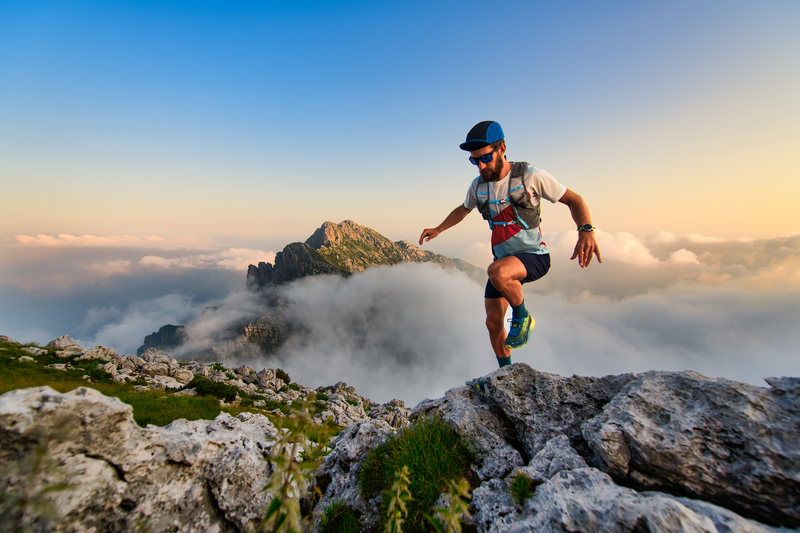
94% of researchers rate our articles as excellent or good
Learn more about the work of our research integrity team to safeguard the quality of each article we publish.
Find out more
REVIEW article
Front. Immunol. , 07 May 2021
Sec. Molecular Innate Immunity
Volume 12 - 2021 | https://doi.org/10.3389/fimmu.2021.665782
This article is part of the Research Topic The Role of Immunometabolism in Tissue Homeostasis and Tissue Resident Immune Cells View all 8 articles
Tissue-resident macrophages exist in unique environments, or niches, that inform their identity and function. There is an emerging body of literature suggesting that the qualities of this environment, such as the types of cells and debris they eat, the intercellular interactions they form, and the length of time spent in residence, collectively what we call habitare, directly inform their metabolic state. In turn, a tissue-resident macrophage’s metabolic state can inform their function, including whether they resolve inflammation and protect the host from excessive perturbations of homeostasis. In this review, we summarize recent work that seeks to understand the metabolic requirements for tissue-resident macrophage identity and maintenance, for how they respond to inflammatory challenges, and for how they perform homeostatic functions or resolve inflammatory insults. We end with a discussion of the emerging technologies that are enabling, or will enable, in situ study of tissue-resident macrophage metabolism.
In his “An Essay Concerning Human Understanding” (1), John Locke famously argued against “innate principles” or the notion that we are born with knowledge that informs our identity. Instead, Locke posited that our identity (and thus uniqueness) arises from sensory experience, “… anything as existing at any determined time and place, we compare it with itself existing at another time, and thereon form the ideas of identity and diversity.” Although Locke’s ideas were ultimately imperfect, his thinking spurred centuries of discussion on the nature of identity: is our identity innately informed or driven by experience? With this as our backdrop, several decades of work on the aptly-named innate immune system has informed a broad understanding of intrinsically-encoded receptors and sensors that serve to fight infection and keep the host healthy. However, with the development of new tools and methods, there is an emerging body of knowledge on how an innate immune cell’s lived experience informs its identity and function. No more so is this true than with the study of tissue-resident macrophages (TRMs).
Specific environmental factors, such as nutrient and oxygen availability, tissue material and debris that TRMs are exposed to, and the length of time spent in this habitare, as well as the events that a TRM experience (such as infectious or sterile insult), all factor in to TRM identity and function, an idea that has been beautifully articulated in a series of reviews and prospective articles (2–5). Building from these insights, we propose that each of these factors contribute to the metabolic state of TRMs, which in turn informs the efficiency and nature of TRM function. In this review, we discuss recent advances in our understanding of TRM metabolism, focusing on studies that explore TRM metabolism in situ/in vivo. We will highlight metabolic regulation of three key aspects of TRM biology: TRM identity, responses during infection and inflammation, and homeostatic/reparative processes. We end with a discussion of methods previously employed to study TRM metabolism as well as emerging technologies that we foresee will lead to a significant advancement in our understanding of TRM metabolism.
Tissue-resident macrophages (TRMs) are a diverse family of cells that arise from a common erythro-myeloid precursor, develop a core macrophage signature during embryogenesis, and acquire specialized transcriptional programs during organogenesis (6–8). TRMs perform a number of canonical immune functions as well as tissue-specific physiological functions throughout their lifespan (3, 4, 9, 10). For instance, cells in the developing organism begin to undergo apoptosis in the yolk sac which continues in all organs throughout development. These dying cells must be cleared by TRMs to ensure proper organogenesis (11). Osteoclasts, a bone-resident TRM (12), are required for bone resorption and homeostasis, such that perturbed osteoclast activity results in osteopetrosis (diminished osteoclast function) or osteoporosis (excessive osteoclast function) (13). Microglia, a brain-resident TRM, are required for synaptic pruning and optimal neurodevelopment. Alveolar macrophages, a lung-resident TRM, are required for surfactant clearance and airway integrity (4). Adipose tissue TRMs contribute to insulin sensitivity, adipogenesis, and adaptive thermogenesis (14). Bone marrow and splenic red pulp macrophages clear millions of spent erythrocytes each day, essential for maintenance of iron homeostasis and prevention of diseases such as hemochromatosis, thalassemia, and chronic anemia (15). Thus, TRMs are exposed and adapt to a multitude of tissue-specific growth factors (16–19), dying cells and debris with varying metabolic properties (20–27), and unique (and often extreme) tissue environments (3, 28, 29) to ensure tissue and organismal homeostasis.
This is important because TRMs are generally long-lived and self-renewing, and the programs they acquire during development are thought to persist throughout life and transmit to daughter cells (30–33). We are beginning to elucidate some of the tissue-specific metabolic factors that drive regulation of TRM identity. For instance, in the liver, Kupffer cells (the main liver TRM population) rely on the cholesterol synthesis intermediate desmosterol to induce the nuclear receptor liver X receptor alpha (LXRα) (34), which in turn is necessary to induce and maintain Kupffer cell transcriptional identity (34–36). These results are particularly intriguing because previous work suggests that the alternative LXRα ligand oxysterol can, in some instances, induce and potentiate pro-inflammatory gene transcription (37, 38) but in other instances induce cholesterol efflux, repress pro-inflammatory responses, and prevent inflammasome activation (39–41). These findings have important implications for TRM metabolism during homeostasis and resolution, which is discussed further below. Similarly, both splenic red pulp and bone marrow-resident macrophages depend on the porphyrin synthesis product heme, which induces expression of the lineage-defined transcription factor Spi-C via degradation of the transcriptional repressor BTB Domain And CNC Homolog 1 (BACH1) (42, 43). However, unlike for Kupffer cells, the source and timing of heme uptake in splenic red pulp or bone marrow-resident macrophages remains unknown. One possibility is that engulfment of dying erythrocytes, which both populations perform millions of times a day (24, 44), provides the key defining source of heme. Alternatively, splenic red pulp macrophages are seeded in a WT1+ stromal network similar to that observed in Kupffer cells (45), which may synthesize and release heme that is subsequently imported via the heme transporter HCP1 (SLC46A1) (46, 47).
The above studies also highlight an important consideration: TRMs are generally evenly distributed throughout a complex meshwork of interacting stromal and tissue-specific cells, each of which contribute to the overall nature of the tissue environment (48). Understanding such interactions is understandably complex when considering the exponential nature at which intercellular interactions scale (49). Nonetheless, recent work has illustrated the contribution of resident stroma to the metabolic regulation of TRM identity. For example, a network of cavity-resident stromal cells produce the metabolite retinoic acid via transcriptional regulation by Wilms’ Tumor 1 (WT1) (50). Retinoic acid is subsequently required for cavity-resident TRM identity and maintenance through induction of the retinoic-acid-responsive transcription factor GATA6 (16, 17, 50), which in turn regulates the enzyme aspartoacylase (Aspa), necessary for the synthesis of acetyl CoA (51). Conversely, rapamycin-insensitive mTORC2 acts as a negative regulator of GATA6, and its suppression is required for peritoneal TRM differentiation (52). Given that WT1+ stromal cells are a major constituent in two unrelated tissue environments (45, 50), it seems reasonable to conjecture that WT1+ stromal cells are a major non-cell-autonomous regulator of TRM metabolism. In support of this hypothesis, two independent studies observed that the liver also features WT1+ hepatic stellate cells that appear to be important regulators of liver fibrosis and scarring (53, 54). How these cells interact and regulate liver TRM metabolism remains unknown. Collectively, this work serves as a demonstration of the importance of understanding the specific environmental factors that contribute to macrophage identity. Beyond these studies, not much else is known about the metabolic regulation of macrophage identity. Some clues come indirectly from previous studies. For instance, it was previously shown that microglia require cholesterol coupled with TGF-β and IL-34 for identity and phagocytic capacity in mixed cell cultures in vitro, which were specifically derived from astrocytes (55). This is consistent with transcriptional analysis of developing microglia indicating significant upregulation of a lipid metabolism program (56). Furthermore, the development of various TRM subsets depend on colony stimulating factor 1 receptor (CSF1R), a receptor for both CSF1 (M-CSF) and IL-34. CSF1R signaling induces macropinocytosis by macrophages (57), suggesting that TRM development and identity depend on lysosomal catabolism of solutes, such as degradation of proteins to generate amino acids (58, 59). Given these findings, it will be interesting to determine how lineage-defining cytokines combine with tissue-specific metabolites to establish and maintain TRM identity.
It is important to note that here, we focus on TRMs that have recently been defined as “resident macrophages” or macrophages that arise in a tissue early on in development. This population is in contrast to monocyte-derived macrophages that can arise in some tissues later in life, during the course of inflammation, or in some cases seed during inflammation and remain after resolution, as reviewed recently (2). This distinction will remain important moving forward not just for basic understanding of macrophage metabolism, but also as a possible therapeutic strategy where these subsets are either beneficial (60) or detrimental (30, 61) to the health of the host. Additionally, most tissues feature multiple types of resident macrophages (2, 5). For example, there are at least three distinct subsets of macrophages in the spleen, defined both by location and physical properties: red pulp, marginal zone, and metallophilic (15). As noted, red pulp macrophages rely on heme metabolism for their identity, whereas neither marginal zone nor metallophillic macrophages depend on heme or Spi-C. Instead, both populations depend on LXRα (62), presumably through metabolic processes similar to Kupffer cells. Given this, it will be important for future studies of TRM metabolism to take into consideration the unique intra-tissue environment differences, including nutrient (63, 64) and oxygen (65, 66) availability, as well as the cell types and debris they engulf (24).
Danger-associated molecular patterns (DAMPs) and pathogen-associated molecular patterns (PAMPs) are at the core of sterile inflammation and microbial infection, respectively (67, 68). Both DAMPs and PAMPs are known to induce an inflammatory response coordinated by innate immune cells, including monocytes, macrophages and dendritic cells, and that uncontrolled chronic inflammation is associated with a myriad of human diseases (68). Notably, the growing field of immunometabolism has underscored how cellular metabolic reprogramming is at the center of this coordinated inflammatory response (69–71). Work more than three decades ago hinted at metabolic changes in macrophages exposed to microbial ligands (72–74), but through a series of elegant in vitro experiments, we have come to better understand the precise nature of these metabolic changes. The specifics of these metabolic changes are detailed in several fantastic reviews. Here, we briefly outline the broader metabolic switch that occurs in canonically pro-inflammatory macrophages. Typically, a naïve macrophage, defined as a macrophage differentiated from bone marrow stem and progenitor cells cultured in high glucose (~20-25mM), normoxic oxygen (~21% or ~160 mmHg O2), and the differentiation cytokine(s) M-CSF/GM-CSF, relies primarily on the tricarboxylic acid (TCA) cycle and mitochondrial respiration to generate much of the ATP used for basic cellular processes. However, seminal work from O’Neill and colleagues demonstrated that activation with the PAMP lipopolysaccharide (LPS) induces macrophages to undergo a metabolic switch from a state of high oxidative phosphorylation (OXPHOS) to a highly glycolytic state (75). Accumulation of glycolytic intermediates and byproducts further support primary functions of activated macrophages, including providing glucose-6-phosphate to the pentose phosphate pathway (PPP) which contributes to both building of biomass and regulating the redox state, as well as induce the production of immune effectors such as itaconate and IL-1β [summarized in depth here (69)]. Building from this work, numerous studies have demonstrated the importance of this metabolic switch for key inflammatory macrophage functions, including bacterial phagocytosis, production of pro-inflammatory cytokines, synthesis of antimicrobial peptides, and generation of reactive oxygen species (ROS) (76–79). Collectively, this work demonstrates that quiescent macrophages undergo striking metabolic changes in support of one of its core immunological roles as a defender against invading pathogens (Figure 1).
Figure 1 Overview of metabolic pathways induced in pro-inflammatory macrophages. Shown is the canonical pro-inflammatory macrophage metabolic program (Canonical Pathways) as well as some of the more predominantly defined intermediates that accumulate in pro-inflammatory macrophages (Intermediates). Also included are some tissue-resident macrophage (TRM)-specific metabolism observations, including that TRMs in some tissues upregulate OXPHOS and glutaminolysis, that lipids (such as oxidized phosphoplipids and high/low-density lipoproteins) can drive a hyper-metabolic state, and in some contexts, glucose is shunted into glycogenesis.
Together with defense against pathogens, macrophages are key orchestrators of the post-injury response (24, 29, 80). This response, canonically known as the wound healing or resolution response, is in many ways the mirror image of the canonical pro-inflammatory response, including broad metabolic changes (29, 81). Generally speaking, these ‘pro-resolving’ macrophages are derived using the same base culturing conditions as pro-inflammatory macrophages, but naïve macrophages are stimulated with IL-4 instead of LPS (or IFNγ). In contrast to canonical pro-inflammatory macrophages, pro-resolving macrophages remain significantly increase TCA cycle activity and OXPHOS, with less dependence on glycolysis, as well as exhibiting significantly increased fatty acid oxidation (FAO) (82, 83). Though it is important to note that the ‘importance’ of glycolysis remains in debate, and likely depends on the nature of the culture conditions (e.g. glucose and oxygen availability) (83, 84). The observed increase in TCA cycle activity is the result of IL-4-induced PPARγ transcriptional activity, which in turn modulates expression of key enzymes, respiratory chain function, and glutamine metabolism (85–87). An additional benefit of enhanced TCA cycle activity is the accumulation of α-ketoglutarate (α-KG), as α-KG was shown to blunt canonical pro-inflammatory cytokine production via suppression of inhibitor of nuclear factor kappa-B kinase subunit beta (IKKβ) and to induce canonical anti-inflammatory cytokine production via activation of the histone demethylase Jumonji domain-containing protein D3 (JMJD3) (88).
One final aspect of this metabolic program worth highlighting, given its macrophage response-defining role, is the importance of arginine and polyamine metabolism (29). In mouse macrophages, it is generally thought that arginine is catabolized via two distinct routes: via an inducible nitric oxide synthase (iNOS) catalyzed dehydration reaction that produces citrulline and nitric oxide or by an arginase 1 (Arg1) catalyzed hydrolysis reaction that produces ornithine and urea (77). The former reaction is thought to be a key component of the pro-inflammatory macrophage response to pathogen invasion (78), whereas the latter is thought to be a key step to the generation of pro-resolving macrophages (81). Ornithine, and its conversion to putrescine via ornithine decarboxylase (ODC), as part of polyamine synthesis, is essential for multiple facets of the induction and maintenance of pro-resolving macrophages (89) (Figure 2).
