- 1Department of Surgery, Hospital Clínico Universitario Virgen de la Arrixaca, Murcia, Spain
- 2Digestive and Endocrine Surgery and Transplantation of Abdominal Organs, Biomedical Research Institute of Murcia (IMIB-Arrixaca), Murcia, Spain
- 3Biomedical Informatic and Bioinformatic Platform, Biomedical Research Institute of Murcia (IMIB-Arrixaca), Murcia, Spain
- 4School of Nursing, Universidad de Murcia, Murcia, Spain
- 5Department of Gastroenterology, Unit of Hepatology, Hospital Clínico Universitario Virgen de la Arrixaca, Murcia, Spain
Background: Although proteomics has been employed in the study of several models of liver injury, proteomic methods have only recently been applied not only to biomarker discovery and validation but also to improve understanding of the molecular mechanisms involved in transplantation.
Methods: The study was conducted following the Preferred Reporting Items for Systematic Reviews and Meta-Analyses (PRISMA) methodology and the guidelines for performing systematic literature reviews in bioinformatics (BiSLR). The PubMed, ScienceDirect, and Scopus databases were searched for publications through April 2020. Proteomics studies designed to understand liver transplant outcomes, including ischemia-reperfusion injury (IRI), rejection, or operational tolerance in human or rat samples that applied methodologies for differential expression analysis were considered.
Results: The analysis included 22 studies after application of the inclusion and exclusion criteria. Among the 497 proteins annotated, 68 were shared between species and 10 were shared between sample sources. Among the types of studies analyzed, IRI and rejection shared a higher number of proteins. The most enriched pathway for liver biopsy samples, IRI, and rejection was metabolism, compared to cytokine-cytokine receptor interactions for tolerance.
Conclusions: Proteomics is a promising technique to detect large numbers of proteins. However, our study shows that several technical issues such as the identification of proteoforms or the dynamic range of protein concentration in clinical samples hinder the successful identification of biomarkers in liver transplantation. In addition, there is a need to minimize the experimental variability between studies, increase the sample size and remove high-abundance plasma proteins.
Introduction
Liver transplantation (LT) for the treatment of end-stage liver diseases remains debatable regarding graft viability, such as acute rejection, ischemia-reperfusion injury (IRI), or primary graft dysfunction. To predict and prevent dysfunction and graft rejection, researchers have tried to identify biomarkers that can predict which grafts are more likely to suffer these complications (1). Many studies have focused on DNA polymorphisms, biopsy RNA expression or donor circulant-free DNA and microRNA for the prediction and diagnosis of several events related to transplantation (2–4). Although proteomics has been applied in the study of liver injury (5), proteomic methods have only recently started to been used for biomarker discovery but also to improving the understanding of the molecular mechanisms in transplantation (6). Proteomics is defined as the unbiased global analysis of the quantitative expression and identification of proteins in a cell, tissue, or organ. Instead of the classical approach in which a candidate gene or protein guides all analyses, protein profiling provides a powerful method to analyze the role of proteins in disease processes in an unbiased manner. Proteomics assays capture a large set of proteins, providing an approach to identify possible biomarkers and mediators and the advantage of elucidating the overall patterns of lesion-induced changes at the protein level (7). Moreover, functional proteomics provides a superior capacity over other techniques to identify modified proteins involved in multiple networks of living cells or body fluids (8). In addition, proteomics complements gene profiling because it represents the regulation of translation, post-translational modifications, protein kinetics, protein-protein interactions, and protein losses. Integrative approaches are expected to unify high-throughput genomics, proteomics and bioinformatics to develop what is known as “transplantomics” in the field of transplantation (9). The aim of proteomics is to unravel all underlying biological mechanisms to obtain information on the physiopathology of the alloimmune response, rejection, or IRI and, thus, to define new therapeutic targets (10). Studies have shown that the proteins involved in lipid and energy metabolism, redox signaling, and oxidative stress are affected during both the hot and cold ischemic phases of transplantation (11). Proteomic research can also be used to identify immunologic markers for operational tolerance in liver or kidney graft recipients. Regarding rejection, the availability of clinical biomarkers of immune activation may allow individualized patient management to avoid acute rejection events (11).
Here, we review the use of proteomics in LT including graft rejection, IRI, immune tolerance, and other transplant derived diseases. To do so, we analyzed different reported techniques and sample sources and compared human and animal studies to describe the principal relevant proteins overlapping among independent studies. Moreover, we highlight the limitations in the proteomic approaches of the different studies as one of the major challenge for the future of this research.
Experimental Procedures
This systematic literature review (SLR) was carried out following the Preferred Reporting Items for Systematic Reviews and Meta-Analyses (PRISMA) checklist (12) and the guidelines for performing SLR in bioinformatics (BiSLR) (13).
Search Strategy
We performed a systematic review of scientific publications that analyzed the proteomic profiles of liver transplant samples. All relevant articles were searched without date limits from the PubMed, Scopus, and ScienceDirect databases. The search terms included a combination of standardized index terms and plain language to cover the terms “liver transplantation”; “liver graft”; “liver transplant”; “liver allograft”; “proteomics”; and “proteome”. These keywords were defined by consensus among authors and customized for each database (Table S1). The search was completed in April 2020.
Selection Criteria
The searches were limited to studies published in the English language using the standard limitations provided by the respective databases. To be eligible for screening, the studies had to meet the following criteria: (a) identification of LT proteomics biomarkers as the main objective, (b) including human or animal models, and (c) application of methodologies for differential expression analysis from proteome profiling. Studies lacking adequate information on the experimental design were not included. Abstracts, book chapters, and review papers were also not considered.
Study Selection
To reduce the risk of bias (13), four researchers from different fields (bioinformatics, biology, proteomics, and medicine) independently reviewed the retrieved articles to assess their suitability for inclusion in this review. After removing duplicates using Mendeley reference management software, study selection was performed in four steps: (a) title screening, (b) abstract screening, (c) diagonal reading (focused on introduction, figures, tables, and conclusion); and (d) full-text reading (13). Only when more than half of the authors accepted inclusion based on steps (a) to (c), those studies were selected for the next step (d), where each reviewer independently evaluated the articles according to a scoring system and the following set of five questions (1): “does the article try to identify biomarkers related to liver transplant?” (2); “has the article generated proteome data?;” (3) “is the methodology based on differential expression analysis of proteome data?” (4); “does the article identify the experimental design, groups, and types or number of samples?”; and (5)”does the article properly report the list of potential biomarkers and the respective fold-change, p-value, or differential patterns observed (up-regulation or down-regulation) in the main text or supplementary material?”. For each question, scores were given following predefined criteria: 0 if the article does not meet any requirement of the question; 1 if it only partially meets the requirements; and 2 if it meets all the requirements. Articles with an average score equal to or higher than 6 among all evaluations were included.
Analysis and Data Collection
Data were independently extracted by all investigators using a standardized form and disagreements were resolved by consensus. A narrative summary of the results was produced according to specific data subjects: (a) the article’s author and year of publication; (b) study characteristics, including the design, study model, study type, sample type, proteomic technology, data analysis methods, presence or absence of validation; and (c) differential expression patterns of potential biomarkers (up- or down-regulated). For each study, the reported proteins were extracted from tables, figures, text, or supplemental material. For evaluation purposes, the protein identifiers were converted to the official gene symbol nomenclature (14).
Pathway Enrichment Design
Pathway and gene ontology (GO) enrichment analyses were performed for the differentially expressed proteins in the different study types (IRI, rejection, tolerance) or sample sources (plasma/serum or biopsies), using the corresponding functions of the limma package in the R statistics language (15).
These functions perform over-representation analyses by computing one-sided hypergeometric tests equivalent to Fisher’s exact tests. The p-values returned by these functions are not adjusted for multiple testing because the terms and pathways are often overlapping and standard methods of p-value adjustment may be very conservative. Therefore, caution should be exercised when interpreting the results. Only very small p-values should be considered.
Risk of Bias Measurement
Due to the large heterogeneity in the study types, we adapted and answered 13 items of the Downs and Black checklist for a critical evaluation of the quality of the cited papers (Table S2) (3).
