- State Key Laboratory of Ophthalmology, Zhongshan Ophthalmic Center, Sun Yat-Sen University, Guangzhou, China
Dendritic cells (DCs) play essential roles in innate and adaptive immunity and show high heterogeneity and intricate ontogeny. Advances in high-throughput sequencing technologies, particularly single-cell RNA sequencing (scRNA-seq), have improved the understanding of DC subsets. In this review, we discuss in detail the remarkable perspectives in DC reclassification and ontogeny as revealed by scRNA-seq. Moreover, the heterogeneity and multifunction of DCs during diseases as determined by scRNA-seq are described. Finally, we provide insights into the challenges and future trends in scRNA-seq technologies and DC research.
Introduction
Dendritic cells (DCs) are the most efficient antigen-presenting cells and form essential interconnections between innate and adaptive immunity (1, 2). As innate immune components, DCs distinguish and monitor the pathogen‐ and danger‐associated signals and subsequently initiate acute immunological responses. During an adaptive immune response, DCs preprocess various extracellular and intracellular antigens and introduce them to naïve T cells via major histocompatibility complex molecules (3). Generally, DCs are the starting point of both innate and adaptive immune system responses.
The incredible heterogeneity and diversity of DCs are well matched to the precise and intricate functions of the immune system (4, 5). In humans, various extraneous threats prime the immune system to develop effective defensive responses. However, excessive immune responses can lead to the intolerance of autologous antigens. Therefore, an appropriate immune response requires the coexistence of multifarious types of DCs, with each subset trained to respond to specific pathogens and collaborate with relevant T cell subtypes. However, limited by technologies and methodologies, it remains challenging to determine a comprehensive DC subtype atlas and evaluate their biomarkers, cell lineage, and role in immune-related diseases (6, 7). Therefore, a new perspective and rational approaches to DC research are urgently needed.
Since the first report on single-cell transcriptome profiling in 2009 (8), single-cell RNA sequencing (scRNA-seq) technology has greatly advanced. Currently, the major available platforms are based on next-generation sequencing (9–15). Shortly after the evolution of this approach, third-generation sequencing technologies emerged, improving the limited read length of next-generation sequencing (16). The appearance and prevalence of scRNA-seq methods have revolutionized DC research, providing insights into DC heterogeneity and ontogeny. A comprehensive literature review focusing on the application of scRNA-seq in DC research is urgently desired by immunologists.
In this review, we discuss recent landmark findings in DC classification and the lineages determined using scRNA-seq technologies. We focus on the applications of scRNA-seq in pathological states, particularly in the tumor environment. Finally, we describe the challenges and future trends of scRNA-seq technologies and DC research.
Strengths of scRNA-seq Technology
scRNA-seq involves the amplification and sequencing of the cell-specific transcriptome to generate a comprehensive gene expression atlas at single-cell resolution (17, 18). Although all body cells share nearly identical genotypes, each cell contains unique transcriptomic information based on its microenvironment and cell conditions. Bulk sequencing of millions of cells in collective samples yields averaged gene expression profiles of all cells and masks heterogeneity among cells (19, 20). Typically, because immune cells are highly heterogeneous and intricate, some subsets accounting for only a small percentage of the total cells are not detected by traditional methods. Hence, considering the sensitivity to minimal subsets, scRNA-seq provides more detailed results than bulk sequencing (21). Additionally, some of the more recent technologies, such as multicolor flow cytometry and cytometry by time-of-flight, can simultaneously measure over 50 protein markers per cell, providing relatively comprehensive insights into the immune proteome at the single-cell level (22, 23). However, scRNA-seq is an unbiased approach for automatically reclassifying immune subsets, which can overcome the limitations of existing methods and enable the identification of new cell types and states (24, 25).
Determining the myeloid developmental trajectory by traditional methods remains difficult. This limitation of bulk RNA sequencing is particularly magnified in studies of cells in dynamic development—for instance, the evolution of progenitor cells into their final differentiated populations through multiplex transitional phases is challenging to detect by bulk sequencing (26). Moreover, the classical hierarchical staged model of hematopoiesis is mostly based on subjectively purified cell populations, which were passively segmented into partitions while overlooking the discrepancies within gates as well as transition states between gates (27, 28). Because pre-set markers that define a cell population are not required and full-scale descriptions of transcriptomic profiling of rare, even transient, transcriptional states can be determined, scRNA-seq has promoted studies of the DC hierarchy.
DCs undergo differentiation, migrate, and respond to environmental stimuli, showing a wide heterogeneity in their markers and transcriptomic characteristics (4, 29). Diseases, particularly carcinogenesis, amplify this nonuniformity. In some cases, the small number of cells is considered responsible for the pathological state, which is difficult to detect (30, 31). By relying on prior knowledge of subpopulation markers in traditional flow cytometry and histology, the substates of disease-specific DCs may be ignored. The superior ability of scRNA-seq to comprehensively capture transcriptional signatures overcomes this challenge and has improved the understanding of the roles of DC substates during homeostasis and diseases.
Applications of scRNA-seq in DC Studies
As the major antigen-presenting cells, DCs participate in both immunological defense and immune tolerance (32, 33). Functioning in both innate and adaptive immunity, DCs monitor infection sites and danger signals or permeate pathologic environments to take up tumor antigens (34). Upon activating naïve T cells, DCs simultaneously produce various cytokines and chemokines to regulate the immune response (35). Furthermore, DCs play an indispensable tolerogenic role by maintaining immune homeostasis and arresting the autoimmune response (33). The outcome of a rapid and precise immune response and balanced immune tolerance greatly relies on the phenotypic and functional heterogeneity of DCs. In the meanwhile, the complex immune microenvironment and immune response requirements remodel the DC subsets, jointly contributing to the intricate categorization and lineage development (36). Thus, establishing a comprehensive DC atlas is urgent for immunological researches and clinical applications. To this end, single-cell transcriptomics has been successfully used to identify new DC subsets, depict unbiased classifications, map cell lineages, and determine the pathological or protective role of DCs in diseases. Several beneficial reviews have been available on the application of scRNA-seq on myeloid cells (37–41). We discuss the landmark findings of DCs in health and disease as determined by scRNA-seq below (Figure 1).
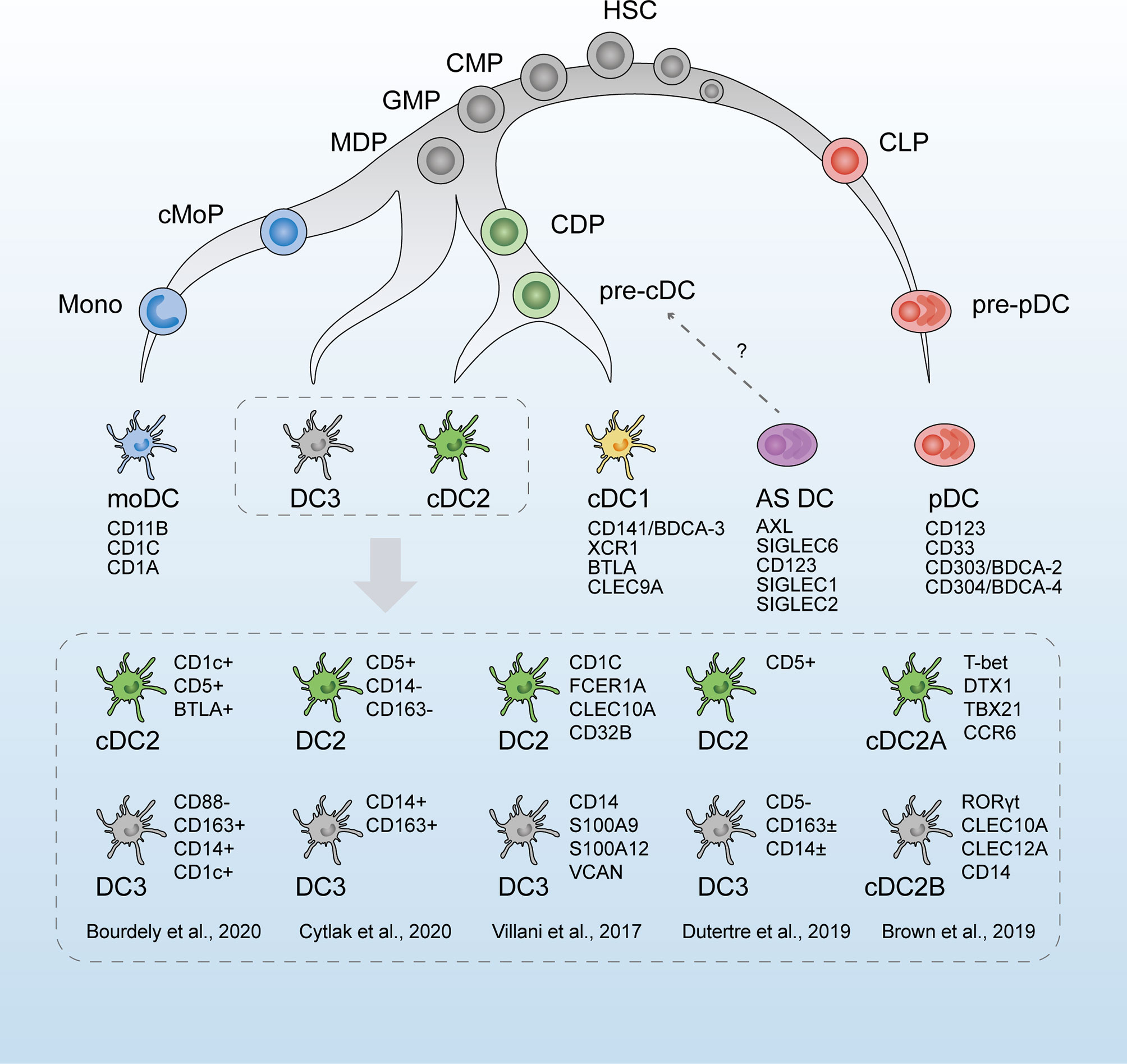
Figure 1 The revised developmental trajectory and classification of human dendritic cells (DCs). The revised developmental trajectory of the DC lineage is continuous, with progenitors already committed to different lineages at the early stages. The classic DC subsets (moDCs, cDC1s, cDC2s, and pDCs) are present. Previous homogenous subset cDC2s is considered heterogeneous, which is subdivided by different markers in scRNA-seq studies by Villani et al. (42), Brown et al. (43), and Dutertre et al. (44). Based on Bourdely et al. (45) and Cytlak et al. (46), DC3s are defined as a new subset, separately derived from MDPs. The origin of pDCs is revised as the lymphoid progenitors. Whether AS DCs (AXL+SIGLEC6+ DCs) are cDC precursors remains to be verified. HSC, hematopoietic stem cells; CMP, common myeloid progenitors; CLP, common lymphoid progenitors; GMP, granulocyte macrophage progenitors; MDP, monocyte–macrophage DC progenitors; CDP, common DC progenitors; cMoP, common monocyte progenitor; Mono, monocytes.
