy lesion type should be considered during mutation profiling analysis to screen the predictors of immunotherapy.
Based on the mutational difference between metastatic and primary lesions, as well as the TP53-affected tumor biology difference, whether the TP53 mutation status combined with the biopsy lesion type is associated with the predictor of immunotherapy remains unclear. Previous studies have shown a higher TP53 mutation frequency in metastatic lesions than in primary lesions, and patients harboring TP53 mutations potentially receiving more OS benefits from immunotherapy (41). In the present cohort, more than 60% of patients with NSCLC harbored TP53 mutations. Among these patients, the metastatic and primary sample cohorts were included. After predictor screening, we found that the predictor of PKZAC for TP53(+) metastatic sample cohort and the predictor of ZANHPN for TP53(+) primary sample cohort could potentially be used for stratification of immunotherapy. For the TP53(−) cohort, the predictor changed to KRPN in the TP53(−) metastatic sample cohort and KEN in the TP53(−) primary sample cohort. These results indicate that the optimal predictor differs according to TP53 mutation status and biopsy lesion type. In addition, previous studies reported that patients harboring KEAP1 or STK11 mutations received shorter OS benefits from immunotherapy (44, 45). Our results provide a new perspective on this issue. We did not observe a difference in the OS for patients harboring TP53 mutations, regardless of KEAP1 and STK11 mutations, after receiving immunotherapy. If the TP53(−) patients harbor KEAP1 and STK11 mutations, the OS is remarkably shorter than those patients without KEAP1 and STK11 mutations, after receiving immunotherapy. One of the limitations of the study was the small sample size, especially in the TP53(−) cohort. In the future, a larger cohort should be collected to validate the phenomena discovered in this study.
Collectively, this study provides a novel perspective for the stratification of immunotherapy via mutational profiling in patients with NSCLC and suggests that TP53 mutation status, as well as the biopsy lesion type, determines the difference in immunotherapy predictors.
Data Availability Statement
The original contributions presented in the study are included in the article/supplementary material. Further inquiries can be directed to the corresponding authors.
Author Contributions
Experiments were conceived and designed by BH, WZ, HW, and JL. Clinical analysis, bioinformatics analysis, and statistical analysis were performed by JL, RZ, YL, BZ, MH, YW, YC, ZY, and WZ. Figures and tables were generated by JL, RZ, and YL, and the manuscript was written by JL. The manuscript was revised by BH. All authors contributed to the article and approved the submitted version.
Funding
This work was supported by the foundation of Shanghai Chest Hospital (Project Nos. 2019YNJCM11 and YJXT20190102); the Shanghai Leading Talents Program (2013), the Shanghai Jiao Tong University (Project Nos. 15ZH4009 and YG2021QN121); the key program of translational medicine from Shanghai Jiao Tong University School of Medicine (Project No. 15ZH1008); the foundation of Chinese Society of Clinical Oncology (Project Nos. Y-2019AZZD-0355 and Y-QL2019-0125); National Natural Science Foundation of China grants (Project No. 31801118).
Conflict of Interest
The authors declare that the research was conducted in the absence of any commercial or financial relationships that could be construed as a potential conflict of interest.
Publisher’s Note
All claims expressed in this article are solely those of the authors and do not necessarily represent those of their affiliated organizations, or those of the publisher, the editors and the reviewers. Any product that may be evaluated in this article, or claim that may be made by its manufacturer, is not guaranteed or endorsed by the publisher.
Acknowledgments
The authors thank the patients for their participation in MSKCC, and the investigators for releasing the sequencing data and clinical data..
