- 1Department of Molecular Biology and Genetics, Federal Research and Clinical Center of Physical-Chemical Medicine of Federal Medical Biological Agency, Moscow, Russia
- 2Department of Biotechnology, Vavilov Institute of General Genetics Russian Academy of Sciences, Moscow, Russia
- 3Phystech School of Biological and Medical Physics, Moscow Institute of Physics and Technology (National Research University), Dolgoprudny, Russia
- 4Laboratory of Plant Genomics, The Institute for Information Transmission Problems of the Russian Academy of Sciences (Kharkevich Institute), Moscow, Russia
- 5Faculty of Ecology, International Institute for Strategic Development of Sectoral Economics Peoples’ Friendship University of Russia (RUDN University), Moscow, Russia
As permanent residents of the normal gut microbiota, bifidobacteria have evolved to adapt to the host’s immune response whose priority is to eliminate pathogenic agents. The mechanisms that ensure the survival of commensals during inflammation and maintain the stability of the core component of the normal gut microbiota in such conditions remain poorly understood. We propose a new in vitro approach to study the mechanisms of resistance to immune response factors based on high-throughput sequencing followed by transcriptome analysis. This approach allowed us to detect differentially expressed genes associated with inflammation. In this study, we demonstrated that the presence of the pro-inflammatory cytokines IL-6 and TNFα to the growth medium of the B. longum subsp. longum GT15 strain changes the latter’s growth rate insignificantly while affecting the expression of certain genes. We identified these genes and performed a COG and a KEGG pathway enrichment analysis. Using phylogenetic profiling we predicted the operons of genes whose expression was triggered by the cytokines TNFα and IL-6 in vitro. By mapping the transcription start points, we experimentally validated the predicted operons. Thus, in this study, we predicted the genes involved in a putative signaling pathway underlying the mechanisms of resistance to inflammatory factors in bifidobacteria. Since bifidobacteria are a major component of the human intestinal microbiota exhibiting pronounced anti-inflammatory properties, this study is of great practical and scientific relevance.
Introduction
Bifidobacteria are a key component of the commensal gut microbiota conferring considerable health benefits and supporting the normal functioning of the host organism (1).
Bifidobacteria exert many immunomodulatory properties that became the subject of many in vitro and in vivo studies (2). As the predominant component of the commensal microbiota of infants (3), bifidobacteria contribute significantly to the formation of the human immune system in the early stages of postnatal development (4, 5). The key factor in this process, ostensibly, is the ability to communicate with the immune system, which results in the regulation of both anti-inflammatory and pro-inflammatory cytokines and other factors of the immune response (2, 6).
As permanent residents of the normal gut microbiota, bifidobacteria have evolved to adapt the host’s immune response whose priority is to eliminate pathogenic agents. The mechanisms that ensure the survival of commensals during inflammation and maintain the stability of the core component of the normal gut microbiota in such conditions remain poorly understood.
Today, researchers have published a few works dedicated to the study of these mechanisms in commensal microorganisms. In one study, the authors suggested that the modification of lipopolysaccharides is a putative mechanism of such stability (7). The modification of lipopolysaccharides by the putative membrane-associated phospholipid phosphatase (BT1854 in B. thetaiotaomicron) increased the resistance to antimicrobial peptides of representatives of Bacteroidetes. Among actinobacteria, only C. aerofaciens became more resistant to antimicrobial peptides (7). Thus, the resistance mechanisms in bifidobacteria have not been studied. Since orthologs of the gene encoding the phosphatase BT1854 were not found in Bifidobacterium genomes [data not shown], we suspected that other genes could be involved in an altogether different pathway, which accounts for the formation of resistance in bifidobacteria.
Microorganisms can sense signaling molecules of the host’s immune system and respond to them by changing their growth rate as well as other characteristics (8). This is corroborated by the fact that interleukin-1 (IL-1) (9), interleukin-2 (IL-2) (10), granulocyte-macrophage colony-stimulating factor (GM-CSF) (10), interleukin-6 (11), interferon-γ (IFNγ) (12), and tumor necrosis factor α (TNFα) (13) all affect bacterial growth. The pronounced response of microorganisms to cytokines and other signaling molecules of the immune system implies changes in the transcription of genes involved in this response. Whole transcriptome analysis is one way to identify these genes.
Microarray-based technology, once the main tool to carry out transcriptome analysis, was replaced later by next-generation RNA-sequencing (RNA-Seq) technology. Today, RNA-seq is best suited for analyzing the fluctuating cellular transcriptome. Bacterial RNA-seq studies have refined considerably our understanding of bacterial gene expression (14). Today, studies on bifidobacteria using this technology are lacking. For example, transcriptome studies were performed: to identify candidate genes involved in the response to oxygen treatment in B. longum BBMN68 (3%, v/v) (15); to identify the genes and operons that are actively transcribed in B. breve UCC2003 during logarithmic growth (16); to examine strain-specific responses to tetracycline in B. animalis subsp. lactis Bl-04 and HN019 (17); to understand the utilization and metabolism of xylo-oligosaccharide in B. adolescentis 15703 and to identify the key regulatory-related genes (18); to identify genes potentially involved in the response to linoleic acid exposure in B. breve DSM 20213 (19); to analyze the changes in the gene expression profile following the exposure of the industrial probiotic strains B. longum JDM301 to acid and JDM301AR (20) to unravel the physiological bases accounting for these differences by pinpointing the transcriptional responses of B. longum NCC2705 and D2957 to sublethal H2O2 exposure (21).
In the present study, we performed transcriptome analysis of the B. longum subsp. longum GT15 strain to investigate the mechanisms lying behind the response of bifidobacteria to signaling molecules of the immune system. B. longum represents one of the most prevalent species of the Bifidobacterium genus in the gut microbiota of both infants and adults (22, 23). The genome of B. longum subsp. longum GT15 was sequenced and characterized by us earlier (24).
Previously we characterized the fn3 gene in Bifidobacterium genomes (25). The gene encodes a protein containing motifs complementary to the cytokine binding region of the gp-130 receptor. The ligand family of this human cytokine receptor includes IL-6 and other cytokines belonging to the IL-6 group (26). In addition, we previously confirmed the ability of FNIII domains of this protein to specifically bind to the cytokine TNFα (27). Therefore, we opted for the pro-inflammatory cytokines IL-6 and TNFα to assess the influence of immune response factors on the expression of B. longum subsp. longum GT15 genes.
The lists of differentially expressed genes (DEGs) revealed by the transcriptome analysis can potentially help us identify the genes involved in the mechanisms of resistance of commensal microorganisms, namely bifidobacteria, to pro-inflammatory immune factors.
Materials and Methods
Bacterial Strain and Growth Condition
In this work, we used the B. longum subsp. longum GT15 strain whose genome was sequenced and submitted to GenBank and assigned the accession no. CP006741 earlier (28). The cultivation of B. longum subsp. longum GT15 was carried out in Lactobacillus MRS Broth culture medium (HiMedia, India) supplemented with 0.5% cysteine (HiMedia, India). B. longum subsp. longum GT15 was cultured at 37°C under anaerobic conditions (HiAnaerobic System - Mark III, AnaeroHiGas Pack 3.5L; HiMedia, India).
Exposure to Pro-Inflammatory Cytokines
A water solution of lyophilized recombinant human cytokines IL-6 and TNFα (Thermo Fisher Scientific, USA) was added to the experimental samples to a final concentration of [100pg/ml], [1ng/ml] and [10ng/ml]. B. longum subsp. longum GT15 grown in MRS-cys supplemented with an equal amount of water without cytokines was used as the control (10, 11). This experiment was conducted at 37°C under anaerobic conditions. Using a SmartSpec Plus spectrophotometer (Bio Rad), we measured the OD600 starting from timepoint 0 and ending at 24 h. The experiment was carried out in three biological replicates.