Figure 2 Overview of metabolic pathways induced in pro-resolving macrophages. Shown is the canonical anti-inflammatory/pro-resolving macrophage metabolic program (Canonical Pathways) as well as some of the more predominantly defined intermediates that accumulate in pro-resolving macrophages (Intermediates). Also included are some tissue-resident macrophage (TRM)-specific metabolism observations, including that apoptotic cells and/or cytokine (e.g., IL-4/IL-13) induce upregulation of glycolysis, that succinate accumulates and signals via SUCNR1 to drive inflammation resolution, and that novel pathways such as desmosterol synthesis are induced in specific tissues, such as the CNS.
Several excellent reviews provide a survey of our general understanding of the metabolic changes that arise in macrophages in response to IL-4 stimulation (29, 69, 71, 81). Although IL-4 has served as an important model for pro-resolving macrophage metabolism, how these macrophages arise in vivo remains an intriguing area of investigation. For instance, one likely situation occurs after the clearance of a pathogen or during the resolution of a sterile injury. Those macrophages that do not die as part of the immune response (e.g., via pyroptosis) switch to a pro-resolving state. A less obvious example arises in the case of type 2 immunity, where the specific infection (e.g., helminths) directly induces a pro-resolving phenotype in macrophages. Intriguingly, macrophages are also thought to assume a wound healing phenotype during homeostatic apoptotic cell clearance (termed ‘efferocytosis’). Recent work suggests that, at least in some contexts, both IL-4 (or IL-13) and exposure to apoptotic cells is required to induce a pre-resolving macrophage response in vivo (90) (Figure 2). Thus, it will be important to understand the shared and unique metabolic features of TRMs in each of these contexts.
Several controversies related to macrophage metabolic (re)programming remain unresolved. This is likely due, in part, to supraphysiological culturing conditions. The use of in vitro systems will remain the key to understanding macrophage metabolism at a mechanistic level, therefore solutions as outlined previously are warranted. An additional complication relates to how metabolic pathways are perturbed. The use of small molecule inhibitors, RNA interference, and increasingly genetic deletion, to perform ‘down’ assays has informed much of our understanding. However, it has also likely led to conflicting results, for instance due to off-target effects in the case of small molecules or genetic compensation in the case of genetic deletion. Furthermore, it remains controversial how different types of macrophages utilize these canonical metabolic programs and how similar (or not) murine and human macrophage metabolism are. For example, whether differential arginine catabolism via iNOS and ARG1 is relevant for inflammatory and resolving functions in human macrophages remains highly debated (91, 92).
The use of complimentary approaches, including the incorporation of more ‘up’ assays in vitro and in vivo, will allow the field to understand these important questions in greater detail.
As noted, much of our understanding regarding metabolic control of macrophage immunity has come from in vitro studies. Recently, groups have begun to extend these findings into in vivo settings, particularly into physiologically relevant models of human disease (93). In this section, we will first summarize the state of in vivo macrophage immunometabolism studies in the context of inflammation. We will particularly focus on the most recent findings of how metabolic reprogramming impacts and shapes the functions of inflammatory macrophages in vivo (Figure 1). However, it is important to note that, aside from work on microglia, the majority of in vivo macrophage metabolism research does not necessarily delineate TRM subsets, which will be important given there is likely spatiotemporal regulation of macrophage metabolism, as seen in the tumor microenvironment (94).
As briefly discussed above, aerobic glycolysis is a key feature of inflammatory macrophages. Interestingly, byproducts of glycolysis are repurposed to support additional metabolic pathways that drive important macrophage functions (69). Most notably, LPS-activated macrophages accumulate TCA cycle intermediates that possess immunoregulatory properties (9). The metabolite succinate was identified as an inflammatory metabolite that induces IL-1β transcription via HIF-1α (75). In these early studies, both macrophage-specific absence of Hif1a, or treatment with the GABA-shunt inhibitor vigabatrin (which prevents succinate production) protected from endotoxin-induced sepsis (75). Interestingly, a recent study from Cardaci and colleagues found that supplementation with D-mannose given intraperitoneally (i.p.) or via water/oral gavage protected mice from endotoxin-induced sepsis and dextran sodium salt (DSS)-induced colitis, respectively (95). Mechanistically, D-mannose treatment acts to competitively inhibit glycolytic production of succinate, triggering a counter-intuitive increase in mannose-6-phosphate levels, possibly owing to low level expression of the enzyme phosphomannose isomerase (MPI) in macrophages (95). Additionally, inflammatory macrophages release succinate into the extracellular environment, which then triggers an autocrine/paracrine amplification loop via signaling through the succinate receptor SUCNR1/GPR91, increasing IL-1β production and exacerbating joint swelling in a mouse model of arthritis (96). Intriguingly, synovial joint macrophages were recently described as a locally-renewing TRM population that provide a key barrier in the synovial joint that, unlike infiltrating monocyte-derived macrophages, act to prevent/dampen inflammation (97). How local succinate production acts on each of these populations of synovial joint macrophages is an intriguing question, especially given succinate can have opposing actions depending on the cell type and context. For instance, inflammatory microglia-derived succinate was shown to instruct neural stem cells, via GPR91, to secrete prostaglandin E2 (PGE2). PGE2 in turn scavenged excess succinate, resulting in decreased macrophage activation and alleviated inflammation in experimental autoimmune encephalomyelitis (EAE), a mouse model of CNS inflammation (12). This work highlights how dynamics in the specific tissue microenvironment can lead to unexpected results in vivo.
As highlighted in recent work from the O’Neill and Artyomov labs, the TCA cycle intermediate itaconate has emerged as a model ‘immuno-metabolite’, given its essential roles for both pro-inflammatory and pro-resolving macrophage function (98). On one hand, itaconate (as well as the cell-permeant derivates dimethyl itaconate or 4-octyl itaconate) acts as a potent anti-inflammatory modulator by suppressing production of various inflammatory cytokines IL-1β, IL-6, and the type I interferon IFNβ. Mechanistically, itaconate (or its derivatives) functions through multiple mechanisms, including inhibiting glycolytic enzyme activity, activation of the oxidative stress response transcription factor NRF2, modification and inhibition of the NLRP3 inflammasome, and induction of ATF3-mediated regulation of IκBζ (99–105). How endogenous itaconate directly affects TRMs in vivo is less clear, especially because itaconate appears to directly inhibit pro-resolving macrophage differentiation (81, 106), and enhance IFNβ production in response to LPS (104). Additionally, in vivo studies have either used mice globally-deficient in the enzyme necessary to produce itaconate from citrate (immune responsive gene 1, Irg1) or delivered exogenous itaconate (or one of the cell-permeant derivates), making conclusions about TRMs versus other tissue-resident cells capable of utilizing exogenous itaconate challenging (99). Nevertheless, absence of Irg1 or delivery of exogenous itaconate derivatives were shown to dampen LPS-induced sepsis (100), alleviated skin pathology in a mouse model of psoriasis (99), limits myocardial infarct size during ischemia-reperfusion injury (103), and suppressed inflammation in urate crystal-induced peritonitis (102).
This seminal series of studies triggered subsequent exploration of immunoregulatory roles of primary metabolites. For instance, Fitzgerald and colleagues found that the TCA cycle intermediate fumarate inhibits pyroptosis via succination of the cysteines in the pyroptosis effector protein gasdermin D (GSDMD). Indeed, treatment of mice with Tecfidera, the fumarate derivative dimethyl fumarate (DMF), alleviated symptoms in both EAE and Familial Mediterranean Fever mouse models as well as providing a mechanistic explanation for the immunomoulatory activity of Tecfidera in MS patients (107). Unlike in classical LPS-activated macrophages, ‘hyperactivation’ of macrophages is induced by endogenous DAMPs, such as oxidized phospholipids (108). Hyperactivated macrophages also exhibit hyperactive metabolic activity, relying on glycolysis, oxidative phosphorylation, and glutamine catabolism (109). Hyperactive macrophages accumulate the TCA cycle intermediate oxaloacetate, likely as a result of multiple substrates feeding the TCA cycle, which exacerbates IL-1β production (109). This phenomenon was observed in plaque-associated macrophages in Ldlr- and Apoe-deficient mice fed high fat diet, which could be prevented by inhibiting either glutaminase or ATP citrate lyase (109, 110). The atherosclerotic plaque is likely a unique microenvironment itself, as the metabolic state of plaque-associated macrophages (also known as Mox macrophages) seems to be characterized by several unique facets (111), reviewed in greater detail elsewhere (26). We highlight Mox macrophages, however, because recent work suggests that adipose TRMs in lean adipose tissue exhibit metabolic characteristics similar to Mox macrophages, possibly because of differences in individual oxidized phospholipid species (112). Contrarily, adipose TRMs isolated from obese mice show characteristics of canonical pro-inflammatory macrophages such as the IL-1 pathway (113).
Unsurprisingly, the TCA cycle is not the only metabolic pathway relevant in inflammatory macrophages. For example, LPS induces translocation of histone deacetylase 3 (HDAC3) to the mitochondria which subsequently deacetylates and inactivates the alpha subunit of hydroxyacyl-CoA dehydrogenase (HADHA), an enzyme essential for fatty acid oxidation (FAO), which was necessary for IL-1β production (114). Myeloid cell-specific deletion of HDAC3 lessened the severity of both LPS-induced sepsis and diet-induced type 2 diabetes, the latter of which appeared to be a direct effect on adipose TRM metabolism (114). Additionally, LPS stimulation upregulates the choline transporter CTL1 (SLC44A1), facilitating increased uptake and generation of phospho-choline species (via the Kennedy Pathway), altering mitochondrial phospholipid and sphingolipid species profiles, and contributing to NLRP3 inflammasome activation (115). Inhibition of choline kinase lessened the inflammation in both LPS-induced sepsis and a urate crystal-induced gout model, as well as reduced splenomegaly and size in a mouse model of Muckle-Wells syndrome (115). The changes in macrophage lipid metabolism observed in response to LPS stimulation are consistent with two recent studies of lipid metabolism in inflammatory macrophages (116, 117). Finally, one carbon, glycogen, and PPP metabolism were shown to regulate numerous aspects of the in vivo inflammatory macrophage response to LPS (118–120). Taken together, it is clear that TLR signaling induces a plethora of metabolic changes in macrophages in vivo. It will be interesting to see how different TRM populations respond to the combination of inflammatory stimuli and unique tissue environment factors, such as the unique factors in adipose tissue highlighted above. This is exemplified by a recent study which found that alveolar macrophages, who reside in a glucose-restricted environment, rely on OXPHOS and not glycolysis to mount an inflammatory response to acute inflammation induced by LPS or to influenza infection (121). Understanding how alveolar macrophages are able to produce the requisite inflammatory cytokines in the absence of glycolytic reserve may also elucidate novel immunoregulatory metabolites. Indeed, elucidating these unique metabolic regulatory pathways, both in alveolar macrophages but also stromal and immune cells that regularly interact with alveolar macrophages, could prove important for managing the devastating consequences of chronic lung disease [the lung metabolic environment is reviewed in detail elsewhere (122)]. As has likely become apparent, the vast majority of in vivo studies of inflammatory macrophage metabolism use variations of the LPS-induced sepsis model. Naturally, the immunometabolism of TRMs in response to tissue-relevant infections is an important and rapidly growing area of research. For instance, alveolar macrophages rely on different metabolic programs depending on the type of infection (121, 123). Additionally, emerging evidence suggests that commensal microbes contribute to metabolic reprogramming of intestinal TRM populations (124–126). Given that tissue-specific infectious defense is a core function of TRMs, moving beyond LPS will certainly provide new insights into unique TRM metabolic programs used during infectious immunity.
Of the long-lived TRM populations, microglia metabolism is arguably the most studied. Beginning with seminal work from the Barres lab, microglia researchers realized very early on that the CNS environment, including the debris that microglia engulf, the abundant (and unique) lipid species present, and the types of damage that occur, are equally important to informing microglia metabolism as intrinsic programming (63, 64, 127, 128). In some ways, microglia behave similarly to other macrophages, such as the metabolic shift away from TCA Cycle activity/mitochondrial ATP generation towards increased glucose uptake (via upregulated GLUT1/SLC2A1) and breakdown into lactate as well as increased mTOR activity, in response to TLR ligands or inflammatory cytokine stimulation (63, 128, 129). These microglia, termed reactive microglia, are particularly clinically relevant because they have been shown to arise in chronic neurodegenerative diseases, such as Alzheimer’s Disease (AD) (130–135). Interestingly, microglia that populate plaques in AD, termed disease-associated microglia (DAMs) (136), appear to transition from an initial glycolytic, reactive state into an apparent hypometabolic state with significantly altered mTOR activity, which is possibly related to alterations in the phagocytic and/or lipid-sensing function of the AD risk-associated triggering receptor on myeloid cells 2 (TREM2) (130, 131, 134). However, microglia also exhibit potentially unique properties, including the ability to utilize alternative energy sources, which may prove especially important given the restrictive CNS parenchyma (63, 137). An additional unique aspect of the CNS that is likely driving microglia metabolism is the presence of diverse lipid species, the ramifications of which have recently been discussed extensively elsewhere (128). Ultimately, it seems apt to look to the various studies of microglia metabolism as a model for how we should study peripheral TRM metabolism moving forward.
As previously discussed, numerous studies suggest that pro-resolving macrophages, typically defined as IL-4-stimulated, rely extensively on TCA cycle activity and OXPHOS for ATP generation, anti-inflammatory cytokine production, and the establishment of the canonical wound healing response (Figure 2). Intriguingly, the relative importance of glycolysis to IL-4-stimulated macrophage polarization remains controversial (82–84, 138). It is possible that there is some level of net increase in glycolytic flux induced by transitioning from a naïve macrophage to a stimulated state (138). It is also possible that the variation in macrophage response depends on the levels of insulin/insulin-like growth factors derived from serum as well as the dynamics of glucose availability, which can affect glucose transporter expression and thus the ability and reliance on glucose uptake (139–142). Interestingly, macrophages engulfing apoptotic cells (termed ‘efferocytosis’), which is a canonical pre-resolving macrophage function, exhibit dynamic changes in metabolic state depending on the stage of clearance. Specifically, receptor engagement of apoptotic cells induces glucose uptake and aerobic glycolysis which is necessary for rapid ATP generation to polymerize actin and promote internalization of apoptotic cells (143). On the other hand, internalization (and presumably digestion) of apoptotic cells induces fatty acid oxidation (FAO) and OXPHOS, which is required for specific aspects of the pro-resolving response including IL-10 (144). This ultimate dependence on FAO for pro-resolving macrophage function was also observed in peritoneal TRMs in two models, IL-4/IL-4R complex injection and Heligmosomoides polygyrus (H. polygyrus) (86). However, in the latter case, the primary source of lipids was exogenous triacylglycerol instead of apoptotic cells. What the source of lipids are, whether different TRMs require FAO to fuel canonical macrophage function, and if the oxygen and substrate availability unique to different tissues changes pro-resolving TRM function (145), remains unknown.
Pro-resolving macrophages are canonically characterized by the preferential hydrolysis of arginine into ornithine and urea via the enzyme Arg1. Ornithine, together with its essential role in detoxification of ammonia in the urea cycle, is also the entry point into the polyamine synthesis pathway. Interestingly, Puleston and colleagues found that pro-resolving macrophages depend on the polyamine biosynthesis pathway to support TCA Cycle and OXPHOS (89). Intriguingly, the polyamine synthesis product spermidine was consumed in a novel post-translational modification termed ‘hypusination’ – or the addition of amino acid hypusine. The only known eukaryotic protein to be modified by hypusine is translation initiation factor 5A (eIF5A), which in macrophages was necessary for the expression of a subset of mitochondrial respiration proteins (89). Importantly, the IL-4/IL-4R and H. polygyrus models of pro-resolving peritoneal TRM function revealed the importance of eIF5A hypusination in vivo. Similar to in vivo findings related to lipid sources for FAO, TRMs may derive arginine, ornithine, or spermidine from various sources, including via regulated release by (23) or engulfment of apoptotic cells (146).
