- 1Department of Pathology, Stanford University School of Medicine, Palo Alto, CA, United States
- 2Histocompatibility, Immunogenetics and Disease Profiling Laboratory, Stanford Blood Center, Palo Alto, CA, United States
- 3Department of Neurology, School of Medicine, University of California San Francisco, San Francisco, CA, United States
- 4Neurology IV Unit Neuroimmunology and Neuromuscular Diseases, Fondazione I.R.C.C.S Istituto Neurologico Carlo Besta (INCB), Milan, Italy
- 5Department of Immunology and Transfusion Medicine, Oslo University Hospital, Oslo, Norway
- 6Institute of Clinical Medicine, University of Oslo, Oslo, Norway
- 7Department of Medical Genetics, University of Oslo and Oslo University Hospital, Oslo, Norway
- 8Department of Immunology, Genetics and Pathology (IGP), Rudbeck Laboratory, Uppsala University and University Hospital, Uppsala, Sweden
- 9Department of Neurology, Oslo University Hospital and University of Oslo, Oslo, Norway
- 10Department of Clinical Neuroscience, Karolinska Institutet, Stockholm, Sweden
- 11Department of Neurology, Oslo University Hospital, Oslo, Norway
- 12The Department of Laboratory Medicine, Karolinska Institutet, Stockholm, Sweden
- 13Department of Clinical Research and Innovation, Fondazione I.R.C.C.S Istituto Neurologico Carlo Besta (INCB), Milan, Italy
Genetic susceptibility to myasthenia gravis (MG) associates with specific HLA alleles and haplotypes at the class I and II regions in various populations. Previous studies have only examined alleles at a limited number of HLA loci that defined only broad serotypes or alleles defined at the protein sequence level. Consequently, genetic variants in noncoding and untranslated HLA gene segments have not been fully explored but could also be important determinants for MG. To gain further insight into the role of HLA in MG, we applied next-generation sequencing to analyze sequence variation at eleven HLA genes in early-onset (EO) and late-onset (LO) non-thymomatous MG patients positive for the acetylcholine receptor (AChR) antibodies and ethnically matched controls from Italy, Norway, and Sweden. For all three populations, alleles and haplotype blocks present on the ancestral haplotype AH8.1 were associated with risk in AChR-EOMG patients. HLA-B*08:01:01:01 was the dominant risk allele in Italians (OR = 3.28, P = 1.83E−05), Norwegians (OR = 3.52, P = 4.41E−16), and in Swedes HLA-B*08:01 was the primary risk allele (OR = 4.24, P <2.2E-16). Protective alleles and haplotype blocks were identified on the HLA-DRB7, and HLA-DRB13.1 class II haplotypes in Italians and Norwegians, whereas in Swedes HLA-DRB7 exhibited the main protective effect. For AChR-LOMG patients, the HLA-DRB15.1 haplotype and associated alleles were significantly associated with susceptibility in all groups. The HLA-DR13–HLA-DR–HLA-DQ haplotype was associated with protection in all AChR-LOMG groups. This study has confirmed and extended previous findings that the immunogenetic predisposition profiles for EOMG and LOMG are distinct. In addition, the results are consistent with a role for non-coding HLA genetic variants in the pathogenesis of MG.
Introduction
Myasthenia gravis (MG) is a rare antibody-mediated, T-cell dependent autoimmune disorder of the neuromuscular junction (NMJ) characterized by fluctuating muscle weakness and abnormal fatigability (1, 2). The global prevalence of MG ranges from 40 to 180 per million with an estimated annual incidence of 1.74 to 12 cases per million persons (3, 4). MG occurs across all ancestral groups and affects individuals of all ages and both sexes, although several studies have shown that MG exhibits a binomial distribution based on age and sex; MG is frequently observed in younger women aged less than 40 years old and older men over 60 years old (4).
In the majority of patients, ~85–90%, MG is a result of pathogenic autoantibodies targeting the nicotinic acetylcholine receptors (AChRs) located at the postsynaptic membrane of the NMJ (5–7). Anti-AChR autoantibodies predominantly consist of immunoglobulin subclasses IgG1 and IgG3, which are potent activators of complement resulting in membrane attack, impaired synaptic signal transmission, and muscle damage that manifests as skeletal muscle weakness (8). In addition, CD4+ T helper cells and the cytokines they secrete play important roles in the pathogenesis of MG. Rare forms of MG are due to autoantibodies targeting other postsynaptic membrane components such as the muscle-specific kinase (MuSK) protein, which is essential for NMJ formation and clustering of AChRs, and the lipoprotein receptor-related protein 4 (LRP4) a receptor that binds to neuronal agrin to activate MuSK (5, 9). Double-seronegative MG cases (meaning negative for AChR and MuSK antibodies) may have other antibodies targeting NMJ proteins (such as LRP4, Agrin, and Cortactin), but further studies are needed to determine their relevance for disease mechanism (5, 9, 10). The MG autoantibodies are important diagnostic factors and are used along with the clinical presentation (ocular vs. generalized), thymus pathology, and age of onset for classifying MG patients and to help guide treatment (3). The demarcation between early-onset MG (EOMG) and late-onset MG (LOMG) is not well-defined, but most studies agree than EOMG occurs at <50 years, and LOMG is >50 years old (3, 11). These two subtypes of MG differ in terms of sex affected and the associated susceptibility genes; EOMG is common in women with a hyperplastic thymus, whereas LOMG mostly afflicts men who have normal or atrophic thymus. The different MG subgroups clearly indicate that MG is a heterogeneous disease, suggesting that many predisposing factors are involved in determining disease pathogenesis. The disease etiology in MG is multifactorial involving a complex interplay of environmental and genetics factors. Familial cases of MG have been reported over the years, implicating the role of genetic factors in MG risk (12–16). Furthermore, a twin study examining MG heritability showed that the concordance rate between monozygotic twins (35%) was greater than dizygotic twins (3–4%), further underscoring the notion of a genetic predisposition (17). However, the incomplete concordance in monozygotic twins also suggests the contribution of a large environmental component to MG risk. The strongest genetic predictors of MG risk are the human leukocyte antigen (HLA) genes (18) located on chromosome 6p21.31. The associated HLA alleles vary according to the different subgroups of MG. Specifically, the HLA-A1-B8-DR3-DQ2 ancestral haplotype, also referred to as AH8.1 (19), or specific alleles which lie on this haplotype have been found to be strongly associated with AChR-MG and EOMG in several European populations (20–24). The HLA-B7-DR2 haplotype and the HLA-DRB1*15:01 allele were found to be associated with LOMG in two cohorts (25, 26). In a recent retrospective study performed in Italian MG patients HLA-DQB1*05:01 was associated with thymoma, and the HLA-DRB1*16~HLA-DQB1*05:02 haplotype with non-thymomatous AChR-MG with >60 years onset (27). These studies highlight the genetic heterogeneity in HLA alleles predisposing to various groups of MG among different populations. However, previous studies were limited by the small number of HLA genes interrogated in a single study, and restricted coverage of genes. Limited sequence coverage of HLA genes means that allele and phase ambiguities are likely to be present, thereby compromising the accuracy of the alleles and haplotypes defined. Therefore, re-examination of HLA associations with MG using extended coverage and advanced molecular typing methods is warranted. Next-generation sequencing (NGS) is an attractive option for variant characterization of HLA genes due to its comparatively low cost, high-accuracy and unbiased variant discovery. NGS of extended HLA gene segments has been applied successfully in population-based and trait-association studies to identify allelic variants in non-coding regions, and to achieve mostly unambiguous HLA genotyping (28–31). In this study, we report the analyses of HLA alleles and haplotypes, defined using high-resolution NGS, associated with susceptibility and protection to EOMG and LOMG in Italian, Norwegian, and Swedish cohorts.
Subjects and Methods
Study Populations
The study populations consisted of MG patients and healthy controls (HC) recruited from Italy (MG = 354, 219 females; HC = 250, 90 females), Norway (MG = 412, 252 females; HC = 500, 319 females), and Sweden (MG = 339, 202 females; HC = 2,120, sex unknown). All participants provided written informed consent at the site of study recruitment and arrived de-identified to the genotyping and analysis laboratories (Stanford University and the University of California San Francisco). All subjects included in the analyses were self-described white European. There was not a requirement for ancestors living in Italy for a certain number of generations, however, patients and controls were reported to be of Italian descent. White Norwegians was classified according to the birth country and ethnicity of the parent. The Swedish cohort is a single-center consecutively collected cohort of MG patients either treated or reviewed for second opinion at the Karolinska University Hospital in Stockholm, Sweden. Self-reported information on ancestry was collected at inclusion. There was no requirement for ancestors having lived in Sweden for a certain number of generations.
HLA Genotyping
HLA typing was performed on genomic DNA extracted from blood samples using local protocols such as the salting-out method or the standard phenol/chloroform method. For all DNA samples with the exception of Swedish HC samples which were typed previously by mid-resolution Sanger sequencing, were retrospectively typed at high-resolution for class I (HLA-A, HLA-C, HLA-B) and class II (HLA-DRB1, HLA-DRB3, HLA-DRB4, HLA-DRB5, HLA-DQA1, HLA-DQB1, HLA-DPA1, HLA-DPB1) HLA loci using the MIA FORA NGS high-throughput (HT) semi-automated typing protocol (Immucor, Inc., Norcross, GA, USA) and Illumina MiniSeq instruments (Illumina, Inc., San Diego, CA, USA). All sample testing including sequence data analysis and HLA genotype assignment using the MIA FORA FLEX v3.0 alignment software (Immucor, Norcross, GA, USA), were performed according to previously published methods (28).
Standardization of STR Ambiguities
The MIA FORA software permits detailed examination of all sequence segments, as well as unambiguous allele assignment, with the exception of short tandem repeat (STR) enriched regions located within introns of some class II genes. These low complexity regions consist of ~1–6 bp nucleotide units that are repeated numerous times and cannot be enumerated accurately by the sequencing methodology. In order to standardize alleles that are indistinguishable due to STRs, alleles were assigned to groups and were given the suffix SG (denoting STR Group) to the lowest numbered allele in that group (28, 30). For example, allele HLA-DRB1*03:01:01:01SG denotes the HLA-DRB1*03:01:01:01 and HLA-DRB1*03:01:01:02 STR ambiguous group. Details of HLA ambiguities are shown in Supplementary Table 1.
