- 1Department of Otolaryngology Head and Neck Surgery, Xiangya Hospital, Central South University, Changsha, China
- 2Otolaryngology Major Disease Research Key Laboratory of Hunan Province, Changsha, China
- 3Clinical Research Center for Pharyngolaryngeal Diseases and Voice Disorders in Hunan Province, Xiangya Hospital, Changsha, China
- 4Department of Otorhinolaryngology, The First Affiliated Hospital of University of South China, Hengyang, China
- 5National Clinical Research Center for Geriatric Disorders, Xiangya Hospital, Changsha, China
Myeloid cells are a major heterogeneous cell population in the tumor immune microenvironment (TIME). Imbalance of myeloid response remains a major obstacle to a favorable prognosis and successful immune therapy. Therefore, we aimed to construct a risk model to evaluate the myeloid contexture, which may facilitate the prediction of prognosis and immune infiltration in patients with head and neck squamous cell carcinoma (HNSCC). In our study, six myeloid signature genes (including CCL13, CCR7, CD276, IL1B, LYVE1 and VEGFC) analyzed from 52 differentially expressed myeloid signature genes were finally pooled to establish a prognostic risk model, termed as myeloid gene score (MGS) in a training cohort and validated in a test cohort and an independent external cohort. Furthermore, based on the MGS subgroups, we were able to effectively identify patients with a poor prognosis, aggressive clinical parameters, immune cell infiltration status and immunotherapy response. Thus, MGS may serve as an effective prognostic signature and predictive indicator for immunotherapy response in patients with HNSCC.
Introduction
The tumor immune microenvironment (TIME) has an enormous impact on carcinogenesis, metastasis and progression (1, 2). Diverse immune cells in TIME have conflicting effects, tumor-antagonizing in some cases and tumor-promoting in others (3). Hence, the heterogeneity of immune components is a pivotal factor affecting the interactions between tumor and TIME (4). Myeloid cells are a major heterogeneous cell population in TIME, including dendritic cells (DC), monocytes/macrophages granulocytes and myeloid-derived suppressor cells (MDSCs) (5). Historically, substantial attention has been focused on their role in tumor angiogenesis, invasion and metastasis (6–8). Recently, increasing evidence revealed that myeloid cells are central compartments of immunosuppressive cells, as a major obstacle to immune therapy (6, 9). For example, programmed death-ligand 1 (PD-L1) expression on myeloid cells hampers T cells differentiation into tumor scavenging effector cells (10) and antagonizes the response to anti-PD-1 therapy (11).
Head and neck squamous cell carcinoma (HNSCC) ranks the sixth most common malignant tumor globally, with increasing incidence and over 300,000 deaths annually (12, 13). HNSCC is remarkably heterogeneous. The heterogeneity of HNSCC in part is derived from molecular changes of the parenchyma (1), but also from the intricate and versatile tumor microenvironment (14, 15). In squamous cell carcinomas, the incidence is lower in immunocompetent patients (16), and low immune infiltration has been shown to associate with a poor prognosis (17). Noteworthy, cancer patients with prospering immune responses are more likely to benefit from immune therapy (18). For patients with recurrent and metastatic cancers, anti-PD-1 immunotherapy has been demonstrated to obtain a durable response and overall survival (OS) benefit (19–21). Although HNSCC exhibits higher immune infiltration than other solid tumors (18, 22, 23), only approximately 15% of patients get benefit from treatment responses (19–21). Thus, novel biomarkers need urgent exploration to evaluate the immune status and predict the prognosis and the immunotherapy response. Previously, myeloid cells have been examined individually, generating piecemeal information about their role in the tumor immune response. Nonetheless, the components of myeloid cells are highly heterogeneous and plastic, which indicates that a valid myeloid signature requires integrative analyses.
During the past decades, unveiled massive data were generated by Gene Expression Omnibus (GEO) and The Cancer Genome Atlas (TCGA) (24, 25), providing unique resources for bioinformatics analysis in tumors. In the present study, we first comprehensively investigated the expression pattern and influence on immune infiltration of myeloid signature genes using the TCGA-HNSCC dataset (26) and GEO dataset (GSE65858) (27). Furthermore, a prognostic signature, termed the myeloid gene score (MGS), was constructed and validated as a prognosis biomarker of HNSCC. Besides, our results also suggested that MGS reflected the immune status, infiltration of immune cells in the tumor and response to immunotherapy.
Materials and Methods
HNSCC Datasets Acquisition and Data Processing
The RNA-seq profile data and clinical information of 500 HNSCC and 44 adjacent normal samples (Table S1) including 32 (27.27%) oral samples and 12 (72.73%) larynx samples, were downloaded from the TCGA database (https://portal.gdc.cancer.gov/) and the cBio Cancer Genomics portal (cBioPortal, https://www.cbioportal.org/). For the TCGA dataset, a total of 498 HNSCC samples with prognosis, two cases with duplication or missing follow-up removed, were finally chosen and randomly assigned into a training cohort (Table S2) and a test cohort (Table S3). The GSE65858 dataset from GEO database (https://www.ncbi.nlm.nih.gov/geo/) as an external verification cohort, including microarray and clinical data of 270 HNSCC samples. The TNM stage was determined according to the 7th American Joint Committee on Cancer (AJCC) grading system. Clinical features of HNSCC patients are shown in Table 1. We performed data analysis using R (v4.0.2, https://www.r-project.org/), and batch effects between different datasets were controlled with the “ComBat” algorithm (28).
The differentially expressed myeloid signature genes between HNSCC and normal samples were conducted using the “limma” R package. The cut-off values were set as |Fold change| >1.5 with FDR <0.05. Then, a heat map and a volcano plot were constructed to show the differentially expressed myeloid signature genes.
Construction of MGS
First, the candidate prognostic genes were identified by Univariate Cox regression analysis, based on p <0.05. Subsequently, a prognostic risk model was constructed by Lasso regression analysis in the TCGA training cohort. Then, the risk score, hereafter termed as the MGS, of each patient was calculated as the following formula:
where n is the number of candidate prognostic genes, Coef (genei) is the coefficient of genes determined by Lasso regression analysis, and Expi is the expression value. Ultimately, the HNSCC patients were divided into MGSlow and MGShigh subgroups based on the median value of MGS in the training cohort.
Gene Set Enrichment Analysis
Gene set enrichment analysis (GSEA) is a computational method conducted to elucidate biological functions enriched in gene sets between two states (29). In our study, GSEA was performed by GSEA v4.0 (https://www.gsea-msigdb.org/), with C2 (c2.cp.kegg. v7.1.symbols.gmt) curated gene sets from the Molecular Signatures Database (MSigDB) (30). After 1,000 permutations for each analysis, the results with p <0.05 and FDR <0.25 were considered significantly enriched sets.
Immune Score and Immune Cell Infiltration Analyses
The immune score and stromal score of each sample were calculated in the TCGA dataset using ESTIMATE (Estimation of Stromal and Immune cells in Malignant Tumor tissues using Expression data) algorithm via the “estimate” R package (31). Additionally, the composition fractions of 22 tumor-infiltrating immune cell types in each HNSCC sample were yielded using the CIBERSORT (cell type identification by estimating relative subsets of RNA transcripts) algorithm (32). The samples were adopted according to p <0.05 with 1,000 permutations.
Immunotherapy Datasets
Due to the lack of a database of immunotherapy in HNSCC, metastatic urothelial cancer (mUC) and skin melanoma (SKCM) receiving immunotherapy were adopted and analyzed to evaluate the therapeutic predictive value of MGS. The data package of the mUC dataset was downloaded from http://research-pub.gene.com/IMvigor210CoreBiologies, and preprocessed data with “IMvigor210CoreBiologies” R package (33). The gene expression and clinical data of the SKCM dataset were obtained from the cBio Cancer Genomics portal (https://www.cbioportal.org/). A total of 298 mUC and 80 SKCM patients with immunotherapy were analyzed to evaluate the MGS.
Statistical Analysis
All statistical analyses were carried out using R (v4.0.2). Student’s t-test and one-way ANOVA were performed for comparisons between subgroups. Benjamini–Hochberg multiple testing correction method was used to correct p-values for controlling the false discovery rate (FDR). Survival curves were generated utilizing the Kaplan–Meier method, compared with the log-rank test. Univariate and multivariate analyses were performed with Cox regression to determine the independent prognostic value of the variables. The diagnostic efficiency of the MGS was estimated using ROC curve analysis with overall survival (OS) data based on the endpoint death. The correlation analysis of the variables was performed using the Pearson correlation test. P <0.05 indicates statistically significant differences.
Results
Identification of Differentially Expressed Myeloid Signature Genes
Initially, we evaluated the expression of 83 human myeloid signature genes (Table S4) in 498 HNSCC and 44 adjacent normal tissues. A total of 52 differentially expressed myeloid signature genes were identified (see the schematic workflow in Figure 1), including 43 upregulated and nine downregulated genes. The differentially expressed genes are shown in Table S5 and are displayed with a heat map and a volcano plot (Figures 2A, B).