RNA Extraction and Sequencing and Annotation
B. longum subsp. longum GT15 cells were collected in the middle of the log-phase in both experimental and control conditions. The cells were treated with RNA Protect Bacteria Reagent (QIAGEN). Total RNA extraction and purification was performed using the RNeasy Mini Kit (QIAGEN). Removal of residual gDNA was performed using the TURBO DNA-free Kit (Invitrogen) and the RNase-Free DNase Set (QIAGEN). The concentration and quality of the extracted RNA were checked using the Quant-iT RiboGreen RNA Assay Kit (Thermo Fisher Scientific) and the Agilent RNA 6000 Pico Kit (Agilent Technologies), respectively.
Total RNA (2 µg) was used for library preparation. Ribosomal RNA was removed from the total RNA using the RiboZero rRNA Removal Kit (Bacteria) (Epicentre/Illumina, Madison, USA) and libraries were prepared using the NEBNext® Ultra II Directional RNA Library Prep Kit (NEB), according to the manufacturer protocol. Subsequently, RNA cleanup was performed with the RNA Clean XP kit (Beckman Coulter, Brea, USA). The library underwent a final cleanup using the Agencourt AMPure XP system (Beckman Coulter, Brea, USA) after which the libraries’ size distribution and quality were assessed using a high sensitivity DNA chip (Agilent Technologies). Libraries were subsequently quantified by Quant-iT DNA Assay Kit, High Sensitivity (Thermo Fisher Scientific). Finally, equimolar quantities of all libraries (12 pM) were sequenced by a high throughput run on the Illumina HiSeq using 2×100 bp paired-end reads and a 5% Phix spike-in control. Before loading the cBot system, the libraries were incubated at 98°C for 2 min and then cooled on ice to improve the hybridization of GC-rich sequences. The dataset of RNA-Seq analysis was deposited to the NCBI under the project name PRJNA628664.
Data Processing and Analysis
Data processing and analysis were performed as previously described (29). Quality control of raw reads was carried out with FASTQC v0.11.5. Adaptors were trimmed with the Trimmomatic v0.33 tool. The Kallisto v0.46.0 software was used for mapping of reads and estimation of transcript abundance. Differential expression analysis was performed using edgeR v3.26.8 package, integrated in the Degust v4.1.1 web-tool. Only genes with count per million (CPM) ≥ 1 were analyzed further. Genes were filtered based on false discovery rate cutoff (FDR) ≤ 0.05 and minimum expression fold change (FC) ≥ 1. The plots were generated using the ggplot2 package in R. DEGs were then subjected to enrichment analysis of COG functions and Kyoto Encyclopedia of Genes and Genomes (KEGG) pathways. Bedtools genomecov (30) was used for coverage calculation. Using the moving average, coverage distribution tables were obtained. Data visualization was performed using GNU/R (31).
Phylogenetic Profiling
Phylogenetic profiling included the identification of ortholog groups for Bifidobacterium genomes, the construction of binary vectors that indicate their presence (1) or absence (0) across several genomes, the construction of a matrix of pairwise distances between vectors, and the grouping of phylogenetic profiles (PP). The construction of PP was performed using the program OrthoFinder v.2.3.7 (32). A matrix of pairwise distances between PP was obtained using values based on the Jaccard Similarity Metric. PPs were constructed based on certain criteria: the distance values in a matrix of pairwise distances be minimal, genes must locate nearby and on the same strain, and all genes in the group must be downregulated or upregulated. Custom Python v.3.7.5 scripts have been used for the analysis (https://github.com/LabGenMO/phylo-profiling/blob/master/Identification%20of%20connected%20genes.ipynb).
Preparation of 5′-Enriched Libraries for Transcriptional Start Sites Identification and Sequencing
Libraries for transcription start site (TSS) identification were prepared as described earlier (33) with some modifications. To prepare 5′-enriched libraries, we enriched whole transcriptome RNA by depleting ribosomal RNA (rRNA) using standard protocol RiboMinus (Thermo Fisher Scientific). mRNA was fragmented with Zn2+, end-repaired with T4 polynucleotide kinase according to the manufacturer protocol (Thermo Fisher Scientific) and treated with Terminator exonuclease (Epicentre/Illumina, Madison, USA). This procedure enriched RNA with 5’-triphosphate protected fragments. Then, the RNA was cleaned up with KAPA Pure Beads in a ratio of 1.8 to 1 and treated with RNA 5’ Pyrophosphohydrolase (RppH) (NEB) to remove the pyrophosphate groups and cleaned up again. The strand-specific libraries for Illumina sequencing were constructed from the obtained RNA. RNA was ligated with ds-adapters with protruding inner ends containing a random hexameric sequence (5’ ds-adapter sequences: RNA oligo 5’-AGUUCUACAGUCCGACGAUC-3’ and 5’-NNNNNNGATCGTCGGA-3’; 3’ ds-adapter sequences: kinated oligo 5’-pTGGAATTCTCGGGTGCCAAGG-3’ and 5’-GAGAATTCCANNNNNN-3’) using T4 DNA ligase (Thermo Fisher Scientific). Then, cDNA was generated using 5’-GGCACCCGAGAATTCCA-3’ primer and Maxima H Minus Reverse Transcriptase (Thermo Fisher Scientific) according to the manufacturer protocol. cDNA was clean up with KAPA Pure Beads, amplified with RNA PCR Index Primers from TruSeq Small RNA kit and was cleaned up using KAPA Pure Beads. Libraries were quantified by Quant-iT DNA Assay Kit, High Sensitivity (Thermo Fisher Scientific). The quality of libraries was evaluated using Bioanalyzer 2100 with high sensitivity DNA chip (Agilent Technologies).
Finally, equimolar quantities of all libraries (12 pM) were sequenced by high-throughput sequencing on Illumina HiSeq using 2×100 bp paired-end reads and a 5% PhiX spike-in control.
Identification of Transcriptional Start Sites
Quality control on raw reads was carried out with FASTQC v0.11.5. Adaptors were trimmed using Trimmomatic v0.33. Bowtie2 was used to align short reads (34). SAM files processing was performed by samtools (35). Identification of TSS and subsequent analysis were performed from sam files with BAC-Browser (36) with standard parameters.
Results
Pro-Inflammatory Cytokines Have Little Effect on Growth of B. Longum Subsp. Longum GT15 Strain
To test the effects of pro-inflammatory cytokines on the growth of the bacterial culture, B. longum subsp. longum GT15 was incubated with various concentrations of IL-6 and TNFα while OD600 values were measured at different timepoints. The OD600 measurements under experimental and control conditions formed the growth curves of B. longum subsp. longum GT15 (Figure S1). We showed that the presence of IL-6 [100pg/ml] and TNFα [10 ng/ml] in the growth medium led to moderate changes in the growth rate of the culture (Figure S1). We used those same cultures for the subsequent transcriptome analysis.
Differences in Gene Transcription Profiles
To further understand the mechanism underlying the response of bifidobacteria to inflammation, we applied Illumina-sequencing technology for sequencing the whole transcriptome from three independent biological replicates of B. longum subsp. longum GT15 at the exponential growth phase (Figure 1).
A total of 12,602,595, 11,947,362, and 12,045,570 unique reads were obtained for the samples supplemented with the pro-inflammatory cytokines IL-6 [100pg/mL] and TNFα [10ng/mL] and a reference sample without pro-inflammatory cytokines (control), respectively. After filtering out poor quality reads, the number of effective reads mapped to the genome of B. longum subsp. longum GT15 was reduced to 11,283,525, 10,683,238, and 10,794,398, respectively. Genes that were significantly differentially expressed (based on a fold change of at least two [log 2 ratio <−1 or >1] and a t-test P-value < 0.001) in response to the presence of cytokines were singled out: a total number of 130 DEGs in the sample of B. longum subsp. longum GT15 grown with IL-6 compared to the control sample, including 68 downregulated and 62 upregulated genes and a total number of 1017 DEGs for the sample of B. longum subsp. longum GT15 grown with TNFα compared to the control sample, among which 505 genes were downregulated and 512 genes – upregulated (Figure 2, Tables S1).
