- 1Department of Clinical and Experimental Medicine, University of Florence, Florence, Italy
- 2Department of Biomedical, Experimental and Clinical Sciences “Mario Serio” University of Florence, Florence, Italy
- 3Department of Statistics, Computer Science, Applications “G. Parenti”, Florence, Italy
- 4Department of Biology, University of Florence, Florence, Italy
- 5SOD of Interdisciplinary Internal Medicine, Azienda Ospedaliera Universitaria Careggi (AOUC), Florence, Italy
Background and aim: Gut microbiota (GM) can support colorectal cancer (CRC) progression by modulating immune responses through the production of both immunostimulatory and/or immunosuppressive cytokines. The role of IL-9 is paradigmatic because it can either promote tumor progression in hematological malignancies or inhibit tumorigenesis in solid cancers. Therefore, we investigate the microbiota–immunity axis in healthy and tumor mucosa, focusing on the correlation between cytokine profile and GM signature.
Methods: In this observational study, we collected tumor (CRC) and healthy (CRC-S) mucosa samples from 45 CRC patients, who were undergoing surgery in 2018 at the Careggi University Hospital (Florence, Italy). First, we characterized the tissue infiltrating lymphocyte subset profile and the GM composition. Subsequently, we evaluated the CRC and CRC-S molecular inflammatory response and correlated this profile with GM composition, using Dirichlet multinomial regression.
Results: CRC samples displayed higher percentages of Th17, Th2, and Tregs. Moreover, CRC tissues showed significantly higher levels of MIP-1α, IL-1α, IL-1β, IL-2, IP-10, IL-6, IL-8, IL-17A, IFN-γ, TNF-α, MCP-1, P-selectin, and IL-9. Compared to CRC-S, CRC samples also showed significantly higher levels of the following genera: Fusobacteria, Proteobacteria, Fusobacterium, Ruminococcus2, and Ruminococcus. Finally, the abundance of Prevotella spp. in CRC samples negatively correlated with IL-17A and positively with IL-9. On the contrary, Bacteroides spp. presence negatively correlated with IL-9.
Conclusions: Our data consolidate antitumor immunity impairment and the presence of a distinct microbiota profile in the tumor microenvironment compared with the healthy mucosa counterpart. Relating the CRC cytokine profile with GM composition, we confirm the presence of bidirectional crosstalk between the immune response and the host’s commensal microorganisms. Indeed, we document, for the first time, that Prevotella spp. and Bacteroides spp. are, respectively, positively and negatively correlated with IL-9, whose role in CRC development is still under debate.
Introduction
Colorectal cancer (CRC) is a complex and widespread disease and is the second cause of cancer-related deaths in the world (1). Usually, it begins as benign polyps that can become (especially the adenomatous type) cancerous if not removed: in humans, a variable percentage of polyps, ranging from 1% to 10%, evolve into malignancies (2) because multistep colorectal tumorigenesis does not involve exclusively genetic factors, but also host factors, such as inflammatory and immune responses (3, 4). Indeed, chronic inflammation increases cancer risk through a deregulated activation of the immune system, which causes a loss of tissue architecture and genotoxic cellular DNA damage (5). In this context, CRC is considered the best example of a chronic inflammation–associated tumor, occurring often in patients with inflammatory bowel disease (IBD): IBD-associated CRC is estimated to be 2% of all CRCs, and the rate of death resulting from CRC in IBD patients ranges from 10% to 15% (6, 7). Moreover, according to the immunoediting theory, the adaptive immune system, in addition to protecting the host from developing tumors (8), can support tumor progression. Specifically, T cells can develop different functional features during cancer growth, affecting the disease progression and/or regression. The protective immunity is mediated by effector cells (Th1 and Th17/Th1), and “not effector” T lymphocyte subsets (Th2, Tregs, Tnull) can promote colon cancer progression (9–11). In this scenario, the microbiota plays an important role as well because it is essential to modulate immune responses favoring the equilibrium between protective immunity and tolerance to commensal bacteria (12). A perturbation of the gut microbiota (GM) composition can disrupt this balanced ecosystem, determining a chronic/abnormal immune activation and supporting tumor growth. In fact, over the past 10 years, both specific bacteria and dysbiotic conditions have been associated with or implicated in colorectal carcinogenesis (13, 14) and, in some cases, through the interaction with the immune system (15). In particular, the role of Fusobacterium nucleatum is paradigmatic because it promotes CRC by either the induction of epithelial cell proliferation (16), thus generating a proinflammatory microenvironment propitious to cancer progression (17), or via the production of proteins able to block the cytotoxic antitumoral activity of T and NK cells (18, 19).
Moreover, microbes can affect cancer cell antigenicity and adjuvanticity (20), determining whether an antigen triggers an immune response and if its nature drives the acquisition of a specific T cell phenotype (effector or regulator). In addition, microbiota can elicit the production of cytokines (and other immune mediators) by influencing the immunostimulatory or immunosuppressive reactions, such as the tendency to mount Th1/Tc1 (characterized by IFN-γ production), Th2/Tc2 (IL-4 and IL-13), Th17/Tc17 (IL-17), or Th9 (IL-9) responses (21–23) that play different roles in colon cancer (11, 24). For example, the commensal bacteria can stimulate the lamina propria dendritic cells to produce the IL-6, TGF-β, and IL-23 needed to elicit Th17 and Th9 lymphocyte development (25), and these play a dual role in CRC promotion (5, 26). Current studies show that Th9 cells play a vital antitumor role in most solid tumors (27), but IL-9, as a lymphocyte growth factor, can also promote cancer progression in hematological tumors (23).
Finally, fermentative bacterial products, such as short chain fatty acids, may affect colorectal carcinogenesis by favoring the expression of the Foxp3 gene and boosting Treg functions (28, 29).
Given these premises, our study aims to investigate immune system–microbiota crosstalk in CRC through the cellular and molecular characterization of immunity and the comparative evaluation of microbiota composition in healthy and tumor mucosa, focusing on the correlation between the cytokine profile and GM composition.