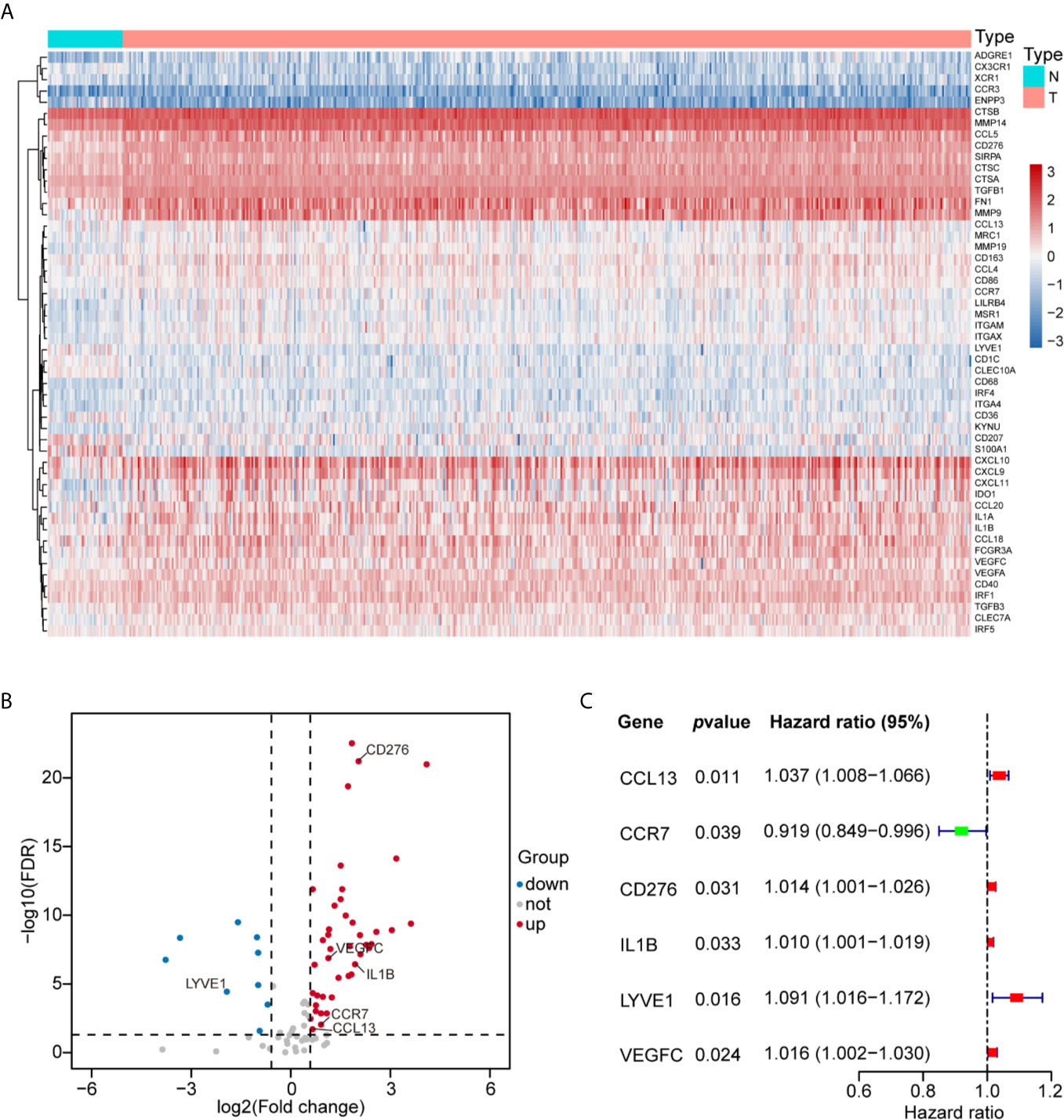
Figure 2 Construction of the MGS with six prognostic myeloid signature genes in HNSCC. (A) Fifty-two differentially expressed myeloid signature genes between normal tissue (N) and tumor tissue (T) are shown in the heat map. (B) Forty-three upregulated and nine downregulated myeloid signature genes are displayed by a volcano plot (|Fold change| >1.5 and FDR <0.05). (C) The six risk myeloid signature genes in the risk model (MGS) are demonstrated by a forest plot.
Construction and Validation of the Prognostic MGS
Then, we evaluated 52 differentially expressed myeloid signature genes in the TCGA training cohort using univariate Cox regression to screen the differentially expressed genes with the prognostic potential to construct a risk model based on the myeloid contexture. Consequently, six prognosis-related myeloid signature genes were identified (Figure 2C), which were used to establish a prognostic risk score (MGS) by LASSO regression analysis (Figure S1). Detailed information and coefficient of the 6 genes are shown in Table 2. MGS of each sample was calculated with the following equation: Risk score (MGS) = CCL13 ∗ 0.0380 + CCR7 ∗ (−0.1049) + CD276 ∗ 0.0073 + IL1B ∗ 0.0034 + LYVE1 ∗ 0.0673 + VEGFC ∗ 0.0020. According to the median value of MGS (0.246), HNSCC patients in the TCGA training and test cohorts were classified into MGSlow and MGShigh subgroups.
Furthermore, OS analysis was performed separately in the TCGA training and test cohorts to evaluate the prognostic value of MGS. In the training cohort, the prognosis of patients in the MGShigh subgroup was markedly worse than patients in the MGSlow subgroup (p <0.001, Figure 3A). The ROC curve analysis indicated that the MGS had a higher ability than other clinical parameters (Figure 3B). The MGS and OS status of patients are depicted in dot plots (Figures 3C, D). The expression patterns of risk genes in the MGSlow and MGShigh subgroups presented with a heat map (Figure 3E), which revealed that high levels of CCL13, CD276, IL1B, LYVE1 and VEGFC acted as risk factors with high MGS, while high expression levels of CCR7 acted as a protective factor with low MGS. Similarly, in the TCGA test cohort and TCGA combining set, the prognoses were also different between the MGSlow and MGShigh subgroups, and the ROC curve analysis also showed that the MGS has a higher ability than other clinical parameters (Figures S2A, B). The relationship between the expression patterns of the 6 genes and MGS was in line with the training cohort (Figures S2C, D).
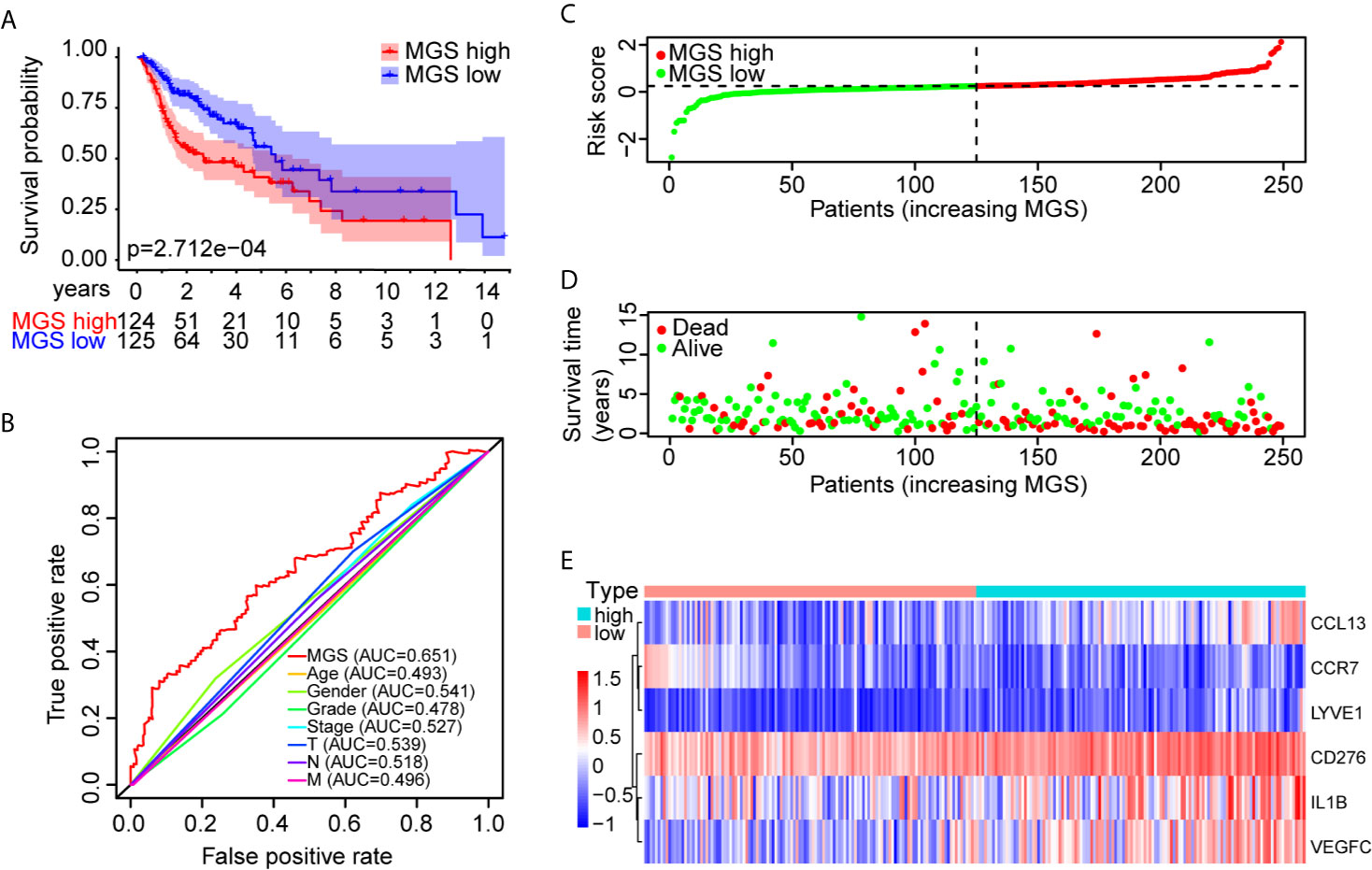
Figure 3 Prognostic performance identification of the MGS in the training cohort. (A) Kaplan–Meier survival curve with OS between the patients of MGSlow and MGShigh subgroups in the TCGA training cohort. (B) The discriminatory power of the MGS and other clinical factors shown by the ROC curve of OS in the training cohort. (C) The distribution of the MGSlow and MGShigh HNSCC patients demonstrated by the risk plot. (D) The survival state of HNSCC patients displayed by the scatter plot. (E) The expression pattern of the six risk genes in the training cohort.
To further validate the prognostic performance of MGS, a GEO data cohort (GSE65858) was adopted as an independent external data cohort. According to the same risk model, patients in the GEO test cohort were also segregated into MGSlow and MGShigh subgroups. As anticipated, prognoses were totally different in these two subgroups (Figure S3A), and MGS also had a higher predictive value than other clinical parameters, except for T stages (Figure S3B). The MGS and OS statuses are depicted using dot plots (Figures S3C, D). The expression profile of the 6 genes was also consistent with the training cohort (Figure S3E). Hence, the above results indicated that MGS was robust in multiple validation data cohorts.