Statistical Analyses
Allele carrier frequencies were determined by direct counting from the sequencing results and were calculated by dividing the number of times a specific allele (either at the homozygous or heterozygous state) is observed in a population by the total number of copies of all alleles at that particular genetic locus in the population. The Bridging Immuno Genomic Data Analysis Workflow Gaps (BIGDAWG) software package v1.8 (32) was used to perform case-control phenotype association analyses. Association analyses were performed using chi-square (χ2) testing. Haplotypes frequencies were estimated using the R ‘haplo.stats’ package to run the expectation maximization (EM) algorithm in the BIGDAWG package. A tilde symbol “~” is used to show alleles located or shared on a specific haplotype block. Calculation of haplotype/allele confidence interval, odds ratio, and P-values were performed using the R epicalc package run in BIGDAWG. The effect sizes of HLA alleles and haplotypes on MG risk were measured by odds ratio (OR) with 95% confidence intervals (CI), and associated probability (P) values were derived from a two-tailed Fischer’s exact test or a Pearson’s chi-squared test when appropriate. Correction of nominal P-values for multiple testing was applied as follows. First the Bonferroni correction method was used to correct P-values generated from tests of overall (locus-level) heterogeneity at the locus. P-values less than 0.05 (α) were considered statistically significant. If the adjusted locus-level heterogeneity P-value at a particular locus was statistically significant allele-level association P-values were not corrected for multiple comparison, However, if the adjusted locus-level heterogeneity P-value at a locus was not statistically significant allele-level association P-values were corrected for multiple testing (i.e. multiplying the P-value by the number of identified alleles at each locus) using the Bonferroni method. Stratification analysis was applied to the primary risk alleles (i.e. the allele(s) with the strongest effect) to detect secondary alleles and haplotypes associated with the disease. Due to the small number of ocular patients from each population (ranging from 13 to 19 in the EOMG group, and three to 30 in the LOMG group), ocular data from all three groups were pooled together then analyzed. All calculated P-values were two-sided and P-values less than 0.05 (α) were considered statistically significant. Standardized linkage disequilibrium (LD) measures were computed using D’ (33) and the Wn statistic (34) at the locus-level and D’ij for alleles at two loci implemented in the PyPop software (35). In control subjects allele frequencies at all loci were tested for deviations from Hardy–Weinberg equilibrium (HWE) proportions using the exact method of Guo and Thompson (36). Analyses were performed using the R statistical program version 3.5.0 and SPSS version 21 software package (IBM SPSS Inc., Chicago, IL, USA).
Results
Demographics of Cohorts
MG datasets were categorized according to age of onset; early-onset MG (EOMG) age of <50 years, and late-onset MG (LOMG) age of >50. In addition, the cohorts were summarized according to ocular and generalized MG subtypes, autoantibody profile, and thymoma cases (Table 1). We first sought to determine whether gender influenced any of the clinical features described. We found that the distribution of male and females were significantly different among EOMG and LOMG patients in all three groups (P <0.001). There was a predominance of female cases in the EOMG group, ranging from 77.6% in the Italian cohort to 79.0% in Norwegians and 80.4% in Swedish patients. In contrast, LOMG was more common in men, occurring at 66.7% in Italians, 62.7% in Norwegians, and 61.1% in Swedes. These finding are in keeping with previous reports of sex differences in MG by age of onset. Similarly, females were prominent in the anti-AChR-Ab seropositive group in all three cohorts, ranging from 57 to 60%. Thymoma was slightly more common in women although the numbers are small. We restricted the analyses to patients that were non-thymomatous and antibody positive for the AChR since the dataset of the rarer antibody positive subsets were too small to analyze and make any inferences.
Locus-Level Heterogeneity in AChR-EOMG Patients
We tested eleven HLA loci characterized at maximum resolution in the Italian and Norwegian case-control cohorts and six loci in the Swedish group for allelic and haplotype heterogeneity and association with MG risk and protection. The Swedish data was examined at 2-field allelic resolution for six HLA genes (HLA-A, HLA-C, HLA-B, HLA-DRB1, HLA-DQB1, and HLA-DPB1) because the control sample used was previously genotyped for only these loci using mid-resolution molecular methods. For all three populations, HLA heterogeneity between AChR-EOMG non-thymomatous cases and controls were analyzed at the overall locus-level, the results of which are shown in Supplementary Table 2. In the Italian cohort following adjustment for multiple testing, the majority of loci and haplotypes were significant with the exception of HLA-A, HLA-C, HLA-DPA1, HLA-DPB1, and HLA-DPA1~HLA-DPB1. Similarly, in the Norwegian group most of the loci survived multiple testing and only the HLA-DP loci and haplotype results were not significant. In the Swedish cohort all loci with the exception of HLA-DPB1 were statistically significant. The significant results indicate differences in the distribution of HLA alleles between cases and controls.
The HLA-B*08~HLA-DR3~HLA-DQA1*05~HLA-DQB1*02 Haplotype Is Associated With AChR-EOMG Risk
The distribution of haplotype blocks encompassing two to eleven loci in cases and controls were compared. For all three case-control populations the strongest associations were identified between AChR-EOMG susceptibility and haplotype blocks present on the conserved ancestral haplotype (AH) 8.1 found in populations originating from Northern and Western Europe (Tables 2–4). Specifically, the risk haplotype blocks included various combinations of class I blocks (e.g. HLA-C*07:01:01:01~HLA-B*08:01:01:01), class II blocks (HLA-DRB1*03:01:01:01SG~HLA-DQA1*05:01:01:02), and class I and II blocks (HLA-B*08:01:01:01~HLA-DRB1*03:01:01:01SG). A similar pattern was observed in the Swedish population that was analyzed at 2-field allelic resolution. These results indicate that both class I and II regions of AH8.1 influence AChR-EOMG risk. It is noteworthy that the shorter haplotype blocks bearing HLA-B*08:01:01:01 have a greater effect on disease risk, compared to haplotype blocks without HLA- B*08:01:01:01, suggesting that HLA-B*08:01:01:01 is the dominant risk allele.
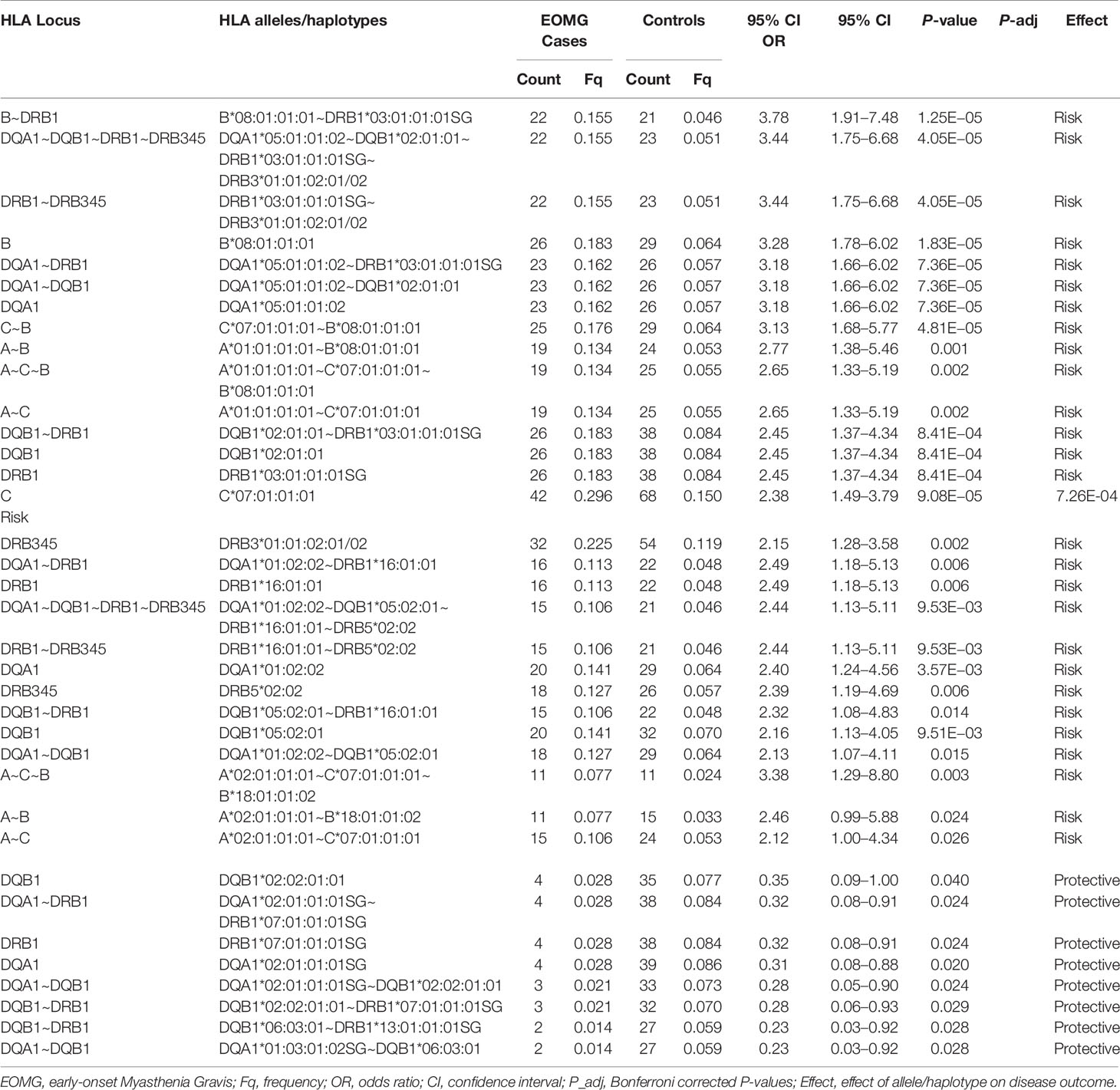
Table 2 Significant associations of HLA alleles and haplotypes in Italian EOMG AChR-antibody positive cases and controls ranked by odds ratios.