Material and Methods
Patient Recruitment
In this observational study, 45 patients affected by nonmetastatic colorectal adenocarcinoma at the preoperative stage for undergoing surgical resection were enrolled in 2018 at the Careggi University Hospital (Florence, Italy) (see Table 1 for a summary of patient characteristics). Exclusion criteria were extraperitoneal rectum localization of the tumor; previous surgery for cancer; previous chemo-radiotherapy treatment; immunodeficiency; travel to exotic areas in the last 5 years; treatment with immunosuppressive drugs, antibiotics, or regular probiotics during the previous 2 months; acute gastrointestinal infections in the month prior to enrollment; and associated presence of established malignancies or chronic intestinal inflammatory diseases (Crohn’s disease and ulcerative recto colitis). Tissue samples of tumor (CRC) and surrounding healthy mucosa (CRC-S) were obtained from the surgical specimen after surgery. The study has received the local ethics committee approval (CE: 11166_spe), and informed written consent has been obtained from each participant.
Immunological Analysis
Analysis of Tissue Infiltrating Lymphocytes (TILs)
Tissue samples were dissociated with the Tumor Dissociation Kit, human (Miltenyi Biotech, UK) in combination with the gentleMACS™ Octo Dissociator (Miltenyi Biotech, GmbH) to obtain a gentle and rapid generation of single-cell suspensions. Then, TILs were magnetically isolated with antihuman CD3 microbeads (Miltenyi Biotech, UK) using the AutoMACS Pro Separator (Miltenyi Biotech, GmbH) and analyzed by polychromatic flow cytometry. In detail, TILs from dissociated tissues were characterized for the expression of CD4, CD8, CD25, CD127, IFN-γ, IL-4, IL-17, IL-9, IL-22, and FoxP3 using intracellular cytokine staining. Briefly, TILs were cultured in RPMI 1640 culture medium (SERO-Med GmbH, Wien) supplemented with 10% FCS HyClone (Gibco Laboratories, Grand Island, NY, USA) and stimulated for 5 h using the Leukocyte Activation Cocktail with BD GolgiPlug™ (BD Pharmingen). Cells were stained for surface antigens and then fixed with 4% (v/v) paraformaldehyde and permeabilized with 0.5% saponin, followed by intracellular staining with anti-IL-4, anti-IL-17, anti-IL-22, anti-IL-9, and anti-IFN-γ mAbs (BD Biosciences). For the detection of peripheral Tregs, TILs were fixed and permeabilized using the BD Pharmingen Human FoxP3 Buffer Set (BD Biosciences). A minimum of 10,000 events were acquired.
Molecular Inflammatory Response Evaluation
In a restricted court of patients (n=14) for whom the tissue was available, we evaluated the tumor and healthy mucosa–associated inflammatory response through the evaluation of 26 cytokines by specifically assembled MixMatch Human kits for Luminex MAGPIX detection system (Affymetrix, eBioscience) and following the manufacturers’ instructions. More specifically, we analyzed macrophage inflammatory protein-1α (MIP-1α), interleukin (IL)-27, IL-1β, IL-2, IL-4, IL-5, interferon gamma-induced protein 10 (IP-10), IL-6, IL-8, IL-10, IL-12p70, IL-13, IL-17A, granulocyte-macrophage colony stimulating factor (GM-CSF), tumor necrosis factor-α (TNF-α), interferon (IFN)-α, IFN-γ, monocyte chemotactic protein 1(MCP-1), IL-9, P-selectin, IL-1α, IL-23, IL-18, IL-21, soluble intercellular adhesion molecule-1 (sICAM-1), and IL-22. The levels of cytokines were estimated using a 5-parameter polynomial curve (ProcartaPlex Analyst 1.0).
The low and upper limit of quantification (LLOQ and ULOQ) used for the cytokines and chemokines are reported in Table 2. A value under the LLOQ was considered to be 0 pg/ml.
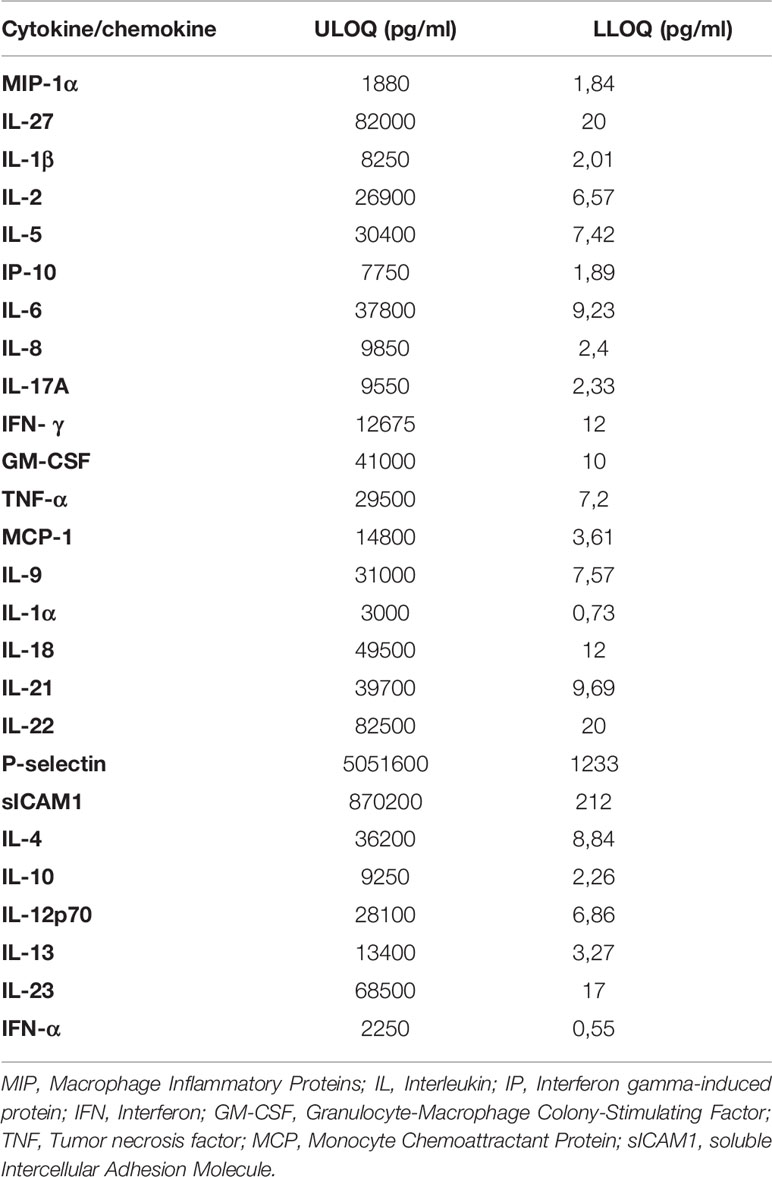
Table 2 Low and Upper Limit of Quantification (LLOQ and ULOQ) for each evaluated cytokine/chemokine.