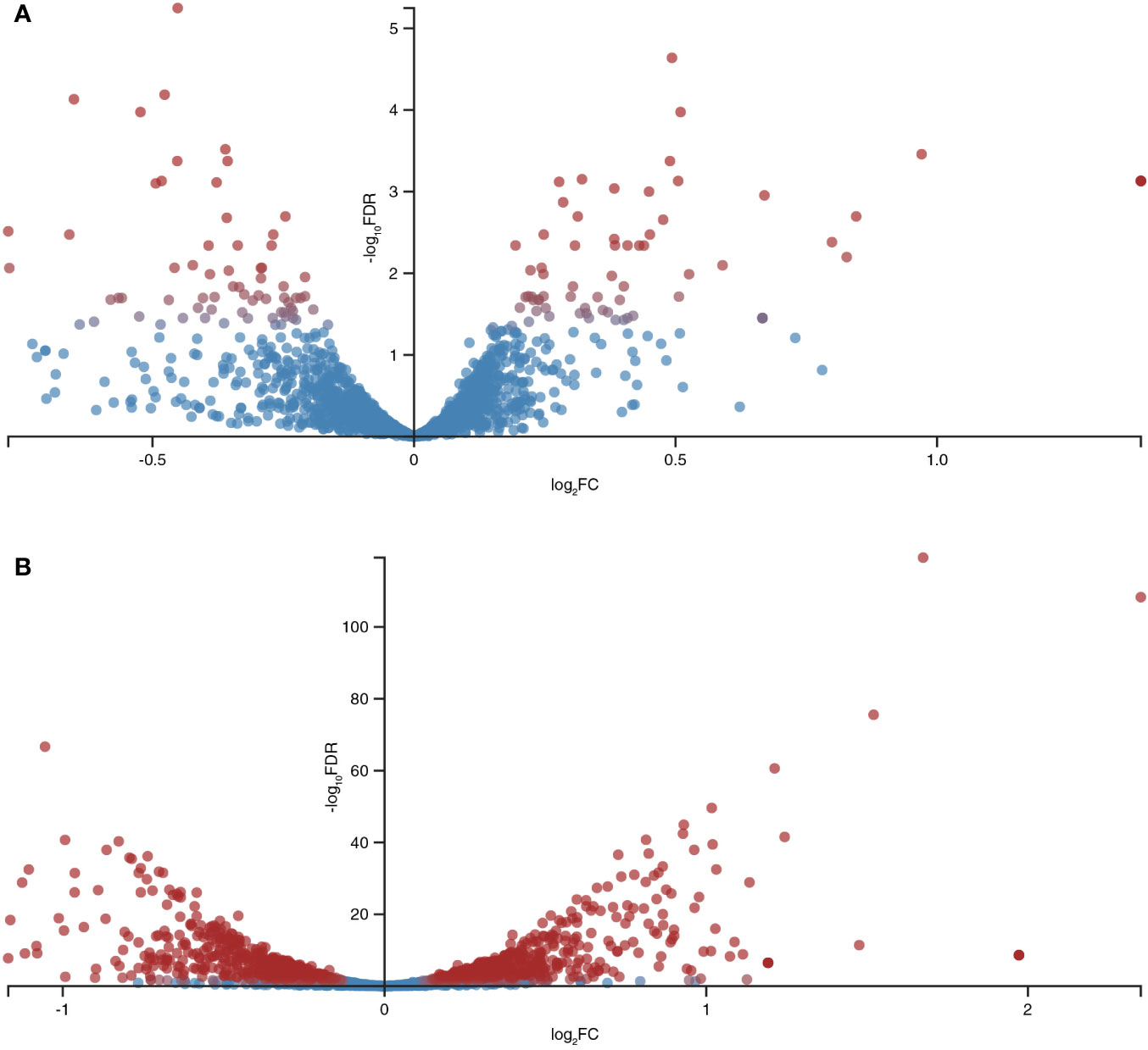
Figure 2 Global differentially expressed genes (DEGs) in B. longum subsp. longum GT15 resulting from (A) exposure to IL-6, (B) exposure to TNFα. Red dots: DEGs; blue dots: not DEGs.
The fold change (FC) in the expression of genes affected by the exposure of B. longum subsp. longum GT15 to IL-6 varied within the range −2>FC<2. In the case of exposure to TNFα, the FC in the expression of 992 genes fell within the range −2>FC<2 and the FC in expression of 25 genes was above 2. The levels of expression of these 25 genes (upregulated and downregulated) are presented in Table 1.
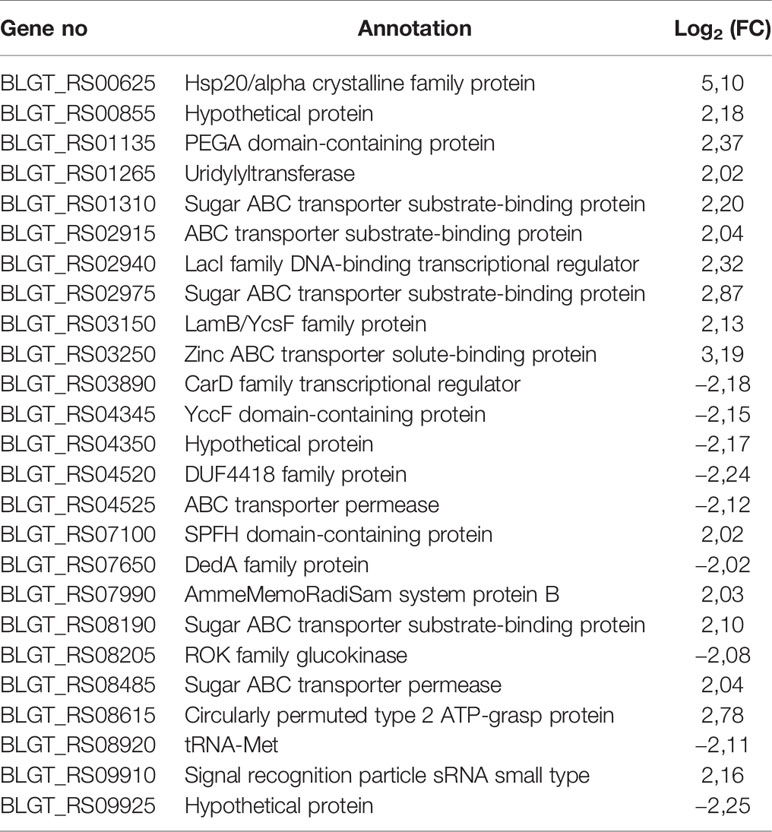
Table 1 Significantly upregulated and downregulated genes (FC≥ 2) upon exposure of B. longum subsp. longum GT15 to TNFα compared to controls by RNAseq.
One of the most differentially expressed 25 genes was BLGT_RS08205, a ROK family glucokinase, encoding the NagC family transcriptional regulator. This gene is involved in many processes: carbohydrate metabolism (glycolysis/gluconeogenesis, galactose metabolism, starch and sucrose metabolism, amino sugar and nucleotide sugar metabolism); biosynthesis of other secondary metabolites (streptomycin biosynthesis, neomycin, kanamycin and gentamicin biosynthesis. Among the remaining genes, seven genes (BLGT_RS08190, BLGT_RS02975, BLGT_RS01310, BLGT_RS08485, BLGT_RS02915, BLGT_RS03250, BLGT_RS04525) encoded transporters involved in signaling and cellular processes, two genes (BLGT_RS03890, BLGT_RS00625) were involved in genetic information processing and the genes BLGT_RS03150, BLGT_RS08190, BLGT_RS01265, BLGT_RS08920 were involved in glutathione metabolism, membrane transport, signal transduction and translation, respectively.