Results
Literature Search and Studies Descriptions
A total of 95 references were collected from the PubMed, ScienceDirect, and Scopus databases. Duplicates were removed, resulting in 85 studies. After the screening phase, 56 studies were excluded. Among the remaining 29 studies, seven were excluded for average scores below 6. Finally, the SLR included 22 studies (Figure 1). Fifty percent of the studies were from Asia, 27% were from the US, and 18% were from Europe. Only one paper was from South America (16). The selected studies were published between 2004 and 2019, with 40% published in the last 5 years (Table 1).
Proteomics Strategies
Two-dimensional gel electrophoresis (2D gel)- based proteomics is the most widely used strategy described in selected publications (50%); however, shotgun proteomics has displaced this strategy, as this proteomic method has been applied most frequently in the last 5 years (62.5%). Only four of the publications used antibody arrays, two of which were in combination with 2D gel-based proteomics analyses (20, 33) (Table 2). The application of prefractionation techniques to reduce the high dynamic range in protein abundance is increasing, as we observed that their use has doubled in the last 5 years (62.5% vs.21.4%). Protein identification was carried out mainly by tandem mass spectrometry (MS/MS) (75%), but only half of the selected publications performed validation, principally by western blot (80%). The protein quantification most used in 2D gel-based proteomics was silver staining (50%), while stable isotope labeling of peptides with isobaric tags for relative and absolute quantitation (iTRAQ) method (62%) was the most often used method for shotgun proteomics (Table 2). In addition, two publications carried out fluorescent multiplexing technology (25, 30). The median number of selected proteins differentially expressed per study was 37, ranging from 7 (33) to 197 (35) (Table 2).
Sample Sources and Study Types
Half of the publications were conducted as clinical trials with human samples, whereas the other half used rats as an animal model (Table 1). The sample size was highly variable for human studies, with a mean of 23 patients per trial, with a range from 3 to 96 patients (Table 1). The mean number of rats per analysis group was 7, with a minimum of 3 and a maximum of 15 (Table 1). Likewise, most of the proteomics were performed with liver biopsies (50%), followed by serum or plasma (41%) (Table 1). Only one study each used peripheral blood mononuclear cells (32) or preservation solution (35) as sample source. Most of the selected publications focused on liver IRI (41%), followed by acute liver graft rejection (27.3%) and immunological LT tolerance (18.2%). Only one paper each analyzed three other scenarios such as primary graft non-function (28), chronic kidney disease (CKD) associated with LT (25) or liver regeneration after living-donor LT (22) (Table 1). The IRI studies were quite variable. Among human samples, two publications analyzed samples only after reperfusion. Zhang et al. (37) compared healthy volunteers to liver transplant patients 1 to 7 days after reperfusion, while Parvanien et al. (24) took samples from the portal vein, the hepatic vein, and the radial artery, and compared changes among the different types of blood. On the other hand, Coskun et al. (35) analyzed cold ischemia using organ preservation solution as a sample source. Three other studies recollected both ischemia and reperfusion samples and compared them to control (pre-ischemia) samples and even between them (18, 29, 36). When rats were used as a model, Knecht et al. (16) carried out ex vivo cold storage and normothermic reperfusion, whereas the other 2 publications performed a model of in vivo selective warm ischemia using vascular clamping followed by reperfusion, such in normal (20) as in steatotic rats (27).
Only one study on rejection analyzed chronic rejection (31), whereas the rest were focused on acute cellular rejection, of which, two were carried out in rats (19, 21) and three with human samples (26, 32, 34), although Massoud et al. (26) enrolled hepatitis C virus -positive patients in their trial.
In contrast, spontaneous tolerance in a rat model of LT was the main model used in the tolerance selected publications (17, 23, 33).
Commonly Identified Proteins Among Independent Studies
A total of 497 proteins detected by proteomics were identified by the selected studies (354 and 211 from humans and rats, respectively) (Table S3). Of these, 68 proteins (13.68%) overlapped between species (Figure 2A; Table S4A). Among 114 proteins identified in serum or plasma (101 in human and 25 in rats), 12 (10.53%) overlapped between species (Figure 2B; Table S4B). Among 250 proteins detected in liver biopsy studies (80 in human and 190 in rat), 20 (8%) overlapped between species (Figure 2C; Table S4C). Almost half of the proteins annotated from serum or plasma (45.6%) were among the 150 most abundant proteins in plasma (38) (Table S4D).
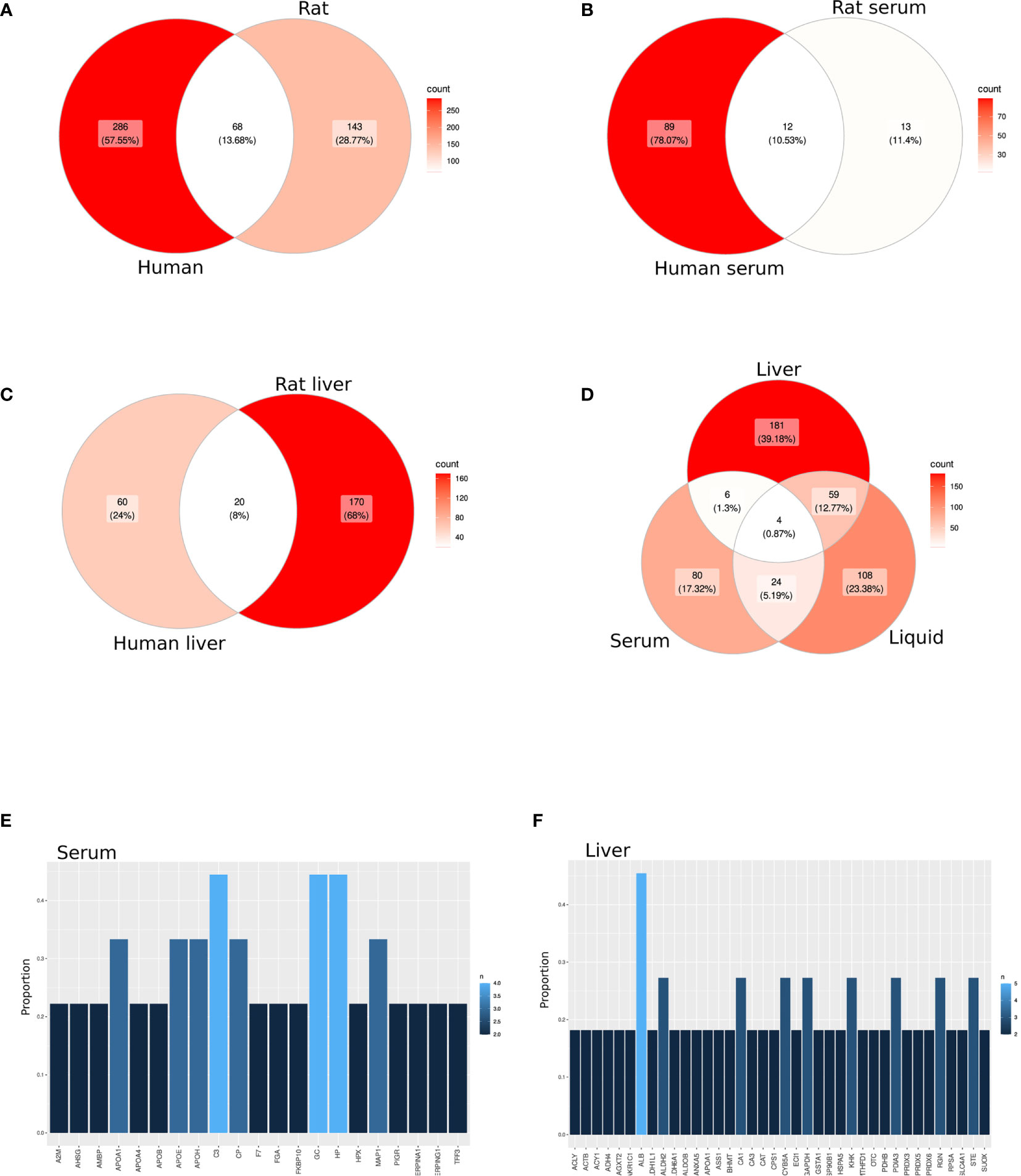
Figure 2 Candidate protein biomarkers from different sample source. (A–D) Venn diagram among all selected studies in a cross-species (A–C) or cross-sample source (D) analysis. (E, F) Bar graph representing the most shared proteins among all selected studies in serum/plasma (E) or liver biopsies (F).