Evaluation of the MGS and the Clinical Parameters
The clinical parameter analysis was performed between the MGSlow and MGShigh subgroups (Figures 4A–F), and the results showed that the MGS of HNSCC patients with Stage III + IV, T3 + 4 and HPV− tumors were higher than those with Stage I + II, T1 + 2 and HPV+ tumors, respectively (p <0.001, p <0.01 and p <0.0001, respectively). Nevertheless, the MGS between the subgroups of Grade, N classification and subsite were not statistically distinct (P = 0.213, P = 0.168 and P = 0.583, respectively). Furthermore, logistic regression was conducted to analyze the association between the MGS and other clinical parameters in the TCGA data cohort (Table 3), which demonstrated that MGS was tightly associated with the stage (p <0.05), T classification (p <0.01) and HPV infection (p <0.0001). The results indicated that MGS was closely related to the progression of HNSCC. Univariate and multivariate analysis of OS was performed with the clinical parameters listed in Table 4. The results showed that MGS was a potential prognostic factor for HNSCC patients.
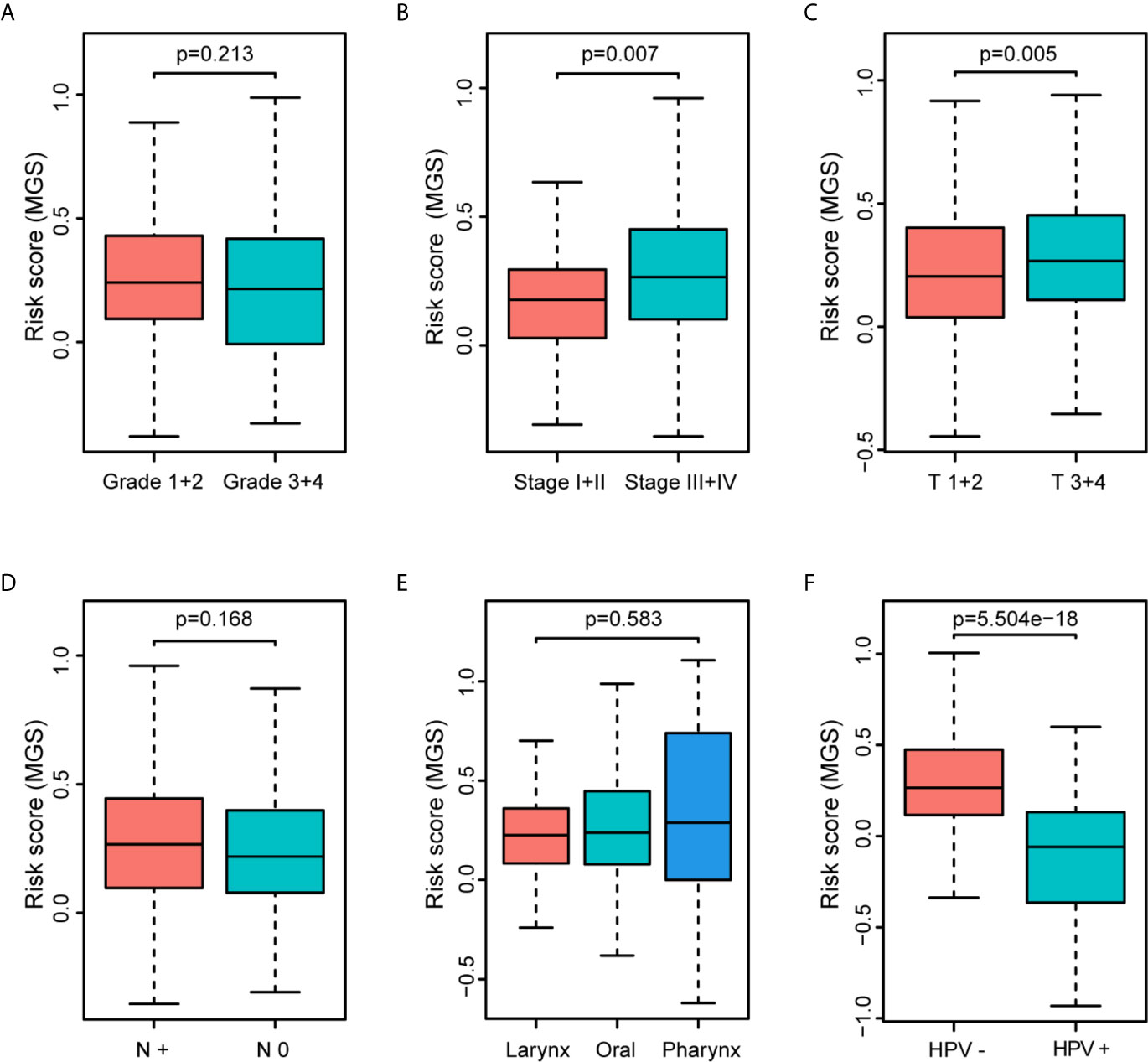
Figure 4 Association of the MGS with clinical parameters of HNSCC patients. Distribution of the MGS stratified by pathologic grade (A), clinical stage (B), T classification (C), N classification (D), subsites (E) and HPV infection (F) in the TCGA data cohort.
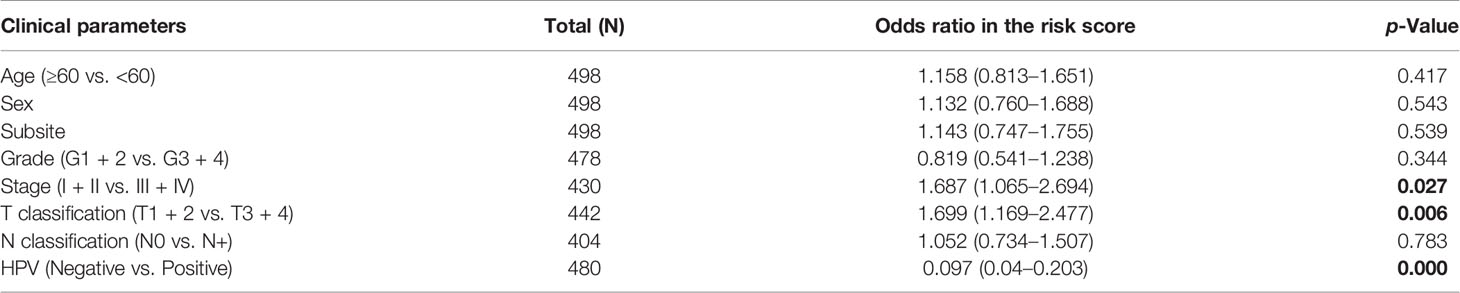
Table 3 Association between the clinical factors and the MGS in HNSCC patients of TCGA data cohort using logistic regression.
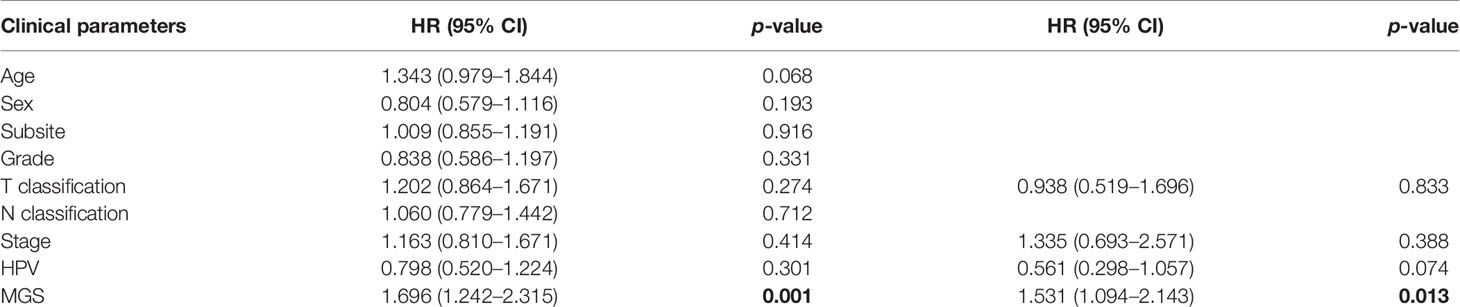
Table 4 Univariate and multivariate Cox regression of overall survival and clinical parameters in TCGA HNSCCs patients.
GSEA of Enriched Pathways in the Subgroups
GSEA was performed to identify the potential function of MGS in MGSlow and MGShigh subgroups in the TCGA data cohort. The top 10 enriched pathways in the MGShigh subgroup and 30 enriched pathways in the MGSlow subgroup were obtained (Table S6), in which signaling pathways with FDR <0.25 and NOM p <0.05 were selected (Table 5). The results indicated that glycometabolism, extracellular matrix (ECM), adhesion and cell cytoskeleton related pathways were enriched in the MGShigh subgroup (Figures 5A, B). However, fatty acid metabolism and immune related pathways were enriched in the MGSlow subgroup (Figures 5C, D). Noteworthy, the B cell and T cell receptor signaling pathway was enriched in the MGSlow subgroup (Figures 5E, F), which suggested that high MGS may be associated with attenuation of B cell and T cell receptor signaling pathways.