KEGG Pathway Mapping of DEGs
The biologically significant DEGs were further analyzed by Kyoto Encyclopedia of Genes and Genomes (KEGG) pathways. As predicted by KEGG, 34 pathways were altered by IL-6 (Figure 3A, Table S2) and 87 pathways were altered by TNFα (Figure 3B, Table S2). A detailed representation of these pathways is given in Figure 3.
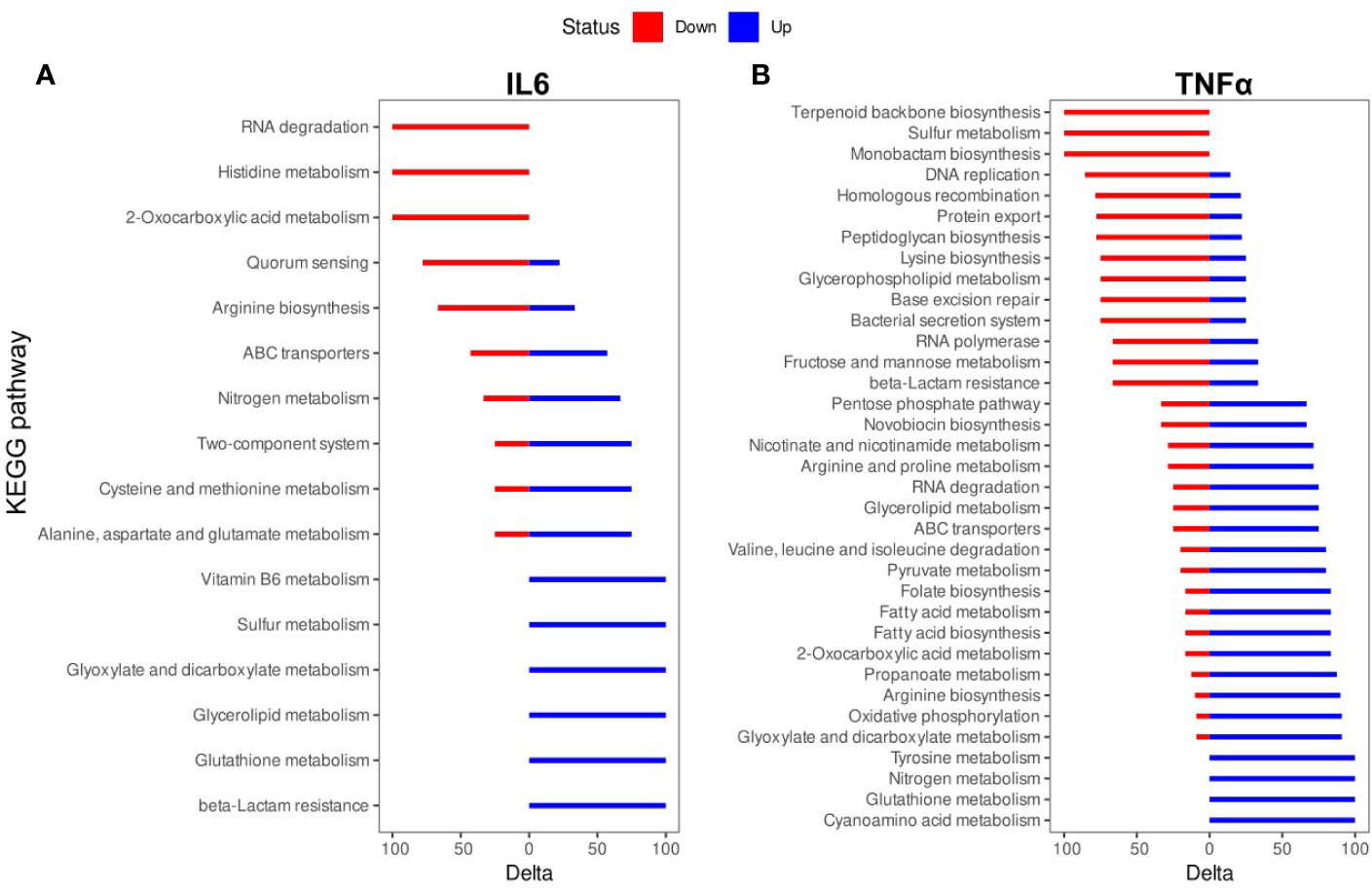
Figure 3 Kyoto Encyclopedia of Genes and Genomes (KEGG) pathway enrichment analysis of differentially expressed genes (DEGs): (A) IL-6 vs. control; (B). TNFα vs. control. The names of KEGG pathways are placed along the Y-axis. The X- axis represents the values of the Delta. Downregulated genes are shown in red color. Upregulated genes are show in blue color.
The TNFα-induced genes were involved in cyanoamino acid metabolism (3 (overexpressed genes)/4 (genes in the pathway)), glutathione metabolism (4/7), nitrogen metabolism (4/6), tyrosine metabolism (3/4), glyoxylate and dicarboxylate metabolism (10/14), oxidative phosphorylation (10/12), arginine biosynthesis (9/12), propanoate metabolism (7/12), 2-oxocarboxylic acid metabolism (10/21), fatty acid biosynthesis (5/7), fatty acid metabolism (5/7), folate biosynthesis (5/7), pyruvate metabolism (8/15), valine, leucine and isoleucine degradation (4/6), ABC transporters (21/54), glycerolipid metabolism (3/7), RNA degradation (6/10), arginine and proline metabolism (5/10), nicotinate and nicotinamide metabolism (5/13), novobiocin biosynthesis (2/4), pentose phosphate pathway (6/17). The TNFα-suppressed genes were involved in beta-Lactam resistance (4/7), fructose and mannose metabolism (2/4), RNA polymerase (2/4), bacterial secretion system (6/9), base excision repair (3/7), glycerophospholipid metabolism (3/6), lysine biosynthesis (6/11), peptidoglycan biosynthesis (7/17), protein export (7/12), DNA replication (6/13), homologous recombination (11/22), monobactam biosynthesis (3/4), sulfur metabolism (2/2), terpenoid backbone biosynthesis (5/9) (Figure 3B).
Functional Annotation of DEGs
The differentially expressed genes were grouped into functional categories according to the Clusters of Orthologous Groups (COG) classification system. COG categories H (coenzyme metabolism), E (amino acid transport and metabolism), G (carbohydrate transport and metabolism), C (energy production and conversion), O (posttranslational modification, protein turnover, chaperones) had a high number of upregulated genes after TNFα treatment compared to controls (Figure 4) whereas F (nucleotide transport and metabolism), U (intracellular trafficking, secretion, and vesicular transport), M (cell wall/membrane/envelope biogenesis), T (signal transduction mechanisms) L (replication, recombination and repair), K (transcription) J (translation, ribosomal structure and biogenesis) had a high number of downregulated genes after TNFα treatment compared to controls (Figure 4).