References
1. Lou Y, Xu J, Zhang Y, Zhang W, Zhang X, Gu P, et al. Akt Kinase LANCL2 Functions as a Key Driver in EGFR-Mutant Lung Adenocarcinoma Tumorigenesis. Cell Death Dis (2021) 12:170. doi: 10.1038/s41419-021-03439-8
2. Zhang LL, Lu J, Liu RQ, Hu MJ, Zhao YM, Tan S, et al. Chromatin Accessibility Analysis Reveals That TFAP2A Promotes Angiogenesis in Acquired Resistance to Anlotinib in Lung Cancer Cells. Acta Pharmacol Sin (2020) 41:1357–65. doi: 10.1038/s41401-020-0421-7
3. Lu J, Zhong H, Chu T, Zhang X, Li R, Sun J, et al. Role of Anlotinib-Induced CCL2 Decrease in Anti-Angiogenesis and Response Prediction for Nonsmall Cell Lung Cancer Therapy. Eur Respir J (2019) 53:1801568. doi: 10.1183/13993003.01562-2018
4. Brahmer J, Reckamp KL, Baas P, Crino L, Eberhardt WE, Poddubskaya E, et al. Nivolumab Versus Docetaxel in Advanced Squamous-Cell Non-Small-Cell Lung Cancer. N Engl J Med (2015) 373:123–35. doi: 10.1056/NEJMoa1504627
5. Chu T, Lu J, Bi M, Zhang H, Zhuang W, Yu Y, et al. Equivalent Efficacy Study of QL1101 and Bevacizumab on Untreated Advanced Non-Squamous Non-Small Cell Lung Cancer Patients: A Phase 3 Randomized, Double-Blind Clinical Trial. Cancer Biol Med (2021) 18:816–24. doi: 10.20892/j.issn.2095-3941.2020.0212
6. Ettinger DS, Wood DE, Aisner DL, Akerley W, Bauman JR, Bharat A, et al. NCCN Guidelines Insights: Non-Small Cell Lung Cancer, Version 2.2021. J Natl Compr Canc Netw (2021) 19:254–66. doi: 10.6004/jnccn.2021.0013
7. Mok TS, Wu YL, Thongprasert S, Yang CH, Chu DT, Saijo N, et al. Gefitinib or Carboplatin-Paclitaxel in Pulmonary Adenocarcinoma. N Engl J Med (2009) 361:947–57. doi: 10.1056/NEJMoa0810699
8. Nie W, Tang L, Zhang H, Shao J, Wang Y, Chen L, et al. Structural Analysis of the EGFR TK Domain and Potential Implications for EGFR Targeted Therapy. Int J Oncol (2012) 40:1763–9. doi: 10.3892/ijo.2012.1356
9. Peters S, Camidge DR, Shaw AT, Gadgeel S, Ahn JS, Kim DW, et al. Alectinib Versus Crizotinib in Untreated ALK-Positive Non-Small-Cell Lung Cancer. N Engl J Med (2017) 377:829–38. doi: 10.1056/NEJMoa1704795
10. Patil T, Simons E, Mushtaq R, Pacheco JM, Doebele RC, Bowles DW. Targeted Therapies for ROS1-Rearranged Non-Small Cell Lung Cancer. Drugs Today (Barc) (2019) 55:641–52. doi: 10.1358/dot.2019.55.10.3030646
11. Rittmeyer A, Barlesi F, Waterkamp D, Park K, Ciardiello F, von Pawel J, et al. Atezolizumab Versus Docetaxel in Patients With Previously Treated Non-Small-Cell Lung Cancer (OAK): A Phase 3, Open-Label, Multicentre Randomised Controlled Trial. Lancet (2017) 389:255–65. doi: 10.1016/S0140-6736(16)32517-X
12. Gadgeel S, Rodriguez-Abreu D, Speranza G, Esteban E, Felip E, Domine M, et al. Updated Analysis From KEYNOTE-189: Pembrolizumab or Placebo Plus Pemetrexed and Platinum for Previously Untreated Metastatic Nonsquamous Non-Small-Cell Lung Cancer. J Clin Oncol (2020) 38:1505–17. doi: 10.1200/JCO.19.03136
13. Pinheiro FD, Teixeira AF, de Brito BB, da Silva FAF, Santos MLC, de Melo FF, et al. Immunotherapy - New Perspective in Lung Cancer. World J Clin Oncol (2020) 11:250–9. doi: 10.5306/wjco.v11.i5.250
14. Kline J, Gajewski TF. Clinical Development of Mabs to Block the PD1 Pathway as an Immunotherapy for Cancer. Curr Opin Investig Drugs (2010) 11:1354–9.