Statistical Analysis of Immunologic Data
Statistical analysis was performed with SPSS statistical software (version 24). Differences between T cells subset data obtained from CRC and CRC-S samples and tissue cytokine levels evaluated in the same groups were assessed with paired Wilcoxon signed-rank tests. P values less than 0.05 were considered statistically significant.
Microbiota Characterization
DNA Extraction
Genomic DNA was extracted using the DNeasy PowerLyzer PowerSoil Kit (Qiagen, Hilden, Germany) from frozen (-80°C) CRC and CRC-S according to the manufacturer’s instructions. Briefly, tissues were added to a bead beating tube and thoroughly homogenized with TissueLyser II for 5 min at 30 Hz. Total genomic DNA was captured on a silica membrane in a spin column format and subsequently washed and eluted. The quality and quantity of extracted DNA was assessed using the NanoDrop ND-1000 (Thermo Fisher Scientific, Waltham, US) and the Qubit Fluorometer (Thermo Fisher Scientific), respectively. Then, genomic DNA was frozen at -20°C.
Bioinformatic Analysis of 16S rRNA
Extracted DNA samples were sent to IGA Technology Services (Udine, Italy), where amplicons of the variable V3–V4 region of the bacterial 16s rRNA gene were sequenced in paired-end (2 × 300) cycles on the Illumina MiSeq platform, according to the Illumina 16S Metagenomic Sequencing Library Preparation protocol (30).
Raw sequences were processed following the software pipeline MICCA (31). Paired-end reads were assembled using the “mergepairs” command, maintaining a minimum overlap of 100 bp and an edit distance in the maximum overlap of 32 bp. Subsequently, the sequences were cut with the “trim” command to remove the primers and eventually eliminate the reads with imperfect primer sequences. All the reads with a length lower than 350 bp and with an error rate higher than or equal to 0.5 were removed with the “filter” command.
Clean reads were eventually merged into a single file with the “merge” command and transformed into a FASTA format file. The operational taxonomic units (OTUs) were generated using the “otu” command in “denovo_greedy” mode, setting a 97% identity and performing an automatic removal of chimeras with the “-c” option. The longest sequence of each OTU was used for taxonomic assignment using the “classify” command in “rdp” mode, i.e., using the RDP Bayesian classifier that is able to obtain classification and confidence for taxonomic ranks up to genus.
Statistical Analysis of Bacterial Communities
Statistical analyses on the bacterial communities were performed in R (R Core Team, 2014) with the help of the packages phyloseq 1.26.1 (32), DESeq2 1.22.2 (33), breakaway 4.6.16 (34, 35), and other packages satisfying their dependencies—in particular, vegan 2.5-5 (36). Rarefaction analysis on OTUs was performed using the function rarecurve (step 50 reads) and further processed to highlight saturated samples (arbitrarily defined as saturated samples with a final slope in the rarefaction curve with an increment in OTU number per reads < 1e-5).
For the cluster analysis (complete clustering on Euclidean distance) of the entire community, the OTU table was first normalized using the total OTU counts of each sample and then adjusted using square root transformation.
The coverage was calculated by Good’s estimator (37) using the formula: (1 - n/N) × 100, where n is the number of sequences found once in a sample (singletons) and N is the total number of sequences in that sample.
Richness, Shannon, Chao 1, and Evenness indices were used to estimate bacterial diversity in each sample using the function estimate_richness from phyloseq (31). The evenness index (38) was calculated using the formula E = S/log(R), where S is the Shannon diversity index and R is the number of OTUs in the sample. Differences in all indices between CRC and CRC-S were tested using a paired Wilcoxon signed-rank test. Sample richness was further measured using the estimator and its associated error introduced in the breakaway package (32). The function betta_random of the breakaway package was further used to evaluate the statistical differences in richness between paired-by-patient CRC and CRC-S samples.
The differential analysis of abundance was performed with DESeq2 (31) at the OTUs and at the different taxonomic ranks (created using the tax_glom function in phyloseq) by using a two-group blocked-by-patient design to perform a paired test.
Statistical Analysis of the Association Between Tissue Microbiota and Cytokines
The association between tissue microbiota and cytokines was investigated with a 2-step analysis separately for the mucosa and tumor tissues. In the first step, we implemented a modified version of the sure independence screening (SIS) procedure (39). SIS uses the notion of marginal correlation—in our case, the correlation of a single cytokine with the dependent variable—to rank the cytokines. The cytokines with the smallest p-value from a Dirichlet regression (40) with that given cytokine as the only predictor are included in step 2. For each cytokine, the p-value is obtained testing the model with the considered cytokine and the intercept against a model with only the intercept with a likelihood-ratio test. Step 1 is necessary only when the list of cytokines is too long compared with the sample size; in our analysis, we selected the three most relevant cytokines from step 1.