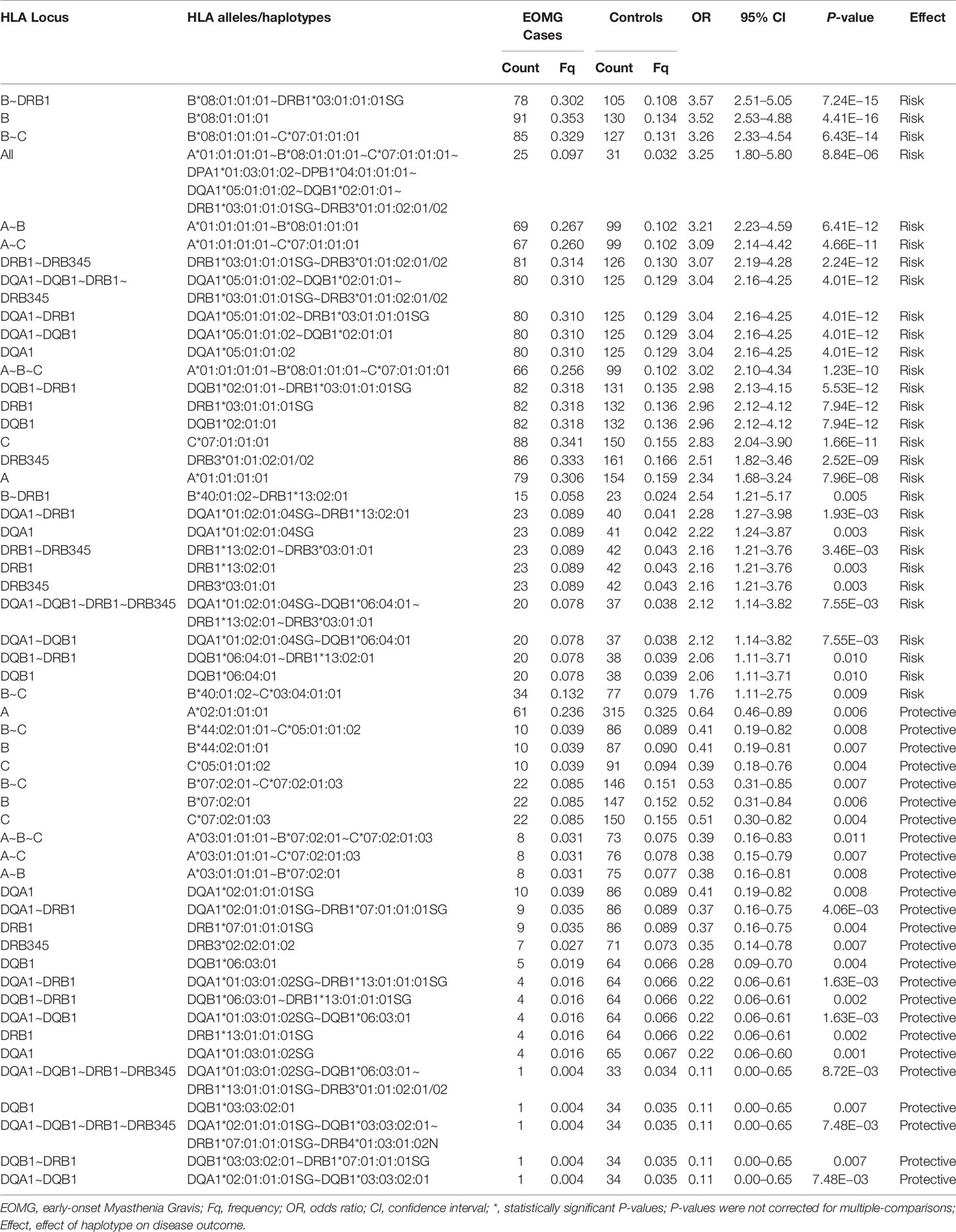
Table 3 Significant associations of HLA alleles and haplotypes in Norwegian Myasthenia Gravis EOMG AChR-antibody positive and controls ranked by odds ratios.
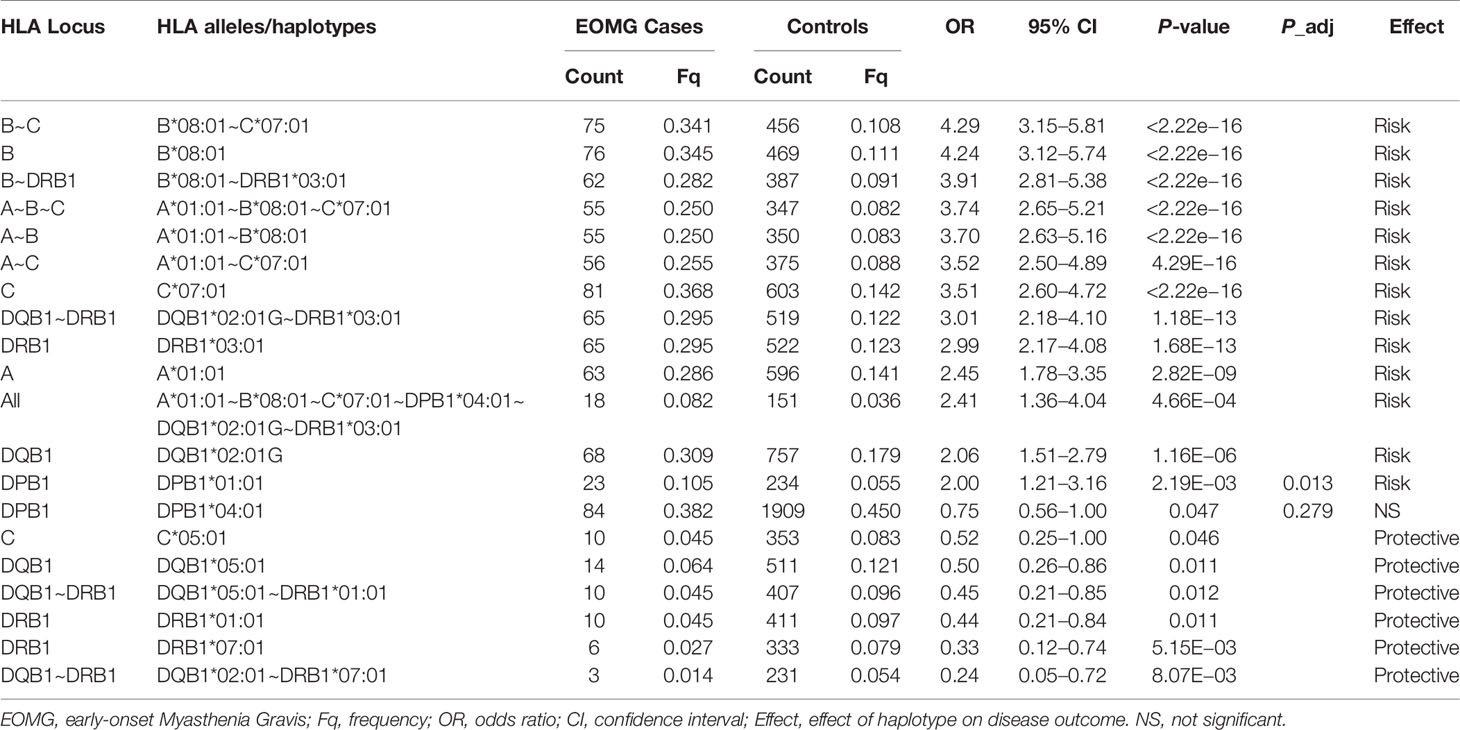
Table 4 Significant associations of HLA alleles and haplotypes in Swedish EOMG AChR-antibody positive cases and controls ranked by odds ratios.
To further investigate the relationship between AChR-EOMG and the AH8.1, we performed allele-level association analyses in each of the three study populations. In the Italian and Norwegian cohorts, HLA-B*08:01:01:01 and HLA-DQA1*05:01:01:02 were the top two alleles strongly associated with AChR-EOMG risk (Tables 2 and 3). HLA-B*08:01:01:01 was the dominant risk allele (Italians, OR = 3.28, CI = 1.78–6.02, P = 1.83E−05; Norwegians, OR = 3.52, CI = 2.53–4.88, P = 4.41E−16).
Likewise HLA-B*08:01 was the strongest risk factor in the Swedish cohort (Table 4; OR = 4.24, CI = 3.12–5.74, P <2.22E−16), followed by HLA-C*07:01 (OR = 3.51, CI = 2.60–4.72, P <2.22E−16); HLA-DQA1 loci were not typed in Swedes. Analyses of alleles reduced to 2-field resolution in the Italian and Norwegian cohorts revealed a similar pattern of significant allele frequency differences between cases and controls (data not shown). In the Italian and Norwegian cohorts it is noteworthy that the effect size of the HLA-DQA1*05:01 allele at the protein level is considerably less than observed at the intronic level: Italians OR = 2.45, CI = 1.37–4.34, P = 8.41E−04; Norwegians OR = 2.96, CI = 2.12–4.12, P = 7.94E−12. The alternative 4-field variants HLA-DQA1*05:01:01:01 and HLA-DQA1*05:01:01:03 allele frequencies are considerably less than HLA-DQA1*05:01:01:02 in these populations. HLA-DQA1*05:01:01:01 and HLA-DQA1*05:01:01:03 are not present on the 8.1 ancestral haplotype. Furthermore allele HLA-DRB3*02:02:01:01 is a marker of HLA-DQA1*05:01:01:01 and HLA-DQA1*05:01:01:03 haplotypes, whereas HLA-DRB3*01:01:02:01/02 is found on the HLA-DQA1*05:01:01:02 haplotype, indicating that the HLA-DQA1*05:01:01 4-field haplotypes are distinct. The association results of HLA-DQA1*05:01 compared to HLA-DQA1*05:01:01:02 clearly suggest that the SNP variant located in intron 2 of the HLA-DQA1*05:01:01:02 gene is an important determinant for AChR-EOMG risk (Figure 1).
