- 1Biosciences and Biotechnology Division, Lawrence Livermore National Laboratory, Livermore, CA, United States
- 2Molecular and Microbiology Department, Sandia National Laboratories, Livermore, CA, United States
Peptide-based subunit vaccines are coming to the forefront of current vaccine approaches, with safety and cost-effective production among their top advantages. Peptide vaccine formulations consist of multiple synthetic linear epitopes that together trigger desired immune responses that can result in robust immune memory. The advantages of linear compared to conformational epitopes are their simple structure, ease of synthesis, and ability to stimulate immune responses by means that do not require complex 3D conformation. Prediction of linear epitopes through use of computational tools is fast and cost-effective, but typically of low accuracy, necessitating extensive experimentation to verify results. On the other hand, identification of linear epitopes through experimental screening has been an inefficient process that requires thorough characterization of previously identified full-length protein antigens, or laborious techniques involving genetic manipulation of organisms. In this study, we apply a newly developed generalizable screening method that enables efficient identification of B-cell epitopes in the proteomes of pathogenic bacteria. As a test case, we used this method to identify epitopes in the proteome of Francisella tularensis (Ft), a Select Agent with a well-characterized immunoproteome. Our screen identified many peptides that map to known antigens, including verified and predicted outer membrane proteins and extracellular proteins, validating the utility of this approach. We then used the method to identify seroreactive peptides in the less characterized immunoproteome of Select Agent Burkholderia pseudomallei (Bp). This screen revealed known Bp antigens as well as proteins that have not been previously identified as antigens. Although B-cell epitope prediction tools Bepipred 2.0 and iBCE-EL classified many of our seroreactive peptides as epitopes, they did not score them significantly higher than the non-reactive tryptic peptides in our study, nor did they assign higher scores to seroreactive peptides from known Ft or Bp antigens, highlighting the need for experimental data instead of relying on computational epitope predictions alone. The present workflow is easily adaptable to detecting peptide targets relevant to the immune systems of other mammalian species, including humans (depending upon the availability of convalescent sera from patients), and could aid in accelerating the discovery of B-cell epitopes and development of vaccines to counter emerging biological threats.
Introduction
Development of an effective vaccine against a biothreat agent or emerging pathogen is a costly and cumbersome process that can take years to decades to complete. The identification of antigens that stimulate protective immunity against a pathogen can represent a significant bottleneck in the vaccine development process, especially for bacterial or fungal pathogens, eukaryotic parasites, or even large DNA viruses, which can contain hundreds to thousands of potential antigens. Our study addressed the need to accelerate this process by testing the feasibility of a screening platform for efficient identification of immunoreactive peptides that could be utilized as candidates for development of peptide-based vaccines.
Peptide-based vaccines represent a potential solution to provide protection against biothreat and emerging pathogens to which current vaccine development strategies have failed. Peptide vaccine formulations consist of multiple synthetic linear epitopes that together trigger immune responses resulting in robust immune memory. This multi-epitope, multi-target approach has the potential to be broadly protective across divergent strains (e.g., the first universal influenza vaccine to enter phase III clinical trials was a peptide vaccine), and could be effective for pathogens with complex life cycles (e.g., several malaria peptide vaccines are currently in clinical trials) (1–3). Although it has been reported that conformational (discontinuous) epitopes make up the majority of B-cell epitopes (4), linear epitopes possess several advantages for vaccine design over conformational epitopes. Due to their short sequence and lack of complex secondary and tertiary structure, short antigenic peptides can be easily synthesized, and multiplexed into vaccine formulations, for high-throughput assessment of efficacy. Consequently, peptide-based vaccines are potentially powerful medical countermeasures that would seem amenable to rapid development in responding to infectious disease outbreaks.
Current strategies for epitope identification depend upon detection of epitopes within an individual full-length protein, a low-throughput approach that requires prior knowledge of the antigenic protein, its sequence, and its conformational structure. Technologies to screen for epitopes at the whole proteome level have been developed (e.g., proteomic microarrays, phage and yeast display); however, these technologies require extensive use of synthetic biology and other time-consuming methodologies (e.g., library construction, peptide/protein array preparation, heterologous protein expression) (3, 5–11). Another major disadvantage of display technologies and use of non-native expression systems is that these methods do not reliably replicate the native properties of the antigenic proteins, including their post-translational modifications, which can lead to inaccurate identification of epitopes.
In this study, proteome-wide screening for linear B-cell epitopes was achieved using total protein extracts isolated from the pathogen of interest, affinity purified using antibodies from convalescent sera from infected animals. This strategy holds several advantages over the currently available methods for epitope discovery: It does not require prior knowledge of antigenicity or antigen structure, and obviates need for complex and laborious experimental techniques such as preparation of display libraries and heterologous protein expression. As with other methods for epitope discovery from serum, it may be less well suited for pathogens for which natural infection does not confer immunity, such as HIV, malaria and TB, although even in those cases protective antibodies may be found in some subsets of patients or animal models (12–14).
Our approach was designed to enable identification of the protein antigen and, importantly, the antigenic regions within the identified antigen, such that these short linear peptides can be immediately synthesized and tested for efficacy in vaccine formulations. Note that several strategies have been previously developed for the identification of T-cell peptide epitopes (15, 16), including techniques similar to that presented here involving purification of MHC-bound peptides and their subsequent identification via LC/MS/MS (17).
In this study, we focused on two intracellular bacterial pathogens, Francisella tularensis (Ft) and Burkholderia pseudomallei (Bp), organisms which pose a high risk for misuse as bioweapons and therefore are considered Tier 1 Select Agents by the US Centers for Disease Control and Prevention. The mortality rates of both pathogens are high, and there is currently no licensed vaccine available for either agent (18–20). Humoral immunity plays an important role in developing immune protection to both of these intracellular pathogens, making them good model organisms for the purposes of this study (21–26). In addition, the immunoproteome of Ft has been thoroughly characterized (19, 27, 28), such that the previously published data could be compared to the datasets generated in our study. We leveraged a merged dataset of 164 previously identified antigens, corresponding to ~10% of Ft proteome. The Bp immunoproteome is not as well characterized compared to that of Ft: our reference dataset contained only 61 previously identified seroreactive proteins, corresponding to ~1% of the Bp proteome (29, 30). Consequently, analysis of the dataset resulting from the Bp screen has revealed many proteins that have not been previously categorized as antigens.
Materials and Methods
Bacterial Strains and Culture Conditions
Francisella tularensis SCHU S4ΔclpB (“Ft-ΔclpB”) was a generous gift from Dr. Wayne Conlan (National Research Council Canada). Stock cultures were prepared by growing Ft-ΔclpB on Chocolate II Agar plates supplemented with hemoglobin and isovitalex (BD 221169) for 48 hours at 37°C. Bacteria were harvested by scraping confluent lawns into Mueller Hinton (MH) broth containing 20% (w/v) sucrose, and stored at -80°C at a concentration 108 - 109 CFU/mL. Burkholderia pseudomallei mutant ΔpurM (“Bp82”) was obtained from BEI resources (NR-51280). Frozen stocks were prepared by growing the bacteria to log phase in Luria-Bertani (LB) broth, adding glycerol to achieve 20% (w/v) with the bacteria at a final concentration of 108 - 109 CFU/mL, and storing aliquots at -80°C. For immunizations, the Ft-ΔclpB and Bp82 bacterial stocks were thawed and diluted in sterile phosphate-buffered saline (PBS) to the specified concentrations used for dosing. For protein extraction purposes, Ft-ΔclpB and Bp82 were propagated to log phase in MH and LB broth, respectively. Both bacterial strains used in this study are classified as Risk Group 2 organisms. All biological materials were handled under standard institutional biosafety and biosecurity procedures, as outlined in an approved Institutional Biosafety Committee (IBC) protocol.
Protein Extraction and Peptide Preparation
Ft-ΔclpB and Bp82 were grown to log phase in 300 mL of MH broth or LB broth, respectively, at 37°C with shaking (250 rpm). The bacteria were harvested by centrifugation at 3200 x g for 10 min at 4°C, washed once with 10 mL of PBS, and the pellet flash frozen using dry ice. The bacteria in the pellet were lysed by subjecting them to two freeze-thaw cycles (alternating between room temperature and dry ice). For protein extraction, the lysate was mixed with Bper Complete Bacterial Protein Extraction Reagent (Thermo Fisher Scientific cat# 89822), and the mixture incubated at room temperature for 15 min with rotational shaking. The mixture was then subjected to two rounds of sonication (1 sec pulses, timed output 10 sec, at 50% power) using a Heat Systems Ultrasonics sonicator (model W-385), and centrifuged at 16,000 x g for 10 min. Proteins were precipitated with acetone and washed twice with ethanol. Air-dried protein pellets were solubilized using 8M urea and Protease Max surfactant (Promega cat# V2071), then digested with trypsin (Promega cat# V5111) using the in-solution digestion protocol provided by the manufacturer (Promega cat# TB373). Completion of the trypsinization reaction was confirmed by gel electrophoresis. The trypsin-digested proteins were filtered using 10K MWCO concentrators (Pierce) at 10,000 x g for 20 min at 20°C, and the filtrates (purified peptides) stored at -20°C. These purified peptides preparations were used as inputs in subsequent experiments.
Mice and Immunizations
Mouse immunization studies were carried out in strict accordance with the recommendations in the Guide for the Care and Use of Laboratory Animals and the National Institutes of Health. Standard institutional safety and biosecurity procedures were followed for in vivo experiments. Appropriate efforts were made to minimize suffering of animals. All animals were housed in ABSL2 conditions in an AAALAC-accredited facility, and the protocol (Protocol 270, renumbered 284) was approved by the LLNL Institutional Animal Care and Use Committee (IACUC). For immunization, 6 week-old female specific-pathogen-free BALB/c-Elite and C57BL/6J-Elite mice (Charles River) were injected subcutaneously with 10^6 CFU Ft-ΔclpB (BALB/c and C57BL/6J), or intradermally with 10^7 CFU Bp82 (BALB/c), and boosted at 2 weeks. No adjuvants were used. Matched PBS-dosed controls were included for each injection route. Course of infection was monitored by performing daily health scoring and weight measurements. Mice that developed infection wounds (Ft only) were topically treated with Dakin’s solution to encourage wound healing, and allowed to remain on test so long as they did not meet humane endpoint criteria (any mice with ~20% body weight loss or overt signs of morbidity were humanely euthanized). Sera from euthanized mice were excluded from analysis due to lack of immunity to the pathogen. Convalescent sera were harvested from resilient mice at 4 weeks post-infection, via cardiac puncture terminal bleeding under inhaled isoflurane anesthesia followed by blood fractionation [centrifugation at 3800 x g for 15 min in microtainer serum separator tubes (BD)]. Sera were stored at -80°C.
