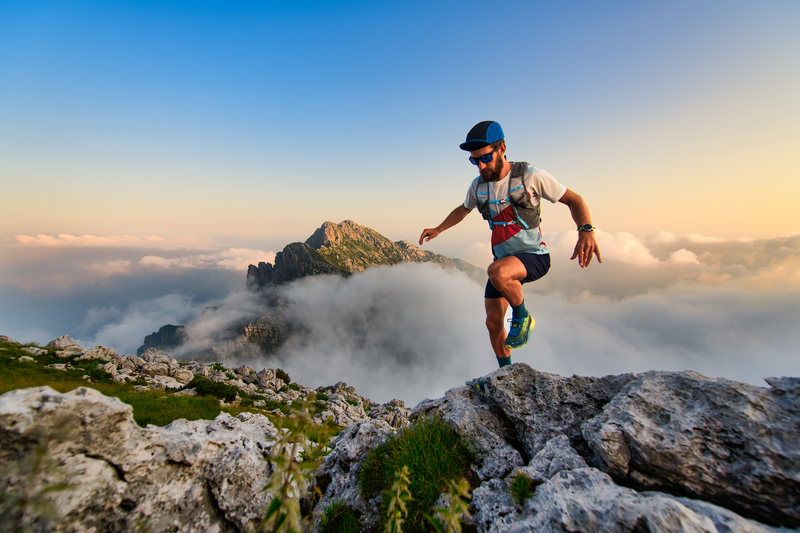
94% of researchers rate our articles as excellent or good
Learn more about the work of our research integrity team to safeguard the quality of each article we publish.
Find out more
REVIEW article
Front. Immunol. , 31 May 2021
Sec. Vaccines and Molecular Therapeutics
Volume 12 - 2021 | https://doi.org/10.3389/fimmu.2021.642383
This article is part of the Research Topic Pre-Conference Research Topic: 16th International Symposium on Schistosomiasis View all 35 articles
Schistosomiasis is a parasitic disease caused by trematode worms of the genus Schistosoma and affects over 200 million people worldwide. The control and treatment of this neglected tropical disease is based on a single drug, praziquantel, which raises concerns about the development of drug resistance. This, and the lack of efficacy of praziquantel against juvenile worms, highlights the urgency for new antischistosomal therapies. In this review we focus on innovative approaches to the identification of antischistosomal drug candidates, including the use of automated assays, fragment-based screening, computer-aided and artificial intelligence-based computational methods. We highlight the current developments that may contribute to optimizing research outputs and lead to more effective drugs for this highly prevalent disease, in a more cost-effective drug discovery endeavor.
Schistosomiasis is a neglected tropical disease (NTD) caused by trematode parasites belonging to the genus Schistosoma. The most clinically-relevant species are S. mansoni, S. japonicum and S. haematobium while S. mekongi, S. guineensis and S. intercalatum have lower prevalence (1, 2). According to the World Health Organization (WHO), approximately 229 million people are infected worldwide, causing around 200,000 deaths annually (2). However, this is probably an underestimation, due to the low sensitivity of the available diagnostic methods to detect low intensity parasite infections (3, 4). It ranks second behind malaria in terms of prevalence and socioeconomic impact, causing the loss of more than 2.6 million disability-adjusted life years (DALYs) (5).
Humans become infected when the cercariae larvae, released by the snail intermediate hosts, penetrate through the skin during contact with contaminated freshwater (1, 6). Then, cercariae access the host circulation and develop into juvenile and adult worms (7). Paired female and male adult schistosomes live in the blood vessels where they produce eggs that are excreted in faeces (S. mansoni, S. japonicum, S. intercalatum, S. guineenses and S. mekongi) or urine (infections by S. haematobium) (6). Eggs become trapped in human tissues causing inflammatory immune reactions (including granulomata) that damage organs resulting in intestinal, hepatosplenic, or urogenital disease (8). The eggs that reach the environment, hatch in the water and release the larval stage miracidia. The Miracidia infect the intermediate hosts and continue the parasite’s life-cycle (9).
Because the development of a schistosomiasis vaccine has proved challenging (10, 11), the treatment and control of schistosomiasis continue to depend, on the almost 50-year-old drug, praziquantel (PZQ) (12, 13). PZQ is generally effective against adult and schistosomula stages of all schistosome species and is well tolerated, causing only mild and transient side effects (14, 15). However, PZQ is ineffective against juvenile schistosomes, which contributes to the failure of the drug to cure the disease and the need for new rounds of treatment (16, 17). Moreover, PZQ is administered as a racemic mixture, wherefore only half of PZQ dose (i.e., R-PZQ stereoisomer) is pharmacologically active. The S-PZQ, besides being pharmacologically inactive, contributes to the bitter taste and the large size of PQZ tablets, both of which decrease patient compliance and are not suitable for children (18, 19). Moreover, PZQ has been used in mass drug administration campaigns for many decades and this may account for a selection pressure that can promote the development of parasitic resistance (20). In fact, reduced PZQ efficacy has been demonstrated both in laboratory and field isolates (21–28). Consequently, there is an urgent need for new antischistosomal drugs.
One of the foremost challenges to the discovery of new anti-schistosomal drugs is the long and complex life cycle of the parasite, which makes screening campaigns technically difficult (29). The phenotypic screening of whole-organisms in vitro and/or in animal models is the approach that is most used for finding hit compounds (i.e., active compounds in vitro based on a defined activity threshold) (30–32), though animal models tend to be costly and time-consuming (33). These assays require the maintenance of the parasites’ life cycle – including both the intermediate (snails) and the definitive hosts (hamsters or mice) – in order to have regular access to parasites. However, these laboratory-based life cycles can only produce a restricted number of adult-stage schistosomes (34). As a consequence, most early compound screening efforts use newly transformed schistosomula (NTS), which are obtained from the mechanically transformed cercariae (35). This can limit the finding of new hits, as the sensitivity to compounds can vary between life cycle stage and gender of the parasite (36, 37).
Screening compounds for anti-schistosomal activity is typically carried out manually where an analyst identifies the 5presence of morphological or behavioral changes in the parasites by microscopy (29). A numerical scale (“severity score”), usually including four (38, 39) or five (29, 40) scores, is used to describe the phenotypes. However, this analysis is subjective, semi-quantitative, time-consuming and the results can vary largely from analyst to analyst (41–43). Nonetheless, severity scoring systems have been successful in identifying hits, defining SAR, and identifying compounds that translate with in vivo efficacy in the mouse model of S. mansoni infection (44).
In this review we focus on innovative approaches to the identification of antischistosomal drug candidates, including the use of automated assays, fragment-based screening, computer-aided and artificial intelligence-based computational methods. We also highlight the current developments that may contribute to optimizing research outputs and lead to more cost-effective drugs for this highly prevalent disease.
Phenotypic screening consists of testing substances that could possibly cause phenotypic changes considered relevant to a biological system. Phenotypic-based drug discovery (PDD), compared to target-based drug discovery (TDD), has the advantage of presenting a greater probability of identifying compounds which will be translated to in vivo tests since they better mimic the complex environment of living systems. For example, in a cellular assay, a test compound may have to cross cellular membranes and/or resist degradation by metabolic enzymes before interacting with its target(s). These factors may have a significant impact in a compound’s biological activity and are not taken into account in a target-based assay. Hence, a hit coming from a phenotypic screen has much more biological value than one coming from a target-based screen (45, 46). Nonetheless, an important consideration must be given to the higher cases of false negatives in PDD campaigns when compared to TDD. As discussed by Geary and colleagues (33), the false negatives result from the inability of some compounds to reach a proper concentration inside a whole organism, which may hinder the detection of potential schistosomicidal molecules.
PDD approach tends to be more time-consuming and costly to develop and run than TDD (47). This is mainly due to the implementation of higher complexity screening assays, and to other factors such as the parallel use of genetic and small molecules screens, as well as more complicated hit validation and target identification efforts (48). Furthermore, the establishment of structure-activity relationship (SAR) is more challenging due to other concurrent factors, such as membrane permeability and off-target binding, though there are several examples of successful SAR studies using schistosome phenotypic assays in the literature (49–51). Hence, PDD approach is limited in this sense due to unknown mechanism of action, potentially targeting different types of proteins, such as receptors, enzymes, transcription factors and even different signaling pathways (45). Additional assays may be necessary to support the SAR in PDD approaches. On the other hand, the multiplicity of potential targets in PDD can be a source of serendipity (33, 46). In contrast, the prior knowledge of the target in TDD helps to accelerate the interpretation of SAR data. In spite of their differences, it is increasingly known that PDD and TDD must be seen as complementary in the R&D of new drugs (48, 52, 53).
In the following topics we will address some of the main phenotypic assays that have been used for schistosomiasis drug discovery. They consist in labelled (employing fluorescent or luminescent dyes) and label-free assays that are able to detect drug-induced phenotypes in different stages of the parasite, such as schistosomula (NTS), juvenile (1-5 weeks post infection) and adult (6-7 weeks or over post infection) (38).
Some methodologies used in schistosome phenotypic screening are based on fluorescent or luminescent dyes commonly employed in cellular viability/cytotoxicity assays (Table 1). Propidium iodide (PI), a DNA intercalator, for instance, is a fluorescent dye that is not able to cross membrane cells and can only stain nucleic acids of cells that have lost their membrane integrity. For this reason, such fluorophore is used to differentiate living and dead cells. Unlike PI, fluorescein diacetate (FDA) can cross biological membranes, and is converted by healthy cells into fluorescein, another fluorescent dye. Peak et al. (54) developed a microplate-based assay to measure schistosomula viability using PI/FDA. In this method, the fluorescence emitted by FDA and PI stained parasites is quantified by a microplate reader and later converted into worm viability using the fluorescence ratio FDA/(PI + FDA). This assay was able to detect the effect of some known schistosomicidal drugs, namely auranofin, gambogic acid and amphotericin b, but failed in measuring the effect of praziquantel and other compounds previously identified as actives by microscopy (55). Braun et al. (56) also used PI and FDA probes in the development of a quantitative HTS (qHTS) fluorescent-based bioassay to identify schistosomula in water samples (56). The results obtained with this method showed no statistically significant differences when compared to visual inspections carried out by manual microscopy.
Mansour et al. (42) used a commercial solution of resazurin (Alamar Blue®), a redox-sensitive probe, to measure the viability of schistosomula in microplates. This assay is based on the principle that only metabolic active worms can reduce resazurin to resorufin, a fluorescent molecule. During validation, this assay proved to be useful in evaluating the effect of compounds that kill or provoke severe damage to the parasite (e.g., oltipraz), but showed less sensitivity towards those that elicited more subtle effects (e.g., praziquantel) detectable by conventional microscopy.
Lalli et al. (38) developed and validated a luminescence-based method for the evaluation of schistosomula viability by quantifying ATP (38). This assay is carried out using a commercial kit (CellTiter-Glo®) which contains luciferase and its substrate luciferin as the main components. In principle, metabolic active worms produce ATP which participates in the reaction catalysed by luciferase. As a result, a luminescence signal is produced and registered in a microplate reader. This medium-throughput method is suitable for semi-automated screening of chemical libraries and has several benefits such as speed in screening, reproducibility and non-subjectivity. Guidi et al. (57) used the same luminescence-based technique, combined with HTS, to search for molecules with schistosomicidal activity. As a result, a few compounds capable of impairing the viability of the larval, juvenile, and adult phases were identified, with potency against juveniles higher than PZQ. In addition, changes in egg formation and production are among the phenotypic modifications.
However, despite its success in generating dose-response curves for some known schistomicidal drugs (e.g., gambogic acid and oltipraz) this method was unable to detect the effect of praziquantel and oxamniquine on schistosomula viability. Maccesi et al. (44) also could not detect the biological effect of some schistomicidal compounds using another phenotypic assay. In their work, S. mansoni schistosomula were screened with the 400 compounds of the MMV Pathogen Box in three different institutions. Two of them employed visual scoring (microscopy) to describe drug-induced phenotypes while the other used a colorimetric assay based on the metabolic reduction of XTT to measure parasites viability. In nearly 74% of the cases, all three methods agreed on the classification of the compounds (active/inactive) after 72h of incubation. Nonetheless, unlike the visual methods, the XTT-based assay did not identify PZQ and other compounds (e.g., auranofin, nitazoxanide) as actives against schistosomula. This may be due to the fact that metabolic-based assays were originally designed to be used in cell and unicellular organisms. Its use in multicellular and more complex models are prone to missing important phenotypes (44) found in these organisms, like the dysregulation of the neuromuscular system, a mechanism attributed to PZQ (58).
Panic et al. (39) performed a review of fluorescent and luminescent markers used on S. mansoni drug assays and confirmed Lalli et al. (38) studies of development and validation of a luminescence-based assay (CellTiter-Glo®). In contrast to resazurin assays, which are also used to determine the viability of Schistosoma parasites, CellTiter-Glo® was able to determine the IC50 of mefloquine and to detect the schistomicidal activity of some FDA-approved compounds, showing results that correlate with microscopic findings.
The assays described in this topic represent a major advance in schistosomiasis drug discovery. Nonetheless, they show some limitations that must be taken into consideration. Compared to colorimetric methods, such as XTT-based assay, fluorescence/luminescence-based assays require black/white microplates which are more expensive (59). In some cases, a given method may not detect the schistomicidal effect of a compound recognized as active by other techniques, including conventional microscopy (39). Moreover, some test compounds may interfere with the assay (e.g., compounds auto-fluorescence), leading to a misinterpretation of the results (38). Therefore, it is advisable to be cautious when considering the results obtained from a single method, being recommended to corroborate the results using at least one orthogonal assay.
Label-free automated assays detect phenotypic alterations in schistosomes in the absence of any kind of label (e.g., fluorescent probe). In general, they can be divided into two main groups: image-based (most common) (60–63) and non-image-based methods (64, 65) (Figure 1A). The former use visual information, such as morphology and/or motility of the parasite, to describe a phenotype (Figures 1A, B). In contrast, non-image-based methods, herein exemplified by electrical impedance spectroscopy (65) and microcalorimetry (64), create phenotypic profiles based on worm’s metabolic activity and/or its motility (Figures 1A, C). Image-based and non-image-based assays have been largely employed in drug discovery campaigns to identify new schistosomicidal compounds and calculate their potencies. Some of these methods and their main characteristics (e.g., type of readout, assay format and the number of parasites required per assay) are shown in Table 2 and will be discussed in more detail in the following section.
Figure 1 Label-free image-based and non-image-based automated methods used in schistosomiasis drug discovery. (A) Overall description of label-free methods, (B) image-based methods, (C) non-image-based methods.
The automatic quantification of phenotypic features from schistosomes images has been addressed in several ways (60–63, 66, 69, 70, 72). Most assays rely on home-made (60) or commercial (63, 70) systems, equipped with digital cameras/camcorders to acquire images of unstained parasites in microplates. Image analysis is carried out, using either commercial (62, 69, 70) or custom-built (60, 68) software and generally consists of three main steps: image processing, parasite(s) detection and features extraction (Figure 1B). By the end of the analysis, a phenotypic profile, composed of a variable number of features, is created for one (63, 69, 70) or more (60) worms. These profiles can be used to identify schistosomicidal compounds, measure their effect and potency, as well compare their responses to those elicited by other compounds (60, 63, 69, 70).
One common strategy to acquire schistosome images is by video microscopy. Ribeiro and colleagues implemented two assays using this technique to measure the motility of NTS (62) and adult (66) forms of S. mansoni. In these assays, parasites are distributed in microplates and their videos recorded over a few minutes. Image frames are analyzed in ImageJ (73), an open-source software. For NTS analysis, worms are detected as ellipsoid objects and their body length are estimated by the size of the major axis of the ellipses. NTS movements (shortening and elongation of the body) are calculated by the frequency of length changes over time (62). For adult analysis, each image frame is submitted to a series of pre-processing steps (e.g., illumination correction and background removal) before representing the worms as binary objects. Then, the difference in pixels between pairs of consecutive images are measured throughout the entire video. The subtracted pixels represent a change in parasite position over time and are used as a metric to measure worm´ motility (66). These methods were employed to evaluate the effect of different compounds on schistosome NTS/adults worms, such as tyrosine-derived signaling agonists/antagonists (74), natural alkaloids (75–77) and analogs (77, 78), as well as others with biological activity in humans (e.g., NPS-2143, a calcium-sensing receptor antagonist) (77).
McCusker and colleagues (67, 72) also applied video microscopy to record morphological and motility aspects of adult schistosomes. In their assay, 1 min videos of adult worms, distributed in 6-well dishes, are acquired, and saved as images Z-stacks. During image analysis in ImageJ, maximum intensity projections are generated to each Z-stack resulting in a composite image for which pixel integrated pixel values are measured. This metric represents the total movement of the parasite over time and can be used to compare treated and non-treated parasites. This method detected the schistosomicidal effect of non-sedating benzodiazepines (72) and FPL-64176 (67), a human L-type Ca2+ channel agonist.
WormAssay is a home-made low-cost solution to screen compounds against adult schistosomes (and other macroscopic parasites) developed by Marcellino et al. (60). This system consists of two devices: an imaging apparatus and a Mac computer (image acquisition control and analysis). The former can be described as a light-tight box containing a high-definition video (HDV) camcorder mounted at the bottom and a hinge lid at the top harboring the microplate chamber. Inside the chamber a white LED strip is used to laterally illuminate the microplate. During acquisition, dark-field videos of 0.5 – 1 min length are recorded from the entire microplate. Image analysis is performed in real-time using a custom open-source free software (Mac application) installed in the computer. The overall motility of worms, measured for each well, is determined by two algorithms: one that calculates the average velocity of moving contours inside the well and another which detects changes in the occupation and vacancy of pixels between a group of frames. WormAssay quantified the effect of several compounds on worm motility, including neuromodulatory drugs (79), phenylpyrimidines (80) and inhibitors of S. mansoni cyclic nucleotide phosphodiesterase 4 (SmPDE4) (81) and proteasome (82). Recently, a modified version of WormAssay software, named WormAssayGP2, was released by Padalino and colleagues (83, 84) and contains minor modifications related to the source code and user interface (85). To date, WormAssayGP2 has been used to detect the schistomicidal activity of putative inhibitors of S. mansoni lysine specific demethylase 1 (SmLSD1) (86) and histone methyltransferase mixed lineage leukemia-1 (SmMLL-1) (84), as well as human ubiquitin-proteasome system (85) inhibitors.
One of the first attempts to describe complex phenotypes is schistosomes was carried out by Singh et al. (87). They developed an automated method to detect, track and classify individual NTS in microscopy videos. Since then, several modifications have been made regarding worms segmentation (88) and phenotypic analysis (63, 68, 89, 90). The algorithms developed in these studies have proved to be useful in quantifying the schistosomicidal activity of PZQ (63), chlorpromazine (63), statins (91) and inhibitors of human polo-like kinase 1 (PLK1) (89, 92). They have also been implemented in the “quantal dose-response calculator” (QDREC), a web server that automatically extracts quantal time- and dose-response information from bright-field images of schistosomes NTS (or other parasites) (68). QDREC quantifies 71 image-based features related to the appearance, shape and texture, of each segmented parasite. These features serve as inputs for supervised machine learning algorithms which classify parasites as “normal” or “degenerate”. The proportion of “degenerate” worms in a well is employed as a metric to estimate the schistosomicidal effect of a given compound and can be used to create dose-response curves. QDREC was validated with 12 schistosomicidal compounds (e.g., statins, PZQ, closantel, niclosamide and sorafenib) showing comparable results with visual annotation for both parasite classification and dose-response curves.
Paveley et al. (70) was a pioneer in implementing automated microscopy, also known as high content screening (HCS), to extract multivariate data from schistosomes. They created an HCS-based automated platform to identify active compounds on NTS of S. mansoni. In this assay, a HCS system collects bright-field images of each well of a 384-wells microplate through two distinct modes: a time-lapse image acquisition (5x6 s interval) using a 4x objective, for motility analysis, and one acquisition of four adjacent images using a 10x objective, for morphology measurements. Image analysis is performed in Pipeline Pilot 8.5 software (Accelrys Inc., San Diego, USA) and includes a series of sequential image operations, such as thresholding, filtering, detecting boundaries prior worm´s segmentation. Morphological (e.g., area, texture, pixel intensity) and motility-related features are quantified for each NTS and summarized into a phenotype (processed through Bayesian models) and a motility score, respectively. The final scores are obtained by averaging the scores of all parasites inside each well. Test compounds are declared “hits” (i.e., actives) if phenotype and motility scores exceed a defined threshold for each metric. This assay was able to detect the anti-worm effect of known schistosomicidal drugs (oltipraz and dihydroartemisinin) (70), several drug candidates from small (93, 94) and large (70, 95) chemical libraries, as well FDA-approved drugs (e.g., kinase inhibitors) (96). This analysis has also been employed by Hoffmann and colleagues (84, 86, 97–100) who measured the schistosomicidal activity of plant-derived compounds [e.g., diterpenoids (97, 99) and triterpenoids (100)] and of potential inhibitors of S. mansoni histone-modifying enzymes (40, 84, 86, 98).
Recently, Chen et al. (61) developed another HCS-based high-throughput assay to extract phenotypic features from treated and non-treated NTS. It consists of a fully integrated platform that can perform multiple automated operations ranging from liquid handling of NTS suspensions to image acquisition/analysis. The latter tasks are carried out as follows: time-lapse bright-field images (30 x 0.66 s interval) are acquired from each well of a U-bottom 96-wells microplate using a 10x objective in a HCS system. During image analysis, each NTS is segmented and classified as “clear” (normal) or “degenerate” (damaged/dying) using 15 phenotypic features based on the appearance of worms (e.g., pixel intensity, area, length). Motility measurements are inferred from the magnitude of a change in a feature over time or how often its sign or direction changes (e.g., when the worm becomes longer than shorter). Two statistical methods are employed to measure significant changes in phenotypes: glass effect size (monoparametric) and Mahalanobis distance (multiparametric). The results are analyzed in “SchistoView”, a graphic interface supported by MySQL database, which allows users to visualize, query and explore NTS data. This method shows several improvements in comparison to the HCS-based assay developed by Paveley et al. (70), including a higher NTS segmentation accuracy, it requires less parasites per assay and quantifies how parasites move instead of simply determine if the movement has occurred or not. In part, these improvements were achieved due to an innovative solution that allows the segmentation of touching objects in bright-field images, a challenging task for image analysis in general. In fact, many techniques implemented in this platform, such as automated liquid handling of 100 µm-sized organisms and statistical analysis of multiparametric data, can be useful to other screening projects. The HCS approach by Chen et al. (61) was successfully used to create the phenotypic profile of known schistosomicidal agents, as well of 1,323 human approved compounds, identifying new potential drug candidates.