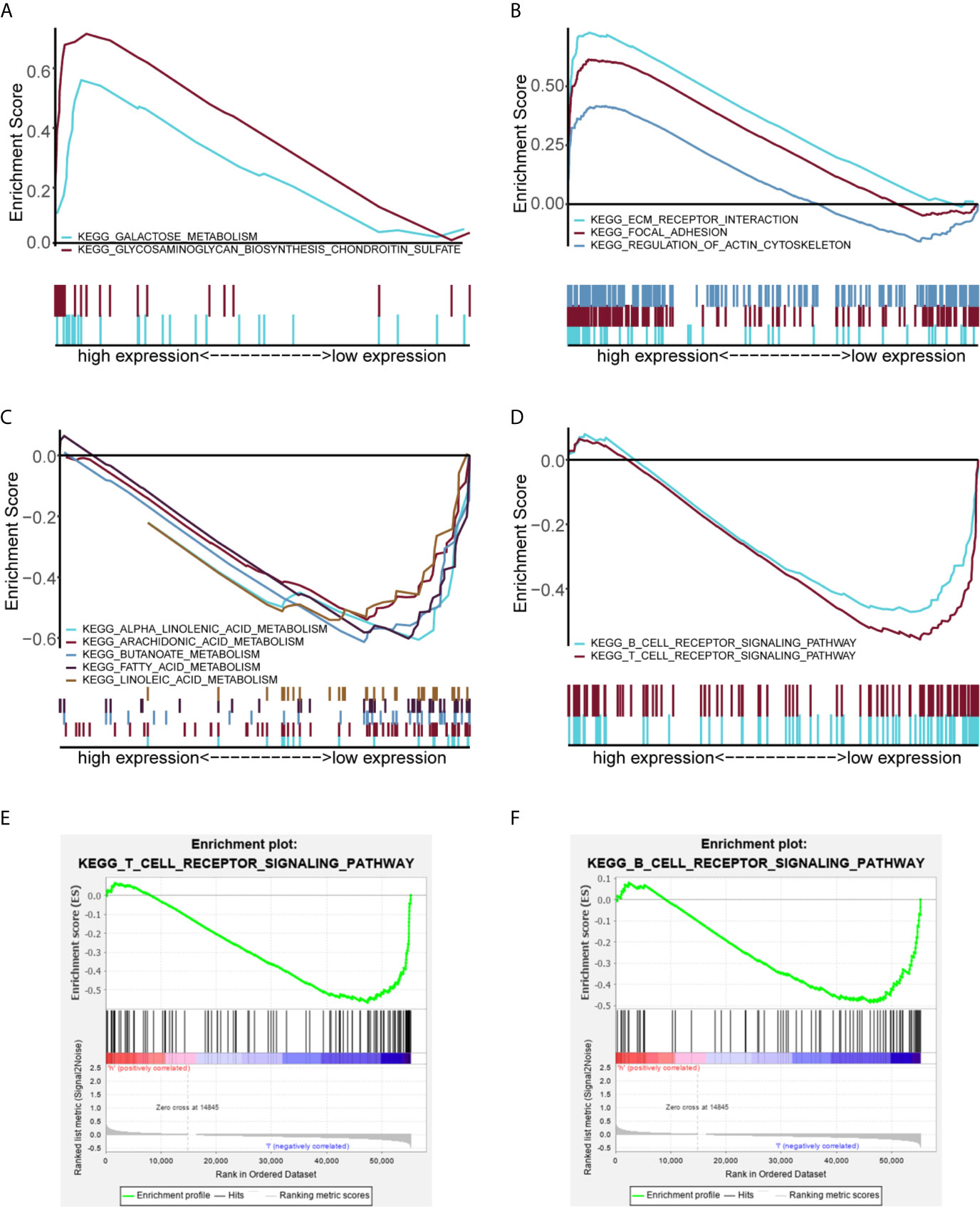
Figure 5 GSEA analysis showing the enriched pathways of the MGSlow and MGShigh subgroups. Multiple GSEA showing glycometabolism related pathways (A) and extracellular matrix (ECM), adhesion and cell cytoskeleton related pathways (B) of the MGShigh subgroup in the TCGA data cohort. Multiple GSEA showing fatty acid metabolism related pathways (C) and immune related pathways (D) of the MGSlow subgroup. Single GSEA showing the B cell receptor signaling pathway (E) and the T cell receptor signaling pathway (F) of the MGSlow subgroup.
Estimation of the MGS With Tumor Immunity
Based on the results of GSEA, MGS was associated with tumor immunity. The immune and stromal scores of the TCGA data cohort were estimated to investigate the effect of MGS on tumor immunity using ESTIMATE. The results showed that the immune score of the MGSlow subgroup was obviously higher than that of the MGShigh subgroup (p <0.01, Figure 6A), and the MGS was negatively correlated with the immune score (R = −0.22, p <0.0001, Figure 6B). In contrast, patients in the MGShigh subgroup had higher stromal scores (p <0.001, Figure 6C), therefore, MGS was positively associated with the stromal score (R = 0.15, p <0.001, Figure 6D).
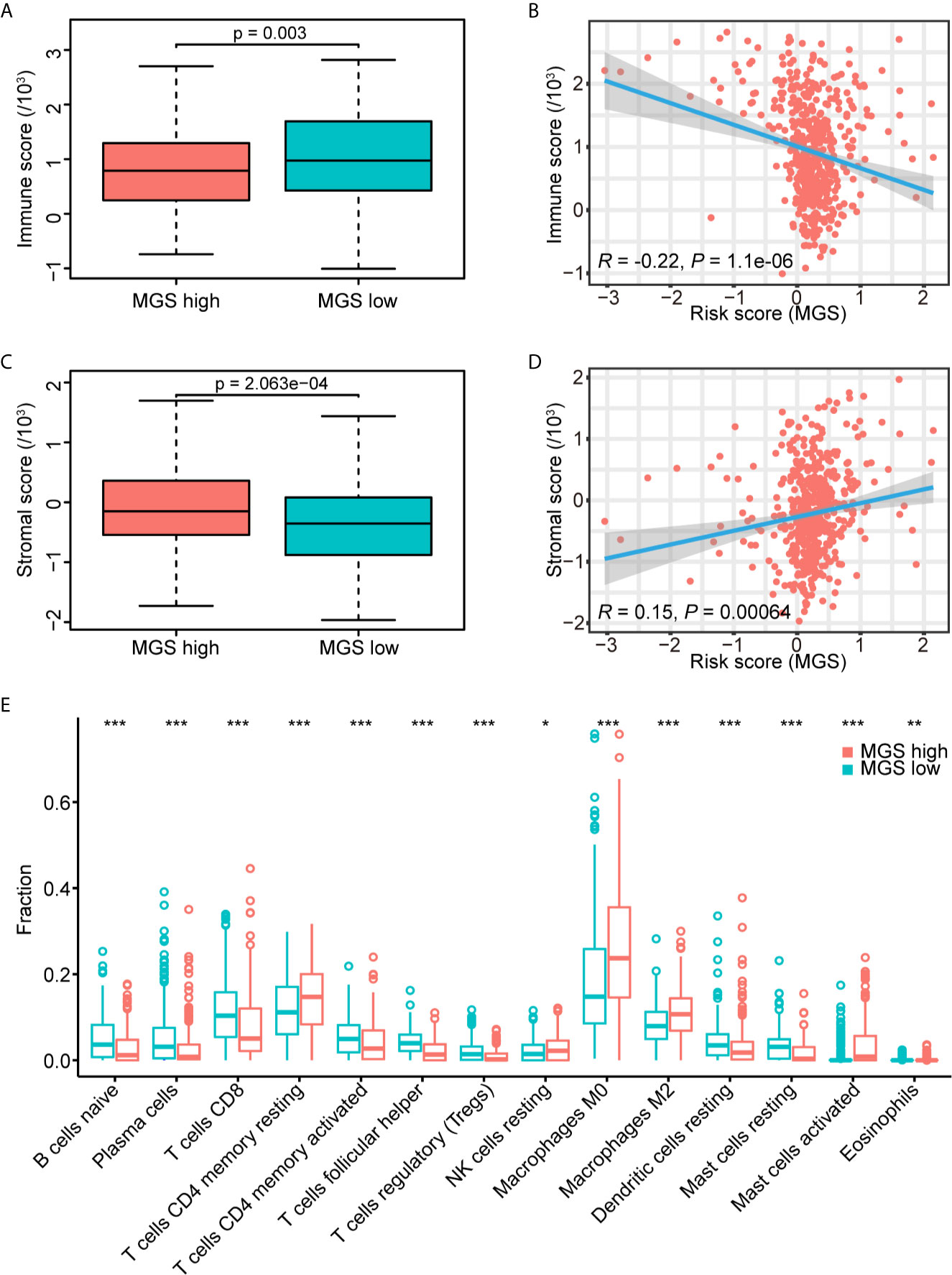
Figure 6 Estimation of the MGS with tumor immunity. (A) Distribution of immune scores in the MGSlow and MGShigh subgroups of the TCGA data cohort. (B) Association between the MGS and immune score in HNSCC patients. (C) Distribution of stromal scores in the MGSlow and MGShigh subgroups. (D) Association between the MGS and stromal score in HNSCC patients. (E) The infiltrating fractions of immune cell types with significant differences in two subgroups. *P < 0.05; **P < 0.01; ***P < 0.001.
Furthermore, the fraction of tumor-infiltrating immune cells in the TCGA data cohort was evaluated using CIBERSORT between MGSlow and MGShigh subgroups (Figure 6E, Figure S4). The results showed that the fraction of macrophages (M0) (p <0.001), alternative macrophages (M2) (p <0.001), activated mast cells (p <0.001) and eosinophils (p <0.01) in the MGShigh subgroup were higher than those in the MGSlow subgroup, and the MGSlow subgroup represented lower resting dendritic cells (p <0.001) and resting mast cells (p <0.001). Consistent with the GSEA results as noted in Figures 5E, F, the fraction of naïve B cells, CD8+ T cells, activated CD4 memory T cells, and follicular helper T cells in the MGShigh subgroup were lower than those in the MGSlow subgroup (all p <0.001), and resting CD4 memory T cells were opposite (p <0.001). These results suggested that high MGS was associated with tumor immunosuppression, potentially caused by attenuation of B cell and T cell proliferation and activation. When HPV+ cases were excluded, the results were somewhat skewed, but basically consistent with the analysis of overall cases (Figure S5).
Association of MGS Genes With the T Cell and B Cell Subpopulations
According to the associations between MGS and the abovementioned five subpopulations of B cells and T cells, we further explored the potential associations of MGS genes and the five subpopulations of B cells and T cells (Figures 7A–F). In line with the expression patterns of the MGS genes, the reduction of naïve B cells was associated with high levels of CD276 (p <0.05), VEGFC (p <0.001), LYVE1 (p <0.001) and low expression of CCR7 (p <0.001). The attenuation of CD8+ T cells was related to a high expression of CD276 (p <0.001), LYVE1 (p <0.001) and low expression of CCR7 (p <0.001). In addition, the decrease of activated CD4 memory T cells was related to the high expression of CD276 (p <0.001), LYVE1 (p <0.05) and low expression of CCR7 (p <0.05). Similarly, the asthenia of follicular helper T cells was linked to high levels of CD276 (p <0.001), VEGFC (p <0.01), LYVE1 (p <0.001), CCL13 (p <0.05) and IL1B (p <0.05), while low levels of CCR7 (p <0.05). Moreover, the increase of resting CD4 memory T cells was associated with high expression of CD276 (p <0.001), VEGFC (p <0.01), LYVE1 (p <0.001), CCL13 (p <0.01) and IL1B (p <0.05). Thus, MGS genes including CD276, CCR7, VEGFC, LYVE1, CCL13 and IL1B were potentially associated with an immunosuppressive status in patients with HNSCC.