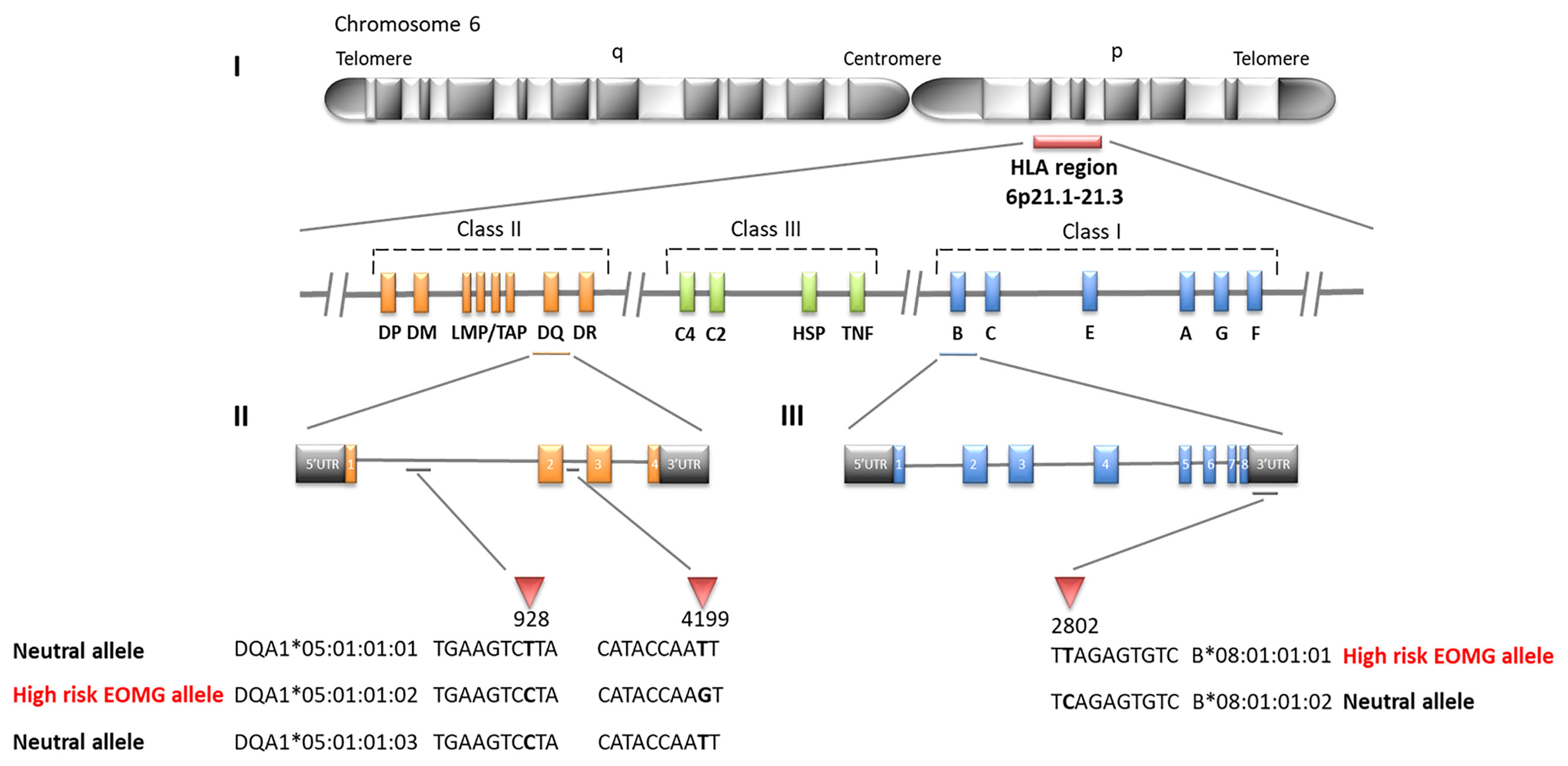
Figure 1 SNP differences in HLA alleles strongly associated with early-onset myasthenia gravis (EOMG) (I) Simplified map of the HLA region (II) Three different 4-field intronic variants of HLA-DQA1*05:01:01, only HLA-DQA1*05:01:01:02 is associated with high risk of EOMG the other alleles have neutral effects (III) Two 4-field variants of HLA-B*08:01:01 are distinguished by a SNP located in the 3’UTR; HLA-B*08:01:01:01 is a high risk EOMG allele whereas HLA-B*08:01:01:02 is neutral.
In addition to the susceptibility factors described above we found that HLA-DR16 alleles and haplotype-blocks (OR = 2.49, CI = 1.18–5.13, P = 0.006), and the HLA-A*02:01:01:01~HLA-C*07:01:01:01~HLA-B*18:01:01:02 haplotype (OR = 3.38, CI = 1.29–8.80, P = 0.003) were significantly associated with risk in Italians (Table 2). In Norwegians, HLA-DRB*13:02 class I and II haplotype blocks were also strong risk factors; HLA-B*40:01:02~HLA-DRB1*13:02:01, OR = 2.54, CI = 1.21–5.17, P = 0.005. Forest plots depicting the strongest statistically significant associated alleles with MG across groups are shown in Figure 2.
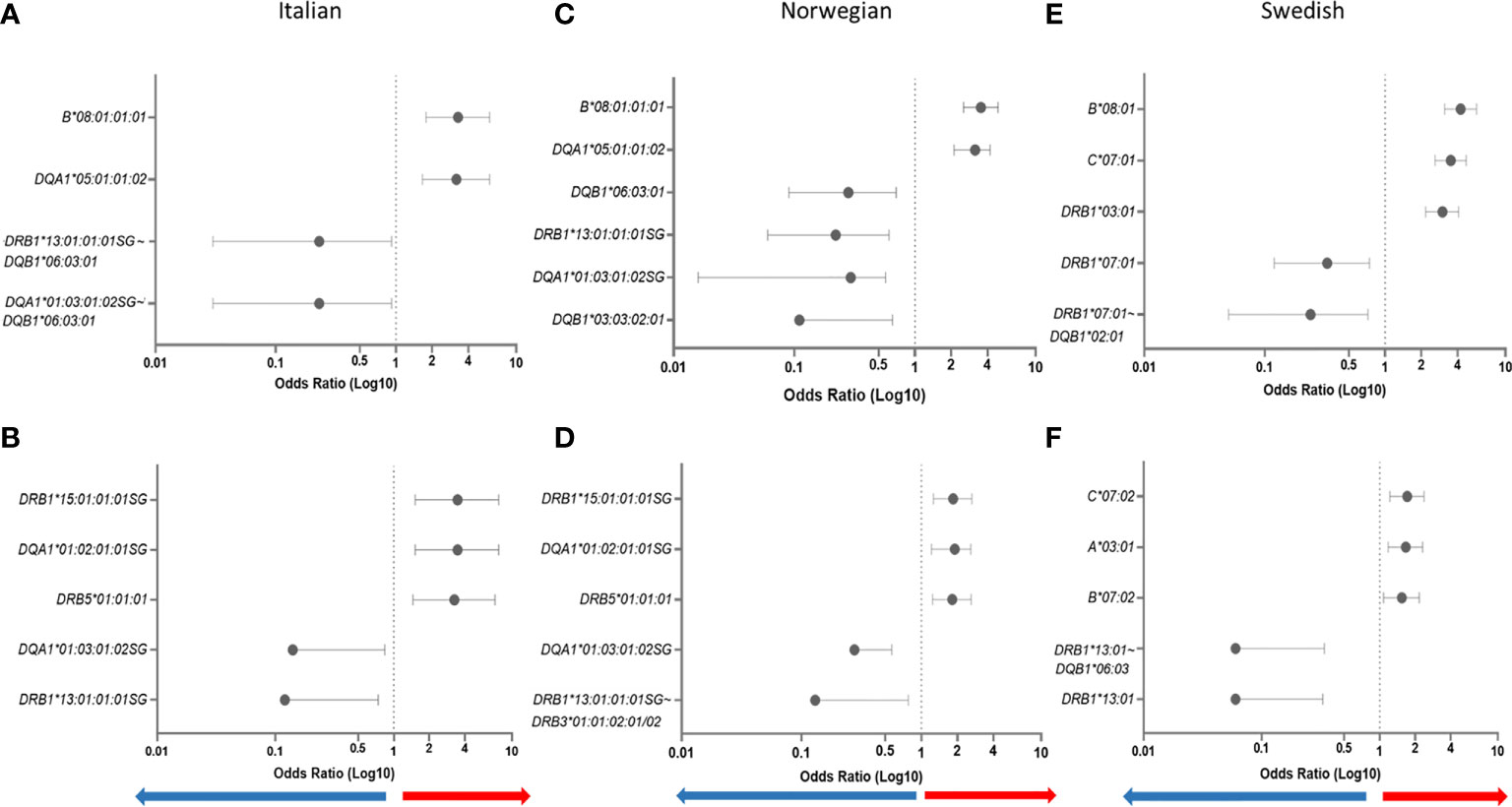
Figure 2 Forest plots depicting odds ratios and 95% confidence intervals of the most strongly associated HLA alleles with MG. HLA alleles/haplotypes in Italians EOMG (A) and LOMG (B); Norwegians EOMG (C) and LOMG (D); Swedish EOMG (E) and LOMG (F).
To determine whether sex influenced the associations observed we performed both case-control analyses stratified by sex and logistic regression adjusting for sex in Italian and Norwegian cohorts; sex data was not available for Swedish controls. Both sets of analyses for Italians and Norwegians produced very similar results to the original/unadjusted results (data not shown). Since the majority of AChR-EOMG cases in each three cohorts are comprised of females the association signals could be attributed to females. In logistic regression male sex had a negative coefficient estimate of ~−1.5 and the P-values were statistically significant indicating a negative correlation between males and AChR-EOMG.
We performed stratification analyses in the absence of the primary allele(s) with the strongest risk effects in order to identify secondary alleles associated with AChR-EOMG. Case-control analyses were performed in the absence of HLA-B*08:01:01:01, HLA-DQA1*05:01:01:02, and HLA-DRB1*03:01:01:01 (data not shown). In Norwegians when HLA-DQA1*05:01:01:02 was absent HLA-B*08:01:01:01 remained the strongest risk factor (OR = 3.72, CI = 1.69–7.78, P-adj = 6.40E−04). Removal of HLA-B*08:01:01:01 did not yield any significant associations after adjustment for multiple testing. In the Italian cohort, alleles of the HLA-DR16 class II region (HLA-DRB1*16:01:01~HLA-DRB5*02:02~HLA-DQA1*01:02:02~HLA-DQB1*05:02:01) had a strong effect on AChR-EOMG risk (OR ~2.0), however, only HLA-DRB1*16:01:01 (average OR = 2.85, P-adj <0.036) and HLA-DQA1*01:02:02 (average OR = 2.58, P-adj <0.044) in the absence of HLA-B*08:01:01:01 and HLA-DQA1*05:01:01:02 were statistically significant when adjusted for multiple alleles. In Swedes, no significant associations were observed following correction for multiple comparisons.
HLA-DRB1*13.1 and HLA-DRB1*07 Haplotypes Are Protective Against AChR-EOMG
Highly protective haplotype blocks in Italians include and HLA-DRB1*13:01:01:01SG~HLA-DQB1*06:03:01 and HLA-DQA1*01:03:01:02~HLA-DQB1*06:03:01 (OR = 0.23, CI = 0.03–0.92, P = 0.028). These haplotype blocks were also highly protective in AChR-EOMG Norwegian patients (OR = 0.22, CI = 0.06–0.61, P = 0.002 and 1.63E−03) as well as HLA-DRB1*13:01:01:01~HLA-DQA1*01:03:01:02, and HLA-DRB3*01:01:02:01/02~HLA-DRB1*13:01:01:01SG~HLA-DQA1*01:03:01:02~HLA-DQB1*06:03:01 which was strongly protective (OR = 0.11, CI = 0.00–0.65, P = 7.48E−03). Collectively these haplotype blocks comprise the extended class II HLA-DRB1*13:01:01:01SG haplotype (abbreviated here as HLA-DRB1*13.1). HLA-DRB*13.1 has been shown to confer protection for several autoimmune diseases such as rheumatoid arthritis and systemic lupus erythematosus, although the negative association with MG is not well established (37). However, it is noteworthy that HLA-DR7 haplotype-blocks were also strongly protective in Norwegians, with OR values similar to those seen for the HLA-DRB1*13 haplotype-blocks. Specifically, haplotype-blocks which lie on the non-expressed HLA-DRB1*07:01:01:01SG~HLA-DRB4*01:03:01:02N~HLA-DQB1*03:03:02:01 were protective, whereas the expressed HLA-DR7~HLA-DRB4 were not associated with protection. HLA-DR7 was also found to be associated with protection in Italians but unlike Norwegians the expressed HLA-DRB4 haplotype, as deduced from the HLA-DQB1 allele, was protective.