Likewise, only 10 proteins (2.17%) overlapped between serum and tissue samples, including both animal and human samples (Figure 2D; Table S4E). However, 63 (13.64%) and 28 (6.1%) proteins overlapped between preservation solution and liver biopsies or serum, respectively (Figure 2D; Tables S4F, G). Moreover, four proteins (0.87%), including C-reactive protein (CRP), Glutathione S-transferase alpha 1 (GSTA1), Hemoglobin subunit beta (HBB) and Hemopexin, were found in all sample sources (Table S4H).
Among the selected studies based on serum or plasma, three proteins were found in 44.44% of the publications (Complement C3 (C3), GC vitamin D binding protein (GC) and Haptoglobin), whereas other five proteins [Apolipoprotein A1 (APOA1), Apolipoprotein E (APOE), Apolipoprotein H (APOH), Ceruloplasmin and Microtubule associated protein 1 (MAP1)] were found in one-third of the studies (Figure 2E; Table S4I). However, when liver biopsies were the sample source for proteomics, only albumin was found in almost half of the publications, followed by eight proteins differentially expressed in at least three publications (27.3%) (Figure 2F; Table S4J).
On the other hand, two proteins [albumin and carbonic anhydrase 1 (CA1)] were described as differentially expressed in four of the nine selected works related to IRI and eight proteins in three publications, whereas other 76 proteins were annotated in two studies (Figure 3A; Table S4K). Likewise, eight proteins were shared in one-third of the graft rejection studies [Actin beta (ACTB), Aldehyde dehydrogenase 2 family member (ALDH2), Catalase, Cofilin 1 (CFL1), Clustering, FKBP Prolyl Isomerase 10 (FKBP10), Regucalcin and Sulfite oxidase (SUOX)] (Figure 3B; Table S4L). Moreover, haptoglobin was shared in 100% of the tolerance publications, while MAP1 was present in 75% of the studies and other four proteins (APOE, C3, Ceruloplasmin and GC) appeared in 50% of the studies (Figure 3C; Table S4M). Moreover, 40 proteins (8.39%) were shared between IRI and rejection studies (Table S4N), 13 (2.73%) between IRI and tolerance (Table S4O), and 8 (1.63%) between rejection and tolerance (Table S4P) studies. A total of 1.05% of the proteins described (albumin, APOA1, C3, GSTA1 and hemopexin) were shared among the three different study types (Figure 3D; Table S4Q).
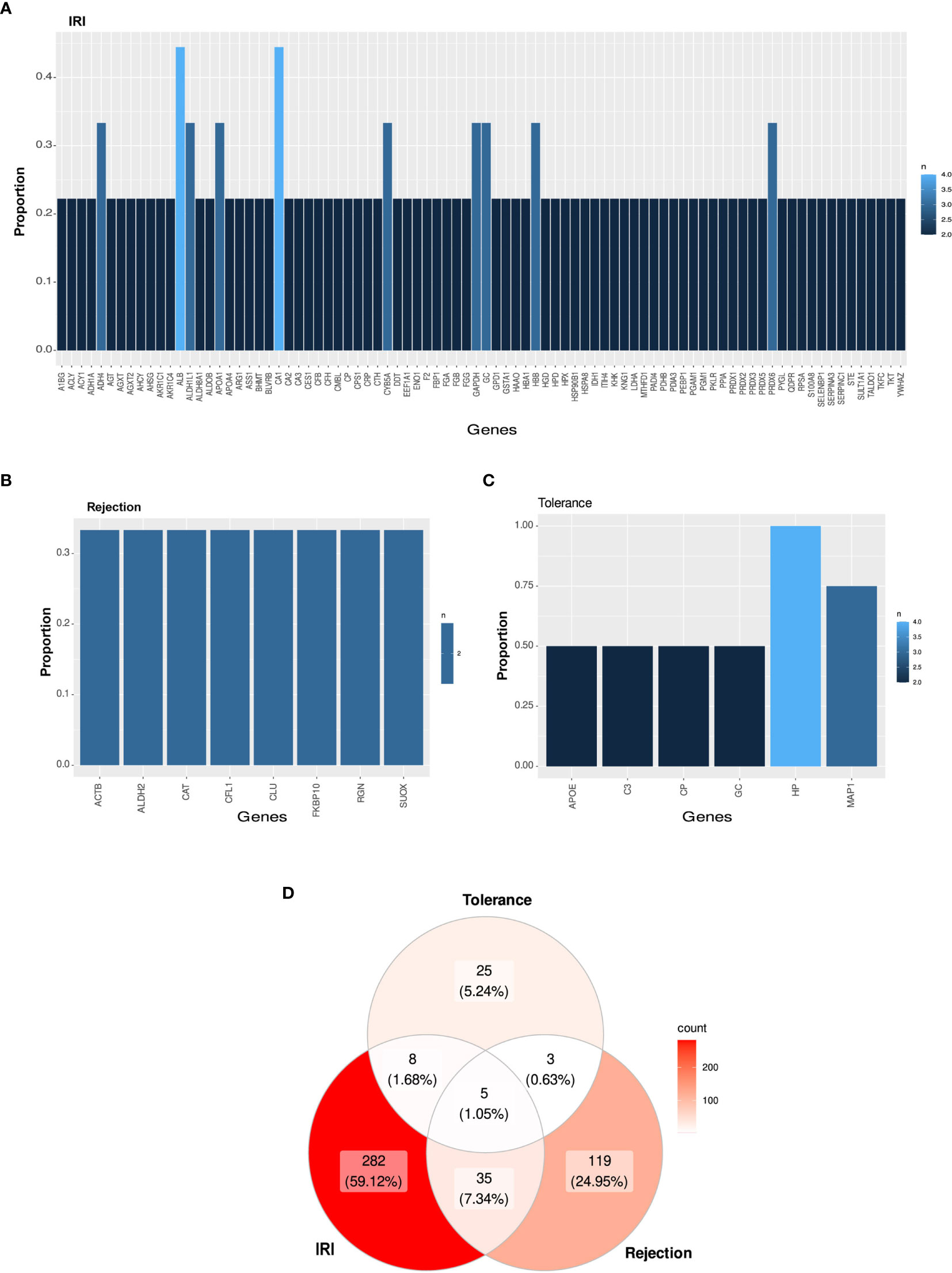
Figure 3 Candidate protein biomarkers from different types of study. (A–C) Bar graph representing the most shared proteins among all selected studies in IRI (A), rejection (B) or tolerance (C). (D) Venn diagram among all selected publications in cross-type of study analysis.
Differential patterns of protein expression were reported for 78.67% of the listed proteins, in which 241 proteins were up-regulated among all study types or sample sources and 190 were down-regulated. Furthermore, 40 of these proteins (10.23%) were described as both up- and down-regulated, in several publications (Figure 4A; Table S4R). When analyzed by study type, IRI showed the most duplicated patterns [14 proteins including Estrogen sulfotransferase (STE), Ribosomal protein SA (RPSA), Protein disulfide isomerase family A member 3 (PDIA3), Heat shock protein 90 alpha family class A member 1 (HSP90B1), GC, Cytochrome B5 type A (CYB5A), CA1, APOA1, Aldehyde dehydrogenase 1 family member L (ALDH1L1), Albumin, Aldo-keto reductase family member C1 (AKR1C1), Alanine-glyoxylate aminotransferase 2 (AGXT2), Alcohol dehydrogenase 4 (ADH4) and ATP citrate lyase (ACLY)], followed by tolerance studies with four proteins (MAP1, Haptoglobin, GC and Ceruloplasmin) and graft rejection with two proteins (CA1 and ACTB) (Figure 4B).
