- 1Department of Research and Development, Gentera Biotechnology, Istanbul, Turkey
- 2Department of Paediatrics, Division of Paediatric Rheumatology, Schulich School of Medicine & Dentistry, University of Western Ontario, London, ON, Canada
- 3Department of Epidemiology and Biostatistics, Schulich School of Medicine & Dentistry, University of Western Ontario, London, ON, Canada
- 4Department of Paediatrics, Division of Paediatric Rheumatology, Hacettepe University, Ankara, Turkey
- 5Department of Paediatrics, Division of Paediatric Rheumatology, Erciyes University, Kayseri, Turkey
- 6Department of Paediatrics, Division of Paediatric Rheumatology, Cerrhapasa Medical School, Istanbul University, Istanbul, Turkey
Background: During the last decade, remarkable progress with massive sequencing has been made in the identification of disease-associated genes for AIDs using next-generation sequencing technologies (NGS). An international group of experts described the ideal genetic screening method which should give information about SNVs, InDels, Copy Number Variations (CNVs), GC rich regions. We aimed to develop and validate a molecular diagnostic method in conjunction with the NGS platform as an inexpensive, extended and uniform coverage and fast screening tool which consists of nine genes known to be associated with various AIDs.
Methods: For the validation of basic and expanded panels, long-range multiplex models were setup on healthy samples without any known variations for MEFV, MVK, TNFRSF1A, NLRP3, PSTPIP1, IL1RN, NOD2, NLRP12 and LPIN2 genes. Patients with AIDs who had already known causative variants in these genes were sequenced for analytical validation. As a last step, multiplex models were validated on patients with pre-diagnosis of AIDs. All sequencing steps were performed on the Illumina NGS platform. Validity steps included the selection of related candidate genes, primer design, development of screening methods, validation and verification of the product. The GDPE (Gentera) bioinformatics pipeline was followed.
Results: Although there was no nonsynonymous variation in 21 healthy samples, 107 synonymous variant alleles and some intronic and UTR variants were detected. In 10 patients who underwent analytical validation, besides the 11 known nonsynonymous variant alleles, 11 additional nonsynonymous variant alleles and a total of 81 synonymous variants were found. In the clinical validation phase, 46 patients sequenced with multiplex panels, genetic and clinical findings were combined for diagnosis.
Conclusion: In this study, we describe the development and validation of an NGS-based multiplex array enabling the “long-amplicon” approach for targeted sequencing of nine genes associated with common AIDs. This screening tool is less expensive and more comprehensive compared to other methods and more informative than traditional sequencing. The proposed panel offers advantages to WES or hybridization probe equivalents in terms of CNV analysis, high sensitivity and uniformity, GC-rich region sequencing, InDel detection and intron covering.
Introduction
As a term, autoinflammation was used to define the diseases not accompanied with high titer of antibodies or T cells. Now it has been understood that these diseases are caused by mutations in genes regulating the innate immune responses (1). Cells that are mediating the pathogenesis of hereditary autoinflammatory diseases (AIDs) are the cells of the innate immune system such as dendritic cells, neutrophils, monocytes and macrophages (2). The diagnosis of AIDs is generally based on the clinical manifestations, symptoms and other biochemical parameters. A detailed clinical history and physical examination are the first steps in the diagnosis and management of autoinflammatory diseases in childhood (3–5). Some of these diseases occur soon after birth and can be fatal if diagnosis and treatment is delayed or unavailable. The differential diagnosis will be maintained so that the treatment modalities can be established before the disease progresses with the help of the genetic screening service. Specific clinical manifestations and genetic analysis are significant for making a differential diagnosis. However, many patients share similar clinical symptoms/features and 50% of patients do not have confirmation by molecular genetic testing (6, 7). Genetic analysis of patients with AIDs allows early and accurate diagnosis and the administration of appropriate treatments. Molecular genetics has greatly contributed to correct diagnosis, especially in atypical presentations (1, 8). These patients are on costly drugs because of empirical treatment modalities due to lack of genetic diagnosis and many patients are using off label or not able to use medications due to not being able to detect genetic mutation. Mutational screening may not be comprehensive related to the used techniques (Sanger Sequencing, Strip Assay etc.), partial gene screening, or screening just known genes and/or mutational hotspots or a subset of coding portions.
During the last decade, remarkable progress with massive sequencing has been made in the identification of disease-associated genes for AIDs using the next generation sequencing technologies (NGS) (1, 8–10). NGS has become an instrumental technology for finding single-gene defects with a comprehensive approach in undiagnosed patients with early onset symptoms (11–14). NGS has advanced the field of autoinflammation by identifying disease-causing genes that point to pathways not known to regulate cytokine signaling or inflammation. Unfortunately, it is still not available for use in routine practice due to great expense in many countries (15). Accurate diagnosis of AIDs is essential to access for the treatment. Overlapping disease manifestations provoke genetic testing among AIDs is the unique way for the diagnosis. International group of experts recently published a guideline and suggested sequencing the 8 genes at a minimum, and if possible additional AIDs genes from the list referenced in Infevers (16). According to this guideline, an ideal genetic screening method should give information about SNVs, InDels, Copy Number Variations (CNVs), GC rich regions, mosaicism and it must be deep sequencing (1000−10,000×). However, there is no tool that currently provides this data for both the clinician and geneticist. Our aim was to develop and validate a molecular diagnostic method in conjunction with NGS platform as an inexpensive, extended and uniform coverage and fast screening tool which consists of nine genes known to be associated with various AIDs.
Material and Methods
Sample Collection and DNA Extraction
DNA extraction from blood samples was performed using the QIAamp DNA mini kit (Qiagen, Germany). The concentration and purity of the DNA were measured by a spectrophotometric method (NanoDrop 2000c, Thermo Scientific) at 260/280 nm wave length (17). The DNA was isolated at 25 ng/µl concentration and stored to use at 4°C.
Primer Design, Multiplex PCR Amplification
Two different multiplex panels were designed as basic and expanded panels. The basic panel is a part of the expanded panel. The basic panel contains MEFV, MVK, TNFRSF1A and NLRP3 genes’ CDS, UTR and some intronic regions. The expanded panel contains PSTPIP1, IL1RN, NOD2, NLRP12 and LPIN2 in addition to the basic panel (Table 1). The regions covered by the panels are shown in Figure 1. First, gene regions were determined and primers were designed to cover all coding sequences (CDS) and UTRs of genes by using NCBI Primer Blast (18). The Long PCR method was chosen to reduce the number of reactions. With this method, amplicons up to 11.78 Kb were obtained. Each of the primers was tested with healthy control DNAs. For multiplex PCR amplification, primers were designed to minimize primer-dimer formation. Attention was paid to ensure that Tm degrees were close. Reaction contents and conditions were also optimized in this context. The gene regions in the basic panel were amplified in one tube while the expanded panel regions were amplified in four tubes. The 25 µl multiplex PCR amplification reaction volume contained 1,25 unit of LA Taq Hot-Start DNA Polymerase, 1,25 unit of PrimeSTAR GXL DNA Polymerase (TaKaRa Bio, Shiga, Japan), 2.5 µl of 10X LA PCR Buffer II (Mg2+ plus), 4 µl of dNTP mixture (2.5 mM each), 5 µl of Betaine (Sigma), 1 µl (0.2 µM each) of primer mixture (Table 2), 1-25 ng of template genomic DNA and the rest ddH20. After determining the appropriate amounts of DNA input and PCR components, the PCR cycle and running parameters were adjusted for effective amplification. The cycling parameters are as follows: initial denaturation at 95°C for 3 min, followed by 34 cycles of 30 second denaturing at 95°C, 40 s annealing at 58°C, and 12 min extension at 68°C. A final extension at 72°C for 12 min concludes the PCR. The PCR reactions were performed using C1000 Thermal Cycler (Bio-Rad). Amplification was seen as a single band in gel electrophoresis. A 1 Kb DNA ladder was used to assess band size. PCR products were purified by Agencourt AMPure XP (Beckman Coulter) magnetic beads and quantified by Qubit 2.0 Fluorometer (Thermo Fisher) with dsDNA BR and HS Reagent Kit.