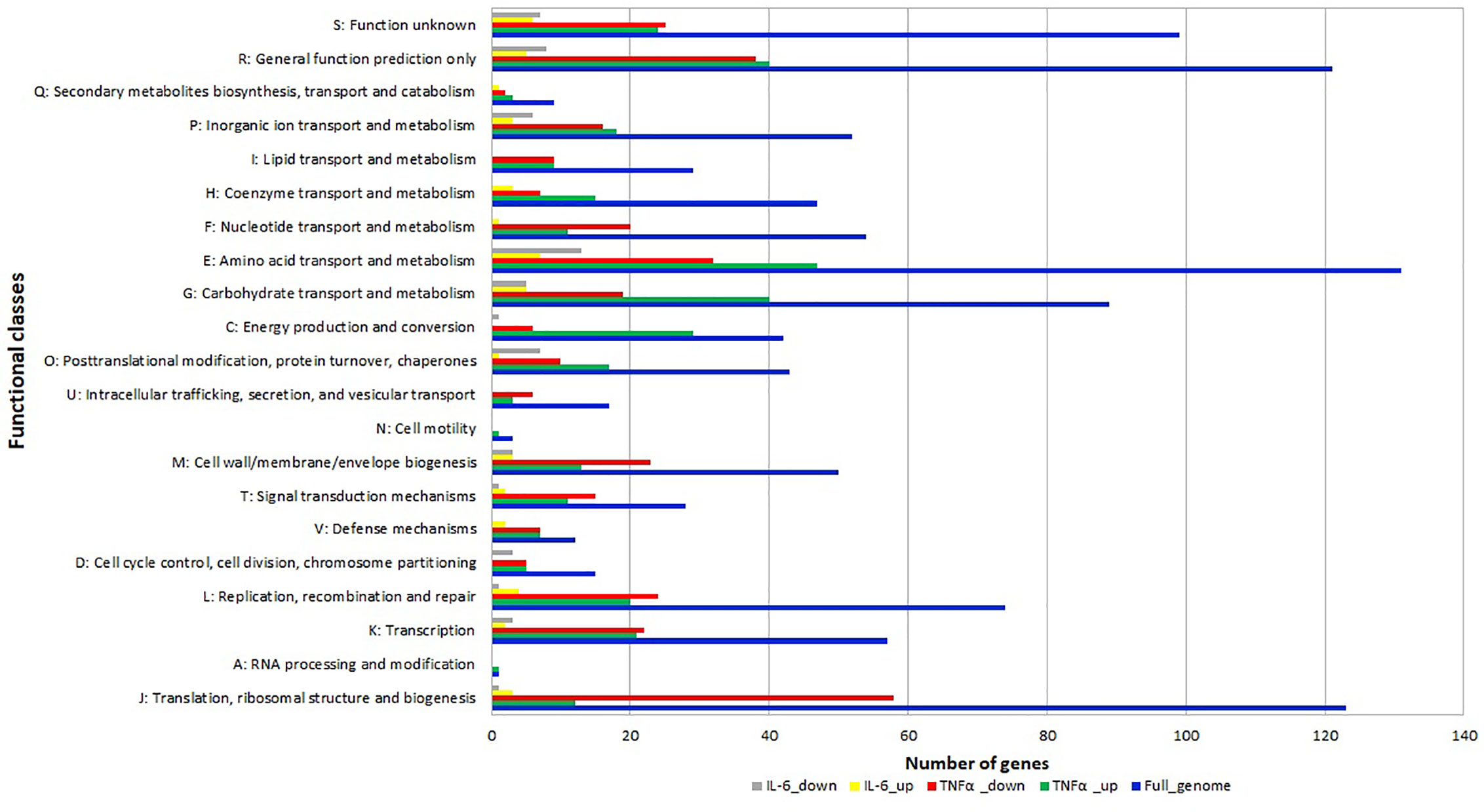
Figure 4 Relative abundance of transcripts grouped into Clusters of Orthologous Groups (COG) functional categories. Functional classification of genes with statistically significant increase (green bar for TNFα and yellow bar for IL-6) or decrease (red bar for TNFα and gray bar for IL-6) in mRNA level compared to controls.
In addition, many genes in categories O (posttranslational modification, protein turnover, chaperones) and E (amino acid transport and metabolism) were also downregulated upon exposure to IL-6.
Evolutionarily Stable Groups of Genes and Transcriptional Organization of DEGs
We used phylogenetic profiling to identify putative operons among the DEGs. We benefited from the open-source RefSeq database to analyze 130 genome sequences belonging to different Bifidobacterium species (Table S3). A phylogenetic profile (PP) is a binary vector describing the presence or absence of the coding sequence of a protein in a set of genomes of a group of organisms (37). It has been proposed that genes involved in the same biological pathway, especially genes in operons, are subjected to the same gain and loss evolutionary events. Therefore, genes with similar PPs are potential functional partners.
Pairs of genes having similar PPs (Jaccard distance less than 0.001), located on the same strand within 10,000 nucleotides, and having the same sign of differential (negative or positive) expression, were grouped together. Such groups were labeled as potential operons (Table S4, Figure 5).
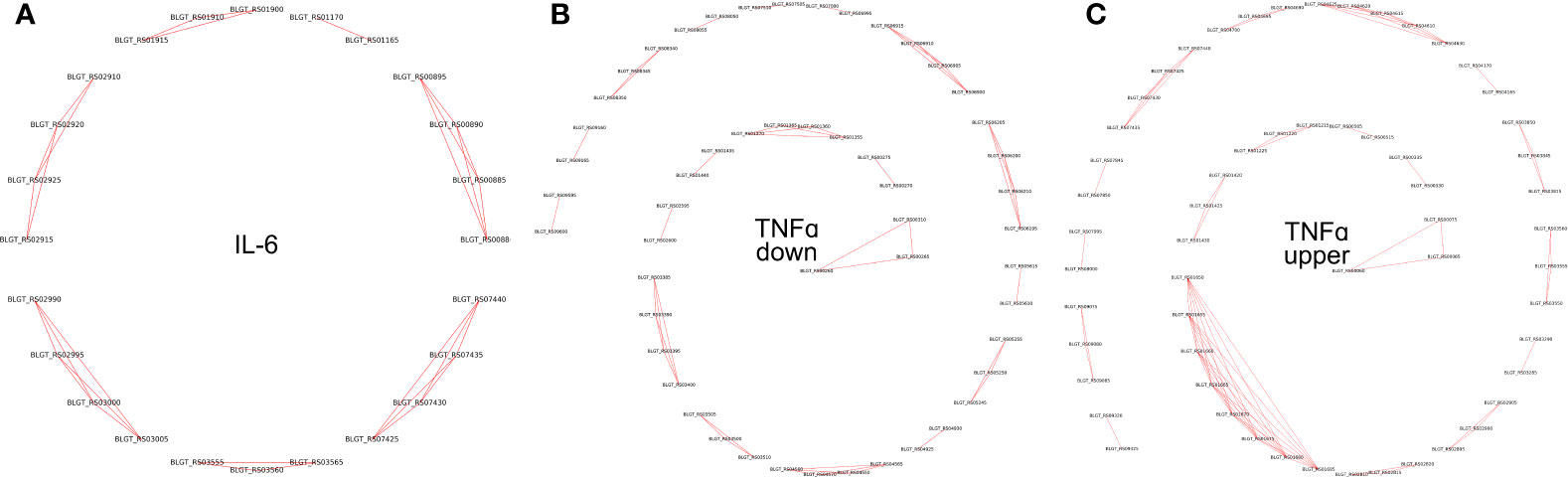
Figure 5 Operons of genes whose expression depends on the presence of (A) IL-6 and (B) TNFα downregulated (C) TNFα upregulated cytokines in the medium.
To validate the predicted operons involved in the response to the pro-inflammatory cytokines IL-6 and TNFα in B. longum subsp. longum GT15, we carried out de novo identifications of transcriptional start sites (TSS). To carry out this task, we prepared RNA libraries enriched with 5’-fragments of mRNA and sequenced them using the high-throughput Illumina technology. The TSS were mapped with single-nucleotide resolution. The search for potential TSSs yielded total of 410 in B. longum subsp. longum GT15. Out of those, 38 TSSs belonged to operons of DEGs after exposure to TNFα, and 7 TSSs belonged to operons regulating DEGs resulting from exposure to IL-6 (Table S3, Figure 5).