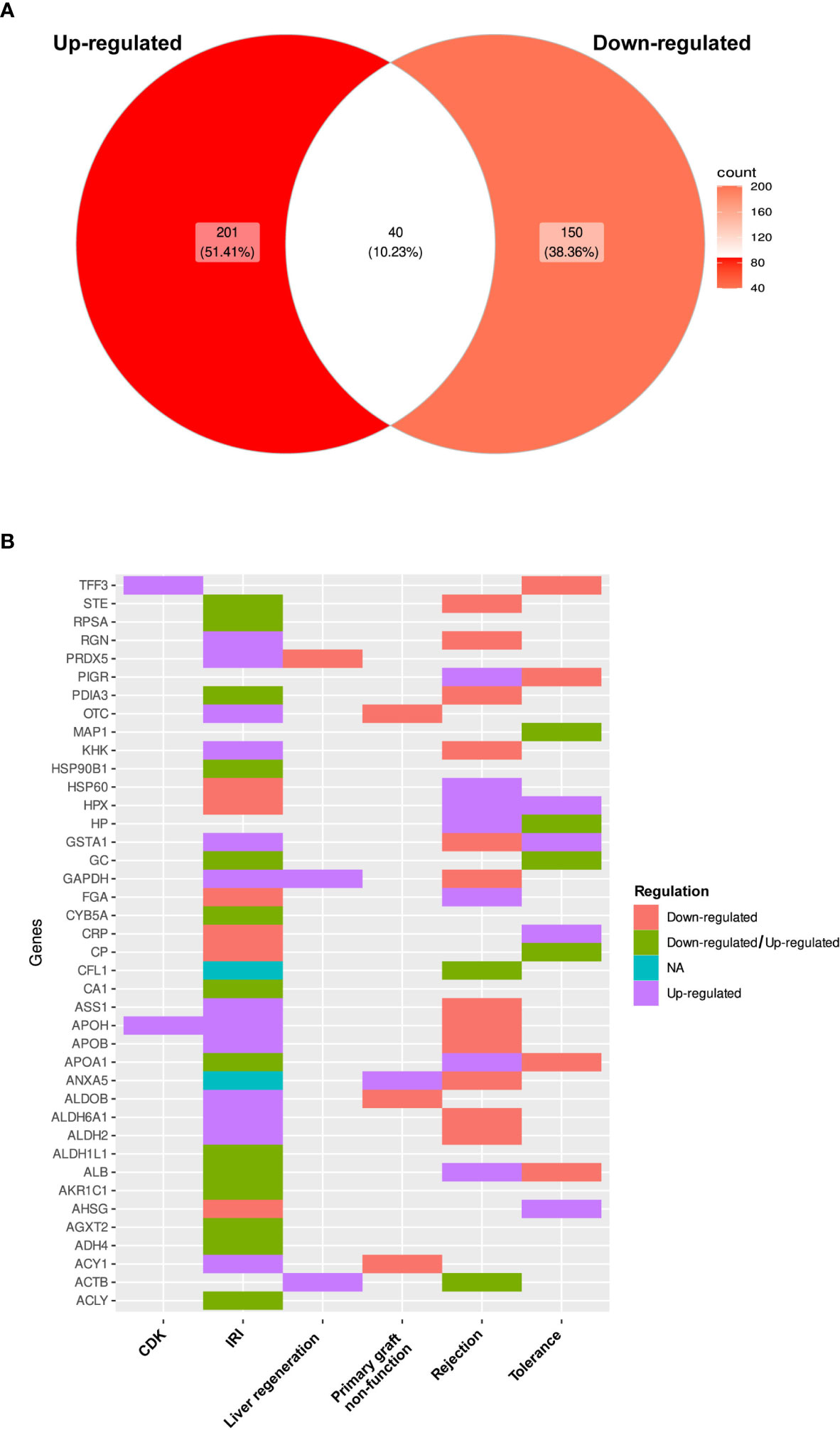
Figure 4 Differential pattern of protein expression. (A) Comparison of differential expression patterns observed for proteins among all selected studies expressed as a Venn diagram. (B) Heat plot of the list of proteins that had evidence supporting both types of expression changes.
Only Huang et al. (36) carried out a correlation analysis of proteomic results with transcriptomic data.
Enriched Pathways
In this study, 47 and 43 pathways were associated, respectively, with the protein list for serum or liver samples. Metabolic pathways were the most representative in both cases, including carbon metabolism (hsa01200), glycolysis/gluconeogenesis (hsa00010), and amino acid biosynthesis (hsa01230) (Tables S5A, B). Although 70% of the pathways were shared (Table S5C), complement/coagulation cascades (hsa04610), and interleukin (IL)-17 signaling pathway (path04657) were specific for serum, whereas protein processing in the endoplasmic reticulum (hsa04141) was related exclusively to liver tissue samples. Analysis of specific studies showed that metabolic pathways (hsa01100) and complement/coagulation cascades were the principal pathways involved in IRI (Table S5D). Likewise, metabolic pathways were the most important in rejection (Table S5E), whereas cytokine-cytokine receptor interaction (hsa04060), complement/coagulation cascades, or IL-17 (path04657) and tumor necrosis factor (TFN) signaling pathways (path04668) were the most prominent in tolerance (Table S5F).
Quality Evaluation
Table S2 shows the methodological quality scores of the included studies according to the Downs and Black methods. The average score was 56.99%, and eight of the 22 studies achieved scores equal to or greater than 60%. In general, the studies clearly stated their objectives, hypotheses, and main findings. However, most of the studies did not properly describe the main confounders. Moreover, the number of participants was reduced in general, so the score in the representative source population and outcome measures was also low.
Discussion
Proteome studies are powerful tools in translational clinical research. However, this relatively new and expensive technique requires a complex infrastructure (39) which might explain why there have only been a few studies and limited work focused in LT and why most have been conducted in the US and China during the last 15 years. Ideally, the techniques applied should be able to identify and quantify all proteins present in any clinical sample. To achieve this goal, it is essential to upgrade protein and peptide separation techniques, mass spectrometry instrumentation, and bioinformatics software together with the accession to complete sequence genome databases (40, 41). Unfortunately, deep proteome analysis is hampered by the high dynamic range in protein abundances (42) of an estimated seven orders of magnitude, while current mass spectrometry instruments can only analyze four orders of magnitude. Therefore, mass spectrometry-based proteomics requires the combination of different separation techniques to resolve complex mixtures of proteins to reach the goal of identifying and quantifying all proteins. 2D gels have been widely used since the first proteomics studies (43, 44), although they have been replaced by bottom-up or shotgun proteomics due to the increased number of proteins detected, reduced sample handling, and higher sampling throughput. The shotgun strategies are based on protease digestion of protein mixtures, peptide separation by liquid chromatography, and the identification by MS/MS of some tryptic peptides of each protein, covering a low percentage of their sequence, but allowing their identification (45). Furthermore, the stable isotope labeling of peptides (iTRAQ or TMT-tags) enables the quantification of the changes in abundance for thousands of proteins in a single experiment by multiplexing up to eleven different peptide samples (46, 47). Despite this yield, shotgun proteomics experiments can only detect half of the proteins present in a proteome (42) and the information obtained is limited to the few tryptic peptides that allow the identification of the protein. In contrast to 2D-gel-based techniques, we cannot distinguish between the different isoforms of a certain protein or if any of them have changed their concentration after LT. In clinical research, the high dynamic range in plasma protein levels is well known (48) for which several strategies have been developed to address this obstacle. Chromatographic methods for depleting the most abundant proteins present in serum/plasma (49) or the application of combinatorial hexapeptide ligand libraries (ProteoMiner™ beads) (50) enable the identification of hundreds of proteins using a shotgun proteomics approach (24, 34). However, the identification of thousands of proteins requires an extensive fractionation of serum/plasma using affinity and/or ionic chromatography before applying mass spectrometry-based proteomics to each fraction (26, 37). In 2D gel proteomics, protein quantification is performed using staining methods before protein digestion and mass spectrometry analysis. Modern sensitive techniques such as difference gel electrophoresis (DIGE) (51) or silver (52) staining protocols allow the detection of hundreds of protein spots. Furthermore, the design of DIGE experiments allows a reduced number of biological replicates compared to other staining methods such as silver or Coomassie Blue staining (53). However, 2D gel spot identification of hundreds of proteins hampers the proper quantification of each protein present in the spot using only the gel staining step (54). The presence of several proteins in the same spot also complicates their identification using Peptide Mass Fingerprinting (18, 19), a common mass spectrometry analysis method linked to 2D gel proteomics (55); thus, MS/MS is required to improve protein identification (56). The proteins identified by both 2D gel and shotgun proteomics approaches require validation using other methodologies since they are inferred from peptides sequenced by MS/MS and not from complete protein. In contrast to bottom-up proteomics strategies that involve protease digestion before mass spectrometry analysis, top-down proteomics strategies identify and quantify intact proteins directly through their fragmentation in the mass spectrometer (57). This methodology enables the study of individual proteoforms (58), which is not possible through “bottom-up” proteomics strategies, opening a new approach in clinical proteomics. Therefore, top-down proteomics can describe protein species generated due to RNA splicing, post-translational modification, or proteolysis. However, several obstacles still hamper complete proteome analysis, such as the proteoform pre-fractionation step and the limitation of high-resolution mass spectrometers. The application of gel-eluted liquid fraction entrapment electrophoresis (59), as a proteoform pre-fractionation method, in addition to high-resolution mass spectrometry, has allowed the identification of proteoforms that describe novel protein biomarkers associated with the liver transplant rejection (32). Another approach to solving the difficulties associated with the high dynamic range present in biological samples is to apply targeted proteomic tools as antibody arrays. This technology allows the quantification of hundreds of proteins previously selected due to their clinical interest. This approach has been applied in the field of LT to identify liver biomarkers using hepatic biopsies (20) or plasma (25, 30). The development of equipment combining fluorescence and multiplexing technologies has allowed a notable increase in the numbers of proteins detected in small volumes of sample [189 proteins (25, 30) compared to 40 for antibody arrays (20)].