Revision of the Classification of DCs and Identification of New Subsets
The exploration of DC subsets dates from the observation that CD8 was expressed on some, but not all, DCs in mice (47). Polychromatic flow cytometry enabled the assessment of multiple cellular markers and initial exploration of DC phenotypic heterogeneity (48).
The DC classification proposed in 2014 was primarily based on ontogeny, followed by the function and phenotype (49). According to the previous view, the common DC progenitor (CDP)-derived DCs could be subdivided into three main subtypes: (i) type 1 conventional or classical DCs (cDC1s), (ii) type 2 conventional or classical DCs (cDC2s), and (iii) plasmacytoid DCs (pDCs) (49). It was believed that cDCs and pDCs, derived from bone marrow (BM) CDPs, underwent an intermediate stage known as pre-DCs. These precursors then migrated to the peripheral blood and tissues where they matured into cDCs and pDCs (50–53). Generally, CD11c+ cDCs are professional antigen-presenting cells that activate CD4+ and CD8+ T cells (54), whereas pDCs generate type I interferon (IFN) during viral infections. Additionally, monocyte-derived DCs (moDCs) are absent from homeostasis but are common at inflammatory sites (55).
Substantial heterogeneity, accompanied by limited cell numbers, further complicates full-scale DC subtype analysis. Previous studies have found that immune cell subsets, which were considered homogeneous populations defined by surface markers, contain highly heterogeneous multi-component cell types (56, 57). Shalek et al. (58) took the lead to employ scRNA-seq to reveal the heterogeneity of DCs, revealing previously unobserved variations among seemingly identical cells. scRNA-seq has been extensively utilized to decipher the heterogeneous cell groups because of its unbiased approach and high sensitivity for detecting rare cell populations, which would otherwise be neglected by traditional approaches (59, 60). Compared with flow cytometry, single-cell transcriptomic techniques facilitate cell type identification and eliminate the requirement for known surface markers. It is, however, absolutely critical to realize, when interpreting clustered scRNA-seq data, that the technology on its own is unable to discriminate bona fide cell subsets from heterogeneous cell states which belong to the same cell lineage. Identification of new bona fide cell subsets requires additional experimental validation.
A newly proposed classification of DCs dependent on scRNA-seq by Villani et al. (42) in 2017 has led to a new perspective in DC research, which is widely referenced and discussed. Following scRNA-seq analysis and unbiased transcriptomic classification, six main DC groups were identified using a bioinformatic clustering approach and were named DC1–6. Further functional and phenotypic analyses confirmed this unsupervised sorting strategy, thus validating the respective physiological roles of the assumed DC subsets. DC1s analogous to the classic CD141+ cDC1s in humans were discriminated by the surface marker CLEC9A. DC2s and DC3s were two subtypes within CD1c+ cDC2s, the former of which was characterized by a major histocompatibility complex II-like gene set and the latter by a CD14+ monocyte-like gene set. Comparing the two clusters, DC3s strongly expressed an inflammatory gene program represented by CD14, S100A9, and S100A8. DC4s were represented as CD1c-CD141-cDCs, and literature regarding these cells is rare (61). DC5 (or “AS DCs”) was described as a new DC population defined by the expression of AXL, SIGLEC1, and SIGLEC6 markers. The gene expression signatures of AS DCs covered a spectrum between cDC2-like and pDC-like gene sets, suggesting a relationship to both pDCs and cDC2s cells. Classic pDCs were named DC6s.
The real identity of AS DCs remains controversial. This newly discovered DC population was reported to occupy 2 to 3% of human blood DC populations (42), and another scRNA-seq study verified their existence in human cord blood (62). Considerable heterogeneity is observed in AS DCs, as they are captured in both traditional pDC and cDC gates by flow cytometry, and their gene expression profiles cover the spectrum between cDC2-like (e.g., IFI30, ITGAX, LY86, GLIPR20, FGR, LYZ, and ENTPD1) and pDC-like (e.g., IL3RA, IGJ, NRP1, MZB1) gene sets. Villani et al. (42) verified the morphological and functional similarity of AS DCs with cDCs and observed their differentiation towards CD1c+ DCs in vitro. Several scRNA-seq studies supported similar populations as cDC precursors (44, 46, 63). Similarly, Lukowski et al. (64) identified a cluster of Sox4+ cDCs in the murine spleen, displaying a continuum of cDC and pDC lineage-mixed gene signatures, which had a similar transcriptional profile with AS DCs in humans. This population expressed elevated levels of pre-DC features (such as CX3CR1, FLT3, CD33, and CSF1R) and was hypothesized to represent a cell state of pre-DC to cDC transition. Alcántara-Hernández et al. (65) argued that AS DCs were lacking in human skin but detectable in lymphoid tissues, in line with observations for pDCs. In addition, they verified that “pure” pDCs (without AS DCs) activated in vitro could increase their antigen presentation potential and convert towards the cDC-like phenotype (upregulated HLA-DR, CD80, and CD11c). Furthermore, they also identified the murine equivalent of AS DCs and named these “transitional DCs” because of the continuum of pDC and cDC characteristics. This XCR1-CD11b-SiglecH+CX3CRhi DC population shares major similarities with pDCs but inefficiently produces type I IFN (66). Before the identification of this presumptive AS DC population, similar undefined subsets with functional properties incompatible with their phenotype were discovered by flow cytometry, such as a CX3CR1+CD8α+ DC subset in the mouse spleen (belonging to cDCs) with pDC-like functions and transcriptome (67), as well as a CD2hi pDC group that can secrete type I IFN and efficiently trigger T cell proliferation (68) and a CD2hiCD5+CD81+ pDC group in human blood, BM, and tonsil that could stimulate T and B cell activation but hardly produces type I IFN (69). Notably, this DC population has a CD2+CD5+ and pDC-like expression profile in common with AS DCs, which might represent two overlapping clusters (69). Whether these DC populations are homologs with diverse phenotypes in their respective microenvironment and developmental phases remains unclear. Additional research on the developmental trajectory of AS DCs would contribute to validating the identity of AS DCs.
Recent studies by scRNA-seq have expanded the understanding of the heterogeneity of cDC2s. According to Villani et al. (42), CD1c+ DCs were discrepant in the gene signatures, as DC3s expressing CD14 and “monocyte-like” gene signature were characterized by acute and chronic inflammatory genes, whereas DC2s were more similar to cDC1s. Later, in 2019, Dutertre et al. (44) proposed this CD14+CD1c+ DCs as a subpopulation of cDC2s. They identified CD5+ cDC2s to correspond to DC2s and CD5- cDC2s to DC3s by single-cell protein and RNA analysis. This demarcation by CD5 is in line with the results of Yin et al. (70) and Korenfeld et al. (71). Similarly, in murine splenic DCs, two subtypes were divided within cDC2s by scRNA-seq, which were characterized by a mutually exclusive expression of T-bet and RORγt, with divergent pro- and anti-inflammatory roles in vivo (43). However, RORγt+ cDC2s were absent from human blood, and the CD1c+CLEC10A+ cDC2s (DC2,3s) corresponded to T-bet+ cDC2s. An analog to the mouse RORγt+ cDC2s subset was found in the human spleen and defined as CD1cloCLEC10A–CLEC4Ahi cDC2s (43). In addition to the intrinsic heterogeneity of cDC2 subpopulations, interindividual variation was observed by scRNA-seq. The phenotypic profiles and subset frequencies of cDC2s varied dramatically among individuals, unlike the other subsets (65). This discrepancy between species and tissues further complicated the understanding of the cDC2 family.
There remained disputes on whether these putative DC3s belonged to monocytes or cDC2s or just represented a bona fide DC lineage independent of cDCs or pDCs (72). moDCs are the most abundant DC subset at the inflammatory site and arise from recruited monocytes (73). Harboring monocyte-like gene profiles, DC3s were once assigned to moDCs. A circulating CD1c+CD14+CD163+ cDC2 subset, related to the DC3s, was found to expand correlatively with disease activity in patients with systemic lupus erythematosus (SLE). These cells exhibited a pro-inflammatory transcriptomic profile and secreted pro-inflammatory mediators that might contribute to SLE physiopathology (44). Bourdely et al. (45) proposed circulating DC3s as immediate precursors of resident inflammatory DCs, and cells with DC3 phenotype were found to infiltrate breast cancer tissues and correlate positively with resident memory T cells. The overlap of their gene signature and functional ability seems to equate DC3s and moDCs. As monocyte derivation is the salient feature of moDCs, the ontogeny of DC3s should be the most convincing evidence of whether these two components are equivalent. As shown by scRNA-seq (45), DC3s developed directly via a DC3-restricted progenitor contained in early granulocyte–monocyte and DC progenitors, distinct from cDC-restricted CDPs or monocytes. In addition, GM-CSF alone, but not FLT3L, efficiently supported the differentiation of DC3s, in contrast to traditionally defined cDCs. Similarly, Cytlak et al. (46) demonstrated that DC3s developed from granulocyte–monocyte progenitors along an IRF8low trajectory, separate from pDCs, cDC1s, or cDC2s. These scRNA-seq studies supported DC3 as a bona fide DC lineage, independent of cDCs, pDCs, or monocytes (74).
Notably, Calzetti et al. (75) pointed out that DC4s corresponded to a group of CD14dim/- CD16 ++ monocytes by using a gating strategy based on the lack of CD14 expression only; thus, these cells could not be considered as a newly discovered DC population. This finding agrees with that of Dutertre et al. (44), who found that signature genes and markers of DC4s showed the highest expression in CD16+ monocytes but were undetectable in any DCs.
The scRNA-seq cluster-based extension of DC diversity represents a breakthrough in the traditional cognition of DCs and has significantly broadened our understanding of these cells. However, newly identified DC populations remain to be validated by ontogenic and functional verifications, which we discuss hereinafter.
Trajectory Analysis of DC Lineages
Inferring the lineage trajectory based on transcriptomic profiles of individual cells is a promising application of scRNA-seq analysis (76, 77). From the static snapshot data of a population of cells at different stages in a developmental process, the pseudotime trajectory analysis calculates and reconstructs the dynamic differentiation processes. Multiple algorithms have been exploited to achieve the single-cell pseudotime trajectory analysis, including Monocle 2 (78), Monocle 3 (76), Slingshot (79), TSCAN (80), and others. No single method works for all the datasets, and the best practice depends on the structure of the trajectory and the sample size of the dataset. Of note is that, in pseudotime inference, cellular trajectories are determined purely by their transcriptional profiles, which, however, might not completely reflect the cell states (81). Indeed the bifurcations in gene expressions are thought to lag behind the actual fate decisions (82). Hence, it is recommended to combine single-cell transcriptomics with other cellular aspects, such as chromatin state, spatial arrangement, and phosphorylation, to validate the inferred cell transitions and developmental trajectory (83, 84).
A recently introduced concept, RNA velocity, has been developed to infer developmental trajectories in scRNA-seq data (85). Based on the ratio of spliced (mature) and unspliced (nascent) mRNA of an individual gene at a given time point, the positive or negative change in mRNA abundance, namely, RNA velocity, can be used to indicate the future states of cells (86). Velocyto, proposed by La Manno et al., is a potent model to estimate RNA velocity using scRNA-seq (85). More recently, the newly developed method, scVelo (86), breaks the central assumptions of a common splicing rate in RNA velocity and largely shortens the time and lessens the memory consumption than that of Velocyto by solving the full transcriptional dynamics of splicing kinetics using a likelihood-based dynamical model.