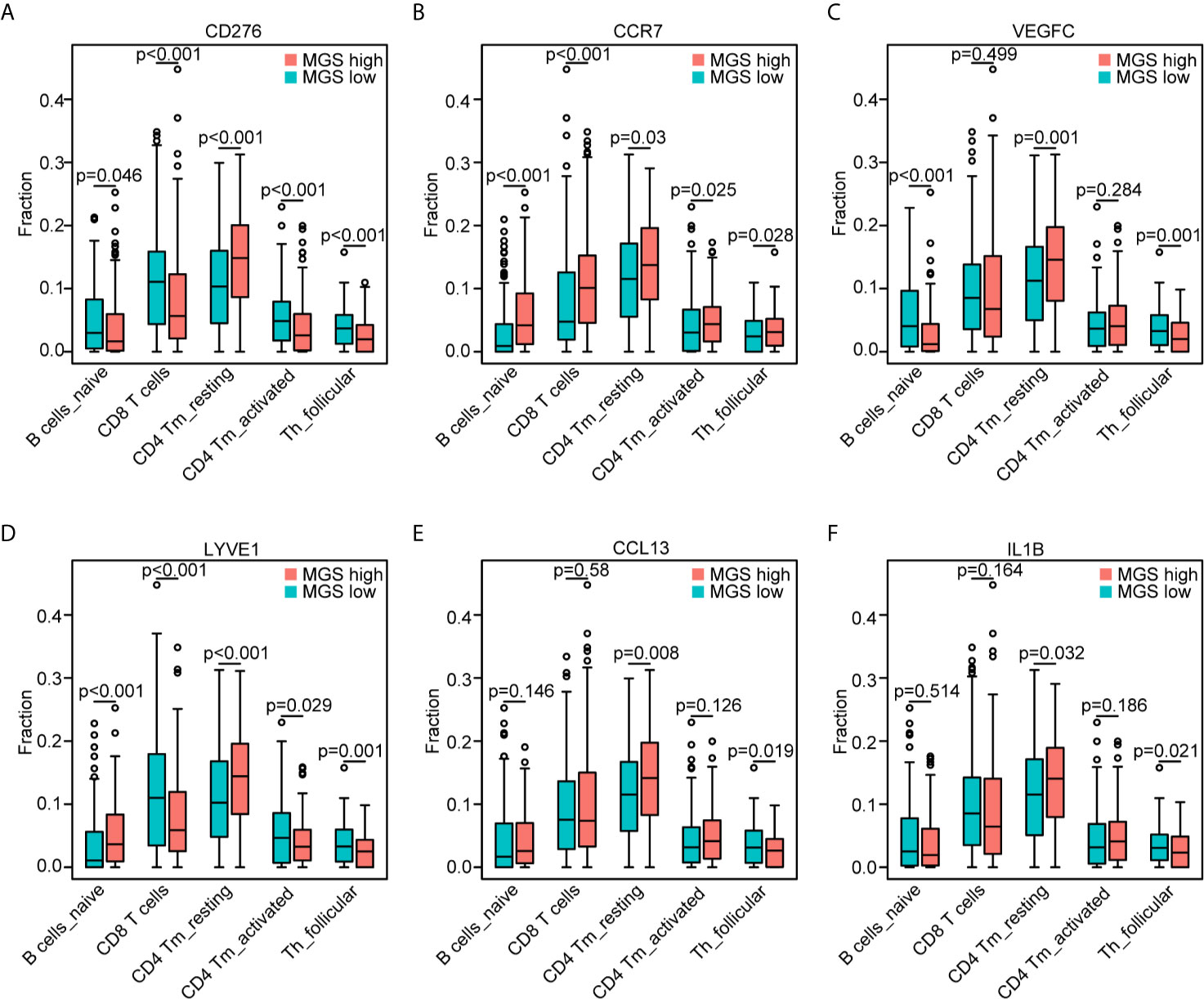
Figure 7 Association of the MGS genes with the T cell and B cell subpopulations. Consistent withFigure 6E, the distribution of the five subpopulations of B cells and T cells based on the high and low expression of CD276 (A), CCR7 (B), VEGFC (C), LYVE1 (D), CCL13 (E) and IL1B (F), respectively.
The Exploration of MGS in Immunotherapeutic Benefits
Emerging immunotherapies for cancer have shown surprising results, but they are only effective in some patients. Hence, we performed subsequent analyses to explore the predictive value of MGS in immunotherapeutic benefits. The mUC and SKCM datasets, which received immunotherapy, were adopted to evaluate the immune score, the expression of PD-L1 and PD1 and response to immunotherapy. Consistently, the immune score of the MGSlow subgroup was elevated compared with the MGShigh subgroup in the SKCM cohort (p <0.0001, Figure S6A). Notably, the results showed that the expression of PD-L1 and PD1 in the MGShigh subgroup was lower than that in the MGSlow subgroup in the SKCM cohort (p <0.01 and p <0.0001, Figures S6B, C). We also found that lower MGS was related to a better response to immunotherapy in the SKCM cohort (Figure S6D). Similar results were similarly obtained in the mUC cohort (Figures S6E–H). Collectively, the results revealed that MGS was associated with patient response to immunotherapy in the SKCM and mUC cohorts.
Discussion
The “hot” and “cold” status of tumors are determined by the infiltration of immune cells, and patients with “cold” tumors have a poor prognosis and benefit less from immunotherapy compared with those with “hot” tumors (34, 35). Myeloid cells, crucial components of the TIME, play a critical role in tumor immune evasion and affect treatment outcome (36). Nonetheless, the component heterogeneity and functional variability of myeloid cells bring a great challenge to distinguish their tumor-antagonizing and tumor-promoting effects in tumors (36, 37). So far, the role of the myeloid cells in HNSCC remains ambiguous, which requires an integrative analysis to explore the expression patterns and prognostic significance of myeloid signature genes, especially their association with TIME in HNSCC patients. Here, for the first time, we comprehensively investigated myeloid signature genes and constructed a myeloid signature MGS to reflect the immune infiltration and immune status in patients with HNSCC.
Myeloid cells are important defenders belonging to the innate immune system and crucial in the orchestration of immune responses. Through directly regulating cancer cells and indirectly impinging the cancer stroma, myeloid cells are multifaceted in cancer progression (36–38). Therefore, integrally evaluating the exact roles of myeloid signature genes in cancers could provide valuable information in the prediction of prognosis and determination of treatment strategies. In our MGS model, high levels of CCL13, CD276, IL1B, LYVE1 and VEGFC were risk factors, while a high level of CCR7 was a protective factor. However, some of the six genes in the heat map by MGSlow and MGShigh subgroups are not very well differentiated, which may be related to poor discriminations between high and low levels of the genes themselves. CD276 (B7-H3), a member of the B7 superfamily, was shown to be overexpressed in colorectal cancer, pancreatic cancer, diffuse brain glioma, non-small cell lung cancer and head and neck cancers (39–43), and enhanced cancer progression (44, 45). Accumulating evidence has demonstrated that elevated expression and polymorphisms of IL1B acted as a crucial risk factor in various cancers, involving cervical cancer, breast cancer, non-small cell lung cancer (46–48). LYVE1 (lymphatic vessel endothelial hyaluronan receptor-1) has been identified as a biomarker of lymphangiogenesis and lymph node metastasis in multiple cancers with poor prognoses (49–51). VEGFC, as previously shown, is a critical determinant in various signaling pathways catalyzing aggressiveness in multiple cancers (52–55). Our results, consistent with the previous studies, indicated that CD276, IL1B, LYVE1 and VEGFC are associated with cancer progression. CCR7 (chemokine receptor 7) can inhibit angiogenesis according to the previous investigation (56, 57), although available studies have shown that it fosters tumor progression (58–60). There are few studies focused on the role of CCL13 in tumors, and for the first time, we explored it as a risk factor for MGS in patients with HNSCC.
Intriguingly, our GSEA data indicated that the B cell receptor (BCR) and T cell receptor (TCR) signaling pathways were enriched in the MGSlow subgroup, which indicated that anti-tumor immune status may be attenuated in the MGShigh subgroup (61–63). Consistently, our study revealed that high MGS was associated with an immunosuppressive status, which was reflected by a diminished immune score and decreased infiltration of naïve B cells, CD8+ T cells, CD4+ memory activated T cells and follicular helper T cells, and elevated infiltration of CD4 memory resting T cells. Meanwhile, the promotion of MGS correlated with the increased infiltration of macrophages (M0), alternative macrophages (M2), activated mast cells and eosinophils. Tumor-associated macrophages (TAMs) composed of multiple subpopulations are generally cataloged as inactivated (M0), classically (M1) and alternatively (M2) activated cells. Indeed, M2 cells can display protumor properties deriving from directly impacting multiple steps in tumorigenesis and development of malignant cells and negatively interacting with other immune cells, involving CD8+ T cells (64–66). Similar to M2 cells, mast cells are often identified as tumor-promoting cells (67–69). Previous studies showed that the attenuation of CD276 can elicit suppression of multiple tumors, which depended on CD8+ T cells (70, 71). The previous study has investigated the tumor immune infiltration characteristics of HNSCC by multiplex immunohistochemistry. The results revealed that differential immune states according to lymphoid and myeloid cell infiltration were associated with HPV infection and prognosis (72). Consistent with our results, CD8+ T cell status correlated with the composition of myeloid populations. Recently, accumulating evidence suggested that immune cell infiltration was closely tied with levels of immune activity in the TIME of HNSCC (15, 73), and immune infiltration could be evaluated using various models constructed by different methods, such as cancer-associated AS events (CASEs) and m6A methylation regulators (74, 75). Some of the myeloid genes in our model have been shown to regulate multiple immune cells by previous studies. IL1B, belonging to the IL1 system, can drive senescence-associated secretory phenotypes (SASPs), which may affect immune cell activation and induce neutrophil accumulation (76, 77). In addition, the shed ectodomain of LYVE1 expressed on M2 cells inhibited cancer cell proliferation (78). Furthermore, VEGFC can catalyze TAMs remodeling and CD8+ T cells deletion leading to immune escape and immune tolerance (79, 80). Hence, MGS on the basis of 6 prognostic myeloid signature genes may afford a method for evaluating “hot tumors” with myeloid contextures and provide new strategies for the optimization of treatment regimens.