Rat LT is a well-established experimental model (60) with hundreds of publications in the last 15 years. Moreover, preclinical studies with rodents have been accepted as a previous step in clinical trials (61). However, only 14% of the proteins found to be differentially expressed in rat studies were replicated in human samples. Although these species share 95% of their genes (62), there are differences in their liver transcriptomes (63). Similarly, hepatocytes synthesize most of serum proteins in the endoplasmic reticulum (ER), so that serum levels of hepatocyte-made proteins comprise significant biomarkers that reflect both systemic processes and liver status (64). However, only 2% of proteins were shared between serum and liver tissue in our SLR. This might be biased by the fact that the tolerance studies always started from serum, whereas IRI and graft rejection studies started mainly with biopsies. Nevertheless, the processes that took place in both samples implied similar enrichment of pathways but included different proteins. Moreover, protein processing in the ER pathway appeared enriched only in liver tissue samples. However, the presence of shared proteins between liver tissue and preservation solution samples increased up to 14%, as many cellular components are released following damage occurring during cold ischemia (65). It is, therefore, not surprising that both samples showed metabolic pathways enrichment, as liver is known as the metabolic factory (66) (Table S5G).
Ischemia-reperfusion is a pathological condition resulting from an initial restriction of blood supply to an organ followed by the restoration of perfusion and reoxygenation (67). Although surgical techniques and immunosuppressants increase transplant success rates, allograft rejection remains a major problem, with a maximum incidence within the first weeks (68). IRI after deceased donor transplantation increases the rate of acute and chronic rejection and is estimated to account for 10% of early organ failure (69). Thus, it is not surprising that IRI and rejection studies shared more proteins. However, 13 of these proteins appeared indistinctly up- or down-regulated in both study. Likewise, 17 proteins could not be compared since they were identified in an IRI study conducted with preservation fluid (35), where the differential expression pattern was not available. In other words, only 25% of the proteins shared between IRI and rejection studies showed the same patterns. Nevertheless, reperfusion injury, results not only from metabolic disturbances but also from an inflammatory immune response (70). However, although there is an enrichment of different metabolic pathways in both IRI and rejection, only the related immune response complement/coagulation cascades and hypoxia inducible factor 1-alpha (HIF-1a) signaling pathways appear to be somewhat prominent in IRI and rejection samples, respectively. Apoptosis plays an important role in IRI during LT. HIF-1a may trigger liver apoptosis following IRI through the induction of hypoxically regulated genes (71).
Operational tolerance is defined as the state in which the allograft is allowed to survive at a level that provides adequate clinical function in the absence of IS (72). The achievement of immune tolerance to an allogeneic graft is a field of intense research, fueled by a critical need to avoid IS-related side effects (73). Different groups have identified biomarkers to distinguish tolerant patients from those who are going to experience rejection, including genes (74, 75), T cell receptors (76–78), activated regulatory T-cells (Treg) and related miRNAs (79). However, proteomic analysis has not been commonly used to study tolerance. The Chen group in Kaohsiung Chang Gung Memorial Hospital (Taiwan) has studied the proteomic signature of spontaneous tolerance in a rat model of LT (17, 23, 33). The rat models of tolerance can be induced spontaneously in LT between different strains (80). In this way, studies in tolerance proteomics were more similar, with haptoglobin reported to be differentially expressed in every publication. Same authors showed haptoglobin to be differentially expressed in serum from a liver transplant recipient after IS withdrawal (81). Moreover, in their proteomic study using Luminex Bead technology, Levitsky et al. (30) reported haptoglobin as significantly differentially expressed. However, haptoglobin is reported to be both down- (23) and up-regulated (17, 33) in different studies by the same authors. Moreover, haptoglobin was also reported in one rejection study (21), when tolerance is a totally antagonistic phenomenon. This protein has also been identified as among the 12 most abundant plasma proteins in serum (38).
Proteomics is a promising technique that can be used to detect a large number of proteins. Even so, progress remains slow and further research is needed to develop a treatment (82). The experimental variability between different study types and the low numbers of participants do not help to identify specific biomarkers for IRI, rejection, or tolerance. Increasing the number of samples by means of cheaper validation methods is a likely possibility. Similarly, there is an urgent need to eliminate the most abundant proteins when working with serum or plasma samples. Selective depletion of a dozen high-abundance proteins extends analyses down about 1–2 orders of magnitude and depletion of about 150 major proteins extends the range to approximately 10,00-fold lower concentrations (38).
Although proteomics in LT, or any other field, is extremely promising on its own, the future of this technique lies in the improvement of the identification of proteoforms and their integration with other techniques such as genomics and metabolomics, which will help to provide a global view of the interactome of all the agents involved in a particular biological process.
Data Availability Statement
The original contributions presented in the study are included in the article/Supplementary Material. Further inquiries can be directed to the corresponding author.
Author Contributions
PR, FS-B, JP, RR-C, and AB-M participated in de design of the study. VL-L, JM-A, FP-S, CT-M, and AB-M participated in the performance of the research. RR-C, VL-L, JM-A, and AB-M participated in data analysis. All authors contributed to the article and approved the submitted version.
Funding
AB-M was funded by Fundación Mutua Madrileña, Grant/Award Number: AP171362019 and Instituto de Salud Carlos III, Grant/Award Number: PI20/00185. JP and RR-C were funded by Instituto de Salud Carlos III, Grant/Award Numbers: PI17/00489 and PI13/02870, respectively. Funding sources provided financial support but had no involvement in study design, collection, analysis and interpretation of data.
Conflict of Interest
The authors declare that the research was conducted in the absence of any commercial or financial relationships that could be construed as a potential conflict of interest.
Publisher’s Note
All claims expressed in this article are solely those of the authors and do not necessarily represent those of their affiliated organizations, or those of the publisher, the editors and the reviewers. Any product that may be evaluated in this article, or claim that may be made by its manufacturer, is not guaranteed or endorsed by the publisher.
Supplementary Material
The Supplementary Material for this article can be found online at: https://www.frontiersin.org/articles/10.3389/fimmu.2021.672829/full#supplementary-material
Abbreviations
ACTB, Actin beta; APO, Apolipoprotein; CA1, Carbonic anhydrase 1; CKD, Chronic kidney disease; C3, Complement C3; DIGE, Difference gel electrophoresis; ER, Endoplasmic reticulum; GC, GC vitamin D binding protein; GSTA1, Glutathione S-transferase alpha 1; IS, Immunosuppression; IL-17, Interleukin-17; IRI, Ischemia reperfusion injury; iTRAQ, Isobaric tags for relative and absolute quantitation; LT, Liver transplantation; MAP1, Microtubule associated protein 1; PRISMA, Preferred Reporting Items for Systematic Reviews and Meta-Analyses; SLR, Systematic literature review; MS/MS, Tandem mass spectrometry; 2D gel, Two-dimensional gel electrophoresis.