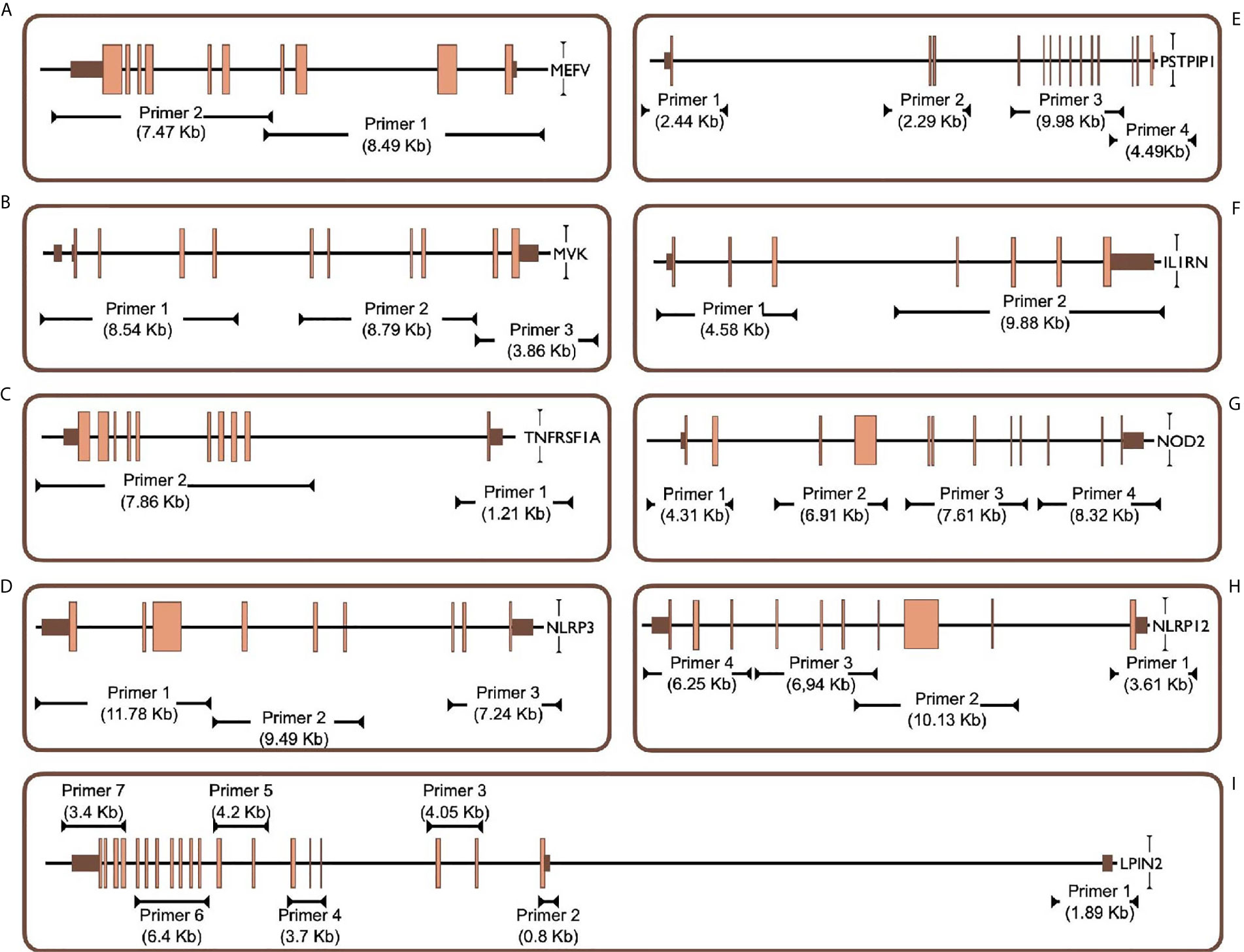
Figure 1 Targeted PCR regions outline in 9 gene. (A) MEFV, Mediterranean Fever, (B) MVK, Mevalonate Kinase (C) TNFRSF1A, Tumor Necrosis Factor Receptor Superfamily Member 1A, (D) NLRP3, NLR Family Pyrin Domain Containing 3, (E) PSTPIP1, Proline-Serine-Threonine Phosphatase Interacting Protein 1, (F) IL1RN, Interleukin-1 Receptor Antagonist, (G) NOD2, Nucleotide-binding Oligomerization Domain-containing Protein 2, (H) NLRP12, NLR Family Pyrin Domain Containing 12, (I) LPIN2, Phosphatidate Phosphatase LPIN2. Brown and orange boxes indicate UTRs and exons, respectively.
NGS Using Illumina NextSeq 500 System
Barcoded library PCR products were prepared with Nextera XT Sample Prep Kit (Illumina) according to manufacturer’s protocol for > 500 bp amplicon read. 1 ng total multiplex PCR products were used for the preparation of each DNA library. PCR products were enzymatically fragmented with Nextera XT kit (19). Each DNA library was tagged with unique index by 12 cycles of PCR. After cleaning up and normalization steps, all libraries were pooled. Sequencing was performed using the NextSeq 500 Sequencing System (Illumina) with 300 cycle Mid-Output Kit.
Bioinformatics
Sequencing data was analyzed for rare pathogenic variants that might be associated with the disease. Gentera Data Processing Engine (GDPE) [Gentera (20), Turkey], an easy-to-use automatic pipeline, was used for analyzing genomes. GDPE provides high accuracy variant detection by using different algorithms. A sample sheet and raw data were used as an input. The 5 ‘and 3’ ends of this DNA sequence data are trimmed to certain lengths considering the quality parameters. The DNA sequence data of targeted genes are aligned with using BWA (21) based on the reference human genome sequence (GRCh38). After the alignment, the following steps were followed with using GATK (22) algorithm. Realignment in InDel regions, recalibrating the quality score, parameter optimizations for variations, variant annotation, filtering variants according to Strand Bias status by taking the upper limit (20%), eliminating unreliable (<15%) variations according to the percentage of variation detected. FastQC (23) was used to evaluate the quality of data. Raw VCF file annotated with Annovar (24). The dbSNP150 (25) database was used to determine SNP annotations, amino acid and nucleotide changes and locations in the final report. “Sorting Intolerant from Tolerant” [SIFT (26)] and “Polymorphism Phenotyping” [PolyPhen (27)] applications were used to evaluate the possible impact assessment of variations on proteins. CNV analysis was performed with coverage-based CNVpytor (a python extension of CNVnator) to determine copy number and large structural variations (28). CNVpytor refined the data with multiple-bandwidth partitioning and GC correction approaches.