SDS-PAGE and Western Analysis
Western analysis was performed to confirm seropositivity of infected mice. Bacterial lysates were prepared using Bper Complete Bacterial Protein Extraction Reagent (Thermo Fisher Scientific cat# 89822), combined with Laemmli loading buffer (BioRad), and boiled at 95°C for 5 min. Samples were loaded onto 4-15% acrylamide gels (Mini-Protean TGX, BioRad) and separated by electrophoresis at 120 V for 1 hr. The proteins were transferred from the gels to nitrocellulose membranes (BioRad). Membranes were blocked with Tris-buffered saline plus 0.05% Tween 20 (TBS-T) plus 5% nonfat dry milk, at room temperature for 1 hr or at 4°C for 16 hrs. The membranes were hybridized with mouse sera at 1:500 dilution in TBS-T plus 5% milk, at room temperature for 2 hrs; washed three times with TBS-T; and then incubated with goat anti-mouse antibodies conjugated to HRP (Pierce cat# 1858413), at 1:5000 dilution in TBS-T plus 5% milk, at room temperature for 1 hr. After three TBS-T washes, the membranes were developed using SuperSignal™ West Pico PLUS Chemiluminescent Substrate (Thermo Fisher Scientific).
Enzyme-Linked Immunosorbent Assay
ELISA was performed to assess the level of seropositivity of infected mice. Wells were coated with bacterial lysates and incubated at 4°C for 16 hrs. After three washes with PBS plus 0.1% Tween-20 (PBS-T), sera from infected mice diluted to 1:100 with PBS were added to the wells and incubated at room temperature for 1 hr. Following four PBS-T washes, the wells were incubated for 1 hr with Recombinant Protein A/G peroxidase (Pierce cat# 32490) diluted at 1:5000 with PBS. After four PBS-T washes, 1-Step ABTS Substrate Solution (Pierce cat# 37615) was added, and after 15 min incubation any colorimetric changes in the wells were detected using a microplate reader (Tecan M200 Pro).
Affinity Purification of Immunoreactive Peptides
Magnetic beads coated with protein G (Invitrogen cat# 10007D) were used to capture antibodies from pools of sera obtained from either infected (experiment) mice or mock-infected (control) mice, following the manufacturer’s protocol (MAN0017348). Each pool was comprised of sera recovered from 3-5 mice, with equal volumes used for each experiment-control pair. The antibody-coated beads were then incubated with peptide preparations (inputs) at room temperature for 45 min. Antibody-coated beads from each experiment-control pair were incubated with the same input peptides; in total, 6 input peptide preparations were used with the 8 Ft experiment-control pairs, and 5 with the 9 Bp experiment-control pairs. Following three PBS washes, immunoreactive peptides were eluted from the beads using citrate buffer (pH 3). Input, unbound, and eluted (output) peptides were flash frozen with dry ice and stored at -20°C.
Mass Spectrometry
The input, unbound, and eluted (output) peptides recovered from the antibody-coated beads (see preceding section) were desalted using an Empore SD solid phase extraction plate; lyophilized; reconstituted in 0.1% TFA; and analyzed via LC-MS/MS by MS Bioworks (Ann Arbor, Michigan), using a Waters M-Class UPLC system interfaced to a ThermoFisher Fusion Lumos mass spectrometer. Peptides were loaded on a trapping column and eluted over a 75 μm analytical column at 350 nL/min. Both columns were packed with Luna C18 resin (Phenomenex). A 2 hr gradient was employed. The mass spectrometer was operated in a data dependent HCD mode, with MS and MS/MS performed in the Orbitrap at 60,000 FWHM resolution and 15,000 FWHM resolution, respectively. The instrument was run with a 3 sec cycle for MS and MS/MS.
MS Data Processing
Data were analyzed using Mascot (Matrix Science) with the following parameters: Enzyme: Trypsin/P; Database: UniProt F. tularensis SCHU S4 or UniProt B. pseudomallei strain 1026b (forward and reverse appended with common contaminants and mouse IgG sequences); Fixed modification: Carbamidomethyl (C); Variable modifications: Oxidation (M), Acetyl (N-term), Pyro-Glu (N-term Q), Deamidation (N/Q); Mass values: Monoisotopic; Peptide Mass Tolerance: 10 ppm; Fragment Mass Tolerance: 0.02 Da; Max Missed Cleavages: 2; Mascot DAT files were parsed into Scaffold Proteome Software for validation, filtering and to create a non-redundant list per sample. Data were filtered using 1% protein and peptide FDR and requiring at least one unique peptide per protein.
Bioinformatic Analysis
Each experiment typically consisted of three sets of data: “Input” (total bacterial peptides without affinity purification), “Control” (peptides purified from beads coated with antibodies from uninfected mice), and “Experiment” (peptides purified from beads coated with antibodies from infected mice).
LC-MS/MS data were analyzed at the peptide level, rather than rolling up peptide scores into a protein abundance metric as would be done in standard proteomics. We used the Total Ion Current (TIC, total area under the MS2 curve) as a metric for the abundance of the peptide in each sample. Input datasets were first normalized against each other based on median ratios for the peptides occurring in every Input dataset. The sparser Control and Experiment datasets were then normalized against their respective Input dataset based on median ratios as well. Since each animal can be expected to raise a different set of antibodies, we counted how often specific output peptides occurred more abundantly in the Experiment vs Control, rather than focusing on the average log fold change in abundance. For each peptide and each Experiment sample, we assigned an enrichment score of +1, 0, or -1 depending on whether the normalized peptide abundance was greater than, equal to, or lower in the Experiment than in the corresponding Control sample, creating a score matrix of peptides × Experiments. The total enrichment score for each peptide is then the sum of its enrichment scores across each Experiment. Statistical significance was evaluated by generating a number of randomized score matrices, where each peptide was randomly assigned a +1, 0, or -1 score for each Experiment, with the same probabilities as in the real matrix, and calculating how frequently peptides reach an specific total enrichment score. This gives us a background level of how many high-scoring peptides we would expect to see even if there was no correlation in peptide abundance across the different experiments, which can then be used to calculate the significance level of observing a given number of high scoring peptides in the real data, using a simple binomial test comparing expected vs observed number of peptides exceeding a given score.
Amino Acid Conservation Scores were calculated using the ConSurf web server (31) with default parameter values, using near full-length protein structure homology models from SWISS-MODEL or crystal structures from PDB where available. These scores are normalized position-specific evolutionary rates, with negative scores indicating the most conserved amino acids. The Average Amino Acid Conservation Score (AAACS), proposed by Ren et al. as a useful tool to identify conserved epitopes that may be targeted by broadly neutralizing antibodies, is the average of the conservation score for the residues in an epitope, with negative scores indicating more highly conserved regions (32).
In addition to AAACS, we also scored peptides based on how many complete sequenced genomes of pathogenic B. pseudomallei and F. tularensis they occurred in, similar to the conservation analysis in EpitoCore (33). We downloaded proteomes for all 110 B. pseudomallei strains with complete genome sequences available through NCBI. For F. tularensis, 36 strains with complete genomes were available through NCBI, but several of these corresponded to the less-pathogenic novicida, holartica and mediasiatica subspecies, so we decided to focus exclusively on the 17 available F. tularensis subsp. tularensis complete genomes. We identified homologs with ≥90% sequence identity to the proteins containing our top scoring peptides in Tables 1, 2, and then scored each peptide based on how often they had a 100% identical hit in each homolog.
We used two state-of-the-art computational B-cell epitope prediction tools to evaluate all of the peptides in our proteomic data that match the proteins in Tables 1, 2. Peptides were submitted to the iBCE-EL web server for scoring (34). iBCE-EL is an ensemble-based method based on extremely randomized tree and gradient boosting classifiers, trained on 5,550 experimentally validated B-cell epitopes and 6,893 non-epitopes from the Immune Epitope Database, to identify linear B-cell epitopes. In addition, proteins were submitted to the Bepipred Linear Epitope Prediction 2.0 tool on the IEDB website (35), and peptides were then scored based on their average predicted residue score. Bepipred 2.0 is a random forest classifier trained on 160 non-redundant antigen-antibody crystal structures, to predict the probability that a given antigen residue is part of an epitope.
Results
Overview of Immunoproteome Screen
In this study, we tested the feasibility of proteome-wide screening for linear B-cell epitopes using peptide extracts from target bacteria and sera from infected animals. The method requires: (1) isolation of peptides from lysates generated from the target bacteria; (2) challenge of the host (in this case, mouse) with the target bacteria, followed by collection of convalescent serum; (3) mixing of the bacterial peptides and convalescent serum, to allow peptide antigens to bind to their cognate antibodies in the serum; and (4) recovery of bound peptides for identification through mass spectrometry (Figure 1). We applied this method to two bacterial Select Agent pathogens: Francisella tularensis and Burkholderia pseudomallei. Infection with attenuated strains of these pathogens [F. tularensis SCHU S4ΔclpB and B. pseudomallei ΔpurM (strain Bp82)] has been shown to stimulate development of protective immunity against their corresponding fully-virulent parental strains (F. tularensis SCHU S4 and B. pseudomallei K96245, respectively) (36, 37), suggesting that convalescent sera recovered from hosts infected with these attenuated pathogens must contain protective antibodies.