In terms of myeloid cells playing a pivotal role in immunotherapy responses (6, 36), patients who received immunotherapy in mUC and SKCM datasets were obtained to evaluate the difference in immunotherapeutic benefits based on MGS status. Our results showed that the immune score of samples with high MGS were also significantly decreasing compared with those samples with low MGS, and patients in the MGShigh subgroup prone to benefit from immunotherapy. These results indicate that the MGS acts as a potential marker to predict immunotherapy response. Therefore, future models utilizing MGS and correlated parameters could facilitate accurately customization of treatment regiments.
However, our investigation has some limitations. Firstly, we performed this study with bioinformatics analysis alone, lacking the validation of solid clinical specimens. The protein expressions of myeloid signature genes in HNSCC at subsites and in different multiplex settings were not verified. Additionally, the research was conducted with a retrospective design rather than a prospective one. The biological basis for MGS differences remains elusive. Furthermore, the heterogeneity of tumor immunity in the subsites of HNSCC deserves further investigation. The immunotherapeutic benefits of MGS were explored with SKCM and mUC datasets, so the evidence for therapeutic response may be insufficient. However, MGS in our study was validated by multiple data cohorts in HNSCC, therefore, our risk model is still reliable and acceptable. Thus, future studies with prospective clinical trials and mechanistic exploration are warranted to further validate the present result and unravel the mechanism modulating the myeloid contexture, however, the evaluation of which will be time-consuming.
In conclusion, our study demonstrated that a novel risk model constructed by six myeloid signature genes could predict prognosis, and assess the myeloid contexture and immune status of HNSCC patients. Moreover, the MGS can facilitate the screening of appropriate candidates for immunotherapy and the development of optimal treatment strategies.
Data Availability Statement
Publicly available datasets were analyzed in this study. This data can be found here: Expression profile and clinical data of HNSCC datasets can be downloaded from The Cancer Genome Atlas (TCGA) (https://portal.gdc.cancer.gov/) and Gene Expression Omnibus (GEO) database (https://www.ncbi.nlm.nih.gov/geo/). The mUC dataset was downloaded from http://research-pub.gene.com/IMvigor210CoreBiologies. The gene expression and clinical data of SKCM dataset can be obtained from the cBio Cancer Genomics portal (https://www.cbioportal.org/).
Author Contributions
YL and XZ designed the study and approved the manuscript. ZL contributed to the data analysis and the manuscript writing. HC, DZ, CL, GL, HL, DH, XW, and FZ conducted data interpretations and depicted the figures. All authors contributed to the article and approved the submitted version.
Funding
This study was supported by the National Natural Science Foundation of China (Nos. 82073009, 81974424, 81874133, 81773243 and 81772903), the National Key Research and Development Project of China (Nos. 2020YFC1316900, 2020YFC1316901), the Natural Science Foundation of Hunan Province (Nos. 2019JJ40481, 2019JJ50944 and 2019JJ50547), the Huxiang Young Talent Project (No. 2018RS3024) and the Fundamental Research Funds for the Central South University (No. 1053320182447).
Conflict of Interest
The authors declare that the research was conducted in the absence of any commercial or financial relationships that could be construed as a potential conflict of interest.
Supplementary Material
The Supplementary Material for this article can be found online at: https://www.frontiersin.org/articles/10.3389/fimmu.2021.659184/full#supplementary-material
Supplementary Figure 1 | LASSO regression analysis conducted to construct the MGS. (A) Expression of 6 risk genes in peritumor and intratumor in HNSCC of TCGA data cohort. (B) LASSO algorithms used to identify prognosis-related myeloid signature genes. (C) LASSO coefficient values used to construct the MGS in the training cohort. (D) Correlation between the 6 risk genes and the MGS. (P < 0.05; Spearman rank correlation).
Supplementary Figure 2 | Verification of the MGS in HNSCC patients with the TCGA data set. (A) The prognostic performance of the MGS in the TCGA test cohort. (B) The risk plot distribution, survival status, and the expression of six genes in the TCGA test cohort. (C) The prognostic performance of the MGS in the TCGA all cohort. (D) The risk plot distribution, survival status, and expression of six genes in the TCGA all cohort.
Supplementary Figure 3 | Validation of the MGS in HNSCC patients using the GEO data cohort. (A) The prognoses in the MGSlow and MGShigh subgroups of the GEO data cohort. (B) The predictive performance of the MGS and other clinical factors shown by the ROC curve in the GEO data cohort. (C) The risk score distribution of MGSlow and MGShigh subgroups. (D) Scatter plot showing the survival statuses of HNSCC patients. (E) The expression of six genes in the GEO data cohort.
Supplementary Figure 4 | Immune cells infiltration in subgroups of the TCGA data cohort. The infiltrating fractions of 22 immune cell types in the MGSlow and MGShigh subgroups.
Supplementary Figure 5 | Estimation of the MGS with immune infiltration in the HNSCC patients excluding the HPV+ cases. The infiltrating fractions of immune cell types with significant differences in the MGSlow and MGShigh subgroups.
Supplementary Figure 6 | The value of the MGS in the prediction of immunotherapeutic benefits. (A) Distribution of immune scores in subgroups of the SKCM cohort. The expression distribution of PD-L1 (B) and PD1 (C) in subgroups of the SKCM cohort. (D) Rate of response, stable disease (SD)/progressive disease (PD) and complete response (CR)/partial response (PR), to immunotherapy in subgroups of the SKCM cohort. (E) Distribution of immune scores in subgroups of the mUC cohort. The expression distribution of PD-L1 (F) and PD1 (G) in subgroups of the mUC cohort. (H) Rate of response, SD/PD and CR/PR, to immunotherapy in subgroups of the mUC cohort.
Table S1 | The list of 44 adjacent normal samples in the TCGA- HNSCC dataset.
Table S2 | The HNSCC patients of the TCGA training cohort.
Table S3 | The HNSCC patients of the TCGA test cohort.
Table S4 | The list of Myeloid signature genes.
Table S5 | Fifty-two differentially expressed Myeloid signature genes.
Table S6 | The top 10 and 30 enriched pathways in MGSlow and MGShigh subgroups, respectively.
References
1. Leemans CR, Snijders PJF, Brakenhoff RH. The Molecular Landscape of Head and Neck Cancer. Nat Rev Cancer (2018) 18(5):269–82. doi: 10.1038/nrc.2018.11
2. Quail DF, Joyce JA. Microenvironmental Regulation of Tumor Progression and Metastasis. Nat Med (2013) 19(11):1423–37. doi: 10.1038/nm.3394
3. Kim IS, Zhang XHF. One Microenvironment Does Not Fit All: Heterogeneity Beyond Cancer Cells. Cancer Metastasis Rev (2016) 35(4):601–29. doi: 10.1007/s10555-016-9643-z
4. Grabovska Y, Mackay A, O’Hare P, Crosier S, Finetti M, Schwalbe EC, et al. Pediatric Pan-Central Nervous System Tumor Analysis of Immune-Cell Infiltration Identifies Correlates of Antitumor Immunity. Nat Commun (2020) 11(1):4324. doi: 10.1038/s41467-020-18070-y
5. Bassler K, Schulte-Schrepping J, Warnat-Herresthal S, Aschenbrenner AC, Schultze JL. The Myeloid Cell Compartment-Cell by Cell. Annu Rev Immunol (2019) 37:269–93. doi: 10.1146/annurev-immunol-042718-041728
6. Gabrilovich DI, Ostrand-Rosenberg S, Bronte V. Coordinated Regulation of Myeloid Cells by Tumours. Nat Rev Immunol (2012) 12(4):253–68. doi: 10.1038/nri3175
7. Qian B-Z, Pollard JW. Macrophage Diversity Enhances Tumor Progression and Metastasis. Cell (2010) 141(1):39–51. doi: 10.1016/j.cell.2010.03.014
8. Biswas SK, Mantovani A. Macrophage Plasticity and Interaction With Lymphocyte Subsets: Cancer as a Paradigm. Nat Immunol (2010) 11(10):889–96. doi: 10.1038/ni.1937
9. Kiss M, Van Gassen S, Movahedi K, Saeys Y, Laoui D. Myeloid Cell Heterogeneity in Cancer: Not a Single Cell Alike. Cell Immunol (2018) 330:188–201. doi: 10.1016/j.cellimm.2018.02.008
10. Baumeister SH, Freeman GJ, Dranoff G, Sharpe AH. Coinhibitory Pathways in Immunotherapy for Cancer. Annu Rev Immunol (2016) 34:539–73. doi: 10.1146/annurev-immunol-032414-112049
11. Gubin MM, Esaulova E, Ward JP, Malkova ON, Runci D, Wong P, et al. High-Dimensional Analysis Delineates Myeloid and Lymphoid Compartment Remodeling During Successful Immune-Checkpoint Cancer Therapy. Cell (2018) 175(4):1014–30. doi: 10.1016/j.cell.2018.09.030
12. Siegel RL, Miller KD, Jemal A. Cancer Statistics, 2020. CA Cancer J Clin (2020) 70(1):7–30. doi: 10.3322/caac.21590
13. Bray F, Ferlay J, Soerjomataram I, Siegel RL, Torre LA, Jemal A. Global Cancer Statistics 2018: GLOBOCAN Estimates of Incidence and Mortality Worldwide for 36 Cancers in 185 Countries. CA: Cancer J Clin (2018) 68(6):394–424. doi: 10.3322/caac.21492