In the second step, we used Dirichlet multinomial regression to determine the joint effect of cytokines on the tissue microbiota. We implemented a Bayesian variable selection (BVS) method based on a thresholding function (41). This approach is based on a Monte Carlo Markov chain algorithm that explores the space of possible models. The method’s output is a list of posterior probability of inclusion (PPI) and the posterior mean of the nonzero regression coefficients. PPI is the probability, between 0 and 1, that a given association cytokine-genera is nonzero, accounting for the effect of all other cytokines. The posterior mean is an estimate of a nonzero association. Each estimated regression coefficient evaluates the taxon–cytokine association, whose sign and magnitude measure the effect of the cytokine on the taxon.
Results
Assessment of Tissue Infiltrating T Cell Subset Distribution in Healthy and Cancer Mucosa
We performed polychromatic flow cytometry analysis of TILs isolated from the dissociated CRC and CRC-S. The percentage of CD4+ and CD8+ TILs in the mucosa sample group did not differ significantly. In detail, the mean percentages (SD) of CD4+ cells were 58.03 (6.88) in CRC vs. 58.15 (6.32) in CRC-S and the mean percentages of CD8+ T cells were 17.42 (5.65) in CRC vs. 14.39 (4.14) in CRC-S.
The analysis of the T cell subsets revealed that the tumor mucosa sample group displayed higher percentages of Th17 (CRC vs. CRC-S: 10.02 (4.32) vs. 5.13 (1.39); p=0.0008), Th2 (CRC vs. CRC-S: 3.45 (1.31) vs. 1.41 (0.93); p=0.0011), and Treg (CRC vs. CRC-S: 4.08 (1.44) vs. 2.10 (0.57); p=0.0040) as shown in Figures 1A, C. Regarding the T cytotoxic cells, the CRC group showed higher percentages of Tc17 (CRC vs. CRC-S: 6.33 (3.98) vs. 1.77 (1.00), p=0.0036), Tc1/Tc17 (CRC vs. CRC-S: 6.25 (4.74) vs. 1.88 (1.48), p=0.0022), and Tcreg (CRC vs. CRC-S: 1.08 (0.81) vs. 0.06 (0.08), p=0.0055) (Figure 1B). Notably, the number of Th9s is major (but not significant) in CRC tissue, and the Tc9s are similar in the two different sites.
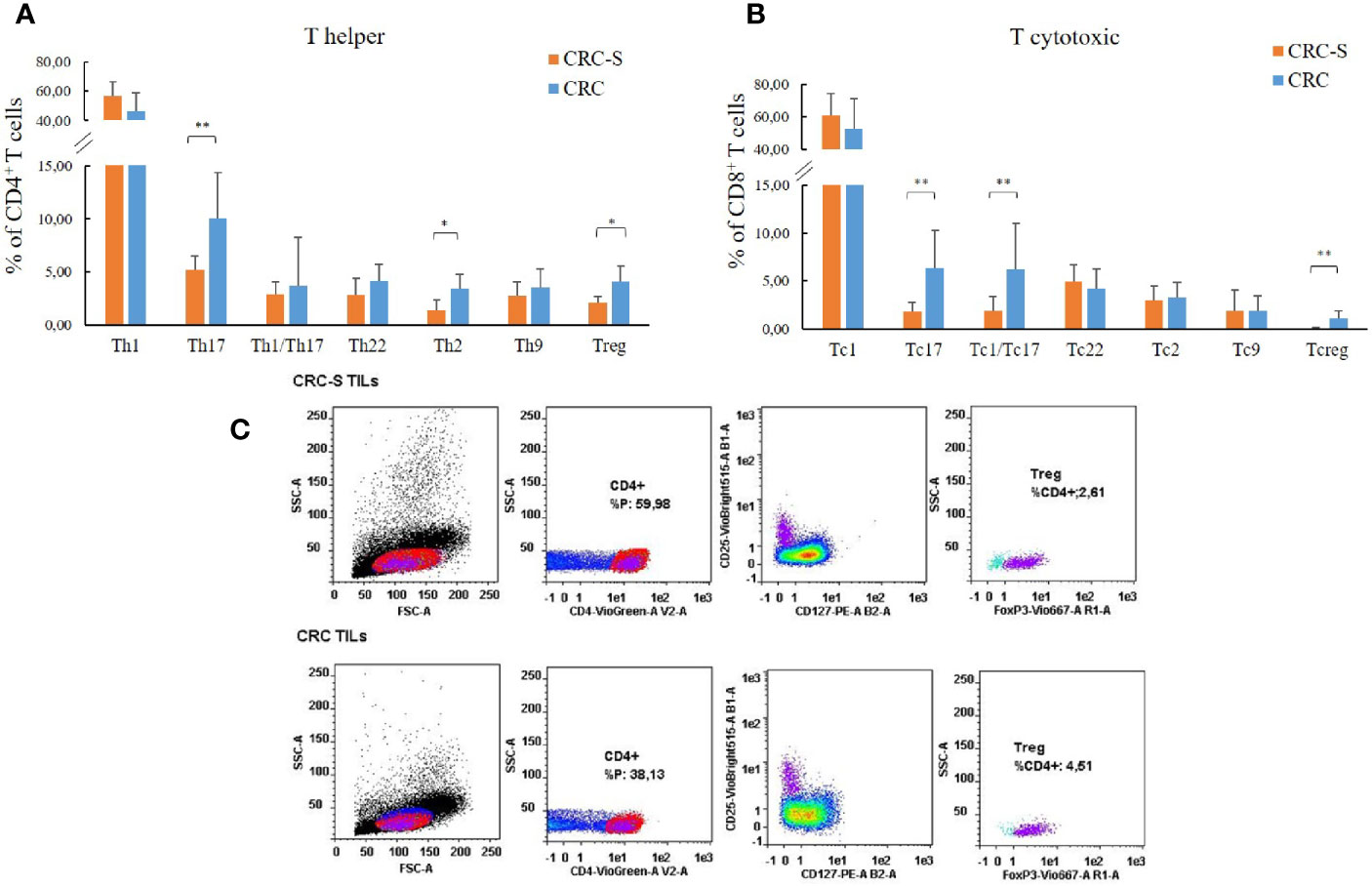
Figure 1 T cell subset distribution in the tumor mucosa and surrounding healthy mucosa samples groups. Panel (A) reports the mean percentages (+SD) of T helper subsets with respect to the percentage of CD4+ T cells, and panel (B) reports the mean percentages (+SD) of T cytotoxic subsets with respect to the percentage of CD8+ T cells. Panel (C) reports the cytofluorimetric analysis of T regulatory cells in the tumor mucosa and surrounding healthy mucosa samples of one representative patient. Statistical analyses were calculated using Wilcoxon signed-rank test. The asterisks (*) represent p-values, *p < 0.01, **p < 0.001. CRC = tumor mucosa; CRC-S = healthy mucosa.