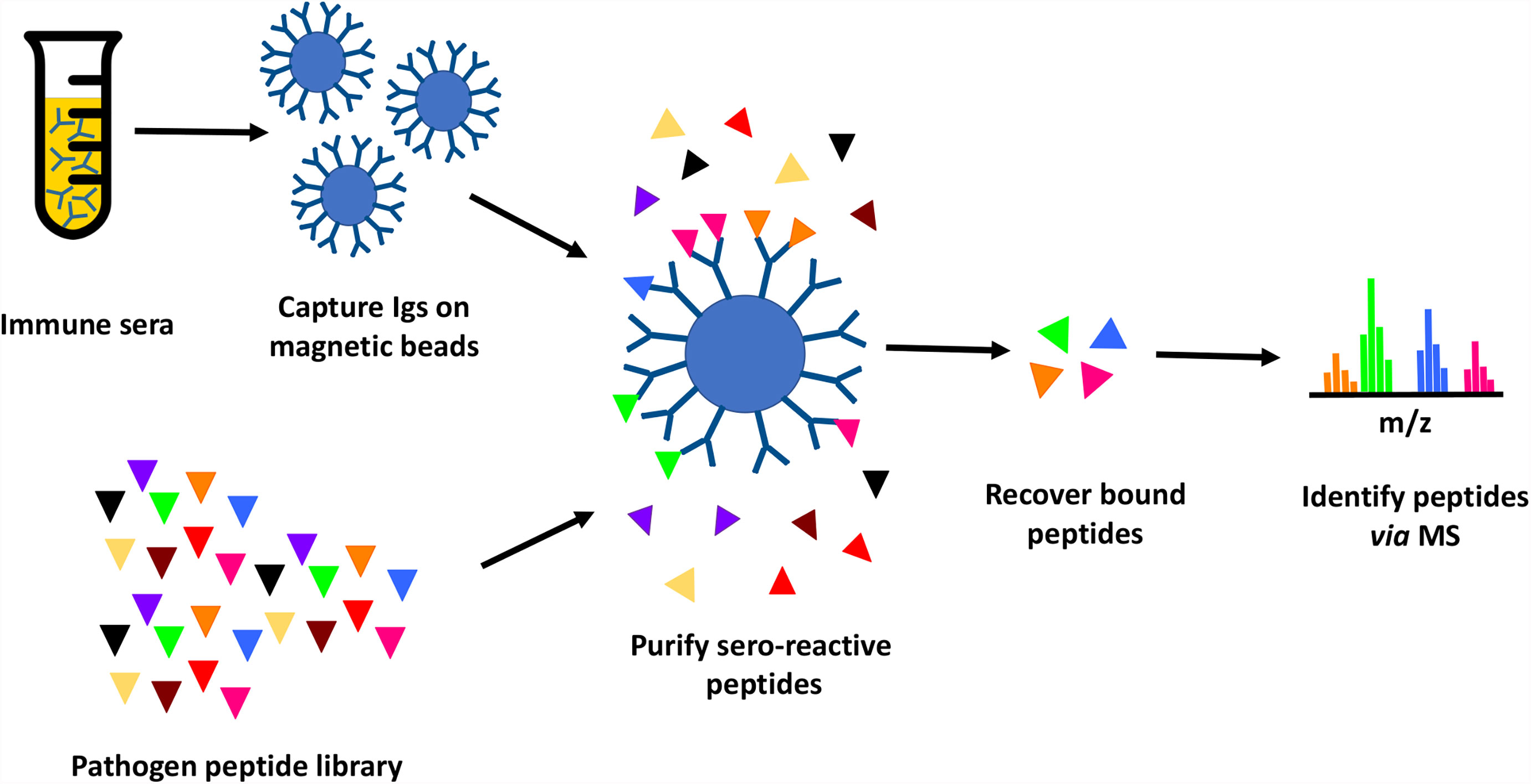
Figure 1 Immunoproteome screening workflow. Schematic overview of high throughput approach for identification of seroreactive peptides in the proteomes of pathogens.
Briefly, proteins purified from pathogen lysates were digested with trypsin to generate a peptide library. Mice were infected with a sublethal dose of Ft-ΔclpB or Bp82, and immune status assessed through observed weight loss and measurement of seroreactivity of mouse sera to pathogen lysates via enzyme-linked immunosorbent assay (ELISA) or Western blot analysis (Figure 2). Antibodies purified from the convalescent sera of infected mice were immobilized on magnetic beads and then incubated with pathogen-derived peptides to allow formation of antigen-antibody complexes. Peptides recovered from the immobilized antibodies were identified via liquid chromatography coupled with tandem mass spectrometry.
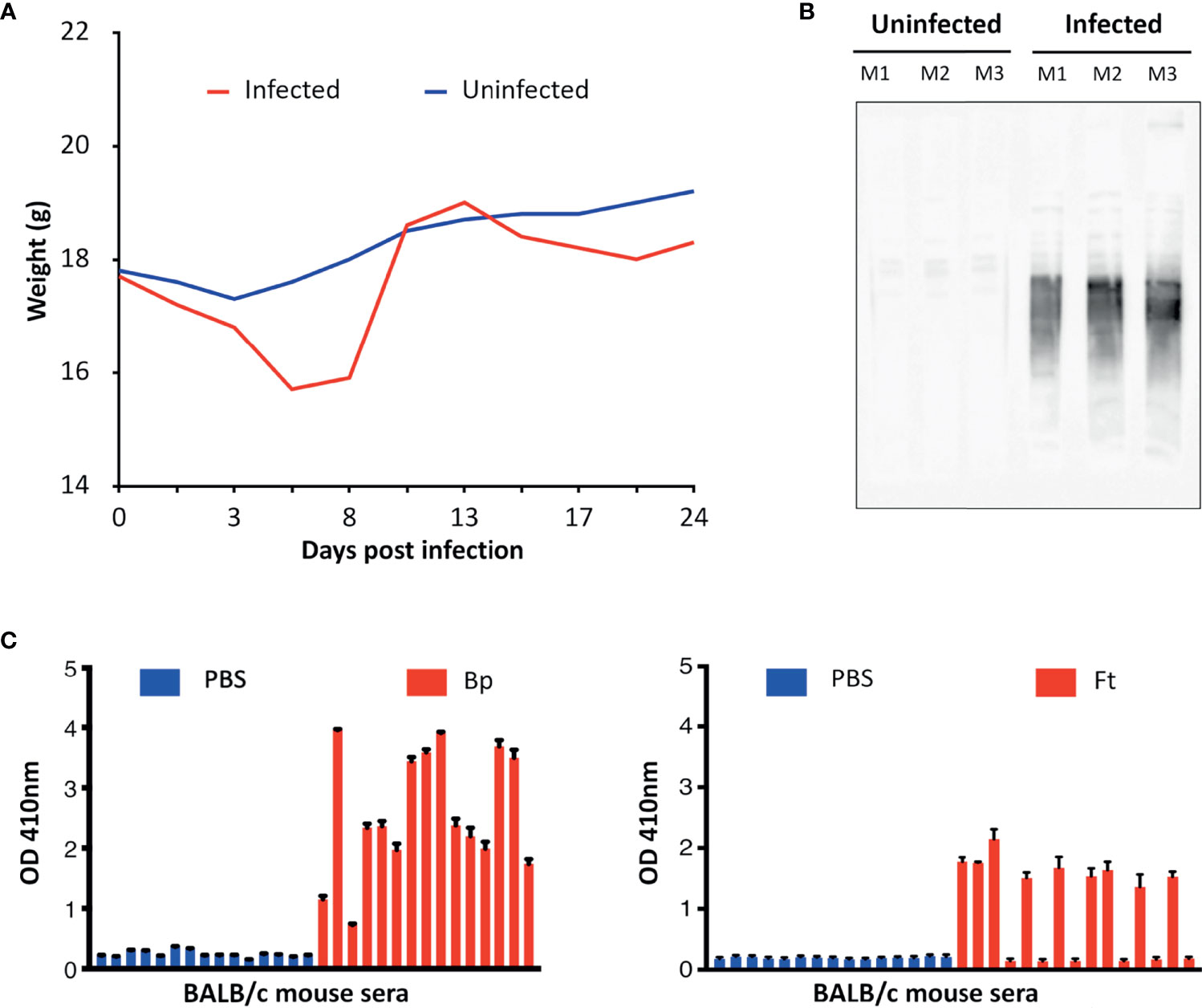
Figure 2 (A) Representative course of mouse infection to obtain immune sera. Mice were infected with a sublethal dose of Bp and their weight monitored. Weight was monitored throughout the course of infection. (B) Representative Western blot of sera from infected vs uninfected mice. Bp protein lysates were analyzed by Western blotting using sera from infected and uninfected mice (Mouse 1–3) and bound antibodies detected using anti-mouse HRP. (C) Representative ELISA results obtained from mice infected with Bp and Ft (red) in comparison with uninfected mice (PBS-treated mice, blue). Seroreactivity of mice sera to microwells coated with corresponding pathogen lysate was assessed using protein-A/G-HRP and measuring sample absorbance (optical density). Sera of some mice infected with Ft did not yield positive results because Ft infection led to lethal outcome and mice had to be euthanized during the course of immunization. Graphs represent two technical replicates for sera collected from each mouse. Antibodies from sera with the strongest Western blot and ELISA signals were purified in this study and used to screen for immunogenic peptides.
Bioinformatic Identification of Enriched Antigenic Peptides
The peptides recovered using pooled sera from infected mice (Experiment peptidome) were compared to those recovered from mock-infected mice (Control peptidome); a total of 8 pairs of Experiment-Control peptidomes were collected for Ft, and 9 pairs for Bp. For Ft, we found that out of the 1923 peptides that were recovered in at least two Experiment peptidomes, 44 had an enrichment score of 6 or greater (Table 1), whereas only 20.1 +/- 6.1 peptides would be expected at random (p=1x10-6). For Bp, out of 2902 peptides that were recovered in at least two Experiment peptidomes, 46 peptides had an enrichment score of 6 or greater (Table 2), whereas only 17.8 +/- 4.3 peptides would be expected at random (p=1.9x10-9). If a more stringent enrichment cutoff is desired, we found 16 Ft peptides with an enrichment score of 7 or greater, versus 3.5+/-1.6 expected at random (p=1.8x10-7), and 20 Bp peptides with an enrichment score of 7 or greater, versus 4.1+/-2.1 expected at random (p=3.9x10-9). The enriched peptides included some that were derived from protective antigens identified in previous studies, as well as predicted outer membrane and extracellular proteins (Tables 1, 2). There were many examples of multiple enriched peptides originating from the same protein (highlighted in bold in the tables), a further indication that enrichment was not random but rather due to immune response to a discrete set of bacterial proteins.
Note that we used C57BL/6J mice for two of the eight Ft experimental samples, because of previously reported differences in protection and antibody response after immunization of C57BL/6J and BALB/c mice with Ft-ΔclpB by Twine et al. (38). Analyzing the BALB/c Ft samples separately yielded a very similar set of results as in Table 1, but with lower p-value for the enrichment due to the smaller number of samples (data not shown). Therefore, we decided to combine the data and focus on antibody responses in common between both strains of mice. Although Twine et al. reported an antibody response against chaperonin protein GroL only in BALB/c mice, our data shows that there are several GroL epitopes that are enriched in samples from both mouse strains (see Table 1 and Figure 4).