According to the previous view, the developmental trajectory of hematopoietic lineage was accepted as stepwise and staged (87, 88). In the BM, the myeloid lineage stemmed from common myeloid progenitors to granulocyte–macrophage progenitors and then transformed into monocyte–macrophage DC progenitors (MDPs). DC-committed precursor cells, known as CDPs, continued. CDPs served as progenitors for pDCs and pre-cDCs. MDP-derived monocytes and CDP-derived pDCs, pre-cDCs, migrated into peripheral tissues through the bloodstream, where monocytes differentiated into moDCs and pre-cDCs into two classical DC subtypes, cDC1s and cDC2s (89).
ScRNA-seq technologies have challenged the previous understandings of the ontogeny of hematopoiesis (90–92). One of the most important notions is that the hematopoietic system is less of a stepwise process than previously suggested. In contrast to the traditional concept of hierarchy deferring to multi-, oligo-, and unipotent progenitors, there is high cell-to-cell variability in the propensity of hematopoietic progenitors to differentiate into separate lineages (93, 94)—for instance, in addition to the reported heterogeneity among CDP populations with distinct differentiation priming towards cDCs and pDCs, Schlitzer et al. (95) focused on the potential differentiation potency towards cDC1s and cDC2s among murine CDPs. Previous studies showed that single CDPs cultured in vitro differentiate preferentially into the cDC1 or cDC2 subsets (50). Single-cell transcriptomic analysis validated that the biased gene expression sets towards cDC1 and cDC2 lineages became visible at the CDP stage in the BM (95). Notably, the transcriptomic signatures overlapped among putative MDP, CDP, and pre-cDC subsets. This finding indicated that cells in the MDP, CDP, or pre-cDC pool had already transformed into the next differentiation stage at the transcriptome level despite the preservation of their protein signatures. Similarly, Bagadia et al. (96) identified the earliest committed cDC1 progenitors within CDPs. This is also the case for pre-cDCs, which already contain committed pre-cDC1s and pre-cDC2s (63).
In contrast to the classical “ball-and-stick” model in which all cells within a population behave equally, Naik et al. (97) proposed a revised continuous model of DC differentiation, where early progenitors have already committed to different lineages. In addition, the “Autobahn” model is introduced by Bassler et al., in which progenitors harbor early cell fate commitment and also multipotent plasticity as an adaptation to environmental changes (39). Two models both highlight the continuum of hierarchical differentiation spanning multiple intermediate states as well as the pre-commitment of progenitors. Comparatively, the Autobahn model stresses more on the changeability of lineage fate to fit in various environmental changes. Lin et al. (98) found that the FLT3 ligand-mediated increase of cDC1 output was achieved by the selective expansion of cDC1-primed progenitors, without comprising other lineages. This observation contradicts the changeable fate mapping of progenitors under external stimuli in the Autobahn model.
pDCs are considered to vary from cDCs in terms of derivation, function, and surface markers (53). The ontogeny of pDCs appeared to be controversial and promiscuous, as contradictory evidence was reported for both a myeloid and lymphoid origin of pDCs (51, 99, 100). Previously, pDCs were classified within the myeloid compartment, but scRNA-seq studies have questioned this hypothesis (101). Herman et al. (102) revealed a common precursor cluster shared by B cells and pDCs, indicating the lymphoid origin of pDCs. Verification was performed by assessing the differentiation potential of sorted precursors. Similarly, Rodrigues et al. (103) proved that pDCs developed mostly from Ly6D+SiglecH+IL-7R+ lymphoid progenitor cells and showed transcriptional and functional heterogeneity from myeloid-derived “pDC-like” cells. Subsequently, in 2019, Dress et al. (104) proposed that pDCs, developed from Ly6D+CD81+ lymphoid progenitors in mice, were completely independent of the myeloid DC lineage. A marked proportion of common lymphoid progenitors harbored a characteristic gene program, which denoted the earliest detected pDC priming. Additionally, they identified the newly discovered pDC-primed Ly6D+ pDC progenitors in every conventionally defined cDC progenitor population, which may account for the inconsistent observations of pDC derivation.
The origin of moDCs and whether the origin depends on environmental factors are other important issues being addressed by many groups. A study involving scRNA-seq showed that the moDC-primed differentiation potency already existed in a separate subset of Ly6C+ monocytes in the murine BM (105). However, an opposing hypothesis was also presented: all human blood CD14+ monocytes are potential moDCs producers, in the case of certain cytokines in conjunction with environmental ligands of the aryl hydrocarbon receptor (106). Consistently, Mildner et al. (107) demonstrated that murine Ly6C+ and Ly6C- monocytes are homogeneous populations according to scRNA-seq.
In summary, scRNA-seq has improved the understanding of the origins and trajectory of DC subset differentiation, identity, and development. Although interesting, some contradictory findings require further exploration.
Application of scRNA-seq in Disease-Related DCs
Tumor-infiltrating myeloid cells (TIM) comprise various immune subsets that could profoundly shape cancer development (108). By directly interacting with malignant cells or indirectly releasing cytokines and chemokines, TIM could condition anti-tumoral protection and also take part in immune surveillance and favor tumor progression under certain circumstances (109). DCs account for a small fraction of TIM but play a key role in presenting tumor antigens and priming T cells (110).
In cancer, cDC1s form the predominant DC population and are specialized to take in the tumor antigens and deliver them to the lymph nodes (LNs); therein, they activate anti-tumoral T cells by cross-presentation (111). In terms of cDC2s, it is considered that they activate CD4+ T cell and Th17 cells but do not deliver antigens to LNs (112). moDCs derived from circulating monocytes can also participate in the tumor microenvironment and function similarly to cDC1s. pDCs uniquely produce pro-inflammatory IFN to promote DC-mediated anti-tumor responses (113). Therefore, deciphering a comprehensive transcriptomic profile of tumor-infiltrating DCs by scRNA-seq has greatly promoted the knowledge of tumor immunity and provided potential targets for anti-tumoral therapy (Figure 2).
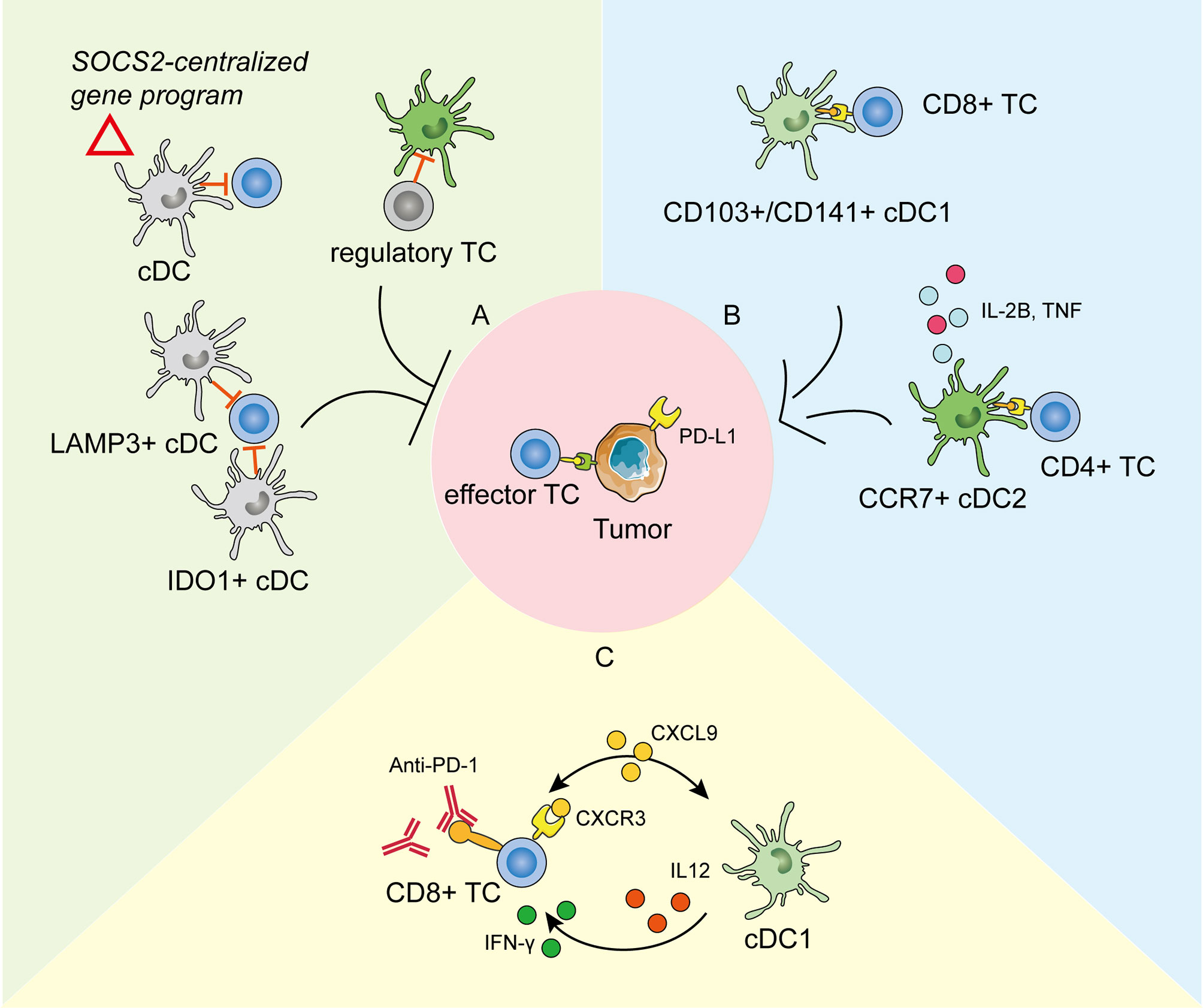
Figure 2 The roles of tumor-infiltrating dendritic cells (DCs) in tumor development and anti-PD-1 immunotherapy as revealed by scRNA-seq. (A) DCs in the tumor environment could be modified to repress the anti-tumoral immunity and promote cancer development in different ways. cDCs expressing the SOCS2-centralized gene program could facilitate tumor immune surveillance (114). Clusters of IDO1+ cDCs and LAMP3+ cDCs are reported to repress the proliferation and function of effector T cells (115, 116). DCs could also be restrained by regulatory T cells (117). (B) cDC1s take in the tumor antigens and activate effector CD8+ T cells (TC). cDC2s activate the CD4+ TCs and secrete pro-inflammatory cytokines to promote anti-tumoral immunity. (C) The crosstalk between cDC1s and CD8+ TCs through chemokines and cytokines is pivotal in response to effective anti-PD-1 immunotherapy.
Generally, the single-cell transcriptomes of DCs in the tumor environment present dynamic alterations during tumor progression (118). Moreover, tumor-infiltrating DCs exhibit unique transcriptional profiles compared with peripheral blood DCs, along with interior heterogeneity (119). Across species, DCs maintain largely conserved, as shown by scRNA-seq of TIM in mice and humans (119, 120). These provide valuable evidence for linking the experimental results in mice with the clinical outcomes and therapeutic responses in humans as well as unfavorable evidence for speculating tumor-infiltrating DCs based on peripheral blood.