Verifications of the Singleplex and Multiplex Models in Healthy Controls
With the primers designed as described, a trial study was carried out without any known variations on the genes being sequenced. Each of the 9 genes was sequenced separately in different DNA with the singleplex model and visualized using gel electrophoresis. The singleplex PCR products controlled on the gel were sequenced as described in the NGS system. The same procedure was performed for basic and expanded panels on healthy controls. Multiplex PCR primers and conditions were prepared as described. They were visualized on gel electrophoresis (Figure 2). After PCR products were sequenced as described in NGS system, optimization was done by decreasing the number of amplicons with high reading in NGS results, increasing the number of amplicons with low reading and changing reaction conditions.
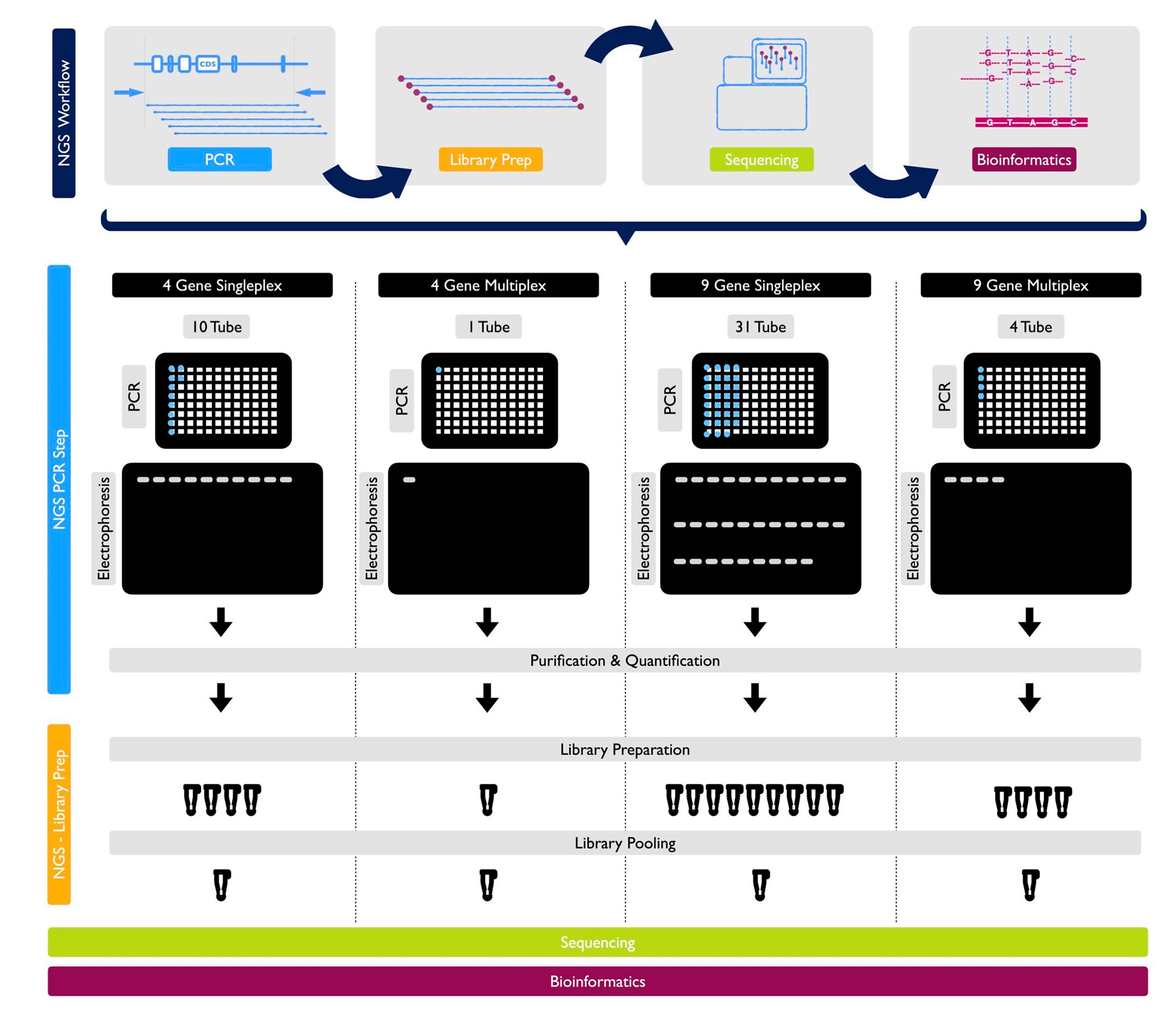
Figure 2 NGS Workflow and detailed protocols for 1 sample by PCR, library prep, sequencing and bioinformatics for 4-gene singleplex, basic multiplex panel, 9-gene singleplex and expanded multiplex panel, respectively.
Analytical Validations of the Multiplex Models in Known Mutation Carriers
Genomic DNA samples from patients with AIDs who had already been found to carry at least one variation in one of the known causative genes previously tested through Sanger sequencing or Strip Assay Kits (ViennaLab) were collected. These variation-positive patients were taken into consideration for developing an NGS-based diagnostic protocol.
Clinical Validations of the Multiplex Models in Undiagnosed Patients
Patients underwent screening history, physical examination, and laboratory evaluation, in the outpatient department in ten different pediatric and adult rheumatology centers where these groups of patients are mainly followed up in Turkey and pre-diagnosed with FMF, MKD (HIDS), TRAPS, CAPS, PAPA, DIRA, Blau, FCAS2 and Majeed syndrome. Initially, data was collected on their clinical parameters such as presence and duration of fever, frequency of attacks, abdominal pain, age of onset, organ system involvement, the presence of visible lesions (rashes, purpura, nodules etc.). Molecular diagnostics were also considered based on their clinical presentations and response to therapies. Blood samples from previously consented patients and, in some cases, unaffected family members, were collected to extract DNA, and perform NGS analysis with basic and expanded panels (Figure 2).
Results
Verifications of the Singleplex and Multiplex Models in Healthy Controls
For singleplex optimization, the PCR procedure was applied to all primer pairs separately on 9 healthy control DNA samples. In gel electrophoresis, the clear unique-band appearance reflects that the targeted PCR product is amplified (Figure 3A). Basic and expanded panels were developed after optimization of primer composition and PCR conditions with using specific primer sets. Ten healthy control DNA samples were screened with the basic panel, similar PCR product patterns were observed with singleplex electrophoresis. Eight PCR products longer than 7.5 Kb were observed as single thick band. Two bands of 3.8 and 1.2 kb were also observed separately (Figure 3B). Adequate quality was observed in all regions covered by the primers. Two healthy control DNA samples were tested with expanded panel and bands were observed in 0.8 kb and 10 kb range. In total, 21 control samples were sequenced, and no nonsynonymous variation was detected. A total of 107 synonymous variations were detected. 55% of these variations were found on the MEFV (n=13) and 25% on the NLRP3 (n=13). Of the 130 UTR variations detected, 48% were on MEFV and 30% were on NLRP3. In addition, 16% of 1221 intronic variations were found on MEFV, 17% on MVK (n=13) and 42% on NLRP3 (Table 3).