HCS has also been applied to screen compounds against adult forms (male and females) of schistosomes. Neves et al. (69) described a HCS-based method to extract morphological and motility measurements from time-lapse bright-field images of S. mansoni. In this assay, schistosomes were distributed in 96-well microplates (1 worm per well) and their images acquired over time (100 x 0.3 s interval) using a 2x objective. Image analysis was carried out in a customized pipeline of the open-source software Cellprofiler (101) comprising a series of image processing modules, including those responsible for detection of wells, illumination correction, parasites segmentation and features extraction. More than 90 features were quantified during this analysis though only two, related to worm motility, were used to describe drug-induced phenotypes. So far, this method has been applied to detect the schistomicidal effect of antidepressant paroxetine (69) as well as putative inhibitors of S. mansoni thioredoxin glutathione reductase (SmTGR) (93, 94).
Electrical impedance spectroscopy (EIS) is noninvasive and label-free method that has been explored in schistosomiasis drug discovery (65, 71, 102, 103) (Figure 1C). In summary, ESI systems quantify dielectrical properties of samples while applying an alternative current (AC) electrical field using electrodes. EIS measurements can be used to detect phenotypic changes in cells/organisms induced by perturbagens, such as small molecules (104). Smout et al. (102) employed the xCELLigence real time cell analysis (RTCA) system to measure the effect of chemical compounds on the motility of helminths, including adult schistosomes. The experiments are carried out in E-plates, commercial microplates with gold electrodes embedded in the base of the wells that allow monitoring electrical resistance. Later, an improved version of this assay (xCELLigence worm real-time motility assay - xWORM) expanded its applications to detect alterations in the motility of schistosomes cercariae and egg hatching (71). xWORM is a sensitive method and was able to reveal the schistosomicidal effect of natural-derived [phytochemicals (105, 106) and puromycin (107)] and synthetic [forchlorfenuron (108) and polyridylruthenium(II) complexes (109)] compounds. Nonetheless, it is not sensitive enough to detect NTS small movements and requires a relativity larger number of samples compared to conventional microscopy (103). These limitations were addressed by Modena et al. (110) who developed a microfluid impedance-based platform to measure changes in NTS motility. Their system consisted of a microfluidic chip, made of polydimethylsiloxane (PDMS), attached to a glass substrate with patterned electrodes. This method showed high sensitivity towards both viable and non-viable parasites and required a lower number of worms per assay to operate in comparison to microscopy. Later, this concept evolved into a parallelized platform which was able to run four experiments simultaneously (103). More recently (65), the system was reformulated, becoming more automated, performing at a higher throughput (32 experiments run in parallel) and allowing long-term culturing of NTS. This assay was successful in determining the EC50 of mefloquine and oxethazaine which were of the same order of magnitude as those calculated by microscopy (65).
Isothermal microcalorimetry (IMC) is a very sensitive technique that measures the heat released or consumed by physical or chemical events under essentially isothermal conditions (111, 112). IMC has been used in different areas of biomedicine, such as in the detection of infection and tumors, antibiotic testing, parasitology and screening for new drugs (111, 113). Manneck et al. (64) developed an IMC-based assay to study the effect of chemical compounds on NTS and adult worms of S. mansoni. In this method, the overall heat production of a suspension of parasites is continuously recorded by the microcalorimeter. After the injection of a schistosomicidal compound it is expected that the heat-flow curves change their behavior indicating compounds effect on worm metabolism and/or motility (Figure 1C). This assay proved to be highly sensitive, capturing subtle effects that were not detected by conventional microscopy and was used to measure the schistosomicidal activity of known anti-schistosome agents (mefloquine, praziquantel) (64, 114), their isomers/racemates (115, 116), as well as mefloquine-related arylmethanols (117) and 3-alkoxy-1,2-dioxolanes (118)
In the previous topics we described the main label-free methods available today for schistosomiasis drug discovery. They represent more automated alternatives for conventional microscopy, overcoming some of its major limitations (e.g., visual phenotypic scoring). These methods vary according to several features, such as equipment/readout (e.g., microscope/image), assay cost (equipment and supplies), automation and screening throughput. They all have their pros and cons and the choice of one over another depends, in many cases, on equipment availability. In low-budget labs, video microscopy and WormAssay represent more affordable solutions for compound screening against schistosomula and/or adult schistosomes, since they require low-cost equipment, and the assays are carried out in regular microplates. On the other hand, HCS-based assays demand a high initial investment but use regular microplates as supplies, operate at a higher throughput and can be readily incorporated into automated platforms. Moreover, HCS systems can be easily coupled with a wide range of objective lens, allowing them to capture images of schistosomula, adults and potentially other parasites forms (e.g., eggs and juveniles). In contrast to image-based, non-image-based methods are less employed in screening campaigns. Overall, they demand expensive (microcalorimeter and RTCA systems) or customized (EIM platform) equipment, more costly supplies (e.g., E-plates), operate at a lower-throughput in comparison with automated microscopy and, in the case of xWORM, it is not able to detect phenotypic changes in schistosomula. Nonetheless, they are highly sensitive, may reveal drug-induced phenotypes which cannot be captured by image-based methods (e.g., metabolic activity), and xWORM already offers protocols for measuring the effect of compounds on schistosome eggs and cercariae. In conclusion, it is our understanding that HCS-based assays represent today the most advanced approaches to schistosomes phenotypic screening due to their ability to describe complex phenotypes of different forms of the parasite at a high throughput.
Target-based drug discovery (TDD) consists of finding ligands for a known biological target, previously identified as having potential relevance in a disease. One of its main advantages is the possibility of knowing characteristics of the target binding site, which allows the optimization of ligands and the development of an efficient structure-activity relationship. Its emergence was made possible by advances in molecular biology and genetics, which allowed the identification of individual biological targets, as well as the possibility of developing compounds that interact with these targets. The genome project, the development of techniques such as RNA interference and gene knockout, advances in structural biology and the development of computational tools were of great importance for the emergence of this alternative to the phenotypic approach in drug discovery (47, 119–121).
Ligand-binding assays are at the core of TDD strategies. In the context of pharmacological screening, the classical assay provides, for example, affinity, potency, and maximum response data of the analyzed molecules. It is also possible to determine the intrinsic activity of ligands (122, 123), through functional binding assays, in addition to assessing the residence time of the ligand to its target molecule (124). In contrast to the classical binding assays, HTS is a strategy that allows for the testing of tens of thousands of compounds per day, for activity against biological targets. Considered one of the most used strategies in TDD, HTS can be performed using different approaches, that can be mainly divided into biochemical assays and cell-based assays (125–127).
Although in-solution assays are commonly used for in vitro screenings, immobilized enzyme reactors (IMER) systems have proved to be a valid alternative drug screening strategy. The immobilization of a target protein to a solid support has the advantage of longer maintenance of the stability of the molecule, and the possibility of extracting the protein from the reaction medium, allowing it to be reused. In addition, IMER can be coupled to different separation systems, such as high performance liquid chromatography (HPLC), which solve the possible problem of product and assayed compounds fluorescing at the same wavelength, since these analytes can be separated and analyzed individually (128). Active anti-cancer compounds (129), enzyme inhibitors (130–133) and G protein-coupled receptors (GPCR) (134) binders have recently been identified using this approach.
After the sequencing and decoding of the S. mansoni genome, several putative drug targets were identified (Table 3), and studies using a the target-based approach emerged (157).
S. mansoni encodes 252 kinases, which have already been shown to have a relevant role in the biology of the parasite (158). S. mansoni polo-like kinase (SmPLK1) is mainly expressed in reproductive organs of the adult parasite, which suggests a contribution of this enzyme to cell division. The screening of a series of analogues compounds, derived from a human PLK1 inhibitor bioactive against S. mansoni parasites, yielded the identification of potent compounds against schistosomula and adults (92). Buskes et al. (49) reported the optimization of a compound, analogous to the tyrosine kinase inhibitor lapatinib which had initially been identified as a potent antitrypanosomal. From this optimization, analogues were selected for a repurposing approach, and screened against S. mansoni parasites. As a result, several potent compounds against the adult form of the parasite were identified and considered promising leads for further assessment as antischistosomal compounds. The main drawback to exploit kinases as drug targets is the difficulty to achieve selectivity among the vastness of homologues present both in schistosomes and humans. One promising route to achieve this selectivity is exploring allosteric binding sites as alternatives to the more conserved active sites.
Targeting histone-modifying enzymes (HMEs) has been a widely explored strategy for the discovery of new drugs to treat parasitic diseases. In S. mansoni, two classes of histone deacetylases (HDAC and sirtuins) have been identified and are considered potential drug targets for the treatment of schistosomiasis (159). Kalinin et al. (135) designed and synthesized a series of compounds, derived from weak human HDAC8 (hsHDAC8) inhibitors, which varied in the size and flexibility of their side chains. These molecules were screened on S. mansoni HDAC8 (SmHDAC8) to assess their ability to inhibit enzyme activity, and a potent and selective SmHDAC8 inhibitor was identified. Crystallographic and docking studies with SmHDAC8 and the compound revealed key interactions between them, which are not observed with the human orthologue hsHDAC8. Another study identified the first S. mansoni sirtuin 2 (SmSirt2) inhibitors with activity in the low micromolar range, potency against larval schistosome and adult worms, and no toxicity to human cells. These inhibitors were previously identified by an in vitro screening of a compound library, comprising potent and specific growth inhibitors of other parasites, such as Leishmania donovani and Trypanosoma cruzi (136).
Another drug target for schistosomiasis is thioredoxin glutathione reductase (TGR), an enzyme responsible for maintaining the redox homeostasis. A high-throughput screening against a compound library comprising 59,360 synthetic compounds was carried out, of which 74 inhibited SmTGR activity by more than 90% at 10 µM. Some of these had potent schistosomicidal activity against the larvae and adult worms (137). After the flood of date coming from the large q-HTS campaign, there was a feeling of certain disappointment since no major pre-clinical or clinical candidate arose from all this effort. However, the work with SmTGR as a drug target is slowly picking up pace again. A recent work selected the most active chemotypes from HTS plus analogues and re-tested against the enzyme. Ninety-seven had SmTGR inhibitory activity confirmed, and five of them killed S. japonicum, S. haematobium and S. mansoni (with LD50 ≤ 10 µM) adult worms, and all other development stages of S. mansoni (138). SmTGR has also been recently explored under the fragment-based drug discovery paradigm, as it will be discussed further ahead in this review.
3-oxoacyl-ACP reductase (OAR) is an enzyme involved in lipid biosynthesis that is absent in mammals. The cloning, expression, and purification of Schistosoma japonicum OAR (SjOAR) was performed by Liu et al. (139), as well as the elaboration of a homology model of the three-dimensional structure of this protein. A library consisting of more than 14,000 small molecules was chosen for an in silico screening against the model of SjOAR, and 30 initial hits were identified. Of these hits, two were shown to have schistosomicidal activity on both juvenile and adult forms, relatively low cytotoxicity, and could significantly inhibit the activity of the purified recombinant enzyme, confirming that SjOAR is the primary target of these compounds.
From a library focused on exploring phosphodiesterases (PDEs) as potential drug targets for several parasites, 265 compounds were obtained and had their antischistosomal activity evaluated (160). In vivo screening revealed that 171 of the compounds had activity against adult parasites. All these hits showed some level of activity in a mouse model, and two of them, when combined with PZQ, managed to a near complete eradication of viable eggs. Despite being structurally related to PDE10 inhibitors, further studies are needed to validate SmPDEs as the targets of these compounds (140). Another important work was carried out by Long and colleagues (81), which undertook considerable efforts to validate S. mansoni PDE4A as a target for a series of benzoxaboroles. From a library of 1085 benzoxaboroles, the authors identified some compounds which induced hypermotility and degeneration of S. mansoni worms. Employing phenotypic assays with transgenic C. elegans, chemical and functional characterization, it was possible to observe a positive correlation between the hypermotile phenotype of the parasite and the inhibition of SmPDE4A, suggesting that this enzyme is a target for the tested bezaxoboroles. In another recent study, inhibitors of SmPDE4A were discovered, using a virtual screening approach. Homology models of the enzyme structure were generated and used to screen a chemical library. 25 hits were selected and tested as inhibitors of the recombinant SmPDE4A, and five of them were able to inhibit its activity (141).
Schistosome aspartic proteases, as well as cysteine proteases, play a major role in life cycle of Schistosoma parasites by breaking down host hemoglobin an essential source of amino acids from the parasite. Studies have shown that reductions in transcript levels of SmCD1, an enzyme of S. mansoni similar to cathepsin D, lead to phenotypic changes in the parasite, such as growth retardation (161). Thus, SmCD1, as well as the orthologue from S. japonicumi (SjCD1), are considered validated targets in antischistosomal drug discovery. A homology modelling study and SAR analysis with peptidomimetic compounds designed against SjCD1 revealed unique structural features for achieving selectivity to this enzyme (144). Recombinant SmCD1 was recently expressed in HEK293 cells, characterized biophysically and biochemically (153). This is an important step towards further exploring this enzyme in TDD, since they can be considered promising druggable targets as demonstrated in the past with the development of HIV-1 protease inhibitors.
With over 10 years of publications, cysteine proteases are some of the oldest targets against schistosomiasis and S. mansoni cysteine protease cathepsin B1 (SmCB1) is one example. The SmCB1 has stood out as an important target for drug development. Some studies have described structural and functional characteristics (152, 162) of how SmCB1 is inhibited. Some of these applied scoring methods based on quantum mechanics (QM) to describe important interactions between vinyl sulfone chemotype inhibitors and SmCB1 (163). Furthermore, these inhibitors were important to map druggable hot spots in SmCB1 (150). The vinyl sulfones inhibitors also showed desirable properties such as activity in phenotypic assays, selectivity for SmCB1 over human cathepsin B and metabolic stability. Besides this new SmCB1 inhibitor class, in a recent publication Jiková et al. (149) showed that azanitriles chemotypes can act as potent covalent inhibitors of SmCB1. Using recombinantly expressed SmCB1, crystal structure determination, QM methods, phenotypic and target-based assays, these authors were able to identify azanitriles with nanomolar range potency. These studies trace an important path to the identification of new molecules with therapeutic potential to treat schistosomiasis, whilst reinforcing the importance of SmCB1 as a valuable S. mansoni drug target.
In addition to enzymes, receptors (164–166) and transporters (62, 166) are also targeted in schistosomiasis drug discovery. Serotonin (5-HT) GPCRs have already been identified in S. mansoni and related to worm movement regulation (66). Marchant et al. (78) characterized the pharmacological profile of the schistosome receptor Sm.5HTR, a GPCR involved in worm movement, and a screening of 143 previously studied compounds was performed, leading to the identification of scaffolds that regulate the activity of this receptor Similarly, a commercial GPCR compound library has been screened against Sm.5HTR, and 23 compounds identified as potential antagonists, with the majority showing selective inhibition of the parasite serotonin receptor (167).
In 2019, a paper published by Park et al. (58) presented important insights regarding the role of praziquantel on schistosome worms. This work showed that PZQ activates a S. mansoni transient receptor potential channel (SmTRPM) showing properties consistent with the observed responses on worms, like nanomolar sensitivity to PZQ, stereoselectivity and sustained Ca2+ entry response. The authors were able to identify nanomolar sensitive of SmTRPM to (R)-PZQ isomer (eutomer), which is approximately 50 times more sensitive to (S)-PZQ. Further screening campaigns will be necessary to assess the therapeutic potential of this target. Nevertheless, these findings elevate the SmTRPM as a promising clinical target to treat schistosomiasis.
In the last decades, fragment-based drug discovery (FBDD) has been established as an efficient approach for the identification of new biologically active compounds (168–172). To date, four marketed drugs have been discovered by FBDD (173), including vemurafenib (174), venetoclax (175), erdafitinib (160), and pexidartinib (176), while over 40 fragment-based drug candidates are in different stages of clinical trials (177). In FBDD campaigns, small and less complex compounds, commonly with molecular weight (MW) <300 Da and <20 heavy atoms, are screened against therapeutic targets (178, 179). The use of very small molecules offers advantages over screening larger compounds, including a more efficient sampling of chemical space with fewer compounds (180), higher hit rates (181), and also better physicochemical properties (182, 183). Besides, lower investments are needed, and FBDD projects progress relatively faster between the research and development (R&D) phases (184). As an example, vemurafenib took only six years from hit identification to the approval by the US Food and Drug Association (FDA) in 2011 (171, 174). Therefore, incorporating FBBD into anti-schistosome drug discovery may help to accelerate the identification and development of drug candidates for schistosomiasis and other NTDs (185, 186). FBDD involves steps of library design, screening, and optimization and these steps are discussed in the subsequent sections.
Most early fragment libraries were designed based on the Rule of Three (RO3), i.e. MW ≤300 Da, the number of hydrogen bond donors ≤3, the number of hydrogen bond acceptors is ≤3 and cLogP is ≤3 (178). However, this paradigm has been changing based on incremental experience in FBDD acquired in the last years and considering the facilitation of fragment screening and/or subsequent fragment optimization chemistry (187, 188). Nowadays, several strategies exist, which cover the use of labeled fragments for nuclear magnetic resonance (NMR) spectroscopy, covalent linkage for mass spectrometry, dynamic combinatorial chemistry, X‐ray crystallographic screening of specialized fragments and fragments optimized for easy elaboration (189).
The last two strategies are blended and available to the community through the XChem fragment screening facility at Diamond Light Source in the UK (190). For this facility, a library of chemical compounds poised for expansion called DSPL (191) was designed to allow rapid and low cost follow-up synthesis and to provide quick SAR data through X-ray crystallography. Poised fragments contain at least one functional group which can be synthesized using a robust, well-characterized reaction. Reactions include amide couplings, Suzuki-type aryl-aryl couplings and reductive aminations, amongst others. The library was designed by analyzing all commercially available fragment space (using the ZINC reference library), yielding nearly 30,000 compounds of which a chemically diverse subset of 800 compounds was selected for the poised library. In practice, the available chemical material shows bias towards the most commonly used chemical reactions (192), however, it still the case that the XChem program delivers between 2% and 10% fragment hit rates (soaks yielding bound fragments in the structure) for projects amenable to multi-crystal soaking and screening by crystallography. A remarkable result exemplified by the more than 100 projects screened since 2016, including the successful screening and follow-up of the SARS-CoV-2 Mpro protein, also released as an open science public service (193, 194).
Screening strategies rely on identifying and ranking chemical fragments that bind to the protein target. Methods need to be sufficiently sensitive to measure low affinity interactions and therefore do not typically rely on activity assays. The NMR, surface plasmon resonance (SPR), thermal shift assays (TSA) (also known as differential scanning fluorimetry (DSF) and X-ray crystallography are the most widely used techniques for high throughput fragment screening.
The NMR spectroscopy is the most robust fragment screening method for detecting very weak binding (KDs in the µM to mM range). The NMR approach used to identify target-ligand interactions can be based on observation of the target (target-observed) or ligand (ligand-observed) (195). In the case of ligand-observed NMR methods, only the resonance of the nuclei present in the ligands is measured (196). This type of approach includes methods such as saturation-transfer difference (STD), water LOGSY, cross saturation (CS) and transferred-cross saturation (TCS), transferred nuclear Overhauser effect (trNOE), NOE editing/filtering diffusion editing, relaxation editing, use of paramagnetic tags and residual dipolar couplings (197, 198). On the other hand, the target-observed methods provide data on the target nuclei that are directly involved in the interaction with the ligand (197). Among the target-observed methods, there are chemical shift mapping using 15N-HSQC, backbone amide hydrogen exchange and solvent paramagnetic relaxation enhancement methods. In comparison with other methods, NMR spectroscopy has the advantage of being conducted in solution, which allows the protein to be as close as possible to its native conformation. In addition, NMR enables both the target and the ligand to be structurally characterized serving as quality control assay to verify the structural integrity of the ligand. However, compared to other methods, fragment screening by NMR is relatively slow (199).
Screening by SPR involves immobilization of the target protein on a gold or silver sensor surface and measurement in the change in reflected light following ligand interaction (200, 201). The method is high throughput and very sensitive (µM to nM range) providing kinetic binding data (Kon and Koff rates), from which, KDs are calculated. The main disadvantage of the technique is the potential difficulty of immobilizing proteins in native conformation and therefore it is important to test a reference compound to assess correct binding behavior. The relatively high concentration of immobilized target and fragment affinities can lead to false positives through non-specific binding (202).
In the thermal shift assay protein denaturation is monitored by fluorescence either intrinsic tryptophan fluorescence or using dyes that preferentially bind partially or completely denatured proteins (203). Ligand binding is measured indirectly as the increase in thermal stability resulting from interaction with the target protein in the native state (204). This method is easy, fast and inexpensive for fragment screening (185). However, could not be appropriate for all target proteins, because indirect readout of the protein’s denaturation, and chances to generate false positives. Thereby, it is necessary to confirm the identified hits with other methods (205).
Crystallography is the current method for delivering atomic resolution information and is arguably the method of choice for primary screening if a project is amenable to this approach, i.e., access to high quality purified protein that can be reproducibly crystallized (206). There are two strategies for obtaining protein-fragment complexes, namely, co-crystallization or soaking (207). Co-crystallization is the mixing of the free protein in solution with a ligand prior to crystallization, which allows the small molecule to bind to the protein prior to crystal lattice formation. This is the preferred method if a protein complex with a specific ligand is required. A potential downside is co-crystallization with different small molecules can lower the success rate for crystallization, or introduce changes in resolution and crystal form, burdening the downstream analysis and limiting high throughput. In crystal soaking, false negatives come from protein failing to crystallize, or protein crystals growing without bound compounds. A simpler approach is soaking, where the compound is added, (dissolved or pure), directly to the crystallization drop which already contains crystals. In this method, compounds diffuse through solvent channels in the crystal accessing binding pockets in the protein (208). However, false negatives can still arise through a lack of fragment binding. Compounds may also dissolve crystals by disrupting the crystal lattice. Both co-crystallization and soaking are sensitive to low compound solubility.
The fragment hits commonly have weak binding affinities (from mM to high µM range) as a consequence of the reduced number of heavy atoms to form attractive interactions with the target (209). Despite the low MW, the fragment hits form high-quality interactions, i.e., highly energetically favorable interactions that surpass the entropic penalties for binding (210, 211). Thus, fragments constitute starting points that can be optimized iteratively into larger higher-affinity compounds – a process known as fragment-to-lead (F2L) (205, 210). The F2L process is guided by the information of binding mode, growth vectors available, and ligand efficiency (LE) and its derivatives (212, 213). LE is a metric used to describe the average free energy of binding per heavy atom (Equation 1) (214), and LE ≥0.3 is frequently used to select the most promising fragment hits to F2L (183). Three main strategies are used to optimize fragments, including fragment growing, merging, and linking (Figure 2).