Molecular Inflammatory Profile in CRC-Associated Tissues
We compared the molecular inflammatory profile of the homogenized CRC and CRC-S of 14 cancer patients through the evaluation of 26 pro- and anti-inflammatory cytokines. Six of the evaluated cytokines (IL-4, IL-10, IL12p70, IL-13, IL-23, and IFN-α) were under the LLOQ (Table 2) in all samples either because levels were very low (not detectable) or these molecules are not produced. The other 20 cytokines showed a common trend in all patients, characterized by higher levels in CRC compared to CRC-S. In particular, IL-1β, IL-2, IFN-γ, P-selectin, MIP-1α, IL-6, IL-17A, TNF-α, MCP-1, IL-9, IL-1α, IP-10, and IL-8 were increased significantly in CRC compared to CRC-S (Figure 2), whereas IL-27, IL-21, IL-22, IL-18, IL-5, GM-CSF, and sICAM1 showed a similar but not significant trend (p > 0,05).
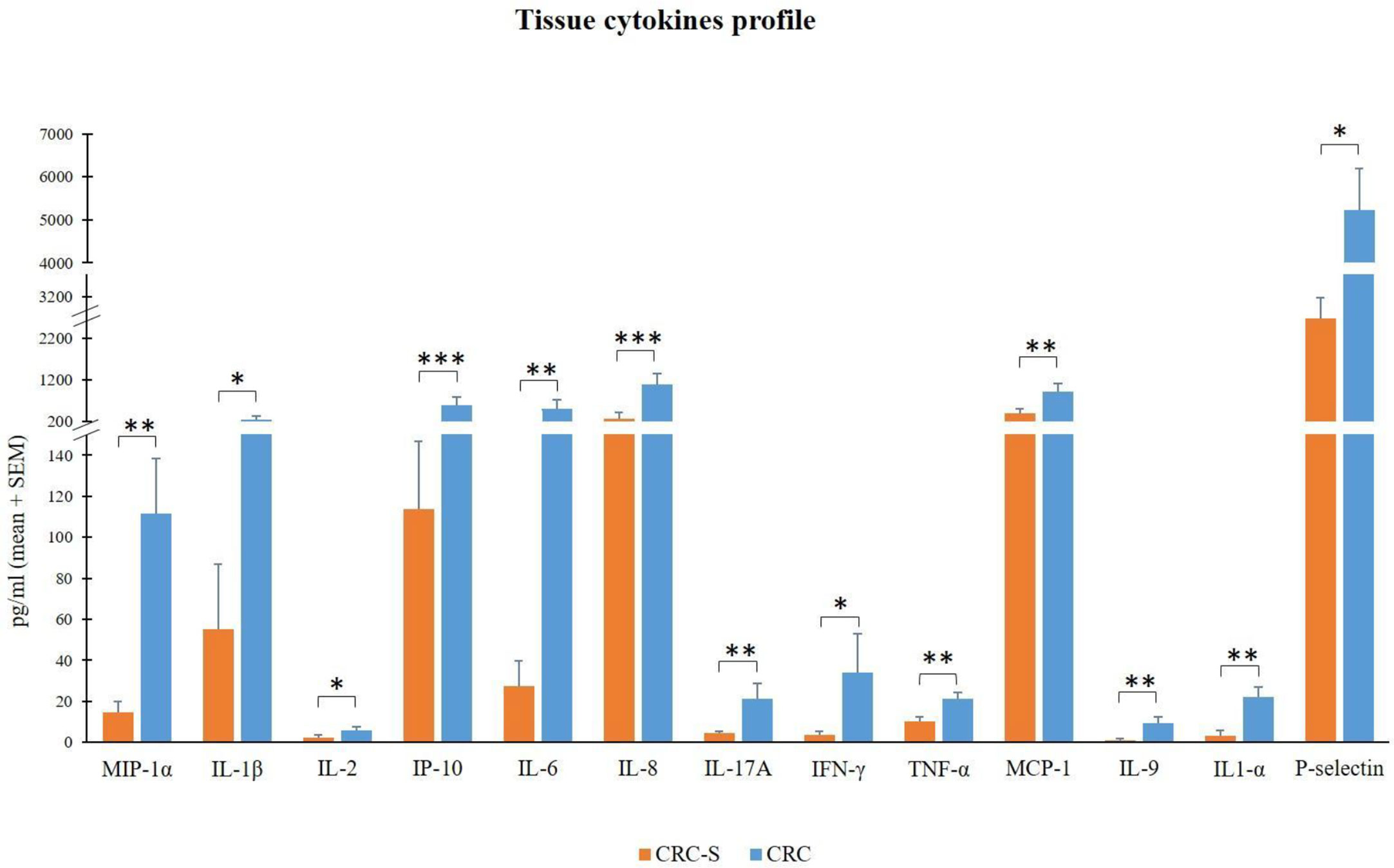
Figure 2 Tissue cytokine levels in 14 CRC patients. The histogram reports the mean (+SEM) cytokine levels (pg/ml) of the evaluated cytokines in CRC-S and CRC of 14 CRC patients. Wilcoxon signed-rank test was performed to test the differences between CRC-S and CRC paired samples. A p-value < 0.05 is considered statistically significant. The asterisks (*) represent p-values, *p < 0.05, **p < 0.01, ***p < 0.001. CRC-S= healthy mucosa; CRC= tumor mucosa.