Surprisingly, the HLA-DRB1*13.1 haplotype was not associated with AChR-EOMG in the Swedish cohort. Instead the HLA*DRB1*07:01~HLA-DQB1*02:01 haplotype was strongly protective (OR = 0.24, CI = 0.05–0.72, P = 8.07E−03), and the HLA-DRB1*01:01~HLA-DQB1*05:01 was moderately protective. At the allele-level only HLA-DRB1*07:01 had the strongest protective effect (OR = 0.33, CI = 0.12–0.74, P = 5.15E−03).
Locus-Level Heterogeneity in AChR-LOMG Patients
Locus-level heterogeneity results for the AChR-LOMG subtype for three cohorts are shown in Supplementary Table 3. In the Italian cohort HLA-DRB1 remained significant after adjustment for multiple comparisons. For the Norwegian group HLA-DRB1, HLA-DQA1, HLA-DQB1, HLA-DRB1~HLA-DQB1, and HLA-DPA1~HLA-DPB1 were significant. In the Swedish group HLA-DRB1, HLA-DQB1, HLA-C~HLA-B, and HLA-DRB1~HLA-DQB1 were significant.
HLA-DRB*15.1 and HLA-DRB1*07 Are Risk Factors for AChR-LOMG Patients
In all three cohorts’ alleles and haplotype blocks of the HLA-DR15~HLA-DR51~HLA-DQA1~HLA-DQB1 extended haplotype were positively associated with AChR-LOMG (Tables 5–7). However the effect sizes were significantly greater in the Italians with OR’s ranging from 3.26 to 3.56, compared to Norwegians; ORs ranging from 1.57 for HLA-C*07:02:01:03 to 3.23 for the extended haplotype encompassing both class I and II regions, and Swedes; OR = 1.54 for HLA-B*07:02 and largest OR of 2.30 for the extended haplotype.
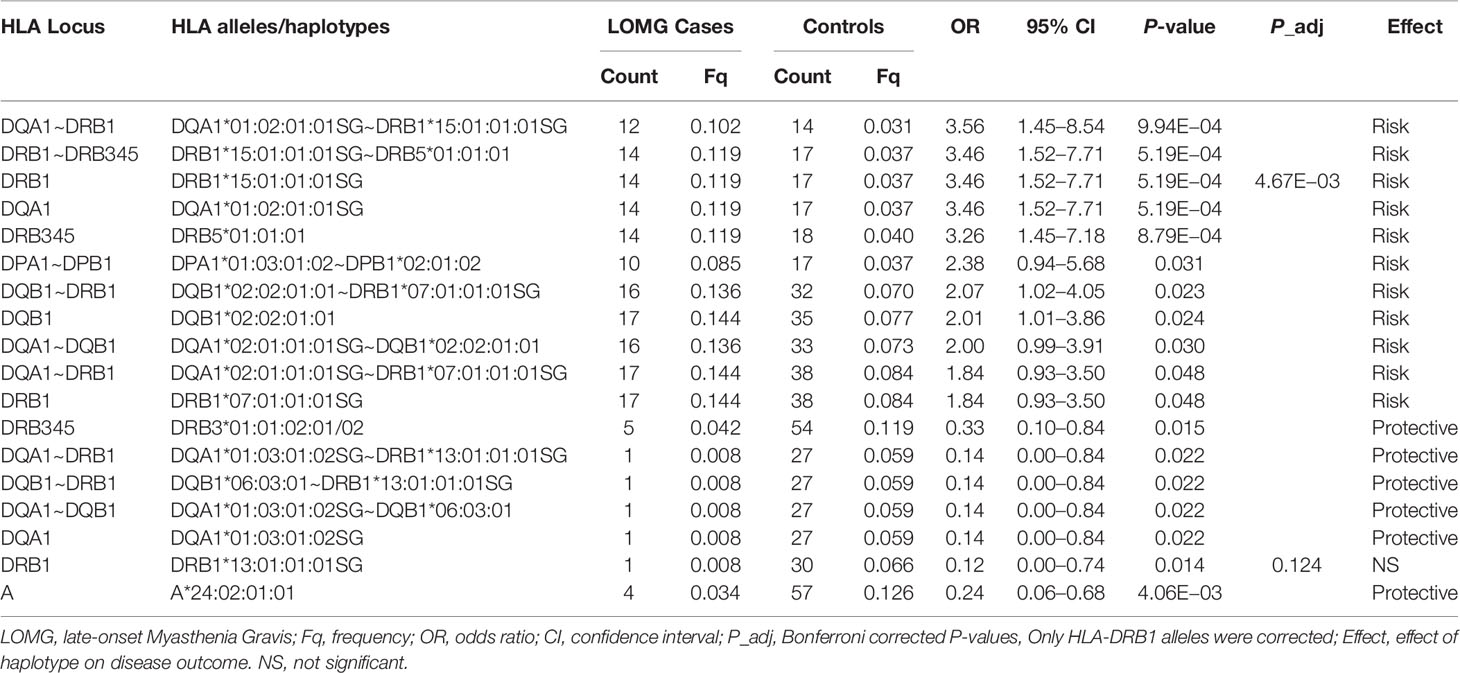
Table 5 Significant associations of HLA alleles and haplotypes in Italian LOMG AChR-antibody positive cases and controls ranked by odds ratios.
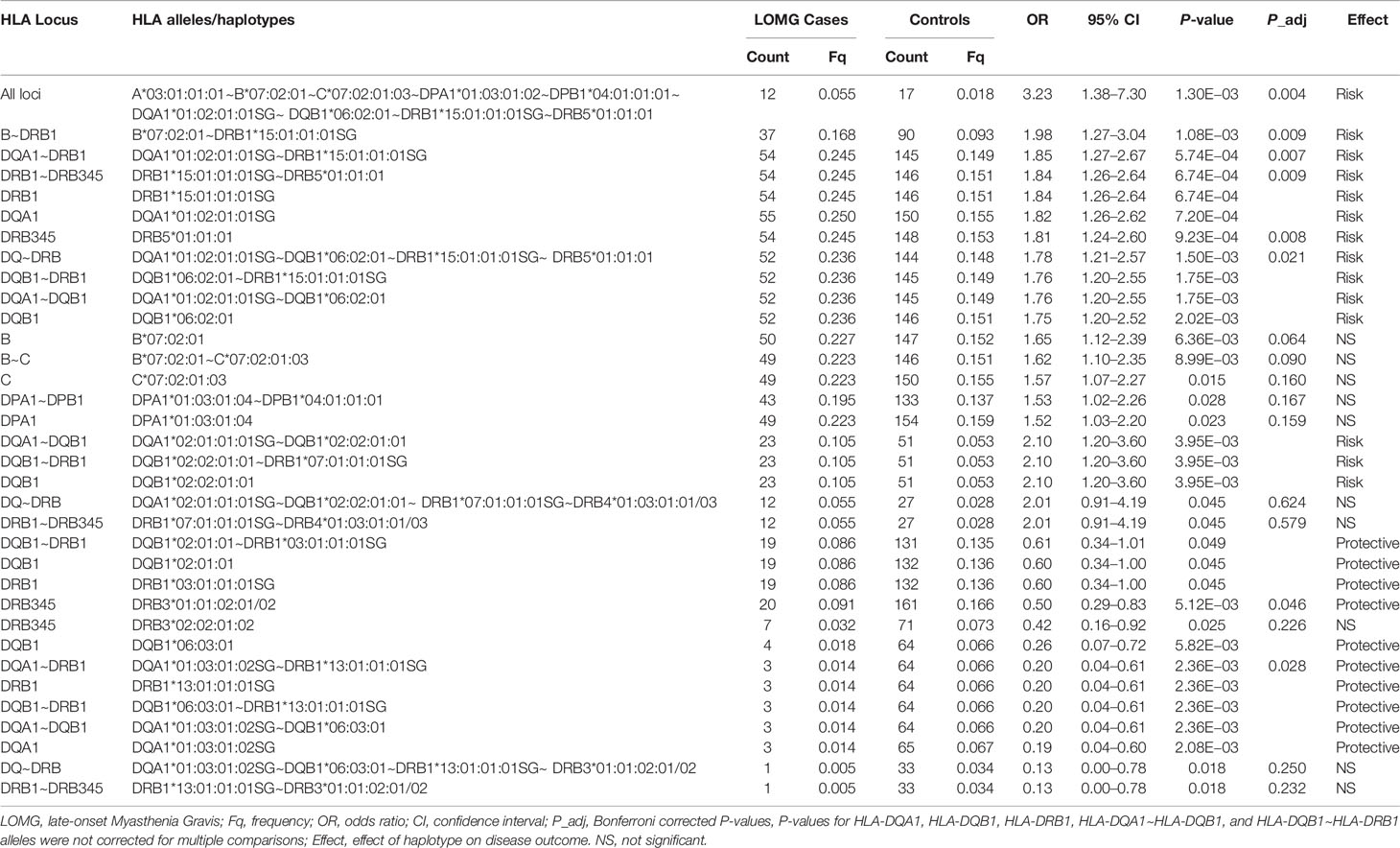
Table 6 Significant associations of HLA alleles and haplotypes in Norwegian LOMG AChR-antibody positive cases and controls ranked by odds ratios.
