- 1Department of Cellular and Molecular Biology, Faculty of Biological Sciences, Kharazmi University, Tehran, Iran
- 2Applied Microbiology Research Center, Systems Biology and Poisonings Institute, Baqiyatallah University of Medical Sciences, Tehran, Iran
- 3Department of Medical Biotechnology, Faculty of Medical Sciences, Tarbiat Modares University, Tehran, Iran
- 4Department of Medical Genetics, Shahid Sadoughi University of Medical Sciences, Yazd, Iran
- 5Immunology Research Center, Tabriz University of Medical Sciences, Tabriz, Iran
- 6Advanced Therapy Medicinal Product (ATMP) Department, Breast Cancer Research Center, Motamed Cancer Institute, Academic Center for Education, Culture and Research (ACECR), Tehran, Iran
- 7Biochemistry Department, Guilan University of Medical Sciences, Rasht, Iran
- 8School of Pharmacy, Shiraz University of Medical Sciences, Shiraz, Iran
- 9Department of Clinical Biochemistry, Faculty of Medical Sciences, Dezful University of Medical Sciences, Dezful, Iran
- 10Department of Biology Sciences, Shahid Rajaee Teacher Training University, Tehran, Iran
- 11Pharmaceutical Sciences Research Center, Shiraz University of Medical Sciences, Shiraz, Iran
Autoimmune diseases (ADs) could occur due to infectious diseases and vaccination programs. Since millions of people are expected to be infected with SARS-CoV-2 and vaccinated against it, autoimmune consequences seem inevitable. Therefore, we have investigated the whole proteome of the SARS-CoV-2 for its ability to trigger ADs. In this regard, the entire proteome of the SARS-CoV-2 was chopped into more than 48000 peptides. The produced peptides were searched against the entire human proteome to find shared peptides with similar experimentally confirmed T-cell and B-cell epitopes. The obtained peptides were checked for their ability to bind to HLA molecules. The possible population coverage was calculated for the most potent peptides. The obtained results indicated that the SARS-CoV-2 and human proteomes share 23 peptides originated from ORF1ab polyprotein, nonstructural protein NS7a, Surface glycoprotein, and Envelope protein of SARS-CoV-2. Among these peptides, 21 peptides had experimentally confirmed equivalent epitopes. Amongst, only nine peptides were predicted to bind to HLAs with known global allele frequency data, and three peptides were able to bind to experimentally confirmed HLAs of equivalent epitopes. Given the HLAs which have already been reported to be associated with ADs, the ESGLKTIL, RYPANSIV, NVAITRAK, and RRARSVAS were determined to be the most harmful peptides of the SARS-CoV-2 proteome. It would be expected that the COVID-19 pandemic and the vaccination against this pathogen could significantly increase the ADs incidences, especially in populations harboring HLA-B*08:01, HLA-A*024:02, HLA-A*11:01 and HLA-B*27:05. The Southeast Asia, East Asia, and Oceania are at higher risk of AD development.
Introduction
In 2019, the severe acute respiratory syndrome coronavirus 2 (SARS-CoV-2) caused a disease called coronavirus disease 2019 (COVID-19). It rapidly turned into a threat to global health and a progressive pandemic disease in many countries worldwide (1, 2). SARS-CoV-2 genome encodes for 14 open reading frames (Orfs). The Orf1a/Orf1ab encodes pp1a and pp1ab polyproteins which are further cleaved by virus-encoded proteases into 16 non-structural proteins (Nsps) (3, 4). The remaining Orfs encode structural proteins such as spike glycoprotein (S), the small envelope glycoprotein (E), the membrane glycoprotein (M), and a nucleocapsid protein (N) (3, 5). SARS-CoV-2 inters into the host cells via the interaction between the S glycoprotein of the virus and the angiotensin-converting enzyme 2 (ACE2) and the type II transmembrane serine protease (TMPRSS2) of the host cells. The S glycoprotein binds to the ACE2 receptor through its receptor-binding domain (RBD), which spans the 331 to 524 residues (4–7).
Autoimmune disease occurs when the body loses its immunological tolerance to its antigens (the failure of self-tolerance). ADs can appear anywhere in the body and three factors that induce and perpetuate autoimmune disease are hampered immune regulation, environmental factors, and genetic predisposition (8–11). Recent data have shown that environmental factors such as infectious agents (including viruses, bacteria, parasites, and fungi), dietary ingredients, and toxic Chemicals contribute more than 70% to loss of self-tolerance and, as result, autoimmunity (12–14). Autoimmune diseases could be induced by viruses (15). There are also some bacteria-induced autoimmune diseases (16). Moreover, immune responses to Candida albicans in peripheral blood lymphocytes and synovial fluids suggested that fungi may also lead to autoimmunity (17). Three major mechanisms of the infectious agents to trigger autoimmune diseases include bystander activation, epitope spreading, and molecular mimicry (12, 18). In the bystander mechanism, infected cells can activate uninfected-cells through intercellular communication (gap junctions), co-receptor expression [natural Killer group 2D (NKG2D), CD122, TLR (toll-like receptor)], and soluble signals (cytokines). Nonspecific activation of B and T cells is called bystander activation and is known by the activation of lymphocytes detached from the BCR/TCR specificity (18). Release of self-antigens during inflammatory or chronic autoimmune responses, can lead to autoimmune reactions against endogenous epitopes in a phenomenon known as epitope spreading (ES) (19). The ES can be induced by changes in protein structure, such as the conversion of arginine to citrulline. This change triggers an immune response to the original protein and its citrullinated form. Moreover, similar responses could be elicited against other citrullinated proteins; this mechanism is a characteristic of rheumatoid arthritis (RA). Pemphigus bullous, Systemic lupus erythematosus (SLE), pemphigoid, multiple sclerosis, and some other autoimmune diseases are all affected by intramolecular and intermolecular B cell epitope spreading. Somatic hyper-mutation, antigen presentation, and endocytic processing are the molecular mechanisms that support epitope spreading. They also enhance the immune response in ADs (20). Molecular mimicry is another mechanism of developing autoimmunity. This mechanism could lead to the activation of cross reactive T and, or B cells. It occurs when infectious agents contain foreign antigens similar in structure and sequence to the human self-antigens (21–23). This mechanism is implicated in the pathogenesis of many autoimmune diseases such as Graves’ disease, MS, spondyloarthropathies, and diabetes mellitus (24–26).
Recently, the association of various diseases with SARS-CoV-2 has been investigated, one of the most well-known of which are ADs. During the studies on ADs, the impact of SARS-CoV-2 in the progression of autoimmunity has been proven. Studies on the correlation of SARS-CoV-2 infection with diseases such as immune thrombocytopenic purpura (ITP), Miller Fisher syndrome (MFS), Kawasaki (KD), RA, Guillain-Barre syndrome (GBS), and SLE suggested that there is a connection between SARS-CoV-2 and autoimmune disorders. Infection with SARS-CoV-2 acts as a turning point for the progression of autoimmune disease. COVID-19 could reduce the threshold of immunological tolerance through molecular mimicry and epitope spreading (27, 28). The study for the feasibility of autoimmune responses against protein targets in SARS-CoV-2 infection demonstrates that different organs could be affected by anti-SARS-CoV-2 immune responses, and cytopathic effects could be directly induced. Moreover, the role of self-reactive antibodies in the infectious process of the viruses should not be overlooked (29). Another correlation study has been conducted on the lupus erythematosus. According to the results of this study, a significant increase in the levels of anti-SARS-CoV-2 antibody is visible in autoantibody-positive patients (50% of 21 ICU China patients & 92% of 11 ICU German patients) (30). A case report on a young woman with recurrent immune‐mediated lymphocytic fulminant myocarditis (FM) has also brought some evidence in to light. The outcome of this study points out that the autoimmune disease can activate or reactivate in patients with an immunogenic background via significant infections such as COVID-19. This means that predisposition to such genetic history can act as a powerful trigger for the immune system to respond beyond normal (31). It has also been revealed that the risk of COVID-19 is higher in the patients suffering from autoimmune diseases (32).
In the present study, we aimed to analyze the whole proteome of the SARS-CoV-2 for potential antigenic regions capable of triggering autoimmune responses. In silico studies have already been widely used to solve biological challenges (33–41). Various in silico tools have been harnessed to analyze the MHC2 binding epitopes of the SARS-CoV-2 proteome and assess their possible involvement in ADs. Moreover, the global HLA susceptibility map of SARS-CoV-2 for ADs was attained.
Methods
Study Flowchart
The study includes the analyses of more than 48000 peptides using various in silico tools to find out the most potent ADs inducing SARS-CoV-2 peptides. The designed procedure includes mutiple steps to gather reliable data about the ability of the SARS-CoV-2 proteome to trigger ADs following the COVID-19 or possible vaccination programs. The following diagram shows the study steps to get a better grasp of the analyses which would be conducted (Figure 1).
Generation of Peptide Library for SARS-Cov-2 Proteome
The sequence for full polyprotein 1ab (ORF1ab), spike (S) protein, envelope (E) protein, nucleocapsid (N) protein, and membrane (M) protein, nonstructural protein NS3, nonstructural protein NS6, nonstructural protein NS7a, nonstructural protein NS7b, and nonstructural protein NS8 were obtained from the National Center of Biotechnology Information (NCBI) (https://www.ncbi.nlm.nih.gov/). The obtained sequences were confirmed with the sequences stored in the UniProtKB (https://www.uniprot.org/). The peptide generator server (https://www.peptide2.com/peptide_generator.php) was employed to dissect the viral proteome into 8mer, 9mer, 10mer, 11mer, and 12mer overlapping peptide libraries, while the overlapping amino acid count was set to be 7, 8, 9, 10, and 11, respectively.
Peptide Similarity Search
The generated peptides for the SARS-CoV-2 proteome were checked against the human proteome to quickly retrieve all occurrences for a given query peptide from the UniProtKB protein sequences. The PIR Peptide Match tool was used to find the peptide matches. Employing the multiple peptide match interface of the PIR (https://research.bioinformatics.udel.edu/peptidematch/batchpeptidematch.jsp), the generated peptide libraries were checked against the human proteome [Homo sapiens [9606] (210556 seq.)] of the UniProtKB release 2020_05 plus isoforms (195,707,930 sequences). The search was set to include the isoforms to encompass all possibilities. In the case of the existing peptide match, the corresponding protein from the Human proteome was searched in UniProtKB. The information about the gene name, cellular functions, and protein-protein interaction network from the String server (https://string-db.org/) were extracted to analyze their possible correlation with autoimmune diseases.