Prior immunoproteomics analysis of the antibody response to F. tularensis using human or mouse sera has identified 164 antibody targets out of a total of 1667 proteins (~10% of the entire Ft proteome) (19, 27, 28). Out of the 1923 peptides that have hits in at least two Ft datasets, 876 peptides match known antigenic proteins. Given those numbers, we would expect only 20 such peptides to show up at random in our list of 44 in Table 1, but instead we observe that 38/44 peptides in the list correspond to known antigens - an almost two-fold enrichment (p=2.79x10-9). Note that despite the extensive literature on antigens in Ft, only five B-cell epitopes have been experimentally determined (Figure 4B), justifying the need for a simple experimental epitope screening method. The immune response to B. pseudomallei has not been studied in as much depth as for Francisella. So even though Bp with 6203 protein coding genes has a genome that is more than three times as large as that of Ft, we found only 61 known antigens identified in previous studies (29, 30) (~1% of the entire proteome). Our list of 46 top Bp peptides in Table 1 includes one known antigen, which does not qualify as a statistically significant enrichment primarily because of the much smaller total number of known antigens for Bp.
Figure 3 shows all 46 Ft DnaK peptides that were detected in at least two Experiment samples, regardless of their degree of enrichment. Eight of these DnaK peptides are in our list of 44 enriched Ft peptides (Table 1 and red line segments in Figure 3A), including two that are enriched in all 8 Experiments (red line segments in Figure 3A). Note the lack of correlation between our experimental enrichment scores and the iBCE-EL and Bepipred scores (Figures 3B, C). All but one of the 8 enriched peptides are conserved in all 17 fully sequenced Ft strains (Figure 3E), but some of the peptides towards the C-terminal show a greater evolutionary rate as measured by their Average Amino Acid conservation Score (AAACS, Figure 3D) and thus may be more prone to immune escape mutants.
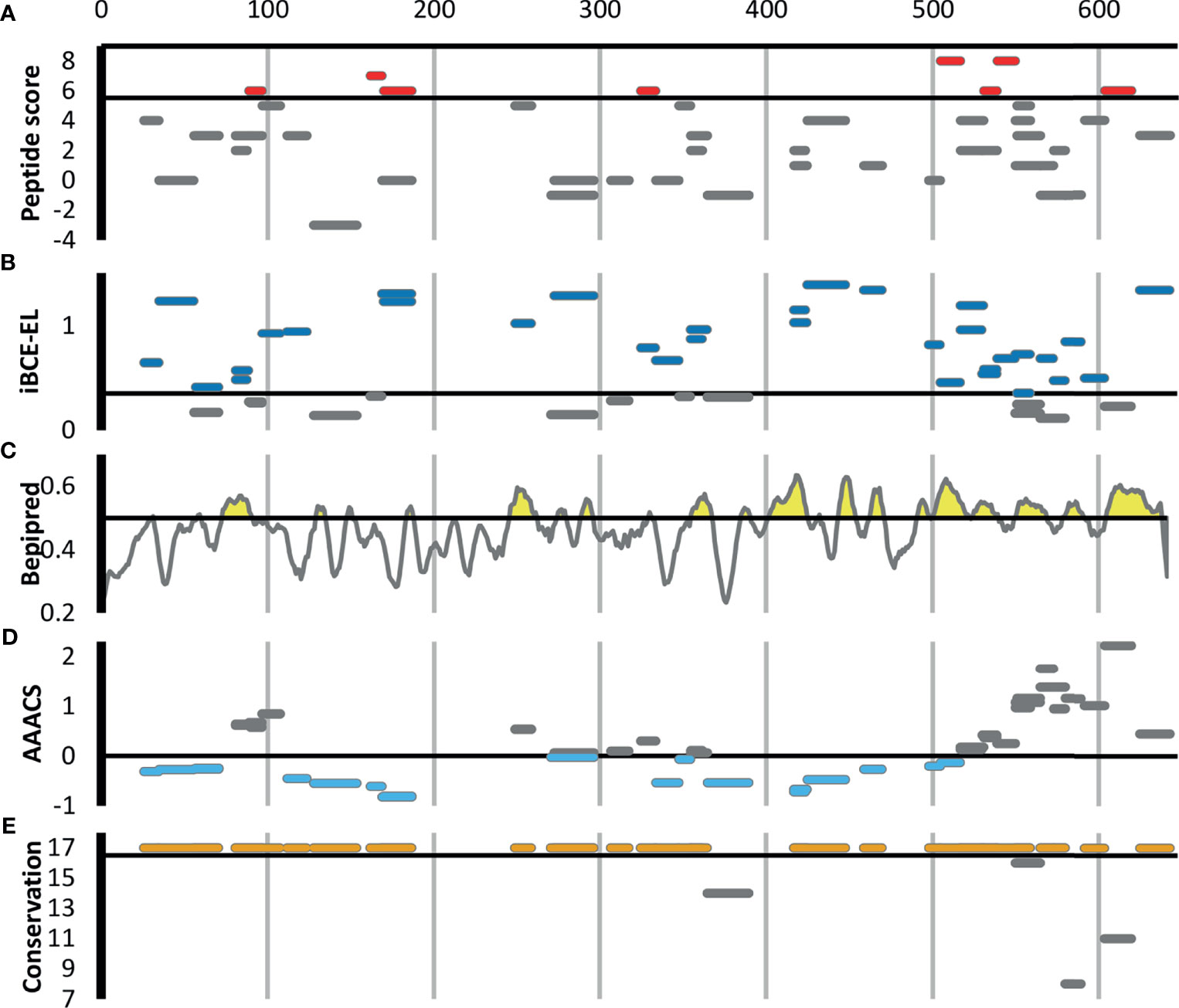
Figure 3 Scoring for the 46 F. tularensis DnaK peptides detected in at least two Experiment samples. The short horizontal line segments in A, B, E and F indicate the position of a peptide along the length of the 642aa DnaK protein, and its vertical position within each figure panel indicates its score for the metric indicated. The default score threshold for each tool is shown with a horizontal line, and the peptides or per-amino acid scores exceeding that threshold are shown in color. (A) Peptide enrichment score based on our proteomics results. An enrichment score of 8 indicates that the peptide was detected in greater abundance in all 8 Experiment samples relative to their respective Control samples. The threshold for inclusion in Table 1 was an enrichment score of ≥6 (shown in red). (B) B-cell epitope prediction score generated using iBCE-EL. At the default iBCE-EL score threshold of 0.35, nearly three quarter of all peptides were predicted to be likely B-cell epitopes (shown in dark blue). (C) B-cell epitope prediction score generated using Bepipred 2.0. The per-amino acid scores are indicated by the line graph. At the default iBCE-EL score threshold of 0.35, 37% of all amino acids were predicted to be in B-cell epitopes (regions of the graph shown in yellow). (D) Average Amino Acid Conservation Score (AAACS) based on Consurf analysis. Negative scores indicate greater degrees of evolutionary conservation (shown in light blue). (E) Number of fully sequenced F. tularensis subsp. tularensis genomes (17 analyzed) in which each peptide occurs. Almost all of the DnaK peptides considered were conserved in all 17 Ft genomes (shown in orange).
Figure 4 shows all 32 Ft GroL peptides that were detected in at least two Experiment samples in our study, regardless of the degree of their enrichment. Four of these GroL peptides are in our list of 44 enriched Ft peptides (Table 1 and red line segments in Figure 4A), including three that are enriched in all eight Experiments. Lu et al. (39) used hydrogen/deuterium exchange–mass spectrometry (DXMS) to experimentally identify one discontinuous and four linear B-cell epitopes for a selection of mouse monoclonal antibodies against GroL (Figure 4B). Note that one of the four enriched peptides in Figure 4A (DNTTIIDGAGEK) overlaps with a linear epitope (NTTIIDGAGEKEAIAKRINVIK) and a discontinuous epitope (SEDLSMKLEETNM—NTTIIDGAGEKEAIA) identified by DXMS in Figure 4B, while a second enriched peptide (EGVITVEEGK) is directly adjacent to another of the linear DXMS epitopes (FEDEL). According to the Immune Epitope Database (IEDB) (40), these are the only experimentally validated B-cell epitopes for Ft (IEDB also lists four B. pseudomallei antigens that have been assayed for B-cell epitopes, none of which overlap with the proteins in Table 1).
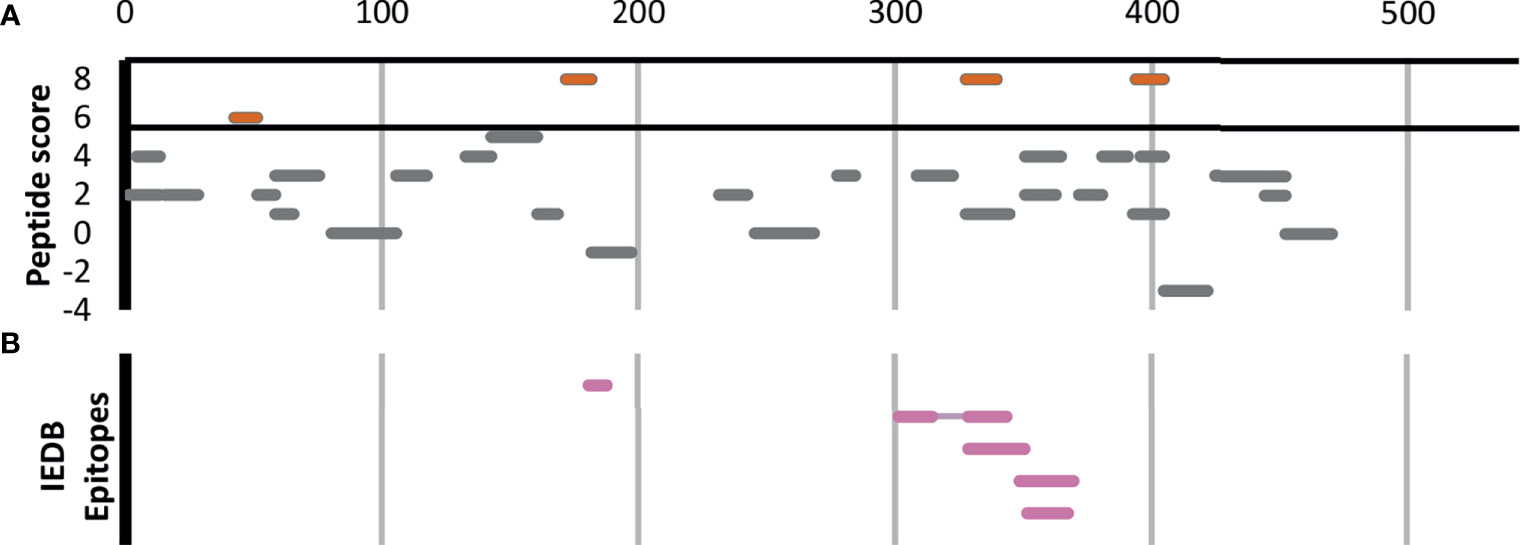
Figure 4 The 32 F. tularensis GroL peptides detected in at least two Experiment samples. Horizontal line segments indicate the position of each peptide along the length of the 544aa GroL protein sequence. (A) Peptide enrichment score based on our proteomics results, with a score of 8 indicating that the peptide was found in greater abundance in all 8 Experiment samples relative to their respective Control samples. The threshold for inclusion in Table 1 was a score of ≥6 or better (shown in red). (B) Five B-cell epitopes identified by DXMS by Lu et al. (39), including one discontinuous epitope.