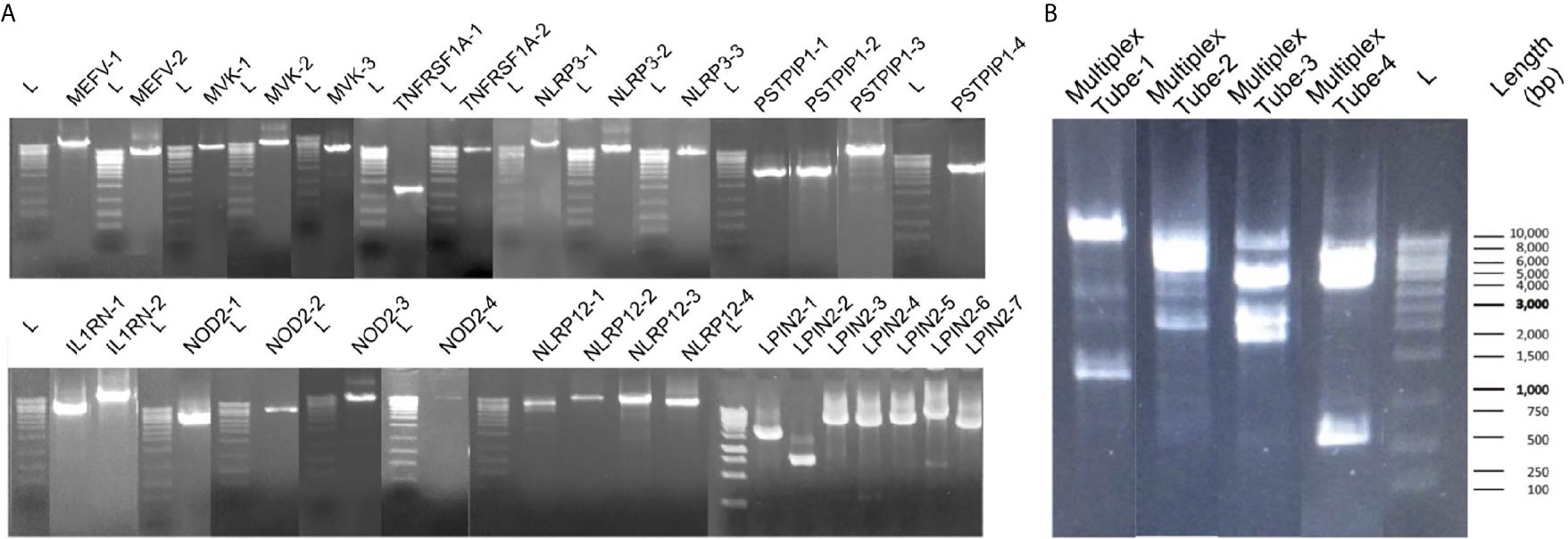
Figure 3 Band patterns of the PCR products. (A) Singleplex 31 amplicons band patterns of MEFV, MVK, TNFRSF1A, PSTPIP1, IL1RN, NOD2, NLRP12 and LPIN2 are shown respectively. L label indicates the 1 kb sized DNA ladder. (B) The multiplex band patterns of the MEFV, MVK, TNFRSF1A and NLRP3 are shown in Multiplex Tube-1. PSTPIP1, IL1RN, NOD2, NLRP12 and LPIN2 patterns are shown in Multiplex Tube 2-4. L label indicates the 1 kb sized DNA ladder.
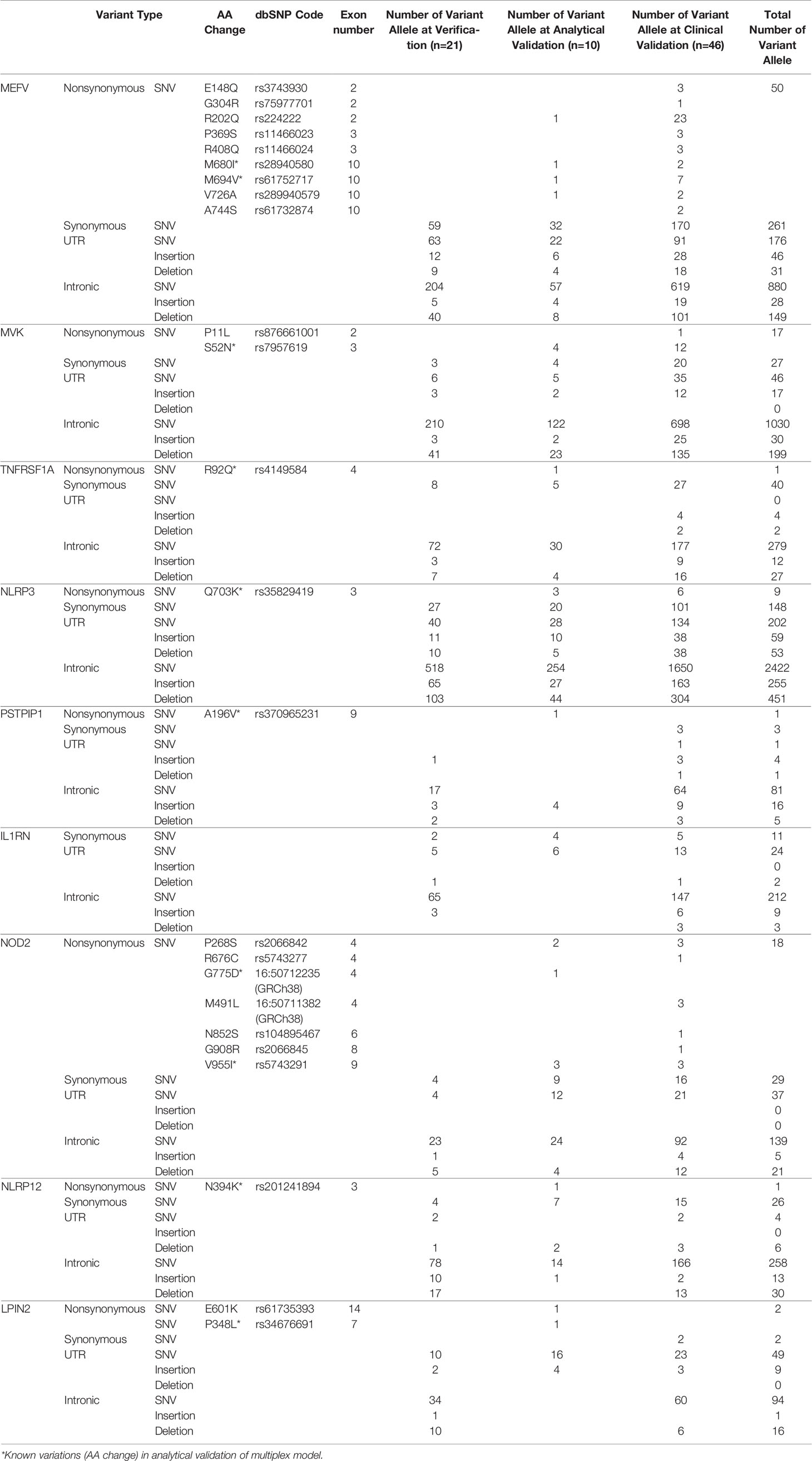
Table 3 Detected variant alleles at verification, analytical validation and clinical validation steps in patients with AID.
Analytical Validations of the Multiplex Models in Known Mutation Carriers:
At this step, 5 patients with known MEFV:M694V, MEFV:M680I, MVK:S52N, TNFRSF1A:R92Q, NLRP3:Q703K/Q703K variations were screened with the basic panel. Another 5 patients with known PSTPIP1:A196V, NOD2:V955I, NOD2:G775D, NLRP12:N394K, LPIN2:P348L variations were screened with the expanded panel. In addition to these nonsynonymous variations, 11 more nonsynonymous allele variants were detected. A total of 81 synonymous variations were detected. 39% of these variations were found on the MEFV (n=10) and 24% on the NLRP3 (n=10). Of the 89 UTR variations detected, 24% were on MEFV and 31% were on NLRP3. In addition, 50% of 501 intronic variations were found on NLRP3 and 24% on MVK (n=10) (Table 3).