Prediction of Peptide-MHC Class I Binding Affinity
The Immune Epitope Database (IEDB) (http://www.iedb.org/) was searched to find equivalent epitopes similar to SARS-CoV-2 peptides. The search was restricted to epitopes which are experimentally tested for their ability to bind to adaptive immune receptors [T cell receptor (TCR), antibody or B cell receptor (BCR), or major histocompatibility complex (MHC)]. The search was also narrowed to include linear human epitopes with 90% identity to SARS-CoV-2 peptides. All of the SARS-CoV-2 peptides with a matching epitope in human proteome were assessed in IEDB search. The corresponding proteins for experimental IEDB epitopes were analyzed in the UniProt database. Moreover, the experimentally confirmed HLAs for equivalent IEDB epitopes and the involved disease were extracted from the IEDB epitope search results. The SARS-CoV-2 peptides with equivalent experimental IEDB epitopes were evaluated for their MHC class I-peptide binding affinity. These predictions were carried out against 145 different HLA alleles. These alleles were selected due to the availability of their global allele frequency data. The NetCTLpan 1.1 Server (http://www.cbs.dtu.dk/services/NetCTLpan/) was used to predict the CTL epitopes in the sequences of the selected peptides. The NetMHCpan 4.1 server (http://www.cbs.dtu.dk/services/NetMHCpan/) was used to predict the peptide-MHC class I binding using artificial neural networks (ANNs). Octamer peptides and the selected 145 HLA alleles were set to conduct the analyses. Moreover, the exact prediction was made for the selected SARS-CoV-2 peptides against the experimentally confirmed HLAs of equivalent IEDB epitopes.
HLA Structures
The protein data bank (RCSB PDB) (https://www.rcsb.org/) and the PDBflex database (www.pdbflex.org) were used to find the 3D structures of the HLA molecules. The structure of the predicted HLAs for SARS-CoV-2 peptides and the structures of the experimentally determined HLAs for IEDB epitopes were obtained from the RCSB PDB. The structures resolved by the x-ray diffraction method, accompanied by a peptide, and had the highest resolution were selected for further evaluations. The Chimera 1.10.2 software was used to remove the redundant (peptide chains and the unwanted non-protein molecules) chains from the HLA structures. This would prepare them for the following docking analyses.
Peptide Modeling
The structures of: (a) SARS-CoV-2 peptides with existing HLA predictions, and (b) the SARS-CoV-2 peptides predicted to bind to experimentally confirmed HLA molecules of the equivalent IEDB epitopes, were required to confirm their ability to bind to corresponding HLAs. Therefore, peptide modeling software was harnessed to determine the 3D structure of these peptides. The PEPSTRMOD server (http://osddlinux.osdd.net/raghava/pepstrmod/nat_ss.php) and PEP-FOLD 3.5 server (https://mobyle.rpbs.univ-paris-diderot.fr/cgi-bin/portal.py#forms::PEP-FOLD3) were employed to model the peptides. These servers are capable of modeling the peptides with natural amino acids. The quality of the modeled peptides was assessed using the QMEAN server (https://swissmodel.expasy.org/qmean/). The Protein Data Bank File Editor software was used to assign chain IDs for modeled epitopes.
Molecular Docking
Molecular docking analysis was done for the modeled peptides with existing resolved HLA structures. The structures of the HLA molecules and the modeled peptides were used to perform the molecular docking study. The CABS-dock (http://biocomp.chem.uw.edu.pl/CABSdock), HPEPDOCK (http://huanglab.phys.hust.edu.cn/hpepdock/), and HADDOCK (https://wenmr.science.uu.nl/haddock2.4/) servers were used to perform the analyses. Both chains of the HLA molecule and the modeled peptides were set as the input molecules. Chimera software was used for visual inspection of the docked complexes to ensure their correct interaction orientation. FireDock (http://bioinfo3d.cs.tau.ac.il/FireDock/) refinement server utilizes a coarse refinement method to optimize the interaction in molecular docking studies. The software was used to refine the docked complexes. The results of the FireDock software were fed to rigid-body orientation and side-chain conformations optimization by the RosettaDock server (http://rosettadock.graylab.jhu.edu/).
Binding Energy Calculation
Aside from the correct interaction orientation, the docked protein-peptide complexes should have strong binding energy to keep them together to properly present on the surface of immune cells. The PRODIGY (PROtein binDIng enerGY prediction) server (https://wenmr.science.uu.nl/prodigy/) was invoked to predict binding affinity in docked complexes. All of the docked complexes were subjected to this analysis. Moreover, the originally selected HLA complexes were checked for their binding affinity against their accompanying peptides as a positive control in comparison with the docked complexes.
Data Validation and Disease Association Search
All identical peptides with human proteome were searched in IEDB to find similar experimentally validated epitopes. The corresponding HLA class I of experimental epitopes was evaluated via an integrated approach for the SARS-CoV-2 peptides. A literature review was conducted to invoke experimental studies as supporting evidence for SARS-CoV-2 and autoimmune disease association. The miPepBase database (http://proteininformatics.org/mkumar/mipepbase/index.html) is a database of experimentally verified peptides involved in molecular mimicry, which was used to find any Molecular Mimicry of matching peptides. Cross reactivity between the human “disease-related” epitopes and the matching peptides of the pathogen could trigger autoimmunity in a process, which is called molecular mimicry. On the other hand, the Gene and Autoimmune Disease association Database (GAAD) (http://gaad.medgenius.info/genes/) was employed to describe the possible association between genes and autoimmune disease. The gene IDs of the human proteins, which were obtained from the peptide match search, were used to run the analyses. The search would be carried out among the 4,186 genes which are found to associate with autoimmune diseases. It was important to know if the IEDB peptides were associated with any autoimmune diseases. Therefore, the miPepBase database was used to find epitopes with the ability to trigger Molecular Mimicry for autoimmune diseases. All of the IEDB epitopes found in the previous section were fed as input for these analyses. The possible association between the genes of the IEDB proteins and autoimmune disease were described by GAAD database. The gene IDs of the proteins (corresponding to the IEDB epitopes) were obtained from UniProt database. Moreover, a thorough literature review was also performed to find existing incidence of auto immune diseases following the COVID-19.
Population Coverage
The population coverage analyses were done using the IEDB population coverage tool (http://tools.iedb.org/population/). This tool is used to calculate the fraction of individuals predicted to respond to a given epitope set based on HLA genotypic frequencies and on the basis of MHC binding and, or T cell restriction data. Two runs of population coverage analyses were executed for the obtained HLA and peptide sets. The first run was done for the SARS-CoV-2 peptides with existing HLA predictions. The second run was done for the SARS-CoV-2 peptides predicted to bind to experimentally confirmed HLA molecules of the equivalent IEDB epitopes. Moreover, to analyze the combined population coverage of all peptides and their predicted HLA molecules, another run was performed. The analyses were limited to the class one HLA molecules, and the selected areas and populations were set to include all of the possible areas and populations within the server.
Results
Peptide Library Generation
The genomic RNA sequences of full polyprotein 1ab (ORF1ab), spike (S) protein, envelope (E) protein, nucleocapsid (N) protein, membrane (M) protein, nonstructural protein NS3, nonstructural protein NS6, nonstructural protein NS7a, nonstructural protein NS7b, and nonstructural protein NS8 were found under the protein IDs of QHR63289.1, QHR63290.2, QHR63292.1, QHR63298.1, QHR63293.1, QHR63291.1, QHR63294.1, QHR63295.1, QHR63296.1, and QHR63297.1, respectively. All possible 8- to 12-mer peptides were generated from the SARS-CoV-2 proteome. 48530 peptides were generated and organized in 8mer, 9mer, 10mer, 11mer, and 12mer overlapping peptide libraries. Since the sliding window of peptide generation has one amino acid step size, each amino acid differed by one amino acid from its previous peptide.
Matching Peptides in the Human Proteome
Searching for matching peptides among the human proteome sequences unveiled the existence of 23 SARS-CoV-2 peptides with exact matches within the human protein. All of the matching peptides were from the octamer library, and no other peptide libraries with different lengths had any matching peptides. Some of the peptides were found in more than one human protein. The list of SARS-CoV-2 octamer peptides and some information about the matching human proteins are presented in Table 1. The protein-protein interaction networks of the found proteins are presented in Supplementary Figure 1. Data regarding the tissue specificity of the found human proteins are represented in Supplementary Table 1. The corresponding proteins of Eight out of 23 SARS-CoV-2 peptides had been shown to be expressed within heart tissue.
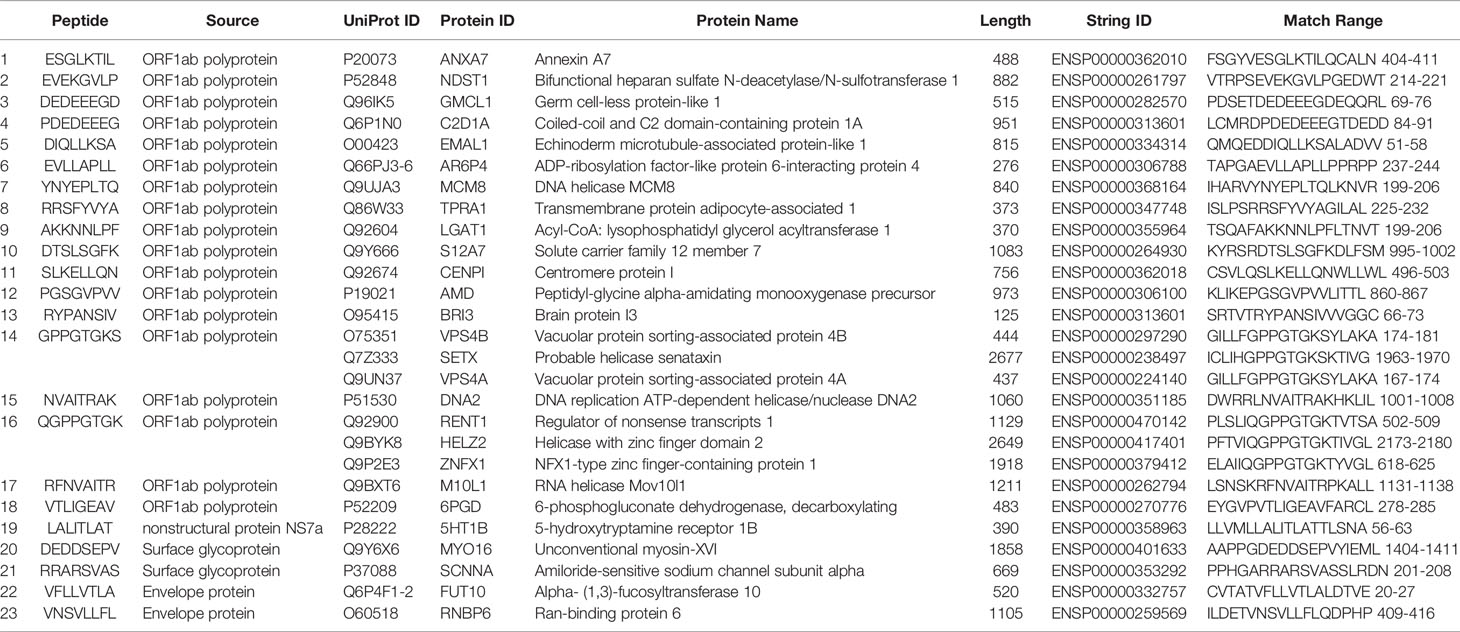
Table 1 The results of peptide (octamers) similarity search between SARS-CoV-2 peptides and human proteome.