14. Thorsson V, Gibbs DL, Brown SD, Wolf D, Bortone DS, Ou Yang T-H, et al. The Immune Landscape of Cancer. Immunity (2018) 48(4):812–30. doi: 10.1016/j.immuni.2018.03.023
15. Mandal R, Şenbabaoğlu Y, Desrichard A, Havel JJ, Dalin MG, Riaz N, et al. The Head and Neck Cancer Immune Landscape and its Immunotherapeutic Implications. JCI Insight (2016) 1(17):e89829. doi: 10.1172/jci.insight.89829
16. Gonzalez JL, Reddy ND, Cunningham K, Silverman R, Madan E, Nguyen BM. Multiple Cutaneous Squamous Cell Carcinoma in Immunosuppressed vs Immunocompetent Patients. JAMA Dermatol (2019) 155(5):625–7. doi: 10.1001/jamadermatol.2018.5595
17. Balermpas P, Michel Y, Wagenblast J, Seitz O, Weiss C, Rödel F, et al. Tumour-Infiltrating Lymphocytes Predict Response to Definitive Chemoradiotherapy in Head and Neck Cancer. Br J Cancer (2014) 110(2):501–9. doi: 10.1038/bjc.2013.640
18. Perri F, Ionna F, Longo F, Della Vittoria Scarpati G, De Angelis C, Ottaiano A, et al. Immune Response Against Head and Neck Cancer: Biological Mechanisms and Implication on Therapy. Transl Oncol (2020) 13(2):262–74. doi: 10.1016/j.tranon.2019.11.008
19. Cohen EEW, Soulières D, Le Tourneau C, Dinis J, Licitra L, Ahn M-J, et al. Pembrolizumab Versus Methotrexate, Docetaxel, or Cetuximab for Recurrent or Metastatic Head-and-Neck Squamous Cell Carcinoma (KEYNOTE-040): A Randomised, Open-Label, Phase 3 Study. Lancet (2019) 393(10167):156–67. doi: 10.1016/S0140-6736(18)31999-8
20. Ferris RL, Blumenschein G, Fayette J, Guigay J, Colevas AD, Licitra L, et al. Nivolumab vs Investigator’s Choice in Recurrent or Metastatic Squamous Cell Carcinoma of the Head and Neck: 2-Year Long-Term Survival Update of CheckMate 141 With Analyses by Tumor PD-L1 Expression. Oral Oncol (2018) 81:45–51. doi: 10.1016/j.oraloncology.2018.04.008
21. Ferris RL, Blumenschein G, Fayette J, Guigay J, Colevas AD, Licitra L, et al. Nivolumab for Recurrent Squamous-Cell Carcinoma of the Head and Neck. N Engl J Med (2016) 375(19):1856–67. doi: 10.1056/NEJMoa1602252
22. Caponigro F, Ionna F, Scarpati GDV, Longo F, Addeo R, Manzo R, et al. Translational Research: A Future Strategy for Managing Squamous Cell Carcinoma of the Head and Neck? Anticancer Agents Med Chem (2018) 18(9):1220–7. doi: 10.2174/1871520618666180411110036
23. Gooden MJM, de Bock GH, Leffers N, Daemen T, Nijman HW. The Prognostic Influence of Tumour-Infiltrating Lymphocytes in Cancer: A Systematic Review With Meta-Analysis. Br J Cancer (2011) 105(1):93–103. doi: 10.1038/bjc.2011.189
24. Liu J, Lichtenberg T, Hoadley KA, Poisson LM, Lazar AJ, Cherniack AD, et al. An Integrated Tcga Pan-Cancer Clinical Data Resource to Drive High-Quality Survival Outcome Analytics. Cell (2018) 173(2):400–16. doi: 10.1016/j.cell.2018.02.052
25. Edgar R, Barrett T. Ncbi GEO Standards and Services for Microarray Data. Nat Biotechnol (2006) 24(12):1471–2. doi: 10.1038/nbt1206-1471
26. Cancer Genome Atlas N. Comprehensive Genomic Characterization of Head and Neck Squamous Cell Carcinomas. Nature (2015) 517(7536):576–82. doi: 10.1038/nature14129
27. Wichmann G, Rosolowski M, Krohn K, Kreuz M, Boehm A, Reiche A, et al. The Role of HPV RNA Transcription, Immune Response-Related Gene Expression and Disruptive TP53 Mutations in Diagnostic and Prognostic Profiling of Head and Neck Cancer. Int J Cancer (2015) 137(12):2846–57. doi: 10.1002/ijc.29649
28. Johnson WE, Li C, Rabinovic A. Adjusting Batch Effects in Microarray Expression Data Using Empirical Bayes Methods. Biostatistics (2007) 8(1):118–27. doi: 10.1093/biostatistics/kxj037
29. Subramanian A, Tamayo P, Mootha VK, Mukherjee S, Ebert BL, Gillette MA, et al. Gene Set Enrichment Analysis: A Knowledge-Based Approach for Interpreting Genome-Wide Expression Profiles. Proc Natl Acad Sci USA (2005) 102(43):15545–50. doi: 10.1073/pnas.0506580102
30. Liberzon A, Subramanian A, Pinchback R, Thorvaldsdóttir H, Tamayo P, Mesirov JP. Molecular Signatures Database (MsigDB) 3.0. Bioinformatics (2011) 27(12):1739–40. doi: 10.1093/bioinformatics/btr260
31. Yoshihara K, Shahmoradgoli M, Martínez E, Vegesna R, Kim H, Torres-Garcia W, et al. Inferring Tumour Purity and Stromal and Immune Cell Admixture From Expression Data. Nat Commun (2013) 4:2612. doi: 10.1038/ncomms3612
32. Newman AM, Liu CL, Green MR, Gentles AJ, Feng W, Xu Y, et al. Robust Enumeration of Cell Subsets From Tissue Expression Profiles. Nat Methods (2015) 12(5):453–7. doi: 10.1038/nmeth.3337
33. Mariathasan S, Turley SJ, Nickles D, Castiglioni A, Yuen K, Wang Y, et al. Tgfβ Attenuates Tumour Response to PD-L1 Blockade by Contributing to Exclusion of T Cells. Nature (2018) 554(7693):544–8. doi: 10.1038/nature25501
34. Bonaventura P, Shekarian T, Alcazer V, Valladeau-Guilemond J, Valsesia-Wittmann S, Amigorena S, et al. Cold Tumors: A Therapeutic Challenge for Immunotherapy. Front Immunol (2019) 10:168. doi: 10.3389/fimmu.2019.00168
35. Kather JN, Suarez-Carmona M, Charoentong P, Weis C-A, Hirsch D, Bankhead P, et al. Topography of Cancer-Associated Immune Cells in Human Solid Tumors. Elife (2018) 7:e36967. doi: 10.7554/eLife.36967
36. Engblom C, Pfirschke C, Pittet MJ. The Role of Myeloid Cells in Cancer Therapies. Nat Rev Cancer (2016) 16(7):447–62. doi: 10.1038/nrc.2016.54
37. Fridman WH, Zitvogel L, Sautès-Fridman C, Kroemer G. The Immune Contexture in Cancer Prognosis and Treatment. Nat Rev Clin Oncol (2017) 14(12):717–34. doi: 10.1038/nrclinonc.2017.101
38. Weston CJ, Zimmermann HW, Adams DH. The Role of Myeloid-Derived Cells in the Progression of Liver Disease. Front Immunol (2019) 10:893. doi: 10.3389/fimmu.2019.00893
39. Zhang T, Jin Y, Jiang X, Li L, Qi X, Mao Y, et al. Clinical and Prognostic Relevance of B7-H3 and Indicators of Glucose Metabolism in Colorectal Cancer. Front Oncol (2020) 10:546110. doi: 10.3389/fonc.2020.546110
40. Inamura K, Takazawa Y, Inoue Y, Yokouchi Y, Kobayashi M, Saiura A, et al. Tumor B7-H3 (Cd276) Expression and Survival in Pancreatic Cancer. J Clin Med (2018) 7(7):172. doi: 10.3390/jcm7070172
41. Wang Z, Wang Z, Zhang C, Liu X, Li G, Liu S, et al. Genetic and Clinical Characterization of B7-H3 (CD276) Expression and Epigenetic Regulation in Diffuse Brain Glioma. Cancer Sci (2018) 109(9):2697–705. doi: 10.1111/cas.13744
42. Zhang C, Hao X. Prognostic Significance of CD276 in Non-small Cell Lung Cancer. Open Med (Wars) (2019) 14:805–12. doi: 10.1515/med-2019-0076
43. Hu J, Jiang C, Zheng M, Guo Y, Tang X, Ren J, et al. Overexpression of B7-H3 as an Opportunity for Targeted Therapy in Head and Neck Cancers. Am J Transl Res (2019) 11(8):5183–96.
44. Kanchan RK, Perumal N, Atri P, Chirravuri Venkata R, Thapa I, Klinkebiel DL, et al. MiR-1253 Exerts Tumor-Suppressive Effects in Medulloblastoma Via Inhibition of CDK6 and CD276 (B7-H3). Brain Pathol (2020) 30(4):732–45. doi: 10.1111/bpa.12829
45. Cheng R, Wang B, Cai X-R, Chen Z-S, Du Q, Zhou L-Y, et al. Cd276 Promotes Vasculogenic Mimicry Formation in Hepatocellular Carcinoma Via the PI3K/AKT/MMPs Pathway. Onco Targets Ther (2020) 13:11485–98. doi: 10.2147/OTT.S271891
46. Wang L, Zhao W, Hong J, Niu F, Li J, Zhang S, et al. Association Between IL1B Gene and Cervical Cancer Susceptibility in Chinese Uygur Population: A Case-Control Study. Mol Genet Genomic Med (2019) 7(8):e779. doi: 10.1002/mgg3.779
47. Tulotta C, Ottewell P. The Role of IL-1B in Breast Cancer Bone Metastasis. Endocr Relat Cancer (2018) 25(7):R421–R34. doi: 10.1530/ERC-17-0309
48. Landvik NE, Hart K, Skaug V, Stangeland LB, Haugen A, Zienolddiny S. A Specific interleukin-1B Haplotype Correlates With High Levels of IL1B mRNA in the Lung and Increased Risk of non-Small Cell Lung Cancer. Carcinogenesis (2009) 30(7):1186–92. doi: 10.1093/carcin/bgp122
49. Yu M, Zhang H, Liu Y, He Y, Yang C, Du Y, et al. The Cooperative Role of S1P3 With LYVE-1 in LMW-HA-induced Lymphangiogenesis. Exp Cell Res (2015) 336(1):150–7. doi: 10.1016/j.yexcr.2015.06.014
50. Ozmen F, Ozmen MM, Ozdemir E, Moran M, Seçkin S, Guc D, et al. Relationship Between LYVE-1, VEGFR-3 and CD44 Gene Expressions and Lymphatic Metastasis in Gastric Cancer. World J Gastroenterol (2011) 17(27):3220–8. doi: 10.3748/wjg.v17.i27.3220
51. Jackson DG, Prevo R, Clasper S, Banerji S. Lyve-1, the Lymphatic System and Tumor Lymphangiogenesis. Trends Immunol (2001) 22(6):317–21. doi: 10.1016/s1471-4906(01)01936-6
52. Chen J-Y, Lai Y-S, Chu P-Y, Chan S-H, Wang L-H, Hung W-C. Cancer-Derived VEGF-C Increases Chemokine Production in Lymphatic Endothelial Cells to Promote Cxcr2-Dependent Cancer Invasion and MDSC Recruitment. Cancers (Basel) (2019) 11(8):1120. doi: 10.3390/cancers11081120
53. Shigetomi S, Imanishi Y, Shibata K, Sakai N, Sakamoto K, Fujii R, et al. Vegf-C/Flt-4 Axis in Tumor Cells Contributes to the Progression of Oral Squamous Cell Carcinoma Via Upregulating VEGF-C Itself and Contactin-1 in an Autocrine Manner. Am J Cancer Res (2018) 8(10):2046–63.