Discussion
We have developed a widely applicable shotgun immunoproteomic method that enables efficient identification of B-cell epitopes in the proteomes of pathogens. The results of this study have revealed a significant enrichment of peptides derived from previously identified antigens and vaccine candidates, validating the method’s efficacy. This method was designed to identify linear epitopes efficiently without the need of genetic manipulation or other experimental techniques that can be costly and labor intensive. Attenuated strains made the optimization of this proof-of-concept study more efficient; however, the availability of an attenuated strain for the target organism does not represent a limitation, as our strategy could be applied to fully virulent strains of pathogens as well. Although the present study was performed using a mouse model, the workflow could be easily adapted to detecting targets relevant to the human immune system, using convalescent sera from patients.
Utilizing peptide antigens for vaccine development has several advantages over typical vaccine development efforts. Similar to other types of subunit vaccines, peptide vaccines represent a safer alternative to attenuated vaccines due to lack of any potentially infectious materials in the vaccine formulation. Use of short peptides sufficient for stimulation of immune response favors exclusion of deleterious sequences that may be present in full length antigenic proteins. Peptide vaccine formulations are defined and their contents fully synthetic, which simplifies quality control procedures and thereby streamlines the regulatory approval process. Production of peptide vaccines is expected to be relatively fast and inexpensive, due to ease of synthesis and recent advances in improved peptide stability (3, 41, 42). Moreover, once antigenic peptides are identified, evaluation of their efficacy could represent a lesser challenge due to the possibility of multiplexing peptides during in vivo trials, rather than use of one-at-a-time testing
Among Ft proteins, the present screen identified multiple peptides for two well-characterized antigens, 60kDa chaperonin GroL (Q5NEE1) and chaperone protein DnaK (Q5NFG7). Both chaperonins have been previously implicated in virulence of Francisella (43–45), and are known to induce antibody production in mice and humans (27, 46, 47). These chaperonin proteins are important for facilitating folding of nascent proteins as well as post-translational modifications. They are also known as heat-shock proteins, as they protect cellular proteins from environmental stresses such as high temperature and low pH (47, 48). Although their cellular localization is predicted to be cytoplasmic, they reportedly also associate with membrane proteins and are released into host cells during infection (47, 49–51) perhaps contributing to their ability to stimulate various immune functions, including innate immunity, humoral immunity and cell-mediated immunity (43, 47, 52–55). Heat-shock proteins are good candidates for subunit vaccine design due to their ability to stimulate various immune responses without the need of adjuvant; in fact, both GroL and DnaK have been exploited for vaccine development efforts targeting Francisella and other pathogens (39, 47, 56, 57).
Highly virulent Type A Francisella strains such as SCHU S4 can bind host plasminogen to the bacterial cell surface where it can be converted to plasmin, a serine protease that degrades opsonizing antibodies, inhibiting antibody-mediated uptake by macrophages (58, 59). Among the 25 Ft proteins listed in Table 1, we find at least 3 that are known to be involved in plasminogen binding in Francisella or other pathogens, including conserved hypothetical lipoprotein LpnA (Q5NGE4) (59), fructose-1,6-bisphosphate aldolase (Q5NF78) (60), and elongation factor Tu (Q5NID9) (61). These proteins could make for particularly attractive vaccine targets, because if we can interfere with their function before the pathogen has activated its plasmin-mediated antibody evasion, that would make it more susceptible to other antibodies as well.
Among the antigenic peptides identified in the Bp proteome are those belonging to Type VI secretion system component Hcp-,1 and previously identified antigen 10kDa chaperonin GroES (62). Hcp-1 was previously found to be a major virulence determinant in Burkholderia and recognized by sera from infected human patients and animals (63–65). Due to this, Hcp-1 has been interrogated as a potential candidate for Burkholderia vaccine development (63–65). Additionally, a peptide from an ankyrin repeat-containing protein (A0A0H3HJC) came up as one of the highest scoring peptides in our study. Ankyrin repeats are typically eukaryotic protein domains involved in protein-protein interactions (66), but have been co-opted by many bacterial pathogens as type IV secreted effector proteins to mimic or manipulate various host functions (67).
Recovery of peptides derived from several supposedly cytosolic enzymes may seem puzzling. However several “housekeeping” enzymes are known to be displayed on the surface of pathogens where they play a role in virulence (68). For example, our top scoring peptides from B. pseudomallei include two derived from enolase (A0A0H3HLA6). While enolase is primarily thought of as a key glycolytic enzyme, it is also expressed on the surface of a wide variety of bacterial and fungal pathogens, where it interacts with host plasminogen and is associated with invasion and virulence (69). Antibodies against enolase have been detected in a large variety of infectious and autoimmune diseases (70). It is as yet unknown whether enolase plays the same role in Burkholderia, but the protein is predicted to be present both in the cytoplasm and on the cell surface, and its production was found to be upregulated upon exposure to human lung epithelial cells (71). Other housekeeping proteins in our top scoring results whose homologs in other pathogens are known to play a role in adhesion, invasion, or virulence include elongation factor Tu (Q5NID9), malic enzyme/malate dehydrogenase (A0A0H3HP28, Q5NHC8), and fructose-1,6-bisphosphate aldolase (Q5NF78) (68).
Overall, this immunoproteomic workflow has identified numerous peptides mapping to previously identified antigens and subunit vaccine targets, predicted membrane-associated proteins, as well as uncharacterized proteins. The Ft datasets revealed a significant enrichment of peptides belonging to previously identified antigenic proteins in Experiment samples relative to their respective Control samples, providing validation to this approach. Interestingly, several of these known antigens also yielded multiple top scoring peptides in our analysis. Despite the large amount of prior immunoproteomic analysis on Ft, covering ~10% of the genome, experimentally validated B-cell epitopes are available for only a single protein, and our analysis captures two out of its five known epitopes. Due to the much smaller number of previously identified antigens for Burkholderia, we were not able to tell whether the enrichment in the Bp datasets was significant. Improved proteome coverage and more comprehensive immunogenic profiles could be achieved with the use of alternative enzymes with different specificities, since there is a risk of ablating epitopes that contain cut sites recognized by specific enzymes such as trypsin. Alternatively, performing incomplete digestion with one enzyme, or a cocktail of enzymes with different specificities, could increase the number of overlapping peptides and thereby improve the yield and diversity of identified epitopes. In addition, since the presented method is dependent upon extraction of proteins from whole cell lysates, it is conceivable that the proteome coverage could be biased toward highly abundant proteins or those proteins that are easier to extract, despite this disadvantage we have detected several membrane-bound antigens in this study.
A variety of computational B-cell epitope prediction tools have been developed to identify epitopes in antigens. However accurate computational prediction of B-cell epitopes still poses a major challenge (72), with sensitivity or specificity typically below 60% (35, 73–76), leading some recent in-silico multi-epitope vaccine design efforts to look at the consensus of up to 8 or 9 B-cell epitope prediction tools simultaneously (77, 78). The recent development of prediction tools using state-of-the-art machine learning models that claim significantly higher performance on large benchmarking datasets seems promising (34, 79). Here we compare the performance of Bepipred 2.0 (35), one of the most widely used B-cell prediction tools, and iBCE-EL (34). Interestingly, we find no significant correlation between the peptides experimentally identified using the method described here and computationally predicted linear B-cell epitope scores generated by Bepipred 2.0 and iBCE-EL, even for those antibody-binding peptides belonging to known Ft or Bp antigens, nor do we find any significant correlation between the Bepipred 2.0 and iBCE-EL scores themselves (see Supplementary Tables S1, S2, as well as Figures 3A–C for Ft DnaK), highlighting the value of an unbiased experimental method to screen for antibody targets, as presented here. At their default score thresholds, iBCE-EL correctly predicts 34/44 of the Ft peptides, and 39/46 of the Bp peptides, while Bepipred 2.0 correctly predicts 21/44 Ft peptides and 13/46 Bp peptides, but that is not actually significantly more than would be expected at random given their hit rates on other un-enriched tryptic peptides in our dataset. Part of the discrepancy between the computational Bepipred 2.0 predictions and our experimental results may be due to the fact that Bepipred 2.0 is trained on antigen-antibody 3D structures, which likely contain a mix of conformational and linear epitopes. In addition, Bepipred 2.0 has a relatively low self-reported 58.6% sensitivity and 57.2% specificity at the default score threshold of 0.5 (80), and thus is expected to exhibit a large number of false positives and false negative predictions. iBCE-EL is reported to have better sensitivity and specificity [73.2% and 72.4% (34)], but explicitly takes into account sequence features at the beginning and end of the epitope that may be missing in the tryptic peptides generated here, affecting their score. In cases where the tryptic peptide is too short to be used directly as a vaccine candidate (some are as short as 6 residues), we may in fact be able to use these computational tools to guide us in how to extend the boundaries of the peptide beyond its flanking trypsin cleavage sites.
Note that computational B-cell prediction tools such as these are trained to distinguish epitopes from non-epitopes in known antigens, but are not an effective alternative to experimentally screening for epitopes across an entire bacterial proteome. For example, on a random selection of 100 Ft and Bp proteins, Bepipred-2.0 using its default epitope threshold of 0.5 classified 40% of all amino acids as being part of an epitope, including an average of 5.5 peptides of length 9 or longer per protein (data not shown). Likewise, on a random selection of 1000 tryptic peptides from all our proteomics data, iBCE-EL classified 81% as B-cell epitopes using its default score threshold of 0.35 (data not shown). Applied across the entire proteome, the computational approach would predict tens of thousands of putative B-cell epitopes, likely with a high false-positive rate and, regardless, providing little guidance in winnowing the possibilities for experimental verification.