There is an emerging body of work exploring immune and non-immune homeostatic functions of TRMs, including local and systemic control of organismal metabolism, metabolite release to promote tissue regeneration, and maintenance of tissue homeostasis via organelle clearance. For example, previous studies suggested that liver inflammation, mediated by generation of inflammatory liver TRMs, underlies the presentation of obesity-induced metabolic disease including altered hepatic glucose production, progression to steatohepatitis, and liver fibrosis (147). However, a recent report presents striking contradictory evidence from flies, mice, and human patients (148). Specifically, they found that obesity-induced insulin resistance does not induce pro-inflammatory activation of liver TRMs, but rather produce insulin-like growth factor-binding protein 7 (IGFBP7) which acts as a non-inflammatory immune cell-derived metabolite to induce lipogenesis and gluconeogenesis via insulin receptor signaling. Interestingly, variations in the IGFBP7 isoform appeared to differentially bind the insulin receptor, possibly explaining why some obese patients develop insulin resistance whereas others do not (148). Importantly, this work highlights the possibility of a TRM-specific tissue metabolic circuit functioning independent of canonical immune pathways (149). It is important to highlight that, although the finding that liver TRMs (specifically Kupffer cells) remain ‘non-inflammatory’ during obesity was recently confirmed, Scott and colleagues found that Kupffer cells are lost over time in the obese mouse, replaced by bone marrow-derived macrophages (150). Intriguingly, a fraction of these macrophages express osteopontin and reside in regions of increased desmin, suggesting that these infiltrating macrophages are contributors to liver fibrosis. One final note, unlike the previous study, Scott and colleagues observed that Igfbp7 expression was restricted to the non-hematopoietic compartment, which is consistent with the observed loss of Kupffer cells (150). Whether these differences are simply differences in the model used, or an interesting observation of the changes in tissue dynamics over the course of the disease, remains an important point of future investigation. Nevertheless, it will be interesting to explore how systemic metabolic stress, an ever-growing reality in many countries (151), affects various TRM populations, in particular how TRMs fight to maintain organismal homeostasis within biologically safe parameters and why/how those safeguards fail (152, 153).
One possibility is that TRMs use different transporters and G-protein coupled receptors (GPCRs) to respond to metabolites present in the extracellular milieu. As an illustration, it was recently shown that adipose TRMs respond to extracellular succinate via the GPCR succinate receptor 1 (SUNCR1) (153). Unlike its role in certain contexts, succinate signaling through SUNCR1 maintained metabolic homeostasis across multiple contexts, including in mice fed normal chow, fed high-fat diet, and exposed to cold temperature (a model of adipose tissue-browning). Importantly, these findings also correlated to comparisons between lean and obese patients (153). This is a striking example of how the function of an immunoregulatory metabolite can vary depending on the tissue and context. As discussed below, emerging methods and technologies will facilitate a better understanding of the in situ dynamics of TRM metabolism, including intercellular interactions. In a recent study, Crawford and colleagues used stable isotope tracing-based untargeted metabolomics to investigate how hepatocyte-derived substrates (glucose and ketone bodies) are metabolized by liver TRMs (154). This method allowed for an extensive characterization of liver TRM metabolic pathways used under various contexts, including elucidating a novel role for acetoacetate oxidation in liver TRMs required to fuel the glycosaminoglycan pathway and prevent of liver fibrosis (154).
Two recent papers highlight the importance of the specific tissue microenvironment on the canonical TRM function of tissue repair and regeneration. In the first study, Berardi, Mazzone, and colleagues elucidate a system of muscle regeneration by which muscle TRMs act as sensors of glutamine availability which is a key determinant of successful regeneration. Specifically, upon muscle injury or in aged muscle, glutamine levels fall in the extracellular milieu, inducing TRMs to switch from glutamate oxidation (via glutamate dehydrogenase 1, GLUD1) to increased glutamine synthesis (via glutamine synthetase, GS) and release (155). Satellite cells, the multipotent muscle stem cell, consume TRM-derived glutamine via the transporter SLC1A5 which facilitates their proliferation and differentiation into mature muscle. This circuit was tunable, allowing for both improved and impaired muscle regeneration across multiple in vivo models (155). In the second study, Nave, Saher, and colleagues report that microglia-mediated CNS repair depends on the synthesis of desmosterol (instead of the sterol synthesis end-product cholesterol) from the engulfed myelin (156). Desmosterol, in turn, signals via the liver X receptor (LXR) to induce lipid efflux and secretion of reparative factors to support remyelination. Indeed, inducing sterol synthesis promoted quicker remyelination in vivo (156). Conceptually, each tissue features unique accessory and primary cell types, as highlighted in the above studies, that function collectively to perform the required functions of that tissue. The types of material transferred to TRMs is not restricted to metabolites, as two recent studies found that mitochondria are transferred to or cleared by TRMs in the adipose tissue and the heart as part of tissue homeostatic processes (157, 158). Collectively, these findings highlight exciting and emerging areas of TRM metabolism and biology.
As noted in the beginning of this review, a TRM’s life-long environment, or habitare, is the sum total of factors that are experienced. Two recent reviews make the conjecture that a TRM’s habitare includes time spent in residence. Although this is a relatively understudied aspect of TRM biology, there is at least some evidence to suggest that TRM metabolism is affected during aging. In two separate studies, the Andreasson lab details how aging peritoneal TRMs and microglia exhibit altered, although potentially reversible, changes in metabolism that accompany perturbed homeostatic function (159, 160). In the first study, the authors show that macrophages synthesize nicotinamide adenine dinucleotide (NAD+) via the kynurenine pathway (KP), which is necessary for mitochondrial respiration and canonical pro-resolving macrophage function (159). Intriguingly, the ability to synthesize NAD+ via the KP is decreased in aging peritoneal TRMs but was reversed via overexpression of the KP pathway enzyme nicotinate-nucleotide pyrophosphorylase (QPRT) or via exogenous delivery of the NAD+ precursor nicotinamide mononucleotide (NMN) (159). In the second study, peritoneal TRMs and microglia exhibited an age-dependent perturbation in OXPHOS resulting from increased PGE2-mediated glycogenesis. Increased PGE2 signaling via its cognate receptor EP2 resulted in a maladaptive pro-inflammatory state that altered mouse cognition, which was reversible when inhibited in vivo (160).
One final point worth highlighting when discussing the time TRMs spend in residence is the effect that chronic, low-grade perturbations have on TRM identity. This is best exemplified by recent studies of Kupffer cells in mouse models of metabolic-associated fatty liver disease (MAFLD). Intriguingly, Kupffer cell numbers and/or identity is lost during the course of the MAFLD sequelae non-alcoholic steatohepatitis (NASH) (62, 150, 161, 162). These cells are, at least in part, replaced by monocyte-derived macrophages that appear to seed the liver long-term and assume transcriptional programs similar to those of their long-term TRM counterparts. This is intriguing because these newcomers, despite exhibiting transcriptional, epigenetic, and functional similarities to their long-lived Kupffer cell counterparts (34, 150, 161), appear to have different responses to lipids (including triglycerides) and contribute to NASH progression differently (161, 162). For instance, on one hand, monocyte-derived liver TRMs appear to less efficiently promote storage of triglycerides and exacerbate liver fibrosis (161). On the other hand, mice lacking CCR2, which prevented monocyte-derived liver TRM seeding, resulted in increased liver fibrosis (162). These seemingly contradictory results may actually represent functional evolution of monocyte-derived liver TRMs, ultimately supporting the notion that the time spent in a particular tissue, particularly as the nature of that tissue changes, informs the function and metabolic state of the TRM. Taken together, it is apparent that future work will need to take into consideration the multiple factors that constitute a TRM’s habitare, especially time spent in residence and the changing nature of that residence.
The majority of what we know about macrophage metabolism comes from a combination of in vitro mechanistic and in vivo genetic/small molecule targeting studies. These approaches will continue to be essential moving forward, and the utilization of methods for modeling the appropriate tissue environment and differentiating unique TRM subsets will further advance our understanding of TRM metabolism (163, 164). However, the emergence of better methods and technologies will allow for a holistic understanding of TRM metabolism, including at the single cell level in situ. For an in-depth overview of single cell analysis of cellular metabolism, including statistical methods for analyzing single cell RNA sequencing, we recommend the perspective from Artyomov and Van den Bossche (165). Here, we outline some of the methods and technologies used to study metabolism in vivo that have been or could easily be applied to study TRM metabolism.
Raman Spectroscopy and Fluorescence Imaging. Molecules, especially complex metabolites, exhibit numerous unique properties that can be exploited to study cell metabolic processes. These approaches generally fall under the umbrella of molecular imaging. One such property is the Raman effect (or Raman scattering), which is the inelastic scattering of photons when a molecule is excited by a laser beam. Raman spectroscopy (RS) imaging combines information obtained from this property together with light microscopic information to perform label-free, non-destructive analysis of cellular metabolism. Proof-of-principle studies have shown that RS imaging can be used to image macrophage heme metabolism (166) as well as macrophage fatty acid and lipid metabolism (167). Additionally, deuterium can be used as a stable isotope label of a metabolite of interest, allowing for both analysis of intracellular distribution and metabolic flux in macrophages (167). RS imaging exhibits high sensitivity and specificity for metabolite detection and is capable of rapid imaging speeds (167). On the other hand, despite improved technology and methods, RS imaging continues to suffer from relatively low spatial resolution (167). Despite this caveat, RS imaging still has the potential to analyze cellular metabolism in situ when combined with confocal, multiphoton, or lightsheet microscopy (168–171).
Cellular metabolism is modulated, in large part, by the redox state of the cell. One major determinant of this redox state is the ratio of oxidized and reduced forms of nicotinamide adenine dinucleotide (NAD+/NADH) and nicotinamide adenine dinucleotide phosphate (NADP+/NADPH). Classically, NAD+ is a key cofactor for ATP production via glycolysis (in the cytosol) and OXPHOS (in the mitochondria), whereas NADP+ (and importantly its reduced form NADPH) is a key cofactor for the synthesis of the antioxidant glutathione (GSH) and for anabolic metabolism pathways, such as lipid synthesis. Naturally, there has been much effort put into developing methods for real-time monitoring of NAD(P)/NAD(P)H pool sizes (172, 173), and more recently subcellular localization (174), using genetically-encoded fluorescent protein sensors in live cells and transparent model organisms. Recently, two independent groups reported the development of ratiometric fluorescence probes that allow for specific monitoring of NADP/NADPH (175, 176). The first, Apollo-NADP+, relies on the principle of fluorescence anisotropy (also known as fluorescence polarization), that occurs because of the Förster resonance energy transfer (FRET) that occurs between homologous fluorescence proteins. Fluorescence anisotropy, similar to the anisotropy exhibited by tissues and exploited by magnetic resonance, is when the emitted light from an excited fluorescent protein exhibits unequal polarization across spatial dimensions and has become an important tool for studying protein-protein interactions (177, 178). Apollo-NADP+ functions by monitoring the single photon-induced anisotropy of monomeric (inactive) glucose-6-phosphate dehydrogenase (G6PD) and dimeric (active) G6PD, based on the knowledge that NADP+ binding to G6PD stabilizes G6PD dimers (179). Importantly, Apollo-NADP+ is a direct metric of NADP/NADPH levels and a more rapid indicator of cellular redox state than fluorescent protein reporters of hydrogen peroxide (176). The second collection, termed iNap (175), is a series of pH-resistant, ratiometric sensors based on the circularly permuted YFP NADH/NAD sensor, SoNar (180). Both iNap and SoNar function by fusing the NAD(H) binding domain Rex from Thermus aquaticus, with iNap including modifications to Rex to facilitate binding to NADP(H) instead of NAD(H). Excitingly, both SoNar and iNap were shown to be capable of monitoring subcellular pools in live cells in vitro (175, 181) as well as single cell metabolic function in vivo (175, 182). Finally, as a proof-of-principle, the authors used iNap to demonstrate the ability to rapidly assess NADPH pool size decrease in response to LPS+IFNγ stimulation of macrophages, consistent with the knowledge that inflammatory macrophages consume NADPH to produce superoxide free radicals via NADPH oxidase activity.
The fluorescent protein sensors described above are just a subset of the numerous reporters developed to monitor cellular metabolism in live cells [reviewed in (172)]. As noted, in vivo use of fluorescent protein reporters of metabolic activity has primarily focused on model organisms that can be imaged easily. However, proof-of-principle studies in mice do exist, for instance with both the SoNar and iNap reporters described above in muscle cells of young and aged mice. One limitation relates to how to introduce a genetically-encoded reporter. Both muscle and neurons (another regularly investigated cell type), for instance, are easily electroporated or transduced with adeno-associated viruses (AAV). Macrophages, especially TRMs that are not replenished by bone marrow-derived progenitors, are generally resistant to such approaches. The natural solution is to introduce these reporters into mice using a conditionally-induced transgene strategy. This has historically been a cost-ineffective strategy: however optimized protocols for using CRISPR/Cas9-induced homology-directed repair to introduce such transgenes into fertilized oocytes has made such an endeavor more realistic. It would be interesting to combine NAD/NADH or NADP/NADPH reporter mice together with recently described tools to manipulate these ratios in vivo (183–186) to study TRM redox state and metabolism in vivo.
It is also worth highlighting that NADH (and NADPH) exhibits fluorescence (460 ± 50 nm) when excited by an ultraviolet laser (340 ± 30 nm) or a tunable infrared two-photon laser (750 ± 30 nm) (137, 187, 188). When excited, free NADH has a much shorter fluorescence lifetime than bound NADH, which can be quantified using fluorescence lifetime imaging microscopy (FLIM). Indeed, as noted above, MacVicar and colleagues used two-photon FLIM to demonstrate that microglia can adapt to hypoglycemia by using glutamine to maintain NAD(P)H levels (137). In a separate study, Keely and colleagues provide proof-of-principle evidence that FLIM can be used to monitor NAD(P)H and FAD levels in stromal-resident macrophages, in this case tumor-associated macrophages in the mammary tumor microenvironment (188). Both of these studies were unable to distinguish between NADH and NADPH because of their similarities. A previous study highlighted FLIM methods that distinguish between NADH and NADPH (187). Given the distinct functional importance, future work should incorporate this method. It will be interesting to see if different TRM populations exhibit unique NAD(P)H profiles in situ.
The methods detailed in this review require special equipment or expertise, therefore methods that are more readily available are warranted. Flow cytometry is a gold standard immunological technique, with instruments that are more readily available and have gone down significantly in price. Two recent studies detail methods that use high-throughput multi-parameter flow cytometry to either 1) specifically detecting important metabolic proteins including rate-limiting enzymes and transporters (189) or 2) quantify puromycin uptake as a surrogate of cellular metabolism (190). Unfortunately, both of these methods could possibly require time-consuming and cumbersome tissue digestions to release TRMs, which could ultimately affect the metabolic state of the cell (191). Similar to RNA sequencing-based approaches, these methods are restricted in their ability to directly assess metabolic activity. Nevertheless, these methods provide additional tools to the immunologist’s repertoire that are easy to adapt to assess TRM metabolism.
One final fluorescence-based method that is important to discuss involves the combination of histocytometry and enzyme histochemistry to probe the enzymatic activity of common metabolic pathways in situ (192). Specifically, Haschemi and colleagues took advantage of the knowledge that intact tissues fresh-frozen in a preservative such as OCT remain metabolically active and performed nitroblue tetrazolium chloride (NBT)-based enzymatic essays on tissue sections to assess the glycolytic pathway (via GAPDH activity), pentose phosphate pathway (via G6PD activity), fermentation (via LDH activity), and the TCA cycle (via both IDH3 and SDH activity). Immediately following the enzymatic assay, the same (or serial) tissue sections are stained and analyzed using whole-tissue histocytometry. By combining these two methods, Haschemi and colleagues were able to resolve the metabolic activity of immune and non-immune cells in situ across multiple tissues and tissue environments, including human colon cancer. For instance, the authors observed that macrophages in the tumor microenvironment generally exhibited decreased metabolic activity across all enzymes assessed relative to tissue macrophages in healthy control tissue (192). This method comes with a few caveats. The first caveat relates to NBT itself. NBT is less sensitive to , more susceptible to reduction by tissue reductases, and more susceptible to autoxidation, than alternatives such as dihydroethidium, each of which can result in non-specific NBT fluorescence (193). Additionally, the enzymatic assays are performed at saturating concentrations of substrates and confactors, which may not faithfully reflect the metabolic activity of a cell in its native environment where substrates and cofactors are limiting. Finally, the activity of these enzymes is a measurement of steady state activity and does not necessarily reflect the flux of substrates nor the ultimate outcome of the intermediates and products formed. Nonetheless, this method is an important advance for measuring TRM metabolic activity in situ.