15. Kennedy LB, Salama AKS. A Review of Cancer Immunotherapy Toxicity. CA Cancer J Clin (2020) 70:86–104. doi: 10.3322/caac.21596
16. O’Donnell JS, Teng MWL, Smyth MJ. Cancer Immunoediting and Resistance to T Cell-Based Immunotherapy. Nat Rev Clin Oncol (2019) 16:151–67. doi: 10.1038/s41571-018-0142-8
17. Gandhi L, Rodriguez-Abreu D, Gadgeel S, Esteban E, Felip E, De Angelis F, et al. Pembrolizumab Plus Chemotherapy in Metastatic Non-Small-Cell Lung Cancer. N Engl J Med (2018) 378:2078–92. doi: 10.1056/NEJMoa1801005
18. Nasser NJ, Gorenberg M, Agbarya A. First Line Immunotherapy for Non-Small Cell Lung Cancer. Pharmaceuticals (Basel) (2020) 13:373. doi: 10.3390/ph13110373
19. Bozorgmehr F, Hommertgen A, Krisam J, Lasitschka F, Kuon J, Maenz M, et al. Fostering Efficacy of Anti-PD-1-Treatment: Nivolumab Plus Radiotherapy in Advanced Non-Small Cell Lung Cancer - Study Protocol of the FORCE Trial. BMC Cancer (2019) 19:1074. doi: 10.1186/s12885-019-6205-0
20. Gibney GT, Weiner LM, Atkins MB. Predictive Biomarkers for Checkpoint Inhibitor-Based Immunotherapy. Lancet Oncol (2016) 17:e542–51. doi: 10.1016/S1470-2045(16)30406-5
21. Chan TA, Yarchoan M, Jaffee E, Swanton C, Quezada SA, Stenzinger A, et al. Development of Tumor Mutation Burden as an Immunotherapy Biomarker: Utility for the Oncology Clinic. Ann Oncol (2019) 30:44–56. doi: 10.1093/annonc/mdy495
22. Patel SP, Kurzrock R. PD-L1 Expression as a Predictive Biomarker in Cancer Immunotherapy. Mol Cancer Ther (2015) 14:847–56. doi: 10.1158/1535-7163.MCT-14-0983
23. Fumet JD, Truntzer C, Yarchoan M, Ghiringhelli F. Tumour Mutational Burden as a Biomarker for Immunotherapy: Current Data and Emerging Concepts. Eur J Cancer (2020) 131:40–50. doi: 10.1016/j.ejca.2020.02.038
24. Rizvi NA, Hellmann MD, Snyder A, Kvistborg P, Makarov V, Havel JJ, et al. Cancer Immunology. Mutational Landscape Determines Sensitivity to PD-1 Blockade in Non-Small Cell Lung Cancer. Science (2015) 348:124–8. doi: 10.1126/science.aaa1348
25. Chang L, Chang M, Chang HM, Chang F. Microsatellite Instability: A Predictive Biomarker for Cancer Immunotherapy. Appl Immunohistochem Mol Morphol (2018) 26:e15–21. doi: 10.1097/PAI.0000000000000575
26. Samstein RM, Lee CH, Shoushtari AN, Hellmann MD, Shen R, Janjigian YY, et al. Tumor Mutational Load Predicts Survival After Immunotherapy Across Multiple Cancer Types. Nat Genet (2019) 51:202–6. doi: 10.1038/s41588-018-0312-8
27. McGrail DJ, Pilie PG, Rashid NU, Voorwerk L, Slagter M, Kok M, et al. High Tumor Mutation Burden Fails to Predict Immune Checkpoint Blockade Response Across All Cancer Types. Ann Oncol (2021) 32:661–72. doi: 10.1016/j.annonc.2021.02.006
28. Hugo W, Zaretsky W, Sun W, Song W, Moreno W, Hu-Lieskovan W, et al. Genomic and Transcriptomic Features of Response to Anti-PD-1 Therapy in Metastatic Melanoma. Cell (2016) 165:35–44. doi: 10.1016/j.cell.2016.02.065
29. Lu J, Zhong H, Wu J, Chu T, Zhang L, Li H, et al. Circulating DNA-Based Sequencing Guided Anlotinib Therapy in Non-Small Cell Lung Cancer. Adv Sci (Weinh) (2019) 6:1900721. doi: 10.1002/advs.201900721
30. Lu J, Shi Q, Zhang L, Wu J, Lou Y, Qian J, et al. Integrated Transcriptome Analysis Reveals KLK5 and L1CAM Predict Response to Anlotinib in NSCLC at 3rd Line. Front Oncol (2019) 9:886. doi: 10.3389/fonc.2019.00886
31. Gandara DR, Paul SM, Kowanetz M, Schleifman E, Zou W, Li Y, et al. Blood-Based Tumor Mutational Burden as a Predictor of Clinical Benefit in Non-Small-Cell Lung Cancer Patients Treated With Atezolizumab. Nat Med (2018) 24:1441–8. doi: 10.1038/s41591-018-0134-3
32. Reck M, Rodriguez-Abreu D, Robinson AG, Hui R, Csoszi T, Fulop A, et al. Pembrolizumab Versus Chemotherapy for PD-L1-Positive Non-Small-Cell Lung Cancer. N Engl J Med (2016) 375:1823–33. doi: 10.1056/NEJMoa1606774