Previous studies have reported that tumor-infiltrating cDC1s could effectively enhance effector T cells and protective responses and correlate with a favorable prognosis in several cancers (121–123). By scRNA-seq of different degrees of precursor lesions of pancreatic ductal adenocarcinoma, Bernard et al. (118) found that the proinflammatory immune component in the tumor environment, including activated DCs and cytotoxic T cells, was progressively depleted during neoplastic progression. Accordingly, recent single-cell profiling of early lung adenocarcinoma revealed that human cDC1s were significantly scarce at the tumor site compared to that at non-tumor lesions, which might account for the enrichment of non-functional T cells in tumor lesions (124).
scRNA-seq helped to (117) identify the specialized role and unique function of cDC2s in tumor immunology. Profiling of tumor-draining LNs of mice showed that migratory cDC2s expressing CCR7 were responsible for initiating CD4+ T cell priming, but not differentiation, leading to defective anti-tumoral effector T cells. The therapeutic depletion of regulatory T cells effectively enhanced cDC2 migration and reverted their phenotypical dysfunction, thus enabling productive anti-tumoral CD4+ T cell priming in the LNs (117). This study revealed that the balance between cDC2s and regulatory T cells determined the protective antitumor effect of CD4+ T cells. Aside from antigen presentation, a tumor-enriched cDC2 cluster was reported to highly express pro-inflammatory mediators such as interleukin-1B and tumor necrosis factor (125), which may resemble the reported circulating pro-inflammatory DC3s (42, 44, 45). In line with the anti-tumoral role, a recent scRNA-seq study suggested that the expression of CD207, the characteristic marker of cDC2s, correlated positively with the survival of patients with lung cancer (119).
Except for the anti-tumorigenic roles, DCs can also be modified to actively support cancerogenesis and promote immune escape (126). Normally, mononuclear phagocytes maintain a shared gene program during differentiation and entry into healthy tissues to keep the immune homeostasis (127). However, in some cases, this conserved physiological homeostatic program expressed by migratory DCs might be made use of by tumor-immune surveillance. Single-cell transcriptome profiles of human melanoma metastases suggested that enrichment of this homeostatic signature in DCs functioned in tumor immune escape, which was IFN γ dependent and SOCS2 (suppressor-of-cytokine-2) centralized (114). Depletion of SOCS2 restored anti-tumoral immunity by expanding DC-priming T cell immunity. In addition, a cluster of IDO1+ cDCs was identified in tumor environments and was predicted to impair T cell proliferation and cytotoxicity by promoting tryptophan depletion and kynurenine production (115). Interestingly, a cluster of tolerogenic and regulatory DCs, highly expressing migration (CCR7)- and maturation (LAMP3)-related genes, along with various chemokine ligands (CCL17, CCL19, and CCL22), was presumably able to interact with regulatory T cells and exhausted CD8+ T cells by binding to corresponding receptors in nasopharyngeal carcinoma at single-cell resolution (116). This immune-suppressive transcriptomic pattern in LAMP3+ DCs has been widely detected in various tumors by scRNA-seq, including in bladder urothelial carcinoma (128), hepatocellular carcinoma (129, 130), and lung cancer (119, 131), suggesting that universal crosstalk fosters an immune-suppressive niche for the tumor microenvironment. A pan-cancer single-cell immune atlas covering 15 cancer types verified the broad presence of LAMP3+ DCs, with variable abundance in different cancers (125). Furthermore, AXL and IL-4 signaling partially drive this immunoregulatory program, and IL-4 blocking efficiently rescued the immune depression, providing an antitumor therapy target (131). Zheng et al. (132) added the higher expression of specific transcription factors (including RELB, IRF1, FOXO1, and ETS1) to the features of LAMP3+ DCs in human esophageal squamous cell carcinoma. To further explore their lineage origins, studies have suggested that the tumor-infiltrating LAMP3+ DCs could arise from both cDC1s and cDC2 (129, 131). Notably, these differently originated LAMP3+ DCs maintained specific transcriptomic properties, were regulated by different ligand–receptor pairs, and might perform diverse functions (125). Therefore, as revealed by scRNA-seq, tumor development is accompanied by the transition or differentiation of functional cDCs into a regulatory phenotype or functional defectiveness to create an immunosuppressive milieu.
The crosstalk between tumor-infiltrating DCs and T cells plays an important role in cancer immunotherapy (133, 134). Immune checkpoint blockade (ICB) has emerged as a promising method in cancer treatment (135). Despite considerable clinical responses received, scRNA-seq has promoted the mechanism study to extend the benefits to resistant tumors. Previous studies have demonstrated that the expansion and activation of tumor-infiltrating cDC1s could enhance the therapeutic effect of the PD-1 blockade (110, 122). Based on scRNA-seq analysis on experimental melanoma mouse models, Lelliott et al. (136) showed that combined BRAF, MEK, and CD4/6 inhibition triple therapy could result in the marked depletion of cDC1s from the tumor milieu, the absence of which might contribute to non-response to ICB and poor survival in patients with melanoma. Moreover, the mechanisms of how tumor-infiltrating DCs influence immunotherapy efficiency have become clearer by scRNA-seq. Garris et al. (137) showed that the tumor-infiltrating DC-derived IL-12 was indispensable in effective anti-PD-1 response. Meanwhile, the IFN-γ released by activated CD8+ T cells further activated cDC1s. In line with this, scRNA-seq analysis on a mouse colorectal cancer model MC38 suggested that anti-CD40 agonist specifically activated a subpopulation of cDC1, leading to the upregulation of IL-12, which could enhance Th1 development and IFN-γ production by CD8+ T cells (120). Chow et al. likewise presented that the cellular crosstalk mediated by cDC1s-derived CXCL9 and CXCR3 on T cells was pivotal for the proliferation and function of intratumoral CD8+ T cells in response to anti-PD-1 treatment. Apart from cDCs in tumor immunity, the scRNA-seq of human melanoma biopsies suggested that moDCs correlated with PD-1 responsiveness and effector T cell activity, and more importantly, targeting moDCs by anti-CD40 antibody could enhanced PD-1 ICB efficacy (138).
As antigen-presenting cells, DCs can influence autoimmunity and infection in a complex, even in a bidirectional, manner, as they can promote either immune tolerance or prime T cell differentiation—for instance, moDCs, also described as inflammatory DCs, have been reported to dominate in various inflammations (139). Janela et al. (140) identified the exact dominant DC population during cutaneous bacterial infection and related pathways. They revealed that a minor cell group of activated EpCAM+CD59+Ly-6D+ cDC1s controls neutrophil recruitment to the inflamed site and survival and function by secreting the cytokine vascular endothelial growth factor-α. Dutertre et al. (44, 140) identified a distinct subset of CD5-CD163+CD14+ cDC2s [DC3s in (42)] as circulating inflammatory DCs. The proliferation of inflammatory CD163+CD14+ DC3s was positively correlated with the severity of SLE. In terms of neuroinflammation, Jordão et al. (141) proposed that, although DCs were scarce in the homeostatic central nervous system, their density highly increased during neuroinflammation. DCs and monocyte-derived cells exhibited a high diversity during experimental autoimmune encephalomyelitis and played major roles in antigen presentation to initiate the disease pathology. Martini et al. (142) used scRNA-seq to map the cardiac immune composition in a standard murine nonischemic, pressure-overload heart failure model and found that the relative abundance of DCs decreased in the early and late disease stages. Furthermore, the DC cluster was not homogeneous but could be subdivided into two subclusters, the larger one of which is transcriptionally active and associated with DC differentiation. In a bleomycin-induced pulmonary fibrosis mouse model, Peyser et al. (143) observed a significant increase in the DC population compared to saline-treated lungs. The role of increased DCs in pathogenesis merits a closer examination. Additionally, DCs have been reported to engage in diverse pathogenic and protective mechanisms during atherogenesis (144). Cochain et al. (145) found a cluster of moDCs as the major atherosclerosis-associated cell population, representing 14.9% of the total CD45+ population in the atherosclerotic aorta.
Collectively, transcriptomic studies at the single-cell level can help to understand the phenotypic and functional profiles of DCs in the pathological state and predict the protective or stimulative role in disease development, thereby providing potential targets for treating immune-related diseases.
Challenges and Future Perspectives
In the past 10 years, the application of scRNA-seq technologies has revolutionized the understanding of DC evolution, differentiation, and heterogeneity in homeostatic and pathological states. The historical delineation of cell populations based on known surface markers has been insufficient for comprehensively discriminating subsets, particularly concerning variable pathological conditions. In contrast, unsupervised analysis based on single-cell resolution transcriptomes performs better than conventional flow cytometry and bulk transcriptomic analysis using a minimal set of pre-selected markers.
However, as scRNA-seq captures a snapshot of transcriptomic profiles and DCs are dynamic cells, this approach is insufficient to define a bona fide DC subset or lineage as opposed to a transitory cell state of the same lineage. As the gene expression signatures of DCs are changing dynamically, responding to environmental stimuli and functional requirements, scRNA-seq-derived cell clusters based on differential transcriptomic gene expression should not be confused with the identification of bona fide cell subsets, but clusters can represent a cell subset or a cell state. This cell state can be related to the cell cycle of the cell, metabolic activity, ontogeny, maturation, or the microenvironment in which it resides. As mentioned above, the same population, cDC2, might be described and interpreted in divergent manners by different researchers. Hence, for a new cell type to be accepted beyond the precondition of discrepant transcriptomic programs, complementary functional and ontogenic evidence, that is, separate developmental pathways controlled by specific transcription factors, is necessary to determine a scRNA-seq-defined subset.
In addition, the challenges of defining subsets also lie in technologies and algorithms (146). Clustering is the key step in defining cell types based on the transcriptome. Despite unsupervised computational methods, the specific cluster resolution is set manually, which determines how many clusters are divided in the dataset. Computational methods aiding the selection of clustering resolution exist, but a final judgment and decision from the user are required (147). Under-clustering can hide a rare but biologically relevant population. By contrast, over-clustering can result in partitioning a population into several clusters that simply represent stochastic variations instead of biological states. Due to the low abundance of RNA captured from single cells, “dropouts” (zero reads detected in some cells but with a relatively high expression in others) are inevitably more common in scRNA-seq than in bulk sequencing, thus reducing the reliability of the results. Some imputation methods are developed to reduce the dropout effects before in-depth analysis (148, 149), but this inference still relies on information from the local cell community.
One promising future direction for scRNA-seq is the integration of the DC atlas across different species. A better understanding of the counterparts of DC subsets in humans and mice will lead to the development of potential clinical benefits. Moreover, single-cell multi-omics studies have received increasing research attention. Thus far, transcriptomes, methylomes, proteomes, and epigenomes from the same cell have been obtained and analyzed. Further studies will facilitate improvements in single-cell metabolomics and single-cell proteomes as well as integration of multi-omics single-cell analysis. scRNA-seq may be useful for mapping the DC atlas across species through an accurate and unbiased classification of subsets, and the intricate ontogeny of development and metabolomics, epigenomics, and proteomics can reveal how DCs function in physiological and pathological conditions.