Molecular imaging of metabolism is not limited to light-based approaches. The molecular imaging umbrella includes three techniques that, while technically lacking in spatial resolution, make up for it with high specificity and minimal invasiveness: nuclear magnetic resonance (NMR), single photon emission computed tomography (SPECT), and positron emission tomography (PET). The use of each of these methods to study metabolism have been broadly reviewed in detail elsewhere (194–198). Both PET and SPECT rely on injection of radioactive tracers and detection of gamma ray emission, both allow for dynamic measurements, and both require registration to structural images (typically obtained using computed tomography), yet each has unique advantages and disadvantages (198). PET imaging generally has higher spatial resolution (4-6 mm for human scanners, 1 mm for small animal scanners), although advancements in micro-SPECT technologies have significantly improved this parameter for small animal imaging (10 mm for human scanners, below 1 mm for small animal scanners) (199). Additionally, the time required to acquire a full sequence of PET slices is significantly less than for SPECT. On the other hand, the tracers used for SPECT are significantly more stable than the tracers used for PET (200).
Both PET and SPECT have been used extensively to study macrophages in vivo in both murines and humans (201), owing largely to the advancements made in radiolabeled antibodies (known as radiopharmaceuticals) which allow for significantly enhanced cellular sensitivity and specificity (202). Examples of SPECT tracers that specifically target macrophages include imaging of a radiolabeled translocator protein (TSPO) small molecule in the inflamed ankles of a murine model of rheumatoid arthritis (203), of a second generation variant of the TSPO-targeting small molecule (iodo-DPA-713) in the pancreas, liver, and intestines of mice treated with cerulein (198), and of combined 14C-methionine and 99mTc-methoxyisobutylisonitrile in a murine model of acute myocardial infarction (204). This latter study is an excellent example of the ability to simultaneously label cells and monitor metabolite uptake in vivo using micro-SPECT (205, 206). Examples of PET tracers that specifically target macrophages including imaging of combined 89Zr-Feraheme (FH) and 18F-Fludeoxyglucose (FDG) in non-human primates experiencing acute open wound injury or arthritis (207), of a long-circulating, dextran-coated nanoparticle labeled with 64Cu in atherosclerotic plaques of ApoE-deficient mice (208), and of phospholipid or apoA-I conjugated 89Zr-high-density lipoprotein (HDL) in an orthotopic mouse model of breast cancer (209). For both PET and SPECT, there are now numerous contrast agents and metabolites that can be imaged (210, 211). Given the significant advances already made, we believe future studies will be able to combine advances in macrophage-specific radiolabeling with unique metabolic tracers which will allow for in situ live animal imaging of TRM metabolism.
Although PET/SPECT allow for specific targeting of macrophages, these methods only allow one to measure uptake of a desired metabolite, not whether, how much, and in what way a given substrate is used. However, there are two alternative non-destructive/non-terminal approaches that are capable of measuring each of these parameters in living tissue, both of which rely on the principles of nuclear magnetic resonance (NMR): magnetic resonance imaging (MRI) and magnetic resonance spectroscopy (NMR). The use of these techniques to measure metabolism in vivo have been reviewed in great detail previously (194, 210, 212–215). Both MRI and MRS rely on the detection of energy exchange between the external magnetic field applied to the tissue/sample and atomic nuclei, however they vary in that MRI detects emitted radio frequency (RF) signal whereas MRS detects the chemical shift induced by the magnetic field interacting with electrons that surround the nucleus (“shielding”). Imaging of living tissue using 1H-MRS (proton MRS) has historically been challenging because of the high background signal that comes from water and the typically low abundance of desired metabolites combine to give low signal-to-noise ratio (SNR). However, several advances have led to improvements of MR-based in vivo imaging of cellular metabolism (194, 210, 212, 213). Specifically, magnets with increased field strengths, such as 11.7 T in humans and 21.1 T in rodents (216, 217), the use of body region-specific RF surface coils and MRI cryoprobes, and the use of infusion to introduce hyperpolarized metabolites [e.g., [6-13C, 15N3]-Arginine (218), for reviews, see (194, 210, 212, 213)], have each significantly improved the SNR for MR imaging of living tissue. Because of the ability to take repeated measurements of animals (or humans), hyperpolarized MR will continue to be an important method for studying TRM metabolism in health and disease.
The workhorse for cellular metabolism broadly, and for macrophage biology specifically, is and will likely remain mass spectrometry (MS). The topic of ex vivo isolated tissue and cellular MS using gas chromatography (GC) or liquid chromatography (LC) have been reviewed in detail elsewhere. However we will highlight a series of excellent reviews on the broader technology, methodology, and approaches (from the Rabinowitz lab) (191, 219), the pioneering use of these techniques in mouse and human cancer (reviewed by the DeBerardinis lab) (220), and the application of these methodologies to study immunometabolism, particularly of T cells (reviewed by Jones and colleagues) (164). Together with the improved sensitivities of MS technology, we are excited by emerging accompanying approaches such as chemical isotope labeling nanoflow (nano)LC-MS, which potentially allows for LC-MS detection of thousands of metabolites in the ultralow cell number (e.g., 100-10,000) range (221). Unfortunately, each of the above MS-based methods requires isolation of cells or tissue, including potentially detrimental digestion and isolation steps. This is particularly problematic for TRMs, as noted above, because TRMs are integrally embedded in the tissue stromal meshwork which makes isolation difficult. Here, we briefly highlight three spatial MS imaging approaches that, if adopted and optimized, will allow for unparalleled investigation of in situ TRM metabolismml: MIBI-TOF, Nano-SIMS, and MALDI-MS imaging.
Both multiplexed ion beam-imaging by time of flight (MIBI-TOF) and nanoscale secondary ion mass spectrometry (Nano-SIMS) rely on detection of released secondary ions. For a review of SIMS principles, we recommend the following reviews (222, 223). Briefly, a surface (in this case the tissue section or tissue culture of cells) is rasterized with a focused primary ion beam which induces ejection of secondary ions. These secondary ions are then collected and analyzed for their mass/charge ratio using MS, typically TOF or sector field MS (for Nano-SIMS) but can also be analyzed using a quadrupole mass analyzer. In MIBI-TOF, a tissue of interest is prepared via standard histological approaches such as formalin-fixed, paraffin-embedded (FFPE) or fresh frozen (for immunofluorescence, and subsequently stained using antibodies conjugated to a unique metal isotope similar to those used for mass cytometry (CyTOF) (224). MIBI-TOF is capable of imaging at resolutions down to 260 nm (near-single molecule) with a modest field of view (800 μm x 800 μm). The detail that makes up the strength of MIBI-TOF is also its weakness. Specifically, MIBI-TOF requires antibodies that are capable of recognizing a protein of interest. Importantly, MIBI-TOF was recently used to study human cytotoxic T cells using a panel of metabolic pathway regulatory proteins such as transport proteins and essential enzymes (225). Thus far, MIBI-TOF has been restricted to proteins, although antibodies do exist against some metabolites, therefore it is theoretically possible to exploit these antibodies for MIBI-TOF in the future. On the other hand, Nano-SIMS improves on the successes of TOF-SIMS (226, 227) imaging by reducing the distance between the primary probe and the sample (228) which theoretically allows for subcellular (nanometer) detection of metabolites (222, 223). A beautiful example of the potential of Nano-SIMS was shown by i.v. injection and imaging of 13C-labeled triglyceride-rich lipoproteins or 13C-labeled fatty acids into mice (222). Nano-SIMS images of the cells in a tissue are generated either from independent analysis of single secondary ions (e.g., 12C− 13,C−) or the ratio of secondary ions (e.g., 12C− to 13C−). Arguably the most important feature of Nano-SIMS is its ability to not only analyze metabolite pool sizes, but also analyze isotopic enrichment in situ at both relatively high lateral resolution and high spatial resolution. On the other hand, Nano-SIMS is limited by the number of unique masses that can be analyzed in parallel (up to 7 with the NanoSIMS 50L). One could imagine future TRM research combining NanoSIMS with other in situ methods such as spatial RNAseq or spatial MALDI imaging (see below) to study both cellular and subcellular TRM metabolism across large tissue areas.
Finally, it is important to imaging mass spectrometry (also known as mass spectrometry imaging, or MSI). Over the last several years, numerous techniques and equipment have been developed to perform MSI [reviewed here (229, 230)]. MSI, broadly speaking, is a collection of tools used to study the spatial distribution of molecules of interest (ranging from small metabolites up to more complex glycans, lipids, and proteins) across a region or the entirety of a tissue section. Here, we highlight matrix-assisted laser desorption/ionization (MALDI) MSI, including the recently released MALDI-2 (231), because of its improved sensitivity to small metabolites (including under 100 kDa) and improved spatial resolution (down to ~5-10-microns). Indeed, several modifications have allowed for dramatically improved mass accuracy, including the use of hybrid mass analyzers such as QTOF or Fourier-transform ion cyclotron resonance (FT-ICR) (232, 233). Much of this work has focused on spatial imaging of lipid metabolism down to 10-micron resolution (234, 235), however several proof-of-principle studies have demonstrated its utility to smaller metabolites including glutamine and glucose (236, 237). Importantly, MALDI MSI was also recently shown to be useful for isotope flux analysis in situ of 13C5-glutamine in the intestines (236). MALDI MSI can principally be used on tissue biopsies or whole tissues to perform qualitative MS of hundreds to thousands of metabolites, as well as perform quantitative and isotopic enrichment analyses of metabolites of interest (Figure 3A).
Figure 3 Schematic of spatial metabolomic analysis using MALDI Mass Spectrometry imaging. (A) 1. Isolated whole or sectioned tissue can be analyzed using a combination of untargeted approaches involving various MALDI matrix substances, such as 9-AA, allowing for profiling of a broad range of metabolite sizes and classes. 2. Quantitative analysis of metabolites of interest can be performed using two interconnected approaches: On-tissue spotting and metabolite concentration curves. On-tissue spotting involves taking a slurry of known metabolites (at known concentrations) and dabbing them onto tissues of interest. This allows for an internal reference standard to control for matrix deposition and laser excitation. Metabolite concentration curves allow for quantification of the concentration of a specific metabolite or finite number of metabolites of interest. 2. Metabolic flux analysis can be performed by adopting conventional in vivo infusion of 13C-labeled metabolites, such as uniformly-labeled 13C-Glucose into mice. (B) 1. Samples are cut entirely into serial sections. Interleaved sections are used for MALDI or H&E/immunofluorescence staining. 2. Slides used for MALDI are sprayed with matrix of interest using a standard MALDI matrix sprayer. Up to two slides can be sprayed and run at a given time, and slides with applied matrix should be imaged immediately. 3. Slides are then analyzed using a MALDI MSI (such as the SolariX XR MALDI-FT-ICR instrument, pictured). Slides are analyzed at a desired pixel resolution within the limits of the machine (e.g., 20-micron), meaning that the MALDI laser is rastered over the whole tissue surface, exciting 20-micron regions at a time. 4) Mass spectrometry peaks are subsequently analyzed for identity (see method) and mapped back onto H&E or IF stained slides.
MALDI involves four key steps (Figure 3B). Tissues of interest must be immediately snap frozen, which are then cut onto appropriate slides using a cryostat (1). This step is essential to ensure minimal change in regional cellular distribution or tissue structure as well as to minimize changes in metabolites. Previous groups have also used FFPE tissues, which are fairly common in clinical settings, although report noticeable loss in abundance of desired metabolites. Principally, serial sections would be kept for immunofluorescence staining (and possibly also spatial RNAseq), which is necessary for subsequent cellular localization of identified metabolites. Then, a matrix material is applied to the tissue section using a matrix sprayer (such as the HTX TM-Sprayer) which helps to evenly distribute gaseous matrix substance onto tissues (2). Then, samples coated with matrix substance are rasterized with a laser at the desired pixel size which causes ablation and desorption of the matrix-coated sample (3). In the case of MALDI-2 (such as the one housed in the timsTOF Flex 2), a second laser has been added that allows for a 10x improvement in sensitivity and resolution (down to single cell) (231). Metabolites are ionized and accelerated for identification via MS, for example using FT-ICR (as illustrated in Figure 3B). Finally, data is first processed similar to typical MS studies, including identification of mass-charge ratios (m/z ratio, 4). However, it is worth mentioning that because of the incredible amount of data generated by scanning an entire tissue (or tissue section) coupled together with the need to register these m/z ratios to the individual pixels in an immunofluorescence image, it is likely that novel computational approaches will be required, especially as we begin to generate 3-dimensional renderings of whole tissues as illustrated. We anticipate registration tools, such as those long used for neuroimaging, may help in this endeavor.
In this Review, we have highlighted exciting new findings on tissue-resident macrophage (TRM) metabolism. We define a macrophage’s time spent in a particular environment (or ‘niche’), and the features that define that environment, as its habitare. We believe habitare is apt, because it carries with it a quality that is more than just the cold residence in which one resides, but instead includes the richness and warmth that makes the place one resides in a home. We make the case that TRM metabolism is defined by their early entrance into this habitare and is informed over time by the factors that the TRM comes into contact with, both during homeostasis and inflammation. Clearly John Locke never imagined that one’s environment would imprint at the biological level, as it does for memory. We argue that TRMs are instructed in a similar way, first informed by intrinsic programs but ultimately, and continually, informed by their lived experience. Finally, we highlight the emerging technologies that we think will help move forward this important field of study. We believe that lowered costs, increased availability, and better analytical algorithms will allow for widespread adoption of these techniques, and we look forward to the future work to come.
GZ, PHVS, KRK and JSAP wrote, revised, and approved the manuscript for publication. All authors contributed to the article and approved the submitted version.
This work was supported by NIH NCI 5R00CA237728-03 and the Parker Institute for Cancer Immunotherapy Career Development Award (Perry laboratory), NIH NCI 1R01CA248364-01A1 (Keshari laboratory), and MSKCC Cancer Center Support Grant P30CA008748.
The authors declare that the research was conducted in the absence of any commercial or financial relationships that could be construed as a potential conflict of interest.
We thank members of the Perry and Keshari laboratories for discussions.