Figure 2 A schematic illustration of fragment optimization strategies. (A) Fragment growing: initial fragment with low affinity is optimized by stepwise addition of functional groups to obtain a larger compound with high affinity. 3D and 2D schemes represents the growing evolution of navoximod, an indoleamine 2,3-deoxygenase 1 (IDO1) inhibitor with antineoplastic properties (solid tumors) (215); (B) Fragment merging: two or more fragments sharing the same pocket are covalently merged to obtain a larger compound with higher affinity. 3D and 2D schemes represent an example of fragment merging to the discovery of inhibitors of the Mycobacterium tuberculosis cytochrome P450 CYP121 (216). (C) Fragment linking: two or more fragments bound independently in proximity are covalently linked with suitable linkers to obtain a larger compound with higher affinity. 3D and 2D schemes represent an example of fragment linking to the discovery of inhibitors of M. tuberculosis pantothenate synthetase (217).
(1) where, ΔG is the free energy of binding and HAC is the heavy atoms count of a compound.
Fragment growing (Figure 2A) is the most commonly applied strategy in F2L (218). The effectiveness of this strategy is shown by its use in F2L process of three out of the four marketed drugs derived from FBDD (173), namely vemurafenib (174), erdafitinib (160), and pexidartinib (176). The fragment growing strategy involves a several steps. Firstly, potential growth vectors are identified in the chemical structure of the fragment hit (212, 219). Then, atoms or chemical groups are added to the fragment hit to explore additional interactions with the binding site and increase the potency (205). Structural information of the binding mode is essential during fragment growing to identify potential sub-pockets to explore and assess the maintenance of the fragment’s original binding mode and additional molecular interactions (198, 220). At each iteration of growing, synthesis and testing, success can be evaluated by LE, monitoring if the extra molecular mass added was beneficial (221).
Fragment merging (Figure 2B) can be applied when two fragments bind in an overlapping position of the binding site and can be merged into a unique and more potent hybrid compound (222, 223). As in fragment linking, both fragments can work additively when merged or even synergistically (224). Here, structural information is also crucial to understand the binding mode (198). Fragment merging is also difficult to achieve and less frequently used because of the challenging task of maintaining the original binding modes of the fragments after merge (225).
Fragment linking consists of the connection of fragments binding to different but adjacent sub-pockets in the binding site by a linker moiety (Figure 2C) (168, 177) and is the most powerful strategy for converting fragments into potent ligands (198). This is due to the potential super additivity effect, where the binding free energy of linked fragments is higher than the sum of binding free energy of the individual fragments (226, 227). The main challenge is the design of a linker group that does not affect the original binding mode of fragment hits (218). As a successful case, the marketed drug venetoclax was optimized by applying the fragment linking method (173, 177).
The SmTGR is a flavoenzyme expressed by schistosomes involved in the detoxification pathways that are pivotal for their survival in the host organism (228–230). Most SmTGR inhibitors are reactive electrophilic compounds, such as metal derivatives or Michael acceptors, presumably targeting the nucleophilic residues (selenocysteine and low pKa redox active cysteines), which may result in low selectivity and toxicity (231, 232). Attempts to obtain crystal structures of SmTGR in complex with such inhibitors have been unsuccessful, reflecting the problem of crystallizing non-homogenous protein preparations resulting from the presence of several redox and nucleophilic centers in the SmTGR, which are the sites of action for electrophilic inhibitor (233).
Given the challenges posed by the redox properties of the enzyme, allosteric and secondary binding sites could be explored, as they present less reactive amino acids which could lead to less toxic and more selective inhibitors. For this reason, Silvestri and coworkers (233) prioritized 1,000 fragment inhibitors of the SmTGR from a quantitative HTS campaign. Then, by X-ray crystallography identified two fragments (1,8-naphthyridine-2-carboxylate and 1-(2-hydroxyethyl)piperazine) that bound in a secondary pocket adjacent to the NADPH binding site (Figure 3), named as “doorstop pocket”. The pockets are separated by the Tyr296 residue, where the aromatic ring of Tyr296 could adopt the closed (Figures 3A, D) and open (Figures 3B, C) conformations. Small molecules bound at the doorstop pocket disturb the well-known and conserved conformational adjustments associated with NADPH binding and enzyme reduction (233). Subsequently, chimeric compounds blending the structural features of the initial fragments into single compounds were synthesized and showed improved SmTGR inhibition activity, ex vivo activity against larval and adult S. mansoni worms at low micromolar concentrations. In addition, the designed compounds tended to have selectivity for SmTGR, as the amino acid residues of the doorstop pocket are not conserved between members of the FAD/NAD-linked reductase family (233). Although strictly this work was not a FBDD campaign (at least not originally designed as one), it was an interesting effort that showed the potential of the fragment-based approach to disclose new binding sites that can be explored to develop novel potent ligands.
Figure 3 Doorstop pocket of SmTGR adjacent to the NADPH binding site. The Tyr296 of the doorstop pocket is represented in (A) closed and (B) open conformation, as well as in the presence of (C) NADPH and (D) two fragments (1,8-naphthyridine-2-carboxylate and 1-(2-hydroxyethyl)piperazine).
Although FBDD has been established as an efficient approach for the identification of new biologically active compounds for several diseases, very few applications had been reported for schistosomiasis. Thus, FBDD has a great potential for anti-schistosomal drug discovery in the future.
In silico approaches have been used in several parts of FBDD pipelines as an alternative or complementary approach, with the benefits of speed and low cost (234, 235). Many fragment libraries are available in the literature (188) and computational methods can be used to design a fragment library with high chemical diversity, synthetically accessible to be easily optimized during F2L, and also select or exclude fragments based on physicochemical properties (236, 237). The biophysical techniques applied for fragment screening are low-to-medium throughput (188), limiting the number of fragments that can practically be screened, and therefore the coverage of chemical space (180, 238). To compensate for this, molecular docking and machine learning can be used to virtually screen a large number of fragments and prioritize the most promising for experimental testing (224, 239–242).
Several in silico methods are also used during the F2L process (243). When no structural information about the binding is available, molecular docking and molecular dynamics are used to predict the binding mode and inform the growing, linking, and merging strategies (244, 245). The fragment hits can also be optimized with the help of de novo, machine learning, and deep learning methods (209, 243). These methods will be discussed in more details in the next sections.
The “-omics” era for schistosomiasis drug discovery started when the first versions of the S. mansoni (157, 246), S. haematobium (247), and S. japonicum (248) genomes were published. Recently, revised versions of the S. japonicum (249) and S. haematobium (250) genomes were released, enhancing the quality of the available genomics data for these three main trematode responsible for the majority of schistosomiasis cases in the world. The latest genome versions of these trematode species, together with other parasitic worms species, are available online at (https://parasite.wormbase.org/species.html#Platyhelminthes).
Most of the genome of schistosomes (like many other organisms) has yet to be explored experimentally. Consequently, bioinformatic tools and resources have become pivotal for the functional annotation and analysis of genes and their products. There are a wide range of general resources available that host biological information (genomics sequences, transcription data, protein structures, metabolomic data and more, see Table 4) as well as bioinformatic tools to perform analysis to unveil useful information within the data. Databases and webservers such as (but not limited to) InterPro (251), CATH-Gene3D (252, 253), the Conserved Domains Database (CDD) (254), HAMAP (196), PANTHER (255), Pfam (256, 257), PROSITE Patterns and Profiles (258), ProDom (196), PIRSF (196), PRINTS (259), SMART (260), Structure-Function Linkage Database (SFLD) (261), SUPERFAMILY (262, 263), TIGRFAMs (264) are integrated to identify specific motifs and domains to classify the protein of interest. Other resources such as the Kyoto Encyclopedia of Genes and Genomes (KEGG) database (265) provide functional annotation that encompasses molecular-level information about biological systems, integrating molecular datasets resulting from genome sequencing or other large-scale experimental technology. The KEGG website (https://www.genome.jp/kegg/) offers several tools to find data-oriented and organism-specific entry points, as well as analytical tools for diverse ends, such as genome and metagenome functional annotation (BlastKOALA and GhostKOALA respectively), pathway mapping tools (KEGG Mapper), sequence and chemical similarity search (BLAST/FASTA and SIMCOMP respectively).
Gene Ontology (GO) model (266) (http://www.geneontology.org) is commonly used for describing genes using a unified and common vocabulary applicable to any organism. GO is a hierarchical way of describing information gathered on genes and proteins at different levels of annotation and is used by many of the databases mentioned above as it provides the top three different categories of high-quality annotation: (i) biological process, (ii) molecular function, and (iii) cellular component; each one referring to the biological objective of the gene/gene product, biochemical activity of the gene/gene product, and place in the cell where the gene product is active respectively (267). The unified annotation/vocabulary provided by GO is dynamic and entirely based on the principle of shared orthology by all eukaryotic organisms. It can be updated as the ontologies become mature through the integration of more experimental results.
As an exemplar of how these tools and resources can be used in the context of Schistosoma is by Padalino and colleagues (40) who identified S. mansoni Lysine Specific Demethylase-1 (SmLSD1) as a druggable epigenetic target, as well as daunorubicin and pirarubicin as potential inhibitors. This was possible using bioinformatics tools, such as Uniprot, PROSITE, InterPro, and Pfam, BLAST in combination with homology modeling, molecular docking, and a whole-organism screening.
Likewise, the latest revised versions of S. haematobium and S. japonicum published by Stroehlein et al. (250) and Luo et al. (249) demonstrate the use of several tools in an extensive way. Luo and colleagues (249) combine tools whose functions range from genome evaluation to RNA, protein prediction and phylogenetic analysis and all of them converge to the evaluation and comparison of the revised genome and the previously published versions. Stroehlein and colleagues (250) used a lesser extent of tools, however a deep comparison between previously published versions of Schistosoma genomes was conducted and a careful data curation was carried out to ensure the quality of the assembled genome.
Since 2017, many efforts in terms of RNA-seq data have been reported in the context of Schistosoma (85, 269–272). A thorough protocol of how to gather, process, reconstruct the transcripts, and identify novel long non-coding RNA (lncRNAs), as well as their expression levels (273). In this protocol, Maciel and Verjovski-Almeida use a set of open-source Unix-based tools combined with several R (274) packages to support the analysis of differential expression of some lncRNAs. Wang and colleagues (85) reported a large RNAi screening against S. mansoni to uncover new therapeutic targets. The authors conducted a GO enrichment to better understand the roles of the essential genes to the parasite development and attachment to substrate. Furthermore, this study demonstrated the essentiality of SmTK25 kinase to maintain the muscular function of the parasite, thus representing a promising therapeutic target.
One of the principal methods for integrating and inferring functional annotation is phylogenetics - the study of the evolutionary story of organisms and their relationships with other organisms or group of organisms. The relationships among the organisms are described in a detailed and hierarchical manner through phylogenomic inference methods, which will result in a phylogeny, represented by a phylogenetic tree (275). It is the best way for identifying and confirming whether two or more sequences are orthologs (276).
Phylogenomic inference methods are applied to assign a biological function to an unannotated gene or protein (277). Their overall accuracy is high and theoretically the topology of a generated phylogenetic tree is correct unless highly dissimilar sequences (identity <25%) are present among the aligned sequences (278). The methods are directly dependent on a multiple sequence alignment (MSA), whereby main objective is to align more than two sequences, allowing the identification of conserved motifs, domains and regions in the compared sequences (nucleic or amino acids) (277). Therefore, the quality of a final phylogenetic tree will strongly depend on the MSA quality and accuracy. The most popular tools for phylogenetic analysis are PhyML (279), RAxML/ExaML (280), FastTree (281), and IQ-TREE (281, 282).
There are many methods that can be used to build phylogenetic trees from an MSA. Distance-based methods such as neighbor-joining and Unweighted Pair Group Method with Arithmetic Mean (UPGMA) are the simplest examples which provide a genetic distance calculation between the multiple sequences aligned, but do not give evolutionary information (275, 282, 283). More complex methods, such as maximum parsimony, minimum evolution, and maximum likelihood can be employed considering the Bayes’ theorem for the estimation of an evolutionary model (284, 285).
Maximum parsimony’s principle relies on the sum of the number of minimum possible sites substitutions in each sequence. The sum will constitute the tree length for the investigated topology and the topology with the minimum length is called maximum parsimony tree (279). Minimum evolution is a distance-based method which generates a tree topology based on the lowest value among the values obtained from the sum of all branches (286). This method has a high time-cost mainly when dealing with too many sequences, e.g. protein superfamilies. The Maximum likelihood statistical method is known as the method which produces the most reliable phylogenetic trees in comparison with distance-based methods and the parsimony method (283). A phylogenetic tree is constructed through the maximum likelihood method accordingly to the following steps: (i) generate a starting tree; (ii) rearrange the starting tree through topological substitutions and evaluate the new tree; (iii) replace the starting tree and repeat the step ii if no better tree is identified; on the contrary, terminate the search (283).
For the cited methods, even for those based on distance, the robustness of the tree is assured by a bootstrap resampling technique (275, 282, 287) which is based on the replacement of nucleotides, codons or amino acids and the construction of a new tree with the new sequences. Next, each interior branch of the original tree is compared to the newly branches and, if the branches are different, a bootstrap score 0 is given while a score 1 is assigned to the other branches. The process is repeated a few hundred times, the percentage of times which the bootstrap score 1 was given is calculated, and the topology can be considered correct if the percentage is equal or greater than 95%. In the context of Schistosoma species and other helminths, these types of phylogenetic analysis have been boosted by the availability of a wide range of high quality genomes captured and analyzed within the 50 Helminth Genome Project (https://www.sanger.ac.uk/collaboration/50hgp/) and well as the other genomes and resources found in WormBase (268).
Despite all advances achieved in the field of automation of screens, FBDD, and also in the understanding of disease biology in the post-genomic era, delivering new drugs to the market remains a highly complex, expensive and time-consuming process (288, 289). Therefore, there is a need for innovative approaches that could bring new drugs for patients at a lower cost-to-market. In this context, computer-assisted drug design approaches (CADD) has been considered as a potential opportunity (290, 291). Cheminformatics is a field of CADD and has the objective of utilizing computer and information sciences to solve problems in the area of chemistry (292, 293). This involves the design, creation, retrieval, storage, management, organization, analysis, visualization, dissemination, and use of chemical information (294). Over the last few years, the advances in data processing power and the development of new artificial intelligence (AI) tools, has fueled the field of CADD and cheminformatics (295, 296). Moreover, AI tools abilities have increasingly been applied to a wide variety of chemical challenges, from improving computational chemistry to end-to-end drug discovery as well as to synthesis planning/prediction (297, 298).
The developments in phenotypic and target-based screening provide data essential for applying computational tools to accelerate the discovery of new drugs to treat schistosomiasis (299, 300). These advances coupled with data storage in public databases such as PubChem (301–303) and ChEMBL (304) have enabled the compilation, curation, analysis, and application of chemical and biological information to support antischistosomal lead generation and optimization (305–307). Thus, cheminformatics has an important role in schistosomiasis drug discovery through the conversion of data to information and information into knowledge (308, 309), supporting data-driven decisions in lead identification and optimization (310, 311).
In the last years, pivotal advances in cheminformatics-driven drug discovery have been achieved in three main sub-fields: molecular de novo design, virtual screening, and synthesis prediction. Machine learning approaches have also progressively been applied in these areas. Therefore, in the next sections, machine learning approaches will be described and important aspects to schistosomiasis drug discovery will be highlighted.
Machine learning (ML), mainly supervised methods, is a growing field of AI that uses different algorithms to enable computers to learn from sample data, known as “training data”, without being explicitly programmed for this task (312). ML algorithms are capable of recognizing complex patterns in chemical structures that evade human rationales because of the enormous number of parallel variables that should be addressed in drug design (313). On the other hand, molecular modeling techniques (e.g., docking, molecular dynamics) are based on explicit physical equations derived from molecular mechanics and quantum mechanics theory (314). Consequently, ML techniques are considered to have higher predictive value than classic molecular modeling methods. Combining human and ML -derived models should enable medicinal chemists to make better decisions and move projects forward more quickly (315, 316).
ML has applications in several stages of drug discovery and development, accelerating the overall process (317), including automation of whole-organism assays (70, 94), lead identification and optimization (318), and clinical development, for example in patient recruitment, prediction of diagnosis, prognosis, treatment planning, and clinical trial outcomes (317, 319). ML provides robust methods such as random forest (RF) (320, 321) for learning from large and multi-dimensional chemical data to make predictions and select new chemical entities for experimental testing (322). The generation of ML models for drug design and discovery consists of a multi-step protocol (323). The first step is the data collection of chemical and biological information from the literature and/or databases, followed by preparation and curation of data employing standardized protocols (324–326). Then, descriptors are calculated from molecular representations varying from one-dimension to n-dimensions (327). These molecular descriptors are derived from a logical and mathematical method that converts the chemical information into a useful number (328). The third step is the model training (learning), where a ML technique is applied to establish Quantitative Structure-Activity Relationships (QSAR) between the molecular descriptors and continuous (e.g., pIC50, Ki, etc.) or categorical/binary (e.g., active, inactive, toxic, nontoxic, etc.) experimental bioactivities or properties (329, 330). The models that are developed need to be validated using appropriate metrics to assess their predictive value (331, 332), and then used to predict the biological activity of new compounds (318).
It is worth pointing out that the initial training data underpins ML models generation. The data should be high-quality and in sufficient quantity to lead in models with high performance (295). However, in the current scenario, the data of pharmaceutical industry is scarce, costly, and need substantial resources, which could limit the use of ML for drug discovery (329).
Some guidelines for model generation and validation should be followed to ensure the reliability of the model. In this context, some principles for assessing the validity of ML-based QSARs have been proposed by the Organization for Economic Cooperation and Development (OECD) (333) stating that they should have:
i. A defined endpoint: Ensure clarity in the endpoint being predicted by a given model, since biological property could be determined by different protocols and under different experimental conditions;
ii. An unambiguous algorithm: ensure reproducibility in the ML algorithm that generates predictions of an endpoint from chemical structure.
iii. A defined applicability domain (AD): the AD is defined as the chemical space containing the features of the compounds used to train the ML-based QSAR models (334). The AD offers means to assess the confidence of prediction to unseen compounds (335). The most common methods to define AD use distance-based metrics to calculate the distance of the features between the training set and a new compound being predicted (335–337).
iv. Appropriate measures of goodness-of-fit, robustness, and predictivity: ensure the distinction between the internal performance of a model (as represented by goodness-of-fit and robustness) and the predictivity of a model (as determined by external validation);
v. Mechanistic interpretation, if possible: ensure that some consideration is given to the possibility of a mechanistic association between the descriptors used in a model and the endpoint being predicted (333).
As an example of application of ML to schistosomiasis drug discovery, Zorn and coworkers (338) used data from phenotypic screens against the schistosomula and adult stages of S. mansoni to develop ML models. Firstly, the authors elaborated two rule books and associated scoring systems used to normalize 3,898 phenotypic data points and transform to categorical data. Then, using the Assay Central software, they generated eight Bayesian machine learning models based on each developmental stage of the parasite and four experimental time points (≤24, 48, 72, and >72 h). Subsequently, the generated models were used to predict the activity of compounds from several libraries of commercial vendors. Finally, 40 compounds predicted as active and 16 compounds predicted as inactive were selected and purchased for in vitro phenotypic assays against schistosomula and adult stages of S. mansoni. In this manner, the authors achieved a prediction accuracy for active and inactives of 61% and 56% for schistosomula and adults, respectively. Additionally, the hit rates achieved were 48% and 34% for schistosomula and adults, respectively (338).
DL is a type of ML that uses a hierarchical recombination of features to extract pertinent information and then learn the patterns represented in the data. In other words, DL uses artificial neural networks (ANNs) with many layers of nonlinear processing units for learning data representations. DL has emerged to deal with the high volume and exponentially growth of sparse data, coming from different sources around the globe (339). Conceptually, DL was conceived in the 1980s, with the development of ANNs, which, at the time, could not out-perform ML algorithms due to the small amounts of data available. As soon as advances in hardware were achieved, in 2010s, with graphic processing units and cloud computing technologies, deep neural networks (DNNs) became more popular and able to be trained and accomplish complex tasks (340).
The basic structure of a classical ANN and DL representations are represented in Figure 4 and are inspired by the structure of the human brain. There are three basic layers in a neural network: the input layer, hidden layer and output layer. Depending on the type of ANN, the nodes, also called neurons, in neighboring layers are either fully connected or partially connected. The major difference between DL and traditional ANN is the complexity of the NNs. Traditional ANNs (Figure 4A) normally only have one hidden layer whereas DL architectures such as Deep Feed Forward Network (Figure 4B) uses larger numbers of hidden layers.
Figure 4 Architecture of several popular neural networks. (A) classical Feed Forward Network; (B) Deep Feed Forward Network; (C) Convolutional Neural Network; (D) Recurrent Neural Network; (E) Variational Autoencoder Network; and (F) Generative Adversarial Network.
Since the advent of QSAR in the 1960s for drug discovery projects, and the use of so called “shallow methods” for the identification of new chemical entities with drug like properties (341), the application of DL methods has been increasing (276, 315, 317–323). The applications of DL can be as diverse as the creativity of those who applies and develops the methods. The possibilities are unlimited in terms of algorithms [see (342)] but are restricted in terms of data quality and chemical space coverage (325). However, DL has broadened even the ability of generating new chemical data, allowing the usage of autoencoders to interpret SMILES data and, within that chemical space, generate new scaffolds sharing a few physicochemical properties with their parental molecules (343). From virtual screening processes to synthesis prediction, DL has been largely used in the field of CADD, and its applications are exemplified in the next sections.
As a fundamental part of CADD strategies, virtual screening (VS) is an in silico screening alternative to the experimental HTS approach to search libraries of small molecules and identify those structures which are most likely to have biological activity (344, 345). VS represents a rapid and low-cost and method for screen promising compounds against pathogens, cells and/or specific biological targets (344, 346). A VS campaign is basically a funnel-like process (347) composed of different filters. A large chemical database can be submitted to those different filters and, throughout the process, the compounds presenting undesired properties will be filtered out. In the end of the process, virtual hits with drug-, lead- or even fragment-like properties are presented in a ranked list.
In the last few years, the success of machine and deep learning has enabled the development of VS methods that can extract task-specific features directly from chemical data (295, 296). Convolutional Neural Networks (CNN, Figure 4C) are a subclass of DL that search for recurring spatial patterns in data and compose them into complex features in a hierarchical manner (348, 349). Chemical descriptors have very high dimensionality, and hence training a standard Feedforward network to recognize chemical patterns would require hundreds of thousands of input cells. This can cause many problems associated with the “curse of dimensionality” in neural networks. The CNNs provide a solution to this (350) by utilizing convolutional and pooling layers to help reduce the dimensionality from compound graphs (351). As convolutional layers are trainable but have significantly fewer parameters than a standard hidden layer, they can highlight important parts of the chemical structure and pass each of them forward.