Author Contributions
WS contributed to the conception and design of this study. BC, LZ, and SY contributed to the drafting and revising of the article. WS gave final approval. All authors contributed to the article and approved the submitted version.
Funding
This study was supported by the National Key Research and Development Program of China (2017YFA0105804).
Conflict of Interest
The authors declare that the research was conducted in the absence of any commercial or financial relationships that could be construed as a potential conflict of interest.
Publisher’s Note
All claims expressed in this article are solely those of the authors and do not necessarily represent those of their affiliated organizations, or those of the publisher, the editors and the reviewers. Any product that may be evaluated in this article, or claim that may be made by its manufacturer, is not guaranteed or endorsed by the publisher.
References
1. Banchereau J, Steinman RM. Dendritic Cells and the Control of Immunity. Nature (1998) 392:245–52. doi: 10.1038/32588
2. Qian C, Cao X. Dendritic Cells in the Regulation of Immunity and Inflammation. Semin Immunol (2018) 35:3–11. doi: 10.1016/j.smim.2017.12.002
3. Reis e Sousa C. Activation of Dendritic Cells: Translating Innate Into Adaptive Immunity. Curr Opin Immunol (2004) 16(1):21–5. doi: 10.1016/j.coi.2003.11.007
4. Patente TA, Pinho MP, Oliveira AA, Evangelista G, Bergami-Santos PC, Barbuto J. Human Dendritic Cells: Their Heterogeneity and Clinical Application Potential in Cancer Immunotherapy. Front Immunol (2018) 9:3176. doi: 10.3389/fimmu.2018.03176
5. Hashimoto D, Miller J, Merad M. Dendritic Cell and Macrophage Heterogeneity In Vivo. Immunity (2011) 35(3):323–35. doi: 10.1016/j.immuni.2011.09.007
6. Geissmann F, Gordon S, Hume DA, Mowat AM, Randolph GJ. Unravelling Mononuclear Phagocyte Heterogeneity. Nat Rev Immunol (2010) 10(6):453–60. doi: 10.1038/nri2784
7. Hume DA. Applications of Myeloid-Specific Promoters in Transgenic Mice Support In Vivo Imaging and Functional Genomics But do Not Support the Concept of Distinct Macrophage and Dendritic Cell Lineages or Roles in Immunity. J Leukoc Biol (2011) 89(4):525–38. doi: 10.1189/jlb.0810472
8. Tang F, Barbacioru C, Wang Y, Nordman E, Lee C, Xu N, et al. mRNA-Seq Whole-Transcriptome Analysis of a Single Cell. Nat Methods (2009) 6(5):377–82. doi: 10.1038/nmeth.1315
9. Ramsköld D, Luo S, Wang YC, Li R, Deng Q, Faridani OR, et al. Full-Length mRNA-Seq From Single-Cell Levels of RNA and Individual Circulating Tumor Cells. Nat Biotechnol (2012) 30(8):777–82. doi: 10.1038/nbt.2282
10. Gierahn TM, Wadsworth MH 2nd, Hughes TK, Bryson BD, Butler A, Satija R, et al. Seq-Well: Portable, Low-Cost RNA Sequencing of Single Cells at High Throughput. Nat Methods (2017) 14(4):395–8. doi: 10.1038/nmeth.4179
11. Zheng GX, Terry JM, Belgrader P, Ryvkin P, Bent ZW, Wilson R, et al. Massively Parallel Digital Transcriptional Profiling of Single Cells. Nat Commun (2017) 8:14049. doi: 10.1038/ncomms14049
12. Klein AM, Mazutis L, Akartuna I, Tallapragada N, Veres A, Li V, et al. Droplet Barcoding for Single-Cell Transcriptomics Applied to Embryonic Stem Cells. Cell (2015) 161(5):1187–201. doi: 10.1016/j.cell.2015.04.044
13. Picelli S, Faridani OR, Björklund AK, Winberg G, Sagasser S, Sandberg R. Full-Length RNA-Seq From Single Cells Using Smart-Seq2. Nat Protoc (2014) 9(1):171–81. doi: 10.1038/nprot.2014.006
14. Islam S, Kjällquist U, Moliner A, Zajac P, Fan JB, Lönnerberg P, et al. Characterization of the Single-Cell Transcriptional Landscape by Highly Multiplex RNA-Seq. Genome Res (2011) 21(7):1160–7. doi: 10.1101/gr.110882.110
15. Jaitin DA, Kenigsberg E, Keren-Shaul H, Elefant N, Paul F, Zaretsky I, et al. Massively Parallel Single-Cell RNA-Seq for Marker-Free Decomposition of Tissues Into Cell Types. Science (2014) 343(6172):776–9. doi: 10.1126/science.1247651
16. van Dijk EL, Jaszczyszyn Y, Naquin D, Thermes C. The Third Revolution in Sequencing Technology. Trends Genet (2018) 34(9):666–81. doi: 10.1016/j.tig.2018.05.008
17. Papalexi E, Satija R. Single-Cell RNA Sequencing to Explore Immune Cell Heterogeneity. Nat Rev Immunol (2018) 18(1):35–45. doi: 10.1038/nri.2017.76
18. Hedlund E, Deng Q. Single-Cell RNA Sequencing: Technical Advancements and Biological Applications. Mol Aspects Med (2018) 59:36–46. doi: 10.1016/j.mam.2017.07.003
19. Suvà ML, Tirosh I. Single-Cell RNA Sequencing in Cancer: Lessons Learned and Emerging Challenges. Mol Cell (2019) 75(1):7–12. doi: 10.1016/j.molcel.2019.05.003
20. Stark R, Grzelak M, Hadfield J. RNA Sequencing: The Teenage Years. Nat Rev Genet (2019) 20(11):631–56. doi: 10.1038/s41576-019-0150-2
21. Kulkarni A, Anderson AG, Merullo DP, Konopka G. Beyond Bulk: A Review of Single Cell Transcriptomics Methodologies and Applications. Curr Opin Biotechnol (2019) 58:129–36. doi: 10.1016/j.copbio.2019.03.001
22. Bandura DR, Baranov VI, Ornatsky OI, Antonov A, Kinach R, Lou X, et al. Mass Cytometry: Technique for Real Time Single Cell Multitarget Immunoassay Based on Inductively Coupled Plasma Time-of-Flight Mass Spectrometry. Anal Chem (2009) 81(16):6813–22. doi: 10.1021/ac901049w
23. Monneret G, Gossez M, Aghaeepour N, Gaudilliere B, Venet F. How Clinical Flow Cytometry Rebooted Sepsis Immunology. Cytomet A (2019) 95(4):431–41. doi: 10.1002/cyto.a.23749
24. Choi YH, Kim JK. Dissecting Cellular Heterogeneity Using Single-Cell RNA Sequencing. Mol Cells (2019) 42(3):189–99. doi: 10.14348/molcells.2019.2446
25. Todorov H, Saeys Y. Computational Approaches for High-Throughput Single-Cell Data Analysis. FEBS J (2019) 286(8):1451–67. doi: 10.1111/febs.14613
26. Philip M, Schietinger A. Heterogeneity and Fate Choice: T Cell Exhaustion in Cancer and Chronic Infections. Curr Opin Immunol (2019) 58:98–103. doi: 10.1016/j.coi.2019.04.014
27. Cheng H, Zheng Z, Cheng T. New Paradigms on Hematopoietic Stem Cell Differentiation. Protein Cell (2020) 11(1):34–44. doi: 10.1007/s13238-019-0633-0
28. Velten L, Haas SF, Raffel S, Blaszkiewicz S, Islam S, Hennig BP, et al. Human Haematopoietic Stem Cell Lineage Commitment Is a Continuous Process. Nat Cell Biol (2017) 19(4):271–81. doi: 10.1038/ncb3493
29. Helft J, Ginhoux F, Bogunovic M, Merad M. Origin and Functional Heterogeneity of Non-Lymphoid Tissue Dendritic Cells in Mice. Immunol Rev (2010) 234(1):55–75. doi: 10.1111/j.0105-2896.2009.00885.x
30. Wing JB, Tanaka A, Sakaguchi S. Human FOXP3+ Regulatory T Cell Heterogeneity and Function in Autoimmunity and Cancer. Immunity (2019) 50(2):302–16. doi: 10.1016/j.immuni.2019.01.020
31. McGranahan N, Swanton C. Clonal Heterogeneity and Tumor Evolution: Past, Present, and the Future. Cell (2017) 168(4):613–28. doi: 10.1016/j.cell.2017.01.018
32. Waisman A, Lukas D, Clausen BE, Yogev N. Dendritic Cells as Gatekeepers of Tolerance. Semin Immunopathol (2017) 39(2):153–63. doi: 10.1007/s00281-016-0583-z
33. Iberg CA, Jones A, Hawiger D. Dendritic Cells As Inducers of Peripheral Tolerance. Trends Immunol (2017) 38(11):793–804. doi: 10.1016/j.it.2017.07.007
34. Steinman RM. Decisions About Dendritic Cells: Past, Present, and Future. Annu Rev Immunol (2012) 30:1–22. doi: 10.1146/annurev-immunol-100311-102839
35. Hugues S, Boissonnas A, Amigorena S, Fetler L. The Dynamics of Dendritic Cell-T Cell Interactions in Priming and Tolerance. Curr Opin Immunol (2006) 18(4):491–5. doi: 10.1016/j.coi.2006.03.021
36. Guilliams M, Henri S, Tamoutounour S, Ardouin L, Schwartz-Cornil I, Dalod M, et al. From Skin Dendritic Cells to a Simplified Classification of Human and Mouse Dendritic Cell Subsets. Eur J Immunol (2010) 40(8):2089–94. doi: 10.1002/eji.201040498
37. Bosteels C, Scott CL. Transcriptional Regulation of DC Fate Specification. Mol Immunol (2020) 121:38–46. doi: 10.1016/j.molimm.2020.02.021
38. Villar J, Segura E. Decoding the Heterogeneity of Human Dendritic Cell Subsets. Trends Immunol (2020) 41(12):1062–71. doi: 10.1016/j.it.2020.10.002
39. Bassler K, Schulte-Schrepping J, Warnat-Herresthal S, Aschenbrenner AC, Schultze JL. The Myeloid Cell Compartment-Cell by Cell. Annu Rev Immunol (2019) 37:269–93. doi: 10.1146/annurev-immunol-042718-041728
40. Schultze JL, Aschenbrenner AC. Systems Immunology Allows a New View on Human Dendritic Cells. Semin Cell Dev Biol (2019) 86:15–23. doi: 10.1016/j.semcdb.2018.02.017
41. Dress RJ, Liu Z, Ginhoux F. Towards the Better Understanding of Myelopoiesis Using Single-Cell Technologies. Mol Immunol (2020) 122:186–92. doi: 10.1016/j.molimm.2020.04.020