MHC-Binding Prediction
The IEBD search indicated that 21 (out of 24 SARS-CoV-2) peptides have experimentally confirmed equivalent epitopes with at least 90% of similarity. There was no exact match between the selected SARS-CoV-2 peptides and the IEBD epitopes. Moreover, there were no predicted posttranslational modifications for SARS-CoV-2 peptides. Since IEDB epitopes are tested for binding to an adaptive immune receptor, the SARS-CoV-2 peptides with over 90% sequence similarity could be expected to show similar immunological outcomes. The corresponding proteins, experimental HLAs, and involved diseases for equivalent IEDB epitopes are presented in Supplementary Table 2. Selected SARS-CoV-2 peptides were also checked against 145 HLAs with known global allele frequency data. The obtained results indicated that some of the selected SARS-CoV-2 peptides are capable of binding to these HLA molecules. It has been predicted that the peptides including the ESGLKTIL (binds to: HLA-B*08:01), EVLLAPLL (binds to: HLA-B*51:07), NVAITRAK (binds to: HLA-A*34:02), RYPANSIV (binds to: HLA-A*24:02, HLA-A*24:03, HLA-A*24:07, HLA-C*14:02, and HLA-C*14:03), RRSFYVYA (binds to: HLA-B*27:02, HLA-B*27:03, HLA-B*27:04, and HLA-B*27:05), and RFNVAITR (binds to: HLA-A*33:03 and HLA-A*74:01) are the only SARS-CoV-2 peptides predicted to bind to some of the examined HLA alleles. The capability to bind to HLA molecule indicates that these epitopes are potentially immunogenic. The results of the similar prediction for selected SARS-CoV-2 peptides against the experimentally confirmed HLAs of equivalent IEDB epitopes indicated that the peptides including the RRSFYVYA (binds to: HLA-B*27:05), NVAITRAK (binds to: HLA-A*11:01), and RFNVAITR (binds to: HLA-A*31:01) could bind to examined HLA molecules.
Preparation of HLA 3D Structures
It is vitally important to gather structural information about the interactions between an epitope and its HLA molecule. The 3D structures of some HLA molecules are already resolved and stored in protein structure databases. The search within the RCSB PDB indicated that four cases of the predicted HLAs for SARS-CoV-2 peptides and 61 cases of experimentally determined HLAs for IEDB epitopes have 3D structures. Some of the HLA molecules had more than one resolved structure. The structure with the highest resolution was selected for further analyses. The structures of the accompanying epitopes were removed from the chosen HLA structures. Supplementary Table 3 listed the RCSB PDB IDs for the HLA molecules, found with a resolved 3D structure. The accompanying peptides of the HLA complexes were removed from the peptide-binding groove for the following docking analyses.
Peptide Modeling
The structures of 4 peptides were modeled by the PEPSTRMOD and FOLD 3.5 servers. All of the peptides were correctly folded. The model quality assessment showed that the modeled peptides are of high structural quality. The modeled peptides were assigned with C or D chain IDs to be distinguished from the HLA chain IDs upon the docking analyses.
Molecular Docking
The employed CABS-dock, HPEPDOCK, and HADDOCK software could dock the HLA structures with the modeled epitopes. All of the resulting complexes were visually inspected for their correct interactions. The obtained results indicated that the peptides were docked within the peptide-binding groove of the HLA molecules. The FireDock and RosettaDock servers optimized the docking orientation and side-chain conformations to have more reliable complexes of HLA and peptide molecules (Supplementary Figure 2). The obtained results demonstrated that the SARS-CoV-2 peptides could interact with the experimentally confirmed HLA molecules.
Binding Energy Calculation
The results of binding energy calculation indicated that all of the modeled peptides can bind to the HLA molecules with an affinity equivalent to the binding affinity of resolves HLA-peptide complexes (Table 2). The predicted HLA (HLA-B*08:01 under the PDB ID of 3X13) showed the highest binding affinity (-13.7 kcal mol-1) against the corresponding SARS-CoV-2 peptide (ESGLKTIL). This binding affinity was even higher than the binding energy of the peptide and HLA molecule within the original 3X13 complex and the binding energy between the similar IEDB peptides and their HLA molecules. This high binding energy could be construed as the high potency of these peptides to invoke a robust immune response.
Disease Association Search
The SARS-CoV-2 octamer peptides with matching peptides in human proteome were analyzed for their association with autoimmune diseases. The results of the search within miPepBase database showed that some of the peptides could trigger the molecular mimicry mechanism and lead to autoimmune diseases. The results of the miPepBase database are presented in Table 3. The possible association between the found human protein matches and the autoimmune disease was analyzed using the GAAD server. The gene names for all of the matching proteins were extracted and used for the analyses. The obtained results revealed that only the SARS-CoV-2 peptide, which matches with the GMCL1 gene name, could be associated with Multiple sclerosis and Rheumatoid arthritis. The rest of the peptides had no associated diseases. The literature review results showed that 21 autoimmune conditions could be triggered following the COVID-19 (Table 4). The literature review results encompasses the results of the miPepBase and GAAD databases.
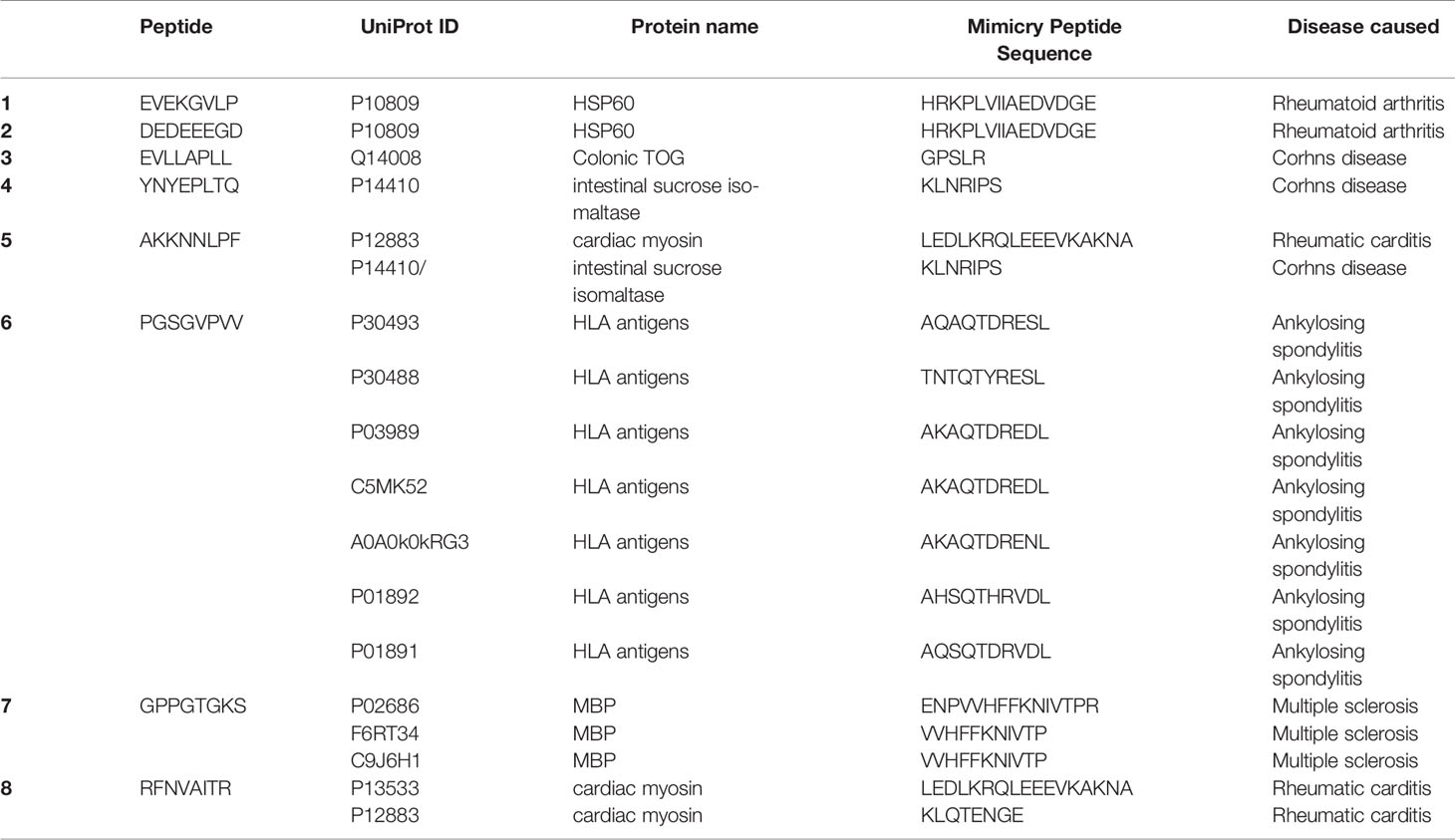
Table 3 The results of the miPepBase. All of the peptides with matching human proteins are analyzed.
Population Coverage Analyses
Performing the population coverage analyses, the possible world coverage of each SARS-CoV-2 peptide for autoimmune responses was evaluated (Table 5). The attained results indicated that regarding the peptides with the highest population coverages, the RYPANSIV (world coverage 25.74%), NVAITRAK (world coverage 15.53%), ESGLKTIL (world coverage 10.55%), RRSFYVYA (world coverage 7.33%), and RFNVAITR (world coverage 6.91%) are the top five peptides. On the other hand, calculation of combined population coverage for all peptides with predicted HLA binding indicates over 57% world coverage (Supplementary Table 4). Among different world regions the Southeast Asia (coverage: 84.12%), East Asia (coverage: 83.78%), and Oceania (coverage: 80.72%) have the highest population coverages. Europe, North America, and South America have over 50 % coverage, while Africa is below 50% coverage. On the contrary, the regions like Lebanon, United Kingdom, and Rwanda have been calculated to have the lowest population coverage for the given peptide and HLA sets (Figure 2).