Although DL have important advantages, the most prominent demonstration of DL’s capability are in areas where large amount of data are available, which is not the reality of all drug discovery campaigns (297, 352). In addition, studies demonstrated that simpler ML methods can outperform DL for activity prediction (353).
Regardless of the advances in cheminformatics, the conception of the large majority of new molecules in drug discovery campaigns comes from the inventiveness of medicinal chemists (354). Since the 1990s, de novo methods have been used to design new molecules from scratch, commonly using structure-based approaches and resulting in compounds that are sterically and electrostatically complementary to the binding site of a protein target (355). However, the molecules generated by early de novo design methods were usually synthetically challenging, with poor pharmacokinetic properties, and the generation process required long runtimes (356, 357).
With the progress in deep learning, a variation of the de novo design method called generative modeling has appeared as a promising approach (358). These methods model the underlying probability distribution of chemical features from a training dataset and, thus, learn the essential aspects that characterize molecules (296, 359). Then, new molecules are generated combining these features by sampling the learned distribution of chemical features (360). The most common deep learning architectures for generative models are Recurrent neural networks (RNNs) (361), generative adversary network (GAN) (362), and variational autoencoder (VAE) (Figure 4) (363).
The RNNs (Figure 4D) are commonly trained with a large number of Simplified Molecular Input Line-Entry System (SMILES) strings, which encode chemical structures (364). Then, the RNN predict the probability of the next SMILES character considering a sequence of preceding characters (365). Thereby, the new molecules are generated by RNN character by character until the required number of characters have been produced (360).
The VAEs (Figure 4E) are composed by an autoencoder model that contains an encoder and a decoder network. The encoder translate a higher-dimensional molecular representation (e.g., SMILES) into a lower-dimensional representation, called latent space (366). The decoder translate the latent-space representation back to the higher-dimensional representation to generate new molecules (295, 366, 367). In addition, this network uses probabilistic hidden cells, which applies a radial basis function to the difference between the test sample and the cells’ mean. In this sense, VAE learns the parameters of a probability distribution representing the chemical structure data. Instead of just learning a function representing the chemical space, it gains a more detailed and nuanced view of the chemical structures, sampling from the distribution and generating new chemical structures (359).
GANs (Figure 4F) consist of two specialized networks that “contest” with each other: a generative network and a discriminative network (367). With careful regulation, these two adversaries compete with each other, each’s drive to succeed improving the other. The end result is a well-trained generator that can spit out a new chemical structure with desired biological property. The generative network (usually a CNN) tries to generates new molecules, while discriminative network tries to discern generated molecules as artificial or real (296). Mechanistically, discriminating network receives either training data or generated content from the generative network. How well the discriminating network was able to correctly predict the biological property is then used as part of the error for the generating network. Both networks are trained alternatively aiming the generation of molecules that are indiscernible from the real data (319).
In addition to the deep learning architectures for generative modeling, it is possible to use techniques such as transfer learning and reinforcement learning to fine-tune the models to generate molecules with the desired properties (e.g., activity against a target and physicochemical properties) and also optimize compounds such as fragments (368, 369). The power of these methodologies is the design of new molecules with ideal properties in shorter periods and lower costs (370, 371).
Despite the innovation of generative models, the novelty and accessibility of generated molecules must be evaluated (372–374). Gao and Coley (375) observed that generative models can produce infeasible molecules even with good performance in benchmarks. On the other hand, in a work for the discovery of discoidin domain receptor family member 1 (DDR1) kinase inhibitors (370), Walters and Murcko (366) pointed out that the top inhibitor is very similar to a known DDR1 inhibitor (366, 371).
The synthetic feasibility of virtual compounds identified in VS campaigns is a key point when considering synthesizing and further optimizing their properties (376, 377). Efforts from several research groups to improve the evaluation of synthetic routes and their inherent accessibility have been published and well-known software has been produced, e.g. SYNCHEM (378), RASA (379), LHASA (376), CAMEO (380), SOPHIA (381), EROS (382), and Reaxys (295, 383). Their main goal is to assess synthetically accessible routes, reaction predictions, and start material selection. To achieve this goal, approaches based on basic rules for organic synthesis, data-driven intelligent systems, sequence-to-sequence, template-based models, knowledge-graph based, and retrosynthetic prediction models have been proposed and published (384–386). To list the main obstacles for reaching a good accuracy in predicting both accessible synthetic routes and retrosynthetic disconnections, we can point out (i) the low number of unsuccessful reactions reported, (ii) the extensive data curation process, which impacts on the data quality and, consequently the predicted outcomes (384, 385). The current state-of-the art relies on treating the task as a text processing problem. Natural Language Processing (NLP) algorithms have been tested, implemented, and shown to provide of promising outcomes. The IBM RXN platform (387) represents a successful application and example of how to deal with chemical reactions as text. The platform uses the simplified molecular-input line-entry system (SMILES) as the source of local and global features potentially involved in a chemical reaction (388).
As well as in FBDD, very few applications of cheminformatics have been reported for schistosomiasis. Thus, cheminformatics also has a great potential for anti-schistosomal drug discovery in the future.
In conclusion, we would like to emphasize that the recent advances in automation of whole organism screening and target-based assays, as well as FBDD, CADD and AI tools integrated in drug design projects, represent a new era in anti-schistosomiasis drug discovery. The phenotypic assay methods described here are more sensitive and faster than traditional microscopy an have enabled the identification of several new antischistosomal candidates. In parallel, significant contributions are coming from the genomics to target-based screening approaches, especially with prospecting and prioritizing biological targets with key/essential roles in parasite survival and/or host-parasite interactions. The automated collection and processing of X-ray crystallography data at synchrotons has transformed fragment-based screening enabling the acquisition of structural data at atomic resolution. The generation of large datasets from advances in the automation of phenotypic screening and target-based approaches has created a fertile ground for drug discovery. These data have enabled the use of artificial intelligence tools, such as machine learning and deep learning, to generate predictive QSAR models for prioritization of VS hits or structural design of novel compounds. These tools can be also used for in silico multi-parameter optimization, for achieving a favorable balance between target potency, selectivity, physicochemical, pharmacokinetic and toxicological properties. Therefore, we see the use of AI tools and QSAR models as a time-, labor-, and cost-effective way to discover hit compounds and to optimize lead candidates in the early stages of drug discovery process. We hope that these new technologies collectively will empower schistosomiasis drug discovery and increase the efficiency of the various processes involved to deliver new drugs to the market.
All authors contributed to the article and approved the submitted version.
The authors would like to thank Brazilian funding agencies, CNPq, CAPES, FAPERJ, FAPEG and FIOCRUZ for financial support and fellowships. JM-F, AS, and LS are supported by CAPES fellowship (Finance code 001). CA and FS-J are CNPq research fellows. AS thanks CAPES for PDSE fellowship to perform part of his Ph.D. studies in UK (#88881.131626/2016-01). NF is supported by the Medical Research Council (Grant No: MR/T000171/1).
The authors declare that the research was conducted in the absence of any commercial or financial relationships that could be construed as a potential conflict of interest.
The reviewer CC declared a past co-authorship with one of the authors CA to the handling Editor.
RO, NF, and JB-N thank Diamond Light Source UK for the XChem facility time and the use of beamline I04-1 (Proposal LB16978). We are grateful to ChemAxon and OpenEye Inc. for providing academic license of their software for our projects. We are also grateful to DataCamp for providing academic license for our students perform training courses.
1. Gryseels B, Polman K, Clerinx J, Kestens L. Human Schistosomiasis. Lancet (2006) 368:1106–18. doi: 10.1016/S0140-6736(06)69440-3
2. WHO. Schistosomiasis. In: Schistosomiasis (2021). Available at: https://www.who.int/en/news-room/fact-sheets/detail/schistosomiasis (Accessed May 5, 2021).
3. Amoah AS, Hoekstra PT, Casacuberta-Partal M, Coffeng LE, Corstjens PLAM, Greco B, et al. Sensitive Diagnostic Tools and Targeted Drug Administration Strategies are Needed to Eliminate Schistosomiasis. Lancet Infect Dis (2020) 20:e165–72. doi: 10.1016/S1473-3099(20)30254-1
4. Hotez PJ, Alvarado M, Basáñez MG, Bolliger I, Bourne R, Boussinesq M, et al. The Global Burden of Disease Study 2010: Interpretation and Implications for the Neglected Tropical Diseases. PloS Negl Trop Dis (2014) 8:e2865. doi: 10.1371/journal.pntd.0002865
5. Kassebaum NJ, Arora M, Barber RM, Bhutta ZA, Brown J, Carter A, et al. Global, Regional, and National Disability-Adjusted Life-Years (DALYs) for 315 Diseases and Injuries and Healthy Life Expectancy (HALE), 1990–2015: A Systematic Analysis for the Global Burden of Disease Study 2015. Lancet (2016) 388:1603–58. doi: 10.1016/S0140-6736(16)31460-X
6. Colley DG, Bustinduy AL, Secor WE, King CH. Human Schistosomiasis. Lancet (2014) 383:2253–64. doi: 10.1016/S0140-6736(13)61949-2
7. Nation CS, Da’dara AA, Marchant JK, Skelly PJ. Schistosome Migration in the Definitive Host. PloS Negl Trop Dis (2020) 14:1–12. doi: 10.1371/journal.pntd.0007951
8. McManus DP, Dunne DW, Sacko M, Utzinger J, Vennervald BJ, Zhou X-N. Schistosomiasis. Nat Rev Dis Primers (2018) 4:13. doi: 10.1038/s41572-018-0013-8
9. Castillo MG, Humphries JE, Mourão MM, Marquez J, Gonzalez A, Montelongo CE. Biomphalaria Glabrata Immunity: Post-genome Advances. Dev Comp Immunol (2020) 104:103557. doi: 10.1016/j.dci.2019.103557
10. Eyayu T, Zeleke AJ, Worku L. Current Status and Future Prospects of Protein Vaccine Candidates Against Schistosoma Mansoni Infection. Parasite Epidemiol Control (2020) 11:e00176. doi: 10.1016/j.parepi.2020.e00176
11. Molehin AJ. Schistosomiasis Vaccine Development: Update on Human Clinical Trials. J BioMed Sci (2020) 27:1–7. doi: 10.1186/s12929-020-0621-y
12. Cioli D, Pica-Mattoccia L, Basso A, Guidi A. Schistosomiasis Control: Praziquantel Forever? Mol Biochem Parasitol (2014) 195:23–9. doi: 10.1016/j.molbiopara.2014.06.002
13. Mäder P, Rennar GA, Ventura AMP, Grevelding CG, Schlitzer M. Chemotherapy for Fighting Schistosomiasis: Past, Present and Future. ChemMedChem (2018) 13:2374–89. doi: 10.1002/cmdc.201800572
14. Gönnert R, Andrews P. Praziquantel, a New Broad-Spectrum Antischistosomal Agent. Z Parasitenkd (1977) 52:129–50. doi: 10.1007/BF00389899
15. Zwang J, Olliaro P. Efficacy and Safety of Praziquantel 40 mg/kg in Preschool-Aged and School-Aged Children: A Meta-Analysis. Parasit Vectors (2017) 10:47. doi: 10.1186/s13071-016-1958-7
16. Secor WE, Montgomery SP. Something Old, Something New: Is Praziquantel Enough for Schistosomiasis Control? Future Med Chem (2015) 7:681–4. doi: 10.4155/fmc.15.9
17. Caffrey CR. Chemotherapy of Schistosomiasis: Present and Future. Curr Opin Chem Biol (2007) 11:433–9. doi: 10.1016/j.cbpa.2007.05.031
18. Olliaro P, Delgado-Romero P, Keiser J. The Little We Know About the Pharmacokinetics and Pharmacodynamics of Praziquantel (Racemate and R-Enantiomer). J Antimicrob Chemother (2014) 69:863–70. doi: 10.1093/jac/dkt491
19. Meyer T, Sekljic H, Fuchs S, Bothe H, Schollmeyer D, Miculka C. Taste, A New Incentive to Switch to (R)-Praziquantel in Schistosomiasis Treatment. PloS Negl Trop Dis (2009) 3:e357. doi: 10.1371/journal.pntd.0000357
20. Wang W, Wang L, Liang Y-S. Susceptibility or Resistance of Praziquantel in Human Schistosomiasis: A Review. Parasitol Res (2012) 111:1871–7. doi: 10.1007/s00436-012-3151-z
21. Fallon PG, Doenhoff MJ. Drug-Resistant Schistosomiasis: Resistance to Praziquantel and Oxamniquine Induced in Schistosoma Mansoni in Mice is Drug Specific. Am J Trop Med Hyg (1994) 51:83–8. doi: 10.4269/ajtmh.1994.51.83
22. Ismail MM, Taha SA, Farghaly AM, El-Azony AS. Laboratory Induced Resistance to Praziquantel in Experimental Schistosomiasis. J Egypt Soc Parasitol (1994) 24:685–95.
23. Couto FF, Coelho PMZ, Araújo N, Kusel JR, Katz N, Jannotti-Passos LK, et al. Schistosoma Mansoni: A Method for Inducing Resistance to Praziquantel Using Infected Biomphalaria Glabrata Snails. Mem Inst Oswaldo Cruz (2011) 106:153–7. doi: 10.1590/S0074-02762011000200006
24. Fallon PG, Sturrock RF, Niang AC, Doenhoff MJ. Short Report: Diminished Susceptibility to Praziquantel in a Senegal Isolate of Schistosoma Mansoni. Am J Trop Med Hyg (1995) 53:61–2. doi: 10.4269/ajtmh.1995.53.61
25. Ismail M, Bennett JL, Tao L-F, Farghaly A, Bruce J, Metwally A. Characterization of Isolates of Schistosoma Mansoni From Egyptian Villagers That Tolerate High Doses of Praziquantel. Am J Trop Med Hyg (1996) 55:214–8. doi: 10.4269/ajtmh.1996.55.214
26. Crellen T, Walker M, Lamberton PHL, Kabatereine NB, Tukahebwa EM, Cotton JA, et al. Reduced Efficacy of Praziquantel Against Schistosoma Mansoni Is Associated With Multiple Rounds of Mass Drug Administration. Clin Inf Dis (2016) 63:1151–9. doi: 10.1093/cid/ciw506
27. Kabuyaya M, Chimbari MJ, Manyangadze T, Mukaratirwa S. Efficacy of Praziquantel on Schistosoma Haematobium and Re-Infection Rates Among School-Going Children in the Ndumo Area of uMkhanyakude District, KwaZulu-Natal, South Africa. Infect Dis Poverty (2017) 6:1–9. doi: 10.1186/s40249-017-0293-3
28. Melman SD, Steinauer ML, Cunningham C, Kubatko LS, Mwangi IN, Wynn NB, et al. Reduced Susceptibility to Praziquantel Among Naturally Occurring Kenyan Isolates of Schistosoma Mansoni. PloS Negl Trop Dis (2009) 3:e504. doi: 10.1371/journal.pntd.0000504
29. Ramirez B, Bickle Q, Yousif F, Fakorede F, Mouries M-A, Nwaka S. Schistosomes: Challenges in Compound Screening. Expert Opin Drug Discov (2007) 2:S53–61. doi: 10.1517/17460441.2.S1.S53
30. Katsuno K, Burrows JN, Duncan K, van Huijsduijnen RH, Kaneko T, Kita K, et al. Hit and Lead Criteria in Drug Discovery for Infectious Diseases of the Developing World. Nat Rev Drug Discov (2015) 14:751–8. doi: 10.1038/nrd4683
31. Caffrey CR, Secor WE. Schistosomiasis: From Drug Deployment to Drug Development. Curr Opin Infect Dis (2011) 24:410–7. doi: 10.1097/QCO.0b013e328349156f
32. Spangenberg T. Alternatives to Praziquantel for the Prevention and Control of Schistosomiasis. ACS Infect Dis (2020). doi: 10.1021/acsinfecdis.0c00542
33. Geary TG, Sakanari JA, Caffrey CR. Anthelmintic Drug Discovery: Into the Future. J Parasitol (2015) 101:125–33. doi: 10.1645/14-703.1
34. Lombardo FC, Pasche V, Panic G, Endriss Y, Keiser J. Life Cycle Maintenance and Drug-Sensitivity Assays for Early Drug Discovery in Schistosoma Mansoni. Nat Protoc (2019) 14:461–81. doi: 10.1038/s41596-018-0101-y
35. Tekwu EM, Anyan WK, Boamah D, Baffour-Awuah KO, Tekwu SK, Beng VP, et al. Mechanically Produced Schistosomula as a Higher-Throughput Tools for Phenotypic Pre-Screening in Drug Sensitivity Assays: Current Research and Future Trends. Biomark Res (2016) 4:21. doi: 10.1186/s40364-016-0075-2
36. Pica-Mattoccia L, Cioli D. Sex- and Stage-Related Sensitivity of Schistosoma Mansoni to In Vivo and In Vitro Praziquantel Treatment. Int J Parasitol (2004) 34:527–33. doi: 10.1016/j.ijpara.2003.12.003
37. Tavares NC, de Aguiar PHN, Gava SG, Oliveira G, Mourão MM. Schistosomiasis: Setting Routes for Drug Discovery. In: Special Topics in Drug Discovery. London, UK: InTech (2016). p. 116–24. doi: 10.5772/65386
38. Lalli C, Guidi A, Gennari N, Altamura S, Bresciani A, Ruberti G. Development and Validation of a Luminescence-based, Medium-Throughput Assay for Drug Screening in Schistosoma Mansoni. PloS Negl Trop Dis (2015) 9:e0003484. doi: 10.1371/journal.pntd.0003484
39. Panic G, Flores D, Ingram-Sieber K, Keiser J. Fluorescence/Luminescence-Based Markers for the Assessment of Schistosoma Mansoni Schistosomula Drug Assays. Parasit Vectors (2015) 8:624. doi: 10.1186/s13071-015-1233-3
40. Padalino G, Ferla S, Brancale A, Chalmers IW, Hoffmann KF. Combining Bioinformatics, Cheminformatics, Functional Genomics and Whole Organism Approaches for Identifying Epigenetic Drug Targets in Schistosoma Mansoni. Int J Parasitol Drugs Drug Resist (2018) 8:559–70. doi: 10.1016/j.ijpddr.2018.10.005
41. Keiser J. In Vitro and In Vivo Trematode Models for Chemotherapeutic Studies. Parasitology (2010) 137:589–603. doi: 10.1017/S0031182009991739
42. Mansour NR, Bickle QD. Comparison of Microscopy and Alamar Blue Reduction in a Larval Based Assay for Schistosome Drug Screening. PloS Negl Trop Dis (2010) 4:e795. doi: 10.1371/journal.pntd.0000795
43. Panic G, Vargas M, Scandale I, Keiser J. Activity Profile of an FDA-Approved Compound Library Against Schistosoma Mansoni. PloS Negl Trop Dis (2015) 9:e0003962. doi: 10.1371/journal.pntd.0003962
44. Maccesi M, Aguiar PHN, Pasche V, Padilla M, Suzuki BM, Montefusco S, et al. Multi-Center Screening of the Pathogen Box Collection for Schistosomiasis Drug Discovery. Parasit Vectors (2019) 12:1–10. doi: 10.1186/s13071-019-3747-6