42. Villani AC, Satija R, Reynolds G, Sarkizova S, Shekhar K, Fletcher J, et al. Single-Cell RNA-Seq Reveals New Types of Human Blood Dendritic Cells, Monocytes, and Progenitors. Science (2017) 356(6335):eaah4573. doi: 10.1126/science.aah4573
43. Brown CC, Gudjonson H, Pritykin Y, Deep D, Lavallée VP, Mendoza A, et al. Transcriptional Basis of Mouse and Human Dendritic Cell Heterogeneity. Cell (2019) 179(4):846–63.e24. doi: 10.1016/j.cell.2019.09.035
44. Dutertre CA, Becht E, Irac SE, Khalilnezhad A, Narang V, Khalilnezhad S, et al. Single-Cell Analysis of Human Mononuclear Phagocytes Reveals Subset-Defining Markers and Identifies Circulating Inflammatory Dendritic Cells. Immunity (2019) 51(3):573–89.e8. doi: 10.1016/j.immuni.2019.08.008
45. Bourdely P, Anselmi G, Vaivode K, Ramos RN, Missolo-Koussou Y, Hidalgo S, et al. Transcriptional and Functional Analysis of CD1c+ Human Dendritic Cells Identifies a CD163+ Subset Priming CD8+CD103+ T Cells. Immunity (2020) 53(2):335–52.e8. doi: 10.1016/j.immuni.2020.06.002
46. Cytlak U, Resteu A, Pagan S, Green K, Milne P, Maisuria S, et al. Differential IRF8 Transcription Factor Requirement Defines Two Pathways of Dendritic Cell Development in Humans. Immunity (2020) 53(2):353–70.e8. doi: 10.1016/j.immuni.2020.07.003
47. Vremec D, Zorbas M, Scollay R, Saunders DJ, Ardavin CF, Wu L, et al. The Surface Phenotype of Dendritic Cells Purified From Mouse Thymus and Spleen: Investigation of the CD8 Expression by a Subpopulation of Dendritic Cells. J Exp Med (1992) 176(1):47–58. doi: 10.1084/jem.176.1.47
48. Gondois-Rey F, Granjeaud S, Kieu Sle T, Herrera D, Hirsch I, Olive D. Multiparametric Cytometry for Exploration of Complex Cellular Dynamics. Cytomet A (2012) 81(4):332–42. doi: 10.1002/cyto.a.22016
49. Guilliams M, Ginhoux F, Jakubzick C, Naik SH, Onai N, Schraml BU, et al. Dendritic Cells, Monocytes and Macrophages: A Unified Nomenclature Based on Ontogeny. Nat Rev Immunol (2014) 14(8):571–8. doi: 10.1038/nri3712
50. Naik SH, Sathe P, Park HY, Metcalf D, Proietto AI, Dakic A, et al. Development of Plasmacytoid and Conventional Dendritic Cell Subtypes From Single Precursor Cells Derived. Vitro Vivo Nat Immunol (2007) 8(11):1217–26. doi: 10.1038/ni1522
51. Onai N, Obata-Onai A, Schmid MA, Ohteki T, Jarrossay D, Manz MG. Identification of Clonogenic Common Flt3+M-CSFR+ Plasmacytoid and Conventional Dendritic Cell Progenitors in Mouse Bone Marrow. Nat Immunol (2007) 8(11):1207–16. doi: 10.1038/ni1518
52. Diao J, Winter E, Chen W, Cantin C, Cattral MS. Characterization of Distinct Conventional and Plasmacytoid Dendritic Cell-Committed Precursors in Murine Bone Marrow. J Immunol (2004) 173(3):1826–33. doi: 10.4049/jimmunol.173.3.1826
53. Reizis B. Plasmacytoid Dendritic Cells: Development, Regulation, and Function. Immunity (2019) 50(1):37–50. doi: 10.1016/j.immuni.2018.12.027
54. Cabeza-Cabrerizo M, Cardoso A, Minutti CM, Pereira da Costa M, Reis E Sousa C. Dendritic Cells Revisited. Annu Rev Immunol (2021) 39:131–66. doi: 10.1146/annurev-immunol-061020-053707
55. Liu K, Nussenzweig MC. Origin and Development of Dendritic Cells. Immunol Rev (2010) 234(1):45–54. doi: 10.1111/j.0105-2896.2009.00879.x
56. Chen Z, Han Y, Gu Y, Liu Y, Jiang Z, Zhang M, et al. CD11c(high)CD8+ Regulatory T Cell Feedback Inhibits CD4 T Cell Immune Response. Via Fas Ligand-Fas Pathway J Immunol (2013) 190(12):6145–54. doi: 10.4049/jimmunol.1300060
57. Tussiwand R, Everts B, Grajales-Reyes GE, Kretzer NM, Iwata A, Bagaitkar J, et al. Klf4 Expression in Conventional Dendritic Cells is Required for T Helper 2 Cell Responses. Immunity (2015) 42(5):916–28. doi: 10.1016/j.immuni.2015.04.017
58. Shalek AK, Satija R, Adiconis X, Gertner RS, Gaublomme JT, Raychowdhury R, et al. Single-Cell Transcriptomics Reveals Bimodality in Expression and Splicing in Immune Cells. Nature (2013) 498(7453):236–40. doi: 10.1038/nature12172
59. Savas P, Virassamy B, Ye C, Salim A, Mintoff CP, Caramia F, et al. Single-Cell Profiling of Breast Cancer T Cells Reveals a Tissue-Resident Memory Subset Associated With Improved Prognosis. Nat Med (2018) 24(7):986–93. doi: 10.1038/s41591-018-0078-7
60. Olah M, Menon V, Habib N, Taga MF, Ma Y, Yung CJ, et al. Single Cell RNA Sequencing of Human Microglia Uncovers a Subset Associated With Alzheimer’s Disease. Nat Commun (2020) 11(1):6129. doi: 10.1038/s41467-020-19737-2
61. Abolhalaj M, Askmyr D, Sakellariou CA, Lundberg K, Greiff L, Lindstedt M. Profiling Dendritic Cell Subsets in Head and Neck Squamous Cell Tonsillar Cancer and Benign Tonsils. Sci Rep (2018) 8(1):8030. doi: 10.1038/s41598-018-26193-y
62. Jin X, Meng L, Yin Z, Yu H, Zhang L, Liang W, et al. Characterization of Dendritic Cell Subtypes in Human Cord Blood by Single-Cell Sequencing. Biophys Rep (2019) 5(4):199. doi: 10.1007/s41048-019-00096-5
63. See P, Dutertre CA, Chen J, Günther P, McGovern N, Irac SE, et al. Mapping the Human DC Lineage Through the Integration of High-Dimensional Techniques. Science (2017) 356(6342):eaag3009. doi: 10.1126/science.aag3009
64. Lukowski SW, Rødahl I, Kelly S, Yu M, Gotley J, Zhou C, et al. Absence of Batf3 Reveals a New Dimension of Cell State Heterogeneity Within Conventional Dendritic Cells. iScience (2021) 24(5):102402. doi: 10.1016/j.isci.2021.102402
65. Alcántara-Hernández M, Leylek R, Wagar LE, Engleman EG, Keler T, Marinkovich MP, et al. High-Dimensional Phenotypic Mapping of Human Dendritic Cells Reveals Interindividual Variation and Tissue Specialization. Immunity (2017) 47(6):1037–50.e6. doi: 10.1016/j.immuni.2017.11.001
66. Leylek R, Alcántara-Hernández M, Lanzar Z, Lüdtke A, Perez OA, Reizis B, et al. Integrated Cross-Species Analysis Identifies a Conserved Transitional Dendritic Cell Population. Cell Rep (2019) 29(11):3736–50.e8. doi: 10.1016/j.celrep.2019.11.042
67. Bar-On L, Birnberg T, Lewis KL, Edelson BT, Bruder D, Hildner K, et al. CX3CR1+ CD8alpha+ Dendritic Cells are a Steady-State Population Related to Plasmacytoid Dendritic Cells. Proc Natl Acad Sci USA (2010) 107(33):14745–50. doi: 10.1073/pnas.1001562107
68. Matsui T, Connolly JE, Michnevitz M, Chaussabel D, Yu CI, Glaser C, et al. CD2 Distinguishes Two Subsets of Human Plasmacytoid Dendritic Cells With Distinct Phenotype and Functions. J Immunol (2009) 182(11):6815–23. doi: 10.4049/jimmunol.0802008
69. Zhang H, Gregorio JD, Iwahori T, Zhang X, Choi O, Tolentino LL, et al. A Distinct Subset of Plasmacytoid Dendritic Cells Induces Activation and Differentiation of B and T Lymphocytes. Proc Natl Acad Sci USA (2017) 114(8):1988–93. doi: 10.1073/pnas.1610630114
70. Yin X, Yu H, Jin X, Li J, Guo H, Shi Q, et al. Human Blood CD1c+ Dendritic Cells Encompass CD5high and CD5low Subsets That Differ Significantly in Phenotype, Gene Expression, and Functions. J Immunol (2017) 198(4):1553–64. doi: 10.4049/jimmunol.1600193
71. Korenfeld D, Gorvel L, Munk A, Man J, Schaffer A, Tung T, et al. A Type of Human Skin Dendritic Cell Marked by CD5 Is Associated With the Development of Inflammatory Skin Disease. JCI Insight (2017) 2(18):e96101. doi: 10.1172/jci.insight.96101
72. Heger L, Hofer TP, Bigley V, de Vries I, Dalod M, Dudziak D, et al. Subsets of CD1c+ DCs: Dendritic Cell Versus Monocyte Lineage. Front Immunol (2020) 11:559166. doi: 10.3389/fimmu.2020.559166
73. León B, López-Bravo M, Ardavín C. Monocyte-Derived Dendritic Cells Formed at the Infection Site Control the Induction of Protective T Helper 1 Responses Against Leishmania. Immunity (2007) 26(4):519–31. doi: 10.1016/j.immuni.2007.01.017
74. Villar J, Segura E. The More, the Merrier: DC3s Join the Human Dendritic Cell Family. Immunity (2020) 53(2):233–5. doi: 10.1016/j.immuni.2020.07.014
75. Calzetti F, Tamassia N, Micheletti A, Finotti G, Bianchetto-Aguilera F, Cassatella MA. Human Dendritic Cell Subset 4 (DC4) Correlates to a Subset of CD14dim/-CD16++ Monocytes. J Allergy Clin Immunol (2018) 141(6):2276–9.e3. doi: 10.1016/j.jaci.2017.12.988
76. Cao J, Spielmann M, Qiu X, Huang X, Ibrahim DM, Hill AJ, et al. The Single-Cell Transcriptional Landscape of Mammalian Organogenesis. Nature (2019) 566(7745):496–502. doi: 10.1038/s41586-019-0969-x
77. Chen H, Albergante L, Hsu JY, Lareau CA, Lo Bosco G, Guan J, et al. Single-Cell Trajectories Reconstruction, Exploration and Mapping of Omics Data With STREAM. Nat Commun (2019) 10(1):1903. doi: 10.1038/s41467-019-09670-4
78. Qiu X, Mao Q, Tang Y, Wang L, Chawla R, Pliner HA, et al. Reversed Graph Embedding Resolves Complex Single-Cell Trajectories. Nat Methods (2017) 14(10):979–82. doi: 10.1038/nmeth.4402