33. Carbone DP, Reck M, Paz-Ares L, Creelan B, Horn L, Steins M, et al. First-Line Nivolumab in Stage IV or Recurrent Non-Small-Cell Lung Cancer. N Engl J Med (2017) 376:2415–26. doi: 10.1056/NEJMoa1613493
34. Garon EB. Cancer Immunotherapy Trials Not Immune From Imprecise Selection of Patients. N Engl J Med (2017) 376:2483–5. doi: 10.1056/NEJMe1705692
35. Ready N, Hellmann MD, Awad MM, Otterson GA, Gutierrez M, Gainor JF, et al. First-Line Nivolumab Plus Ipilimumab in Advanced Non-Small-Cell Lung Cancer (CheckMate 568): Outcomes by Programmed Death Ligand 1 and Tumor Mutational Burden as Biomarkers. J Clin Oncol (2019) 37:992–1000. doi: 10.1200/JCO.18.01042
36. Nie W, Qian J, Xu MD, Gu K, Qian FF, Hu MJ, et al. A Non-Linear Association Between Blood Tumor Mutation Burden and Prognosis in NSCLC Patients Receiving Atezolizumab. Oncoimmunology (2020) 9:1731072. doi: 10.1080/2162402X.2020.1731072
37. Nie W, Qian J, Zhang B, Zhong H, Han B. Tumour Mutational Burden in Treatment-Resistant Tumours. Lancet Oncol (2020) 21:e551. doi: 10.1016/S1470-2045(20)30617-3
38. Mogi A, Kuwano H. TP53 Mutations in Nonsmall Cell Lung Cancer. J BioMed Biotechnol (2011) 2011:583929. doi: 10.1155/2011/583929
39. Jamal-Hanjani M, Wilson GA, McGranahan N, Birkbak NJ, Watkins TBK, Veeriah S, et al. Tracking the Evolution of Non-Small-Cell Lung Cancer. N Engl J Med (2017) 376:2109–21. doi: 10.1056/NEJMoa1616288
40. Bailey MH, Tokheim C, Porta-Pardo E, Sengupta S, Bertrand D, Weerasinghe A, et al. Comprehensive Characterization of Cancer Driver Genes and Mutations. Cell (2018) 173:371–85.e318.
41. Birkbak NJ, McGranahan N. Cancer Genome Evolutionary Trajectories in Metastasis. Cancer Cell (2020) 37:8–19. doi: 10.1016/j.ccell.2019.12.004
42. Priestley P, Baber J, Lolkema MP, Steeghs N, de Bruijn E, Shale C, et al. Pan-Cancer Whole-Genome Analyses of Metastatic Solid Tumours. Nature (2019) 575:210–6. doi: 10.1038/s41586-019-1689-y
43. Lu J, Zhang YW, Lou YQ, Yan B, Zou BK, Hu MJ, et al. ctDNA-Profiling-Based UBL Biological Process Mutation Status as a Predictor of Atezolizumab Response Among TP53 Negative NSCLC Patients. Front Genet (2021) 12:723670. doi: 10.3389/fgene.2021.723670
44. Marinelli D, Mazzotta M, Scalera S, Terrenato I, Sperati F, D'Ambrosio L, et al. KEAP1-Driven Co-Mutations in Lung Adenocarcinoma Unresponsive to Immunotherapy Despite High Tumor Mutational Burden. Ann Oncol (2020) 31:1746–54. doi: 10.1016/j.annonc.2020.08.2105
Keywords: immunotherapy, biomarker, TP53 mutation, source of tissue, non-small-cell lung cancer
Citation: Lu J, Zhong R, Lou Y, Hu M, Yang Z, Wang Y, Chen Y, Zou B, Zhang W, Wang H and Han B (2021) TP53 Mutation Status and Biopsy Lesion Type Determine the Immunotherapeutic Stratification in Non-Small-Cell Lung Cancer. Front. Immunol. 12:732125. doi: 10.3389/fimmu.2021.732125
Received: 28 June 2021; Accepted: 31 August 2021;
Published: 17 September 2021.
Edited by:
Tao Jiang, Shanghai Pulmonary Hospital, ChinaReviewed by:
Rongzhong Huang, Second Affiliated Hospital of Chongqing Medical University, ChinaHaipeng Liu, Tongji University, China
Copyright © 2021 Lu, Zhong, Lou, Hu, Yang, Wang, Chen, Zou, Zhang, Wang and Han. This is an open-access article distributed under the terms of the Creative Commons Attribution License (CC BY). The use, distribution or reproduction in other forums is permitted, provided the original author(s) and the copyright owner(s) are credited and that the original publication in this journal is cited, in accordance with accepted academic practice. No use, distribution or reproduction is permitted which does not comply with these terms.
*Correspondence: Baohui Han, 18930858216@163.com; Wei Zhang, zhwei2002@hotmail.com; Huimin Wang, chestwhm@126.com
†These authors have contributed equally to this work