If so desired, peptides can be downselected for vaccine development by focusing only on those with the most stringent enrichment scores, or based on consensus with computational epitope prediction tools. Further downselection may include prioritizing highly conserved epitopes that can induce broadly protective immunity, and reduce the risk that emergence of pathogen variants will render the vaccine ineffective (81). ~90% of the top scoring peptides were found to be present in 90% or more of the fully sequenced pathogenic F. tularensis and B. pseudomallei strains (see Supplementary Tables S1, S2, and Figure 3F for the case of Ft DnaK). In addition, we can target peptides that show even deeper evolutionary conservation based on their Average Amino Acid Conservation Score (AAACS), reflecting parts of the protein that may be important for its function (31) (see Supplementary Tables S1, S2, and Figure 3E for the case of Ft DnaK). Peptides that are only one or two amino acids different from human or mouse versions are likely less suitable as vaccine candidates and are marked with a subscript 1 or 2 respectively in Tables 1, 2. Note that while some of the proteins in Tables 1, 2 have homologs in human and mouse (e.g. mitochondrial DnaK), the peptides recovered here are unique to the bacterial versions. For vaccine design, we may also want to prioritize peptides which do not tend to occur in healthy human microbiomes, by comparing them against some of the large human metaproteomics datasets recently generated (82–86).
Further confirmation that the identified sequences are B-cell epitopes could be achieved through additional in vitro and in vivo experimentation (e.g., testing the reactivity of immune sera with synthesized candidate epitopes via ELISA or immunization studies). High throughput screening of peptides for efficacy is feasible due to recent advancements in solid phase peptide synthesis (SPPS), which enables efficient and cost-effective production of peptide candidates (3). For immunization studies, pools of multiple peptides could be incorporated into vaccine delivery systems containing adjuvants and T-helper epitopes known to stimulate the induction of adaptive immune response against peptide antigens, as reviewed in Skwarczynski et al. (3).
The method presented here identifies peptides that are immunoreactive, that is, they interact with antibodies in serum from previously infected individuals. Further experimental test would be needed to confirm immunogenicity, that is, whether they can stimulate antibody production themselves, and protectivity, that is, whether they can protect against infection or disease after immunization. Our immunoproteomic method represents a new tool for precise mapping of linear B-cell epitopes. Generation of such immunogenic profiles for pathogens could provide an ample pool of candidates for further experimental validation and efficient vaccine development. Accelerating the discovery of B-cell epitopes in the proteomes of pathogens will help fuel the development of peptide-based vaccines that have the potential to provide rapid solutions to biothreat agents and emerging pathogens.
Data Availability Statement
The datasets presented in this study can be found in online repositories. The names of the repository/repositories and accession number(s) can be found below: https://www.ebi.ac.uk/pride/archive/, PXD026300 (87).
Ethics Statement
The animal study was reviewed and approved by LLNL Institutional Animal Care and Use Committee.
Author Contributions
PD’h, NC, and MF contributed to the conception and design of the study. NC performed the in vivo experiments. VL provided laboratory support. VL and MF performed in vitro experimentation. PD performed the bioinformatics analysis. BS and SB provided critical input. All authors contributed to the article and approved the submitted version.
Funding
This work was supported by Lawrence Livermore National Laboratory Directed Research and Development Program (LLNL LDRD) Labwide grant (18-LW-039) to MF, and by the LDRD program (grant 218309) at Sandia National Laboratories, a multi-mission laboratory managed and operated by National Technology and Engineering Solutions of Sandia, LLC, a wholly owned subsidiary of Honeywell International, Inc., for the U.S. Department of Energy’s National Nuclear Security Administration under contract DE-NA0003525. Work at LLNL was performed under the auspices of the U.S. Department of Energy by Lawrence Livermore National Laboratory under Contract DE-AC52-07NA27344. LLNL IM release number LLNL-JRNL-822446. The funders were not involved in the study design, collection, analysis, interpretation of data, the writing of this article or the decision to submit it for publication.
Conflict of Interest
MF, NC, and PD’h are inventors on a provisional patent application for the method for rapid detection of immunogenic epitopes, filed by Lawrence Livermore National Security, LLC.
The remaining authors declare that the research was conducted in the absence of any commercial or financial relationships that could be construed as a potential conflict of interest.
Publisher’s Note
All claims expressed in this article are solely those of the authors and do not necessarily represent those of their affiliated organizations, or those of the publisher, the editors and the reviewers. Any product that may be evaluated in this article, or claim that may be made by its manufacturer, is not guaranteed or endorsed by the publisher.
Acknowledgments
We thank Dr. Wayne Conlan (National Research Council Canada) for providing Francisella tularensis SCHU S4ΔclpB strain. Bp 82 reagent was obtained through BEI Resources, NIAID, NIH: Burkholderia pseudomallei, Strain Bp82 (ΔpurM), NR-51280. Our thanks go to Michael Ford and MS Bioworks team for help with sample preparation troubleshooting and specialized mass spectrometry analyses. We also thank past and present members of our laboratories - Drs. Sahar El-Etr, José Peña, Amy Rasley, and Emilio Garcia - for useful discussions and critical input.
Supplementary Material
The Supplementary Material for this article can be found online at: https://www.frontiersin.org/articles/10.3389/fimmu.2021.716676/full#supplementary-material
References
1. Li W, Joshi MD, Singhania S, Ramsey KH, Murthy AK. Peptide Vaccine: Progress and Challenges. Vaccines Basel (2014) 2(3):515–36. doi: 10.3390/vaccines2030515
2. Malonis RJ, Lai JR, Vergnolle O. Peptide-Based Vaccines: Current Progress and Future Challenges. Chem Rev (2020) 120(6):3210–29. doi: 10.1021/acs.chemrev.9b00472
3. Skwarczynski M, Toth I. Peptide-Based Synthetic Vaccines. Chem Sci (2016) 7(2):842–54. doi: 10.1039/C5SC03892H
4. Sun P, Ju H, Liu Z, Ning Q, Zhang J, Zhao X, et al. Bioinformatics Resources and Tools for Conformational B-Cell Epitope Prediction. Comput Math Methods Med (2013) 2013:943636. doi: 10.1155/2013/943636
5. Dudek NL, Perlmutter P, Aguilar MI, Croft NP, Purcell AW. Epitope Discovery and Their Use in Peptide Based Vaccines. Curr Pharm Des (2010) 16(28):3149–57. doi: 10.2174/138161210793292447
6. Wang AP, Li N, Zhou JM, Chen YM, Jiang M, Qi YH, et al. Mapping the B Cell Epitopes Within the Major Capsid Protein L1 of Human Papillomavirus Type 16. Int J Biol Macromol (2018) 118:1354–61. doi: 10.1016/j.ijbiomac.2018.06.094
7. Zhao DM, Han KK, Huang XM, Zhang LJ, Wang HL, Liu N, et al. Screening and Identification of B-Cell Epitopes Within Envelope Protein of Tembusu Virus. Virol J (2018) 15:142. doi: 10.1186/s12985-018-1052-1
8. Bi Y, Jin Z, Wang Y, Mou S, Wang W, Wei Q, et al. Identification of Two Distinct Linear B Cell Epitopes of the Matrix Protein of the Newcastle Disease Virus Vaccine Strain LaSota. Viral Immunol (2019) 32(5):221–9. doi: 10.1089/vim.2019.0007
9. Jaenisch T, Heiss K, Fischer N, Geiger C, Bischoff FR, Moldenhauer G, et al. High-Density Peptide Arrays Help to Identify Linear Immunogenic B-Cell Epitopes in Individuals Naturally Exposed to Malaria Infection. Mol Cell Proteomics (2019) 18(4):642–56. doi: 10.1074/mcp.RA118.000992
10. Yang W-J, Lai J-F, Peng K-C, Chiang H-J, Weng C-N, Shiuan D. Epitope Mapping of Mycoplasma Hyopneumoniae Using Phage Displayed Peptide Libraries and the Immune Responses of the Selected Phagotopes. J Immunol Methods (2005) 304(1–2):15–29. doi: 10.1016/j.jim.2005.05.009
11. Mullen LM, Nair SP, Ward JM, Rycroft AN, Henderson B. Phage Display in the Study of Infectious Diseases. Trends Microbiol (2006) 14(3):141–7. doi: 10.1016/j.tim.2006.01.006
12. Ndam NT, Denoeud-Ndam L, Doritchamou J, Viwami F, Salanti A, Nielsen MA, et al. Protective Antibodies Against Placental Malaria and Poor Outcomes During Pregnancy, Benin. Emerg Infect Dis (2015) 21(5):813–23. doi: 10.3201/eid2105.141626
13. Gonzales SJ, Reyes RA, Braddom AE, Batugedara G, Bol S, Bunnik EM. Naturally Acquired Humoral Immunity Against Plasmodium Falciparum Malaria. Front Immunol (2020) 11:594653. doi: 10.3389/fimmu.2020.594653
14. Li H, Wang X-X, Wang B, Fu L, Liu G, Lu Y, et al. Latently and Uninfected Healthcare Workers Exposed to TB Make Protective Antibodies Against Mycobacterium Tuberculosis. Proc Natl Acad Sci USA (2017) 114(19):5023–8. doi: 10.1073/pnas.1611776114
15. Sharma G, Holt RA. T-Cell Epitope Discovery Technologies. Hum Immunol (2014) 75(6):514–9. doi: 10.1016/j.humimm.2014.03.003
16. Sharma G, Rive CM, Holt RA. Rapid Selection and Identification of Functional CD8+ T Cell Epitopes From Large Peptide-Coding Libraries. Nat Commun (2019) 10:4553. doi: 10.1038/s41467-019-12444-7
17. Caron E, Kowalewski DJ, Chiek Koh C, Sturm T, Schuster H, Aebersold R. Analysis of Major Histocompatibility Complex (MHC) Immunopeptidomes Using Mass Spectrometry. Mol Cell Proteomics MCP (2015) 14(12):3105–17. doi: 10.1074/mcp.O115.052431