1. Locke J. An Essay Concerning Human Understanding. Penguin Classics (1959). Reprint edition (February 1, 1998).
2. Guilliams M, Svedberg FR. Does Tissue Imprinting Restrict Macrophage Plasticity? Nat Immunol (2021) 22:118–27. doi: 10.1038/s41590-020-00849-2
3. Guilliams M, Thierry GR, Bonnardel J, Bajenoff M. Establishment and Maintenance of the Macrophage Niche. Immunity (2020) 52:434–51. doi: 10.1016/j.immuni.2020.02.015
4. Okabe Y, Medzhitov R. Tissue Biology Perspective on Macrophages. Nat Immunol (2016) 17:9–17. doi: 10.1038/ni.3320
5. Blériot C, Chakarov S, Ginhoux F. Determinants of Resident Tissue Macrophage Identity and Function. Immunity (2020) 52:957–70. doi: 10.1016/j.immuni.2020.05.014
6. Gomez Perdiguero E, Klapproth K, Schulz C, Busch K, Azzoni E, Crozet L, et al. Tissue-Resident Macrophages Originate From Yolk-Sac-Derived Erythro-Myeloid Progenitors. Nature (2015) 518:547–51. doi: 10.1038/nature13989
7. Yona S, Kim K-W, Wolf Y, Mildner A, Varol D, Breker M, et al. Fate Mapping Reveals Origins and Dynamics of Monocytes and Tissue Macrophages Under Homeostasis. Immunity (2013) 38:79–91. doi: 10.1016/j.immuni.2012.12.001
8. Hashimoto D, Chow A, Noizat C, Teo P, Beasley MB, Leboeuf M, et al. Tissue-Resident Macrophages Self-Maintain Locally Throughout Adult Life With Minimal Contribution From Circulating Monocytes. Immunity (2013) 38:792–804. doi: 10.1016/j.immuni.2013.04.004
9. Wang A, Luan HH, Medzhitov R. An Evolutionary Perspective on Immunometabolism. Science (2019) 363:eaar3932. doi: 10.1126/science.aar3932
10. Gordon S, Martinez-Pomares L. Physiological Roles of Macrophages. Pflügers Arch - Eur J Physiol (2017) 469:365–74. doi: 10.1007/s00424-017-1945-7
11. Henson PM. Cell Removal: Efferocytosis. Annu Rev Cell Dev Biol (2017) 33:127–44. doi: 10.1146/annurev-cellbio-111315-125315
12. Jacome-Galarza CE, Percin GI, Muller JT, Mass E, Lazarov T, Eitler J, et al. Developmental Origin, Functional Maintenance and Genetic Rescue of Osteoclasts. Nature (2019) 568:541–5. doi: 10.1038/s41586-019-1105-7
13. Tondravi MM, McKercher SR, Anderson K, Erdmann JM, Quiroz M, Maki R, et al. Osteopetrosis in Mice Lacking Haematopoietic Transcription Factor PU.1. Nature (1997) 386:81–4. doi: 10.1038/386081a0
14. Kane H, Lynch L. Innate Immune Control of Adipose Tissue Homeostasis. Trends Immunol (2019) 40:857–72. doi: 10.1016/j.it.2019.07.006
15. A-Gonzalez N, Castrillo A. Origin and Specialization of Splenic Macrophages. Cell Immunol (2018) 330:151–8. doi: 10.1016/j.cellimm.2018.05.005
16. Okabe Y, Medzhitov R. Tissue-Specific Signals Control Reversible Program of Localization and Functional Polarization of Macrophages. Cell (2014) 157:832–44. doi: 10.1016/j.cell.2014.04.016
17. Rosas M, Davies LC, Giles PJ, Liao C-T, Kharfan B, Stone TC, et al. The Transcription Factor Gata6 Links Tissue Macrophage Phenotype and Proliferative Renewal. Science (2014) 344:645–8. doi: 10.1126/science.1251414
18. Lavin Y, Winter D, Blecher-Gonen R, David E, Keren-Shaul H, Merad M, et al. Tissue-Resident Macrophage Enhancer Landscapes are Shaped by the Local Microenvironment. Cell (2014) 159:1312–26. doi: 10.1016/j.cell.2014.11.018
19. Gosselin D, Link VM, Romanoski ,CE, Fonseca GJ, Eichenfield DZ, Spann NJ, et al. Environment Drives Selection and Function of Enhancers Controlling Tissue-Specific Macrophage Identities. Cell (2014) 159:1327–40. doi: 10.1016/j.cell.2014.11.023
20. Roberts AW, Lee BL, Deguine J, John S, Shlomchik MJ, Barton GM. Tissue-Resident Macrophages are Locally Programmed for Silent Clearance of Apoptotic Cells. Immunity (2017) 47:913–27.e916.
21. Blander JM. The Many Ways Tissue Phagocytes Respond to Dying Cells. Immunol Rev (2017) 277:158–73. doi: 10.1111/imr.12537
22. A-Gonzalez N, Quintana JA, García-Silva S, Mazariegos M, González de la Aleja A, Nicolás-Ávila JA, et al. Phagocytosis Imprints Heterogeneity in Tissue-Resident Macrophages. J Exp Med (2017) 214:1281–96. doi: 10.1084/jem.20161375
23. Medina CB, Mehrotra P, Arandjelovic S, Perry JSA, Guo Y, Morioka S, et al. Metabolites Released From Apoptotic Cells Act as Tissue Messengers. Nature (2020) 580:130–5. doi: 10.1038/s41586-020-2121-3
24. Morioka S, Maueröder C, Ravichandran KS. Living on the Edge: Efferocytosis At the Interface of Homeostasis and Pathology. Immunity (2019) 50:1149–62. doi: 10.1016/j.immuni.2019.04.018
25. Yvan-Charvet L, Ivanov S. Metabolic Reprogramming of Macrophages in Atherosclerosis: Is It All About Cholesterol? J Lipid Atheroscler (2020) 9:231–42. doi: 10.12997/jla.2020.9.2.231
26. Tabas I, Bornfeldt KE. Intracellular and Intercellular Aspects of Macrophage Immunometabolism in Atherosclerosis. Circ Res (2020) 126:1209–27. doi: 10.1161/CIRCRESAHA.119.315939
27. van Tuijl J, Joosten LAB, Netea MG, Bekkering S, Riksen NP. Immunometabolism Orchestrates Training of Innate Immunity in Atherosclerosis. Cardiovasc Res (2019) 115:1416–24. doi: 10.1093/cvr/cvz107
28. Tiwari P, Blank A, Cui C, Schoenfelt KQ, Zhou G, Xu Y, et al. Metabolically Activated Adipose Tissue Macrophages Link Obesity to Triple-Negative Breast Cancer. J Exp Med (2019) 216:1345–58. doi: 10.1084/jem.20181616
29. Singer BD, Chandel NS. Immunometabolism of Pro-Repair Cells. J Clin Invest (2019) 129:2597–607. doi: 10.1172/JCI124613
30. Roquilly A, Jacqueline C, Davieau M, Mollé A, Sadek A, Fourgeux C, et al. Alveolar Macrophages are Epigenetically Altered After Inflammation, Leading to Long-Term Lung Immunoparalysis. Nat Immunol (2020) 21:636–48. doi: 10.1038/s41590-020-0673-x
31. Ayata P, Badimon A, Strasburger HJ, Duff MK, Montgomery SE, Loh Y-HE, et al. Epigenetic Regulation of Brain Region-Specific Microglia Clearance Activity. Nat Neurosci (2018) 21:1049–60. doi: 10.1038/s41593-018-0192-3
32. Logie C, Stunnenberg HG. Epigenetic Memory: A Macrophage Perspective. Semin Immunol (2016) 28:359–67. doi: 10.1016/j.smim.2016.06.003
33. Amit I, Winter DR, Jung S. The Role of the Local Environment and Epigenetics in Shaping Macrophage Identity and Their Effect on Tissue Homeostasis. Nat Immunol (2016) 17:18–25. doi: 10.1038/ni.3325
34. Sakai M, Troutman TD, Seidman JS, Ouyang Z, Spann NJ, Abe Y, et al. Liver-Derived Signals Sequentially Reprogram Myeloid Enhancers to Initiate and Maintain Kupffer Cell Identity. Immunity (2019) 51:655–70.e658.
35. Bonnardel J, T’Jonck W, Gaublomme D, Browaeys R, Scott CL, Martens L, et al. Stellate Cells, Hepatocytes, and Endothelial Cells Imprint the Kupffer Cell Identity on Monocytes Colonizing the Liver Macrophage Niche. Immunity (2019) 51:638–54.e639.
36. Scott CL, T’Jonck W, Martens L, Todorov H, Sichien D, Soen B, et al. The Transcription Factor ZEB2 is Required to Maintain the Tissue-Specific Identities of Macrophages. Immunity (2018) 49:312–25.e315.
37. Töröcsik D, Szanto A, Nagy L. Oxysterol Signaling Links Cholesterol Metabolism and Inflammation Via the Liver X Receptor in Macrophages. Mol A Med (2009) 30:134–52. doi: 10.1016/j.mam.2009.02.002
38. Evans TD, Sergin I, Zhang X, Razani B. Modulating Oxysterol Sensing to Control Macrophage Apoptosis and Atherosclerosis. Circ Res (2016) 119:1258–61. doi: 10.1161/CIRCRESAHA.116.310155
39. Dang EV, McDonald JG, Russell DW, Cyster JG. Oxysterol Restraint of Cholesterol Synthesis Prevents Aim2 Inflammasome Activation. Cell (2017) 171:1057–71.e1011.
40. Olkkonen VM. Macrophage Oxysterols and Their Binding Proteins: Roles in Atherosclerosis. Curr Opin lipidology (2012) 23:462–70. doi: 10.1097/MOL.0b013e328356dba0
41. Cyster JG, Dang EV, Reboldi A, Yi T. 25-Hydroxycholesterols in Innate and Adaptive Immunity. Nat Rev Immunol (2014) 14:731–43. doi: 10.1038/nri3755
42. Haldar M, Kohyama M, So A-L, Kc W, Wu X, Briseño CG, et al. Heme-Mediated SPI-C Induction Promotes Monocyte Differentiation Into Iron-Recycling Macrophages. Cell (2014) 156:1223–34. doi: 10.1016/j.cell.2014.01.069
43. Kohyama M, Ise W, Edelson BT, Wilker PR, Hildner K, Mejia C, et al. Role for Spi-C in the Development of Red Pulp Macrophages and Splenic Iron Homeostasis. Nature (2009) 457:318–21. doi: 10.1038/nature07472
44. Kurotaki D, Uede T, Tamura T. Functions and Development of Red Pulp Macrophages. Microbiol Immunol (2015) 59:55–62. doi: 10.1111/1348-0421.12228
45. Bellomo A, Mondor I, Spinelli L, Lagueyrie M, Stewart BJ, Brouilly N, et al. Reticular Fibroblasts Expressing the Transcription Factor WT1 Define a Stromal Niche That Maintains and Replenishes Splenic Red Pulp Macrophages. Immunity (2020) 53:127–42.e127.
46. Shayeghi M, Latunde-Dada GO, Oakhill JS, Laftah AH, Takeuchi K, Halliday N, et al. Identification of an Intestinal Heme Transporter. Cell (2005) 122:789–801. doi: 10.1016/j.cell.2005.06.025
47. Le Blanc S, Garrick MD, Arredondo M. Heme Carrier Protein 1 Transports Heme and is Involved in heme-Fe Metabolism. Am J Physiol Cell Physiol (2012) 302:C1780–5. doi: 10.1152/ajpcell.00080.2012
48. Gordon S, Plüddemann A. Tissue Macrophages: Heterogeneity and Functions. BMC Biol (2017) 15:53. doi: 10.1186/s12915-017-0392-4
49. Zhou X, Franklin RA, Adler M, Jacox JB, Bailis W, Shyer JA, et al. Circuit Design Features of a Stable Two-Cell System. Cell (2018) 172:744–57.e717.
50. Buechler MB, Kim K-W, Onufer EJ, Williams JW, Little CC, Dominguez CX, et al. A Stromal Niche Defined by Expression of the Transcription Factor WT1 Mediates Programming and Homeostasis of Cavity-Resident Macrophages. Immunity (2019) 51:119–30.e115.
51. Gautier EL, Ivanov S, Williams JW, Huang SC, Marcelin G, Fairfax K, et al. Gata6 Regulates Aspartoacylase Expression in Resident Peritoneal Macrophages and Controls Their Survival. J Exp Med (2014) 211:1525–31. doi: 10.1084/jem.20140570
52. Oh M-H, Collins SL, Sun I-H, Tam AJ, Patel CH, Arwood ML, et al. Mtorc2 Signaling Selectively Regulates the Generation and Function of Tissue-Resident Peritoneal Macrophages. Cell Rep (2017) 20:2439–54. doi: 10.1016/j.celrep.2017.08.046
53. Dobie R, Wilson-Kanamori JR, Henderson BEP, Smith JR, Matchett KP, Portman JR, et al. Single-Cell Transcriptomics Uncovers Zonation of Function in the Mesenchyme During Liver Fibrosis. Cell Rep (2019) 29:1832–47.e1838.
54. Kendall TJ, Duff CM, Boulter L, Wilson DH, Freyer E, Aitken S, et al. Embryonic Mesothelial-Derived Hepatic Lineage of Quiescent and Heterogenous Scar-Orchestrating Cells Defined But Suppressed by WT1. Nat Commun (2019) 10:4688. doi: 10.1038/s41467-019-12701-9
55. Bohlen CJ, Bennett FC, Tucker AF, Collins HY, Mulinyawe SB, Barres BA. Diverse Requirements for Microglial Survival, Specification, and Function Revealed by Defined-Medium Cultures. Neuron (2017) 94:759–73.e758. doi: 10.1016/j.neuron.2017.04.043
56. Li Q, Cheng Z, Zhou L, Darmanis S, Neff NF, Okamoto J, et al. Developmental Heterogeneity of Microglia and Brain Myeloid Cells Revealed by Deep Single-Cell RNA Sequencing. Neuron (2019) 101:207–23.e210. doi: 10.1016/j.neuron.2018.12.006
57. Racoosin EL, Swanson JA. M-CSF-induced Macropinocytosis Increases Solute Endocytosis But Not Receptor-Mediated Endocytosis in Mouse Macrophages. J Cell Sci (1992) 102:867–80.
58. Palm W. Metabolic Functions of Macropinocytosis. Philos Trans R Soc Lond B Biol Sci (2019) 374:20180285. doi: 10.1098/rstb.2018.0285
59. Palm W, Park Y, Wright K, Pavlova NN, Tuveson DA, Thompson CB. The Utilization of Extracellular Proteins as Nutrients is Suppressed by Mtorc1. Cell (2015) 162:259–70. doi: 10.1016/j.cell.2015.06.017
60. Aegerter H, Kulikauskaite J, Crotta S, Patel H, Kelly G, Hessel EM, et al. Influenza-Induced Monocyte-Derived Alveolar Macrophages Confer Prolonged Antibacterial Protection. Nat Immunol (2020) 21:145–57. doi: 10.1038/s41590-019-0568-x
61. Devalaraja S, To TKJ, Folkert IW, Natesan R, Alam MZ, Li M, et al. Tumor-Derived Retinoic Acid Regulates Intratumoral Monocyte Differentiation to Promote Immune Suppression. Cell (2020) 180:1098–14.e1016. doi: 10.1016/j.cell.2020.02.042
62. Seidman JS, Troutman TD, Sakai M, Gola A, Spann NJ, Bennett H, et al. Niche-Specific Reprogramming of Epigenetic Landscapes Drives Myeloid Cell Diversity in Nonalcoholic Steatohepatitis. Immunity (2020) 52:1057–74.e1057. doi: 10.1016/j.immuni.2020.04.001
63. Bernier L-P, York EM, MacVicar BA. Immunometabolism in the Brain: How Metabolism Shapes Microglial Function. Trends Neurosci (2020) 43:854–69. doi: 10.1016/j.tins.2020.08.008
64. Ghosh S, Castillo E, Frias ES, Swanson RA. Bioenergetic Regulation of Microglia. Glia (2018) 66:1200–12. doi: 10.1002/glia.23271
65. Lee P, Chandel NS, Simon MC. Cellular Adaptation to Hypoxia Through Hypoxia Inducible Factors and Beyond. Nat Rev Mol Cell Biol (2020) 21:268–83. doi: 10.1038/s41580-020-0227-y
66. Carreau A, El Hafny-Rahbi B, Matejuk A, Grillon C, Kieda C. Why is the Partial Oxygen Pressure of Human Tissues a Crucial Parameter? Small Molecules and Hypoxia. J Cell Mol Med (2011) 15:1239–53. doi: 10.1111/j.1582-4934.2011.01258
67. Roh JS, Sohn DH. Damage-Associated Molecular Patterns in Inflammatory Diseases. Immune Netw (2018) 18:e27–7. doi: 10.4110/in.2018.18.e27
68. Liston A, Masters SL. Homeostasis-Altering Molecular Processes as Mechanisms of Inflammasome Activation. Nat Rev Immunol (2017) 17:208–14. doi: 10.1038/nri.2016.151
69. O’Neill LAJ, Kishton RJ, Rathmell J. A Guide to Immunometabolism for Immunologists. Nat Rev Immunol (2016) 16:553–65. doi: 10.1038/nri.2016.70
70. Caputa G, Castoldi A, Pearce EJ. Metabolic Adaptations of Tissue-Resident Immune Cells. Nat Immunol (2019) 20:793–801. doi: 10.1038/s41590-019-0407-0
71. Buck MD, Sowell RT, Kaech SM, Pearce EL. Metabolic Instruction of Immunity. Cell (2017) 169:570–86. doi: 10.1016/j.cell.2017.04.004
72. Fukuzumi M, Shinomiya H, Shimizu Y, Ohishi K, Utsumi S. Endotoxin-Induced Enhancement of Glucose Influx Into Murine Peritoneal Macrophages Via GLUT1. Infect Immun (1996) 64:108–12. doi: 10.1128/IAI.64.1.108-112.1996
73. Newsholme P, Curi R, Gordon S, Newsholme EA. Metabolism of Glucose, Glutamine, Long-Chain Fatty Acids and Ketone Bodies by Murine Macrophages. Biochem J (1986) 239:121–5. doi: 10.1042/bj2390121
74. Oren R, Farnham AE, Saito K, Milofsky E, Karnovsky ML. Metabolic Patterns in Three Types of Phagocytizing Cells. J Cell Biol (1963) 17:487–501. doi: 10.1083/jcb.17.3.487
75. Tannahill GM, Curtis AM, Adamik J, Palsson-McDermott EM, McGettrick AF, Goel G, et al. Succinate is an Inflammatory Signal That Induces IL-1β Through HIF-1α. Nature (2013) 496:238–42. doi: 10.1038/nature11986
76. O’Neill LA, Pearce EJ. Immunometabolism Governs Dendritic Cell and Macrophage Function. J Exp Med (2016) 213:15–23. doi: 10.1084/jem.20151570
77. Kelly B, O’Neill LAJ. Metabolic Reprogramming in Macrophages and Dendritic Cells in Innate Immunity. Cell Res (2015) 25:771–84. doi: 10.1038/cr.2015.68
78. Muri J, Kopf M. Redox Regulation of Immunometabolism. Nat Rev Immunol (2020). doi: 10.1038/s41577-020-00478-8
79. Cameron AM, Castoldi A, Sanin DE, Flachsmann LJ, Field CS, Puleston DJ, et al. Inflammatory Macrophage Dependence on NAD+ Salvage is a Consequence of Reactive Oxygen Species–Mediated DNA Damage. Nat Immunol (2019) 20:420–32. doi: 10.1038/s41590-019-0336-y
80. Boada-Romero E, Martinez J, Heckmann BL, Green DR. The Clearance of Dead Cells by Efferocytosis. Nat Rev Mol Cell Biol (2020) 21:398–414. doi: 10.1038/s41580-020-0232-1
81. Viola A, Munari F, Sánchez-Rodríguez R, Scolaro T, Castegna A. The Metabolic Signature of Macrophage Responses. Front Immunol (2019) 10:1462–2. doi: 10.3389/fimmu.2019.01462
82. Covarrubias AJ, Aksoylar HI, Yu J, Snyder NW, Worth AJ, Iyer SS, et al. Akt-mTORC1 Signaling Regulates Acly to Integrate Metabolic Input to Control of Macrophage Activation. eLife (2016) 5:e11612.