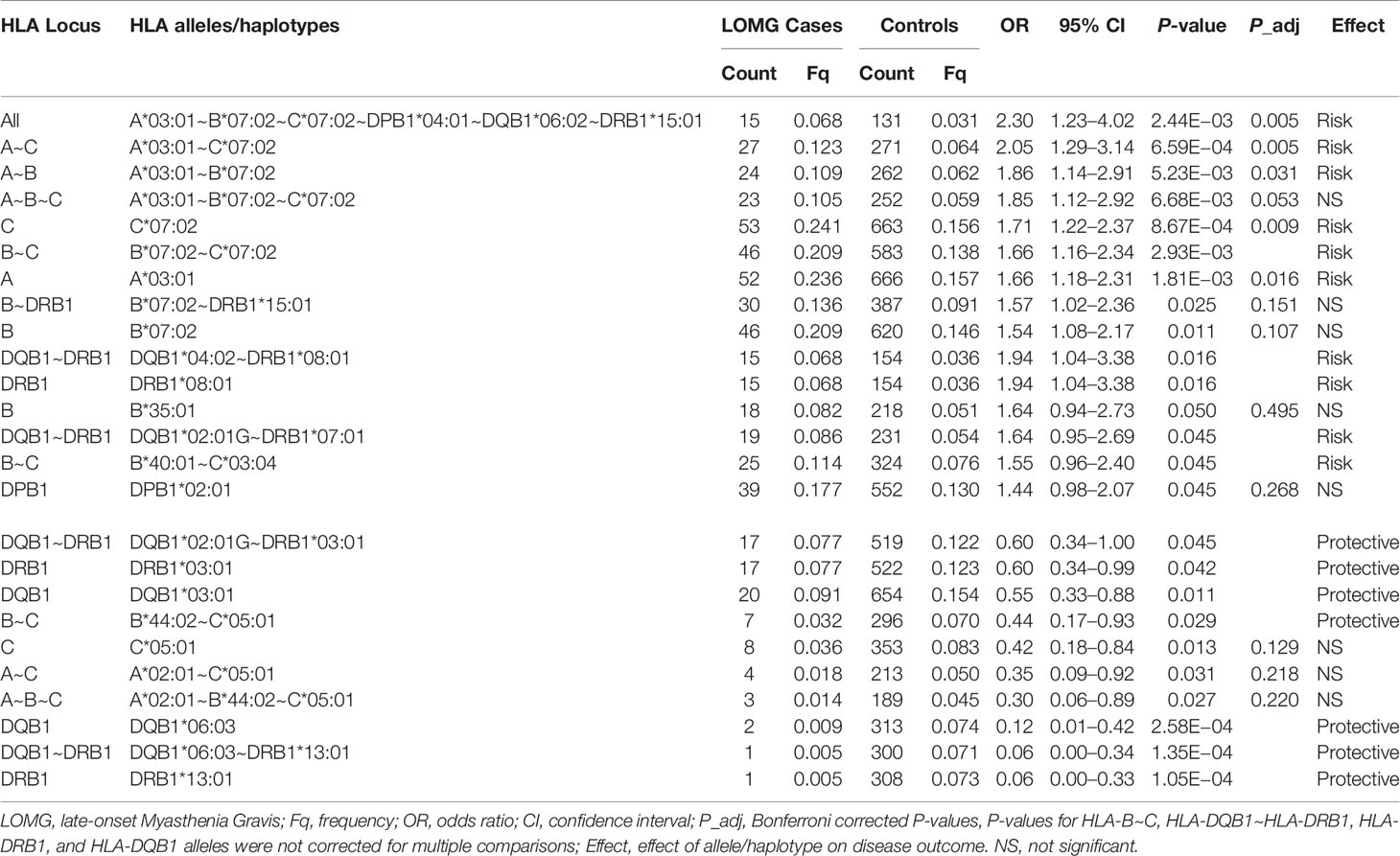
Table 7 Significant associations of HLA alleles and haplotypes in Swedish LOMG AChR-antibody positive cases and controls ranked by odds ratios.
The association of HLA-DRB1*15:01 with LOMG risk has been previously reported (26). However, in this study we find that HLA-DQA1*01:02:01:01 has an equivalent strength of association as HLA-DRB1*15:01:01:01SG for AChR-LOMG risk in Italians (OR = 3.46, CI = 1.52–7.71, P = 5.19E−04) and in Norwegians very similar effect sizes were found (HLA-DRB1*15:01:01:01, OR = 1.84, CI = 1.26–2.64, P = 6.74E−04; HLA-DQA1*01:02:01:01, OR = 1.82, CI = 1.26–2.62, P = 7.20E−04). Furthermore, at the haplotypic-level in the Italian cohort, HLA-DQA1*01:02:01:01SG~HLA-DRB1*15:01:01:01SG (D’ = 0.89) was more strongly associated than the ‘fixed’ HLA-DRB1*15:01:01:01~HLA-DRB5*01:01:01 haplotype where D’ = 1. These results indicate that both alleles are the strongest determinants of AChR-LOMG risk. In Norwegians a similar pattern was observed (Table 6).
Also it is noteworthy that the HLA-DQA1*02:01:01:01SG~HLA-DQB1*02:02:01:01, which had the second largest effect size for risk outside of the HLA-DRB*15 haplotype in the Norwegian cohort (OR = 2.10, CI = 1.20–3.60, P = 3.95E−03) and a similar effect size in Italians (OR = 2.00, CI = 0.99–3.91, P = 0.030) forms part of the HLA-DRB1*07:01:01:01SG~HLA-DRB4*01 expressed haplotype block. These results suggest that the HLA-DQ region of the HLA-DR7 haplotype is an additional risk factor for AChR-LOMG.
Restricting the dataset to individuals without alleles located on the HLA-DR15 class II haplotype lends further support to the involvement of HLA-DR7 alleles as secondary risk factors in AChR-LOMG. In the Norwegian group HLA-DQB1*02:02:01:01 (OR = 2.7, P = 5.0E−04) and HLA-DRB1*07:01:01:01SG (OR = 1.98, P = 8.4E−03) were significantly associated with susceptibility in the absence of both, HLA-DQA1*01:02:01:01SG and HLA-DRB1*15:01:01:01SG alleles (data not shown). Finally, in the stratification analyses of Italians these alleles were positively associated with AChR-LOMG but only the adjusted HLA-DQB1*02:02:01:01 P-value were significant (OR = 2.39, CI = 1.17 to 4.71, P = 0.046).
As found for the AChR-EOMG cohort, strongly protective alleles and haplotypes were observed at the HLA-DRB1*13:01:01:01SG class II region haplotype in all three populations. Somewhat surprisingly, the HLA-DRB1*03:01~HLA-DQB1*02:01 haplotype block, which was a susceptibility factor for AChR-EOMG, was found to be weakly protective for AChR-LOMG Swedish patients; OR = 0.60, CI = 0.34–1.00, P = 0.045.
Generalized and Ocular MG Subsets
We examined whether the allele distribution varied with generalized and ocular subsets within the aforementioned EOMG and LOMG non-thymomatous AChR positive antibody datasets. In the generalized subset similar associations were observed as described above. For both EOMG and LOMG ocular tests, no significant associations were found following adjustment for multiple testing.
Discussion
We provide a detailed MG association analyses data derived from high-depth sequencing of complete HLA genes (HLA-A, HLA-C, HLA-B, HLA-DQA1, HLA-DPA1) and wide coverage of genomic regions (HLA-DRB1, HLA-DRB3/4/5, HLA-DQB1, HLA-DPB1). In contrast to previous reports of HLA associations with MG, which have often utilized serological and low to mid-resolution molecular typing methods, we evaluated individuals from three European populations in a single study and utilized next-generation sequencing to capture variation in intronic and untranslated regions.
Our results have extended and refined previous observations of HLA alleles with MG risk. Consistent with previous HLA MG association studies conducted in European populations (20, 22, 26, 38), we showed that the ancestral haplotype 8.1 most common in Northern European populations is associated with AChR-EOMG risk in Italian, Norwegian, and Swedish cohorts. However, in the Italian and Norwegian cohorts, who were examined at maximum HLA allelic resolution, we successfully sequenced the intronic and UTR variants for most alleles composing the AH8.1 haplotype. This is clearly illustrated when we consider the dominant AChR-EOMG risk allele HLA-B*08:01 which according to the IMGT/HLA Database release 3.250 has five 3-field variants (alleles that differ by synonymous mutations and are translated into the same protein), and two 4-field variants named HLA-B*08:01:01:01 and HLA-B*08:01:01:02 that are distinguishable due to a single SNP T > C at 2,802 bp in the 3’ UTR of the HLA-B gene. In addition, we showed conclusively that the frequency of HLA-B*01:01:01:01 was over represented in Italian and Norwegian AChR-EOMG patients compared to controls; in fact HLA-B*08:01:01:02 occurs at much lower frequencies (AF ~0.005, n = 1 or 2) in the Italian group regardless of disease status, and was not observed at all in Norwegians. Although not shown, HLA-B*01:01:01:02 was not observed in the Swedish AChR-EOMG patient cohort therefore HLA-B*08:01:01:01 is also the risk allele in this group. Furthermore, we show that HLA-B*08:01:01:01 is almost exclusively linked to HLA-C*07:01:01:01 (D’ >0.95) of which there are five other 4-field variants that differ due to various SNPs located within introns. These observations also extend to the class II region of the AH8.1 haplotype; HLA-DQA1*05:01:01:02 was identified as the risk allele whereas HLA-DQA1*05:01:01:01 and HLA-DQA1*05:01:01:03 are neutral. Due to the presence of a prominent low-complexity sequence region near the 5’ end of intron 2 in the HLA-DRB loci and unsequenced regions it was not possible to define the 4-field variant of HLA-DRB1*03:01:01 and HLA-DRB3*01:01:02. We note that increased allelic resolution has decreased the statistical power of the association analyses by increasing the number of categories. We observed this event when we compare the 4-field association results to the 2-field data where overall the effect size is greater and P-values smaller. The downside is that lower resolution typing pools a large number of HLA alleles together and therefore masks the effects of individual yet pertinent alleles. One of the main take home messages from this study is that specific intronic and UTR variants of HLA alleles contribute to MG pathogenesis.
Since the AH8.1 haplotype is most frequent and highly conserved in Europeans it is not too surprising that alleles of this haplotype and extended regions have been found to be associated with several immune system dysfunctions including chronic inflammation and autoimmune diseases such as Type I diabetes (39) and systemic lupus erythematosus (40). Autoimmunity is thought, in part, to result from the downregulation of type II cytokine responses, which is influenced by the interaction of alleles on AH8.1, leading to enhanced humoral responses and increased production of autoantibodies (41). On the other hand, the stability of the AH8.1 haplotype, and relatively low recombination rate across the haplotype suggests that this haplotype is positively selected and has a survival advantage. The AH8.1 contributes to EOMG in European populations (26, 38, 42) but not populations from East Asia where HLA-DRB1*09:01 is the main risk allele for EOMG (43, 44). The numerous studies conducted in Europeans have shown that HLA-B*08:01 appears to be the dominant risk allele for EOMG and the other associated alleles located on AH8.1 are simply a consequence of strong LD between them and HLA*08:01. In this present study, this notion appears to be the case when we only consider the reduced 2-field data for all three groups; HLA-B*08:01 has the strongest effect. However, at the 4-field resolution HLA-DQA1*05:01:01:02 has a similar effect size to HLA-B*08:01:01:01 for risk in Italian patients. This finding is of interest as to the best of our knowledge the HLA-DQA1*05:01:01 allele has never been investigated in previous MG association studies.
We identified additional risk factors for EOMG that appeared to be ethnic specific such as the HLA-DRB1*16:01:01 class II haplotype-blocks in Italians, and the HLA-DRB1*13:02:01 haplotype-blocks encompassing class I and II alleles in Norwegians. These findings are novel and should be confirmed and further examined to refine the SNPs associated with EOMG in these European populations.