45. Zheng W, Thorne N, McKew JC. Phenotypic Screens as a Renewed Approach for Drug Discovery. Drug Discov Today (2013) 18:1067–73. doi: 10.1016/j.drudis.2013.07.001
46. Ban TA. The Role of Serendipity in Drug Discovery. Dialogues Clin Neurosci (2006) 8:335–44. doi: 10.31887/dcns.2006.8.3/tban
47. Croston GE. The Utility of Target-Based Discovery. Expert Opin Drug Discov (2017) 12:427–9. doi: 10.1080/17460441.2017.1308351
48. Moffat JG, Vincent F, Lee JA, Eder J, Prunotto M. Opportunities and Challenges in Phenotypic Drug Discovery: An Industry Perspective. Nat Rev Drug Discov (2017) 16:531–43. doi: 10.1038/nrd.2017.111
49. Buskes MJ, Clements M, Bachovchin KA, Jalani HB, Leonard A, Bag S, et al. Structure–Bioactivity Relationships of Lapatinib Derived Analogs Against Schistosoma Mansoni. ACS Med Chem Lett (2020) 11:258–65. doi: 10.1021/acsmedchemlett.9b00455
50. Miraj CMAG, Fan WH, Song LJ, Yu CX, Feng B. Synthesis and SAR Studies of New Oxadiazole-2-Oxide Derivatives With Remarkable In Vitro Activity Against Schistosoma Japonicum. J Microb Biochem Technol (2017) 09:535–43. doi: 10.4172/1948-5948.1000339
51. Wu J, Wang C, Leas D, Vargas M, White KL, Shackleford DM, et al. Progress in Antischistosomal N,N′-diaryl Urea SAR. Bioorg Med Chem Lett (2018) 28:244–8. doi: 10.1016/j.bmcl.2017.12.064
52. Aulner N, Danckaert A, Ihm JE, Shum D, Shorte SL. Next-Generation Phenotypic Screening in Early Drug Discovery for Infectious Diseases. Trends Parasitol (2019) 35:559–70. doi: 10.1016/j.pt.2019.05.004
53. Swinney DC, Lee JA. Recent Advances in Phenotypic Drug Discovery. F1000Research (2020) 9:944. doi: 10.12688/f1000research.25813.1
54. Peak E, Chalmers IW, Hoffmann KF. Development and Validation of a Quantitative, High-Throughput, Fluorescent-Based Bioassay to Detect Schistosoma Viability. PloS Negl Trop Dis (2010) 4:e759. doi: 10.1371/journal.pntd.0000759
55. Abdulla M-H, Ruelas DS, Wolff B, Snedecor J, Lim K-C, Xu F, et al. Drug Discovery for Schistosomiasis: Hit and Lead Compounds Identified in a Library of Known Drugs by Medium-Throughput Phenotypic Screening. PloS Negl Trop Dis (2009) 3:e478. doi: 10.1371/journal.pntd.0000478
56. Braun L, Hazell L, Webb AJ, Allan F, Emery AM, Templeton MR. Determining the Viability of Schistosoma Mansoni Cercariae Using Fluorescence Assays: An Application for Water Treatment. PloS Negl Trop Dis (2020) 14:e0008176. doi: 10.1371/journal.pntd.0008176
57. Guidi A, Lalli C, Gimmelli R, Nizi E, Andreini M, Gennari N, et al. Discovery by Organism Based High-Throughput Screening of New Multi-Stage Compounds Affecting Schistosoma Mansoni Viability, Egg Formation and Production. PloS Negl Trop Dis (2017) 11:e0005994. doi: 10.1371/journal.pntd.0005994
58. Park SK, Gunaratne GS, Chulkov EG, Moehring F, McCusker P, Dosa PI, et al. The Anthelmintic Drug Praziquantel Activates a Schistosome Transient Receptor Potential Channel. J Biol Chem (2019) 294:18873–80. doi: 10.1074/jbc.AC119.011093
59. Aguiar PHN, Fernandes NMGS, Zani CL, Mourão MM. A High-Throughput Colorimetric Assay for Detection of Schistosoma Mansoni Viability Based on the Tetrazolium Salt XTT. Parasit Vectors (2017) 10:300. doi: 10.1186/s13071-017-2240-3
60. Marcellino C, Gut J, Lim KC, Singh R, McKerrow J, Sakanari J. WormAssay: A Novel Computer Application for Whole-Plate Motion-Based Screening of Macroscopic Parasites. PloS Negl Trop Dis (2012) 6:e1494. doi: 10.1371/journal.pntd.0001494
61. Chen S, Suzuki BM, Dohrmann J, Singh R, Arkin MR, Caffrey CR. A Multi-Dimensional, Time-Lapse, High Content Screening Platform Applied to Schistosomiasis Drug Discovery. Commun Biol (2020) 3:747. doi: 10.1038/s42003-020-01402-5
62. El-shehabi F, Taman A, Moali LS, El-sakkary N, Ribeiro P. A Novel G Protein-Coupled Receptor of Schistosoma Mansoni (SmGPR-3) Is Activated by Dopamine and Is Widely Expressed in the Nervous System. PloS Negl Trop Dis (2012) 6:e1523. doi: 10.1371/journal.pntd.0001523
63. Lee H, Moody-Davis A, Saha U, Suzuki BM, Asarnow D, Chen S, et al. Quantification and Clustering of Phenotypic Screening Data Using Time-Series Analysis for Chemotherapy of Schistosomiasis. BMC Genomics (2012) 13:1–24. doi: 10.1186/1471-2164-13-S1-S4
64. Manneck T, Braissant O, Haggenmuller Y, Keiser J. Isothermal Microcalorimetry to Study Drugs Against Schistosoma Mansoni. J Clin Microbiol (2011) 49:1217–25. doi: 10.1128/JCM.02382-10
65. Ravaynia PS, Lombardo FC, Biendl S, Dupuch MA, Keiser J, Hierlemann A, et al. Parallelized Impedance-Based Platform for Continuous Dose-Response Characterization of Antischistosomal Drugs. Adv Biosyst (2020) 4:1900304. doi: 10.1002/adbi.201900304
66. Patocka N, Sharma N, Rashid M, Ribeiro P. Serotonin Signaling in Schistosoma Mansoni: A Serotonin–Activated G Protein-Coupled Receptor Controls Parasite Movement. PloS Pathog (2014) 10:e1003878. doi: 10.1371/journal.ppat.1003878
67. McCusker P, Chan JD. Anti-Schistosomal Action of the Calcium Channel Agonist FPL-64176. Int J Parasitol Drugs Drug Resist (2019) 11:30–8. doi: 10.1016/j.ijpddr.2019.08.006
68. Asarnow D, Rojo-Arreola L, Suzuki BM, Caffrey CR, Singh R. The QDREC Web Server: Determining Dose-Response Characteristics of Complex Macroparasites in Phenotypic Drug Screens. Bioinformatics (2015) 31:1515–8. doi: 10.1093/bioinformatics/btu831
69. Neves BJ, Dantas RF, Senger MR, Valente WCG, Rezende-Neto J de M, Chaves WT, et al. The Antidepressant Drug Paroxetine as a New Lead Candidate in Schistosome Drug Discovery. Med Chem Commun (2016) 7:1176–82. doi: 10.1039/C5MD00596E
70. Paveley RA, Mansour NR, Hallyburton I, Bleicher LS, Benn AE, Mikic I, et al. Whole Organism High-Content Screening by Label-Free, Image-Based Bayesian Classification for Parasitic Diseases. PloS Negl Trop Dis (2012) 6:e1762. doi: 10.1371/journal.pntd.0001762
71. Rinaldi G, Loukas A, Brindley PJ, Irelan JT, Smout MJ. Viability of Developmental Stages of Schistosoma Mansoni Quantified With xCELLigence Worm Real-Time Motility Assay (xWORM). Int J Parasitol Drugs Drug Resist (2015) 5:141–8. doi: 10.1016/j.ijpddr.2015.07.002
72. McCusker P, Mian MY, Li G, Olp MD, Tiruveedhula VVNPB, Rashid F, et al. Non-Sedating Benzodiazepines Cause Paralysis and Tissue Damage in the Parasitic Blood Fluke Schistosoma Mansoni. PloS Negl Trop Dis (2019) 13:e0007826. doi: 10.1371/journal.pntd.0007826
73. Schneider CA, Rasband WS, Eliceiri KW. NIH Image to ImageJ: 25 Years of Image Analysis. Nat Methods (2012) 9:671–5. doi: 10.1038/nmeth.2089
74. El-Sakkary N, Chen S, Arkin MR, Caffrey CR, Ribeiro P. Octopamine Signaling in the Metazoan Pathogen Schistosoma Mansoni: Localization, Small-Molecule Screening and Opportunities for Drug Development. Dis Model Mech (2018) 11:dmm033563. doi: 10.1242/dmm.033563
75. Chan JD, Agbedanu PN, Grab T, Zamanian M, Dosa PI, Day TA, et al. Ergot Alkaloids (Re)Generate New Leads as Antiparasitics. PloS Negl Trop Dis (2015) 9:e0004063. doi: 10.1371/journal.pntd.0004063
76. Chan JD, Acharya S, Day TA, Marchant JS. Pharmacological Profiling an Abundantly Expressed Schistosome Serotonergic GPCR Identifies Nuciferine as a Potent Antagonist. Int J Parasitol Drugs Drug Resist (2016) 6:364–70. doi: 10.1016/j.ijpddr.2016.06.001
77. Duguet TB, Glebov A, Hussain A, Kulkarni S, Mochalkin I, Geary TG, et al. Identification of Annotated Bioactive Molecules That Impair Motility of the Blood Fluke Schistosoma Mansoni. Int J Parasitol Drugs Drug Resist (2020) 13:73–88. doi: 10.1016/j.ijpddr.2020.05.002
78. Marchant JS, Harding WW, Chan JD. Structure-Activity Profiling of Alkaloid Natural Product Pharmacophores Against a Schistosoma Serotonin Receptor. Int J Parasitol Drugs Drug Resist (2018) 8:550–8. doi: 10.1016/j.ijpddr.2018.09.001
79. Weeks JC, Roberts WM, Leasure C, Suzuki BM, Robinson KJ, Currey H, et al. Sertraline, Paroxetine, and Chlorpromazine are Rapidly Acting Anthelmintic Drugs Capable of Clinical Repurposing. Sci Rep (2018) 8:1–17. doi: 10.1038/s41598-017-18457-w
80. Monti L, Cornec A, Oukoloff K, Kovalevich J, Prijs K, Alle T, et al. Congeners Derived From Microtubule-Active Phenylpyrimidines Produce a Potent and Long-Lasting Paralysis of Schistosoma Mansoni In Vitro. ACS Infect Dis (2020). doi: 10.1021/acsinfecdis.0c00508
81. Long T, Rojo-Arreola L, Shi D, El-Sakkary N, Jarnagin K, Rock F, et al. Phenotypic, Chemical and Functional Characterization of Cyclic Nucleotide Phosphodiesterase 4 (PDE4) as a Potential Anthelmintic Drug Target. PloS Negl Trop Dis (2017) 11:e0005680. doi: 10.1371/journal.pntd.0005680
82. Bibo-Verdugo B, Wang SC, Almaliti J, Ta AP, Jiang Z, Wong DA, et al. The Proteasome as a Drug Target in the Metazoan Pathogen, Schistosoma Mansoni. ACS Infect Dis (2019) 5:1802–12. doi: 10.1021/acsinfecdis.9b00237
84. Padalino G, Chalmers IW, Brancale A, Hoffmann KF. Identification of 6-(piperazin-1-yl)-1,3,5-triazine as a Chemical Scaffold With Broad Anti-Schistosomal Activities. Wellcome Open Res (2020) 5:169. doi: 10.12688/wellcomeopenres.16069.1
85. Wang J, Paz C, Padalino G, Coghlan A, Lu Z, Gradinaru I, et al. Large-Scale RNAi Screening Uncovers Therapeutic Targets in the Parasite Schistosoma Mansoni. Science (2020) 369:1649–53. doi: 10.1126/science.abb7699
86. Padalino G, Celatka CA, Kalin JH, Cole PA, Lassalle D, Chalmers IW, et al. Schistosoma Mansoni Lysine Specific Demethylase 1 (SmLSD1 ) is a Druggable Target Involved in Parasite Survival , Oviposition and Stem Cell Proliferation. (2020). doi: 10.1101/2020.09.17.301184
87. Singh R, Pittas M, Heskia I, Xu F, McKerrow J, Caffrey CR. Automated Image-Based Phenotypic Screening for High-Throughput Drug Discovery. In: 2009 22nd IEEE International Symposium on Computer-Based Medical Systems. New Jersey, USA: IEEE (2009). p. 1–8. doi: 10.1109/CBMS.2009.5255338
88. Asarnow DE, Singh R. Segmenting the Etiological Agent of Schistosomiasis for High-Content Screening. IEEE Trans Med Imaging (2013) 32:1007–18. doi: 10.1109/TMI.2013.2247412
89. Singh R, Beasley R, Long T, Caffrey CR. Algorithmic Mapping and Characterization of the Drug-Induced Phenotypic-Response Space of Parasites Causing Schistosomiasis. IEEE/ACM Trans Comput Biol Bioinform (2018) 15:469–81. doi: 10.1109/TCBB.2016.2550444
90. Asarnow D, Singh R. Determining Dose-Response Characteristics of Molecular Perturbations in Whole-Organism Assays Using Biological Imaging and Machine Learning. In: 2018 IEEE International Conference on Bioinformatics and Biomedicine (BIBM). New Jersey, USA: IEEE (2018). p. 283–90. doi: 10.1109/BIBM.2018.8621083
91. Rojo-Arreola L, Long T, Asarnow D, Suzuki BM, Singh R, Caffrey CR. Chemical and Genetic Validation of the Statin Drug Target to Treat the Helminth Disease, Schistosomiasis. PloS One (2014) 9:e87594. doi: 10.1371/journal.pone.0087594
92. Long T, Neitz RJ, Beasley R, Kalyanaraman C, Suzuki BM, Jacobson MP, et al. Structure-Bioactivity Relationship for Benzimidazole Thiophene Inhibitors of Polo-Like Kinase 1 (PLK1), a Potential Drug Target in Schistosoma Mansoni. PloS Negl Trop Dis (2016) 10:e0004356. doi: 10.1371/journal.pntd.0004356
93. Melo-Filho CC, Dantas RF, Braga RC, Neves BJ, Senger MR, Valente WCG, et al. QSAR-Driven Discovery of Novel Chemical Scaffolds Active Against Schistosoma Mansoni. J Chem Inf Model (2016) 56:1357–72. doi: 10.1021/acs.jcim.6b00055
94. Neves BJ, Dantas RF, Senger MR, Melo-Filho CC, Valente WCG, de Almeida ACM, et al. Discovery of New Anti-Schistosomal Hits by Integration of QSAR-Based Virtual Screening and High Content Screening. J Med Chem (2016) 59:7075–88. doi: 10.1021/acs.jmedchem.5b02038
95. Mansour NR, Paveley R, Gardner JMF, Bell AS, Parkinson T, Bickle Q. High Throughput Screening Identifies Novel Lead Compounds With Activity Against Larval, Juvenile and Adult Schistosoma Mansoni. PloS Negl Trop Dis (2016) 10:e0004659. doi: 10.1371/journal.pntd.0004659
96. Giuliani S, Silva AC, Borba JVVB, Ramos PIP, Paveley RA, Muratov EN, et al. Computationally-Guided Drug Repurposing Enables the Discovery of Kinase Targets and Inhibitors as New Schistosomicidal Agents. PloS Comput Biol (2018) 14:e1006515. doi: 10.1371/journal.pcbi.1006515
97. Crusco A, Whiteland H, Baptista R, Forde-Thomas JE, Beckmann M, Mur LAJ, et al. Antischistosomal Properties of Sclareol and Its Heck-Coupled Derivatives: Design, Synthesis, Biological Evaluation, and Untargeted Metabolomics. ACS Infect Dis (2019) 5:1188–99. doi: 10.1021/acsinfecdis.9b00034
98. Whatley KCL, Padalino G, Whiteland H, Geyer KK, Hulme BJ, Chalmers IW, et al. The Repositioning of Epigenetic Probes/Inhibitors Identifies New Anti-Schistosomal Lead Compounds and Chemotherapeutic Targets. PloS Negl Trop Dis (2019) 13:e0007693. doi: 10.1371/journal.pntd.0007693
99. Crusco A, Bordoni C, Chakroborty A, Whatley KCL, Whiteland H, Westwell AD, et al. Design, Synthesis and Anthelmintic Activity of 7-Keto-Sempervirol Analogues. Eur J Med Chem (2018) 152:87–100. doi: 10.1016/j.ejmech.2018.04.032
100. Whiteland HL, Chakroborty A, Forde-Thomas JE, Crusco A, Cookson A, Hollinshead J, et al. An Abies Procera-Derived Tetracyclic Triterpene Containing a Steroid-Like Nucleus Core and a Lactone Side Chain Attenuates In Vitro Survival of Both Fasciola Hepatica and Schistosoma Mansoni. Int J Parasitol Drugs Drug Resist (2018) 8:465–74. doi: 10.1016/j.ijpddr.2018.10.009
101. Lamprecht MR, Sabatini DM, Carpenter AE. CellProfilerTM: Free, Versatile Software for Automated Biological Image Analysis. Biotechniques (2007) 42:71–5. doi: 10.2144/000112257
102. Smout MJ, Kotze AC, McCarthy JS, Loukas A. A Novel High Throughput Assay for Anthelmintic Drug Screening and Resistance Diagnosis by Real-Time Monitoring of Parasite Motility. PloS Negl Trop Dis (2010) 4:e885. doi: 10.1371/journal.pntd.0000885
103. Chawla K, Modena MM, Ravaynia PS, Lombardo FC, Leonhardt M, Panic G, et al. Impedance-Based Microfluidic Assay for Automated Antischistosomal Drug Screening. ACS Sens (2018) 3:2613–20. doi: 10.1021/acssensors.8b01027
104. Modena MM, Chawla K, Misun PM, Hierlemann A. Smart Cell Culture Systems: Integration of Sensors and Actuators Into Microphysiological Systems. ACS Chem Biol (2018) 13:1767–84. doi: 10.1021/acschembio.7b01029
105. Wangchuk P, Pearson MS, Giacomin PR, Becker L, Sotillo J, Pickering D, et al. Compounds Derived From the Bhutanese Daisy, Ajania Nubigena, Demonstrate Dual Anthelmintic Activity Against Schistosoma Mansoni and Trichuris Muris. PloS Negl Trop Dis (2016) 10:e0004908. doi: 10.1371/journal.pntd.0004908
106. Wangchuk P, Giacomin PR, Pearson MS, Smout MJ, Loukas A. Identification of Lead Chemotherapeutic Agents From Medicinal Plants Against Blood Flukes and Whipworms. Sci Rep (2016) 6:32101. doi: 10.1038/srep32101
107. Yan H-B, Smout MJ, Ju C, Folley AE, Skinner DE, Mann VH, et al. Developmental Sensitivity in Schistosoma Mansoni to Puromycin to Establish Drug Selection of Transgenic Schistosomes. Antimicrob Agents Chemother (2018) 62:e02568–17. doi: 10.1128/AAC.02568-17
108. Zeraik AE, Galkin VE, Rinaldi G, Garratt RC, Smout MJ, Loukas A, et al. Reversible Paralysis of Schistosoma Mansoni by Forchlorfenuron, a Phenylurea Cytokinin That Affects Septins. Int J Parasitol (2014) 44:523–31. doi: 10.1016/j.ijpara.2014.03.010
109. Sundaraneedi MK, Tedla BA, Eichenberger RM, Becker L, Pickering D, Smout MJ, et al. Polypyridylruthenium(II) Complexes Exert Anti-Schistosome Activity and Inhibit Parasite Acetylcholinesterases. PloS Negl Trop Dis (2017) 11:e0006134. doi: 10.1371/journal.pntd.0006134
110. Modena MM, Chawla K, Lombardo F, Burgel SC, Panic G, Keiser J, et al. Impedance-Based Detection of Schistosoma Mansoni Larvae Viability for Drug Screening. In: 2017 IEEE Biomedical Circuits and Systems Conference (BioCAS). New Jersey, USA: IEEE (2017). p. 1–4. doi: 10.1109/BIOCAS.2017.8325227
111. Braissant O, Keiser J, Meister I, Bachmann A, Wirz D, Göpfert B, et al. Isothermal Microcalorimetry Accurately Detects Bacteria, Tumorous Microtissues, and Parasitic Worms in a Label-Free Well-Plate Assay. Biotechnol J (2015) 10:460–8. doi: 10.1002/biot.201400494
112. Braissant O, Bachmann A, Bonkat G. Microcalorimetric Assays for Measuring Cell Growth and Metabolic Activity: Methodology and Applications. Methods (2015) 76:27–34. doi: 10.1016/j.ymeth.2014.10.009
113. Braissant O, Wirz D, Göpfert B, Daniels AU. Biomedical Use of Isothermal Microcalorimeters. Sensors (2010) 10:9369–83. doi: 10.3390/s101009369
114. Manneck T, Keiser J, Müller J. Mefloquine Interferes With Glycolysis in Schistosomula of Schistosoma Mansoni Via Inhibition of Enolase. Parasitology (2012) 139:497–505. doi: 10.1017/S0031182011002204
115. Manneck T, Braissant O, Ellis W, Keiser J. Schistosoma Mansoni: Antischistosomal Activity of the Four Optical Isomers and the Two Racemates of Mefloquine on Schistosomula and Adult Worms In Vitro and In Vivo. Exp Parasitol (2011) 127:260–9. doi: 10.1016/j.exppara.2010.08.011
116. Meister I, Ingram-Sieber K, Cowan N, Todd M, Robertson MN, Meli C, et al. Activity of Praziquantel Enantiomers and Main Metabolites Against Schistosoma Mansoni. Antimicrob Agents Chemother (2014) 58:5466–72. doi: 10.1128/AAC.02741-14
117. Ingram K, Ellis W, Keiser J. Antischistosomal Activities of Mefloquine-Related Arylmethanols. Antimicrob Agents Chemother (2012) 56:3207–15. doi: 10.1128/AAC.06177-11
118. Ingram K, Schiaffo CE, Sittiwong W, Benner E, Dussault PH, Keiser J. In Vitro and In Vivo Activity of 3-alkoxy-1,2-dioxolanes Against Schistosoma Mansoni. J Antimicrob Chemother (2012) 67:1979–86. doi: 10.1093/jac/dks141
119. Al-Ali H. The Evolution of Drug Discovery: From Phenotypes to Targets, and Back. Medchemcomm (2016) 7:788–98. doi: 10.1039/c6md00129g
120. Heilker R, Lessel U, Bischoff D. The Power of Combining Phenotypic and Target-Focused Drug Discovery. Drug Discov Today (2019) 24:526–32. doi: 10.1016/j.drudis.2018.10.009
121. Lage OM, Ramos MC, Calisto R, Almeida E, Vasconcelos V, Vicente F. Current Screening Methodologies in Drug Discovery for Selected Human Diseases. Mar Drugs (2018) 16:1–31. doi: 10.3390/md16080279
122. Noël F, do Monte FM. Validation of a Na+-shift Binding Assay for Estimation of the Intrinsic Efficacy of Ligands at the A2A Adenosine Receptor. J Pharmacol Toxicol Methods (2017) 84:51–6. doi: 10.1016/j.vascn.2016.10.009
123. Noël F, Pompeu TET, Moura BC. Functional Binding Assays for Estimation of the Intrinsic Efficacy of Ligands at the 5-HT1A Receptor: Application for Screening Drug Candidates. J Pharmacol Toxicol Methods (2014) 70:12–8. doi: 10.1016/j.vascn.2014.03.002
124. De Jong LAA, Uges DRA, Franke JP, Bischoff R. Receptor-Ligand Binding Assays: Technologies and Applications. J Chromatogr B Anal Technol BioMed Life Sci (2005) 829:1–25. doi: 10.1016/j.jchromb.2005.10.002
125. Ashok Hajare A, Sachin Salunkhe S, Sachin MS, Sonali Gorde S, Sammer Nadaf J, Sachi Pishawikar A. Review on : High-throughput Screening is an Approach to Drug Discovery. Am J Pharmtech Res (2014) 4:113–29.