79. Street K, Risso D, Fletcher RB, Das D, Ngai J, Yosef N, et al. Slingshot: Cell Lineage and Pseudotime Inference for Single-Cell Transcriptomics. BMC Genomics (2018) 19(1):477. doi: 10.1186/s12864-018-4772-0
80. Ji Z, Ji H. TSCAN: Pseudo-Time Reconstruction and Evaluation in Single-Cell RNA-Seq Analysis. Nucleic Acids Res (2016) 44(13):e117. doi: 10.1093/nar/gkw430
81. Tritschler S, Büttner M, Fischer DS, Lange M, Bergen V, Lickert H, et al. Concepts and Limitations for Learning Developmental Trajectories From Single Cell Genomics. Development (2019) 146(12):dev170506. doi: 10.1242/dev.170506
82. Weinreb C, Rodriguez-Fraticelli A, Camargo FD, Klein AM. Lineage Tracing on Transcriptional Landscapes Links State to Fate During Differentiation. Science (2020) 367(6479):eaaw3381. doi: 10.1126/science.aaw3381
83. McKenna A, Gagnon JA. Recording Development With Single Cell Dynamic Lineage Tracing. Development (2019) 146(12):dev169730. doi: 10.1242/dev.169730
84. Ludwig CH, Bintu L. Mapping Chromatin Modifications at the Single Cell Level. Development (2019) 146(12):dev170217. doi: 10.1242/dev.170217
85. La Manno G, Soldatov R, Zeisel A, Braun E, Hochgerner H, Petukhov V, et al. RNA Velocity of Single Cells. Nature (2018) 560(7719):494–8. doi: 10.1038/s41586-018-0414-6
86. Bergen V, Lange M, Peidli S, Wolf FA, Theis FJ. Generalizing RNA Velocity to Transient Cell States Through Dynamical Modeling. Nat Biotechnol (2020) 38(12):1408–14. doi: 10.1038/s41587-020-0591-3
87. Akashi K, Traver D, Miyamoto T, Weissman IL. A Clonogenic Common Myeloid Progenitor That Gives Rise to All Myeloid Lineages. Nature (2000) 404(6774):193–7. doi: 10.1038/35004599
88. Chao MP, Seita J, Weissman IL. Establishment of a Normal Hematopoietic and Leukemia Stem Cell Hierarchy. Cold Spring Harb Symp Quant Biol (2008) 73:439–49. doi: 10.1101/sqb.2008.73.031
89. Merad M, Sathe P, Helft J, Miller J, Mortha A. The Dendritic Cell Lineage: Ontogeny and Function of Dendritic Cells and Their Subsets in the Steady State and the Inflamed Setting. Annu Rev Immunol (2013) 31:563–604. doi: 10.1146/annurev-immunol-020711-074950
90. Jacobsen S, Nerlov C. Haematopoiesis in the Era of Advanced Single-Cell Technologies. Nat Cell Biol (2019) 21(1):2–8. doi: 10.1038/s41556-018-0227-8
91. Athanasiadis EI, Botthof JG, Andres H, Ferreira L, Lio P, Cvejic A. Single-Cell RNA-Sequencing Uncovers Transcriptional States and Fate Decisions in Haematopoiesis. Nat Commun (2017) 8(1):2045. doi: 10.1038/s41467-017-02305-6
92. Giladi A, Paul F, Herzog Y, Lubling Y, Weiner A, Yofe I, et al. Single-Cell Characterization of Haematopoietic Progenitors and Their Trajectories in Homeostasis and Perturbed Haematopoiesis. Nat Cell Biol (2018) 20(7):836–46. doi: 10.1038/s41556-018-0121-4
93. Paul F, Arkin Y, Giladi A, Jaitin DA, Kenigsberg E, Keren-Shaul H, et al. Transcriptional Heterogeneity and Lineage Commitment in Myeloid Progenitors. Cell (2015) 163(7):1663–77. doi: 10.1016/j.cell.2015.11.013
94. Helft J, Anjos-Afonso F, van der Veen AG, Chakravarty P, Bonnet D, Reis e Sousa C. Dendritic Cell Lineage Potential in Human Early Hematopoietic Progenitors. Cell Rep (2017) 20(3):529–37. doi: 10.1016/j.celrep.2017.06.075
95. Schlitzer A, Sivakamasundari V, Chen J, Sumatoh HR, Schreuder J, Lum J, et al. Identification of Cdc1- and Cdc2-Committed DC Progenitors Reveals Early Lineage Priming at the Common DC Progenitor Stage in the Bone Marrow. Nat Immunol (2015) 16(7):718–28. doi: 10.1038/ni.3200
96. Bagadia P, Huang X, Liu TT, Durai V, Grajales-Reyes GE, Nitschké M, et al. An Nfil3-Zeb2-Id2 Pathway Imposes Irf8 Enhancer Switching During Cdc1 Development. Nat Immunol (2019) 20(9):1174–85. doi: 10.1038/s41590-019-0449-3
97. Naik SH. Dendritic Cell Development at a Clonal Level Within a Revised ‘Continuous’ Model of Haematopoiesis. Mol Immunol (2020) 124:190–7. doi: 10.1016/j.molimm.2020.06.012
98. Lin DS, Tian L, Tomei S, Amann-Zalcenstein D, Baldwin TM, Weber TS, et al. Single-Cell Analyses Reveal the Clonal and Molecular Aetiology of Flt3L-Induced Emergency Dendritic Cell Development. Nat Cell Biol (2021) 23(3):219–31. doi: 10.1038/s41556-021-00636-7
99. Sathe P, Vremec D, Wu L, Corcoran L, Shortman K. Convergent Differentiation: Myeloid and Lymphoid Pathways to Murine Plasmacytoid Dendritic Cells. Blood (2013) 121(1):11–9. doi: 10.1182/blood-2012-02-413336
100. Shigematsu H, Reizis B, Iwasaki H, Mizuno S, Hu D, Traver D, et al. Plasmacytoid Dendritic Cells Activate Lymphoid-Specific Genetic Programs Irrespective of Their Cellular Origin. Immunity (2004) 21(1):43–53. doi: 10.1016/j.immuni.2004.06.011
101. Rodrigues PF, Tussiwand R. Novel Concepts in Plasmacytoid Dendritic Cell (pDC) Development and Differentiation. Mol Immunol (2020) 126:25–30. doi: 10.1016/j.molimm.2020.07.006
102. Herman JS, Sagar, Grün D. FateID Infers Cell Fate Bias in Multipotent Progenitors From Single-Cell RNA-Seq Data. Nat Methods (2018) 15(5):379–86. doi: 10.1038/nmeth.4662
103. Rodrigues PF, Alberti-Servera L, Eremin A, Grajales-Reyes GE, Ivanek R, Tussiwand R. Distinct Progenitor Lineages Contribute to the Heterogeneity of Plasmacytoid Dendritic Cells. Nat Immunol (2018) 19(7):711–22. doi: 10.1038/s41590-018-0136-9
104. Dress RJ, Dutertre CA, Giladi A, Schlitzer A, Low I, Shadan NB, et al. Plasmacytoid Dendritic Cells Develop From Ly6D+ Lymphoid Progenitors Distinct From the Myeloid Lineage. Nat Immunol (2019) 20(7):852–64. doi: 10.1038/s41590-019-0420-3
105. Yáñez A, Coetzee SG, Olsson A, Muench DE, Berman BP, Hazelett DJ, et al. Granulocyte-Monocyte Progenitors and Monocyte-Dendritic Cell Progenitors Independently Produce Functionally Distinct Monocytes. Immunity (2017) 47(5):890–902.e4. doi: 10.1016/j.immuni.2017.10.021
106. Goudot C, Coillard A, Villani AC, Gueguen P, Cros A, Sarkizova S, et al. Aryl Hydrocarbon Receptor Controls Monocyte Differentiation Into Dendritic Cells Versus Macrophages. Immunity (2017) 47(3):582–96.e6. doi: 10.1016/j.immuni.2017.08.016
107. Mildner A, Schönheit J, Giladi A, David E, Lara-Astiaso D, Lorenzo-Vivas E, et al. Genomic Characterization of Murine Monocytes Reveals C/Ebpβ Transcription Factor Dependence of Ly6C- Cells. Immunity (2017) 46(5):849–62.e7. doi: 10.1016/j.immuni.2017.04.018
108. Tran Janco JM, Lamichhane P, Karyampudi L, Knutson KL. Tumor-Infiltrating Dendritic Cells in Cancer Pathogenesis. J Immunol (2015) 194(7):2985–91. doi: 10.4049/jimmunol.1403134
109. Engblom C, Pfirschke C, Pittet MJ. The Role of Myeloid Cells in Cancer Therapies. Nat Rev Cancer (2016) 16(7):447–62. doi: 10.1038/nrc.2016.54
110. Sánchez-Paulete AR, Cueto FJ, Martínez-López M, Labiano S, Morales-Kastresana A, Rodríguez-Ruiz ME, et al. Cancer Immunotherapy With Immunomodulatory Anti-CD137 and Anti-PD-1 Monoclonal Antibodies Requires BATF3-Dependent Dendritic Cells. Cancer Discovery (2016) 6(1):71–9. doi: 10.1158/2159-8290.CD-15-0510
111. Spranger S, Dai D, Horton B, Gajewski TF. Tumor-Residing Batf3 Dendritic Cells Are Required for Effector T Cell Trafficking and Adoptive T Cell Therapy. Cancer Cell (2017) 31(5):711–23.e4. doi: 10.1016/j.ccell.2017.04.003
112. Laoui D, Keirsse J, Morias Y, Van Overmeire E, Geeraerts X, Elkrim Y, et al. The Tumour Microenvironment Harbours Ontogenically Distinct Dendritic Cell Populations With Opposing Effects on Tumour Immunity. Nat Commun (2016) 7:13720. doi: 10.1038/ncomms13720
113. Veglia F, Gabrilovich DI. Dendritic Cells in Cancer: The Role Revisited. Curr Opin Immunol (2017) 45:43–51. doi: 10.1016/j.coi.2017.01.002
114. Nirschl CJ, Suárez-Fariñas M, Izar B, Prakadan S, Dannenfelser R, Tirosh I, et al. Ifnγ-Dependent Tissue-Immune Homeostasis Is Co-Opted in the Tumor Microenvironment. Cell (2017) 170(1):127–41.e15. doi: 10.1016/j.cell.2017.06.016
115. Elyada E, Bolisetty M, Laise P, Flynn WF, Courtois ET, Burkhart RA, et al. Cross-Species Single-Cell Analysis of Pancreatic Ductal Adenocarcinoma Reveals Antigen-Presenting Cancer-Associated Fibroblasts. Cancer Discovery (2019) 9(8):1102–23. doi: 10.1158/2159-8290.CD-19-0094
116. Liu Y, He S, Wang XL, Peng W, Chen QY, Chi DM, et al. Tumour Heterogeneity and Intercellular Networks of Nasopharyngeal Carcinoma at Single Cell Resolution. Nat Commun (2021) 12(1):741. doi: 10.1038/s41467-021-21043-4
117. Binnewies M, Mujal AM, Pollack JL, Combes AJ, Hardison EA, Barry KC, et al. Unleashing Type-2 Dendritic Cells to Drive Protective Antitumor CD4+ T Cell Immunity. Cell (2019) 177(3):556–71.e16. doi: 10.1016/j.cell.2019.02.005