Clinical Validations of the Multiplex Models in Undiagnosed Patients
Panels validated with prediagnosed AIDs patients (n=46) who were diagnosed by primary attending physician, reported symptoms which were associated with episodes of the patients with undiagnosed AIDs were screened with panel in this step summarized in Table 4. The most common symptoms were fever>38C (82.6%), abdominal pain (52.2%), arthralgia (52.2%). Skin involvement was described in 50% of the patients such an urticarial rash, maculo-papular rash, pseudo-folliculitis, and erysipelas-like rash. The number of episodes in a year were reported as more than 12 (10.9%), 6-12 (34,8%) and between 2 and 6 (54.3%) by the patients or their parents. The duration of episodes in our cohort were 2-5 days in 71.7%, 5-10 days in 8.7% and >10 days in 19.6%.
Expanded panels were tested in 14 samples, basic panels tested in 32 samples according to their possible clinical diagnosis. Primary physicians requested to screen a total of 129 genes from 46 patients with the preliminary diagnosis of FMF (n=18), CAPS (n= 25), MKD (n=19), TRAPS (n=23), Blau (n=10), DIRA (n=9), Majeed (n=9), PAPA (n=8) and NALP12 (n=8). Exonic and intronic variations were detected and grouped according to their variant types and summarized in Table 3. A total of 77 nonsynonymous variations were detected. 59% of these were found on MEFV (n=46), 17% were on MVK (n=46), 15% were on NOD2 (n=14) and 7% were on NLRP3 (n=46). A total of 359 synonymous variations were detected and 47% of these variations were found on MEFV and 28% on the NLRP3. Of the 320 UTR variations detected, 28% were on MEFV and 41% were on NLRP3. In addition, 50% of 3673 intronic variations were found on NLRP3, 19% on MVK and 16% on MEFV. Uniform coverage was obtained from exonic, intronic and UTRregions (Figure 4). Also, the CNV alteration was not seen in any sample (Figure 5).
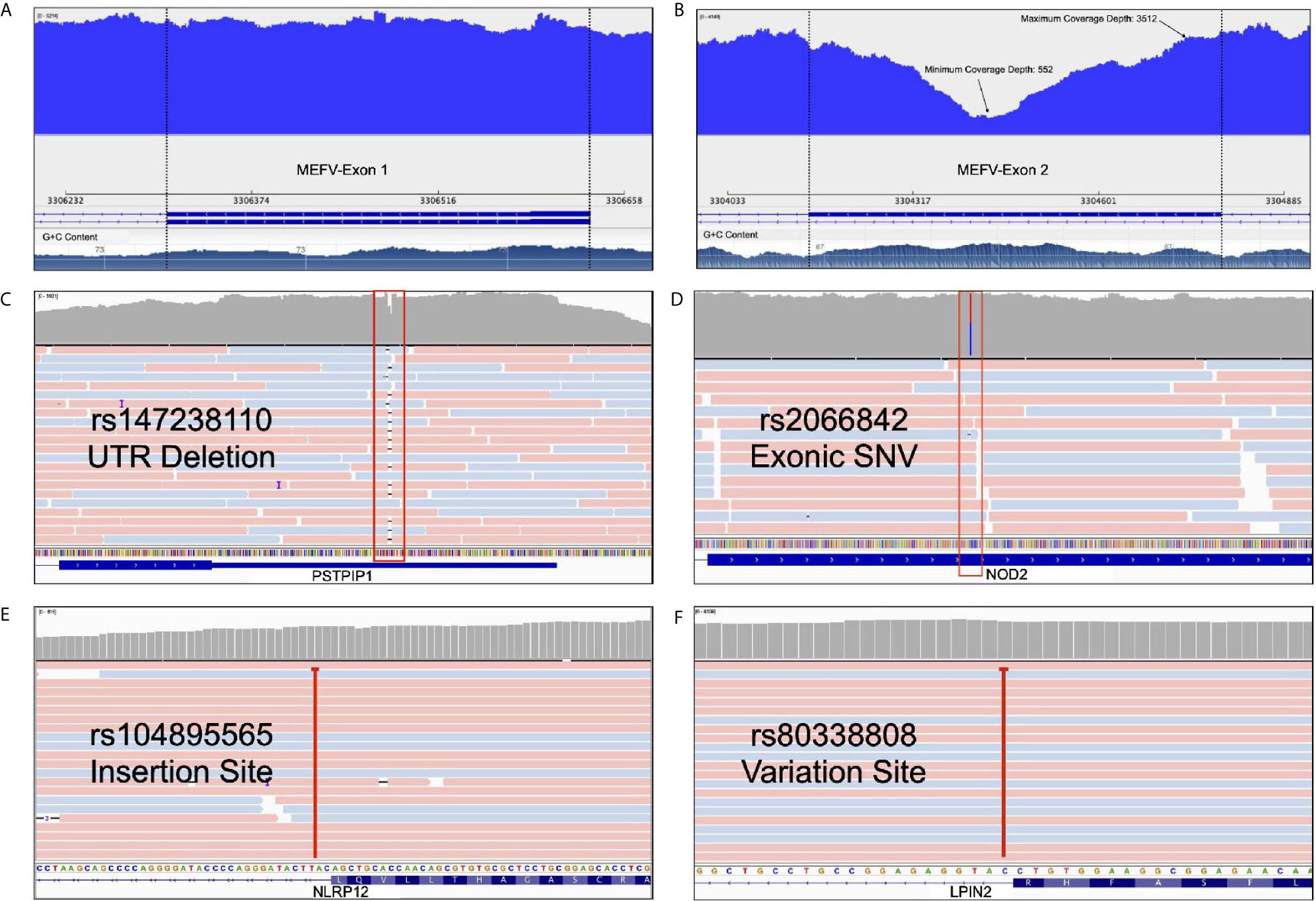
Figure 4 Advantages of our multiplex model. (A) Uniform coverage demonstration on MEFV first exon* (B) Minimum and maximum coverage depth of the MEFV second exon with high GC content* (C) A deletion from PSTPIP1’s UTR as an example of InDel determination. (D) A heterozygosis SNV detection of NOD2 with deep sequencing rate (E) An intronic NLRP12 insertion site that deeply covered (F) An intronic LPIN2 variation site that cause frameshift. *These figures show the change of coverage depth along the exon. G+C content graph can be seen at the bottom.
Discussion
In this study, we describe the development and validation of NGS-based multiplex array enabling the “long-amplicon” approach, which allows compatibility for both long and short read NGS systems including Illumina (Miseq, Hiseq and Nextseq500), Oxford Nanopore and other NGS platforms for targeted sequencing of the nine genes associated with the most common AIDS. Validity steps included the selection of related candidate genes, primer design, development of screening methods, validation and verification of the product. This screening tool is less expensive and more comprehensive compared to other methods and more informative than traditional sequencing. Regarding the complex clinical and molecular diagnosis for AIDs, it should be emphasized that this diagnostic kit will simultaneously test all known genes and variants.