126. Rohman M, Wingfield J. High-Throughput Screening Using Mass Spectrometry Within Drug Discovery. In: Methods in Molecular Biology. New York, NY, USA: Humana Press Inc (2016). p. 47–63. doi: 10.1007/978-1-4939-3673-1_3
127. Henderson MJ, Holbert MA, Simeonov A, Kallal LA. High-Throughput Cellular Thermal Shift Assays in Research and Drug Discovery. SLAS Discov (2020) 25:137–47. doi: 10.1177/2472555219877183
128. De Simone A, Naldi M, Bartolini M, Davani L, Andrisano V. Immobilized Enzyme Reactors: An Overview of Applications in Drug Discovery From 2008 to 2018. Chromatographia (2019) 82:425–41. doi: 10.1007/s10337-018-3663-5
129. Moraes MC, Cardoso C, Seidl C, Moaddel R, Cass Q. Targeting Anti-Cancer Active Compounds: Affinity-Based Chromatographic Assays. Curr Pharm Des (2016) 22:5976–87. doi: 10.2174/1381612822666160614080506
130. Imaduwage KP, Lakbub J, Go EP, Desaire H. Rapid LC-MS Based High-Throughput Screening Method, Affording No False Positives or False Negatives, Identifies a New Inhibitor for Carbonic Anhydrase. Sci Rep (2017) 7:1–10. doi: 10.1038/s41598-017-08602-w
131. Wang L, Zhao Y, Zhang Y, Zhang T, Kool J, Somsen GW, et al. Online Screening of Acetylcholinesterase Inhibitors in Natural Products Using Monolith-Based Immobilized Capillary Enzyme Reactors Combined With Liquid Chromatography-Mass Spectrometry. J Chromatogr A (2018) 1563:135–43. doi: 10.1016/j.chroma.2018.05.069
132. Ferreira Lopes Vilela A, Cardoso CL. An on-Flow Assay for Screening of β-Secretase Ligands by Immobilised Capillary Reactor-Mass Spectrometry. Anal Methods (2017) 9:2189–96. doi: 10.1039/c7ay00284j
133. Rodrigues MVN, Rodrigues-Silva C, Boaventura S, Oliveira ASS, Rath S, Cass QB. On-Flow LC-MS/MS Method for Screening of Xanthine Oxidase Inhibitors. J Pharm BioMed Anal (2020) 181:113097. doi: 10.1016/j.jpba.2020.113097
134. Zhuo T, Zhou S, Zhang W, Lambertucci C, Volpini R. Synthesis and Ability of New Ligands for G Protein-Coupled Receptors 17 (GPR17). Med Sci Monit (2017) 23:953–9. doi: 10.12659/MSM.902048
135. Kalinin DV, Jana SK, Pfafenrot M, Chakrabarti A, Melesina J, Shaik TB, et al. Structure-Based Design, Synthesis, and Biological Evaluation of Triazole-Based smHDAC8 Inhibitors. ChemMedChem (2020) 15:571–84. doi: 10.1002/cmdc.201900583
136. Monaldi D, Rotili D, Lancelot J, Marek M, Wössner N, Lucidi A, et al. Structure-Reactivity Relationships on Substrates and Inhibitors of the Lysine Deacylase Sirtuin 2 From Schistosoma Mansoni (SmSirt2). J Med Chem (2019) 62:8733–59. doi: 10.1021/acs.jmedchem.9b00638
137. Li T, Ziniel PD, He P, Kommer VP, Crowther GJ, He M, et al. High-Throughput Screening Against Thioredoxin Glutathione Reductase Identifies Novel Inhibitors With Potential Therapeutic Value for Schistosomiasis. Infect Dis Poverty (2015) 4:40. doi: 10.1186/s40249-015-0071-z
138. Lyu H, Petukhov PA, Banta PR, Jadhav A, Lea WA, Cheng Q, et al. Characterization of Lead Compounds Targeting the Selenoprotein Thioredoxin Glutathione Reductase for Treatment of Schistosomiasis. ACS Infect Dis (2020) 6:393–405. doi: 10.1021/acsinfecdis.9b00354
139. Liu J, Dyer D, Wang J, Wang S, Du X, Xu B, et al. 3-oxoacyl-ACP Reductase From Schistosoma Japonicum: Integrated In Silico-In Vitro Strategy for Discovering Antischistosomal Lead Compounds. PloS One (2013) 8:e64984. doi: 10.1371/journal.pone.0064984
140. Botros SS, William S, Sabra ANA, El-Lakkany NM, Seif el-Din SH, García-Rubia A, et al. Screening of a PDE-focused Library Identifies Imidazoles With In Vitro and In Vivo Antischistosomal Activity. Int J Parasitol Drugs Drug Resist (2019) 9:35–43. doi: 10.1016/j.ijpddr.2019.01.001
141. Sebastián-Pérez V, Schroeder S, Munday JC, Van Der Meer T, Zaldívar-Díez J, Siderius M, et al. Discovery of Novel Schistosoma Mansoni PDE4A Inhibitors as Potential Agents Against Schistosomiasis. Future Med Chem (2019) 11:1703–20. doi: 10.4155/fmc-2018-0592
142. Jurberg AD, Brindley PJ. Gene Function in Schistosomes: Recent Advances Towards a Cure. Front Genet (2015) 6:1–4. doi: 10.3389/fgene.2015.00144
143. Huang W, Gu M, Cheng W, Zhao QP, Ming Z, Dong H. Characteristics and Function of Cathepsin L3 From Schistosoma Japonicum. Parasitol Res (2020) 119:1619–28. doi: 10.1007/s00436-020-06647-x
144. Caffrey CR, Placha L, Barinka C, Hradilek M, Dostál J, Sajid M, et al. Homology Modeling and SAR Analysis of Schistosoma Japonicum Cathepsin D (SjCD) With Statin Inhibitors Identify a Unique Active Site Steric Barrier With Potential for the Design of Specific Inhibitors. Biol Chem (2005) 386:339–49. doi: 10.1515/BC.2005.041
145. Ekici ÖD, Götz MG, James KE, Li ZZ, Rukamp BJ, Asgian JL, et al. Aza-Peptide Michael Acceptors: A New Class of Inhibitors Specific for Caspases and Other Clan CD Cysteine Proteases. J Med Chem (2004) 47:1889–92. doi: 10.1021/jm049938j
146. Ovat A, Muindi F, Fagan C, Brouner M, Hansell E, Dvořák J, et al. Aza-Peptidyl Michael Acceptor and Epoxide Inhibitors—Potent and Selective Inhibitors of Schistosoma Mansoni and Ixodes Ricinus Legumains (Asparaginyl Endopeptidases). J Med Chem (2009) 52:7192–210. doi: 10.1021/jm900849h
147. Götz MG, James KE, Hansell E, Dvořák J, Seshaadri A, Sojka D, et al. Aza-Peptidyl Michael Acceptors. A New Class of Potent and Selective Inhibitors of Asparaginyl Endopeptidases (Legumains) From Evolutionarily Diverse Pathogens. J Med Chem (2008) 51:2816–32. doi: 10.1021/jm701311r
148. Fonseca NC, da Cruz LF, da Silva Villela F, do Nascimento Pereira GA, de Siqueira-Neto JL, Kellar D, et al. Synthesis of a Sugar-Based Thiosemicarbazone Series and Structure-Activity Relationship Versus the Parasite Cysteine Proteases Rhodesain, Cruzain, and Schistosoma Mansoni Cathepsin B1. Antimicrob Agents Chemother (2015) 59:2666–77. doi: 10.1128/AAC.04601-14
149. Jílková A, Horn M, Fanfrlík J, Küppers J, Pachl P, Řezáčová P, et al. Azanitrile Inhibitors of the SmCB1 Protease Target are Lethal to Schistosoma Mansoni : Structural and Mechanistic Insights Into Chemotype Reactivity. ACS Infect Dis (2021) 7:189–201. doi: 10.1021/acsinfecdis.0c00644
150. Jílková A, Rubešová P, Fanfrlík J, Fajtová P, Řezáčová P, Brynda J, et al. Druggable Hot Spots in the Schistosomiasis Cathepsin B1 Target Identified by Functional and Binding Mode Analysis of Potent Vinyl Sulfone Inhibitors. ACS Infect Dis (2020). doi: 10.1021/acsinfecdis.0c00501
151. Horn M, Jílková A, Vondrášek J, Marešová L, Caffrey CR, Mareš M. Mapping the Pro-Peptide of the Schistosoma Mansoni Cathepsin B1 Drug Target: Modulation of Inhibition by Heparin and Design of Mimetic Inhibitors. ACS Chem Biol (2011) 6:609–17. doi: 10.1021/cb100411v
152. Jílková A, Řezáčová P, Lepšík M, Horn M, Váchová J, Fanfrlík J, et al. Structural Basis for Inhibition of Cathepsin B Drug Target From the Human Blood Fluke, Schistosoma Mansoni. J Biol Chem (2011) 286:35770–81. doi: 10.1074/jbc.M111.271304
153. Araujo-Montoya BO, Senger MR, Gomes BF, Harris G, Owens RJ, Silva-Jr FP. Schistosoma Mansoni Cathepsin D1: Biochemical and Biophysical Characterization of the Recombinant Enzyme Expressed in HEK293T Cells. Protein Expr Purif (2020) 167:105532. doi: 10.1016/j.pep.2019.105532
154. Brady CP, Dowd AJ, Brindley PJ, Ryan T, Day SR, Dalton JP. Recombinant Expression and Localization ofSchistosoma Mansoni Cathepsin L1 Support its Role in the Degradation of Host Hemoglobin. Infect Immun (1999) 67:368–74. doi: 10.1128/IAI.67.1.368-374.1999
155. Dvořák J, Mashiyama ST, Sajid M, Braschi S, Delcroix M, Schneider EL, et al. SmCL3, a Gastrodermal Cysteine Protease of the Human Blood Fluke Schistosoma Mansoni. PloS Negl Trop Dis (2009) 3:e449. doi: 10.1371/journal.pntd.0000449
156. Bezerra IC, Jorge MSB, Gondim APS, De LLL, Vasconcelos MGF. “Fui Lá No Posto E O Doutor Me Mandou Foi Pra Cá”: Processo De Medicamentalização E (Des)Caminhos Para O Cuidado Em Saúde Mental Na Atenção Primária. Interface - Comun Saúde Educ (2014) 18:61–74. doi: 10.1590/1807-57622013.0650
157. Berriman M, Haas BJ, LoVerde PT, Wilson RA, Dillon GP, Cerqueira GC, et al. The Genome of the Blood Fluke Schistosoma Mansoni. Nature (2009) 460:352–8. doi: 10.1038/nature08160
158. Grevelding CG, Langner S, Dissous C. Kinases: Molecular Stage Directors for Schistosome Development and Differentiation. Trends Parasitol (2018) 34:246–60. doi: 10.1016/j.pt.2017.12.001
159. Fioravanti R, Mautone N, Rovere A, Rotili D, Mai A. Targeting Histone Acetylation/Deacetylation in Parasites: An Update (2017–2020). Curr Opin Chem Biol (2020) 57:65–74. doi: 10.1016/j.cbpa.2020.05.008
160. Perera TPS, Jovcheva E, Mevellec L, Vialard J, De Lange D, Verhulst T, et al. Discovery and Pharmacological Characterization of JNJ-42756493 (Erdafitinib), a Functionally Selective Small-Molecule FGFR Family Inhibitor. Mol Cancer Ther (2017) 16:1010–20. doi: 10.1158/1535-7163.MCT-16-0589
161. Morales ME, Rinaldi G, Gobert GN, Kines KJ, Tort JF, Brindley PJ. RNA Interference of Schistosoma Mansoni Cathepsin D, the Apical Enzyme of the Hemoglobin Proteolysis Cascade. Mol Biochem Parasitol (2008) 157:160–8. doi: 10.1016/j.molbiopara.2007.10.009
162. Jílková A, Horn M, Mareš M. Structural and Functional Characterization of Schistosoma Mansoni Cathepsin B1. In: 1 ed. Schistosoma Mansoni: Methods and Protocols. New York, NY, USA: Springer US (2020). p. 145–58. doi: 10.1007/978-1-0716-0635-3_12
163. Fanfrlík J, Brahmkshatriya PS, Řezáč J, Jílková A, Horn M, Mareš M, et al. Quantum Mechanics-Based Scoring Rationalizes the Irreversible Inactivation of Parasitic Schistosoma Mansoni Cysteine Peptidase by Vinyl Sulfone Inhibitors. J Phys Chem B (2013) 117:14973–82. doi: 10.1021/jp409604n
164. Buro C, Oliveira KC, Lu Z, Leutner S, Beckmann S, Dissous C, et al. Transcriptome Analyses of Inhibitor-Treated Schistosome Females Provide Evidence for Cooperating Src-kinase and TGFβ Receptor Pathways Controlling Mitosis and Eggshell Formation. PloS Pathog (2013) 9:e1003448. doi: 10.1371/journal.ppat.1003448
165. Hahnel S, Quack T, Parker-Manuel SJ, Lu Z, Vanderstraete M, Morel M, et al. Gonad RNA-specific qRT-PCR Analyses Identify Genes With Potential Functions in Schistosome Reproduction Such as SmFz1 and SmFGFRs. Front Genet (2014) 5:170. doi: 10.3389/fgene.2014.00170
166. You H, Stephenson RJ, Gobert GN, McManus DP. Revisiting Glucose Uptake and Metabolism in Schistosomes: New Molecular Insights for Improved Schistosomiasis Therapies. Front Genet (2014) 5:176. doi: 10.3389/fgene.2014.00176
167. Chan JD, McCorvy JD, Acharya S, Johns ME, Day TA, Roth BL, et al. A Miniaturized Screen of a Schistosoma Mansoni Serotonergic G Protein-Coupled Receptor Identifies Novel Classes of Parasite-Selective Inhibitors. PloS Pathog (2016) 12:1–26. doi: 10.1371/journal.ppat.1005651
168. Shuker SB, Hajduk PJ, Meadows RP, Fesik SW. Discovering High-Affinity Ligands for Proteins: SAR by NMR. Science (1996) 274:1531–4. doi: 10.1126/science.274.5292.1531
169. Rees DC, Congreve M, Murray CW, Carr R. Fragment-Based Lead Discovery. Nat Rev Drug Discov (2004) 3:660–72. doi: 10.1038/nrd1467
170. Schulz MN, Hubbard RE. Recent Progress in Fragment-Based Lead Discovery. Curr Opin Pharmacol (2009) 9:615–21. doi: 10.1016/j.coph.2009.04.009
171. Erlanson DA, Fesik SW, Hubbard RE, Jahnke W, Jhoti H. Twenty Years on: The Impact of Fragments on Drug Discovery. Nat Rev Drug Discov (2016) 15:605–19. doi: 10.1038/nrd.2016.109
172. Erlanson DA, De Esch IJP, Jahnke W, Johnson CN, Mortenson PN. Fragment-to-Lead Medicinal Chemistry Publications in 2018. J Med Chem (2020) 63:4430–44. doi: 10.1021/acs.jmedchem.9b01581
173. Osborne J, Panova S, Rapti M, Urushima T, Jhoti H. Fragments: Where are We Now? Biochem Soc Trans (2020) 48:271–80. doi: 10.1042/BST20190694
174. Bollag G, Tsai J, Zhang J, Zhang C, Ibrahim P, Nolop K, et al. Vemurafenib: The First Drug Approved for BRAF-mutant Cancer. Nat Rev Drug Discov (2012) 11:873–86. doi: 10.1038/nrd3847
175. Souers AJ, Leverson JD, Boghaert ER, Ackler SL, Catron ND, Chen J, et al. ABT-199, a Potent and Selective BCL-2 Inhibitor, Achieves Antitumor Activity While Sparing Platelets. Nat Med (2013) 19:202–8. doi: 10.1038/nm.3048
176. Tap WD, Wainberg ZA, Anthony SP, Ibrahim PN, Zhang C, Healey JH, et al. Structure-Guided Blockade of CSF1R Kinase in Tenosynovial Giant-Cell Tumor. N Engl J Med (2015) 373:428–37. doi: 10.1056/nejmoa1411366
177. Bancet A, Raingeval C, Lomberget T, Le Borgne M, Guichou J-F, Krimm I. Fragment Linking Strategies for Structure-Based Drug Design. J Med Chem (2020) 63:11420–35. doi: 10.1021/acs.jmedchem.0c00242
178. Congreve M, Carr R, Murray C, Jhoti H. A ‘Rule of Three’ for Fragment-Based Lead Discovery? Drug Discov Today (2003) 8:876–7. doi: 10.1016/S1359-6446(03)02831-9
179. Giordanetto F, Jin C, Willmore L, Feher M, Shaw DE. Fragment Hits: What do They Look Like and How do They Bind? J Med Chem (2019) 62:3381–94. doi: 10.1021/acs.jmedchem.8b01855
180. Hall RJ, Mortenson PN, Murray CW. Efficient Exploration of Chemical Space by Fragment-Based Screening. Prog Biophys Mol Biol (2014) 116:82–91. doi: 10.1016/j.pbiomolbio.2014.09.007
181. Hann MM, Leach AR, Harper G. Molecular Complexity and Its Impact on the Probability of Finding Leads for Drug Discovery. J Chem Inf Comput Sci (2001) 41:856–64. doi: 10.1021/ci000403i
182. Ferenczy GG, Keserű GM. On the Enthalpic Preference of Fragment Binding. Medchemcomm (2016) 7:332–7. doi: 10.1039/C5MD00542F
183. Hopkins AL, Keserü GM, Leeson PD, Rees DC, Reynolds CH. The Role of Ligand Efficiency Metrics in Drug Discovery. Nat Rev Drug Discov (2014) 13:105–21. doi: 10.1038/nrd4163
184. Davis BJ, Roughley SD. Fragment-Based Lead Discovery. 1st ed. Annual Reports in Medicinal Chemistry. Cambridge, MA, USA: Elsevier Inc (2017). doi: 10.1016/bs.armc.2017.07.002
185. Bulfer SL, Jean-Francois FL, Arkin MR. Making FBDD Work in Academia. In: Erlanson DA, Jahnke W, editors. Fragment-Based Drug Discovery: Lessons and Outlook. Weinheim, Germany: Wiley-VCH Verlag GmbH & Co. KGaA (2016). p. 223–46. doi: 10.1002/9783527683604.ch10
186. Mello JDFRE, Gomes RA, Vital-Fujii DG, Ferreira GM, Trossini GHG. Fragment-Based Drug Discovery as Alternative Strategy to the Drug Development for Neglected Diseases. Chem Biol Drug Des (2017) 90:1067–78. doi: 10.1111/cbdd.13030
187. Keeley A, Petri L, Ábrányi-Balogh P, Keserű GM. Covalent Fragment Libraries in Drug Discovery. Drug Discov Today (2020) 25:983–96. doi: 10.1016/j.drudis.2020.03.016
188. Keseru GM, Erlanson DA, Ferenczy GG, Hann MM, Murray CW, Pickett SD. Design Principles for Fragment Libraries: Maximizing the Value of Learnings From Pharma Fragment-Based Drug Discovery (FBDD) Programs for Use in Academia. J Med Chem (2016) 59:8189–206. doi: 10.1021/acs.jmedchem.6b00197
189. Troelsen NS, Clausen MH. Library Design Strategies To Accelerate Fragment-Based Drug Discovery. Chem - A Eur J (2020) 26:11391–403. doi: 10.1002/chem.202000584
190. Fragment Screening - Xchem. Available at: https://www.diamond.ac.uk/Instruments/Mx/Fragment-Screening.html (Accessed December 11, 2020).