We also confirmed previous findings of a protective effect conferred by the HLA-DRB1*13:01:01:01SG class II allele and haplotype in both AChR-EOMG and AChR-LOMG patients from Italy, Norway, and Sweden. HLA-DRB1*13:01:01:01SG has a frequency of ~0.05 in several European populations (unpublished observations). The protective effect of the HLA-DRB1*13:01 allele is not exclusive to MG but has also been found to be negatively associated with a plethora of autoimmune diseases in Europeans, and Asians (37, 45). It has been hypothesized that the protective effect of HLA-DRB1*13:01 is due to the high binding affinity/avidity of T-cell receptors on autoreactive regulatory T-cells (Tregs) for HLA-DRB1*13:01 molecules. Consequently, thymic negative selection and autoreactive Tregs development are enhanced resulting in decreased pathogenicity (41).
A novel EOMG protective allele HLA-DRB1*07:01 was detected in all three cohorts. Specifically, the HLA-DRB1*07:01:01:01SG~HLA-DRB4*01:03:01:02N~HLA-DQA1*02:01:01:01SG~HLA-DQB1*03:03:02:01 class II extended haplotype and the 2-loci HLA-DRB1~HLA-DQB1, HLA-DQA1-DQB1 blocks of this haplotype were equally strongly protective (OR = 0.11) in the Norwegian cohort. Due to strong LD across this region and similarity of effect sizes we cannot easily discern which allele is causative from the haplotype association analyses. HLA-DRB1*07:01:01:01SG of the expressed HLA-DR7 haplotype also occurred at higher allele frequencies in Italian control subjects (AF = 0.084) compared to AChR-EOMG patients (AF = 0.028). HLA-DRB1*07:01 has been found to be associated with protection in systemic sclerosis in European origin individuals (46). The amino acid differences between the protective alleles HLA-DRB1*13:01 and HLA-DRB1*07:01 are substantial, therefore they are highly likely to present different repertoires of peptides suggesting that the immunological mechanisms underlying protection differs. Interestingly, we find that the HLA-DRB1*07:01:01:01SG negatively associated in EOMG has the opposite predisposing effect in LOMG, further underscoring the fact that they are distinct subtypes of MG with different immunogenetic backgrounds.
LOMG risk has been shown to be positively associated with the HLA-DRB1*15:01 allele in a Norwegian population (26), in this study we replicate and extrapolate those findings. Specifically, we find that the extended haplotype, HLA-A*03:01:01:01~HLA-C*07:02:01:03~ HLA-B*07:02:01~HLA-DRB1*15:01:01:01SG~HLA-DRB5*01:01:01~HLA-DQA1*01:02:01:01SG~HLA-DQB1*06:02:01~HLA-DPA1*01:03:01:02~HLA-DPB1*04:01:01:01 strongly predisposes to risk (OR = 3.23), in which the associations of the HLA-DP loci reflect LD with the susceptible HLA-DR-HLA-DQ haplotype. The effect of the extended haplotype was substantially greater than the alleles and the various two to three loci haplotype blocks associated with risk. These findings allude to a synergistic risk effect of the HLA-DR15 alleles, although the independent effects of HLA-DRB1*15:01:01:01SG, HLA-DRB5*01:01:01, and HLA-DQA1*01:02:01:01 were the only alleles statistically significant after adjustment for multiple alleles. We observed a similar HLA-DR15 distribution pattern in Italian AChR-LOMG patients, however the strongest haplotype associated was HLA-DRB1*15:01:01:01SG~HLA-DQA1*01:02:01:01SG (OR = 3.56). The sample size of the Norwegian AChR-LOMG cohort is larger than the Italian counterparts, thus this could account for the slight discrepancy in results. Interestingly, in Italians and Norwegians the strongest signal for susceptibility resided in the class II component of the HLA-DR15 haplotype, whereas in Swedes with the exception of the extended haplotype the strongest effect was seen in the class I region of the HLA-DR15 haplotype. These findings indicate that it is difficult to map the susceptible factor based on HLA alleles, and further studies are needed to identify the causative SNP(s).
In conclusion, this study provides additional evidence that specific HLA alleles and haplotypes play essential roles in the pathogenesis of different subtypes of MG. Distinctive HLA factors for susceptibility and resistance were found in EOMG and LOMG, and open avenues for investigating the mechanisms for these distinct diseases. The extensive LD within the HLA region requires that populations of non-European background should be investigated to distinguish between primary and secondary effects. Due to the location of many of the causative polymorphisms in non-coding regions that could affect expression functional assays of candidate genes are optimal to further understand MG pathogenesis. A deeper understanding of the biological mechanisms underlying MG and could offer new insights for diagnostic approaches and the development of novel therapies.
Data Availability Statement
The datasets presented in this study can be found in online repositories. The names of the repository/repositories and accession number(s) can be found below: (www.immport.org/shared/home), ImmPort/.
Author Contributions
LC was involved in the conception and design of the study, performed the statistical analyses, interpreted the results, and wrote the manuscript. MF-V and JO were involved in the conception and design of the study, contributed to the interpretation of the results, and contributed to the final version of the manuscript. JH contributed to the conception of the study and interpretation of the results. PC, RF, BL, HH, SB, JO, PB, AH, LH, and RM contributed to collection of samples and clinical data. SC was responsible for sample and data collection at the UCSF-DNA bank. LC and SG performed NGS genotyping assays. MB contributed genotyping data. All authors contributed to the article and approved the submitted version.
Funding
This work was supported by grant U19NS095774 (JO and MF-V) from the U.S. National Institutes of Health (NIH). The UCSF DNA biorepository is supported by RG-1611-26299 from the National Multiple Sclerosis Society.
Conflict of Interest
The authors declare that the research was conducted in the absence of any commercial or financial relationships that could be construed as a potential conflict of interest.
Acknowledgments
This manuscript is dedicated to the memory of Pia Bernasconi, a dedicated scientist and generous colleague. We are grateful to the individuals who participated in this study and to Ms. R. Guerrero, Mr. N. Lee, and Dr. Elisa Faggiani for sample processing. We also thank Mrs. Romina Gibertoni and the Italian Association of Myasthenia Gravis (AIM—Associazione Italiana Miastenia e Malattie Immunodegenerative—Amici del Besta Onlus, Milan, Italy) for the kind support to MG patients’ recruitment, Dr. Fernando Ravagnani and Dr. Carmela Guarino of the Immunohematology and Transfusion Medicine Service of the Fondazione I.R.C.C.S. Istituto Nazionale dei Tumori (Milan, Italy) for collection of Italian healthy donor blood samples, and Dr. Carlo Antozzi and Mrs. Silvia Gandini for clinical data collection.
Supplementary Material
The Supplementary Material for this article can be found online at: https://www.frontiersin.org/articles/10.3389/fimmu.2021.667336/full#supplementary-material
Abbreviations
MG, myasthenia gravis; HLA, human leukocyte antigen; NGS, next-generation sequencing; EOMG, early onset myasthenia gravis; LOMG, late onset myasthenia gravis; AChR, acetylcholine receptor.
References
1. Gilhus NE, Tzartos S, Evoli A, Palace J, Burns TM, Verschuuren JJGM. Myasthenia Gravis. Nat Rev Dis Prim (2019) 5:30. doi: 10.1038/s41572-019-0079-y
3. Gilhus NE, Verschuuren JJ. Myasthenia Gravis: Subgroup Classification and Therapeutic Strategies. Lancet Neurol (2015) 14:1023–36. doi: 10.1016/S1474-4422(15)00145-3
4. Carr AS, Cardwell CR, McCarron PO, McConville J. A Systematic Review of Population Based Epidemiological Studies in Myasthenia Gravis. BMC Neurol (2010) 10:46. doi: 10.1186/1471-2377-10-46
5. Gilhus NE, Skeie GO, Romi F, Lazaridis K, Zisimopoulou P, Tzartos S. Myasthenia Gravis — Autoantibody Characteristics and Their Implications for Therapy. Nat Rev Neurol (2016) 12:259–68. doi: 10.1038/nrneurol.2016.44
6. Romi F, Hong Y, Gilhus NE. Pathophysiology and Immunological Profile of Myasthenia Gravis and Its Subgroups. Curr Opin Immunol (2017) 49:9–13. doi: 10.1016/J.COI.2017.07.006
7. Berrih-Aknin S, Le Panse R. Myasthenia Gravis: A Comprehensive Review of Immune Dysregulation and Etiological Mechanisms. J Autoimmun (2014) 52:90–100. doi: 10.1016/j.jaut.2013.12.011
8. Tüzün E, Christadoss P. Complement Associated Pathogenic Mechanisms in Myasthenia Gravis. Autoimmun Rev (2013) 12:904–11. doi: 10.1016/J.AUTREV.2013.03.003
9. Zisimopoulou P, Evangelakou P, Tzartos J, Lazaridis K, Zouvelou V, Mantegazza R, et al. A Comprehensive Analysis of the Epidemiology and Clinical Characteristics of Anti-LRP4 in Myasthenia Gravis. J Autoimmun (2014) 52:139–45. doi: 10.1016/J.JAUT.2013.12.004
10. Hong Y, Zisimopoulou P, Trakas N, Karagiorgou K, Stergiou C, Skeie GO, et al. Multiple Antibody Detection in ‘Seronegative’ Myasthenia Gravis Patients. Eur J Neurol (2017) 24:844–50. doi: 10.1111/ene.13300
11. Andersen JB, Heldal AT, Engeland A, Gilhus NE. Myasthenia Gravis Epidemiology in a National Cohort; Combining Multiple Disease Registries. Acta Neurol Scand (2014) 129:26–31. doi: 10.1111/ane.12233