Our long-range multiplex model is enabled to screen exon-intron boundaries and deep introns. WGS approach shows that there is an augmenting number of pathogenic variants positioned within introns at least 100 bp away from the exon-intron junction (29). Moreover, Genome-Wide Association Studies (GWAS) revealed that many intronic variants have a significant association with diseases (30, 31). Protein translation and expression are affected by deep intronic variants which were observed in cystic fibrosis or collagenopathies (32). Current deep intronic variants which can cause AIDs have not been described. WES, hybridization probe-based sequencing and microarray-based analysis failed to detect intronic variations (29). The relationship between intronic variants and AIDs has been reported. In a patient with periodic fever syndrome, an intronic NLRP12 pathogenic variant (rs104895565) activated a cryptic splice site upstream in exon 3 and caused a frameshift followed by an early stop codon (33). Similarly, an intronic LPIN2 pathogenic variant (rs80338808) reported in a patient with chronic recurrent multifocal osteomyelitis (CRMO) and congenital dyserythropoietic anemia (CDA) caused a frameshift in exon 17 which resulted in early stop codon (34). Our results showed that our panel detects potential intronic variations such as previously described rs104895565 and rs80338808 and able to extend in the light of new information (Figures 4E, F). We found the least intron coverage in the LPIN2 gene with 20% and the highest coverage in the MEFV gene (100%). We are able to cover 80% of the intronic regions in six genes among our panel.
Long-read sequencing instruments perform with high accuracy in detecting small insertions, deletions (InDels) and large complex structural variants compared to short-read systems (35). Our panel is compatible with long read sequencing systems which allow high coverage and more accurate results. Rowczenio et al. performed targeted autoinflammatory panel to investigate the molecular cause of persistent fevers resulting in a 24 nt pathogenic mutation being identified in a patient with TRAPS and confirmed as the first known case of gonosomal TNFRSF1A mosaicism (36). Our panels allow us to detect inDels in exonic, UTR (Figure 4C) and intronic regions.
Methods with high specificity and uniform coverage can achieve adequate quality even with a lower sequence data. This also makes sequencing more cost effective. The specificity and uniformity of amplicon-based studies are too high to compare with such WES or targeted hybrid capture based methods (37). Our panels are highly specific to the exons of the genes they contain (Figure 4D). We performed in-silico analysis and BLAST for our panel to increase specificity not to hit a different region in the human genome. Our NGS results showed that, uniform coverage depth was observed except for GC-rich and repetitive regions (Figure 4A). Sequencing difficulties through GC-rich sequences in AIDs related genes (such as MEFV exon 2) underlined as an important concern for the diagnosis (38). We obtained minimum 552X and maximum 3512X coverage depth for MEFV exon 2 with our multiplex panel (Figure 4B). To improve GC-rich sequence reading quality, we used betaine in the PCR step (39). Also, PCR conditions are optimized for the best GC-rich sequence amplification. Our results revealed that high quality sequence readings obtained targeted GC-rich regions.
Copy number variations (CNVs), intermediate structural variants, refers to DNA copy number changes between 1 Kb to 5 Mb (40). There are different CNV calling strategies according to analysis type of NGS including paired-end read (41), read-depth (42) and split read (43). Whole exome sequencing (WES) studies require much effort for CNV analysis (44). Nevertheless, previous studies demonstrate the limitation such as low sensitivity and high false positive rates (45). Shinar et al. have highlighted the importance of CNVs in the genetic analysis of patients with AIDs (16). For sensitive CNV analysis, above 1000X average reading depth (46) and uniform sequence coverage are needed (47). In particular, detection of structural variants is a crucial and recommended for diagnosis of the AIDS (16). Mosaicism derives as a result of single nucleotide variations (SNVs) and CNVs and NGS is a useful method for identifying, categorizing, verifying and validating (48). The 1000X over coverage obtained outside of GC-rich and repetitive regions and uniform read depth made our multiplex model suitable for CNV analysis. Attention was paid to ensure that the algorithm chosen for data analysis was suitable for these parameters. However, no major structural changes and copy number changes were encountered (Figure 5).
Depending on the method and chemistry used, our panels have some limitations. The panel containing 9 genes does not include all genes related to AIDS. Our effort continues to expand the panel content to increase the number of genes. Focusing on specific regions of the genome reduces the possibility of finding novel variations (49). Compared to WGS, our panel is limited in detecting large structural variants and CNVs (50). Both PCR amplification and library preparation, DNA polymerase is used which causes artifacts. The artifacts cause the base substitution errors between 1/3.200 to 1/300.000 errors/base rates (51). In order to reduce the error caused by DNA polymerase and increase the amplification efficiency, we preferred high compatibility enzyme in our studies (52).
As a conclusion, in this study, we described the development and validation of NGS-based multiplex array enabling the “long-amplicon” approach for targeted sequencing of nine AIDs genes. This screening tool is less expensive and more comprehensive compared to other methods and more informative than traditional sequencing. The proposed panel has an advantage compared to WES or hybridization probe equivalents in terms of CNV analysis, high sensitivity and uniformity, GC-rich region sequencing, InDel detection and intron covering (Table 5). Currently, there are 29 genes that have been associated with more than 30 hereditary auto-inflammatory disorders (http://fmf.igh.cnrs.fr/ISSAID/infevers/). The screening tool will be updated from time to time to incorporate the newly discovered genes.
Data Availability Statement
All data relevant to the study are included in the article or uploaded as supplementary information. The raw datasets presented in this article are not readily available because of institutional restrictions and patient privacy. Requests to access the datasets should be directed to corresponding author. The semi processed data supporting the conclusions of this article will be made available by the authors, without undue reservation.
Ethics Statement
The studies involving human participants were reviewed and approved by Cerrahpasa Medical Faculty, Istanbul, Turkey, with the registry number of 83045809/604.01/02-312418/A-31 on 07.10.2015. Written informed consent to participate in this study was provided by the participants’ legal guardian/next of kin.
Author Contributions
ED and FG coordinated the study, drafted the manuscript analyzed the data. FG and EK performed experiments. FG, DP, and MR performed statistical analysis. ED, SO, HP, and OK participated in writing the manuscript with input from all authors. ED, HP, and OK included patients, provided clinical information and samples. ED revised the manuscript critically for important intellectual content and have given final approval of the version to be submitted for publication. ED as a PI had full access to all of the data in the study and takes responsibility for the integrity of the data and the accuracy of the analysis. All authors contributed to the article and approved the submitted version.
Conflict of Interest
The authors declare that the research was conducted in the absence of any commercial or financial relationships that could be construed as a potential conflict of interest.
Acknowledgments
The authors thank all the patients who took part in this study. MR is the recipient of matching fund (Department of Paediatrics, University of Western Ontario, Canada) bursary for international clinical fellowship in BE AID Center. The authors would like to thank Dr. Daniel L. Kastner from NHGRI/NIH from Bethesda-USA for invaluable insight and inputs during the preparation of project proposal.