83. Huang ,SC-C, Smith ,AM, Everts B, Colonna M, Pearce ,EL, Schilling JD, et al. Metabolic Reprogramming Mediated by the Mtorc2-IRF4 Signaling Axis Is Essential for Macrophage Alternative Activation. Immunity (2016) 45:817–30. doi: 10.1016/j.immuni.2016.09.016
84. Wang F, Zhang S, Vuckovic I, Jeon R, Lerman A, Folmes CD, et al. Glycolytic Stimulation is Not a Requirement for M2 Macrophage Differentiation. Cell Metab (2018) 28:463–475.e464. doi: 10.1016/j.cmet.2018.08.012
85. Vats D, Mukundan L, Odegaard JI, Zhang L, Smith KL, Morel CR, et al. Oxidative Metabolism and PGC-1beta Attenuate Macrophage-Mediated Inflammation. Cell Metab (2006) 4:13–24. doi: 10.1016/j.cmet.2006.05.011
86. Huang SC-C, Everts B, Ivanova Y, O’Sullivan D, Nascimento M, Smith AM, et al. Cell-Intrinsic Lysosomal Lipolysis is Essential for Alternative Activation of Macrophages. Nat Immunol (2014) 15:846–55. doi: 10.1038/ni.2956
87. Sanin DE, Matsushita M, Klein Geltink RI, Grzes KM, van Teijlingen Bakker N, Corrado M, et al. Mitochondrial Membrane Potential Regulates Nuclear Gene Expression in Macrophages Exposed to Prostaglandin E2. Immunity (2018) 49:1021–33.e1026. doi: 10.1016/j.immuni.2018.10.011
88. Liu P-S, Wang H, Li X, Chao T, Teav T, Christen S, et al. α-Ketoglutarate Orchestrates Macrophage Activation Through Metabolic and Epigenetic Reprogramming. Nat Immunol (2017) 18:985–94. doi: 10.1038/ni.3796
89. Puleston DJ, Buck MD, Klein Geltink RI, Kyle RL, Caputa G, O’Sullivan D, et al. Polyamines and Eif5a Hypusination Modulate Mitochondrial Respiration and Macrophage Activation. Cell Metab (2019) 30:352–63.e358. doi: 10.1016/j.cmet.2019.05.003
90. Bosurgi L, Cao YG, Cabeza-Cabrerizo M, Tucci A, Hughes LD, Kong Y, et al. Macrophage Function in Tissue Repair and Remodeling Requires IL-4 or IL-13 With Apoptotic Cells. Science (2017) 356:1072–6. doi: 10.1126/science.aai8132
91. Murray PJ, Wynn TA. Obstacles and Opportunities for Understanding Macrophage Polarization. J leukocyte Biol (2011) 89:557–63. doi: 10.1189/jlb.0710409
92. Thomas AC, Mattila JT. “Of Mice and Men”: Arginine Metabolism in Macrophages. Front Immunol (2014) 5:479. doi: 10.3389/fimmu.2014.00479
93. Makowski L, Chaib M, Rathmell JC. Immunometabolismml: From Basic Mechanisms to Translation. Immunol Rev (2020) 295:5–14. doi: 10.1111/imr.12858
94. Carmona-Fontaine C, Deforet M, Akkari L, Thompson CB, Joyce JA, Xavier JB. Metabolic Origins of Spatial Organization in the Tumor Microenvironment. Proc Natl Acad Sci (2017) 114:2934. doi: 10.1073/pnas.1700600114
95. Torretta S, Scagliola A, Ricci L, Mainini F, Di Marco S, Cuccovillo I, et al. D-Mannose Suppresses Macrophage IL-1β Production. Nat Commun (2020) 11:6343. doi: 10.1038/s41467-020-20164-6
96. Littlewood-Evans A, Sarret S, Apfel V, Loesle P, Dawson J, Zhang J, et al. GPR91 Senses Extracellular Succinate Released From Inflammatory Macrophages and Exacerbates Rheumatoid Arthritis. J Exp Med (2016) 213:1655–62. doi: 10.1084/jem.20160061
97. Culemann S, Grüneboom A, Nicolás-Ávila J.Á., Weidner D, Lämmle KF, Rothe T, et al. Locally Renewing Resident Synovial Macrophages Provide a Protective Barrier for the Joint. Nature (2019) 572:670–5.
98. O’Neill LAJ, Artyomov MN. Itaconate: The Poster Child of Metabolic Reprogramming in Macrophage Function. Nat Rev Immunol (2019) 19:273–81. doi: 10.1038/s41577-019-0128-5
99. Bambouskova M, Gorvel L, Lampropoulou V, Sergushichev A, Loginicheva E, Johnson K, et al. Electrophilic Properties of Itaconate and Derivatives Regulate The Iκbζ–ATF3 Inflammatory Axis. Nature (2018) 556:501–4. doi: 10.1038/s41586-018-0052-z
100. Cordes T, Wallace M, Michelucci A, Divakaruni AS, Sapcariu SC, Sousa C, et al. Immunoresponsive Gene 1 and Itaconate Inhibit Succinate Dehydrogenase to Modulate Intracellular Succinate Levels *. J Biol Chem (2016) 291:14274–84. doi: 10.1074/jbc.M115.685792
101. Mills EL, Ryan DG, Prag HA, Dikovskaya D, Menon D, Zaslona Z, et al. Itaconate is an Anti-Inflammatory Metabolite That Activates Nrf2 Via Alkylation of KEAP1. Nature (2018) 556:113–7.
102. Hooftman A, Angiari S, Hester S, Corcoran SE, Runtsch MC, Ling C, et al. The Immunomodulatory Metabolite Itaconate Modifies NLRP3 and Inhibits Inflammasome Activation. Cell Metab (2020) 32:468–78.e467.
103. Lampropoulou V, Sergushichev A, Bambouskova M, Nair S, Vincent ,EE, Loginicheva E, et al. Itaconate Links Inhibition of Succinate Dehydrogenase With Macrophage Metabolic Remodeling and Regulation of Inflammation. Cell Metab (2016) 24:158–66. doi: 10.1016/j.cmet.2016.06.004
104. Swain A, Bambouskova M, Kim H, Andhey PS, Duncan D, Auclair K, et al. Comparative Evaluation of Itaconate and its Derivatives Reveals Divergent Inflammasome and Type I Interferon Regulation in Macrophages. Nat Metab (2020) 2:594–602. doi: 10.1038/s42255-020-0210-0
105. Liao S-T, Han C, Xu D-Q, Fu X-W, Wang J-S, Kong L-Y. 4-Octyl Itaconate Inhibits Aerobic Glycolysis by Targeting GAPDH to Exert Anti-Inflammatory Effects. Nat Commun (2019) 10:5091. doi: 10.1038/s41467-019-13078-5
106. Runtsch MC, O’Neill L. Itaconate Inhibits Alternative Activation of Macrophages by Targeting Janus Kinase. J Immunol (2019) 1:202, 58.11–58.11.
107. Humphries F, Shmuel-Galia L, Ketelut-Carneiro N, Li S, Wang B, Nemmara VV, et al. Succination Inactivates Gasdermin D and Blocks Pyroptosis. Science (2020) 369:1633–7. doi: 10.1126/science.abb9818
108. Zanoni I, Tan Y, Di Gioia M, Springstead JR, Kagan JC. By Capturing Inflammatory Lipids Released From Dying Cells, the Receptor Cd14 Induces Inflammasome-Dependent Phagocyte Hyperactivation. Immunity (2017) 47:697–709.e693.
109. Di Gioia M, Spreafico R, Springstead JR, Mendelson MM, Joehanes R, Levy D, et al. Endogenous Oxidized Phospholipids Reprogram Cellular Metabolism and Boost Hyperinflammation. Nat Immunol (2020) 21:42–53. doi: 10.1038/s41590-019-0539-2
110. Baardman J, Verberk SGS, van der Velden S, Gijbels MJJ, van Roomen CPPA, Sluimer JC, et al. Macrophage ATP Citrate Lyase Deficiency Stabilizes Atherosclerotic Plaques. Nat Commun (2020) 11:6296. doi: 10.1038/s41467-020-20141-z
111. Serbulea V, Upchurch CM, Ahern KW, Bories G, Voigt P, DeWeese DE, et al. Macrophages Sensing Oxidized DAMPs Reprogram Their Metabolism to Support Redox Homeostasis and Inflammation Through a TLR2-Syk-ceramide Dependent Mechanism. Mol Metab (2018) 7:23–34. doi: 10.1016/j.molmet.2017.11.002
112. Serbulea V, Upchurch CM, Schappe MS, Voigt P, DeWeese DE, Desai BN, et al. Macrophage Phenotype and Bioenergetics are Controlled by Oxidized Phospholipids Identified in Lean and Obese Adipose Tissue. Proc Natl Acad Sci United States America (2018) 115:E6254–e6263. doi: 10.1073/pnas.1800544115
113. Brykczynska U, Geigges M, Wiedemann SJ, Dror E, Böni-Schnetzler M, Hess C, et al. Distinct Transcriptional Responses Across Tissue-Resident Macrophages to Short-Term and Long-Term Metabolic Challenge. Cell Rep (2020) 30:1627–1643.e1627.
114. Chi Z, Chen S, Xu T, Zhen W, Yu W, Jiang D, et al. Histone Deacetylase 3 Couples Mitochondria to Drive Il-1β-Dependent Inflammation by Configuring Fatty Acid Oxidation. Mol Cell (2020) 80:43–58.e47.
115. Sanchez-Lopez E, Zhong Z, Stubelius A, Sweeney SR, Booshehri LM, Antonucci L, et al. Choline Uptake and Metabolism Modulate Macrophage Il-1β and IL-18 Production. Cell Metab (2019) 29:1350–1362.e1357.
116. Hsieh W-Y, Zhou QD, York AG, Williams KJ, Scumpia PO, Kronenberger EB, et al. Toll-Like Receptors Induce Signal-Specific Reprogramming of the Macrophage Lipidome. Cell Metab (2020) 32:128–43.e125. doi: 10.1016/j.cmet.2020.05.003
117. Castoldi A, Monteiro LB, van Teijlingen Bakker N, Sanin DE, Rana N, Corrado M, et al. Triacylglycerol Synthesis Enhances Macrophage Inflammatory Function. Nat Commun (2020) 11:4107. doi: 10.1038/s41467-020-17881-3
118. Yu W, Wang Z, Zhang K, Chi Z, Xu T, Jiang D, et al. One-Carbon Metabolism Supports S-Adenosylmethionine and Histone Methylation to Drive Inflammatory Macrophages. Mol Cell (2019) 75:1147–1160.e1145. doi: 10.1016/j.molcel.2019.06.039
119. Rodriguez AE, Ducker GS, Billingham LK, Martinez CA, Mainolfi N, Suri V, et al. Serine Metabolism Supports Macrophage Il-1β Production. Cell Metab (2019) 29:1003–1011.e1004. doi: 10.1016/j.cmet.2019.01.014
120. Ma J, Wei K, Liu J, Tang K, Zhang H, Zhu L, et al. Glycogen Metabolism Regulates Macrophage-Mediated Acute Inflammatory Responses. Nat Commun (2020) 11:1769. doi: 10.1038/s41467-020-15636-8
121. Woods PS, Kimmig LM, Meliton AY, Sun KA, Tian Y, O’Leary EM, et al. Tissue-Resident Alveolar Macrophages Do Not Rely on Glycolysis for LPS-induced Inflammation. Am J Respir Cell Mol Biol (2020) 62:243–55. doi: 10.1165/rcmb.2019-0244OC
122. Ogger PP, Byrne AJ. Macrophage Metabolic Reprogramming During Chronic Lung Disease. Mucosal Immunol (2021) 14:282–95. doi: 10.1038/s41385-020-00356-5
123. Gonçalves SM, Duarte-Oliveira C, Campos CF, Aimanianda V, ter Horst R, Leite L, et al. Phagosomal Removal of Fungal Melanin Reprograms Macrophage Metabolism to Promote Antifungal Immunity. Nat Commun (2020) 11:2282. doi: 10.1038/s41467-020-16120-z
124. Schulthess J, Pandey S, Capitani M, Rue-Albrecht KC, Arnold I, Franchini F, et al. The Short Chain Fatty Acid Butyrate Imprints an Antimicrobial Program in Macrophages. Immunity (2019) 50:432–45.e437. doi: 10.1016/j.immuni.2018.12.018
125. Gabanyi I, Muller PA, Feighery L, Oliveira TY, Costa-Pinto FA, Mucida D. Neuro-Immune Interactions Drive Tissue Programming in Intestinal Macrophages. Cell (2016) 164:378–91. doi: 10.1016/j.cell.2015.12.023
126. Castro-Dopico T, Fleming A, Dennison TW, Ferdinand JR, Harcourt K, Stewart BJ, et al. Gm-Csf Calibrates Macrophage Defense and Wound Healing Programs During Intestinal Infection and Inflammation. Cell Rep (2020) 32:107857. doi: 10.1016/j.celrep.2020.107857
127. Shippy DC, Ulland TK. Microglial Immunometabolism in Alzheimer’s Disease. Front Cell Neurosci (2020) 14:563446. doi: 10.3389/fncel.2020.563446
128. Chausse B, Kakimoto PA, Kann O. Microglia and Lipids: How Metabolism Controls Brain Innate Immunity. Semin Cell Dev Biol (2021) 112:137–44. doi: 10.1016/j.semcdb.2020.08.001
129. York EM, Zhang J, Choi HB, MacVicar BA. Neuroinflammatory Inhibition of Synaptic Long-Term Potentiation Requires Immunometabolic Reprogramming of Microglia. Glia (2020) 69(3):567–78. doi: 10.1002/glia.23913
130. Baik SH, Kang S, Lee W, Choi H, Chung S, Kim J-I, et al. A Breakdown in Metabolic Reprogramming Causes Microglia Dysfunction in Alzheimer’s Disease. Cell Metab (2019) 30:493–507.e496. doi: 10.1016/j.cmet.2019.06.005
131. Kleinberger G, Brendel M, Mracsko E, Wefers B, Groeneweg L, Xiang X, et al. The FTD-like Syndrome Causing TREM2 T66M Mutation Impairs Microglia Function, Brain Perfusion, and Glucose Metabolism. EMBO J (2017) 36:1837–53. doi: 10.15252/embj.201796516
132. Kamphuis W, Kooijman L, Schetters S, Orre M, Hol EM. Transcriptional Profiling of CD11c-positive Microglia Accumulating Around Amyloid Plaques in a Mouse Model for Alzheimer’s Disease. Biochim Biophys Acta (BBA) - Mol Basis Dis (2016) 1862:1847–60. doi: 10.1016/j.bbadis.2016.07.007
133. Johnson ECB, Dammer EB, Duong DM, Ping L, Zhou M, Yin L, et al. Large-Scale Proteomic Analysis of Alzheimer’s Disease Brain and Cerebrospinal Fluid Reveals Early Changes in Energy Metabolism Associated With Microglia and Astrocyte Activation. Nat Med (2020) 26:769–80.