54. Morita Y, Hata K, Nakanishi M, Omata T, Morita N, Yura Y, et al. Cellular Fibronectin 1 Promotes VEGF-C Expression, Lymphangiogenesis and Lymph Node Metastasis Associated With Human Oral Squamous Cell Carcinoma. Clin Exp Metastasis (2015) 32(7):739–53. doi: 10.1007/s10585-015-9741-2
55. Ou J-J, Wei X, Peng Y, Zha L, Zhou R-B, Shi H, et al. Neuropilin-2 Mediates Lymphangiogenesis of Colorectal Carcinoma Via a VEGFC/VEGFR3 Independent Signaling. Cancer Lett (2015) 358(2):200–9. doi: 10.1016/j.canlet.2014.12.046
56. Zhou R, Sun J, He C, Huang C, Yu H. CCL19 Suppresses Gastric Cancer Cell Proliferation, Migration, and Invasion Through the CCL19/CCR7/AIM2 Pathway. Hum Cell (2020) 33(4):1120–32. doi: 10.1007/s13577-020-00375-1
57. Correale P, Rotundo MS, Botta C, Del Vecchio MT, Ginanneschi C, Licchetta A, et al. Tumor Infiltration by T Lymphocytes Expressing Chemokine Receptor 7 (CCR7) is Predictive of Favorable Outcome in Patients With Advanced Colorectal Carcinoma. Clin Cancer Res (2012) 18(3):850–7. doi: 10.1158/1078-0432.CCR-10-3186
58. Boyle ST, Gieniec KA, Gregor CE, Faulkner JW, McColl SR, Kochetkova M. Interplay Between CCR7 and Notch1 Axes Promotes Stemness in MMTV-PyMT Mammary Cancer Cells. Mol Cancer (2017) 16(1):19. doi: 10.1186/s12943-017-0592-0
59. Xu B, Zhou M, Qiu W, Ye J, Feng Q. CCR7 Mediates Human Breast Cancer Cell Invasion, Migration by Inducing Epithelial-Mesenchymal Transition and Suppressing Apoptosis Through AKT Pathway. Cancer Med (2017) 6(5):1062–71. doi: 10.1002/cam4.1039
60. Chen Y, Shao Z, Jiang E, Zhou X, Wang L, Wang H, et al. CCL21/CCR7 Interaction Promotes EMT and Enhances the Stemness of OSCC Via a JAK2/STAT3 Signaling Pathway. J Cell Physiol (2020) 235(9):5995–6009. doi: 10.1002/jcp.29525
61. Berry CT, Liu X, Myles A, Nandi S, Chen YH, Hershberg U, et al. Bcr-Induced Ca Signals Dynamically Tune Survival, Metabolic Reprogramming, and Proliferation of Naive B Cells. Cell Rep (2020) 31(2):107474. doi: 10.1016/j.celrep.2020.03.038
62. Takeuchi Y, Hirota K, Sakaguchi S. Impaired T Cell Receptor Signaling and Development of T Cell-Mediated Autoimmune Arthritis. Immunol Rev (2020) 294(1):164–76. doi: 10.1111/imr.12841
63. Burger JA, Wiestner A. Targeting B Cell Receptor Signalling in Cancer: Preclinical and Clinical Advances. Nat Rev Cancer (2018) 18(3):148–67. doi: 10.1038/nrc.2017.121
64. Ruffell B, Affara NI, Coussens LM. Differential Macrophage Programming in the Tumor Microenvironment. Trends Immunol (2012) 33(3):119–26. doi: 10.1016/j.it.2011.12.001
65. Najafi M, Hashemi Goradel N, Farhood B, Salehi E, Nashtaei MS, Khanlarkhani N, et al. Macrophage Polarity in Cancer: A Review. J Cell Biochem (2019) 120(3):2756–65. doi: 10.1002/jcb.27646
66. Mantovani A, Sozzani S, Locati M, Allavena P, Sica A. Macrophage Polarization: Tumor-Associated Macrophages as a Paradigm for Polarized M2 Mononuclear Phagocytes. Trends Immunol (2002) 23(11):549–55. doi: 10.1016/S1471-4906(02)02302-5
67. Andreu P, Johansson M, Affara NI, Pucci F, Tan T, Junankar S, et al. FcRgamma Activation Regulates Inflammation-Associated Squamous Carcinogenesis. Cancer Cell (2010) 17(2):121–34. doi: 10.1016/j.ccr.2009.12.019
68. Derakhshani A, Vahidian F, Alihasanzadeh M, Mokhtarzadeh A, Lotfi Nezhad P, Baradaran B. Mast Cells: A Double-Edged Sword in Cancer. Immunol Lett (2019) 209:28–35. doi: 10.1016/j.imlet.2019.03.011
69. Komi DEA, Redegeld FA. Role of Mast Cells in Shaping the Tumor Microenvironment. Clin Rev Allergy Immunol (2020) 58(3):313–25. doi: 10.1007/s12016-019-08753-w
70. Lee Y-H, Martin-Orozco N, Zheng P, Li J, Zhang P, Tan H, et al. Inhibition of the B7-H3 Immune Checkpoint Limits Tumor Growth by Enhancing Cytotoxic Lymphocyte Function. Cell Res (2017) 27(8):1034–45. doi: 10.1038/cr.2017.90
71. Yang S, Wei W, Zhao Q. B7-H3, a Checkpoint Molecule, as a Target for Cancer Immunotherapy. Int J Biol Sci (2020) 16(11):1767–73. doi: 10.7150/ijbs.41105
72. Tsujikawa T, Kumar S, Borkar RN, Azimi V, Thibault G, Chang YH, et al. Quantitative Multiplex Immunohistochemistry Reveals Myeloid-Inflamed Tumor-Immune Complexity Associated With Poor Prognosis. Cell Rep (2017) 19(1):203–17. doi: 10.1016/j.celrep.2017.03.037
73. Zhang X, Shi M, Chen T, Zhang B. Characterization of the Immune Cell Infiltration Landscape in Head and Neck Squamous Cell Carcinoma to Aid Immunotherapy. Mol Ther Nucleic Acids (2020) 22:298–309. doi: 10.1016/j.omtn.2020.08.030
74. Li Z-X, Zheng Z-Q, Wei Z-H, Zhang L-L, Li F, Lin L, et al. Comprehensive Characterization of the Alternative Splicing Landscape in Head and Neck Squamous Cell Carcinoma Reveals Novel Events Associated With Tumorigenesis and the Immune Microenvironment. Theranostics (2019) 9(25):7648–65. doi: 10.7150/thno.36585
75. Yi L, Wu G, Guo L, Zou X, Huang P. Comprehensive Analysis of the PD-L1 and Immune Infiltrates of Ma RNA Methylation Regulators in Head and Neck Squamous Cell Carcinoma. Mol Ther Nucleic Acids (2020) 21:299–314. doi: 10.1016/j.omtn.2020.06.001
76. Acosta JC, Banito A, Wuestefeld T, Georgilis A, Janich P, Morton JP, et al. A Complex Secretory Program Orchestrated by the Inflammasome Controls Paracrine Senescence. Nat Cell Biol (2013) 15(8):978–90. doi: 10.1038/ncb2784
77. Nakamura Y, Aihara R, Iwata H, Kuwayama T, Shirasuna K. IL1B Triggers Inflammatory Cytokine Production in Bovine Oviduct Epithelial Cells and Induces Neutrophil Accumulation Via CCL2. Am J Reprod Immunol (2020) 85(5):e13365. doi: 10.1111/aji.13365
78. Dollt C, Becker K, Michel J, Melchers S, Weis C-A, Schledzewski K, et al. The Shedded Ectodomain of Lyve-1 Expressed on M2-like Tumor-Associated Macrophages Inhibits Melanoma Cell Proliferation. Oncotarget (2017) 8(61):103682–92. doi: 10.18632/oncotarget.21771
79. Lund AW, Duraes FV, Hirosue S, Raghavan VR, Nembrini C, Thomas SN, et al. VEGF-C Promotes Immune Tolerance in B16 Melanomas and Cross-Presentation of Tumor Antigen by Lymph Node Lymphatics. Cell Rep (2012) 1(3):191–9. doi: 10.1016/j.celrep.2012.01.005
Keywords: tumor immune microenvironment, myeloid cells, head and neck squamous cell carcinoma, prognosis, immune infiltration, immunotherapy
Citation: Liu Z, Zhang D, Liu C, Li G, Chen H, Ling H, Zhang F, Huang D, Wang X, Liu Y and Zhang X (2021) Comprehensive Analysis of Myeloid Signature Genes in Head and Neck Squamous Cell Carcinoma to Predict the Prognosis and Immune Infiltration. Front. Immunol. 12:659184. doi: 10.3389/fimmu.2021.659184
Received: 27 January 2021; Accepted: 08 April 2021;
Published: 29 April 2021.
Edited by:
Jonathan Pol, Institut National de la Santé et de la Recherche Médicale (INSERM), FranceReviewed by:
Simon Laban, Ulm University Medical Center, GermanyRieneke Van De Ven, VU University Medical Center, Netherlands
Copyright © 2021 Liu, Zhang, Liu, Li, Chen, Ling, Zhang, Huang, Wang, Liu and Zhang. This is an open-access article distributed under the terms of the Creative Commons Attribution License (CC BY). The use, distribution or reproduction in other forums is permitted, provided the original author(s) and the copyright owner(s) are credited and that the original publication in this journal is cited, in accordance with accepted academic practice. No use, distribution or reproduction is permitted which does not comply with these terms.
*Correspondence: Yong Liu, bGl1eW9uZ2VudEBjc3UuZWR1LmNu; Xin Zhang, eGluemhhbmdAY3N1LmVkdS5jbg==