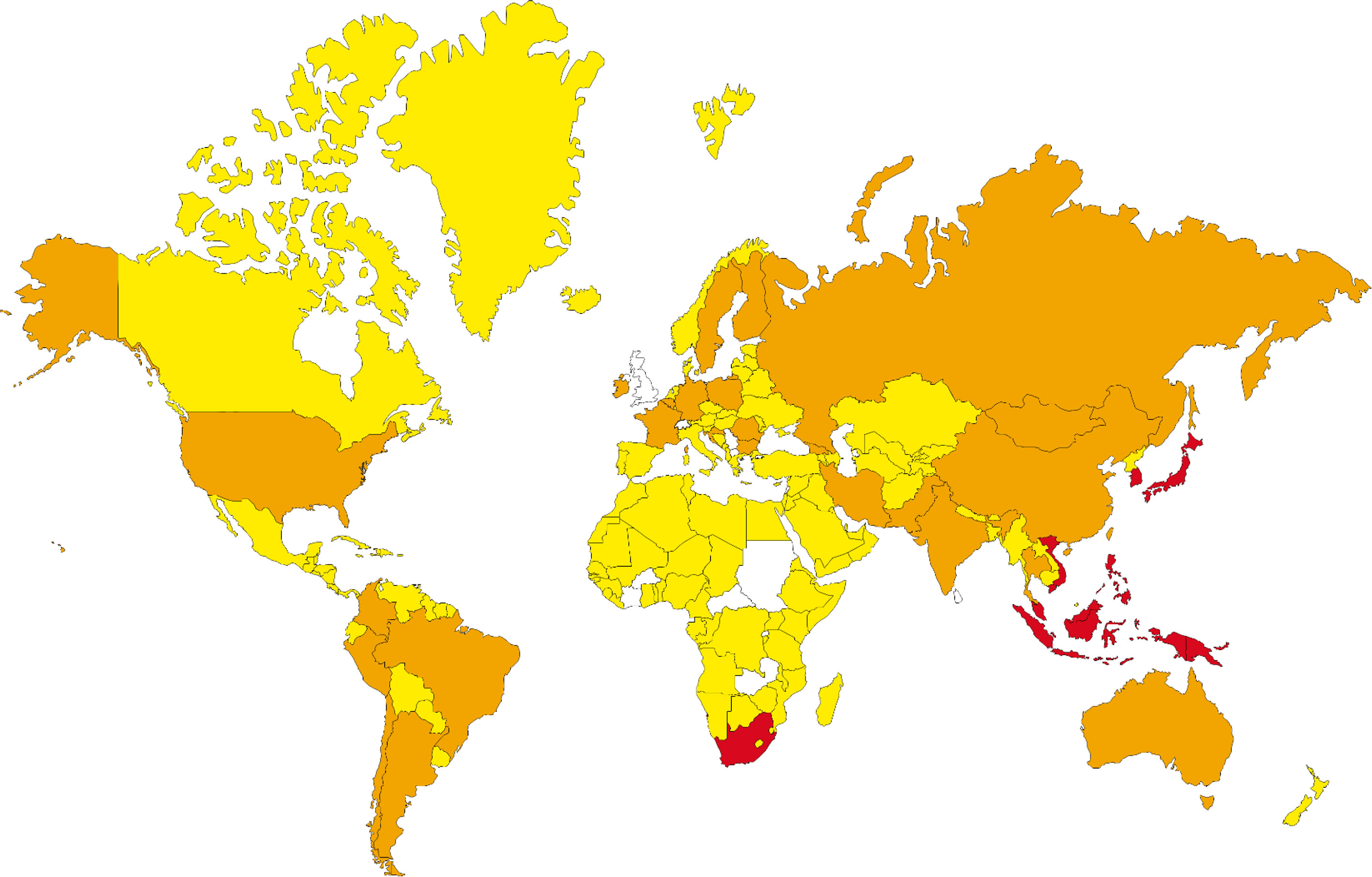
Figure 2 The population coverage prediction for most potent SARS-CoV-2 peptides (red: 100%-75%, orange: 75%-50%, yellow: 50%-25% and regions without data, white: 25%-0% of coverage).
Discussion
Infections could trigger host immune responses against their immunogenic epitopes. Among these responses, some immunological cross-reactions against the epitopes of host proteins could occur (23). Epidemiological studies revealed that infectious diseases could trigger autoimmunity via cross-reactivity. Immunological mechanisms such as molecular mimicry and significant homology between microbial and human antigens could break off the self-tolerance and consequently induce the post-infection ADs. However, these cross-reactions could act as a double-edged sword in autoimmunity (23). Some reports have already suggested that a correlation exists between the COVID-19 and autoimmune diseases (77). Meanwhile, vaccines’ safety regarding their potential to trigger autoimmune responses remains a challenging issue (78). Molecular mimicry between the host antigens and the infectious agent or between the host antigens and the vaccine is a leading mechanism to induce autoimmunity (23, 78).
To find peptide matches within the human proteome, the proteome of SARS-CoV-2 was chopped to peptides with different lengths (8-12mer). Among the various lengths of HLA binders, only the shortest lengths of HLA class I binders (8-meric peptides) matched with peptides in the human proteome. This length of the peptide could also be considered as a B-cell epitope. Therefore, peptides with this length and their related HLAs (HLA class I) were considered for further analyses. Following the 2009 H1N1 pandemic in which tens of millions of vaccine doses against H1N1 have been delivered, reports have revealed an increased incidence of narcolepsy and Guillain-Barre syndrome (GBS) (23). This increase was attributed to epitope mimicry, and the genetic susceptibility of populations that received the vaccines. Kanduc et al. have revealed that two octapeptides of human proteome are shared with HPV16 proteome (79). HPV vaccination remains to be a challenging issue regarding the induction of ADs (23). Since the number of shared octapeptides between the human and SARS-CoV-2 proteome is more than 11-fold compared to the HPV16 and human proteome, the risk of triggering ADs could be significantly higher via SARS-CoV-2 infection and, or vaccination. In this regard, the study by Venkatakrishnan et al. has arrived at some epitopes with possible autoimmune consequences (80). Hence, subunit vaccines lacking these peptides would be safer concerning ADs development.
The SARS-CoV-2 proteome contains four proteins with matching peptides identical to peptides from the human proteome. Amongst, ORF1ab polyprotein encompasses the highest number of these peptides (18 peptides). Thus, this protein would outweigh other SARS-CoV-2 antigens regarding the risk of autoimmune responses. Aside from significant homology between pathogen and human peptides, genetic (e.g., some HLA alleles) and environmental factors (e.g., adjuvant administration) are involved in autoimmunity etiology. Finding positive experimental assays for SARS-CoV-2 epitopes assures the elicitation of the immune system against these peptides. Moreover, positive experimental assays for self-epitopes and HLA-binders, sharing identity with 8-meric peptides (identical to human proteome) increase the risk of cross-reactivity. However, peptides with “perfect fit” and identical homology are not necessarily more pathogenic than peptides with “almost identical” homology (23). Various counterexamples have already been reported for peptides of “almost identical” homology with higher pathogenicity (81, 82). Apparently, identical/similar peptides of infectious agents would be more harmful if they are assigned as binders of HLAs, which have already been determined to be involved in autoimmune diseases. It has been demonstrated that several HLAs are highly associated with ADs (e.g., A*24, A*68, B*08, B*15, B*27, B*42, B*51 and DRB1) (81, 83–86). Various mechanisms such as alternate docking, altered peptide-binding register, and hotspot molecular mimicry had been described for autoreactive T cell activation via HLAs-mediated peptide presentation (81). Therefore, the most deleterious epitopes share sequence identity with experimentally validated self-epitopes and bind to HLAs involved in autoimmune diseases. All of the SARS-CoV-2, predicted to be HLA binders, could potentially bind to HLAs involved in autoimmune diseases, among which five peptides were derived from the ORF1ab polyprotein.
The ESGLKTIL peptide was found within a 9-meric epitope of Annexin 7. Based on experimental assays, this epitope could bind to HLA-B*40:01 and HLA-B*40:02. Moreover, ESGLKTIL was also found within a 9-meric epitope of SARS-CoV-2, which is known as an HLA-B*40:01 binder. Although HLA-B*40:01 and HLA-B*40:02 are protective for autoimmune hepatitis (87), these alleles are associated with ankylosing spondylitis (AS) (88, 89). Ankylosing spondylitis is associated with SARS-CoV-2 (76).Besides, positive experimental assays revealed that ESGLKTIL shares sequence identity (more than 90%) with 11-meric and 16-meric peptides from phosphoglycolate phosphatase. These two peptides are reported to respectively bind to HLA-B*57:01 and HLA-F*01:03. It has been demonstrated that HLA-B*57:01 could be susceptible to drug-induced autoimmunity. Abacavir is a carbocyclic 2′-deoxyguanosine nucleoside analog which is suggested to be involved in loading novel self-peptides onto HLA-B*57:01 (90). A non-classical HLA, the HLA-F*01:03, could be associated with ankylosing spondylitis (91). The other autoimmune-related HLAs predicted to bind the SARS-CoV-2 are the HLA-B*08:01, HLA-A*24, and HLA-B*51. The HLA-B*08:01 is involved in myasthenia gravis, while the HLA-A*24 and HLA-B*51 are associated with Behçet disease (BD). Therefore, populations harboring these alleles are highly at risk of ADs following SARS-CoV-2 infection and/or vaccination.
The RYPANSIV peptide could bind to the HLA-A*024:02. This allele is associated with type 1 diabetes (92), myasthenia gravis (93), Sjogren’s syndrome (94), and systemic lupus erythematosus (95). Evidences are available about association of SARS-CoV-2 and systemic lupus erythematosus (63, 64). Moreover, this peptide shares identity with human peptides known as binders of autoimmune-associated HLAs. NVAITRAK is a binder of HLA-A*11:01, which is associated with autoimmune and inflammatory diseases such as type 1 diabetes (92), diffuse panbronchiolitis (96), and otosclerosis (97). Various studies revealed that SARS-CoV-2 is associated with type 1 diabetes (55–57). The RRARSVAS peptide is a binder for HLA-B*27:05. This allele is a well-known HLA associated with ankylosing spondylitis (98).