References
1. Roedder S, Vitalone M, Khatri P, Sarwal MM. Biomarkers in Solid Organ Transplantation: Establishing Personalized Transplantation Medicine. Genome Med (2011) 3(6):37. doi: 10.1186/gm253gm253
2. Farid WR, Verhoeven CJ, de Jonge J, Metselaar HJ, Kazemier G, van der Laan LJ. The Ins and Outs of microRNAs as Biomarkers in Liver Disease and Transplantation. Transpl Int (2014) 27(12):1222–32. doi: 10.1111/tri.12379
3. Paladini SV, Pinto GH, Bueno RH, Calloni R, Recamonde-Mendoza M. Identification of Candidate Biomarkers for Transplant Rejection From Transcriptome Data: A Systematic Review. Mol Diagn Ther (2019) 23(4):439–58. doi: 10.1007/s40291-019-00397-y
4. Perez-Sanz F, Revilla-Nuin B, Martinez-Alarcon L, Herrero JI, Ramirez P, Pons JA, et al. Tolerance Biomarkers in Liver Transplantation: Independent External Validation of the Predictive Strength of SENP6 and FEM1C Gene Expression. Transplantation (2019) 103(9):1887–92. doi: 10.1097/TP.0000000000002587
5. Parent R, Beretta L. Proteomics in the Study of Liver Pathology. J Hepatol (2005) 43(1):177–83. doi: 10.1016/j.jhep.2005.04.001
6. Sigdel TK, Sarwal MM. Recent Advances in Biomarker Discovery in Solid Organ Transplant by Proteomics. Expert Rev Proteomics (2011) 8(6):705–15. doi: 10.1586/epr.11.66
7. Christians U, Klawitter J, Klawitter J. Biomarkers in Transplantation—Proteomics and Metabolomics. Ther Drug Monit (2016) 38:S70–4. doi: 10.1097/ftd.0000000000000243
8. Colland F, Jacq X, Trouplin V, Mougin C, Groizeleau C, Hamburger A, et al. Functional Proteomics Mapping of a Human Signaling Pathway. Genome Res (2004) 14(7):1324–32. doi: 10.1101/gr.2334104
9. Kienzl-Wagner K, Brandacher G. Proteomics in Transplantation. Adv Clin Chem (2014) 67:215–44. doi: 10.1016/bs.acc.2014.09.004
10. Traum AZ, Schachter AD. Transplantation Proteomics. Pediatr Transplant (2005) 9(6):700–11. doi: 10.1111/j.1399-3046.2005.00408.x
11. McManus CA, Rose ML, Dunn MJ. Proteomics of Transplant Rejection. Transplant Rev (2006) 20(4):195–207. doi: 10.1016/j.trre.2006.08.001
12. Moher D, Liberati A, Tetzlaff J, Altman DG. Preferred Reporting Items for Systematic Reviews and Meta-Analyses: The PRISMA Statement. BMJ (2009) 339:b2535. doi: 10.1136/bmj.b2535
13. Mariano DCB, Leite C, Santos LHS, Rocha REO, de Melo-Minardi RC. A Guide to Performing Systematicquery Literature Reviews in Bioinformatics. Technical Report RT.DCC.002/2017. arXiv (2017) arXiv:1707.05813.
14. Wain HM, Bruford EA, Lovering RC, Lush MJ, Wright MW, Povey S. Guidelines for Human Gene Nomenclature. Genomics (2002) 79(4):464–70. doi: 10.1006/geno.2002.6748
15. Ritchie ME, Phipson B, Wu D, Hu Y, Law CW, Shi W, et al. Limma Powers Differential Expression Analyses for RNA-Sequencing and Microarray Studies. Nucleic Acids Res (2015) 43(7):e47. doi: 10.1093/nar/gkv007
16. Knecht C, Balaban CL, Rodríguez JV, Ceccarelli EA, Guibert EE, Rosano GL. Proteome Variation of the Rat Liver After Static Cold Storage Assayed in an Ex Vivo Model. Cryobiology (2018) 85:47–55. doi: 10.1016/j.cryobiol.2018.10.003
17. Pan T-L, Wang P-W, Huang C-C, Goto S, Chen C-L. Expression, by Functional Proteomics, of Spontaneous Tolerance in Rat Orthotopic Liver Transplantation. Immunology (2004) 113(1):57–64. doi: 10.1111/j.1365-2567.2004.01924.x
18. Vascotto C, Cesaratto L, D'Ambrosio C, Scaloni A, Avellini C, Paron I, et al. Proteomic Analysis of Liver Tissues Subjected to Early Ischemia/Reperfusion Injury During Human Orthotopic Liver Transplantation. Proteomics (2006) 6(11):3455–65. doi: 10.1002/pmic.200500770
19. Zhang C, Zhu F, Wei J, Zheng S, Li L. A Proteomic Analysis of Allograft Rejection in Rats After Liver Transplantation. Sci China Ser C: Life Sci (2007) 50(3):312–9. doi: 10.1007/s11427-007-0038-9
20. Svetlov SI, Xiang Y, Oli MW, Foley DP, Huang G, Hayes RL, et al. Identification and Preliminary Validation of Novel Biomarkers of Acute Hepatic Ischaemia/Reperfusion Injury Using Dual-Platform Proteomic/Degradomic Approaches. Biomarkers (2008) 11(4):355–69. doi: 10.1080/13547500600775110
21. Cheng J, Zhou L, Jiang JW, Qin YS, Xie HY, Feng XW, et al. Proteomic Analysis of Differentially Expressed Proteins in Rat Liver Allografts Developed Acute Rejection. Eur Surg Res (2010) 44(1):43–51. doi: 10.1159/000264602
22. Wu J, Tang Q, Shen J, Yao A, Wang F, Pu L, et al. Comparative Proteome Profile During the Early Period of Small-for-Size Liver Transplantation in Rats Revealed the Protective Role of Prdx5. J Hepatol (2010) 53(1):73–83. doi: 10.1016/j.jhep.2010.01.032
23. Pan T-L, Wang P-W, Chen S-T, Fang J-Y, Hsu T-K, Sintupisut N, et al. Prospective Highlights of Serum Glycoproteins in Spontaneous Tolerance After Orthotopic Liver Transplantation. Clin Chim Acta (2011) 412(7-8):604–13. doi: 10.1016/j.cca.2010.12.014
24. Parviainen V, Joenvaara S, Tukiainen E, Ilmakunnas M, Isoniemi H, Renkonen R. Relative Quantification of Several Plasma Proteins During Liver Transplantation Surgery. J BioMed Biotechnol (2011) 2011:248613. doi: 10.1155/2011/248613
25. Levitsky J, Salomon DR, Abecassis M, Langfelder P, Horvath S, Friedewald J, et al. Clinical and Plasma Proteomic Markers Correlating With Chronic Kidney Disease After Liver Transplantation. Am J Transplant (2011) 11(9):1972–8. doi: 10.1111/j.1600-6143.2011.03669.x
26. Massoud O, Heimbach J, Viker K, Krishnan A, Poterucha J, Sanchez W, et al. Noninvasive Diagnosis of Acute Cellular Rejection in Liver Transplant Recipients: A Proteomic Signature Validated by Enzyme-Linked Immunosorbent Assay. Liver Transplant (2011) 17(6):723–32. doi: 10.1002/lt.22266
27. Tiriveedhi V, Conzen KD, Liaw-Conlin J, Upadhya G, Malone J, Townsend RR, et al. The Role of Molecular Chaperonins in Warm Ischemia and Reperfusion Injury in the Steatotic Liver: A Proteomic Study. BMC Biochem (2012) 13:17. doi: 10.1186/1471-2091-13-17
28. Kornasiewicz O, Bojarczuk K, Bugajski M, Golab J, Krawczyk M. Application of a Proteomic Approach to Identify Proteins Associated With Primary Graft non-Function After Liver Transplantation. Int J Mol Med (2012) 30(4):755–64. doi: 10.3892/ijmm.2012.1062
29. Wu B, Wu H, Chen J, Hua X, Li N, Lu M. Comparative Proteomic Analysis of Human Donor Tissues During Orthotopic Liver Transplantation: Ischemia Versus Reperfusion. Hepatol Int (2012) 7(1):286–98. doi: 10.1007/s12072-012-9346-7