References
1. Oda H, Kastner DL. Genomics, Biology, and Human Illness: Advances in the Monogenic Autoinflammatory Diseases. Rheumatic Dis Clinics North Am (2017) 43(3):327–45. doi: 10.1016/j.rdc.2017.04.011
2. Frizinsky S, Haj-Yahia S, Machnes Maayan D, Lifshitz Y, Maoz-Segal R, Offengenden I, et al. The Innate Immune Perspective of Autoimmune and Autoinflammatory Conditions. Rheumatol (Oxford England) (2019) 58(Suppl 6):vi1–8. doi: 10.1093/rheumatology/kez387
3. Federici S, Sormani MP, Ozen S, Lachmann HJ, Amaryan G, Woo P, et al. Evidence-Based Provisional Clinical Classification Criteria for Autoinflammatory Periodic Fevers. Ann Rheum Dis (2015) 74(5):799–805. doi: 10.1136/annrheumdis-2014-206580
4. Gattorno M, Hofer M, Federici S, Vanoni F, Bovis F, Aksentijevich I, et al. Classification Criteria for Autoinflammatory Recurrent Fevers. Ann Rheum Dis (2019) 78(8):1025–32. doi: 10.1136/annrheumdis-2019-215048
5. Ter Haar N, Lachmann H, Ozen S, Woo P, Uziel Y, Modesto C, et al. Treatment of Autoinflammatory Diseases: Results From the Eurofever Registry and A Literature Review. Ann Rheum Dis (2013) 72(5):678–85. doi: 10.1136/annrheumdis-2011-201268
6. Zen M, Gatto M, Domeneghetti M, Palma L, Borella E, Iaccarino L, et al. Clinical Guidelines and Definitions of Autoinflammatory Diseases: Contrasts and Comparisons With Autoimmunity-A Comprehensive Review. Clin Rev Allergy Immunol (2013) 45(2):227–35. doi: 10.1007/s12016-013-8355-1
7. Ter Haar NM, Eijkelboom C, Cantarini L, Papa R, Brogan PA, Kone-Paut I, et al. Clinical Characteristics and Genetic Analyses of 187 Patients With Undefined Autoinflammatory Diseases. Ann Rheum Dis (2019) 78(10):1405–11. doi: 10.1136/annrheumdis-2018-214472
8. Manthiram K, Zhou Q, Aksentijevich I, Kastner DL. The Monogenic Autoinflammatory Diseases Define New Pathways in Human Innate Immunity and Inflammation. Nat Immunol (2017) 18(8):832–42. doi: 10.1038/ni.3777
9. Demirkaya E, Arici ZS, Romano M, Berard RA, Aksentijevich I. Current State of Precision Medicine in Primary Systemic Vasculitides. Front Immunol (2019) 10:2813. doi: 10.3389/fimmu.2019.02813
10. Demirkaya E, Sahin S, Romano M, Zhou Q, Aksentijevich I. New Horizons in the Genetic Etiology of Systemic Lupus Erythematosus and Lupus-Like Disease: Monogenic Lupus and Beyond. J Clin Med (2020) 9(3):1–20. doi: 10.3390/jcm9030712
11. Demirkaya E, Zhou Q, Smith CK, Ombrello MJ, Deuitch N, Tsai WL, et al. Brief Report: Deficiency of Complement 1r Subcomponent in Early-Onset Systemic Lupus Erythematosus: The Role of Disease-Modifying Alleles in a Monogenic Disease. Arthritis Rheumatol (Hoboken NJ) (2017) 69(9):1832–9. doi: 10.1002/art.40158
12. Zhou Q, Wang H, Schwartz DM, Stoffels M, Park YH, Zhang Y, et al. Loss-of-Function Mutations in TNFAIP3 Leading to A20 Haploinsufficiency Cause An Early-Onset Autoinflammatory Disease. Nat Genet (2016) 48(1):67–73. doi: 10.1038/ng.3459
13. Zhou Q, Yu X, Demirkaya E, Deuitch N, Stone D, Tsai WL, et al. Biallelic Hypomorphic Mutations in a Linear Deubiquitinase Define Otulipenia, An Early-Onset Autoinflammatory Disease. Proc Natl Acad Sci USA (2016) 113(36):10127–32. doi: 10.1073/pnas.1612594113
14. Lalaoui N, Boyden SE, Oda H, Wood GM, Stone DL, Chau D, et al. Mutations That Prevent Caspase Cleavage of RIPK1 Cause Autoinflammatory Disease. Nature (2020) 577(7788):103–8. doi: 10.1038/s41586-019-1828-5
15. Helmy M, Awad M, Mosa KA. Limited Resources of Genome Sequencing in Developing Countries: Challenges and Solutions. Appl Transl Genom (2016) 9:15–9. doi: 10.1016/j.atg.2016.03.003
16. Shinar Y, Ceccherini I, Rowczenio D, Aksentijevich I, Arostegui J, Ben-Chetrit E, et al. Issaid/Emqn Best Practice Guidelines for the Genetic Diagnosis of Monogenic Autoinflammatory Diseases in the Next-Generation Sequencing Era. Clin Chem (2020) 66(4):525–36. doi: 10.1093/clinchem/hvaa024
17. Desjardins P, Conklin D. NanoDrop Microvolume Quantitation of Nucleic Acids. J Vis Exp (2010) 45:1–4. doi: 10.3791/2565
18. Ye J, Coulouris G, Zaretskaya I, Cutcutache I, Rozen S, Madden TL. Primer-BLAST: A Tool to Design Target-Specific Primers for Polymerase Chain Reaction. BMC Bioinf (2012) 13:134. doi: 10.1186/1471-2105-13-134
19. Lee H-R, Yoo S-J, Kim J, Yoo IS, Park CK, Kang SW. Sat0023 the Differential Production OF Reactive Oxygen Species in T Cell Subsets in Peripheral Blood OF Rheumatoid Arthritis Patients. Ann Rheumatic Dis (2019) 78(Suppl 2):1076. doi: 10.1136/annrheumdis-2019-eular.3850
20. Gentera Biotechnology. (2019). Available at: https://www.microsoft.com/en-us/genomics/partners/.
21. Li H, Durbin R. Fast and Accurate Short Read Alignment With Burrows-Wheeler Transform. Bioinformatics (2009) 25(14):1754–60. doi: 10.1093/bioinformatics/btp324
22. McKenna A, Hanna M, Banks E, Sivachenko A, Cibulskis K, Kernytsky A, et al. The Genome Analysis Toolkit: A MapReduce Framework for Analyzing Next-Generation DNA Sequencing Data. Genome Res (2010) 20(9):1297–303. doi: 10.1101/gr.107524.110
23. Andrews S. Fastqc. A Quality Control Tool for High Throughput Sequence Data [Online]. (2010). Available at: http://www.bioinformatics.babraham.ac.uk/projects/fastqc/.
24. Wang K, Li M, Hakonarson H. ANNOVAR: Functional Annotation of Genetic Variants From High-Throughput Sequencing Data. Nucleic Acids Res (2010) 38(16):e164. doi: 10.1093/nar/gkq603
25. Sherry ST, Ward MH, Kholodov M, Baker J, Phan L, Smigielski EM, et al. dbSNP: The NCBI Database of Genetic Variation. Nucleic Acids Res (2001) 29(1):308–11. doi: 10.1093/nar/29.1.308
26. Ng PC, Henikoff S. Sift: Predicting Amino Acid Changes That Affect Protein Function. Nucleic Acids Res (2003) 31(13):3812–4. doi: 10.1093/nar/gkg509
27. Adzhubei I, Jordan DM, Sunyaev SR. Predicting Functional Effect of Human Missense Mutations Using Polyphen-2. Curr Protoc Hum Genet (2013) 76:Chapter 7:Unit7 20. doi: 10.1002/0471142905.hg0720s76
28. Abyzov A, Urban AE, Snyder M, Gerstein M. Cnvnator: An Approach to Discover, Genotype, and Characterize Typical and Atypical CNVs From Family and Population Genome Sequencing. Genome Res (2011) 21(6):974–84. doi: 10.1101/gr.114876.110
29. Vaz-Drago R, Custodio N, Carmo-Fonseca M. Deep Intronic Mutations and Human Disease. Hum Genet (2017) 136(9):1093–111. doi: 10.1007/s00439-017-1809-4
30. Hsiao YH, Bahn JH, Lin X, Chan TM, Wang R, Xiao X. Alternative Splicing Modulated by Genetic Variants Demonstrates Accelerated Evolution Regulated by Highly Conserved Proteins. Genome Res (2016) 26(4):440–50. doi: 10.1101/gr.193359.115
31. Xiong HY, Alipanahi B, Lee LJ, Bretschneider H, Merico D, Yuen RK, et al. RNA Splicing. The Human Splicing Code Reveals New Insights Into the Genetic Determinants of Disease. Science (2015) 347(6218):1254806. doi: 10.1126/science.1254806