191. Cox OB, Krojer T, Collins P, Monteiro O, Talon R, Bradley A, et al. A Poised Fragment Library Enables Rapid Synthetic Expansion Yielding the First Reported Inhibitors of PHIP(2), an Atypical Bromodomain. Chem Sci (2016) 7:2322–30. doi: 10.1039/c5sc03115j
192. Roughley SD, Jordan AM. The Medicinal Chemist ‘ s Toolbox : An Analysis of Reactions Used in the Pursuit of Novel Drug Candidates. J Med Chem (2011) 54:3451–79. doi: 10.1021/jm200187y
193. Douangamath A, Fearon D, Gehrtz P, Krojer T, Lukacik P, Owen CD, et al. Crystallographic and Electrophilic Fragment Screening of the SARS-CoV-2 Main Protease. Nat Commun (2020) 11:1–11. doi: 10.1038/s41467-020-18709-w
194. Erlanson DA. Many Small Steps Towards a COVID-19 Drug. Nat Commun (2020) 11:1–4. doi: 10.1038/s41467-020-18710-3
195. Coyle J, Walser R. Applied Biophysical Methods in Fragment-Based Drug Discovery. SLAS Discov (2020) 25:471–90. doi: 10.1177/2472555220916168
196. Begley DW, Moen SO, Pierce PG, Zartler ER. Saturation Transfer Difference NMR for Fragment Screening. In: . Current Protocols in Chemical Biology. Hoboken, NJ, USA: John Wiley & Sons, Inc (2013). p. 251–68. doi: 10.1002/9780470559277.ch130118
197. Becker W, Bhattiprolu KC, Gubensäk N, Zangger K. Investigating Protein-Ligand Interactions by Solution Nuclear Magnetic Resonance Spectroscopy. ChemPhysChem (2018) 19:895–906. doi: 10.1002/cphc.201701253
198. Li Q. Application of Fragment-Based Drug Discovery to Versatile Targets. Front Mol Biosci (2020) 7:180. doi: 10.3389/fmolb.2020.00180
199. Arroyo X, Goldflam M, Feliz M, Belda I, Giralt E. Computer-Aided Design of Fragment Mixtures for NMR-Based Screening. PloS One (2013) 8:e58571. doi: 10.1371/journal.pone.0058571
200. Navratilova I, Hopkins AL. Fragment Screening by Surface Plasmon Resonance. ACS Med Chem Lett (2010) 1:44–8. doi: 10.1021/ml900002k
201. Giannetti AM. From Experimental Design to Validated Hits a Comprehensive Walk-Through of Fragment Lead Identification Using Surface Plasmon Resonance. In: 1st ed. Methods in Enzymology. Cambridge, MA, USA: Elsevier Inc (2011). doi: 10.1016/B978-0-12-381274-2.00008-X
202. Giannetti AM, Koch BD, Browner MF. Surface Plasmon Resonance Based Assay for the Detection and Characterization of Promiscuous Inhibitors. J Med Chem (2008) 51:574–80. doi: 10.1021/jm700952v
203. Niesen FH, Berglund H, Vedadi M. The Use of Differential Scanning Fluorimetry to Detect Ligand Interactions That Promote Protein Stability. Nat Protoc (2007) 2:2212–21. doi: 10.1038/nprot.2007.321
204. Kranz JK, Schalk-Hihi C. Protein Thermal Shifts to Identify Low Molecular Weight Fragments. In: 1st ed. Methods in Enzymology. Cambridge, MA, USA: Elsevier Inc (2011). doi: 10.1016/B978-0-12-381274-2.00011-X
205. Kirsch P, Hartman AM, Hirsch AKH, Empting M. Concepts and Core Principles of Fragment-Based Drug Design. Molecules (2019) 24:4309. doi: 10.3390/molecules24234309
206. Collins PM, Douangamath A, Talon R, Dias A, Brandao-Neto J, Krojer T, et al. Achieving a Good Crystal System for Crystallographic X-Ray Fragment Screening. In: 1st ed. Methods in Enzymology. Cambridge, MA, USA: Elsevier Inc (2018). doi: 10.1016/bs.mie.2018.09.027
207. Hassell AM, An G, Bledsoe RK, Bynum JM, Carter HL, Deng SJJ, et al. Crystallization of Protein-Ligand Complexes. Acta Crystallogr Sect D Biol Crystallogr (2006) 63:72–9. doi: 10.1107/S0907444906047020
208. Danley DE. Crystallization to Obtain Protein-Ligand Complexes for Structure-Aided Drug Design. Acta Crystallogr Sect D Biol Crystallogr (2006) 62:569–75. doi: 10.1107/S0907444906012601
209. Hoffer L, Muller C, Roche P, Morelli X. Chemistry-Driven Hit-to-lead Optimization Guided by Structure-based Approaches. Mol Inform (2018) 37:1800059. doi: 10.1002/minf.201800059
210. Murray CW, Rees DC. The Rise of Fragment-Based Drug Discovery. Nat Chem (2009) 1:187–92. doi: 10.1038/nchem.217
211. Chilingaryan Z, Yin Z, Oakley AJ. Fragment-Based Screening by Protein Crystallography: Successes and Pitfalls. Int J Mol Sci (2012) 13:12857–79. doi: 10.3390/ijms131012857
212. Murray CW, Rees DC. Opportunity Knocks: Organic Chemistry for Fragment-Based Drug Discovery (FBDD). Angew Chem Int Ed (2016) 55:488–92. doi: 10.1002/anie.201506783
213. Valenti D, Hristeva S, Tzalis D, Ottmann C. Clinical Candidates Modulating Protein-Protein Interactions: The Fragment-Based Experience. Eur J Med Chem (2019) 167:76–95. doi: 10.1016/j.ejmech.2019.01.084
214. Hopkins AL, Groom CR, Alex A. Ligand Efficiency: A Useful Metric for Lead Selection. Drug Discov Today (2004) 9:430–1. doi: 10.1016/S1359-6446(04)03069-7
215. Kumar S, Waldo JP, Jaipuri FA, Marcinowicz A, Van Allen C, Adams J, et al. Discovery of Clinical Candidate (1 R,4 R)-4-((R)-2-((S)-6-Fluoro-5 H-imidazo[5,1-A[isoindol-5-yl)-1-hydroxyethyl)cyclohexan-1-ol (Navoximod), a Potent and Selective Inhibitor of Indoleamine 2,3-Dioxygenase 1. J Med Chem (2019) 62:6705–33. doi: 10.1021/acs.jmedchem.9b00662
216. Hudson SA, McLean KJ, Surade S, Yang YQ, Leys D, Ciulli A, et al. Application of Fragment Screening and Merging to the Discovery of Inhibitors of the Mycobacterium Tuberculosis Cytochrome P450 CYP121. Angew Chem Int Ed (2012) 51:9311–6. doi: 10.1002/anie.201202544
217. Hung AW, Silvestre HL, Wen S, Ciulli A, Blundell TL, Abell C. Application of Fragment Growing and Fragment Linking to the Discovery of Inhibitors of Mycobacterium Tuberculosis Pantothenate Synthetase. Angew Chem Int Ed (2009) 48:8452–6. doi: 10.1002/anie.200903821
218. Lamoree B, Hubbard RE. Current Perspectives in Fragment-Based Lead Discovery (FBLD). Essays Biochem (2017) 61:453–64. doi: 10.1042/EBC20170028
219. Kidd SL, Osberger TJ, Mateu N, Sore HF, Spring DR. Recent Applications of Diversity-Oriented Synthesis Toward Novel, 3-Dimensional Fragment Collections. Front Chem (2018) 6:460. doi: 10.3389/fchem.2018.00460
220. Drwal MN, Bret G, Perez C, Jacquemard C, Desaphy J, Kellenberger E. Structural Insights on Fragment Binding Mode Conservation. J Med Chem (2018) 61:5963–73. doi: 10.1021/acs.jmedchem.8b00256
221. Scott DE, Coyne AG, Hudson SA, Abell C. Fragment-Based Approaches in Drug Discovery and Chemical Biology. Biochemistry (2012) 51:4990–5003. doi: 10.1021/bi3005126
222. Singh M, Tam B, Akabayov B. NMR-Fragment Based Virtual Screening: A Brief Overview. Molecules (2018) 23:233. doi: 10.3390/molecules23020233
223. Miyake Y, Itoh Y, Hatanaka A, Suzuma Y, Suzuki M, Kodama H, et al. Identification of Novel Lysine Demethylase 5-Selective Inhibitors by Inhibitor-Based Fragment Merging Strategy. Bioorg Med Chem (2019) 27:1119–29. doi: 10.1016/j.bmc.2019.02.006
224. Bian Y, Xie X-Q. Computational Fragment-Based Drug Design: Current Trends, Strategies, and Applications. AAPS J (2018) 20:59. doi: 10.1208/s12248-018-0216-7
225. Moreira-Filho JT, Dantas RF, Senger MR, Silva AC, Campos DMB, Muratov E, et al. Shortcuts to Schistosomiasis Drug Discovery: The State-of-the-Art. In: 1 ed. Annual Reports in Medicinal Chemistry. Cambridge, MA, USA: Elsevier (2019). p. 139–80. doi: 10.1016/bs.armc.2019.06.004
226. Nazaré M, Matter H, Will DW, Wagner M, Urmann M, Czech J, et al. Fragment Deconstruction of Small, Potent Factor Xa Inhibitors: Exploring the Superadditivity Energetics of Fragment Linking in Protein-Ligand Complexes. Angew Chem Int Ed (2012) 51:905–11. doi: 10.1002/anie.201107091
227. Mondal M, Radeva N, Fanlo-Virgós H, Otto S, Klebe G, Hirsch AKH. Fragment Linking and Optimization of Inhibitors of the Aspartic Protease Endothiapepsin: Fragment-Based Drug Design Facilitated by Dynamic Combinatorial Chemistry. Angew Chem Int Ed (2016) 55:9422–6. doi: 10.1002/anie.201603074
228. Kuntz AN, Davioud-Charvet E, Sayed AA, Califf LL, Dessolin J, Arnér ESJ, et al. Thioredoxin Glutathione Reductase From Schistosoma Mansoni: An Essential Parasite Enzyme and a Key Drug Target. PloS Med (2007) 4:e206. doi: 10.1371/journal.pmed.0040206
229. Prast-Nielsen S, Huang HH, Williams DL. Thioredoxin Glutathione Reductase: Its Role in Redox Biology and Potential as a Target for Drugs Against Neglected Diseases. Biochim Biophys Acta - Gen Subj (2011) 1810:1262–71. doi: 10.1016/j.bbagen.2011.06.024
230. Song L, Li J, Xie S, Qian C, Wang J, Zhang W, et al. Thioredoxin Glutathione Reductase as a Novel Drug Target: Evidence From Schistosoma Japonicum. PloS One (2012) 7:e31456. doi: 10.1371/journal.pone.0031456
231. Simeonov A, Jadhav A, Sayed AA, Wang Y, Nelson ME, Thomas CJ, et al. Quantitative High-Throughput Screen Identifies Inhibitors of the Schistosoma Mansoni Redox Cascade. PloS Negl Trop Dis (2008) 2:e127. doi: 10.1371/journal.pntd.0000127
232. Lea WA, Jadhav A, Rai G, Sayed AA, Cass CL, Inglese J, et al. A 1,536-Well-Based Kinetic HTS Assay for Inhibitors of Schistosoma Mansoni Thioredoxin Glutathione Reductase. Assay Drug Dev Technol (2008) 6:551–5. doi: 10.1089/adt.2008.149
233. Silvestri I, Lyu H, Fata F, Boumis G, Miele AE, Ardini M, et al. Fragment-Based Discovery of a Regulatory Site in Thioredoxin Glutathione Reductase Acting as “Doorstop” for NADPH Entry. ACS Chem Biol (2018) 13:2190–202. doi: 10.1021/acschembio.8b00349
234. Sheng C, Zhang W. Fragment Informatics and Computational Fragment-Based Drug Design: An Overview and Update. Med Res Rev (2013) 33:554–98. doi: 10.1002/med.21255
235. Grove LE, Vajda S, Kozakov D. Computational Methods to Support Fragment-Based Drug Discovery. In: Erlanson DA, Jahnke W, editors. Fragment-Based Drug Discovery: Lessons and Outlook. Weinheim, Germany: Wiley-VCH Verlag GmbH & Co. KGaA (2016). p. 197–222. doi: 10.1002/9783527683604.ch09
236. Gianti E, Sartori L. Identification and Selection of “Privileged Fragments” Suitable for Primary Screening. J Chem Inf Model (2008) 48:2129–39. doi: 10.1021/ci800219h
237. Hoffer L, Renaud JP, Horvath D. In Silico Fragment-Based Drug Discovery: Setup and Validation of a Fragment-to-Lead Computational Protocol Using S4MPLE. J Chem Inf Model (2013) 53:836–51. doi: 10.1021/ci4000163
238. Barelier S, Eidam O, Fish I, Hollander J, Figaroa F, Nachane R, et al. Increasing Chemical Space Coverage by Combining Empirical and Computational Fragment Screens. ACS Chem Biol (2014) 9:1528–35. doi: 10.1021/cb5001636
239. Kumar A, Voet A, Zhang KYJ. Fragment Based Drug Design: From Experimental to Computational Approaches. Curr Med Chem (2012) 19:5128–47. doi: 10.2174/092986712803530467
240. Rudling A, Gustafsson R, Almlöf I, Homan E, Scobie M, Warpman Berglund U, et al. Fragment-Based Discovery and Optimization of Enzyme Inhibitors by Docking of Commercial Chemical Space. J Med Chem (2017) 60:8160–9. doi: 10.1021/acs.jmedchem.7b01006
241. Lyu J, Wang S, Balius TE, Singh I, Levit A, Moroz YS, et al. Ultra-Large Library Docking for Discovering New Chemotypes. Nature (2019) 566:224–9. doi: 10.1038/s41586-019-0917-9
242. Kleandrova VV, Speck-Planche A. The QSAR Paradigm in Fragment-Based Drug Discovery: From the Virtual Generation of Target Inhibitors to Multi-Scale Modeling. Mini Rev Med Chem (2020) 20:1357–74. doi: 10.2174/1389557520666200204123156
243. de Souza Neto LR, Moreira-Filho JT, Neves BJ, Maidana RLBR, Guimarães ACR, Furnham N, et al. In Silico Strategies to Support Fragment-to-Lead Optimization in Drug Discovery. Front Chem (2020) 8:93. doi: 10.3389/fchem.2020.00093
244. Erlanson DA, Davis BJ, Jahnke W. Fragment-Based Drug Discovery: Advancing Fragments in the Absence of Crystal Structures. Cell Chem Biol (2019) 26:9–15. doi: 10.1016/j.chembiol.2018.10.001
245. Bissaro M, Sturlese M, Moro S. The Rise of Molecular Simulations in Fragment-Based Drug Design (FBDD): An Overview. Drug Discov Today (2020) 25:1693–701. doi: 10.1016/j.drudis.2020.06.023
246. Protasio AV, Tsai IJ, Babbage A, Nichol S, Hunt M, Aslett MA, et al. A Systematically Improved High Quality Genome and Transcriptome of the Human Blood Fluke Schistosoma Mansoni. PloS Negl Trop Dis (2012) 6:e1455. doi: 10.1371/journal.pntd.0001455
247. Young ND, Jex AR, Li B, Liu S, Yang L, Xiong Z, et al. Whole-Genome Sequence of Schistosoma Haematobium. Nat Genet (2012) 44:221–5. doi: 10.1038/ng.1065
248. Zhou Y, Zheng H, Chen Y, Zhang L, Wang K, Guo J, et al. The Schistosoma Japonicum Genome Reveals Features of Host–Parasite Interplay. Nature (2009) 460:345–51. doi: 10.1038/nature08140
249. Luo F, Yin M, Mo X, Sun C, Wu Q, Zhu B, et al. An Improved Genome Assembly of the Fluke Schistosoma Japonicum. PloS Negl Trop Dis (2019) 13:e0007612. doi: 10.1371/journal.pntd.0007612
250. Stroehlein AJ, Korhonen PK, Chong TM, Lim YL, Chan KG, Webster B, et al. High-Quality Schistosoma Haematobium Genome Achieved by Single-Molecule and Long-Range Sequencing. Gigascience (2019) 8:1–12. doi: 10.1093/gigascience/giz108
251. Mitchell AL, Attwood TK, Babbitt PC, Blum M, Bork P, Bridge A, et al. InterPro in 2019: Improving Coverage, Classification and Access to Protein Sequence Annotations. Nucleic Acids Res (2019) 47:D351–60. doi: 10.1093/nar/gky1100
252. Lam SD, Dawson NL, Das S, Sillitoe I, Ashford P, Lee D, et al. Gene3D: Expanding the Utility of Domain Assignments. Nucleic Acids Res (2016) 44:D404–9. doi: 10.1093/nar/gkv1231
253. Sillitoe I, Lewis TE, Cuff A, Das S, Ashford P, Dawson NL, et al. CATH: Comprehensive Structural and Functional Annotations for Genome Sequences. Nucleic Acids Res (2015) 43:D376–81. doi: 10.1093/nar/gku947
254. Lu S, Wang J, Chitsaz F, Derbyshire MK, Geer RC, Gonzales NR, et al. CDD/SPARCLE: The Conserved Domain Database in 2020. Nucleic Acids Res (2020) 48:D265–8. doi: 10.1093/nar/gkz991
255. Thomas PD, Campbell MJ, Kejariwal A, Mi H, Karlak B, Daverman R, et al. PANTHER: A Library of Protein Families and Subfamilies Indexed by Function. Genome Res (2003) 13:2129–41. doi: 10.1101/gr.772403
256. Finn RD, Coggill P, Eberhardt RY, Eddy SR, Mistry J, Mitchell AL, et al. The Pfam Protein Families Database: Towards a More Sustainable Future. Nucleic Acids Res (2015) 44:D279–85. doi: 10.1093/nar/gkv1344
257. Mistry J, Chuguransky S, Williams L, Qureshi M, Salazar GA, Sonnhammer ELL, et al. Pfam: The Protein Families Database in 2021. Nucleic Acids Res (2021) 49:D412–9. doi: 10.1093/nar/gkaa913
258. Sigrist CJA, Cerutti L, Hulo N, Gattiker A, Falquet L, Pagni M, et al. Prosite: A Documented Database Using Patterns and Profiles as Motif Descriptors. Brief Bioinform (2002) 3:265–74. doi: 10.1093/bib/3.3.265
259. Attwood TK, Coletta A, Muirhead G, Pavlopoulou A, Philippou PB, Popov I, et al. The PRINTS Database: A Fine-Grained Protein Sequence Annotation and Analysis Resource-its Status in 2012. Database (2012) 2012:1–9. doi: 10.1093/database/bas019
260. Letunic I, Khedkar S, Bork P. SMART: Recent Updates, New Developments and Status in 2020. Nucleic Acids Res (2021) 49:D458–60. doi: 10.1093/nar/gkaa937
261. Akiva E, Brown S, Almonacid DE, Barber AE, Custer AF, Hicks MA, et al. The Structure-Function Linkage Database. Nucleic Acids Res (2014) 42:521–30. doi: 10.1093/nar/gkt1130
262. Pandurangan AP, Stahlhacke J, Oates ME, Smithers B, Gough J. The SUPERFAMILY 2.0 Database: A Significant Proteome Update and a New Webserver. Nucleic Acids Res (2019) 47:D490–4. doi: 10.1093/nar/gky1130
263. Gough J, Karplus K, Hughey R, Chothia C. Assignment of Homology to Genome Sequences Using a Library of Hidden Markov Models That Represent All Proteins of Known Structure. J Mol Biol (2001) 313:903–19. doi: 10.1006/jmbi.2001.5080
264. Haft DH, Loftus BJ, Richardson DL, Yang F, Eisen JA, Paulsen IT, et al. TIGRFAMs: A Protein Family Resource for the Functional Identification of Proteins. Nucleic Acids Res (2001) 29:41–3. doi: 10.1093/nar/29.1.41
265. Du J, Li M, Yuan Z, Guo M, Song J, Xie X, et al. A Decision Analysis Model for KEGG Pathway Analysis. BMC Bioinformatics (2016) 17:407. doi: 10.1186/s12859-016-1285-1
266. Ashburner M, Ball CA, Blake JA, Botstein D, Butler H, Cherry JM, et al. Gene Ontology : Tool for the Unification of Biology. Nat Genet (2011) 25:25–9. doi: 10.1038/75556
267. Furnham N, de Beer TAP, Thornton JM. Current Challenges in Genome Annotation Through Structural Biology and Bioinformatics. Curr Opin Struct Biol (2012) 22:594–601. doi: 10.1016/j.sbi.2012.07.005
268. Harris TW, Arnaboldi V, Cain S, Chan J, Chen WJ, Cho J, et al. WormBase: A Modern Model Organism Information Resource. Nucleic Acids Res (2019) 48:D762–7. doi: 10.1093/nar/gkz920
269. Xia T, Giri BR, Liu J, Du P, Li X, Li X, et al. RNA Sequencing Analysis of Altered Expression of Long Noncoding RNAs Associated With Schistosoma Japonicum Infection in the Murine Liver and Spleen. Parasit Vectors (2020) 13:1–15. doi: 10.1186/s13071-020-04457-9
270. Vasconcelos EJR, Dasilva LF, Pires DS, Lavezzo GM, Pereira ASA, Amaral MS, et al. The Schistosoma Mansoni Genome Encodes Thousands of Long non-Coding RNAs Predicted to be Functional at Different Parasite Life-Cycle Stages. Sci Rep (2017) 7:1–17. doi: 10.1038/s41598-017-10853-6
271. Amaral MS, Maciel LF, Silveira GO, Olberg GGO, Leite JVP, Imamura LK, et al. Long non-Coding RNA Levels can be Modulated by 5-Azacytidine in Schistosoma Mansoni. Sci Rep (2020) 10:1–17. doi: 10.1038/s41598-020-78669-5
272. Wendt G, Zhao L, Chen R, Liu C, O’Donoghue AJ, Caffrey CR, et al. A Single-Cell RNAseq Atlas of the Pathogenic Stage of Schistosoma Mansoni Identifies a Key Regulator of Blood Feeding. bioRxiv (2020) 1649:1644–9. doi: 10.1101/2020.02.03.932004
273. Maciel LF, Verjovski-Almeida S. Step-by-Step Bioinformatics Analysis of Schistosoma Mansoni Long non-Coding RNA Sequences. In: Timson DJ, editor. Methods in Molecular Biology. New York: Humana (2020). p. 109–33. doi: 10.1007/978-1-0716-0635-3_10
275. Kumar S, Filipski AJ, Battistuzzi FU, Pond SLK, Tamura K. Statistics and Truth in Phylogenomics. Mol Biol Evol (2012) 29:457–72. doi: 10.1093/molbev/msr202
276. Koonin EV. Orthologs, Paralogs, and Evolutionary Genomics. Annu Rev Genet (2005) 39:309–38. doi: 10.1146/annurev.genet.39.073003.114725
277. Engelhardt BE, Jordan MI, Muratore KE, Brenner SE. Protein Molecular Function Prediction by Bayesian Phylogenomics. PloS Comput Biol (2005) 1:e45. doi: 10.1371/journal.pcbi.0010045
278. Brown D, Sjo K. Functional Classification Using Phylogenomic Inference. PloS Comput Biol (2006) 2:e77. doi: 10.1371/journal.pcbi.0020077
279. Guindon S, Dufayard JF, Lefort V, Anisimova M, Hordijk W, Gascuel O. New Algorithms and Methods to Estimate Maximum-Likelihood Phylogenies: Assessing the Performance of PhyML 3.0. Syst Biol (2010) 59:307–21. doi: 10.1093/sysbio/syq010
280. Stamatakis A. RaxML Version 8: A Tool for Phylogenetic Analysis and Post-Analysis of Large Phylogenies. Bioinformatics (2014) 30:1312–3. doi: 10.1093/bioinformatics/btu033
281. Price MN, Dehal PS, Arkin AP. FastTree 2 – Approximately Maximum-Likelihood Trees for Large Alignments. PloS One (2010) 5:e9490. doi: 10.1371/journal.pone.0009490