Comparison of Mucosal Microbiota Composition in CRC and CRC-S
Our sequencing efforts in assessing microbiota composition encompassed a total of 12,475,251 reads for 40 sample pairs. After all the preprocessing steps, which included pair merging, trimming, quality filtering, and chimera detection, a total of 8,458,126 (67.8%) were available for further analysis.
Saturation curves (Figure 3) revealed that most specimens were sufficiently sampled. Samples showed a Good’s coverage ranging from 99% to 100%, indicating that less than 1% of the reads in a given sample came from OTUs that appeared only once in that sample.
As shown in Figure 4, the alpha diversity of samples did not display significant differences for Shannon index and Evenness. On the contrary, a significant (p = 0.011) Chao1 index evidenced that rare OTUs are enriched in CRC-S vs. CRC, denoting a higher diversity.
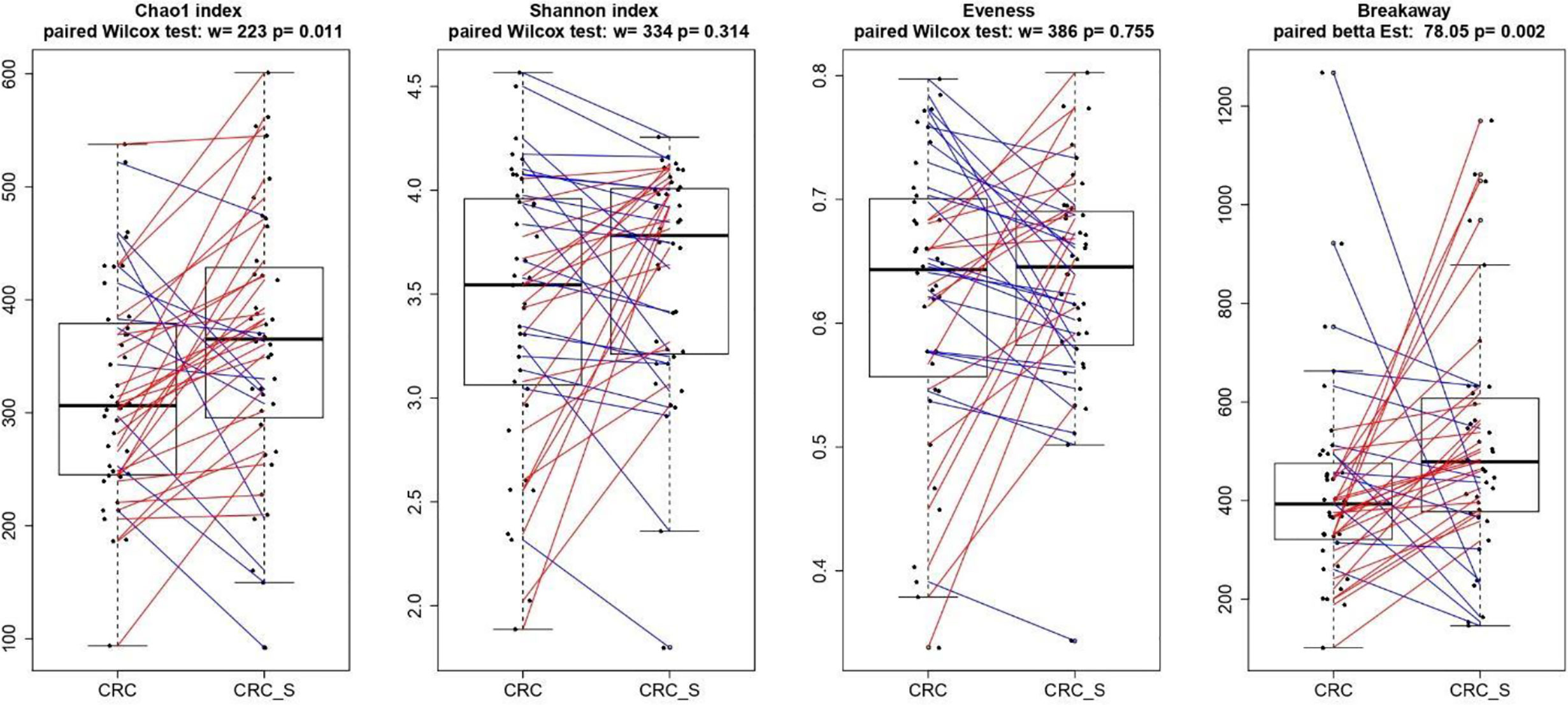
Figure 4 Boxplots showcasing alpha diversity indices (Chao1 index, Shannon index, Evenness, and Breakaway) in CRC and CRC-S samples. Statistical differences were evaluated using paired Wilcoxon signed-rank test for Chao, Shannon, and Evenness indices and using the paired betta analysis implemented in the Breakaway R package. P-values less than 0.05 were considered statistically significant.
Taxonomic analysis detailed in Table 3 reveals for the 2454 OTUs formed the confident (<20% error) presence of 29 phyla (>99% reads), 50 classes (>98% reads), 87 orders (>98% of reads), 176 families (>96% reads), and 372 genera (>86% reads).