Thus, we predicted and then experimentally validated the operons of genes whose expression was affected by the cytokines TNFα and IL-6 (Figure 5).
Discussion
As the analysis of differentially expressed genes showed, TNFα altered the expression of a higher number of genes than IL-6. This may be due to the difference in the concentrations of used cytokines. According to earlier studies, the response of bacteria to treatment with cytokines may become dose-dependent after reaching a certain concentration (38). It is also possible that B. longum subsp. longum GT15 reacts differently to those two types of cytokines. Since cytokines are small peptide signaling molecules, the cytokine-mediated response of bacterial cells appears to be the result of interaction with receptor-like structures. Bacterial sensors enabling the recognition of immune signals have been described for pathogenic microorganisms (8). Moreover, we previously confirmed the ability of FNIII domains of this protein to specifically bind to cytokine TNFα but not IL-6 (27). Different cytokines, acting as ligands to specific receptors, could activate in bacterial cells different signaling pathways consisting of a different number of genes.
The results obtained in the in vitro experiment are subject to two different interpretations. One possible interpretation is that the response of B. longum subsp. longum GT15 to the pro-inflammatory cytokines is an adaptive reaction to stress aimed at maintaining normal functioning. Another interpretation is that the response of B. longum subsp. longum GT15 is intended to quench inflammation in the host organism, particularly in the gut. The cytokine-triggered release of mediators such as short-chain fatty acids, antioxidants, and other molecules that regulate the level of pro-inflammatory cytokines, supports well this hypothesis.
Nagasawa’s and Yu’s study highlighted the important role of hsp20 in reducing inflammation. The heat shock protein 20 (HSP20/HSPB6) belongs to the HSP family (HSPB) of small proteins with monomeric molecular masses ranging from 15 to 30 kDa. Overexpression of hsp20 leads to a marked decrease in TNF-α expression (39, 40). The transcription of the gene encoding hsp20, BLGT_RS00625, was upregulated 5-fold in the B. longum subsp. longum GT15 strain exposed to TNFα. Since the response of B. longum subsp. longum GT15 could be directed at bringing down TNFα levels, we suspect this mechanism to be one of the many ways used by bifidobacteria to dampen inflammation.
The transcription of ABC transporters (BLGT_RS02915, BLGT_RS02975, BLGT_RS03250, BLGT_RS04525, BLGT_RS08190, BLGT_RS08485) showed over two-fold upregulation. This can be explained by the cell’s struggle to maintain homeostasis during inflammation. An increase in the expression of ABC transporters, which facilitates the influx of sugars, leads to their accumulation in the cell followed by increased synthesis of ATP. In addition, a gene encoding Zinc ABC transporter (BLGT_RS03250) was 3,19-fold upregulated. Effective zinc absorption is crucial to an adequate response of the host’s immune system. One strategy adopted by the immune system is to decrease the concentration of zinc, iron and manganese (41–43). Therefore, TNF-α can stimulate the sequestration of zinc by bifidobacteria while it is still available.
The DedA family protein encoded by BLGT_RS07650 was also downregulated (Table 1). This protein has been shown to increase sensitivity of bifidobacteria to temperature and pH (44, 45). Hypersensitivity to temperatures can account for the increased expression of BLGT_RS00625.
Glutathione carries out many functions in biological systems among which are antioxidant activity, immune stimulation and cellular detoxification. Glutathione helps maintain the intracellular redox homeostasis, which ensures the protection of cells against oxidative damage. Most of the biological functions of glutathione depend on the conversion of reduced glutathione (GSH) to its oxidized form by the enzyme glutathione peroxidase and its transformation back to GSH by glutathione reductase. Glutathione, a potent reducing agent in the cell cytoplasm, is crucial for imparting antioxidant properties to bacteria. Glutathione defends cells against oxidative radicals, which pose a serious threat to the survival and performance of bacterial cells. Thus, both GSH synthesis and buildup in the cell could be correlated with the antioxidant potential of bacteria and their ability to grow in an aerobic environment, although other factors are also involved (46). The protective mechanism of GSH is suggested to be either its sacrificial action which prevents the rapid fall of intracellular pH or glutathionylation of the enzyme glyceraldehyde 3-phosphate dehydrogenase (GAPDH) which helps keeping the glycolysis rate under control. Epithelial cells require a continuous supply of GSH for proper functioning. It has been shown that exogenously supplied GSH not only maintains the necessary level in the intestine, but also provides protection against oxidizing agents such as tert-butyl hydroperoxide or menadione (47).
Ammonia has often been used as a marker of colonic bacterial protein metabolism. It is generally considered a potentially toxic metabolite causing direct damage to colonocytes. Nevertheless, ammonia is the main source of nitrogen for bacteria (48). Therefore, exposure to TNFα can signal to bifidobacteria an increase in the concentration of ammonia leading to increased expression of genes, involved in nitrogen metabolism. Similarly, the expression of genes involved in the metabolism of amino acids such as valine, leucine, arginine and proline could rise. The increased expression of genes involved in fatty acid metabolism could reflect the demand for acquiring resources for cell wall reparation during inflammation.
Recently, fecal metabolome analysis of 987 samples revealed altered pathways related to amino acid metabolism (e.g. aminoacyl-tRNA biosynthesis pathway, arginine biosynthesis pathway, and valine, leucine and isoleucine biosynthesis pathway), which correlated with changes in the core microbiome and inflammation (49, 50). Therefore, the changes in the expression of genes involved in biosynthesis and degradation of amino acids, in particular arginine, valine, leucine, and isoleucine, require a closer look in a future study.
Exposure to TNFα has also stimulated the expression of genes involved in propanoate metabolism. Propionate is potentially one of the main metabolites accounting for the probiotic action of bifidobacteria. Previously, propionate was shown to reduce inflammation by lowering blood pressure at the site of inflammation (51).
According to functional analysis, exposure to TNFα altered mainly the expression of genes included in energy pathways. This may be explained by the accumulation of resources caused by inflammation and activation of ATP-dependent ABC transporters to maintain homeostasis. Overall, IL-6 did not affect the expression of functional groups.
The observed effects of cytokines on B. longum subsp. longum GT15 were cumulative and prolonged, features that are conducive for the adaptation to the new conditions.
Data Availability Statement
The datasets presented in this study can be found in online repositories. The names of the repository/repositories and accession number(s) can be found in the article/Supplementary Material.
Author Contributions
VV, KK, and MD designed the experiments, performed the experiments, analyzed the samples, and contributed to the manuscript preparation. ES and DB analyzed the transcriptomic data. EM and AK analyzed the transcriptomic data and found COG. EO and KK contributed to KEGG pathway analysis and figures constructed. PP and MD made the phylogenetic profiling. VV and TS contributed to the transcriptional organization of DEGs. EI and VD contributed to the manuscript preparation. All authors contributed to the article and approved the submitted version.
Funding
This research is supported by the Russian Science Foundation grant № 19-74-00146.
Conflict of Interest
The authors declare that the research was conducted in the absence of any commercial or financial relationships that could be construed as a potential conflict of interest.
Acknowledgments
We thank the Center for Precision Genome Editing and Genetic Technologies for Biomedicine, Federal Research and Clinical Center of Physical-Chemical Medicine of Federal Medical Biological Agency for the opportunity to use computational and sequencing resources.
Supplementary Material
The Supplementary Material for this article can be found online at: https://www.frontiersin.org/articles/10.3389/fimmu.2020.595877/full#supplementary-material
Supplementary Figure 1 | B. longum subsp. longum GT15 growth curves upon exposure to pro-inflammatory cytokines IL-6 and TNFα compared to cultivation without the cytokines.
Supplementary Table 1 | The lists of DEGs.