282. Kind KK, Sgaramella-zonta LA. Phylogenetic Analysis : Concepts and Methods. Am J Hum Genet (1971) 23:235–52.
283. Zhou X, Shen X-X, Hittinger CT, Rokas A. Evaluating Fast Maximum Likelihood-Based Phylogenetic Programs Using Empirical Phylogenomic Data Sets. Mol Biol Evol (2018) 35:486–503. doi: 10.1093/molbev/msx302
285. Penny D, Hendy MD, Steel MA. Progress With Methods for Constructing Evolutionary Trees. Trends Ecol Evol (1992) 7:73–9. doi: 10.1016/0169-5347(92)90244-6
286. Kumar S, Stecher G, Tamura K. MEGA7: Molecular Evolutionary Genetics Analysis Version 7.0 for Bigger Datasets. Mol Biol Evol (2016) 33:1870–4. doi: 10.1093/molbev/msw054
287. Doolittle WF. Phylogenetic Classification and the Universal Tree. Science (1999) 284:2124–8. doi: 10.1126/science.284.5423.2124
288. Mullard A. 2018 FDA Drug Approvals. Nat Rev Drug Discov (2019) 18:85–9. doi: 10.1038/d41573-019-00014-x
289. Smietana K, Siatkowski M, Møller M. Trends in Clinical Success Rates. Nat Rev Drug Discov (2016) 15:379–80. doi: 10.1038/nrd.2016.85
290. Hopfinger A. Computer-Assisted Drug Design. J Med Chem (1985) 28:1133–9. doi: 10.1021/jm00147a001
291. Baig MH, Ahmad K, Roy S, Ashraf JM, Adil M, Siddiqui MH, et al. Computer Aided Drug Design: Success and Limitations. Curr Pharm Des (2016) 22:572–81. doi: 10.2174/1381612822666151125000550
292. Gasteiger J. Introduction. In: Gasteiger J, Engel T, editors. Chemoinformatics. Weinheim, FRG: Wiley-VCH Verlag GmbH & Co. KGaA (2003). p. 1–13. doi: 10.1002/3527601643.ch1
293. Brown FK. Chemoinformatics: What is it and How Does it Impact Drug Discovery. In: 1 ed . Annual Reports in Medicinal Chemistry. Cambridge, MA, USA: Elsevier (1998). p. 375–84. doi: 10.1016/S0065-7743(08)61100-8
294. Engel T. Basic Overview of Chemoinformatics. J Chem Inf Model (2006) 46:2267–77. doi: 10.1021/ci600234z
295. Yang X, Wang Y, Byrne R, Schneider G, Yang S. Concepts of Artificial Intelligence for Computer-Assisted Drug Discovery. Chem Rev (2019) 119:10520–94. doi: 10.1021/acs.chemrev.8b00728
296. Schneider P, Walters WP, Plowright AT, Sieroka N, Listgarten J, Goodnow RA, et al. Rethinking Drug Design in the Artificial Intelligence Era. Nat Rev Drug Discov (2020) 19:353–64. doi: 10.1038/s41573-019-0050-3
297. Mater AC, Coote ML. Deep Learning in Chemistry. J Chem Inf Model (2019) 59:2545–59. doi: 10.1021/acs.jcim.9b00266
298. Ekins S, Puhl AC, Zorn KM, Lane TR, Russo DP, Klein JJ, et al. Exploiting Machine Learning for End-to-End Drug Discovery and Development. Nat Mater (2019) 18:435–41. doi: 10.1038/s41563-019-0338-z
299. Caffrey CR, El-Sakkary N, Mäder P, Krieg R, Becker K, Schlitzer M, et al. Drug Discovery and Development for Schistosomiasis. In: 1 ed. Neglected Tropical Diseases: Drug Discovery and Development. Weinheim, Germany: Wiley‐VCH Verlag GmbH & Co. KGaA (2019). p. 187–225. doi: 10.1002/9783527808656.ch8
300. Zhu H. Big Data and Artificial Intelligence Modeling for Drug Discovery. Annu Rev Pharmacol Toxicol (2020) 60:573–89. doi: 10.1146/annurev-pharmtox-010919-023324
301. Wang Y, Bolton E, Dracheva S, Karapetyan K, Shoemaker BA, Suzek TO, et al. An Overview of the PubChem BioAssay Resource. Nucleic Acids Res (2010) 38:D255–66. doi: 10.1093/nar/gkp965
302. Wang Y, Xiao J, Suzek TO, Zhang J, Wang J, Zhou Z, et al. Pubchem’s BioAssay Database. Nucleic Acids Res (2012) 40:D400–12. doi: 10.1093/nar/gkr1132
303. Kim S, Chen J, Cheng T, Gindulyte A, He J, He S, et al. PubChem in 2021: New Data Content and Improved Web Interfaces. Nucleic Acids Res (2021) 49:D1388–95. doi: 10.1093/nar/gkaa971
304. Gaulton A, Bellis LJ, Bento AP, Chambers J, Davies M, Hersey A, et al. ChEMBL: A Large-Scale Bioactivity Database for Drug Discovery. Nucleic Acids Res (2012) 40:D1100–7. doi: 10.1093/nar/gkr777
305. Duffy BC, Zhu L, Decornez H, Kitchen DB. Early Phase Drug Discovery: Cheminformatics and Computational Techniques in Identifying Lead Series. Bioorg Med Chem (2012) 20:5324–42. doi: 10.1016/j.bmc.2012.04.062
306. Neves BJ, Muratov E, Machado RB, Andrade CH, Cravo PVL. Modern Approaches to Accelerate Discovery of New Antischistosomal Drugs. Expert Opin Drug Discov (2016) 11:557–67. doi: 10.1080/17460441.2016.1178230
307. Martinez-Mayorga K, Madariaga-Mazon A, Medina-Franco JL, Maggiora G. The Impact of Chemoinformatics on Drug Discovery in the Pharmaceutical Industry. Expert Opin Drug Discov (2020) 15:293–306. doi: 10.1080/17460441.2020.1696307
308. Chen H, Kogej T, Engkvist O. Cheminformatics in Drug Discovery, an Industrial Perspective. Mol Inform (2018) 37:1800041. doi: 10.1002/minf.201800041
309. Gasteiger J. Chemoinformatics: Achievements and Challenges, a Personal View. Molecules (2016) 21:151. doi: 10.3390/molecules21020151
310. Humbeck L, Koch O. What Can We Learn From Bioactivity Data? Chemoinformatics Tools and Applications in Chemical Biology Research. ACS Chem Biol (2017) 12:23–35. doi: 10.1021/acschembio.6b00706
311. Bajorath J. Foundations of Data-Driven Medicinal Chemistry. Futur Sci OA (2018) 4:FSO320. doi: 10.4155/fsoa-2018-0057
312. Mak KK, Pichika MR. Artificial Intelligence in Drug Development: Present Status and Future Prospects. Drug Discov Today (2019) 24:773–80. doi: 10.1016/j.drudis.2018.11.014
313. Schneider G. Automating Drug Discovery. Nat Rev Drug Discov (2017) 17:97–113. doi: 10.1038/nrd.2017.232
314. Lo Y-C, Rensi SE, Torng W, Altman RB. Machine Learning in Chemoinformatics and Drug Discovery. Drug Discov Today (2018) 23:1538–46. doi: 10.1016/j.drudis.2018.05.010
315. Schneider G. Mind and Machine in Drug Design. Nat Mach Intell (2019) 1:128–30. doi: 10.1038/s42256-019-0030-7
316. Griffen EJ, Dossetter AG, Leach AG. Chemists: AI is Here; Unite To Get the Benefits. J Med Chem (2020) 63:8695–704. doi: 10.1021/acs.jmedchem.0c00163
317. Vamathevan J, Clark D, Czodrowski P, Dunham I, Ferran E, Lee G, et al. Applications of Machine Learning in Drug Discovery and Development. Nat Rev Drug Discov (2019) 18:463–77. doi: 10.1038/s41573-019-0024-5
318. Neves BJ, Braga RC, Melo-Filho CC, Moreira-Filho JT, Muratov EN, Andrade CH. QSAR-Based Virtual Screening: Advances and Applications in Drug Discovery. Front Pharmacol (2018) 9:1275. doi: 10.3389/fphar.2018.01275
319. Zhavoronkov A, Vanhaelen Q, Oprea TI. Will Artificial Intelligence for Drug Discovery Impact Clinical Pharmacology? Clin Pharmacol Ther (2020) 107:780–5. doi: 10.1002/cpt.1795
320. Svetnik V, Liaw A, Tong C, Culberson JC, Sheridan RP, Feuston BP. Random Forest: A Classification and Regression Tool for Compound Classification and QSAR Modeling. J Chem Inf Comput Sci (2003) 43:1947–58. doi: 10.1021/ci034160g
322. Cova TFGG, Pais AACC. Deep Learning for Deep Chemistry: Optimizing the Prediction of Chemical Patterns. Front Chem (2019) 7:809. doi: 10.3389/fchem.2019.00809
323. Tropsha A. Best Practices for QSAR Model Development, Validation, and Exploitation. Mol Inform (2010) 29:476–88. doi: 10.1002/minf.201000061
324. Fourches D, Muratov E, Tropsha A. Trust, But Verify: on the Importance of Chemical Structure Curation in Cheminformatics and QSAR Modeling Research. J Chem Inf Model (2010) 50:1189–204. doi: 10.1021/ci100176x
325. Fourches D, Muratov E, Tropsha A. Curation of Chemogenomics Data. Nat Chem Biol (2015) 11:535. doi: 10.1038/nchembio.1881
326. Fourches D, Muratov EN, Tropsha A. Trust, But Verify II: A Practical Guide to Chemogenomics Data Curation. J Chem Inf Model (2016) 56:1243–52. doi: 10.1021/acs.jcim.6b00129
327. Danishuddin, Khan AU. Descriptors and Their Selection Methods in QSAR Analysis: Paradigm for Drug Design. Drug Discov Today (2016) 21:1291–302. doi: 10.1016/j.drudis.2016.06.013
328. Todeschini R, Consonni V. Molecular Descriptors for Chemoinformatics. Weinheim, Germany: WILEY-VCH (2009).
329. Muratov EN, Bajorath J, Sheridan RP, Tetko IV, Filimonov D, Poroikov V, et al. QSAR Without Borders. Chem Soc Rev (2020) 49:3525–64. doi: 10.1039/d0cs00098a
330. Cherkasov A, Muratov EN, Fourches D, Varnek A, Baskin II, Cronin M, et al. QSAR Modeling: Where Have You Been? Where are You Going to? J Med Chem (2014) 57:4977–5010. doi: 10.1021/jm4004285
331. Tropsha A, Gramatica P, Gombar VK. The Importance of Being Earnest: Validation is the Absolute Essential for Successful Application and Interpretation of QSPR Models. QuantStructActRelatCombSci (2003) 22:69–77. doi: 10.1002/qsar.200390007
332. Roy K, Mitra I. On Various Metrics Used for Validation of Predictive QSAR Models With Applications in Virtual Screening and Focused Library Design. Comb Chem High Throughput Screen (2011) 14:450–74. doi: 10.2174/138620711795767893
333. OECD Principles for the Validation, for Regulatory Purposes, of (Quantitative) Structure-Activity Relationship Models. Organ Econ Coop Dev (2004), 1–2.
334. Eriksson L, Jaworska J, Worth AP, Cronin MTD, McDowell RM, Gramatica P. Methods for Reliability and Uncertainty Assessment and for Applicability Evaluations of Classification- and Regression-Based QSARs. Environ Health Perspect (2003) 111:1361–75. doi: 10.1289/ehp.5758
335. Mathea M, Klingspohn W, Baumann K. Chemoinformatic Classification Methods and Their Applicability Domain. Mol Inform (2016) 35:160–80. doi: 10.1002/minf.201501019
336. Gadaleta D, Mangiatordi GF, Catto M, Carotti A, Nicolotti O. Applicability Domain for QSAR Models. Int J Quant Struct Relat (2016) 1:45–63. doi: 10.4018/IJQSPR.2016010102
337. Netzeva TI, Worth A, Aldenberg T, Benigni R, Cronin MTD, Gramatica P, et al. Current Status of Methods for Defining the Applicability Domain of (Quantitative) Structure-Activity Relationships. The Report and Recommendations of ECVAM Workshop 52. Altern Lab Anim (2005) 33:155–73. doi: 10.1177/026119290503300209
338. Zorn KM, Sun S, McConnon CL, Ma K, Chen EK, Foil DH, et al. A Machine Learning Strategy for Drug Discovery Identifies Anti-Schistosomal Small Molecules. ACS Infect Dis (2021) 7:406–20. doi: 10.1021/acsinfecdis.0c00754
339. Ciallella HL, Zhu H. Advancing Computational Toxicology in the Big Data Era by Artificial Intelligence: Data-Driven and Mechanism-Driven Modeling for Chemical Toxicity. Chem Res Toxicol (2019) 32:536–47. doi: 10.1021/acs.chemrestox.8b00393
341. Klambauer G, Hochreiter S, Rarey M. Machine Learning in Drug Discovery. J Chem Inf Model (2019) 59:945–6. doi: 10.1021/acs.jcim.9b00136
342. Yu CH, Qin Z, Martin-Martinez FJ, Buehler MJ. A Self-Consistent Sonification Method to Translate Amino Acid Sequences Into Musical Compositions and Application in Protein Design Using Artificial Intelligence. ACS Nano (2019) 13:7471–82. doi: 10.1021/acsnano.9b02180
343. Bjerrum EJ. SMILES Enumeration as Data Augmentation for Neural Network Modeling of Molecules (2017). Available at: http://arxiv.org/abs/1703.07076.
344. Klebe G. Virtual Ligand Screening: Strategies, Perspectives and Limitations. Drug Discov Today (2006) 11:580–94. doi: 10.1016/j.drudis.2006.05.012
345. Braga RC, Alves VM, Silva AC, Liao LM, Andrade CH. Virtual Screening Strategies in Medicinal Chemistry: The State of the Art and Current Challenges. Curr Top Med Chem (2014) 14:1899–912. doi: 10.2174/1568026614666140929120749
346. Badrinarayan P, Sastry GN. Virtual High Throughput Screening in New Lead Identification. Comb Chem High Throughput Screen (2011) 14:840–60. doi: 10.2174/138620711797537102
347. Maia EHB, Assis LC, de Oliveira TA, da Silva AM, Taranto AG. Structure-Based Virtual Screening: From Classical to Artificial Intelligence. Front Chem (2020) 8:343. doi: 10.3389/fchem.2020.00343
348. Schmidhuber J. Deep Learning in Neural Networks: An Overview. Neural Netw (2015) 61:85–117. doi: 10.1016/j.neunet.2014.09.003
349. Valueva MV, Nagornov NN, Lyakhov PA, Valuev GV, Chervyakov NI. Application of the Residue Number System to Reduce Hardware Costs of the Convolutional Neural Network Implementation. Math Comput Simul (2020) 177:232–43. doi: 10.1016/j.matcom.2020.04.031
350. Hadsell R, Chopra S, LeCun Y. Dimensionality Reduction by Learning an Invariant Mapping. Proc IEEE Comput Soc Conf Comput Vis Pattern Recognit (2006) 2:1735–42. doi: 10.1109/CVPR.2006.100
351. Hinton GE, Salakhutdinov RR. Reducing the Dimensionality of. Science (2006) 313:504–7. doi: 10.1126/science.1127647
352. Bender A, Cortes-Ciriano I. Artificial Intelligence in Drug Discovery: What is Realistic, What are Illusions? Part 2: A Discussion of Chemical and Biological Data. Drug Discov Today (2021). doi: 10.1016/j.drudis.2020.11.037.
353. Lane TR, Foil DH, Minerali E, Urbina F, Zorn KM, Ekins S. Bioactivity Comparison Across Multiple Machine Learning Algorithms Using Over 5000 Datasets for Drug Discovery. Mol Pharm (2021) 18:403–15. doi: 10.1021/acs.molpharmaceut.0c01013
354. Bajorath J, Kearnes S, Walters WP, Meanwell NA, Georg GI, Wang S. Artificial Intelligence in Drug Discovery: Into the Great Wide Open. J Med Chem (2020) 63:8651–2. doi: 10.1021/acs.jmedchem.0c01077
355. Schneider G, Fechner U. Computer-Based De Novo Design of Drug-Like Molecules. Nat Rev Drug Discov (2005) 4:649–63. doi: 10.1038/nrd1799
356. Schneider P, Schneider G. De Novo Design at the Edge of Chaos. J Med Chem (2016) 59:4077–86. doi: 10.1021/acs.jmedchem.5b01849
357. Schneider G, Clark DE. Automated De Novo Drug Design: Are We Nearly There Yet? Angew Chem Int Ed (2019) 58:10792–803. doi: 10.1002/anie.201814681
358. Chen H, Engkvist O. Has Drug Design Augmented by Artificial Intelligence Become a Reality? Trends Pharmacol Sci (2019) 40:806–9. doi: 10.1016/j.tips.2019.09.004
359. Sanchez-Lengeling B, Aspuru-Guzik A. Inverse Molecular Design Using Machine Learning:Generative Models for Matter Engineering. Science (2018) 361:360–5. doi: 10.1126/science.aat2663
360. Segler MHS, Kogej T, Tyrchan C, Waller MP. Generating Focused Molecule Libraries for Drug Discovery With Recurrent Neural Networks. ACS Cent Sci (2018) 4:120–31. doi: 10.1021/acscentsci.7b00512
361. Olivecrona M, Blaschke T, Engkvist O, Chen H. Molecular De-Novo Design Through Deep Reinforcement Learning. J Cheminform (2017) 9:1–14. doi: 10.1186/s13321-017-0235-x
362. Putin E, Asadulaev A, Ivanenkov Y, Aladinskiy V, Sanchez-Lengeling B, Aspuru-Guzik A, et al. Reinforced Adversarial Neural Computer for De Novo Molecular Design. J Chem Inf Model (2018) 58:1194–204. doi: 10.1021/acs.jcim.7b00690
363. Gómez-Bombarelli R, Wei JN, Duvenaud D, Hernández-Lobato JM, Sánchez-Lengeling B, Sheberla D, et al. Automatic Chemical Design Using a Data-Driven Continuous Representation of Molecules. ACS Cent Sci (2018) 4:268–76. doi: 10.1021/acscentsci.7b00572
364. Sattarov B, Baskin II, Horvath D, Marcou G, Bjerrum EJ, Varnek A. De Novo Molecular Design by Combining Deep Autoencoder Recurrent Neural Networks With Generative Topographic Mapping. J Chem Inf Model (2019) 59:1182–96. doi: 10.1021/acs.jcim.8b00751
365. Yasonik J. Multiobjective De Novo Drug Design With Recurrent Neural Networks and Nondominated Sorting. J Cheminform (2020) 12:14. doi: 10.1186/s13321-020-00419-6
366. Walters WP, Murcko M. Assessing the Impact of Generative AI on Medicinal Chemistry. Nat Biotechnol (2020) 38:143–5. doi: 10.1038/s41587-020-0418-2
367. Xu Y, Lin K, Wang S, Wang L, Cai C, Song C, et al. Deep Learning for Molecular Generation. Future Med Chem (2019) 11:567–97. doi: 10.4155/fmc-2018-0358
368. Arús-Pous J, Patronov A, Bjerrum EJ, Tyrchan C, Reymond JL, Chen H, et al. SMILES-Based Deep Generative Scaffold Decorator for De-Novo Drug Design. J Cheminform (2020) 12:1–18. doi: 10.1186/s13321-020-00441-8
369. Ståhl N, Falkman G, Karlsson A, Mathiason G, Boström J. Deep Reinforcement Learning for Multiparameter Optimization in De Novo Drug Design. J Chem Inf Model (2019) 59:3166–76. doi: 10.1021/acs.jcim.9b00325
370. Zhavoronkov A, Ivanenkov YA, Aliper A, Veselov MS, Aladinskiy VA, Aladinskaya AV, et al. Deep Learning Enables Rapid Identification of Potent DDR1 Kinase Inhibitors. Nat Biotechnol (2019) 37:1038–40. doi: 10.1038/s41587-019-0224-x
371. Zhavoronkov A, Aspuru-Guzik A. Reply to ‘Assessing the Impact of Generative AI on Medicinal Chemistry’. Nat Biotechnol (2020) 38:146–6. doi: 10.1038/s41587-020-0417-3
372. Bush JT, Pogany P, Pickett SD, Barker M, Baxter A, Campos S, et al. A Turing Test for Molecular Generators. J Med Chem (2020) 63:11964–71. doi: 10.1021/acs.jmedchem.0c01148
373. Brown N, Fiscato M, Segler MHS, Vaucher AC. GuacaMol: Benchmarking Models for De Novo Molecular Design. J Chem Inf Model (2019) 59:1096–108. doi: 10.1021/acs.jcim.8b00839
374. Polykovskiy D, Zhebrak A, Sanchez-Lengeling B, Golovanov S, Tatanov O, Belyaev S, et al. Molecular Sets (MOSES): A Benchmarking Platform for Molecular Generation Models. Front Pharmacol (2020) 11:565644. doi: 10.3389/fphar.2020.565644
375. Gao W, Coley CW. The Synthesizability of Molecules Proposed by Generative Models. J Chem Inf Model (2020) 60:5714–23. doi: 10.1021/acs.jcim.0c00174
376. Corey E, Long A, Rubenstein S. Computer-Assisted Analysis in Organic Synthesis. Science (1985) 228:408–18. doi: 10.1126/science.3838594
377. Zheng S, Rao J, Zhang Z, Xu J, Yang Y. Predicting Retrosynthetic Reactions Using Self-Corrected Transformer Neural Networks. J Chem Inf Model (2020) 60:47–55. doi: 10.1021/acs.jcim.9b00949
378. Gelernter HL, Sanders AF, Larsen DL, Agarwal KK, Boivie RH, Spritzer GA, et al. Empirical Explorations of SYNCHEM. Science (80- ) (1977) 197:1041–9. doi: 10.1126/science.197.4308.1041
379. Huang Q, Li L-L, Yang S-Y. RASA: A Rapid Retrosynthesis-Based Scoring Method for the Assessment of Synthetic Accessibility of Drug-like Molecules. J Chem Inf Model (2011) 51:2768–77. doi: 10.1021/ci100216g
380. Jorgensen WL, Laird ER, Gushurst AJ, Fleischer JM, Gothe SA, Helson HE, et al. CAMEO: A Program for the Logical Prediction of the Products of Organic Reactions. Pure Appl Chem (1990) 62:1921–32. doi: 10.1351/pac199062101921
381. Satoh H, Funatsu K. SOPHIA, a Knowledge Base-Guided Reaction Prediction System - Utilization of a Knowledge Base Derived From a Reaction Database. J Chem Inf Model (1995) 35:34–44. doi: 10.1021/ci00023a005
382. Röse P, Gasteiger J. EROS 6.0, a Knowledge Based System for Reaction Prediction — Application to the Regioselectivity of the Diels-Alder Reaction. In: Software Development in Chemistry 4. Berlin, Heidelberg: Springer Berlin Heidelberg (1990). p. 275–88. doi: 10.1007/978-3-642-75430-2_30
383. Szymkuć S, Gajewska EP, Klucznik T, Molga K, Dittwald P, Startek M, et al. Computer-Assisted Synthetic Planning: The End of the Beginning. Angew Chem Int Ed (2016) 55:5904–37. doi: 10.1002/anie.201506101
384. Nair VH, Schwaller P, Laino T. Data-Driven Chemical Reaction Prediction and Retrosynthesis. Chimia (Aarau) (2019) 73:997–1000. doi: 10.2533/chimia.2019.997
385. Struble TJ, Alvarez JC, Brown SP, Chytil M, Cisar J, Desjarlais RL, et al. Current and Future Roles of Artificial Intelligence in Medicinal Chemistry Synthesis. J Med Chem (2020) 63:8667–82. doi: 10.1021/acs.jmedchem.9b02120
386. Coley CW, Green WH, Jensen KF. Machine Learning in Computer-Aided Synthesis Planning. Acc Chem Res (2018) 51:1281–9. doi: 10.1021/acs.accounts.8b00087
387. IBM RXN Platform . Available at: https://rxn.res.ibm.com/ (Accessed May 5, 2021).
Keywords: schistosomiasis, drug discovery, artificial intelligence, fragment-based drug discovery, phenotypic screening, target-based screening
Citation: Moreira-Filho JT, Silva AC, Dantas RF, Gomes BF, Souza Neto LR, Brandao-Neto J, Owens RJ, Furnham N, Neves BJ, Silva-Junior FP and Andrade CH (2021) Schistosomiasis Drug Discovery in the Era of Automation and Artificial Intelligence. Front. Immunol. 12:642383. doi: 10.3389/fimmu.2021.642383
Received: 15 December 2020; Accepted: 30 April 2021;
Published: 31 May 2021.
Edited by:
Thiago Almeida Pereira, Stanford University, United StatesReviewed by:
Conor R. Caffrey, University of California, San Diego, United StatesCopyright © 2021 Moreira-Filho, Silva, Dantas, Gomes, Souza Neto, Brandao-Neto, Owens, Furnham, Neves, Silva-Junior and Andrade. This is an open-access article distributed under the terms of the Creative Commons Attribution License (CC BY). The use, distribution or reproduction in other forums is permitted, provided the original author(s) and the copyright owner(s) are credited and that the original publication in this journal is cited, in accordance with accepted academic practice. No use, distribution or reproduction is permitted which does not comply with these terms.
*Correspondence: Carolina H. Andrade, Y2Fyb2xpbmFAdWZnLmJy
Disclaimer: All claims expressed in this article are solely those of the authors and do not necessarily represent those of their affiliated organizations, or those of the publisher, the editors and the reviewers. Any product that may be evaluated in this article or claim that may be made by its manufacturer is not guaranteed or endorsed by the publisher.
Research integrity at Frontiers
Learn more about the work of our research integrity team to safeguard the quality of each article we publish.