134. Ulland TK, Song WM, Huang SC-C, Ulrich JD, Sergushichev A, Beatty WL, et al. Trem2 Maintains Microglial Metabolic Fitness in Alzheimer's Disease. Cell (2017) 170:649–63.e613. doi: 10.1016/j.cell.2017.07.023
135. Holland R, McIntosh AL, Finucane OM, Mela V, Rubio-Araiz A, Timmons G, et al. Inflammatory Microglia are Glycolytic and Iron Retentive and Typify the Microglia in APP/PS1 Mice. Brain Behav Immun (2018) 68:183–96. doi: 10.1016/j.bbi.2017.10.017
136. Keren-Shaul H, Spinrad A, Weiner A, Matcovitch-Natan O, Dvir-Szternfeld R, Ulland TK, et al. A Unique Microglia Type Associated With Restricting Development of Alzheimer’s Disease. Cell (2017) 169:1276–1290.e1217. doi: 10.1016/j.cell.2017.05.018
137. Bernier L-P, York EM, Kamyabi A, Choi HB, Weilinger NL, MacVicar BA. Microglial Metabolic Flexibility Supports Immune Surveillance of the Brain Parenchyma. Nat Commun (2020) 11:1559. doi: 10.1038/s41467-020-15267-z
138. Fuchs AL, Schiller SM, Keegan WJ, Ammons MCB, Eilers B, Tripet B, et al. Quantitative 1H NMR Metabolomics Reveal Distinct Metabolic Adaptations in Human Macrophages Following Differential Activation. Metabolites (2019) 9:248. doi: 10.3390/metabo9110248
139. Tu C, Fiandalo MV, Pop E, Stocking JJ, Azabdaftari G, Li J, et al. Proteomic Analysis of Charcoal-Stripped Fetal Bovine Serum Reveals Changes in the Insulin-like Growth Factor Signaling Pathway. J Proteome Res (2018) 17:2963–77. doi: 10.1021/acs.jproteome.8b00135
140. Pavlou S, Lindsay J, Ingram R, Xu H, Chen M. Sustained High Glucose Exposure Sensitizes Macrophage Responses to Cytokine Stimuli But Reduces Their Phagocytic Activity. BMC Immunol (2018) 19:24. doi: 10.1186/s12865-018-0261-0
141. Fernandes R, Carvalho AL, Kumagai AK, Seiça R, Hosoya KI, Terasaki T, et al. Downregulation of Retinal GLUT1 in Diabetes by the Ubiquitin Proteasome Pathway. Invest Ophthalmol Visual Sci (2004) 45:3255–5.
142. Mandarino LJ, Finlayson J, Hassell JR. High Glucose Downregulates Glucose Transport Activity in Retinal Capillary Pericytes But Not Endothelial Cells. Invest Ophthalmol Visual Sci (1994) 35:964–72.
143. Morioka S, Perry JSA, Raymond MH, Medina CB, Zhu Y, Zhao L, et al. Efferocytosis Induces a Novel SLC Program to Promote Glucose Uptake and Lactate Release. Nature (2018) 563:714–8. doi: 10.1038/s41586-018-0735-5
144. Zhang S, Weinberg S, DeBerge M, Gainullina A, Schipma M, Kinchen JM, et al. Efferocytosis Fuels Requirements of Fatty Acid Oxidation and the Electron Transport Chain to Polarize Macrophages for Tissue Repair. Cell Metab (2019) 29:443–56.e445.
145. Svedberg FR, Brown SL, Krauss MZ, Campbell L, Sharpe C, Clausen M, et al. The Lung Environment Controls Alveolar Macrophage Metabolism and Responsiveness in Type 2 Inflammation. Nat Immunol (2019) 20:571–80. doi: 10.1038/s41590-019-0352-y
146. Yurdagul A, Subramanian M, Wang X, Crown SB, Ilkayeva OR, Darville L, et al. Macrophage Metabolism of Apoptotic Cell-Derived Arginine Promotes Continual Efferocytosis and Resolution of Injury. Cell Metab (2020) 31:518–33.e510. doi: 10.1016/j.cmet.2020.01.001
147. Lackey DE, Olefsky JM. Regulation of Metabolism by the Innate Immune System. Nat Rev Endocrinol (2016) 12:15–28. doi: 10.1038/nrendo.2015.189
148. Morgantini C, Jager J, Li X, Levi L, Azzimato V, Sulen A, et al. Liver Macrophages Regulate Systemic Metabolism Through non-Inflammatory Factors. Nat Metab (2019) 1:445–59. doi: 10.1038/s42255-019-0044-9
149. Ye J, Medzhitov R. Control Strategies in Systemic Metabolism. Nat Metab (2019) 1:947–57. doi: 10.1038/s42255-019-0118-8
150. Remmerie A, Martens L, Thoné T, Castoldi A, Seurinck R, Pavie B, et al. Osteopontin Expression Identifies a Subset of Recruited Macrophages Distinct From Kupffer Cells in the Fatty Liver. Immunity (2020) 53:641–57.e614. doi: 10.1016/j.immuni.2020.08.004
151. Christ A, Lauterbach M, Latz E. Western Diet and the Immune Systemml: An Inflammatory Connection. Immunity (2019) 51:794–811. doi: 10.1016/j.immuni.2019.09.020
152. Silva HM, Báfica A, Rodrigues-Luiz GF, Chi J, Santos ,P, Reis BS, et al. Vasculature-Associated Fat Macrophages Readily Adapt to Inflammatory and Metabolic Challenges. J Exp Med (2019) 216:786–806. doi: 10.1084/jem.20181049
153. Keiran N, Ceperuelo-Mallafré V, Calvo E, Hernández-Alvarez MI, Ejarque M, Núñez-Roa C, et al. SUCNR1 Controls an Anti-Inflammatory Program in Macrophages to Regulate the Metabolic Response to Obesity. Nat Immunol (2019) 20:581–92. doi: 10.1038/s41590-019-0372-7
154. Puchalska P, Martin SE, Huang X, Lengfeld JE, Daniel B, Graham MJ, et al. Hepatocyte-Macrophage Acetoacetate Shuttle Protects Against Tissue Fibrosis. Cell Metab (2019) 29:383–98.e387. doi: 10.1016/j.cmet.2018.10.015
155. Shang M, Cappellesso F, Amorim R, Serneels J, Virga F, Eelen G, et al. Macrophage-Derived Glutamine Boosts Satellite Cells and Muscle Regeneration. Nature (2020) 587:626–31. doi: 10.1038/s41586-020-2857-9
156. Berghoff SA, Spieth L, Sun T, Hosang L, Schlaphoff L, Depp C, et al. Microglia Facilitate Repair of Demyelinated Lesions Via Post-Squalene Sterol Synthesis. Nat Neurosci (2021) 24:47–60. doi: 10.1038/s41593-020-00757-6
157. Brestoff JR, Wilen CB, Moley JR, Li Y, Zou W, Malvin NP, et al. Intercellular Mitochondria Transfer to Macrophages Regulates White Adipose Tissue Homeostasis and Is Impaired in Obesity. Cell Metab (2021) 33:270–82.e278. doi: 10.1016/j.cmet.2020.11.008
158. Nicolás-Ávila JA, Lechuga-Vieco AV, Esteban-Martínez L, Sánchez-Díaz M, Díaz-García E, Santiago DJ, et al. A Network of Macrophages Supports Mitochondrial Homeostasis in the Heart. Cell (2020) 183:94–109.e123. doi: 10.1016/j.cell.2020.08.031
159. Minhas PS, Liu L, Moon PK, Joshi AU, Dove C, Mhatre S, et al. Macrophage De Novo NAD+ Synthesis Specifies Immune Function in Aging and Inflammation. Nat Immunol (2019) 20:50–63. doi: 10.1038/s41590-018-0255-3
160. Minhas PS, Latif-Hernandez A, McReynolds MR, Durairaj AS, Wang Q, Rubin A, et al. Restoring Metabolism of Myeloid Cells Reverses Cognitive Decline in Ageing. Nature (2021) 590:122–8. doi: 10.1038/s41586-020-03160-0
161. Tran S, Baba I, Poupel L, Dussaud S, Moreau M, Gélineau A, et al. Impaired Kupffer Cell Self-Renewal Alters the Liver Response to Lipid Overload During Non-alcoholic Steatohepatitis. Immunity (2020) 53:627–40.e625. doi: 10.1016/j.immuni.2020.06.003
162. Daemen S, Gainullina A, Kalugotla G, He L, Chan MM, Beals JW, et al. Dynamic Shifts in the Composition of Resident and Recruited Macrophages Influence Tissue Remodeling in NASH. Cell Rep (2021) 34:108626. doi: 10.1016/j.celrep.2020.108626
163. Cantor JR. The Rise of Physiologic Media. Trends Cell Biol (2019) 29:854–61. doi: 10.1016/j.tcb.2019.08.009
164. Kaymak I, Williams KS, Cantor JR, Jones RG. Immunometabolic Interplay in the Tumor Microenvironment. Cancer Cell (2021) 39:28–37. doi: 10.1016/j.ccell.2020.09.004
165. Artyomov MN, Van den Bossche J. Immunometabolism in the Single-Cell Era. Cell Metab (2020) 32:710–25. doi: 10.1016/j.cmet.2020.09.013
166. Dybas J, Grosicki M, Baranska M, Marzec KM. Raman Imaging of Heme Metabolism in Situ in Macrophages and Kupffer Cells. Analyst (2018) 143:3489–98. doi: 10.1039/C8AN00282G
167. Matthäus C, Krafft C, Dietzek B, Brehm BR, Lorkowski S, Popp J. Noninvasive Imaging of Intracellular Lipid Metabolism in Macrophages by Raman Microscopy in Combination With Stable Isotopic Labeling. Anal Chem (2012) 84:8549–56. doi: 10.1021/ac3012347
168. Yue S, Cheng J-X. Deciphering Single Cell Metabolism by Coherent Raman Scattering Microscopy. Curr Opin Chem Biol (2016) 33:46–57. doi: 10.1016/j.cbpa.2016.05.016
169. Høgset H, Horgan CC, Armstrong JPK, Bergholt MS, Torraca V, Chen Q, et al. In Vivo Biomolecular Imaging of Zebrafish Embryos Using Confocal Raman Spectroscopy. Nat Commun (2020) 11:6172. doi: 10.1038/s41467-020-19827-1
170. Shi L, Zheng C, Shen Y, Chen Z, Silveira ES, Zhang L, et al. Optical Imaging of Metabolic Dynamics in Animals. Nat Commun (2018) 9:2995. doi: 10.1038/s41467-018-05401-3
171. Caspers PJ, Bruining HA, Puppels GJ, Lucassen GW, Carter EA. In Vivo Confocal Raman Microspectroscopy of the Skin: Noninvasive Determination of Molecular Concentration Profiles. J Invest Dermatol (2001) 116:434–42. doi: 10.1046/j.1523-1747.2001.01258.x
172. Zhang Z, Cheng X, Zhao Y, Yang Y. Lighting Up Live-Cell and In Vivo Central Carbon Metabolism With Genetically Encoded Fluorescent Sensors. Annu Rev Anal Chem (2020) 13:293–314. doi: 10.1146/annurev-anchem-091619-091306
173. Hung YP, Albeck JG, Tantama M, Yellen G. Imaging Cytosolic NADH-NAD(+) Redox State With a Genetically Encoded Fluorescent Biosensor. Cell Metab (2011) 14:545–54. doi: 10.1016/j.cmet.2011.08.012
174. Cambronne XA, Stewart ML, Kim D, Jones-Brunette AM, Morgan RK, Farrens DL, et al. Biosensor Reveals Multiple Sources for Mitochondrial NAD+. Science (2016) 352:1474–7. doi: 10.1126/science.aad5168
175. Tao R, Zhao Y, Chu H, Wang A, Zhu J, Chen X, et al. Genetically Encoded Fluorescent Sensors Reveal Dynamic Regulation of NADPH Metabolism. Nat Methods (2017) 14:720–8. doi: 10.1038/nmeth.4306
176. Cameron WD, Bui CV, Hutchinson A, Loppnau P, Gräslund S, Rocheleau JV. Apollo-NADP+: A Spectrally Tunable Family of Genetically Encoded Sensors for NADP+. Nat Methods (2016) 13:352–8. doi: 10.1038/nmeth.3764
177. Tramier M, Coppey-Moisan M. Fluorescence Anisotropy Imaging Microscopy for homo-FRET in Living Cells. Methods Cell Biol (2008) 85:395–414. doi: 10.1016/S0091-679X(08)85017-0
178. Lidke DS, Nagy P, Barisas BG, Heintzmann R, Post JN, Lidke KA, et al. Imaging Molecular Interactions in Cells by Dynamic and Static Fluorescence Anisotropy (rFLIM and Emfret). Biochem Soc Trans (2003) 31:1020–7. doi: 10.1042/bst0311020
179. Au SWN, Gover S, Lam VMS, Adams MJ. Human glucose-6-phosphate Dehydrogenase: The Crystal Structure Reveals a Structural NADP+ Molecule and Provides Insights Into Enzyme Deficiency. Structure (2000) 8:293–303. doi: 10.1016/S0969-2126(00)00104-0
180. Zhao Y, Hu Q, Cheng F, Su N, Wang A, Zou Y, et al. SoNar, a Highly Responsive NAD+/NADH Sensor, Allows High-Throughput Metabolic Screening of Anti-tumor Agents. Cell Metab (2015) 21:777–89. doi: 10.1016/j.cmet.2015.04.009
181. Zhao Y, Yang Y. Real-Time and High-Throughput Analysis of Mitochondrial Metabolic States in Living Cells Using Genetically Encoded NAD+/NADH Sensors. Free Radical Biol Med (2016) 100:43–52. doi: 10.1016/j.freeradbiomed.2016.05.027
182. Zhao Y, Wang A, Zou Y, Su N, Loscalzo J, Yang Y. In Vivo Monitoring of Cellular Energy Metabolism Using SoNar, a Highly Responsive Sensor for NAD+/NADH Redox State. Nat Protoc (2016) 11:1345–59. doi: 10.1038/nprot.2016.074
183. Cracan V, Titov DV, Shen H, Grabarek Z, Mootha VK. A Genetically Encoded Tool for Manipulation of NADP(+)/NADPH in Living Cells. Nat Chem Biol (2017) 13:1088–95. doi: 10.1038/nchembio.2454
184. Goodman RP, Markhard AL, Shah H, Sharma R, Skinner OS, Clish CB, et al. Hepatic NADH Reductive Stress Underlies Common Variation in Metabolic Traits. Nature (2020) 583:122–6. doi: 10.1038/s41586-020-2337-2
185. Patgiri A, Skinner OS, Miyazaki Y, Schleifer G, Marutani E, Shah H, et al. An Engineered Enzyme That Targets Circulating Lactate to Alleviate Intracellular NADH:NAD(+) Imbalance. Nat Biotechnol (2020) 38:309–13. doi: 10.1038/s41587-019-0377-7
186. Titov DV, Cracan V, Goodman RP, Peng J, Grabarek Z, Mootha VK. Complementation of Mitochondrial Electron Transport Chain by Manipulation of the NAD+/NADH Ratio. Science (2016) 352:231–5. doi: 10.1126/science.aad4017
187. Blacker TS, Mann ZF, Gale JE, Ziegler M, Bain AJ, Szabadkai G, et al. Separating NADH and NADPH Fluorescence in Live Cells and Tissues Using FLIM. Nat Commun (2014) 5:3936. doi: 10.1038/ncomms4936
188. Szulczewski JM, Inman DR, Entenberg D, Ponik SM, Aguirre-Ghiso J, Castracane J, et al. In Vivo Visualization of Stromal Macrophages Via Label-Free FLIM-based Metabolite Imaging. Sci Rep (2016) 6:25086. doi: 10.1038/srep25086
189. Ahl PJ, Hopkins RA, Xiang WW, Au B, Kaliaperumal N, Fairhurst A-M, et al. Met-Flow, a Strategy for Single-Cell Metabolic Analysis Highlights Dynamic Changes in Immune Subpopulations. Commun Biol (2020) 3:305. doi: 10.1038/s42003-020-1027-9
190. Argüello RJ, Combes AJ, Char R, Gigan J-P, Baaziz AI, Bousiquot E, et al. Scenith: A Flow Cytometry-Based Method to Functionally Profile Energy Metabolism With Single-Cell Resolution. Cell Metab (2020) 32:1063–1075.e1067.