The RRARSVAS peptide is found in a neutralizing B-cell epitope of SARS-CoV-2 surface glycoprotein (99). Moreover, RRARSVAS is located near to motif responsible for super-antigenicity property of the spike protein. This epitope is located close to the S1/S2 cleavage site of the spike protein and resembles the super-antigen motif from Staphylococcal enterotoxin B (SEB). This sequence could be the possible explanation for cytokine storm in severe COVID-19 patients and multisystem-inflammatory syndrome (MIS-C) observed in children (100, 101). This sequence is also found in T-cell epitopes of SARS-CoV-2 spike protein. These T-cell epitopes are known to bind to various HLAs, including those associated with ADs (HLA-B*08:01 and HLA-B*07:02) (102–104). Moreover, the RARSVA sequence is found in an 11-meric epitope derived from a human antigen. This 11-meric epitope is known to bind to HLA-A*66:01 allele. The HLA-B*08:01 is associated with myasthenia gravis (105), and the HLA-B*07:02 is associated with neurological ADs (85). Reports are available about myasthenia gravis associated with SARS-CoV-2 infection (68, 106–108). Surface glycoprotein is the leading antigen of interest for vaccine development against SARS-CoV-2 (109). DEDDSEPV peptide is conserved in B-cell epitopes of SARS-CoV-2 and SARS-CoV-1 surface glycoproteins (110–113). Moreover, this peptide has recently been found in a 10-meric T-cell epitope of SARS-CoV-2 spike protein. This epitope is reported to bind to HLA-B*40:01 allele (102). The DDSEPV sequence has also been found in an epitope derived from a human antigen. This epitope is known to bind to various HLAs, including those associated with ADs (e.g., HLA-B*27:05 and HLA-C*08:01) (114, 115). Hence, vaccine candidates harboring either of these two peptides (DEDDSEPV and RRARSVAS) could be harmful considering the ADs development. The RRARSVAS peptide could be assigned as the most harmful peptide, since it is found in a neutralizing B-cell epitope of SARS-CoV-2. Therefore, this epitope could be of interest in vaccine design and formulation.
The EVEKGVLP peptide shares > 66% identity with GLVEVEKGV, which is a 9-meric epitope of SARS-CoV-2. This epitope is known to bind to the HLA-A*02:01 allele. This HLA allele is associated with vitiligo autoimmune disease (116). The DIQLLK sequence of the DIQLLKSA peptide (from SARS-CoV-2) was found in one SARS-CoV-1 (GEDIQLLKA) and two human (KRDIQLLK and DIQLLKRTV) HLA binders. The GEDIQLLKA peptide could bind to HLA-B*44:03, which is associated with vitiligo (117). Another study has also shown its association with autoimmune encephalitis (AE) as a neurological autoimmune disease (118). Interestingly, HLA-B*44 has a permissive role in SARS-CoV-2 infection (119). Association of autoimmune encephalitis with COVID-19 was also reported (69). The HLA-B*18:01 allele is associated with an inflammatory thyroid disease known as subacute thyroiditis (SAT) (120). Recently, it has been demonstrated that SARS-CoV-2 could cause thyroid dysfunction (50, 51). As mentioned above, HLA-B*40:02 is associated with ankylosing spondylitis. The DIQLLKRTV peptide is an HLA-A*33:01 binder which is associated with vitiligo (117) and drug-induced liver injury (DILI) (121). Although no positive assays have confirmed the EVLLAPLL peptide as an epitope or HLA binder from SARS-CoV-2, LLAPLL sequence was found in human epitopes, which acts as HLA binders associated with ADs.
Data validation study showed that our predicted autoimmune concerns already have conforming clinical records. These data instances are mostly accumulated since 2020. More than 21 autoimmune conditions are reported to be followed by COVID-19. Although these are preliminary studies of possible role of COVID-19 in triggering or exacerbating the autoimmune conditions, this number shows the perturbing nature of possible consequences. There is even a compatible tissue specificity profile between the predicted epitopes and the resulting autoimmune condition. For instance, myocardial involvement is characteristic for children with MIS-C associated with previous SARS-CoV-2 infection (101). The Annexin 7 is reported to be expressed within the heart tissue. The presence of the ESGLKTIL peptide within this antigen could be related to this post COVID-19 observation. The Amiloride-sensitive sodium channel subunit alpha is also reported to be expressed within the heart tissue. The presence of the RRARSVAS peptide within this antigen could also be related to myocardial involvement in children with MIS-C. Future studies about the exact mechanism of autoimmunity following COVID-19 would shed light on the roles played by mimicking peptides. We believe that getting a better grasp of autoimmune consequences of these mimicking peptides could be drastically important when the threat is global. This is more prominent when the whole population of the world is the target for vaccination programs and the disease would most likely become a seasonal infection for the next decades.
In conclusion, it would be expected that pandemic COVID-19 and the vaccination against this pathogen could significantly increase ADs, particularly those associated with HLA-B*08:01, HLA-A*024:02, HLA-A*11:01, and HLA-B*27:05. Populations harboring these alleles are highly at risk for the associated ADs. ESGLKTIL, RYPANSIV, NVAITRAK, and RRARSVAS are the most harmful peptides of the SARS-CoV-2 proteome. These peptides are binders for HLA-B*08:01, HLA-A*024:02, HLA-A*11:01, and HLA-B*27:05, respectively which could cover a high percentage of different populations throughout the world. Given these peptides and corresponding HLAs, the populations of Southeast Asia, East Asia, and Oceania are predicted to be at higher risk of AD development following the SARS-CoV-2 infection or vaccination.
Data Availability Statement
The original contributions presented in the study are included in the article/Supplementary Material. Further inquiries can be directed to the corresponding author.
Author Contributions
All authors designed the analysis, carried out the experiment, and collected the data. All authors contributed to the article and approved the submitted version.
Funding
This work was supported by Shahid Rajaee Teacher Training University under contract number 23273.
Conflict of Interest
The authors declare that the research was conducted in the absence of any commercial or financial relationships that could be construed as a potential conflict of interest.
Publisher’s Note
All claims expressed in this article are solely those of the authors and do not necessarily represent those of their affiliated organizations, or those of the publisher, the editors and the reviewers. Any product that may be evaluated in this article, or claim that may be made by its manufacturer, is not guaranteed or endorsed by the publisher.
Supplementary Material
The Supplementary Material for this article can be found online at: https://www.frontiersin.org/articles/10.3389/fimmu.2021.705772/full#supplementary-material
References
1. Rodriguez-Morales AJ, Bonilla-Aldana DK, Balbin-Ramon GJ, Rabaan AA, Sah R, Paniz-Mondolfi A, et al. History Is Repeating Itself: Probable Zoonotic Spillover as the Cause of the 2019 Novel Coronavirus Epidemic. Infez Med (2020) 28(1):3–5.
2. Dos Santos WG. Natural History of COVID-19 and Current Knowledge on Treatment Therapeutic Options. Biomed Pharmacother (2020) 129:110493. doi: 10.1016/j.biopha.2020.110493
3. Gordon DE, Jang GM, Bouhaddou M, Xu J, Obernier K, White KM, et al. A SARS-CoV-2 Protein Interaction Map Reveals Targets for Drug Repurposing. Nature (2020) 583:1–13. doi: 10.1038/s41586-020-2286-9
4. Kumar S, Nyodu R, Maurya VK, Saxena SK. Morphology, Genome Organization, Replication, and Pathogenesis of Severe Acute Respiratory Syndrome Coronavirus 2 (SARS-CoV-2). Coronavirus Dis 2019 (COVID-19): Springer (2020), 23–31. doi: 10.1007/978-981-15-4814-7_3
5. Astuti I. Severe Acute Respiratory Syndrome Coronavirus 2 (SARS-CoV-2): An Overview of Viral Structure and Host Response. Diabetes Metab Syndr: Clin Res Rev (2020) 14:407–12. doi: 10.1016/j.dsx.2020.04.020
6. Romano M, Ruggiero A, Squeglia F, Maga G, Berisio R. A Structural View of SARS-CoV-2 RNA Replication Machinery: RNA Synthesis, Proofreading and Final Capping. Cells (2020) 9(5):1267. doi: 10.3390/cells9051267
7. Shang J, Wan Y, Luo C, Ye G, Geng Q, Auerbach A, et al. Cell Entry Mechanisms of SARS-CoV-2. Proc Natl Acad Sci (2020) 117(21):11727–34. doi: 10.1073/pnas.2003138117