Supplementary Table 2 | KEGG pathway mapping of DEGs.
Supplementary Table 3 | Species IDs.
Supplementary Table 4 | Predicted operons.
References
1. O’Callaghan A, van Sinderen D. Bifidobacteria and their role as members of the human gut microbiota. Front Microbiol (2016) 7:925. doi: 10.3389/fmicb.2016.00925
2. Ruiz L, Delgado S, Ruas-Madiedo P, Sánchez B, Margolles A. Bifidobacteria and their molecular communication with the immune system. Front Microbiol (2017) 8:2345. doi: 10.3389/fmicb.2017.02345
3. Adlerberth I, Strachan DP, Matricardi PM, Ahrné S, Orfei L, Åberg N, et al. Gut microbiota and development of atopic eczema in 3 European birth cohorts. J Allergy Clin Immunol (2007) 120:343–50. doi: 10.1016/j.jaci.2007.05.018
4. Kalliomäki M, Kirjavainen P, Eerola E, Kero P, Salminen S, Isolauri E. Distinct patterns of neonatal gut microflora in infants in whom atopy was and was not developing. J Allergy Clin Immunol (2001) 107:129–34. doi: 10.1067/mai.2001.111237
5. Fujimura KE, Sitarik AR, Havstad S, Lin DL, Levan S, Fadrosh D, et al. Neonatal gut microbiota associates with childhood multisensitized atopy and T cell differentiation. Nat Med (2016) 22:1187–91. doi: 10.1038/nm.4176
6. Averina OV, Ermolenko EI, Ratushniy AY, Tarasova EA, Borschev YY, Leontieva GF, et al. Influence of probiotics on cytokine production in the in Vitro and in Vivo Systems. Med Immunol (2015) 17:443–54. doi: 10.15789/1563-0625-2015-5-443-454
7. Cullen TW, Schofield WB, Barry NA, Putnam EE, Rundell EA, Trent MS, et al. Antimicrobial peptide resistance mediates resilience of prominent gut commensals during inflammation. Sci (80- ) (2015) 347:170–5. doi: 10.1126/science.1260580
8. Lesouhaitier O, Veron W, Chapalain A, Madi A, Blier AS, Dagorn A, et al. Gram-negative bacterial sensors for eukaryotic signal molecules. Sensors (2009) 9:6967–90. doi: 10.3390/s90906967
9. Porat R, Clark BD, Wolff SM, Dinarello CA. Enhancement of growth of virulent strains of Escherichia coli by interleukin-1. Sci (80- ) (1991) 254:430–2. doi: 10.1126/science.1833820
10. Denis M, Campbell D, Gregg EO. Interleukin-2 and granulocyte-macrophage colony-stimulating factor stimulate growth of a virulent strain of Escherichia coli. Infect Immun (1991) 59:1853–6. doi: 10.1128/iai.59.5.1853-1856.1991
11. Umberto Meduri G, Kanangat S, Stefan J, Tolley E, Schaberg D. Cytokines IL-1β, IL-6, and TNF-α enhance in vitro growth of bacteria. Am J Respir Crit Care Med (1999) 160:961–7. doi: 10.1164/ajrccm.160.3.9807080
12. Hogan JS, Todhunter DA, Smith KL, Schoenberger PS, Sordillo LM. Growth Responses of Coliform Bacteria to Recombinant Bovine Cytokines. J Dairy Sci (1993) 76:978–82. doi: 10.3168/jds.S0022-0302(93)77425-1
13. Luo G, Niesel DW, Shaban RA, Grimm EA, Klimpel GR. Tumor necrosis factor alpha binding to bacteria: Evidence for a high- affinity receptor and alteration of bacterial virulence properties. Infect Immun (1993) 61:830–5. doi: 10.1128/iai.61.3.830-835.1993
14. Croucher NJ, Thomson NR. Studying bacterial transcriptomes using RNA-seq. Curr Opin Microbiol (2010) 13:619–24. doi: 10.1016/j.mib.2010.09.009
15. Zuo F, Yu R, Xiao M, Khaskheli GB, Sun X, Ma H, et al. Transcriptomic analysis of Bifidobacterium longum subsp. longum BBMN68 in response to oxidative shock. Sci Rep (2018) 8:1–12. doi: 10.1038/s41598-018-35286-7
16. Bottacini F, Zomer A, Milani C, Ferrario C, Lugli GA, Egan M, et al. Global transcriptional landscape and promoter mapping of the gut commensal Bifidobacterium breve UCC2003. BMC Genomics (2017) 18:991. doi: 10.1186/s12864-017-4387-x
17. Morovic W, Roos P, Zabel B, Hidalgo-Cantabrana C, Kiefer A, Barrangou R. Transcriptional and functional analysis of Bifidobacterium animalis subsp. lactis exposure to tetracycline. Appl Environ Microbiol (2018) 84:e01999–18. doi: 10.1128/AEM.01999-18
18. Yang J, Tang Q, Xu L, Li Z, Ma Y, Yao D. Combining of transcriptome and metabolome analyses for understanding the utilization and metabolic pathways of Xylo-oligosaccharide in Bifidobacterium adolescentis ATCC 15703. Food Sci Nutr (2019) 7:3480–93. doi: 10.1002/fsn3.1194
19. Senizza A, Callegari ML, Senizza B, Minuti A, Rocchetti G, Morelli L, et al. Effects of linoleic acid on gut-derived Bifidobacterium breve DSM 20213: A transcriptomic approach. Microorganisms (2019) 7:710. doi: 10.3390/microorganisms7120710
20. Wei Y, Gao J, Liu D, Li Y, Liu W. Adaptational changes in physiological and transcriptional responses of Bifidobacterium longum involved in acid stress resistance after successive batch cultures. Microb Cell Fact (2019) 18:156. doi: 10.1186/s12934-019-1206-x
21. Oberg TS, Ward RE, Steele JL, Broadbent JR. Transcriptome analysis of Bifidobacterium longum strains that show a differential response to hydrogen peroxide stress. J Biotechnol (2015) 212:58–64. doi: 10.1016/j.jbiotec.2015.06.405
22. Turroni F, Peano C, Pass DA, Foroni E, Severgnini M, Claesson MJ, et al. Diversity of bifidobacteria within the infant gut microbiota. PloS One (2012) 7:e36957. doi: 10.1371/journal.pone.0036957
23. Turroni F, Foroni E, Pizzetti P, Giubellini V, Ribbera A, Merusi P, et al. Exploring the diversity of the bifidobacterial population in the human intestinal tract. Appl Environ Microbiol (2009) 75:1534–45. doi: 10.1128/AEM.02216-08
24. Zakharevich NV, Averina OV, Klimina KM, Kudryavtseva AV, Kasianov AS, Makeev VJ, et al. Complete Genome Sequence of Bifidobacterium longum GT15: Identification and Characterization of Unique and Global Regulatory Genes. Microb Ecol (2015) 70:819–34. doi: 10.1007/s00248-015-0603-x
25. Nezametdinova VZ, Mavletova DA, Alekseeva MG, Chekalina MS, Zakharevich NV, Danilenko VN. Species-specific serine-threonine protein kinase Pkb2 of Bifidobacterium longum subsp. longum: Genetic environment and substrate specificity. Anaerobe (2018) 51:26–35. doi: 10.1016/j.anaerobe.2018.03.003
26. Nimo Bravo J, Staunton D, Heath JK, Jones EY. Crystal structure of a cytokine-binding region of gp130. EMBO (1998) 17:1665–74. doi: 10.2210/pdb1bqu/pdb
27. Ilya D, Mavletova D, Chernyshova I, Snegireva N, Gavrilova M, Bushkova K, et al. FN3 Protein Fragment Containing two type III fibronectin domains from B. longum subsp. longum GT15 Binds to Human Tumor Necrosis Factor Alpha in vitro. Anaerobe (2020) 65:102247. doi: 10.1016/j.anaerobe.2020.102247
28. Zakharevich NV, Averina OV, Klimina KM, Kudryavtseva AV, Kasianov AS, Makeev VJ, et al. Complete genome sequence of Bifidobacterium longum GT15: Unique genes for Russian strains. Genome Announc (2014) 2:1348–62. doi: 10.1128/genomeA.01348-14
29. Bespyatykh J, Shitikov E, Bespiatykh D, Guliaev A, Klimina K, Veselovsky V, et al. Metabolic Changes of Mycobacterium tuberculosis during the Anti-Tuberculosis Therapy. Pathogens (2020) 9:131. doi: 10.3390/pathogens9020131
30. Quinlan AR, Hall IM. BEDTools: a flexible suite of utilities for comparing genomic features. Bioinformatics (2010) 26:841–2. doi: 10.1093/bioinformatics/btq033
31. Team, R. Core. R: A language and environment for statistical computing. Vienna, Austria: R Foundation for Statistical Computing (2013).