12. Liu F-C, Kuo C-F, See L-C, Tsai H-I, Yu H-P. Familial Aggregation of Myasthenia Gravis in Affected Families: A Population-Based Study. Clin Epidemiol (2017) 9:527–35. doi: 10.2147/CLEP.S146617
13. Salvado M, Canela M, Ponseti JM, Lorenzo L, Garcia C, Cazorla S, et al. Study of the Prevalence of Familial Autoimmune Myasthenia Gravis in a Spanish Cohort. J Neurol Sci (2016) 360:110–4. doi: 10.1016/J.JNS.2015.11.049
14. Maselli RA, Arredondo J, Nguyen J, Lara M, Ng F, Ngo M, et al. Exome Sequencing Detection of Two Untranslated. GFPT1 Mutations Family Limb-Girdle Myasthenia Clin Genet (2014) 85:166–71. doi: 10.1111/cge.12118
15. Chen Y, Wang W, Wei D, Yang L. Three Cases of Myasthenia Gravis From One Family With Variations in Clinical Features and Serum Antibodies. Neuromuscul Disord (2012) 22:286–8. doi: 10.1016/J.NMD.2011.10.004
16. Corda D, Deiana GA, Mulargia M, Pirastru MI, Serra M, Piluzza MG, et al. Familial Autoimmune MuSK Positive Myasthenia Gravis. J Neurol (2011) 258:1559–60. doi: 10.1007/s00415-011-5964-6
17. Ramanujam R, Pirskanen R, Ramanujam S, Hammarström L. Utilizing Twins Concordance Rates to Infer the Predisposition to Myasthenia Gravis. Twin Res Hum Genet (2011) 14:129–36. doi: 10.1375/twin.14.2.129
18. Fritze D, Naeim F, Herrman C, Smith G, Walford R. Hl-a ANTIGENS in MYASTHENIA Gravis. Lancet (1974) 303:240–3. doi: 10.1016/S0140-6736(74)92548-3
19. Gambino CM, Aiello A, Accardi G, Caruso C, Candore G. Autoimmune Diseases and 8.1 Ancestral Haplotype: An Update. HLA (2018) 92:137–43. doi: 10.1111/tan.13305
20. Vandiedonck C, Beaurain G, Giraud M, Hue-Beauvais C, Eymard B, Tranchant C, et al. Pleiotropic Effects of the 8.1 HLA Haplotype in Patients With Autoimmune Myasthenia Gravis and Thymus Hyperplasia. Proc Natl Acad Sci (2004) 101:15464–9. doi: 10.1073/pnas.0406756101
21. Giraud M, Beaurain G, Eymard B, Tranchant C, Gajdos P, Garchon H-J. Genetic Control of Autoantibody Expression in Autoimmune Myasthenia Gravis: Role of the Self-Antigen and of HLA-linked Loci. Genes Immun (2004) 5:398–404. doi: 10.1038/sj.gene.6364110
22. Janer M, Cowland A, Picard J, Campbell D, Pontarotti P, Newsom-Davis J, et al. A Susceptibility Region for Myasthenia Gravis Extending Into the HLA-Class I Sector Telomeric to HLA-C. Hum Immunol (1999) 60:909–17. doi: 10.1016/S0198-8859(99)00062-2
23. Fang F, Sveinsson O, Thormar G, Granqvist M, Askling J, Lundberg IE, et al. The Autoimmune Spectrum of Myasthenia Gravis: A Swedish Population-Based Study. J Intern Med (2015) 277:594–604. doi: 10.1111/joim.12310
24. Varade J, Wang N, Lim CK, Zhang T, Zhang Y, Liu X, et al. Novel Genetic Loci Associated HLA-B*08:01 Positive Myasthenia Gravis. J Autoimmun (2018) 88:43–9. doi: 10.1016/J.JAUT.2017.10.002
25. Seldin MF, Alkhairy OK, Lee AT, Lamb JA, Sussman J, Pirskanen-Matell R, et al. Genome-Wide Association Study of Late-Onset Myasthenia Gravis: Confirmation of TNFRSF11A and Identification of ZBTB10 and Three Distinct HLA Associations. Mol Med (2016) 21:769–81. doi: 10.2119/molmed.2015.00232
26. Maniaol AH, Elsais A, Lorentzen ÅR, Owe JF, Viken MK, Sæther H, et al. Late Onset Myasthenia Gravis Is Associated With HLA DRB1*15:01 in the Norwegian Population. PloS One (2012) 7:e36603. doi: 10.1371/journal.pone.0036603
27. Massa R, Greco G, Testi M, Rastelli E, Terracciano C, Frezza E, et al. Thymomatous Myasthenia Gravis: Novel Association With HLA DQB1*05:01 and Strengthened Evidence of High Clinical and Serological Severity. J Neurol (2019) 266:982–9. doi: 10.1007/s00415-019-09225-z
28. Creary LE, Gangavarapu S, Mallempati KC, Montero-Martín G, Caillier SJ, Santaniello A, et al. Next-Generation Sequencing Reveals New Information About HLA Allele and Haplotype Diversity in a Large European American Population. Hum Immunol (2019) 80(10):807–22. doi: 10.1016/J.HUMIMM.2019.07.275
29. Creary LE, Guerra SG, Chong W, Brown CJ, Turner TR, Robinson J, et al. Next-Generation HLA Typing of 382 International Histocompatibility Working Group Reference B-Lymphoblastoid Cell Lines: Report From the 17th International HLA and Immunogenetics Workshop. Hum Immunol (2019) 80(7):449–60. doi: 10.1016/J.HUMIMM.2019.03.001
30. Creary LE, Mallempati KC, Gangavarapu S, Caillier SJ, Oksenberg JR, Fernández-Viňa MA. Deconstruction of HLA-DRB1*04:01:01 and HLA-DRB1*15:01:01 Class II Haplotypes Using Next-Generation Sequencing in European-Americans With Multiple Sclerosis. Mult Scler J (2018) 25(6):772–82. doi: 10.1177/1352458518770019 135245851877001.
31. Hollenbach JA, Norman PJ, Creary LE, Damotte V, Montero-Martin G, Caillier S, et al. A Specific Amino Acid Motif of HLA-DRB1 Mediates Risk and Interacts With Smoking History in Parkinson’s Disease. Proc Natl Acad Sci USA (2019) 116:7419–24. doi: 10.1073/pnas.1821778116
32. Pappas DJ, Marin W, Hollenbach JA, Mack SJ. Bridging ImmunoGenomic Data Analysis Workflow Gaps (Bigdawg): An Integrated Case-Control Analysis Pipeline. Hum Immunol (2016) 77:283–7. doi: 10.1016/j.humimm.2015.12.006
33. Hedrick PW. Gametic Disequilibrium Measures: Proceed With Caution. Genetics (1987) 117:331–41. doi: 10.1093/genetics/117.2.331
34. Cramer H. Mathematical Methods of Statistics. I., Ed. H. Cramer Princeton: Princeton University (1946).
35. Lancaster AK, Single RM, Solberg OD, Nelson MP, Thomson G. PyPop Update–a Software Pipeline for Large-Scale Multilocus Population Genomics. Tissue Antigens (2007) 69(Suppl 1):192–7. doi: 10.1111/j.1399-0039.2006.00769.x
36. Guo SW, Thompson EA. Performing the Exact Test of Hardy-Weinberg Proportion for Multiple Alleles. Biometrics (1992) 48:361–72. doi: 10.2307/2532296
37. Bettencourt A, Carvalho C, Leal B, Brás S, Lopes D, Martins da Silva A, et al. The Protective Role of HLA-DRB1(∗)13 in Autoimmune Diseases. J Immunol Res (2015) 2015:948723. doi: 10.1155/2015/948723
38. Saruhan-Direskeneli G, Hughes T, Yilmaz V, Durmus H, Adler A, Alahgholi-Hajibehzad M, et al. Genetic Heterogeneity Within the HLA Region in Three Distinct Clinical Subgroups of Myasthenia Gravis. Clin Immunol (2016) 166–167:81–8. doi: 10.1016/j.clim.2016.05.003
39. Noble JA, Valdes AM. Genetics of the HLA Region in the Prediction of Type 1 Diabetes. Curr Diabetes Rep (2011) 11:533–42. doi: 10.1007/s11892-011-0223-x
40. Wong M, Tsao BP. Current Topics in Human SLE Genetics. Springer Semin Immunopathol (2006) 28:97–107. doi: 10.1007/s00281-006-0031-6
41. Tsai S, Santamaria P. Mhc Class Ii Polymorphisms, Autoreactive T-Cells, and Autoimmunity. Front Immunol (2013) 4:321. doi: 10.3389/fimmu.2013.00321
42. Santos E, Bettencourt A, da Silva AM, Boleixa D, Lopes D, Brás S, et al. HLA and Age of Onset in Myasthenia Gravis. Neuromuscul Disord (2017) 27:650–4. doi: 10.1016/J.NMD.2017.04.002
43. Xie Y, Qu Y, Sun L, Li H, Zhang H, Shi H, et al. Association Between HLA-DRB1 and Myasthenia Gravis in a Northern Han Chinese Population. J Clin Neurosci (2011) 18:1524–7. doi: 10.1016/J.JOCN.2011.05.002
44. Feng H, Yang L, Liu W, Huang X, Qiu L, Li Y. The HLA-B*4601-DRB1*0901 Haplotype Is Positively Correlated With Juvenile Ocular Myasthenia Gravis in a Southern Chinese Han Population. Neurol Sci (2015) 36:1135–40. doi: 10.1007/s10072-015-2235-9
45. Furukawa H, Oka S, Tsuchiya N, Shimada K, Hashimoto A, Tohma S, et al. The Role of Common Protective Alleles HLA-DRB1*13 Among Systemic Autoimmune Diseases. Genes Immun (2017) 18:1–7. doi: 10.1038/gene.2016.40
46. Arnett FC, Gourh P, Shete S, Ahn CW, Honey RE, Agarwal SK, et al. Major Histocompatibility Complex (MHC) Class II Alleles, Haplotypes and Epitopes Which Confer Susceptibility or Protection in Systemic Sclerosis: Analyses in 1300 Caucasian, African-American and Hispanic Cases and 1000 Controls. Ann Rheum Dis (2010) 69:822–7. doi: 10.1136/ard.2009.111906
Keywords: myasthenia gravis, human leukocyte antigen, next-generation sequencing, European, susceptibility, protection
Citation: Creary LE, Gangavarapu S, Caillier SJ, Cavalcante P, Frangiamore R, Lie BA, Bengtsson M, Harbo HF, Brauner S, Hollenbach JA, Oksenberg JR, Bernasconi P, Maniaol AH, Hammarström L, Mantegazza R and Fernández-Viña MA (2021) Next-Generation Sequencing Identifies Extended HLA Class I and II Haplotypes Associated With Early-Onset and Late-Onset Myasthenia Gravis in Italian, Norwegian, and Swedish Populations. Front. Immunol. 12:667336. doi: 10.3389/fimmu.2021.667336
Received: 12 February 2021; Accepted: 27 April 2021;
Published: 07 June 2021.
Edited by:
Kutty Selva Nandakumar, Southern Medical University, ChinaReviewed by:
Jeannine M. Heckmann, University of Cape Town, South AfricaHenry Kaminski, George Washington University, United States
Copyright © 2021 Creary, Gangavarapu, Caillier, Cavalcante, Frangiamore, Lie, Bengtsson, Harbo, Brauner, Hollenbach, Oksenberg, Bernasconi, Maniaol, Hammarström, Mantegazza and Fernández-Viña. This is an open-access article distributed under the terms of the Creative Commons Attribution License (CC BY). The use, distribution or reproduction in other forums is permitted, provided the original author(s) and the copyright owner(s) are credited and that the original publication in this journal is cited, in accordance with accepted academic practice. No use, distribution or reproduction is permitted which does not comply with these terms.
*Correspondence: Lisa E. Creary, lcreary@stanford.edu; Marcelo A. Fernández-Viña, marcelof@stanford.edu