18. Dienst FT. Tularemia: A Perusal of Three Hundred Thirty-Nine Cases. J State Med Soc (1963) 115:114–27.
19. Fulton KM, Zhao X, Petit MD, Kilmury SLN, Wolfraim LA, House RV, et al. Immunoproteomic Analysis of the Human Antibody Response to Natural Tularemia Infection With Type A or Type B Strains or LVS Vaccination. Int J Med Microbiol IJMM (2011) 301(7):591–601. doi: 10.1016/j.ijmm.2011.07.002
20. Gibney KB, Cheng AC. Reducing the Melioidosis Burden: Public Health, Chronic Disease Prevention, or Improved Case Management? Lancet Infect Dis (2019) 19(8):800–2. doi: 10.1016/S1473-3099(19)30303-2
21. Mara-Koosham G, Hutt JA, Lyons CR, Wu TH. Antibodies Contribute to Effective Vaccination Against Respiratory Infection by Type A Francisella Tularensis Strains. Infect Immun (2011) 79(4):1770–8. doi: 10.1128/IAI.00605-10
22. Ray HJ, Cong Y, Murthy AK, Selby DM, Klose KE, Barker JR, et al. Oral Live Vaccine Strain-Induced Protective Immunity Against Pulmonary Francisella Tularensis Challenge Is Mediated by CD4+ T Cells and Antibodies, Including Immunoglobulin a. Clin Vaccine Immunol CVI (2009) 16(4):444–52. doi: 10.1128/CVI.00405-08
23. Rhinehart-Jones TR, Fortier AH, Elkins KL. Transfer of Immunity Against Lethal Murine Francisella Infection by Specific Antibody Depends on Host Gamma Interferon and T Cells. Infect Immun (1994) 62(8):3129–37. doi: 10.1128/iai.62.8.3129-3137.1994
24. Hogan RJ, Lafontaine ER. Antibodies Are Major Drivers of Protection Against Lethal Aerosol Infection With Highly Pathogenic Burkholderia spp. mSphere (2019) 4:e00674–18. doi: 10.1128/mSphere.00674-18
25. Jones SM, Ellis JF, Russell P, Griffin KF, Oyston PCF. Passive Protection Against Burkholderia Pseudomallei Infection in Mice by Monoclonal Antibodies Against Capsular Polysaccharide, Lipopolysaccharide or Proteins. J Med Microbiol (2002) 51(12):1055–62. doi: 10.1099/0022-1317-51-12-1055
26. Khakhum N, Bharaj P, Myers JN, Tapia D, Kilgore PB, Ross BN, et al. Burkholderia Pseudomallei ΔtonB Δhcp1 Live Attenuated Vaccine Strain Elicits Full Protective Immunity Against Aerosolized Melioidosis Infection. mSphere (2019) 4:e00670–18. doi: 10.1128/mSphere.00570-18
27. Kilmury SLN, Twine SM. The Francisella Tularensis Proteome and its Recognition by Antibodies. Front Microbiol (2010) 1:143. doi: 10.3389/fmicb.2010.00143
28. Nakajima R, Escudero R, Molina DM, Rodríguez-Vargas M, Randall A, Jasinskas A, et al. Towards Development of Improved Serodiagnostics for Tularemia by Use of Francisella Tularensis Proteome Microarrays. J Clin Microbiol (2016) 54(7):1755–65. doi: 10.1128/JCM.02784-15
29. Felgner PL, Kayala MA, Vigil A, Burk C, Nakajima-Sasaki R, Pablo J, et al. A Burkholderia Pseudomallei Protein Microarray Reveals Serodiagnostic and Cross-Reactive Antigens. Proc Natl Acad Sci U S A (2009) 106(32):13499–504. doi: 10.1073/pnas.0812080106
30. Yi J, Simpanya MF, Settles EW, Shannon AB, Hernandez K, Pristo L, et al. Caprine Humoral Response to Burkholderia Pseudomallei Antigens During Acute Melioidosis From Aerosol Exposure. PloS Negl Trop Dis (2019) 13(2):e0006851. doi: 10.1371/journal.pntd.0006851
31. Ashkenazy H, Abadi S, Martz E, Chay O, Mayrose I, Pupko T, et al. ConSurf 2016: An Improved Methodology to Estimate and Visualize Evolutionary Conservation in Macromolecules. Nucleic Acids Res (2016) 44(W1):W344–50. doi: 10.1093/nar/gkw408
32. Ren J, Ellis J, Li J. Influenza A Ha’s Conserved Epitopes and Broadly Neutralizing Antibodies: A Prediction Method. J Bioinform Comput Biol (2014) 12(5):1450023. doi: 10.1142/S0219720014500231
33. Fiuza TS, Lima JPMS, de Souza GA. EpitoCore: Mining Conserved Epitope Vaccine Candidates in the Core Proteome of Multiple Bacteria Strains. Front Immunol (2020) 11:816. doi: 10.3389/fimmu.2020.00816
34. Manavalan B, Govindaraj RG, Shin TH, Kim MO, Lee G. iBCE-EL: A New Ensemble Learning Framework for Improved Linear B-Cell Epitope Prediction. Front Immunol (2018) 9:1695. doi: 10.3389/fimmu.2018.01695
35. Jespersen MC, Peters B, Nielsen M, Marcatili P. BepiPred-2.0: Improving Sequence-Based B-Cell Epitope Prediction Using Conformational Epitopes. Nucleic Acids Res (2017) 45(W1):W24–9. doi: 10.1093/nar/gkx346
36. Conlan JW, Shen H, Golovliov I, Zingmark C, Oyston PC, Chen W, et al. Differential Ability of Novel Attenuated Targeted Deletion Mutants of Francisella Tularensis Subspecies Tularensis Strain SCHU S4 to Protect Mice Against Aerosol Challenge With Virulent Bacteria: Effects of Host Background and Route of Immunization. Vaccine (2010) 28(7):1824–31. doi: 10.1016/j.vaccine.2009.12.001
37. Propst KL, Mima T, Choi KH, Dow SW, Schweizer HP. A Burkholderia Pseudomallei deltapurM Mutant is Avirulent in Immunocompetent and Immunodeficient Animals: Candidate Strain for Exclusion From Select-Agent Lists. Infect Immun (2010) 78(7):3136–43. doi: 10.1128/IAI.01313-09
38. Twine S, Shen H, Harris G, Chen W, Sjostedt A, Ryden P, et al. BALB/c Mice, But Not C57BL/6 Mice Immunized With a ΔclpB Mutant of Francisella tularensis Subspecies Tularensis are Protected Against Respiratory Challenge With Wild-Type Bacteria: Association of Protection With Post-Vaccination and Post-Challenge Immune Responses. Vaccine (2012) 30(24):3634–45. doi: 10.1016/j.vaccine.2012.03.036
39. Lu Z, Rynkiewicz MJ, Madico G, Li S, Yang CY, Perkins HM, et al. B-Cell Epitopes in GroEL of Francisella tularensis. PloS One (2014) 9(6):e99847. doi: 10.1371/journal.pone.0099847
40. Vita R, Mahajan S, Overton JA, Dhanda SK, Martini S, Cantrell JR, et al. The Immune Epitope Database (IEDB): 2018 Update. Nucleic Acids Res (2019) 47(D1):D339–43. doi: 10.1093/nar/gky1006
41. Purcell AW, McCluskey J, Rossjohn J. More Than One Reason to Rethink the Use of Peptides in Vaccine Design. Nat Rev Drug Discovery (2007) 6(5):404–14. doi: 10.1038/nrd2224
42. Sun T, Han H, Hudalla GA, Wen Y, Pompano RR, Collier JH. Thermal Stability of Self-Assembled Peptide Vaccine Materials. Acta Biomater (2016) 30:62–71. doi: 10.1016/j.actbio.2015.11.019
43. Noah CE, Malik M, Bublitz DC, Camenares D, Sellati TJ, Benach JL, et al. GroEL and Lipopolysaccharide From Francisella Tularensis Live Vaccine Strain Synergistically Activate Human Macrophages. Infect Immun (2010) 78(4):1797–806. doi: 10.1128/IAI.01135-09
44. Pechous RD, McCarthy TR, Zahrt TC. Working Toward the Future: Insights Into Francisella tularensis Pathogenesis and Vaccine Development. Microbiol Mol Biol Rev (2009) 73(4):684–711. doi: 10.1128/MMBR.00028-09
45. Weiss DS, Brotcke A, Henry T, Margolis JJ, Chan K, Monack DM. In Vivo Negative Selection Screen Identifies Genes Required for Francisella Virulence. Proc Natl Acad Sci USA (2007) 104(14):6037–42. doi: 10.1073/pnas.0609675104
46. Havlasova J, Hernychova L, Brychta M, Hubalek M, Lenco J, Larsson P, et al. Proteomic Analysis of Anti-Francisella Tularensis LVS Antibody Response in Murine Model of Tularemia. Proteomics (2005) 5(8):2090–103. doi: 10.1002/pmic.200401123
47. Huntley JF, Conley PG, Hagman KE, Norgard MV. Characterization of Francisella tularensis Outer Membrane Proteins. J Bacteriol (2007) 189(2):561–74. doi: 10.1128/JB.01505-06
48. Ericsson M, Tarnvik A, Kuoppa K, Sandstrom G, Sjostedt A. Increased Synthesis of DnaK, GroEL, and GroES Homologs by Francisella Tularensis LVS in Response to Heat and Hydrogen Peroxide. Infect Immun (1994) 62(1):178–83. doi: 10.1128/iai.62.1.178-183.1994
49. Henderson B, Allan E, Coates AR. Stress Wars: The Direct Role of Host and Bacterial Molecular Chaperones in Bacterial Infection. Infect Immun (2006) 74(7):3693–706. doi: 10.1128/IAI.01882-05
50. Hickey TB, Thorson LM, Speert DP, Daffe M, Stokes RW. Mycobacterium Tuberculosis Cpn60.2 and DnaK are Located on the Bacterial Surface, Where Cpn60.2 Facilitates Efficient Bacterial Association With Macrophages. Infect Immun (2009) 77(8):3389–401. doi: 10.1128/IAI.00143-09
51. Lee BY, Horwitz MA, Clemens DL. Identification, Recombinant Expression, Immunolocalization in Macrophages, and T-Cell Responsiveness of the Major Extracellular Proteins of Francisella tularensis. Infect Immun (2006) 74(7):4002–13. doi: 10.1128/IAI.00257-06
52. Ashtekar AR, Zhang P, Katz J, Deivanayagam CC, Rallabhandi P, Vogel SN, et al. TLR4-Mediated Activation of Dendritic Cells by the Heat Shock Protein DnaK From Francisella tularensis. J Leukoc Biol (2008) 84(6):1434–46. doi: 10.1189/jlb.0308215
53. Kol A, Bourcier T, Lichtman AH, Libby P. Chlamydial and Human Heat Shock Protein 60s Activate Human Vascular Endothelium, Smooth Muscle Cells, and Macrophages. J Clin Invest (1999) 103(4):571–7. doi: 10.1172/JCI5310