32. Emms DM, Kelly S. OrthoFinder: solving fundamental biases in whole genome comparisons dramatically improves orthogroup inference accuracy. Genome Biol (2015) 16:157. doi: 10.1186/s13059-015-0721-2
33. Mazin PV, Fisunov GY, Gorbachev AY, Kapitskaya KY, Altukhov IA, Semashko TA, et al. Transcriptome analysis reveals novel regulatory mechanisms in a genome-reduced bacterium. Nucleic Acids Res (2014) 42:13254–68. doi: 10.1093/nar/gku976
34. Langmead B, Salzberg SL. Fast gapped-read alignment with Bowtie 2. Nat Methods (2012) 9:357–9. doi: 10.1038/nmeth.1923
35. Li H, Handsaker B, Wysoker A, Fennell T, Ruan J, Homer N, et al. The Sequence Alignment/Map format and SAMtools. Bioinformatics (2009) 25:2078–9. doi: 10.1093/bioinformatics/btp352
36. Garanina IA, Fisunov GY, Govorun VM. BAC-BROWSER: The Tool for Visualization and Analysis of Prokaryotic Genomes. Front Microbiol (2018) 9:2827. doi: 10.3389/fmicb.2018.02827
37. Pellegrini M, Marcotte EM, Thompson MJ, Eisenberg D, Yeates TO. Assigning protein functions by comparative genome analysis: Protein phylogenetic profiles. Proc Natl Acad Sci USA (1999) 96:4285–8. doi: 10.1073/pnas.96.8.4285
38. Meduri GU. Clinical review: A paradigm shift: The bidirectional effect of inflammation on bacterial growth. Clinical implications for patients with acute respiratory distress syndrome. Crit Care (2002) 6:24–9. doi: 10.1186/cc1450
39. Nagasawa T, Matsushima-Nishiwaki R, Yasuda E, Matsuura J, Toyoda H, Kaneoka Y, et al. Heat shock protein 20 (HSPB6) regulates TNF-αinduced intracellular signaling pathway in human hepatocellular carcinoma cells. Arch Biochem Biophys (2015) 565:1–8. doi: 10.1016/j.abb.2014.10.010
40. Yu DW, Ge PP, Liu AL, Yu XY, Liu TT. HSP20-mediated cardiomyocyte exosomes improve cardiac function in mice with myocardial infarction by activating Akt signaling pathway. Eur Rev Med Pharmacol Sci (2019) 23:4873–81. doi: 10.26355/eurrev_201906_18075
41. Neumann W, Gulati A, Nolan EM. Metal homeostasis in infectious disease: recent advances in bacterial metallophores and the human metal-withholding response. Curr Opin Chem Biol (2017) 37:10–8. doi: 10.1016/j.cbpa.2016.09.012
42. Liu JZ, Jellbauer S, Poe AJ, Ton V, Pesciaroli M, Kehl-Fie TE, et al. Zinc sequestration by the neutrophil protein calprotectin enhances salmonella growth in the inflamed gut. Cell Host Microbe (2012) 11:227–39. doi: 10.1016/j.chom.2012.01.017
43. Kehl-Fie TE, Skaar EP. Nutritional immunity beyond iron: a role for manganese and zinc. Curr Opin Chem Biol (2010) 14:218–24. doi: 10.1016/j.cbpa.2009.11.008
44. Thompkins K, Chattopadhyay B, Xiao Y, Henk MC, Doerrler WT. Temperature sensitivity and cell division defects in an Escherichia coli strain with mutations in yghB and yqjA, encoding related and conserved inner membrane proteins. J Bacteriol (2008) 190:4489–500. doi: 10.1128/JB.00414-08
45. Krulwich TA, Sachs G, Padan E. Molecular aspects of bacterial pH sensing and homeostasis. Nat Rev Microbiol (2011) 9:330–43. doi: 10.1038/nrmicro2549
46. Yoon YH, Byun JR. Occurrence of glutathione sulphydryl (GSH) and antioxidant activities in probiotic Lactobacillus spp. Asian-Australasian J Anim Sci (2004) 17:1582–5. doi: 10.5713/ajas.2004.1582
47. Pophaly SD, Singh R, Pophaly SD, Kaushik JK, Tomar SK. Current status and emerging role of glutathione in food grade lactic acid bacteria. Microb Cell Fact (2012) 11:114. doi: 10.1186/1475-2859-11-114
48. Metges CC. Contribution of Microbial Amino Acids to Amino Acid Homeostasis of the Host. J Nutr (2000) 130:1857S–64S. doi: 10.1093/jn/130.7.1857S
49. Gou W, Fu Y, Yue L, Chen G, Cai X, Shuai M, et al. Gut microbiota may underlie the predisposition of healthy individuals to COVID-19. medRXiv (2020) 2020.2004.2022.20076091. doi: 10.1101/2020.04.22.20076091
50. Catanzaro M, Fagiani F, Racchi M, Corsini E, Govoni S, Lanni C. Immune response in COVID-19: addressing a pharmacological challenge by targeting pathways triggered by SARS-CoV-2. Signal Transduct Target Ther (2020) 5:1–10. doi: 10.1038/s41392-020-0191-1
Keywords: Bifidobacterium longum, RNA sequencing, transcriptome, pro-inflammatory cytokines, inflammatory process, transcription start site
Citation: Veselovsky VA, Dyachkova MS, Menyaylo EA, Polyaeva PS, Olekhnovich EI, Shitikov EA, Bespiatykh DA, Semashko TA, Kasianov AS, Ilina EN, Danilenko VN and Klimina KM (2020) Gene Networks Underlying the Resistance of Bifidobacterium longum to Inflammatory Factors. Front. Immunol. 11:595877. doi: 10.3389/fimmu.2020.595877
Received: 18 August 2020; Accepted: 20 October 2020;
Published: 16 November 2020.
Edited by:
Marina De Bernard, University of Padua, ItalyReviewed by:
Mary Catherine O’Connell Motherway, University College Cork, IrelandJonathan Hansen, University of North Carolina at Chapel Hill, United States
Copyright © 2020 Veselovsky, Dyachkova, Menyaylo, Polyaeva, Olekhnovich, Shitikov, Bespiatykh, Semashko, Kasianov, Ilina, Danilenko and Klimina. This is an open-access article distributed under the terms of the Creative Commons Attribution License (CC BY). The use, distribution or reproduction in other forums is permitted, provided the original author(s) and the copyright owner(s) are credited and that the original publication in this journal is cited, in accordance with accepted academic practice. No use, distribution or reproduction is permitted which does not comply with these terms.
*Correspondence: Ksenia M. Klimina, ppp843@yandex.ru
†These authors have contributed equally to this work