To investigate and confirm the paired nature of sampling (i.e., tumor tissue vs. surrounding healthy tissue), we performed a cluster analysis on normalized OTU counts. As shown in Figure 5, we verified that 36/40 paired samples were, in effect, also paired in terms of microbial composition, a result robust to changes in distance metrics (e.g., Bray-Curtis) and the clustering method (data not shown). The analysis of the taxonomic composition reported that 6 phyla dominated the data set (98% sequences), namely Firmicutes (41.24%), Bacteroidetes (35.89%), Proteobacteria (13.37%), Fusobacteria (4.68%), Verrucomicrobia (2.10%), and Actinobacteria (1.56%) as shown in Figure 6. Stacked boxplots of taxa abundance at different taxonomic ranks are available as (Supplementary Figures S1–S4).
The paired comparison of the abundance of single OTUs revealed significant (adj. p<0.05, abs (logFC)>=1) differences between CRC and CRC-S sample groups with 6.6% OTUs involved. At the phylum level, Fusobacteria and Proteobacteria were significantly higher in CRC compared to CRC-S (logFC = -2.92, adj. p = 6.17e-15 and logFC = -0.95, adj. p = 1.92e-05, respectively). At the genus level, 14.7% genera were observed as significantly (adj. p<0.05, abs (logFC)>=1) different, the most abundant being Fusobacterium (average OTUs 6199, log2FC = -2.93, adj. p = 1.06e-08), Ruminococcus2 (Lachnospiraceae family, average OTUs 2911, log2FC = 1.31, adj. p = 1.38e-3), and Ruminococcus (Ruminococcaceae family, average OTUs 1640, log2FC = 1.82, adj. p = 4.12e-05).
Correlation of the Cytokine Profile With the Microbiota Composition in CRC-Associated Tissues
To evaluate this critical and crucial point, we first applied the SIS screening procedure (step 1). We considered the OTU counts of the three most abundant genera and aggregated all other genera in a residual category. Our dependent variable was then defined by four categories: Bacteroides spp., Prevotella spp., and Escherichia/Shigella spp. (plus the residual category). The four top-ranked cytokines were IL-18, IFN-γ, IL-5, and IL-2. Using these four cytokines (plus the intercept), we ran the BVS method (step 2). In the tumor tissues, we detected the association between IL-5 and Prevotella spp. with a PPI=0.81; the same association was found to be supported by the data collected from the mucosa tissues as well (PPI=0.77). The effect of IL-5 on Prevotella is estimated to be positive and equal to 0.64 (posterior mean) and 0.86 for tumor and mucosa tissues, respectively. However, this analysis hardly describes the entire picture because many cytokines with very small p-values in the first step were not included in the second step due to computational constraints.
Moreover, we decided to perform a second analysis that includes directly into step 2 the cytokines that showed differential abundances in comparative analysis between CRC and CRC-S samples. However, due to the computational constraints that impose a limit on the sample size, we selected some of the cytokines that were significantly increased in CRC compared to CRC-S samples, according to their relevance in the current literature. In particular, we chose IFN-γ, IL-17A, IL-8, IL-1β, IL-1α, IP-10, MIP-1α, and IL-9. The results of the BVS Dirichlet multinomial regression (step 2) are reported in Tables 4 and 5. From the results, we notice that Prevotella spp. is associated with both IL-17A and IL-9. The first association is negative, and the effect is 1.08 (posterior mean), whereas the second is positive, with a posterior mean of 1.37. Bacteroides spp. and Escherichia/Shigella spp. show a negative association with IL-9 and IP-10, respectively, with posterior means equal to -0.91 and -0.89, respectively.
Discussion
In this study, we first explored the immunity–microbiota axis in human CRC, comparing the distribution of TILs, the cytokine profile, and the GM composition in cancerous and surrounding mucosa. In agreement with our previous findings (11), the TIL assessment revealed higher percentages of tumor-supporting T cell subsets (Th17, Th2, Th9, and Tregs) in CRC samples compared to CRC-S. Contextually, a Th2 shift in the tumor microenvironment, especially for CRC, strongly contributes to cancer relapse, metastasis, and worse prognosis (42, 43) although, according to existing contradictory evidence, excess inflammation caused by CD4+ and CD8+ IL-17-producing T cells or the immunosuppression induced by Tregs may lead to carcinogenesis (44, 45). Indeed, tumor-infiltrating Th17 and Tc17 cells have been found in various human cancers, confirming their protumorigenic properties (46, 47). According to our results, different studies found higher percentages of Th17 and Tc1/Tc17 cells in tumor tissues compared to adjacent nontumor tissues (48–50). Through their immunosuppressive properties, Tregs can favor immune escape mechanisms of tumor cells, and that is why high amounts of peripheral or tumor-infiltrating Tregs are often associated with poor clinical outcome in gastrointestinal cancer (51, 52). According to our findings, a high number of tumor-infiltrating Tregs is widely documented (53–55).
Finally, Th9 cells, a relatively new subset, seem to have a dual role in tumor progression. Generally, the Th9 cells (activating both the innate and adaptive immune responses) and the Tc9s play an important role in antitumor immunity (56, 57), but IL-9 can exert a tumorigenic role in both hematological and solid tumors (58).
In addition to the TIL analysis, although many studies investigate the levels of several plasma cytokines in CRC patients (59–62), we assessed for the first time the molecular inflammatory profile of CRC mucosa through the evaluation of an exhaustive panel of 26 cytokines of which 20 were increased in CRC compared to CRC-S. In particular, CRC samples revealed significantly higher levels of MIP-1α, IL-1β, IL-2, IP-10, IL-6, IL-8, IL-17A, IFN-γ, TNF-α, MCP-1, IL-1α, P-selectin, and IL-9.
The relevant higher percentages of chemokines MCP-1, MIP-1α, IL-8, and IP-10 reflect the high colonic inflammation, and many studies demonstrate their role in the development of a tumor-favoring microenvironment due to their abilities to favor angiogenesis and to stimulate macrophages and CD8+ T cell recruitment in situ (63–65). In accordance with our results, different studies found high levels of these chemokines in CRC tissues (66–68).
In addition, the cytokines IL-1α, IL-1β, IL-6, IL-17A, and TNF-α promote tumor initiation, progression, angiogenesis, and metastasis in many human malignancies, including CRC (69–71), and our findings are consistent with previous studies (72–75).