8. Ermann J, Fathman CG. Autoimmune Diseases: Genes, Bugs and Failed Regulation. Nat Immunol (2001) 2(9):759–61. doi: 10.1038/ni0901-759
9. Cortés JR, Sánchez-Díaz R, Bovolenta ER, Barreiro O, Lasarte S, Matesanz-Marín A, et al. Maintenance of Immune Tolerance by Foxp3+ Regulatory T Cells Requires CD69 Expression. J Autoimmun (2014) 55:51–62. doi: 10.1016/j.jaut.2014.05.007
10. Berrih-Aknin S. Myasthenia Gravis: Paradox Versus Paradigm in Autoimmunity. J Autoimmun (2014) 52:1–28. doi: 10.1016/j.jaut.2014.05.001
11. Bao Y, Cao X. The Immune Potential and Immunopathology of Cytokine-Producing B Cell Subsets: A Comprehensive Review. J Autoimmun (2014) 55:10–23. doi: 10.1016/j.jaut.2014.04.001
12. Floreani A, Leung PS, Gershwin ME. Environmental Basis of Autoimmunity. Clin Rev Allergy Immunol (2016) 50(3):287–300. doi: 10.1007/s12016-015-8493-8
13. Pollard KM. Environment, Autoantibodies, and Autoimmunity. Front Immunol (2015) 6:60. doi: 10.3389/fimmu.2015.00060
14. Kivity S, Arango MT, Ehrenfeld M, Tehori O, Shoenfeld Y, Anaya J-M, et al. Infection and Autoimmunity in Sjogren’s Syndrome: A Clinical Study and Comprehensive Review. J Autoimmun (2014) 51:17–22. doi: 10.1016/j.jaut.2014.02.008
15. Smatti MK, Cyprian FS, Nasrallah GK, Al Thani AA, Almishal RO, Yassine HM. Viruses and Autoimmunity: A Review on the Potential Interaction and Molecular Mechanisms. Viruses (2019) 11(8):762. doi: 10.3390/v11080762
16. De Luca F, Shoenfeld Y. The Microbiome in Autoimmune Diseases. Clin Exp Immunol (2019) 195(1):74–85. doi: 10.1111/cei.13158
17. Hermann E, Mayet W-J, Klein O, Lohse AW, Trautwein C, Michiels I, et al. Candida Arthritis: Cellular Immune Responses of Synovial Fluid and Peripheral Blood Lymphocytes to Candida Albicans. Ann Rheum Dis (1991) 50(10):697–701. doi: 10.1136/ard.50.10.697
18. Pacheco Y, Acosta-Ampudia Y, Monsalve DM, Chang C, Gershwin ME, Anaya J-M. Bystander Activation and Autoimmunity. J Autoimmun (2019) 103:102301. doi: 10.1016/j.jaut.2019.06.012
19. Vanderlugt CJ, Miller SD. Epitope Spreading. Curr Opin Immunol (1996) 8(6):831. doi: 10.1016/S0952-7915(96)80012-4
20. Cornaby C, Gibbons L, Mayhew V, Sloan CS, Welling A, Poole BD. B Cell Epitope Spreading: Mechanisms and Contribution to Autoimmune Diseases. Immunol Lett (2015) 163(1):56–68. doi: 10.1016/j.imlet.2014.11.001
21. Cusick MF, Libbey JE, Fujinami RS. Molecular Mimicry as a Mechanism of Autoimmune Disease. Clin Rev Allergy Immunol (2012) 42(1):102–11. doi: 10.1007/s12016-011-8294-7
22. Floreani A, Restrepo-Jiménez P, Secchi MF, De Martin S, Leung PS, Krawitt E, et al. Etiopathogenesis of Autoimmune Hepatitis. J Autoimmun (2018) 95:133–43. doi: 10.1016/j.jaut.2018.10.020
23. Rojas M, Restrepo-Jiménez P, Monsalve DM, Pacheco Y, Acosta-Ampudia Y, Ramírez-Santana C, et al. Molecular Mimicry and Autoimmunity. J Autoimmun (2018) 95:100–23. doi: 10.1016/j.jaut.2018.10.012
24. Fujinami RS, Oldstone M. Amino Acid Homology Between the Encephalitogenic Site of Myelin Basic Protein and Virus: Mechanism for Autoimmunity. Science (1985) 230(4729):1043–5. doi: 10.1126/science.2414848
25. Ebringer A, Baines M, Ptaszynska T. Spondyloarthritis, Uveitis, HLA-B27 and Klebsiella. Immunol Rev (1985) 86:101–16. doi: 10.1111/j.1600-065X.1985.tb01140.x
26. Quaratino S, Thorpe CJ, Travers PJ, Londei M. Similar Antigenic Surfaces, Rather Than Sequence Homology, Dictate T-Cell Epitope Molecular Mimicry. Proc Natl Acad Sci (1995) 92(22):10398–402. doi: 10.1073/pnas.92.22.10398
27. Ehrenfeld M, Tincani A, Andreoli L, Cattalini M, Greenbaum A, Kanduc D, et al. Covid-19 and Autoimmunity. Autoimmun Rev (2020) 19:102597. doi: 10.1016/j.autrev.2020.102597
28. Rodríguez Y, Novelli L, Rojas M, De Santis M, Acosta-Ampudia Y, Monsalve DM, et al. Autoinflammatory and Autoimmune Conditions at the Crossroad of COVID-19. J Autoimmun (2020) 114:102506. doi: 10.1016/j.jaut.2020.102506
29. Kanduc D. From Anti-SARS-CoV-2 Immune Responses to COVID-19 Via Molecular Mimicry. Antibodies (2020) 9(3):33. doi: 10.3390/antib9030033
30. Lerma LA, Chaudhary A, Bryan A, Morishima C, Wener MH, Fink SL. Prevalence of Autoantibody Responses in Acute Coronavirus Disease 2019 (COVID-19). J Trans Autoimmun (2020) 3:100073. doi: 10.1016/j.jtauto.2020.100073
31. Caraffa R, Marcolongo R, Bottio T, Rizzo S, Bifulco O, Bagozzi L, et al. Recurrent Autoimmune Myocarditis in a Young Woman During the Coronavirus Disease 2019 Pandemic. ESC Heart Fail (2020) 8:756–60. doi: 10.1002/ehf2.13028
32. Akiyama S, Hamdeh S, Micic D, Sakuraba A. Prevalence and Clinical Outcomes of COVID-19 in Patients With Autoimmune Diseases: A Systematic Review and Meta-Analysis. Ann Rheum Dis (2020) 80:384–91. doi: 10.1136/annrheumdis-2020-219337
33. Khalili S, Rasaee MJ, Mousavi SL, Amani J, Jahangiri A, Borna H. In Silico Prediction and In Vitro Verification of a Novel Multi-Epitope Antigen for HBV Detection. Mol Gen Microbiol Virol (2017) 32(4):230–40. doi: 10.3103/S0891416817040097
34. Mard-Soltani M, Rasaee MJ, Khalili S, Sheikhi A-K, Hedayati M, Ghaderi-Zefrehi H, et al. The Effect of Differentially Designed Fusion Proteins to Elicit Efficient Anti-Human Thyroid Stimulating Hormone Immune Responses. Iran J Allergy Asthma Immunol (2018) 17(2):158–70.
35. Rahbar MR, Zarei M, Jahangiri A, Khalili S, Nezafat N, Negahdaripour M, et al. Trimeric Autotransporter Adhesins in Acinetobacter Baumannii, Coincidental Evolution at Work. Infect Genet Evol (2019) 71:116–27. doi: 10.1016/j.meegid.2019.03.023
36. Bahrami AA, Payandeh Z, Khalili S, Zakeri A, Bandehpour M. Immunoinformatics: In Silico Approaches and Computational Design of a Multi-Epitope, Immunogenic Protein. Int Rev Immunol (2019) 38(6):307–22. doi: 10.1080/08830185.2019.1657426
37. Payandeh Z, Rahbar MR, Jahangiri A, Hashemi ZS, Zakeri A, Jafarisani M, et al. Design of an Engineered ACE2 as a Novel Therapeutics Against COVID-19. J Theor Biol (2020) 505:110425. doi: 10.1016/j.jtbi.2020.110425
38. Khodashenas S, Khalili S, Moghadam MF. A Cell ELISA Based Method for Exosome Detection in Diagnostic and Therapeutic Applications. Biotechnol Lett (2019) 41(4):523–31. doi: 10.1007/s10529-019-02667-5
39. Rahbar MR, Zarei M, Jahangiri A, Khalili S, Nezafat N, Negahdaripour M, et al. Non-Adaptive Evolution of Trimeric Autotransporters in Brucellaceae. Front Microbiol (2020) 11:1–23. doi: 10.3389/fmicb.2020.560667
40. Rahbar MR, Zarei M, Jahangiri A, Khalili S, Nezafat N, Negahdaripour M, et al. Pierce Into the Native Structure of Ata, a Trimeric Autotransporter of Acinetobacter Baumannii ATCC 17978. Int J Pept Res Ther (2019) 26:1–14. doi: 10.1007/s10989-019-09920-5
41. Rezaei T, Khalili S, Baradaran B, Mosafer J, Rezaei S, Mokhtarzadeh A, et al. Recent Advances on HIV DNA Vaccines Development: Stepwise Improvements to Clinical Trials. J Control Release (2019) 316:116–37. doi: 10.1016/j.jconrel.2019.10.045
42. Xiao M, Zhang Y, Zhang S, Qin X, Xia P, Cao W, et al. Antiphospholipid Antibodies in Critically Ill Patients With COVID-19. Arthritis Rheumatol (2020) 72(12):1998–2004. doi: 10.1002/art.41425
43. Bertin D, Brodovitch A, Beziane A, Hug S, Bouamri A, Mege JL, et al. Anticardiolipin IgG Autoantibody Level Is an Independent Risk Factor for COVID-19 Severity. Arthritis Rheumatol (2020) 72(11):1953–5. doi: 10.1002/art.41409
44. Vlachoyiannopoulos PG, Magira E, Alexopoulos H, Jahaj E, Theophilopoulou K, Kotanidou A, et al. Autoantibodies Related to Systemic Autoimmune Rheumatic Diseases in Severely Ill Patients With COVID-19. Ann Rheum Dis (2020) 79(12):1661–3. doi: 10.1136/annrheumdis-2020-218009
45. Toscano G, Palmerini F, Ravaglia S, Ruiz L, Invernizzi P, Cuzzoni MG, et al. Guillain–Barré Syndrome Associated With SARS-CoV-2. N Engl J Med (2020) 382(26):2574–6. doi: 10.1056/NEJMc2009191
46. Arnaud S, Budowski C, Tin SNW, Degos B. Post SARS-CoV-2 Guillain-Barré Syndrome. Clin Neurophysiol (2020) 131(7):1652–4. doi: 10.1016/j.clinph.2020.05.003
47. Rahimi K. Guillain-Barre Syndrome During COVID-19 Pandemic: An Overview of the Reports. Neurol Sci (2020) 41:1–8. doi: 10.1007/s10072-020-04693-y
48. Gutiérrez-Ortiz C, Méndez-Guerrero A, Rodrigo-Rey S, San Pedro-Murillo E, Bermejo-Guerrero L, Gordo-Mañas R, et al. Miller Fisher Syndrome and Polyneuritis Cranialis in COVID-19. Neurology (2020) 95(5):e601–5. doi: 10.1212/WNL.0000000000009619
49. Lantos JE, Strauss S, Lin E. COVID-19–Associated Miller Fisher Syndrome: MRI Findings. Am J Neuroradiol (2020) 41(7):1184–6. doi: 10.3174/ajnr.A6609
50. Lui DTW, Lee CH, Chow WS, Lee ACH, Tam AR, Fong CHY, et al. Thyroid Dysfunction in Relation to Immune Profile, Disease Status, and Outcome in 191 Patients With COVID-19. J Clin Endocrinol Metab (2020) 106(2):e926–35. doi: 10.1210/clinem/dgaa813