118. Bernard V, Semaan A, Huang J, San Lucas FA, Mulu FC, Stephens BM, et al. Single-Cell Transcriptomics of Pancreatic Cancer Precursors Demonstrates Epithelial and Microenvironmental Heterogeneity as an Early Event in Neoplastic Progression. Clin Cancer Res (2019) 25(7):2194–205. doi: 10.1158/1078-0432.CCR-18-1955
119. Zilionis R, Engblom C, Pfirschke C, Savova V, Zemmour D, Saatcioglu HD, et al. Single-Cell Transcriptomics of Human and Mouse Lung Cancers Reveals Conserved Myeloid Populations Across Individuals and Species. Immunity (2019) 50(5):1317–34.e10. doi: 10.1016/j.immuni.2019.03.009
120. Zhang L, Li Z, Skrzypczynska KM, Fang Q, Zhang W, O’Brien SA, et al. Single-Cell Analyses Inform Mechanisms of Myeloid-Targeted Therapies in Colon Cancer. Cell (2020) 181(2):442–59.e29. doi: 10.1016/j.cell.2020.03.048
121. Broz ML, Binnewies M, Boldajipour B, Nelson AE, Pollack JL, Erle DJ, et al. Dissecting the Tumor Myeloid Compartment Reveals Rare Activating Antigen-Presenting Cells Critical for T Cell Immunity. Cancer Cell (2014) 26(5):638–52. doi: 10.1016/j.ccell.2014.09.007
122. Salmon H, Idoyaga J, Rahman A, Leboeuf M, Remark R, Jordan S, et al. Expansion and Activation of CD103(+) Dendritic Cell Progenitors at the Tumor Site Enhances Tumor Responses to Therapeutic PD-L1 and BRAF Inhibition. Immunity (2016) 44(4):924–38. doi: 10.1016/j.immuni.2016.03.012
123. Roberts EW, Broz ML, Binnewies M, Headley MB, Nelson AE, Wolf DM, et al. Critical Role for CD103(+)/CD141(+) Dendritic Cells Bearing CCR7 for Tumor Antigen Trafficking and Priming of T Cell Immunity in Melanoma. Cancer Cell (2016) 30(2):324–36. doi: 10.1016/j.ccell.2016.06.003
124. Lavin Y, Kobayashi S, Leader A, Amir ED, Elefant N, Bigenwald C, et al. Innate Immune Landscape in Early Lung Adenocarcinoma by Paired Single-Cell Analyses. Cell (2017) 169(4):750–65.e17. doi: 10.1016/j.cell.2017.04.014
125. Cheng S, Li Z, Gao R, Xing B, Gao Y, Yang Y, et al. A Pan-Cancer Single-Cell Transcriptional Atlas of Tumor Infiltrating Myeloid Cells. Cell (2021) 184(3):792–809.e23. doi: 10.1016/j.cell.2021.01.010
126. Domagala M, Laplagne C, Leveque E, Laurent C, Fournié JJ, Espinosa E, et al. Cancer Cells Resistance Shaping by Tumor Infiltrating Myeloid Cells. Cancers (Basel) (2021) 13(2):165. doi: 10.3390/cancers13020165
127. Anandasabapathy N, Feder R, Mollah S, Tse SW, Longhi MP, Mehandru S, et al. Classical Flt3L-Dependent Dendritic Cells Control Immunity to Protein Vaccine. J Exp Med (2014) 211(9):1875–91. doi: 10.1084/jem.20131397
128. Chen Z, Zhou L, Liu L, Hou Y, Xiong M, Yang Y, et al. Single-Cell RNA Sequencing Highlights the Role of Inflammatory Cancer-Associated Fibroblasts in Bladder Urothelial Carcinoma. Nat Commun (2020) 11(1):5077. doi: 10.1038/s41467-020-18916-5
129. Zhang Q, He Y, Luo N, Patel SJ, Han Y, Gao R, et al. Landscape and Dynamics of Single Immune Cells in Hepatocellular Carcinoma. Cell (2019) 179(4):829–45.e20. doi: 10.1016/j.cell.2019.10.003
130. Sun Y, Wu L, Zhong Y, Zhou K, Hou Y, Wang Z, et al. Single-Cell Landscape of the Ecosystem in Early-Relapse Hepatocellular Carcinoma. Cell (2021) 184(2):404–21.e16. doi: 10.1016/j.cell.2020.11.041
131. Maier B, Leader AM, Chen ST, Tung N, Chang C, LeBerichel J, et al. A Conserved Dendritic-Cell Regulatory Program Limits Antitumour Immunity. Nature (2020) 580(7802):257–62. doi: 10.1038/s41586-020-2134-y
132. Zheng Y, Chen Z, Han Y, Han L, Zou X, Zhou B, et al. Immune Suppressive Landscape in the Human Esophageal Squamous Cell Carcinoma Microenvironment. Nat Commun (2020) 11(1):6268. doi: 10.1038/s41467-020-20019-0
133. Gardner A, de Mingo Pulido Á, Ruffell B. Dendritic Cells and Their Role in Immunotherapy. Front Immunol (2020) 11:924:521. doi: 10.3389/fimmu.2020.00924
134. Wylie B, Macri C, Mintern JD, Waithman J. Dendritic Cells and Cancer: From Biology to Therapeutic Intervention. Cancers (Basel) (2019) 11(4). doi: 10.3390/cancers11040521
135. Bagchi S, Yuan R, Engleman EG. Immune Checkpoint Inhibitors for the Treatment of Cancer: Clinical Impact and Mechanisms of Response and Resistance. Annu Rev Pathol (2021) 16:223–49. doi: 10.1146/annurev-pathol-042020-042741
136. Lelliott EJ, Mangiola S, Ramsbottom KM, Zethoven M, Lim L, Lau P, et al. Combined BRAF, MEK, and CDK4/6 Inhibition Depletes Intratumoral Immune-Potentiating Myeloid Populations in Melanoma. Cancer Immunol Res (2021) 9(2):136–46. doi: 10.1158/2326-6066.CIR-20-0401
137. Garris CS, Arlauckas SP, Kohler RH, Trefny MP, Garren S, Piot C, et al. Successful Anti-PD-1 Cancer Immunotherapy Requires T Cell-Dendritic Cell Crosstalk Involving the Cytokines IFN-γ and IL-12. Immunity (2018) 49(6):1148–61.e7. doi: 10.1016/j.immuni.2018.09.024
138. Schetters S, Rodriguez E, Kruijssen L, Crommentuijn M, Boon L, Van den Bossche J, et al. Monocyte-Derived APCs Are Central to the Response of PD1 Checkpoint Blockade and Provide a Therapeutic Target for Combination Therapy. J Immunother Cancer (2020) 8(2):e000588. doi: 10.1136/jitc-2020-000588
139. Segura E, Amigorena S. Inflammatory Dendritic Cells in Mice and Humans. Trends Immunol (2013) 34(9):440–5. doi: 10.1016/j.it.2013.06.001
140. Janela B, Patel AA, Lau MC, Goh CC, Msallam R, Kong WT, et al. A Subset of Type I Conventional Dendritic Cells Controls Cutaneous Bacterial Infections Through Vegfα-Mediated Recruitment of Neutrophils. Immunity (2019) 50(4):1069–83.e8. doi: 10.1016/j.immuni.2019.03.001
141. Jordão M, Sankowski R, Brendecke SM, Sagar, Locatelli G, Tai YH, et al. Single-Cell Profiling Identifies Myeloid Cell Subsets With Distinct Fates During Neuroinflammation. Science (2019) 363(6425):eaat7554. doi: 10.1126/science.aat7554
142. Martini E, Kunderfranco P, Peano C, Carullo P, Cremonesi M, Schorn T, et al. Single-Cell Sequencing of Mouse Heart Immune Infiltrate in Pressure Overload-Driven Heart Failure Reveals Extent of Immune Activation. Circulation (2019) 140(25):2089–107. doi: 10.1161/CIRCULATIONAHA.119.041694
143. Peyser R, MacDonnell S, Gao Y, Cheng L, Kim Y, Kaplan T, et al. Defining the Activated Fibroblast Population in Lung Fibrosis Using Single-Cell Sequencing. Am J Respir Cell Mol Biol (2019) 61(1):74–85. doi: 10.1165/rcmb.2018-0313OC
144. Zernecke A. Dendritic Cells in Atherosclerosis: Evidence in Mice and Humans. Arterioscler Thromb Vasc Biol (2015) 35(4):763–70. doi: 10.1161/ATVBAHA.114.303566
145. Cochain C, Vafadarnejad E, Arampatzi P, Pelisek J, Winkels H, Ley K, et al. Single-Cell RNA-Seq Reveals the Transcriptional Landscape and Heterogeneity of Aortic Macrophages in Murine Atherosclerosis. Circ Res (2018) 122(12):1661–74. doi: 10.1161/CIRCRESAHA.117.312509
146. Kiselev VY, Andrews TS, Hemberg M. Challenges in Unsupervised Clustering of Single-Cell RNA-Seq Data. Nat Rev Genet (2019) 20(5):273–82. doi: 10.1038/s41576-018-0088-9
147. Xu C, Su Z. Identification of Cell Types From Single-Cell Transcriptomes Using a Novel Clustering Method. Bioinformatics (2015) 31(12):1974–80. doi: 10.1093/bioinformatics/btv088
148. Li WV, Li JJ. An Accurate and Robust Imputation Method Scimpute for Single-Cell RNA-Seq Data. Nat Commun (2018) 9(1):997. doi: 10.1038/s41467-018-03405-7
Keywords: dendritic cells, single-cell RNA sequencing, cellular heterogeneity, the ontogeny of dendritic cells, tumor-infiltrating dendritic cells
Citation: Chen B, Zhu L, Yang S and Su W (2021) Unraveling the Heterogeneity and Ontogeny of Dendritic Cells Using Single-Cell RNA Sequencing. Front. Immunol. 12:711329. doi: 10.3389/fimmu.2021.711329
Received: 18 May 2021; Accepted: 23 August 2021;
Published: 09 September 2021.
Edited by:
Shalin Naik, Walter and Eliza Hall Institute of Medical Research, AustraliaReviewed by:
Janin Chandra, University of Queensland, AustraliaAnne B. Krug, Ludwig Maximilian University of Munich, Germany
Copyright © 2021 Chen, Zhu, Yang and Su. This is an open-access article distributed under the terms of the Creative Commons Attribution License (CC BY). The use, distribution or reproduction in other forums is permitted, provided the original author(s) and the copyright owner(s) are credited and that the original publication in this journal is cited, in accordance with accepted academic practice. No use, distribution or reproduction is permitted which does not comply with these terms.
*Correspondence: Wenru Su, suwr3@mail.sysu.edu.cn
†These authors have contributed equally to this work and share first authorship