30. Levitsky J, Mathew JM, Abecassis M, Tambur A, Leventhal J, Chandrasekaran D, et al. Systemic Immunoregulatory and Proteogenomic Effects of Tacrolimus to Sirolimus Conversion in Liver Transplant Recipients. Hepatology (2013) 57(1):239–48. doi: 10.1002/hep.25579
31. Wei W, Huang X-H, Liang D, Zeng Y-Y, Ma C, Wu Y-B, et al. A Proteomic Analysis of Transplanted Liver in a Rat Model of Chronic Rejection. Clinics Res Hepatol Gastroenterol (2015) 39(3):340–50. doi: 10.1016/j.clinre.2014.10.005
32. Toby TK, Abecassis M, Kim K, Thomas PM, Fellers RT, LeDuc RD, et al. Proteoforms in Peripheral Blood Mononuclear Cells as Novel Rejection Biomarkers in Liver Transplant Recipients. Am J Transplant (2017) 17(9):2458–67. doi: 10.1111/ajt.14359
33. Wang P-W, Wu T-H, Pan T-L, Chen M-H, Goto S, Chen C-L. Integrated Proteome and Cytokine Profiles Reveal Ceruloplasmin Eliciting Liver Allograft Tolerance via Antioxidant Cascades. Front Immunol (2018) 9:2216. doi: 10.3389/fimmu.2018.02216
34. Jiang Q, Ru Y, Yu Y, Li K, Jing Y, Wang J, et al. iTRAQ-Based Quantitative Proteomic Analysis Reveals Potential Early Diagnostic Markers in Serum of Acute Cellular Rejection After Liver Transplantation. Transplant Immunol (2019) 53:7–12. doi: 10.1016/j.trim.2018.11.005
35. Coskun A, Baykal AT, Oztug M, Kazan D, Kaya E, Emiroglu R, et al. Proteomic Analysis of Liver Preservation Solutions Prior to Liver Transplantation. Curr Proteomics (2019) 16(2):119–35. doi: 10.2174/1570164615666180905104543
36. Huang S, Ju W, Zhu Z, Han M, Sun C, Tang Y, et al. Comprehensive and Combined Omics Analysis Reveals Factors of Ischemia-Reperfusion Injury in Liver Transplantation. Epigenomics (2019) 11(5):527–42. doi: 10.2217/epi-2018-0189
37. Zhang R, Ou M, Zhang Y, Yan Q, Chen H, Lai L, et al. Comparative Proteomic Analysis of Human Serum Before and After Liver Transplantation Using Quantitative Proteomics. Oncotarget (2019) 10(26):2508–14. doi: 10.18632/oncotarget.26761
38. Anderson NL, Sviridov D, Hortin GL. High-Abundance Polypeptides of the Human Plasma Proteome Comprising the Top 4 Logs of Polypeptide Abundance. Clin Chem (2008) 54(10):1608–16. doi: 10.1373/clinchem.2008.108175
39. Cho WCS. Proteomics Technologies and Challenges. Genomics Proteomics Bioinf (2007) 5(2):77–85. doi: 10.1016/s1672-0229(07)60018-7
40. Aebersold R, Mann M. Mass Spectrometry-Based Proteomics. Nature (2003) 422(6928):198–207. doi: 10.1038/nature01511
41. Ong SE, Mann M. Mass Spectrometry-Based Proteomics Turns Quantitative. Nat Chem Biol (2005) 1(5):252–62. doi: 10.1038/nchembio736
42. Zubarev RA. The Challenge of the Proteome Dynamic Range and its Implications for in-Depth Proteomics. Proteomics (2013) 13(5):723–6. doi: 10.1002/pmic.201200451
43. Gorg A, Weiss W, Dunn MJ. Current Two-Dimensional Electrophoresis Technology for Proteomics. Proteomics (2004) 4(12):3665–85. doi: 10.1002/pmic.200401031
44. O'Farrell PH. High Resolution Two-Dimensional Electrophoresis of Proteins. J Biol Chem (1975) 250(10):4007–21. doi: 10.1016/S0021-9258(19)41496-8
45. Nesvizhskii AI, Aebersold R. Interpretation of Shotgun Proteomic Data: The Protein Inference Problem. Mol Cell Proteomics (2005) 4(10):1419–40. doi: 10.1074/mcp.R500012-MCP200
46. Ross PL, Huang YN, Marchese JN, Williamson B, Parker K, Hattan S, et al. Multiplexed Protein Quantitation in Saccharomyces Cerevisiae Using Amine-Reactive Isobaric Tagging Reagents. Mol Cell Proteomics (2004) 3(12):1154–69. doi: 10.1074/mcp.M400129-MCP200
47. Thompson A, Schafer J, Kuhn K, Kienle S, Schwarz J, Schmidt G, et al. Tandem Mass Tags: A Novel Quantification Strategy for Comparative Analysis of Complex Protein Mixtures by MS/MS. Anal Chem (2003) 75(8):1895–904. doi: 10.1021/ac0262560
48. Anderson NL, Anderson NG. The Human Plasma Proteome: History, Character, and Diagnostic Prospects. Mol Cell Proteomics (2002) 1(11):845–67. doi: 10.1074/mcp.r200007-mcp200
49. Pieper R, Su Q, Gatlin CL, Huang ST, Anderson NL, Steiner S. Multi-Component Immunoaffinity Subtraction Chromatography: An Innovative Step Towards a Comprehensive Survey of the Human Plasma Proteome. Proteomics (2003) 3(4):422–32. doi: 10.1002/pmic.200390057
50. Boschetti E, Righetti PG. The Art of Observing Rare Protein Species in Proteomes With Peptide Ligand Libraries. Proteomics (2009) 9(6):1492–510. doi: 10.1002/pmic.200800389
51. Unlu M, Morgan ME, Minden JS. Difference Gel Electrophoresis: A Single Gel Method for Detecting Changes in Protein Extracts. Electrophoresis (1997) 18(11):2071–7. doi: 10.1002/elps.1150181133
52. Shevchenko A, Wilm M, Vorm O, Mann M. Mass Spectrometric Sequencing of Proteins Silver-Stained Polyacrylamide Gels. Anal Chem (1996) 68(5):850–8. doi: 10.1021/ac950914h
53. Karp NA, Lilley KS. Investigating Sample Pooling Strategies for DIGE Experiments to Address Biological Variability. Proteomics (2009) 9(2):388–97. doi: 10.1002/pmic.200800485
54. Zhan X, Yang H, Peng F, Li J, Mu Y, Long Y, et al. How Many Proteins can be Identified in a 2DE Gel Spot Within an Analysis of a Complex Human Cancer Tissue Proteome? Electrophoresis (2018) 39(7):965–80. doi: 10.1002/elps.201700330
55. Henzel WJ, Watanabe C, Stults JT. Protein Identification: The Origins of Peptide Mass Fingerprinting. J Am Soc Mass Spectrom (2003) 14(9):931–42. doi: 10.1016/S1044030503002149
56. Lu B, Motoyama A, Ruse C, Venable J, Yates JR. 3rd: Improving Protein Identification Sensitivity by Combining MS and MS/MS Information for Shotgun Proteomics Using LTQ-Orbitrap High Mass Accuracy Data. Anal Chem (2008) 80(6):2018–25. doi: 10.1021/ac701697w
57. Tran JC, Zamdborg L, Ahlf DR, Lee JE, Catherman AD, Durbin KR, et al. Mapping Intact Protein Isoforms in Discovery Mode Using Top-Down Proteomics. Nature (2011) 480(7376):254–8. doi: 10.1038/nature10575
58. Smith LM, Kelleher NL. Proteoform: A Single Term Describing Protein Complexity. Nat Methods (2013) 10(3):186–7. doi: 10.1038/nmeth.2369
59. Tran JC, Doucette AA. Multiplexed Size Separation of Intact Proteins in Solution Phase for Mass Spectrometry. Anal Chem (2009) 81(15):6201–9. doi: 10.1021/ac900729r
60. Yagi S, Uemoto S, Kobayashi E. Orthotopic Liver Transplantation in Rats. In: Chen H, Qian S, editors. Experimental Organ Transplantation. New York: Nova Science Publishers (2013).