51. Muller I, Cannavaro D, Dazzi D, Covelli D, Mantovani G, Muscatello A, et al. SARS-CoV-2-Related Atypical Thyroiditis. Lancet Diabetes Endocrinol (2020) 8(9):739–41. doi: 10.1016/S2213-8587(20)30266-7
52. Mateu-Salat M, Urgell E, Chico A. SARS-COV-2 as a Trigger for Autoimmune Disease: Report of Two Cases of Graves’ Disease After COVID-19. J Endocrinol Invest (2020) 43(10):1527–8. doi: 10.1007/s40618-020-01366-7
53. Kumar G, Pillai S, Norwick P, Bukulmez H. Leucocytoclastic Vasculitis Secondary to COVID-19 Infection in a Young Child. BMJ Case Rep (2021) 14(4):e242192. doi: 10.1136/bcr-2021-242192
54. Akca UK, Kesici S, Ozsurekci Y, Aykan HH, Batu ED, Atalay E, et al. Kawasaki-Like Disease in Children With COVID-19. Rheumatol Int (2020) 40(12):2105–15. doi: 10.1007/s00296-020-04701-6
55. Rubino F, Amiel SA, Zimmet P, Alberti G, Bornstein S, Eckel RH, et al. New-Onset Diabetes in Covid-19. N Engl J Med (2020) 383(8):789–90. doi: 10.1056/NEJMc2018688
56. Unsworth R, Wallace S, Oliver NS, Yeung S, Kshirsagar A, Naidu H, et al. New-Onset Type 1 Diabetes in Children During COVID-19: Multicenter Regional Findings in the U.K. Diabetes Care (2020) 43(11):e170–e1. doi: 10.2337/dc20-1551
57. Marchand L, Pecquet M, Luyton C. Type 1 Diabetes Onset Triggered by COVID-19. Acta Diabetol (2020) 57(10):1265–6. doi: 10.1007/s00592-020-01570-0
58. Hindilerden F, Yonal-Hindilerden I, Akar E, Yesilbag Z, Kart-Yasar K. Severe Autoimmune Hemolytic Anemia in COVID-19 İnfection, Safely Treated With Steroids. Mediterr J Hematol Infect Dis (2020) 12(1):e2020053–e. doi: 10.4084/MJHID.2020.053
59. Lopez C, Kim J, Pandey A, Huang T, DeLoughery TG. Simultaneous Onset of COVID-19 and Autoimmune Haemolytic Anaemia. Br J Haematol (2020) 190(1):31–2. doi: 10.1111/bjh.16786
60. Lazarian G, Quinquenel A, Bellal M, Siavellis J, Jacquy C, Re D, et al. Autoimmune Haemolytic Anaemia Associated With COVID-19 Infection. Br J Haematol (2020) 190(1):29–31. doi: 10.1111/bjh.16794
61. Zulfiqar A-A, Lorenzo-Villalba N, Hassler P, Andrès E. Immune Thrombocytopenic Purpura in a Patient With Covid-19. N Engl J Med (2020) 382(18):e43. doi: 10.1056/NEJMc2010472
62. Bomhof G, Mutsaers PGNJ, Leebeek F, te Boekhorst P, Hofland J, Croles FN, et al. COVID-19-Associated Immune Thrombocytopenia. Br J Haematol (2020) 190:e61–4. doi: 10.1111/bjh.16850
63. Mantovani Cardoso E, Hundal J, Feterman D, Magaldi J. Concomitant New Diagnosis of Systemic Lupus Erythematosus and COVID-19 With Possible Antiphospholipid Syndrome. Just a Coincidence? A Case Report and Review of Intertwining Pathophysiology. Clin Rheumatol (2020) 39(9):2811–5. doi: 10.1007/s10067-020-05310-1
64. Bonometti R, Sacchi MC, Stobbione P, Lauritano EC, Tamiazzo S, Marchegiani A, et al. The First Case of Systemic Lupus Erythematosus (SLE) Triggered by COVID-19 Infection. Eur Rev Med Pharmacol Sci (2020) 24(18):9695–7. doi: 10.26355/eurrev_202009_23060
65. Miglis MG, Prieto T, Shaik R, Muppidi S, Sinn D-I, Jaradeh S. A Case Report of Postural Tachycardia Syndrome After COVID-19. Clin Auton Res (2020) 30(5):449–51. doi: 10.1007/s10286-020-00727-9
66. Talarico R, Stagnaro C, Ferro F, Carli L, Mosca M. Symmetric Peripheral Polyarthritis Developed During SARS-CoV-2 Infection. Lancet Rheumatol (2020) 2(9):e518–9. doi: 10.1016/S2665-9913(20)30216-2
67. Parisi S, Borrelli R, Bianchi S, Fusaro E. Viral Arthritis and COVID-19. Lancet Rheumatol (2020) 2(11):e655–7. doi: 10.1016/S2665-9913(20)30348-9
68. Muhammed L, Baheerathan A, Cao M, Leite MI, Viegas S. MuSK Antibody–Associated Myasthenia Gravis With SARS-CoV-2 Infection: A Case Report. Ann Internal Med (2021) 174(6):872–3. doi: 10.7326/L20-1298
69. Ayatollahi P, Tarazi A, Wennberg R. Possible Autoimmune Encephalitis With Claustrum Sign in Case of Acute SARS-CoV-2 Infection. Can J Neurol Sci (2020) 48(3):430–2. doi: 10.1017/cjn.2020.209
70. Derksen VFAM, Kissel T, Lamers-Karnebeek FBG, van der Bijl AE, Venhuizen AC, Huizinga TWJ, et al. Onset of Rheumatoid Arthritis After COVID-19: Coincidence or Connected? Ann Rheum Dis (2021) annrheumdis-2021-219859:1096–8. doi: 10.1136/annrheumdis-2021-219859
71. Gao Z-W, Wang X, Lin F, Dong K. The Correlation Between SARS-CoV-2 Infection and Rheumatic Disease. Autoimmun Rev (2020) 19(7):102557–. doi: 10.1016/j.autrev.2020.102557
72. Pizzanelli C, Milano C, Canovetti S, Tagliaferri E, Turco F, Verdenelli S, et al. Autoimmune Limbic Encephalitis Related to SARS-CoV-2 Infection: Case-Report and Review of the Literature. Brain Behav Immun Health (2021) 12:100210. doi: 10.1016/j.bbih.2021.100210
73. Palao M, Fernández-Díaz E, Gracia-Gil J, Romero-Sánchez CM, Díaz-Maroto I, Segura T. Multiple Sclerosis Following SARS-CoV-2 Infection. Mult Scler Relat Disord (2020) 45:102377. doi: 10.1016/j.msard.2020.102377
74. Barzegar M, Vaheb S, Mirmosayyeb O, Afshari-Safavi A, Nehzat N, Shaygannejad V. Can Coronavirus Disease 2019 (COVID-19) Trigger Exacerbation of Multiple Sclerosis? A Retrospective Study. Mult Scler Relat Disord (2021) 52:102947. doi: 10.1016/j.msard.2021.102947
75. Taxonera C, Fisac J, Alba C. Can COVID-19 Trigger De Novo Inflammatory Bowel Disease? Gastroenterology (2021) 160(4):1029–30. doi: 10.1053/j.gastro.2020.11.026
76. Rumiantceva DG, Urumova MM, Erdes SF. New Coronavirus Infection COVID-19 as a Trigger for the Development of Symptoms of Ankylosing Spondylitis. Case Report. TA (2021) 93(5):609–12—12. doi: 10.26442/00403660.2021.05.200793
77. Liu M, Gao Y, Zhang Y, Shi S, Chen Y, Tian J. The Association Between Severe or Dead COVID-19 and Autoimmune Diseases: A Systematic Review and Meta-Analysis. J Infect (2020) 81(3):e93–5. doi: 10.1016/j.jinf.2020.05.065
78. Segal Y, Shoenfeld Y. Vaccine-Induced Autoimmunity: The Role of Molecular Mimicry and Immune Crossreaction. Cell Mol Immunol (2018) 15:586–94. doi: 10.1038/cmi.2017.151
79. Kanduc D. Quantifying the Possible Cross-Reactivity Risk of an HPV16 Vaccine. J Exp Ther Oncol (2009) 8(1):65–76.
80. Venkatakrishnan A, Kayal N, Anand P, Badley AD, Church GM, Soundararajan V. Multi-Pronged Human Protein Mimicry by SARS-CoV-2 Reveals Bifurcating Potential for MHC Detection and Immune Evasion. bioRxiv (2020). doi: 10.1101/2020.06.19.161620
81. Dendrou CA, Petersen J, Rossjohn J, Fugger L. HLA Variation and Disease. Nat Rev Immunol (2018) 18(5):325. doi: 10.1038/nri.2017.143
82. Ehser J, Holdener M, Christen S, Bayer M, Pfeilschifter JM, Hintermann E, et al. Molecular Mimicry Rather Than Identity Breaks T-Cell Tolerance in the CYP2D6 Mouse Model for Human Autoimmune Hepatitis. J Autoimmun (2013) 42:39–49. doi: 10.1016/j.jaut.2012.11.001
83. Wysocki T, Olesińska M, Paradowska-Gorycka A. Current Understanding of an Emerging Role of HLA-DRB1 Gene in Rheumatoid Arthritis–From Research to Clinical Practice. Cells (2020) 9(5):1127. doi: 10.3390/cells9051127
84. Chen Y, Li S, Huang R, Zhang Z, Petersen F, Zheng J, et al. Comprehensive Meta-Analysis Reveals an Association of the HLA-DRB1* 1602 Allele With Autoimmune Diseases Mediated Predominantly by Autoantibodies. Autoimmun Rev (2020) 19(6):102532. doi: 10.1016/j.autrev.2020.102532
85. Muñiz-Castrillo S, Vogrig A, Honnorat J. Associations Between HLA and Autoimmune Neurological Diseases With Autoantibodies. Autoimmun Highlights (2020) 11(1):2. doi: 10.1186/s13317-019-0124-6
86. Elfishawi MM, Elgengehy F, Mossallam G, Elfishawi S, Alfishawy M, Gad A, et al. HLA Class I in Egyptian Patients With Behçet’s Disease: New Association With Susceptibility, Protection, Presentation and Severity of Manifestations. Immunol Invest (2019) 48(2):121–9. doi: 10.1080/08820139.2018.1517364
87. Lammert C, McKinnon EJ, Chalasani N, Phillips EJ. Novel HLA Class I Alleles Outside the Extended DR3 Haplotype Are Protective Against Autoimmune Hepatitis. Clin Trans Gastroenterol (2019) 10(6):e00032. doi: 10.14309/ctg.0000000000000032
88. Lorente E, Redondo-Antón J, Martín-Esteban A, Guasp P, Barnea E, Lauzurica P, et al. Substantial Influence of ERAP2 on the HLA-B* 40: 02 Peptidome: Implications for HLA-B* 27-Negative Ankylosing Spondylitis. Mol Cell Proteomics (2019) 18(11):2298–309. doi: 10.1074/mcp.RA119.001710
89. Díaz-Peña R, Vidal-Castiñeira JR, López-Vázquez A, López-Larrea C. HLA-B* 40: 01 Is Associated With Ankylosing Spondylitis in HLA-B27–positive Populations. J Rheumatol (2016) 43(6):1255–6. doi: 10.3899/jrheum.151096
90. Norcross MA, Luo S, Lu L, Boyne MT, Gomarteli M, Rennels AD, et al. Abacavir Induces Loading of Novel Self-Peptides Into HLA-B* 57: 01: An Autoimmune Model for HLA-Associated Drug Hypersensitivity. AIDS (London England) (2012) 26(11):F21. doi: 10.1097/QAD.0b013e328355fe8f