54. Wallin RP, Lundqvist A, More SH, von Bonin A, Kiessling R, Ljunggren HG. Heat-Shock Proteins as Activators of the Innate Immune System. Trends Immunol (2002) 23(3):130–5. doi: 10.1016/S1471-4906(01)02168-8
55. Valentino MD, Maben ZJ, Hensley LL, Woolard MD, Kawula TH, Frelinger JA, et al. Identification of T-Cell Epitopes in Francisella Tularensis Using an Ordered Protein Array of Serological Targets. Immunology (2011) 132(3):348–60. doi: 10.1111/j.1365-2567.2010.03387.x
56. Ashtekar AR, Katz J, Xu Q, Michalek SM. A Mucosal Subunit Vaccine Protects Against Lethal Respiratory Infection With Francisella tularensis LVS. PloS One (2012) 7(11):e50460. doi: 10.1371/journal.pone.0050460
57. Khan MN, Shukla D, Bansal A, Mustoori S, Ilavazhagan G. Immunogenicity and Protective Efficacy of GroEL (Hsp60) of Streptococcus Pneumoniae Against Lethal Infection in Mice. FEMS Immunol Med Microbiol (2009) 56(1):56–62. doi: 10.1111/j.1574-695X.2009.00548.x
58. Crane DD, Warner SL, Bosio CM. A Novel Role for Plasmin Mediated Degradation of Opsonizing Antibody in the Evasion of Host Immunity by Virulent, But Not Attenuated, Francisella tularensis. J Immunol Baltim Md 1950 (2009) 183(7):4593–600. doi: 10.4049/jimmunol.0901655
59. Clinton SR, Bina JE, Hatch TP, Whitt MA, Miller MA. Binding and Activation of Host Plasminogen on the Surface of Francisella tularensis. BMC Microbiol (2010) 10:76. doi: 10.1186/1471-2180-10-76
60. Shams F, Oldfield NJ, Wooldridge KG, Turner DPJ. Fructose-1,6-Bisphosphate Aldolase (FBA)-A Conserved Glycolytic Enzyme With Virulence Functions in Bacteria: “Ill Met by Moonlight.” Biochem Soc Trans (2014) 42(6):1792–5. doi: 10.1042/BST20140203
61. Kunert A, Losse J, Gruszin C, Hühn M, Kaendler K, Mikkat S, et al. Immune Evasion of the Human Pathogen Pseudomonas Aeruginosa: Elongation Factor Tuf is a Factor H and Plasminogen Binding Protein. J Immunol Baltim Md 1950 (2007) 179(5):2979–88. doi: 10.4049/jimmunol.179.5.2979
62. Varga JJ, Vigil A, DeShazer D, Waag DM, Felgner P, Goldberg JB. Distinct Human Antibody Response to the Biological Warfare Agent Burkholderia mallei. Virulence (2012) 3(6):510–4. doi: 10.4161/viru.22056
63. Burtnick MN, Brett PJ, Harding SV, Ngugi SA, Ribot WJ, Chantratita N, et al. The Cluster 1 Type VI Secretion System Is a Major Virulence Determinant in Burkholderia pseudomallei. Infect Immun (2011) 79(4):1512–25. doi: 10.1128/IAI.01218-10
64. Schell MA, Ulrich RL, Ribot WJ, Brueggemann EE, Hines HB, Chen D, et al. Type VI Secretion is a Major Virulence Determinant in Burkholderia mallei. Mol Microbiol (2007) 64(6):1466–85. doi: 10.1111/j.1365-2958.2007.05734.x
65. Whitlock GC, Deeraksa A, Qazi O, Judy BM, Taylor K, Propst KL, et al. Protective Response to Subunit Vaccination Against Intranasal Burkholderia mallei and B. pseudomallei Challenge. Proc Vaccinol (2010) 2:73–7. doi: 10.1016/j.provac.2010.03.013
66. Li J, Mahajan A, Tsai M-D. Ankyrin Repeat: A Unique Motif Mediating Protein-Protein Interactions. Biochemistry (2006) 45(51):15168–78. doi: 10.1021/bi062188q
67. Pan X, Lührmann A, Satoh A, Laskowski-Arce MA, Roy CR. Ankyrin Repeat Proteins Comprise a Diverse Family of Bacterial Type IV Effectors. Science (2008) 320(5883):1651–4. doi: 10.1126/science.1158160
68. Pancholi V, Chhatwal GS. Housekeeping Enzymes as Virulence Factors for Pathogens. Int J Med Microbiol IJMM (2003) 293(6):391–401. doi: 10.1078/1438-4221-00283
69. Lottenberg R, Minning-Wenz D, Boyle MD. Capturing Host Plasmin(Ogen): A Common Mechanism for Invasive Pathogens? Trends Microbiol (1994) 2(1):20–4. doi: 10.1016/0966-842X(94)90340-9
70. Terrier B, Degand N, Guilpain P, Servettaz A, Guillevin L, Mouthon L. Alpha-Enolase: A Target of Antibodies in Infectious and Autoimmune Diseases. Autoimmun Rev (2007) 6(3):176–82. doi: 10.1016/j.autrev.2006.10.004
71. Al-Maleki AR, Mariappan V, Vellasamy KM, Tay ST, Vadivelu J. Altered Proteome of Burkholderia Pseudomallei Colony Variants Induced by Exposure to Human Lung Epithelial Cells. PloS One (2015) 10(5):e0127398. doi: 10.1371/journal.pone.0127398
72. Sun P, Guo S, Sun J, Tan L, Lu C, Ma Z. Advances in In-Silico B-Cell Epitope Prediction. Curr Top Med Chem (2019) 19(2):105–15. doi: 10.2174/1568026619666181130111827
73. Larsen JEP, Lund O, Nielsen M. Improved Method for Predicting Linear B-Cell Epitopes. Immunome Res (2006) 2:2. doi: 10.1186/1745-7580-2-2
74. Ponomarenko J, Bui H-H, Li W, Fusseder N, Bourne PE, Sette A, et al. ElliPro: A New Structure-Based Tool for the Prediction of Antibody Epitopes. BMC Bioinf (2008) 9:514. doi: 10.1186/1471-2105-9-514
75. Kringelum JV, Lundegaard C, Lund O, Nielsen M. Reliable B Cell Epitope Predictions: Impacts of Method Development and Improved Benchmarking. PloS Comput Biol (2012) 8(12):e1002829. doi: 10.1371/journal.pcbi.1002829
76. Singh H, Ansari HR, Raghava GPS. Improved Method for Linear B-Cell Epitope Prediction Using Antigen’s Primary Sequence. PloS One (2013) 8(5):e62216. doi: 10.1371/journal.pone.0062216
77. Safavi A, Kefayat A, Mahdevar E, Abiri A, Ghahremani F. Exploring the Out of Sight Antigens of SARS-CoV-2 to Design a Candidate Multi-Epitope Vaccine by Utilizing Immunoinformatics Approaches. Vaccine (2020) 38(48):7612–28. doi: 10.1016/j.vaccine.2020.10.016
78. Devi YD, Devi A, Gogoi H, Dehingia B, Doley R, Buragohain AK, et al. Exploring Rotavirus Proteome to Identify Potential B- and T-Cell Epitope Using Computational Immunoinformatics. Heliyon (2020) 6(12):e05760. doi: 10.1016/j.heliyon.2020.e05760
79. Liu T, Shi K, Li W. Deep Learning Methods Improve Linear B-Cell Epitope Prediction. BioData Min (2020) 13:1. doi: 10.1186/s13040-020-00211-0
80. B Cell Help. Available at: http://tools.iedb.org/bcell/help/#Bepipred-2.0.
81. Eickhoff CS, Terry FE, Peng L, Meza KA, Sakala IG, Van Aartsen D, et al. Highly Conserved Influenza T Cell Epitopes Induce Broadly Protective Immunity. Vaccine (2019) 37(36):5371–81. doi: 10.1016/j.vaccine.2019.07.033
82. Grassl N, Kulak NA, Pichler G, Geyer PE, Jung J, Schubert S, et al. Ultra-Deep and Quantitative Saliva Proteome Reveals Dynamics of the Oral Microbiome. Genome Med (2016) 8:44. doi: 10.1186/s13073-016-0293-0
83. Zhang X, Chen W, Ning Z, Mayne J, Mack D, Stintzi A, et al. Deep Metaproteomics Approach for the Study of Human Microbiomes. Anal Chem (2017) 89(17):9407–15. doi: 10.1021/acs.analchem.7b02224
84. Blakeley-Ruiz JA, Erickson AR, Cantarel BL, Xiong W, Adams R, Jansson JK, et al. Metaproteomics Reveals Persistent and Phylum-Redundant Metabolic Functional Stability in Adult Human Gut Microbiomes of Crohn’s Remission Patients Despite Temporal Variations in Microbial Taxa, Genomes, and Proteomes. Microbiome (2019) 7:18. doi: 10.1186/s40168-019-0631-8
85. Long S, Yang Y, Shen C, Wang Y, Deng A, Qin Q, et al. Metaproteomics Characterizes Human Gut Microbiome Function in Colorectal Cancer. NPJ Biofilms Microbiomes (2020) 6:14. doi: 10.1038/s41522-020-0123-4
86. Park SK (Robin), Jung T, Thuy-Boun PS, Wang AY, Yates JR, Wolan DW. ComPIL 2.0: An Updated Comprehensive Metaproteomics Database. J Proteome Res (2019) 18(2):616–22. doi: 10.1021/acs.jproteome.8b00722
Keywords: Francisella, Burkholderia, immunoproteome, B-cell epitope, antigen, peptide vaccine
Citation: D’haeseleer P, Collette NM, Lao V, Segelke BW, Branda SS and Franco M (2021) Shotgun Immunoproteomic Approach for the Discovery of Linear B-Cell Epitopes in Biothreat Agents Francisella tularensis and Burkholderia pseudomallei. Front. Immunol. 12:716676. doi: 10.3389/fimmu.2021.716676
Received: 29 May 2021; Accepted: 02 September 2021;
Published: 29 September 2021.
Edited by:
Shisong Jiang, University of Oxford, United KingdomReviewed by:
Carolyn M. Nielsen, University of Oxford, United KingdomWayne Robert Thomas, University of Western Australia, Australia
Copyright © 2021 D’haeseleer, Collette, Lao, Segelke, Branda and Franco. This is an open-access article distributed under the terms of the Creative Commons Attribution License (CC BY). The use, distribution or reproduction in other forums is permitted, provided the original author(s) and the copyright owner(s) are credited and that the original publication in this journal is cited, in accordance with accepted academic practice. No use, distribution or reproduction is permitted which does not comply with these terms.
*Correspondence: Patrik D’haeseleer, ZGhhZXNlbGVlcjJAbGxubC5nb3Y=