191. Jang C, Chen L, Rabinowitz JD. Metabolomics and Isotope Tracing. Cell (2018) 173:822–37. doi: 10.1016/j.cell.2018.03.055
192. Miller A, Nagy C, Knapp B, Laengle J, Ponweiser E, Groeger M, et al. Exploring Metabolic Configurations of Single Cells Within Complex Tissue Microenvironments. Cell Metab (2017) 26:788–800.e786.
193. Miller FJ, Griendling KK. [20] - Functional Evaluation of Nonphagocytic NAD(P)H Oxidases. Methods Enzymol (2002) Vol. 353:220–33.
194. Comment A, Merritt ME. Hyperpolarized Magnetic Resonance as a Sensitive Detector of Metabolic Function. Biochemistry (2014) 53:7333–57. doi: 10.1021/bi501225t
195. Unterrainer M, Eze C, Ilhan H, Marschner S, Roengvoraphoj O, Schmidt-Hegemann NS, et al. Recent Advances of PET Imaging in Clinical Radiation Oncology. Radiat Oncol (2020) 15:88. doi: 10.1186/s13014-020-01519-1
196. Walrand S, Hesse M, Jamar F. Update on Novel Trends in PET/CT Technology and its Clinical Applications. Br J Radiol (2018) 91:20160534. doi: 10.1259/bjr.20160534
197. Israel O, Pellet O, Biassoni L, De Palma D, Estrada-Lobato E, Gnanasegaran G, et al. Two Decades of SPECT/CT – the Coming of Age of a Technology: An Updated Review of Literature Evidence. Eur J Nucl Med Mol Imaging (2019) 46:1990–2012. doi: 10.1007/s00259-019-04404-6
198. Foss CA, Liu L, Mease RC, Wang H, Pasricha P, Pomper MG. Imaging Macrophage Accumulation in a Murine Model of Chronic Pancreatitis With 125I-Iodo-DPA-713 Spect/Ct. J Nucl Med (2017) 58:1685–90. doi: 10.2967/jnumed.117.189571
199. Khalil MM, Tremoleda JL, Bayomy TB, Gsell W. Molecular SPECT Imaging: An Overview. Int J Mol Imaging (2011) 2011:796025. doi: 10.1155/2011/796025
200. Slomka PJ, Pan T, Berman DS, Germano G. Advances in SPECT and PET Hardware. Prog Cardiovasc Dis (2015) 57:566–78. doi: 10.1016/j.pcad.2015.02.002
201. Van De Wiele C, Sathekge M, Maes A. Targeting Monocytes and Macrophages by Means of SPECT and PET. Q J Nucl Med Mol Imaging Off Publ Ital Assoc Nucl Med (AIMN) [and] Int Assoc Radiopharmacology (IAR) [and] Sect So (2014) 58:269–75.
202. Pacilio M, Lauri C, Prosperi D, Petitti A, Signore A. New SPECT and PET Radiopharmaceuticals for Imaging Inflammatory Diseases: A Narrative Review. Semin Nucl Med (2018) 48:261–76. doi: 10.1053/j.semnuclmed.2017.12.004
203. Peng L, Rongshui Y, Fu S, Zhenyu H, Wentao D, Zhide G, et al. 99mtc-Labeled TSPO Ligand CB86 Targeting Macrophages for Rheumatoid Arthritis SPECT Imaging and Preliminary Evaluation of Anti-Inflammatory Effect. Res Square (2021). Preprint.
204. Mori H, Taki J, Wakabayashi H, Hiromasa T, Inaki A, Ogawa K, et al. Colchicine Treatment Early After Infarction Attenuates Myocardial Inflammatory Response Demonstrated by 14C-Methionine Imaging and Subsequent Ventricular Remodeling by Quantitative Gated SPECT. Ann Nucl Med (2021) 35:253–9. doi: 10.1007/s12149-020-01559-3
205. Taki J, Matsunari I. Metabolic Imaging Using SPECT. Eur J Nucl Med Mol Imaging (2007) 34:34–48. doi: 10.1007/s00259-007-0439-9
206. Taegtmeyer H. Tracing Cardiac Metabolism In Vivo: One Substrate At a Time. J Nucl Med (2010) 51:80S–7S. doi: 10.2967/jnumed.109.068205
207. Wilks M, Yuan H, Josephson L, Normandin M, El Fakhri G. In Vivo PET Imaging of Monocyte/Macrophage Inflammation in Non-Human Primates. J Nucl Med (2019) 60:408–8.
208. Nahrendorf M, Zhang H, Hembrador S, Panizzi P, Sosnovik DE, Aikawa E, et al. Nanoparticle PET-CT Imaging of Macrophages in Inflammatory Atherosclerosis. Circulation (2008) 117:379–87. doi: 10.1161/CIRCULATIONAHA.107.741181
209. Pérez-Medina C, Tang J, Abdel-Atti D, Hogstad B, Merad M, Fisher EA, et al. Pet Imaging of Tumor-Associated Macrophages With 89Zr-Labeled High-Density Lipoprotein Nanoparticles. J Nucl Med Off publ Soc Nucl Med (2015) 56:1272–7. doi: 10.2967/jnumed.115.158956
210. Tee S-S, Keshari KR. Novel Approaches to Imaging Tumor Metabolism. Cancer J (2015) 21:165–73. doi: 10.1097/PPO.0000000000000111
211. Xin Y, Cai H. Improved Radiosynthesis and Biological Evaluations of L- and D-1-[18F]Fluoroethyl-Tryptophan for PET Imaging of IDO-Mediated Kynurenine Pathway of Tryptophan Metabolism. Mol Imaging Biol (2017) 19:589–98. doi: 10.1007/s11307-016-1024-z
212. Keshari KR, Wilson DM. Chemistry and Biochemistry of 13C Hyperpolarized Magnetic Resonance Using Dynamic Nuclear Polarization. Chem Soc Rev (2014) 43:1627–59. doi: 10.1039/C3CS60124B
213. Kurhanewicz J, Vigneron DB, Ardenkjaer-Larsen JH, Bankson JA, Brindle K, Cunningham CH, et al. Hyperpolarized (13)C MRI: Path to Clinical Translation in Oncology. Neoplasia (New York N.Y.) (2019) 21:1–16. doi: 10.1016/j.neo.2018.09.006
214. Li Y, Park I, Nelson SJ. Imaging Tumor Metabolism Using In Vivo Magnetic Resonance Spectroscopy. Cancer J (2015) 21:123–8. doi: 10.1097/PPO.0000000000000097
215. Hyder F, Rothman DL. Advances in Imaging Brain Metabolism. Annu Rev Biomed Eng (2017) 19:485–515. doi: 10.1146/annurev-bioeng-071516-044450
216. Schepkin VD, Brey WW, Gor’kov PL, Grant SC. Initial In Vivo Rodent Sodium and Proton MR Imaging At 21.1 T. Magn Reson Imaging (2010) 28:400–7. doi: 10.1016/j.mri.2009.10.002
217. Ladd ME, Bachert P, Meyerspeer M, Moser E, Nagel AM, Norris DG, et al. Pros and Cons of Ultra-High-Field MRI/MRS for Human Application. Prog Nucl Magn Reson Spectrosc (2018) 109:1–50. doi: 10.1016/j.pnmrs.2018.06.001
218. Cho A, Eskandari R, Granlund KL, Keshari KR. Hyperpolarized [6-13c,15n3]-Arginine as a Probe for in Vivo Arginase Activity. ACS Chem Biol (2019) 14:665–73. doi: 10.1021/acschembio.8b01044
219. Su X, Lu W, Rabinowitz JD. Metabolite Spectral Accuracy on Orbitraps. Anal Chem (2017) 89:5940–8. doi: 10.1021/acs.analchem.7b00396
220. Kaushik AK, DeBerardinis RJ. Applications of Metabolomics to Study Cancer Metabolism. Biochim Biophys Acta (BBA) - Rev Cancer (2018) 1870:2–14. doi: 10.1016/j.bbcan.2018.04.009
221. Luo X, Li L. Metabolomics of Small Numbers of Cells: Metabolomic Profiling of 100, 1000, and 10000 Human Breast Cancer Cells. Anal Chem (2017) 89:11664–71. doi: 10.1021/acs.analchem.7b03100
222. He C, Fong LG, Young SG, Jiang H. NanoSIMS Imaging: An Approach for Visualizing and Quantifying Lipids in Cells and Tissues. J Investig Med (2017) 65:669–72. doi: 10.1136/jim-2016-000239
223. Nuñez J, Renslow R, CliffIII JB, Anderton CR. NanoSIMS for Biological Applications: Current Practices and Analyses. Biointerphases (2018) 13:03B301. doi: 10.1116/1.4993628
224. Keren L, Bosse M, Thompson S, Risom T, Vijayaragavan K, McCaffrey E, et al. Mibi-Tof: A Multiplexed Imaging Platform Relates Cellular Phenotypes and Tissue Structure. Sci Adv (2019) 5:eaax5851. doi: 10.1126/sciadv.aax5851
225. Hartmann FJ, Mrdjen D, McCaffrey E, Glass DR, Greenwald NF, Bharadwaj A, et al. Single-Cell Metabolic Profiling of Human Cytotoxic T Cells. Nat Biotechnol (2021) 39:186–97. doi: 10.1038/s41587-020-0651-8
226. Bich C, Touboul D, Brunelle A. Cluster TOF-SIMS Imaging as a Tool for Micrometric Histology of Lipids in Tissue. Mass spectrom Rev (2014) 33:442–51. doi: 10.1002/mas.21399
227. Fletcher JS. Latest Applications of 3D ToF-SIMS Bio-Imaging. Biointerphases (2015) 10:018902. doi: 10.1116/1.4907727
228. Herrmann AM, Ritz K, Nunan N, Clode PL, Pett-Ridge J, Kilburn MR, et al. Nano-Scale Secondary Ion Mass Spectrometry — A New Analytical Tool in Biogeochemistry and Soil Ecology: A Review Article. Soil Biol Biochem (2007) 39:1835–50. doi: 10.1016/j.soilbio.2007.03.011
229. Buchberger AR, DeLaney K, Johnson J, Li L. Mass Spectrometry Imaging: A Review of Emerging Advancements and Future Insights. Anal Chem (2018) 90:240–65. doi: 10.1021/acs.analchem.7b04733
230. Gilmore IS, Heiles S, Pieterse CL. Metabolic Imaging At the Single-Cell Scale: Recent Advances in Mass Spectrometry Imaging. Annu Rev Anal Chem (2019) 12:201–24. doi: 10.1146/annurev-anchem-061318-115516
231. Niehaus M, Soltwisch J, Belov ME, Dreisewerd K. Transmission-Mode MALDI-2 Mass Spectrometry Imaging of Cells and Tissues At Subcellular Resolution. Nat Methods (2019) 16:925–31. doi: 10.1038/s41592-019-0536-2
232. Kompauer M, Heiles S, Spengler B. Atmospheric Pressure MALDI Mass Spectrometry Imaging of Tissues and Cells At 1.4-μm Lateral Resolution. Nat Methods (2017) 14:90–6. doi: 10.1038/nmeth.4071
233. Spraggins JM, Rizzo DG, Moore JL, Noto MJ, Skaar EP, Caprioli RM. Next-Generation Technologies for Spatial Proteomics: Integrating Ultra-High Speed MALDI-TOF and High Mass Resolution MALDI FTICR Imaging Mass Spectrometry for Protein Analysis. Proteomics (2016) 16:1678–89. doi: 10.1002/pmic.201600003
234. Kettling H, Vens-Cappell S, Soltwisch J, Pirkl A, Haier J, Müthing J, et al. Maldi Mass Spectrometry Imaging of Bioactive Lipids in Mouse Brain With a Synapt G2-s Mass Spectrometer Operated At Elevated Pressure: Improving the Analytical Sensitivity and the Lateral Resolution to Ten Micrometers. Anal Chem (2014) 86:7798–805. doi: 10.1021/ac5017248
235. Ellis SR, Paine MRL, Eijkel GB, Pauling JK, Husen P, Jervelund MW, et al. Automated, Parallel Mass Spectrometry Imaging and Structural Identification of Lipids. Nat Methods (2018) 15:515–8. doi: 10.1038/s41592-018-0010-6
236. Najumudeen AK, Ceteci F, Fey SK, Hamm G, Steven RT, Hall H, et al. The Amino Acid Transporter SLC7A5 is Required for Efficient Growth of KRAS-mutant Colorectal Cancer. Nat Genet (2021) 53:16–26. doi: 10.1038/s41588-020-00753-3
Keywords: tissue-resident macrophages, metabolism, homeostasis, inflammation, development
Citation: Zago G, Saavedra PHV, Keshari KR and Perry JSA (2021) Immunometabolism of Tissue-Resident Macrophages – An Appraisal of the Current Knowledge and Cutting-Edge Methods and Technologies. Front. Immunol. 12:665782. doi: 10.3389/fimmu.2021.665782
Received: 08 February 2021; Accepted: 07 April 2021;
Published: 07 May 2021.
Edited by:
Daniel Puleston, Max Planck Institute for Immunobiology and Epigenetics, GermanyReviewed by:
Charlotte Scott, Flanders Institute for Biotechnology, BelgiumCopyright © 2021 Zago, Saavedra, Keshari and Perry. This is an open-access article distributed under the terms of the Creative Commons Attribution License (CC BY). The use, distribution or reproduction in other forums is permitted, provided the original author(s) and the copyright owner(s) are credited and that the original publication in this journal is cited, in accordance with accepted academic practice. No use, distribution or reproduction is permitted which does not comply with these terms.
*Correspondence: Justin S. A. Perry, cGVycnlqQG1za2NjLm9yZw==
†These authors have contributed equally to this work
Disclaimer: All claims expressed in this article are solely those of the authors and do not necessarily represent those of their affiliated organizations, or those of the publisher, the editors and the reviewers. Any product that may be evaluated in this article or claim that may be made by its manufacturer is not guaranteed or endorsed by the publisher.
Research integrity at Frontiers
Learn more about the work of our research integrity team to safeguard the quality of each article we publish.