61. Bryda EC. The Mighty Mouse: The Impact of Rodents on Advances in Biomedical Research. Mo Med (2013) 110(3):207–11.
62. Gibbs RA, Weinstock GM, Metzker ML, Muzny DM, Sodergren EJ, Scherer S, et al. Genome Sequence of the Brown Norway Rat Yields Insights Into Mammalian Evolution. Nature (2004) 428(6982):493–521. doi: 10.1038/nature02426
63. Yu Y, Ping J, Chen H, Jiao L, Zheng S, Han Z-G, et al. A Comparative Analysis of Liver Transcriptome Suggests Divergent Liver Function Among Human, Mouse and Rat. Genomics (2010) 96(5):281–9. doi: 10.1016/j.ygeno.2010.08.003
64. Kuscuoglu D, Janciauskiene S, Hamesch K, Haybaeck J, Trautwein C, Strnad P. Liver – Master and Servant of Serum Proteome. J Hepatol (2018) 69(2):512–24. doi: 10.1016/j.jhep.2018.04.018
65. Konishi T, Lentsch AB. Hepatic Ischemia/Reperfusion: Mechanisms of Tissue Injury, Repair, and Regeneration. Gene Expression (2017) 17(4):277–87. doi: 10.3727/105221617x15042750874156
66. Cascante M, Marí E. Organization and Regulation of the Metabolic Factory. In: Cornish-Bowden A, editor. New Beer in an Old Bottle: Eduard Buchner and the Growth of Biochemical Knowlegde. Valencia: Universitat de Valencia (1997).
67. Eltzschig HK, Eckle T. Ischemia and Reperfusion—From Mechanism to Translation. Nat Med (2011) 17(11):1391–401. doi: 10.1038/nm.2507
68. Choudhary NS, Saigal S, Bansal RK, Saraf N, Gautam D, Soin AS. Acute and Chronic Rejection After Liver Transplantation: What A Clinician Needs to Know. J Clin Exp Hepatol (2017) 7(4):358–66. doi: 10.1016/j.jceh.2017.10.003
69. Rampes S, Ma D. Hepatic Ischemia-Reperfusion Injury in Liver Transplant Setting: Mechanisms and Protective Strategies. J BioMed Res (2019) 33(4):221–34. doi: 10.7555/JBR.32.20180087
70. Zhai Y, Petrowsky H, Hong JC, Busuttil RW, Kupiec-Weglinski JW. Ischaemia–reperfusion Injury in Liver Transplantation—From Bench to Bedside. Nat Rev Gastroenterol Hepatol (2012) 10(2):79–89. doi: 10.1038/nrgastro.2012.225
71. Cursio R, Miele C, Filippa N, Van Obberghen E, Gugenheim J. Liver HIF-1 Alpha Induction Precedes Apoptosis Following Normothermic Ischemia-Reperfusion in Rats. Transplant Proc (2008) 40(6):2042–5. doi: 10.1016/j.transproceed.2008.05.037
72. Chandrasekharan D, Issa F, Wood KJ. Achieving Operational Tolerance in Transplantation: How can Lessons From the Clinic Inform Research Directions? Transplant Int (2013) 26(6):576–89. doi: 10.1111/tri.12081
73. Baroja-Mazo A, Revilla-Nuin B, Parrilla P, Martínez-Alarcón L, Ramírez P, Pons JA. Tolerance in Liver Transplantation: Biomarkers and Clinical Relevance. World J Gastroenterol (2016) 22(34):7676. doi: 10.3748/wjg.v22.i34.7676
74. Li L, Wozniak LJ, Rodder S, Heish S, Talisetti A, Wang Q, et al. A Common Peripheral Blood Gene Set for Diagnosis of Operational Tolerance in Pediatric and Adult Liver Transplantation. Am J Transplant (2012) 12(5):1218–28. doi: 10.1111/j.1600-6143.2011.03928.x
75. Martínez-Llordella M, Lozano JJ, Puig-Pey I, Orlando G, Tisone G, Lerut J, et al. Using Transcriptional Profiling to Develop a Diagnostic Test of Operational Tolerance in Liver Transplant Recipients. J Clin Invest (2008) 118(8):2845–57. doi: 10.1172/jci35342
76. Bohne F, Londono MC, Benitez C, Miquel R, Martinez-Llordella M, Russo C, et al. HCV-Induced Immune Responses Influence the Development of Operational Tolerance After Liver Transplantation in Humans. Sci Transl Med (2014) 6(242):242ra81. doi: 10.1126/scitranslmed.3008793
77. Li Y, Koshiba T, Yoshizawa A, Yonekawa Y, Masuda K, Ito A, et al. Analyses of Peripheral Blood Mononuclear Cells in Operational Tolerance After Pediatric Living Donor Liver Transplantation. Am J Transplant (2004) 4(12):2118–25. doi: 10.1111/j.1600-6143.2004.00611.x
78. Martínez-Llordella M, Puig-Pey I, Orlando G, Ramoni M, Tisone G, Rimola A, et al. Multiparameter Immune Profiling of Operational Tolerance in Liver Transplantation. Am J Transplant (2007) 7(2):309–19. doi: 10.1111/j.1600-6143.2006.01621.x
79. Revilla-Nuin B, de Bejar A, Martinez-Alarcon L, Herrero JI, Martinez-Caceres CM, Ramirez P, et al. Differential Profile of Activated Regulatory T Cell Subsets and microRNAs in Tolerant Liver Transplant Recipients. Liver Transpl (2017) 23(7):933–45. doi: 10.1002/lt.24691
80. Zimmermann FA, Davies HS, Knoll PP, Gokel JM, Schmidt T. Orthotopic Liver Allografts in the Rat. The Influence of Strain Combination on the Fate of the Graft. Transplantation (1984) 37(4):406–10. doi: 10.1097/00007890-198404000-00019
81. Hsu L-W, Goto S, Nakano T, Lai C-Y, Lin Y-C, Kao Y-H, et al. Immunosuppressive Activity of Serum Taken From a Liver Transplant Recipient After Withdrawal of Immunosuppressants. Transplant Immunol (2007) 17(2):137–46. doi: 10.1016/j.trim.2006.06.001
Keywords: rejection, tolerance, mass spectrometry, ischemia – reperfusion, PRISMA
Citation: López-López V, Pérez-Sánz F, de Torre-Minguela C, Marco-Abenza J, Robles-Campos R, Sánchez-Bueno F, Pons JA, Ramírez P and Baroja-Mazo A (2021) Proteomics in Liver Transplantation: A Systematic Review. Front. Immunol. 12:672829. doi: 10.3389/fimmu.2021.672829
Received: 26 February 2021; Accepted: 12 July 2021;
Published: 26 July 2021.
Edited by:
Jukka Partanen, Finnish Red Cross Blood Service, FinlandReviewed by:
Zhiguang Huo, University of Florida, United StatesMichael Merchant, University of Louisville, United States
Copyright © 2021 López-López, Pérez-Sánz, de Torre-Minguela, Marco-Abenza, Robles-Campos, Sánchez-Bueno, Pons, Ramírez and Baroja-Mazo. This is an open-access article distributed under the terms of the Creative Commons Attribution License (CC BY). The use, distribution or reproduction in other forums is permitted, provided the original author(s) and the copyright owner(s) are credited and that the original publication in this journal is cited, in accordance with accepted academic practice. No use, distribution or reproduction is permitted which does not comply with these terms.
*Correspondence: Alberto Baroja-Mazo, alberto.baroja@ffis.es
†These authors have contributed equally to this work