32. Hashkes PJ, Laxer RM, Simon A. Textbook of Autoinflammation. Switzerland: Springer International Publishing (2019).
33. Yu JW, Fernandes-Alnemri T, Datta P, Wu J, Juliana C, Solorzano L, et al. Pyrin Activates the ASC Pyroptosome in Response to Engagement by Autoinflammatory PSTPIP1 Mutants. Mol Cell (2007) 28(2):214–27. doi: 10.1016/j.molcel.2007.08.029
34. Al-Mosawi ZS, Al-Saad KK, Ijadi-Maghsoodi R, El-Shanti HI, Ferguson PJ. A Splice Site Mutation Confirms the Role of LPIN2 in Majeed Syndrome. Arthritis Rheum (2007) 56(3):960–4. doi: 10.1002/art.22431
35. Kai Ye GH, Zemin N. Structural Variation Detection From Next Generation Sequencing. Next Generat Sequenc Applic (2016) 3:1–6. doi: 10.4172/2469-9853.S1-007
36. Rowczenio DM, Trojer H, Omoyinmi E, Arostegui JI, Arakelov G, Mensa-Vilaro A, et al. Brief Report: Association of Tumor Necrosis Factor Receptor-Associated Periodic Syndrome With Gonosomal Mosaicism of a Novel 24-Nucleotide TNFRSF1A Deletion. Arthritis Rheumatol (2016) 68(8):2044–9. doi: 10.1002/art.39683
37. Mamanova L, Coffey AJ, Scott CE, Kozarewa I, Turner EH, Kumar A, et al. Target-Enrichment Strategies for Next-Generation Sequencing. Nat Methods (2010) 7(2):111–8. doi: 10.1038/nmeth.1419
38. Rowczenio D, Shinar Y, Ceccherini I, Sheils K, Van Gijn M, Patton SJ, et al. Current Practices for the Genetic Diagnosis of Autoinflammatory Diseases: Results of a European Molecular Genetics Quality Network Survey. Eur J Hum Genet (2019) 27(10):1502–8. doi: 10.1038/s41431-019-0439-9
39. Henke W, Herdel K, Jung K, Schnorr D, Loening SA. Betaine Improves the PCR Amplification of GC-rich DNA Sequences. Nucleic Acids Res (1997) 25(19):3957–8. doi: 10.1093/nar/25.19.3957
40. Freeman JL, Perry GH, Feuk L, Redon R, McCarroll SA, Altshuler DM, et al. Copy Number Variation: New Insights in Genome Diversity. Genome Res (2006) 16(8):949–61. doi: 10.1101/gr.3677206
41. Korbel JO, Urban AE, Affourtit JP, Godwin B, Grubert F, Simons JF, et al. Paired-End Mapping Reveals Extensive Structural Variation in the Human Genome. Science (2007) 318(5849):420–6. doi: 10.1126/science.1149504
42. Yoon S, Xuan Z, Makarov V, Ye K, Sebat J. Sensitive and Accurate Detection of Copy Number Variants Using Read Depth of Coverage. Genome Res (2009) 19(9):1586–92. doi: 10.1101/gr.092981.109
43. Roca I, Gonzalez-Castro L, Fernandez H, Couce ML, Fernandez-Marmiesse A. Free-Access Copy-Number Variant Detection Tools for Targeted Next-Generation Sequencing Data. Mutat Res (2019) 779:114–25. doi: 10.1016/j.mrrev.2019.02.005
44. Miyatake S, Koshimizu E, Fujita A, Fukai R, Imagawa E, Ohba C, et al. Detecting Copy-Number Variations in Whole-Exome Sequencing Data Using the Exome Hidden Markov Model: An ‘Exome-First’ Approach. J Hum Genet (2015) 60(4):175–82. doi: 10.1038/jhg.2014.124
45. Guo Y, Sheng Q, Samuels DC, Lehmann B, Bauer JA, Pietenpol J, et al. Comparative Study of Exome Copy Number Variation Estimation Tools Using Array Comparative Genomic Hybridization as Control. BioMed Res Int (2013) 2013:915636. doi: 10.1155/2013/915636
46. Shen W, Szankasi P, Durtschi J, Kelley TW, Xu X. Genome-Wide Copy Number Variation Detection Using Ngs: Data Analysis and Interpretation. Methods Mol Biol (Clifton NJ) (2019) 1908:113–24. doi: 10.1007/978-1-4939-9004-7_8
47. Kerkhof J, Schenkel LC, Reilly J, McRobbie S, Aref-Eshghi E, Stuart A, et al. Clinical Validation of Copy Number Variant Detection From Targeted Next-Generation Sequencing Panels. J Mol Diagn (2017) 19(6):905–20. doi: 10.1016/j.jmoldx.2017.07.004
48. Gajecka M. Unrevealed Mosaicism in the Next-Generation Sequencing Era. Mol Genet Genomics (2016) 291(2):513–30. doi: 10.1007/s00438-015-1130-7
49. Dilliott AA, Farhan SMK, Ghani M, Sato C, Liang E, Zhang M, et al. Targeted Next-generation Sequencing and Bioinformatics Pipeline to Evaluate Genetic Determinants of Constitutional Disease. J Vis Exp (2018) 134:1–10. doi: 10.3791/57266
50. Bewicke-Copley F, Arjun Kumar E, Palladino G, Korfi K, Wang J. Applications and Analysis of Targeted Genomic Sequencing in Cancer Studies. Comput Struct Biotechnol J (2019) 17:1348–59. doi: 10.1016/j.csbj.2019.10.004
51. Eckert KA, Kunkel TA. High Fidelity DNA Synthesis by the Thermus Aquaticus DNA Polymerase. Nucleic Acids Res (1990) 18(13):3739–44. doi: 10.1093/nar/18.13.3739
Keywords: autoinflammation, next generation sequencing - NGS, long range PCR, multiplex, genetic diagnosis
Citation: Guzel F, Romano M, Keles E, Piskin D, Ozen S, Poyrazoglu H, Kasapcopur O and Demirkaya E (2021) Next Generation Sequencing Based Multiplex Long-Range PCR for Routine Genotyping of Autoinflammatory Disorders. Front. Immunol. 12:666273. doi: 10.3389/fimmu.2021.666273
Received: 09 February 2021; Accepted: 14 May 2021;
Published: 09 June 2021.
Edited by:
Federico Alberici, University of Brescia, ItalyReviewed by:
Matteo Doglio, San Raffaele Hospital (IRCCS), ItalyKlaus Tenbrock, RWTH Aachen University, Germany
Copyright © 2021 Guzel, Romano, Keles, Piskin, Ozen, Poyrazoglu, Kasapcopur and Demirkaya. This is an open-access article distributed under the terms of the Creative Commons Attribution License (CC BY). The use, distribution or reproduction in other forums is permitted, provided the original author(s) and the copyright owner(s) are credited and that the original publication in this journal is cited, in accordance with accepted academic practice. No use, distribution or reproduction is permitted which does not comply with these terms.
*Correspondence: Erkan Demirkaya, Erkan.Demirkaya@lhsc.on.ca