91. Santos MR, Couto AR, Foroni I, Bettencourt BF, Li Z, Meneses R, et al. Non-Classical Human Leucocyte Antigens in Ankylosing Spondylitis: Possible Association With HLA-E and HLA-F. RMD Open (2018) 4(1):e000677. doi: 10.1136/rmdopen-2018-000677
92. Noble JA, Valdes AM, Varney MD, Carlson JA, Moonsamy P, Fear AL, et al. HLA Class I and Genetic Susceptibility to Type 1 Diabetes: Results From the Type 1 Diabetes Genetics Consortium. Diabetes (2010) 59(11):2972–9. doi: 10.2337/db10-0699
93. Machens A, Löliger C, Pichlmeier U, Emskötter T, Busch C, Izbicki J. Correlation of Thymic Pathology With HLA in Myasthenia Gravis. Clin Immunol (1999) 91(3):296–301. doi: 10.1006/clim.1999.4710
94. Loiseau P, Lepage V, Djelal F, Busson M, Tamouza R, Raffoux C, et al. HLA Class I and Class II Are Both Associated With the Genetic Predisposition to Primary Sjögren Syndrome. Hum Immunol (2001) 62(7):725–31. doi: 10.1016/S0198-8859(01)00253-1
95. Adamashvili I, Wolf R, Aultman D, Milford EL, Jaffe S, Hall V, et al. Soluble HLA-I (s-HLA-I) Synthesis in Systemic Lupus Erythematosus. Rheumatol Int (2003) 23(6):294–300. doi: 10.1007/s00296-003-0306-3
96. Keicho N, Ohashi J, Tamiya G, Nakata K, Taguchi Y, Azuma A, et al. Fine Localization of a Major Disease-Susceptibility Locus for Diffuse Panbronchiolitis. Am J Hum Genet (2000) 66(2):501–7. doi: 10.1086/302786
97. Singhal SK, Datta U, Panda NK, Gupta AK. Genetic Correlation in Otosclerosis. Am J Otolaryngol (1999) 20(2):102–5. doi: 10.1016/S0196-0709(99)90019-4
98. Dashti N, Mahmoudi M, Aslani S, Jamshidi A. HLA-B* 27 Subtypes and Their Implications in the Pathogenesis of Ankylosing Spondylitis. Gene (2018) 670:15–21. doi: 10.1016/j.gene.2018.05.092
99. Lu S, Xie X-X, Zhao L, Wang B, Zhu J, Yang T-R, et al. The Immunodominant and Neutralization Linear Epitopes for SARS-CoV-2. Cell Rep (2021) 34(4):108666. doi: 10.1016/j.celrep.2020.108666
100. Cheng MH, Porritt RA, Rivas MN, Krieger JM, Ozdemir AB, Garcia G, et al. A Monoclonal Antibody Against Staphylococcal Enterotoxin B Superantigen Inhibits SARS-CoV-2 Entry In Vitro. Structure (2021). doi: 10.1016/j.str.2021.04.005
101. Esposito S, Principi N. Multisystem Inflammatory Syndrome in Children Related to SARS-CoV-2. Pediatr Drugs (2021) 23(2):119–29. doi: 10.1007/s40272-020-00435-x
102. Tarke A, Sidney J, Kidd CK, Dan JM, Ramirez SI, Yu ED, et al. Comprehensive Analysis of T Cell Immunodominance and Immunoprevalence of SARS-CoV-2 Epitopes in COVID-19 Cases. Cell Rep Med (2021) 2(2):100204. doi: 10.1016/j.xcrm.2021.100204
103. Schulien I, Kemming J, Oberhardt V, Wild K, Seidel LM, Killmer S, et al. Characterization of Pre-Existing and Induced SARS-CoV-2-Specific CD8+ T Cells. Nat Med (2021) 27(1):78–85. doi: 10.1038/s41591-020-01143-2
104. Saini SK, Hersby DS, Tamhane T, Povlsen HR, Hernandez SPA, Nielsen M, et al. SARS-CoV-2 Genome-Wide Mapping of CD8 T Cell Recognition Reveals Strong Immunodominance and Substantial CD8 T Cell Activation in COVID-19 Patients. bioRxiv (2020). doi: 10.1101/2020.10.19.344911
105. Varade J, Wang N, Lim CK, Zhang T, Zhang Y, Liu X, et al. Novel Genetic Loci Associated HLA-B* 08: 01 Positive Myasthenia Gravis. J Autoimmun (2018) 88:43–9. doi: 10.1016/j.jaut.2017.10.002
106. Restivo DA, Centonze D, Alesina A, Marchese-Ragona R. Myasthenia Gravis Associated With SARS-CoV-2 Infection. Ann Internal Med (2020) 173(12):1027–8. doi: 10.7326/L20-0845
107. Assini A, Gandoglia I, Damato V, Rikani K, Evoli A, Del Sette M. Myasthenia Gravis Associated With Anti-MuSK Antibodies Developed After SARS-CoV-2 Infection. Eur J Neurol (2021). doi: 10.1111/ene.14721
108. Huber M, Rogozinski S, Puppe W, Framme C, Höglinger G, Hufendiek K, et al. Postinfectious Onset of Myasthenia Gravis in a COVID-19 Patient. Front Neurol (2020) 11:576153. doi: 10.3389/fneur.2020.576153
109. Kaur SP, Gupta V. COVID-19 Vaccine: A Comprehensive Status Report. Virus Res (2020) 288:198114. doi: 10.1016/j.virusres.2020.198114
110. Yi Z, Ling Y, Zhang X, Chen J, Hu K, Wang Y, et al. Functional Mapping of B-Cell Linear Epitopes of SARS-CoV-2 in COVID-19 Convalescent Population. Emerg Microbes Infect (2020) 9(1):1988–96. doi: 10.1080/22221751.2020.1815591
111. Shrock E, Fujimura E, Kula T, Timms RT, Lee I-H, Leng Y, et al. Viral Epitope Profiling of COVID-19 Patients Reveals Cross-Reactivity and Correlates of Severity. Science (2020) 370(6520):eabd4250. doi: 10.1126/science.abd4250
112. Chow SC, Ho CY, Tam TT, Wu C, Cheung T, Chan PK, et al. Specific Epitopes of the Structural and Hypothetical Proteins Elicit Variable Humoral Responses in SARS Patients. J Clin Pathol (2006) 59(5):468–76. doi: 10.1136/jcp.2005.029868
113. Chan WS, Wu C, Chow SC, Cheung T, To K-F, Leung W-K, et al. Coronaviral Hypothetical and Structural Proteins Were Found in the Intestinal Surface Enterocytes and Pneumocytes of Severe Acute Respiratory Syndrome (SARS). Mod Pathol (2005) 18(11):1432–9. doi: 10.1038/modpathol.3800439
114. Sarkizova S, Klaeger S, Le PM, Li LW, Oliveira G, Keshishian H, et al. A Large Peptidome Dataset Improves HLA Class I Epitope Prediction Across Most of the Human Population. Nat Biotechnol (2020) 38(2):199–209. doi: 10.1038/s41587-019-0322-9
115. Marcilla M, Alvarez IA, Ramos-Fernández A, Lombardía M, Paradela A, Albar JP. Comparative Analysis of the Endogenous Peptidomes Displayed by HLA-B* 27 and Mamu-B* 08: Two MHC Class I Alleles Associated With Elite Control of HIV/SIV Infection. J Proteome Res (2016) 15(3):1059–69. doi: 10.1021/acs.jproteome.5b01146
116. Hayashi M, Jin Y, Yorgov D, Santorico SA, Hagman J, Ferrara TM, et al. Autoimmune Vitiligo Is Associated With Gain-of-Function by a Transcriptional Regulator That Elevates Expression of HLA-A* 02: 01 In Vivo. Proc Natl Acad Sci (2016) 113(5):1357–62. doi: 10.1073/pnas.1525001113
117. Singh A, Sharma P, Kar HK, Sharma VK, Tembhre MK, Gupta S, et al. HLA Alleles and Amino-Acid Signatures of the Peptide-Binding Pockets of HLA Molecules in Vitiligo. J Invest Dermatol (2012) 132(1):124–34. doi: 10.1038/jid.2011.240
118. Kim TJ, Lee ST, Moon J, Sunwoo JS, Byun JI, Lim JA, et al. Anti-LGI1 Encephalitis Is Associated With Unique HLA Subtypes. Ann Neurol (2017) 81(2):183–92. doi: 10.1002/ana.24860
119. Correale P, Mutti L, Pentimalli F, Baglio G, Saladino RE, Sileri P, et al. HLA-B* 44 and C* 01 Prevalence Correlates With Covid19 Spreading Across Italy. Int J Mol Sci (2020) 21(15):5205. doi: 10.3390/ijms21155205
120. Stasiak M, Tymoniuk B, Michalak R, Stasiak B, Kowalski ML, Lewiński A. Subacute Thyroiditis Is Associated With HLA-B* 18: 01,-DRB1* 01 and-C* 04: 01—the Significance of the New Molecular Background. J Clin Med (2020) 9(2):534. doi: 10.3390/jcm9020534
121. Nicoletti P, Aithal GP, Bjornsson ES, Andrade RJ, Sawle A, Arrese M, et al. Association of Liver Injury From Specific Drugs, or Groups of Drugs, With Polymorphisms in HLA and Other Genes in a Genome-Wide Association Study. Gastroenterology (2017) 152(5):1078–89. doi: 10.1053/j.gastro.2016.12.016
Keywords: autoimmune disease, SARS-CoV-2, vaccination, peptide, HLA, population coverage
Citation: Karami Fath M, Jahangiri A, Ganji M, Sefid F, Payandeh Z, Hashemi ZS, Pourzardosht N, Hessami A, Mard-Soltani M, Zakeri A, Rahbar MR and Khalili S (2021) SARS-CoV-2 Proteome Harbors Peptides Which Are Able to Trigger Autoimmunity Responses: Implications for Infection, Vaccination, and Population Coverage. Front. Immunol. 12:705772. doi: 10.3389/fimmu.2021.705772
Received: 06 May 2021; Accepted: 23 July 2021;
Published: 10 August 2021.
Edited by:
Attila Mócsai, Semmelweis University, HungaryReviewed by:
Tadej Avcin, University Medical Centre Ljubljana, SloveniaBrian Abel, Aegis Life Inc., United States
Sean McIlwain, University of Wisconsin-Madison, United States
Copyright © 2021 Karami Fath, Jahangiri, Ganji, Sefid, Payandeh, Hashemi, Pourzardosht, Hessami, Mard-Soltani, Zakeri, Rahbar and Khalili. This is an open-access article distributed under the terms of the Creative Commons Attribution License (CC BY). The use, distribution or reproduction in other forums is permitted, provided the original author(s) and the copyright owner(s) are credited and that the original publication in this journal is cited, in accordance with accepted academic practice. No use, distribution or reproduction is permitted which does not comply with these terms.
*Correspondence: Saeed Khalili, c2FlZWQua2hhbGlsaUBzcnUuYWMuaXI=
†These authors share first authorship