Finally, although the overexpression of IFN-γ in CRC tissues can be considered positive (for its established robust antitumor activity) (76, 77), IL-2 and IL-9 display both pro- and antitumor potentials (78, 79). About IL-9, in contrast with our results, Wang et al. show that IL-9 is less expressed in human colon carcinoma (80). Nevertheless, Huang et al. report low IL-9 amounts in CRC patients, but these low levels are associated with tumor progression (81). Interestingly, Tian and colleagues show that IL-9 expression in colitis-associated cancer tissue is significantly higher than that in adjacent tissues, and Lentiviral vector–mediated IL-9 overexpression in the colon cancer cells lines RKO and Caco2 could promote their proliferation (82).
The relationship between CRC development and GM imbalance has been well established in past years (83–85), and numerous studies document distinct fecal and mucosal microbiota profiles in CRC patients compared with healthy subjects (86–88). Indeed, CRC-associated microbiota profiles differ from those in healthy subjects (87), and a brilliant meta-analysis of eight geographically and technically diverse fecal shotgun metagenomic studies of CRC identifies a peculiar colon cancer signature (88). Hence, we characterized the microbiota composition of tumor and adjacent healthy mucosa in the enrolled CRC patients, and according to previous findings, a significant Chao1 index evidences that rare OTUs are enriched in CRC-S vs. CRC (89, 90). We also confirm our recent data (86), demonstrating that Fusobacteria and Fusobacterium spp. are associated with CRC and are amplified during colorectal carcinogenesis (91–93), and we find a significantly higher percentage of Proteobacteria in CRC, according to evidence that an imbalanced GM is often associated with a sustained increase in Proteobacteria phylum members (94, 95). Consistent with Weir et al (96), we find that Ruminococcus spp. are more represented in CRC even if some authors report that, in CRC patients, Ruminococcus spp. have low prevalence (97, 98).
Moreover, to explore the mucosal microbiota–local immune response axis, we correlate—for the first time—the cytokine profile and GM composition using BVS Dirichlet multinomial regression. The application of the SIS screening procedure and the OTU counts of the three most abundant genera (Bacteroides spp., Prevotella spp., Escherichia/Shigella spp., and all other genera aggregated in a residual category), allowed us to identify the four top-ranked cytokines (IL-18, IFN-γ, IL-5, and IL-2) that we used to run the BVS method. Although these analyses cannot adequately describe the complex scenario of the relationship between secreted cytokines and intestinal composition, we observed a positive association between IL-5 and Prevotella spp. in both tumor and mucosa tissues.
As is well known, IL-5 is essential for eosinophil differentiation, and eosinophilia has been observed in various cancers, including CRC, with a controversial prognosis link. Eosinophil infiltration is considered unfavorable in Hodgkin’s lymphoma but positive in breast and prostate cancers (99). As recently reviewed (100), higher numbers of infiltrating eosinophils detected in CRC tissue were repeatedly shown to be prognostically favorable (101–103), but the mechanisms of CRC growth inhibition remain poorly understood. A recently developed CRC mouse model shows that tumor-homing eosinophils secrete chemoattractants for CD8+ effector T cells, eventually causing tumor rejection (104).
Furthermore, abundant IL-5 levels are documented in the synovium of rheumatoid arthritis patients (105), and notably, Scher et al. show that the presence of Prevotella copri is strongly correlated to rheumatoid arthritis (106). Therefore, taking into account all these reported data, our results—showing a positive correlation between IL-5 and Prevotella spp.—and the IL-5 anti-inflammatory role, we can assume an attempt to restore a eubiotic ecosystem in the colon mucosa contrasting the CRC development.
In the BVS method, we include all cytokines that show differential abundances in the comparative analysis of CRC and CRC-S: IFN-γ, IL-17A, IL-8, IL-1β, IL-1α, IP-10, MIP-1α, and IL-9. For the first time, we find that, again, Prevotella spp. is negatively associated with IL-17A but positively related to IL-9. In addition, Bacteroides spp. and Escherichia/Shigella spp. show a negative association with IL-9 and IP-10, respectively.
Despite the negative correlation between Prevotella spp. and IL-17A, it has recently been discovered that Prevotellaceae are able to promote Th17 cell differentiation, and Prevotella spp. are associated with Th17-mediated diseases, including periodontitis and rheumatoid arthritis (107). These conflicting data could be explained by speculating that the same bacterial taxa, in different environmental conditions, may exert contrasting effects. However, we cannot exclude that contrasting results may derive from the relatively low level of taxonomic resolution of 16S rRNA gene metagenomics, which cannot fully discriminate between different species of the same genus (as Prevotella spp.). Indeed, different Prevotella species colonize the human body districts. The periodontal Prevotella spp. related to Th17 cell stimulation could not be subdued to a dysbiotic environment, such as the inflamed CRC mucosa. In addition, in a study in which Prevotella histicola was used to modulate immune response and treat arthritis in a humanized mouse model, Marietta et al. report that treated mice showed significantly lower levels of IL-17 in agreement with our data. The authors also report decreased level of IL-9 as compared to placebo-treated mice in contrast with our results (108). Finally, Campisciano (109) detected an increase of the relative vaginal abundance of Prevotella timonensis in women infected with HPV, which showed a decreased concentration of the IL-15, IL-7, and IL-9 that they associated with the virus infection.
Regarding the negative correlation between Bacteroides spp. and IL-9, we have not found studies documenting this association, but because it is well known that IL-9 is produced by Th17, we have indirect evidence of this correlation. Round et al. find that Bacteroides fragilis inhibits Th17 development, inducing Treg accumulation, and Vaahtovuo et al. demonstrate the lower abundance of Bacteroides spp. in rheumatoid arthritis patients (110, 111).
Our results also show that Escherichia/Shigella spp. are negatively correlated with the IP-10 that is induced in many viral, bacterial, and parasite infections, i.e., shigellosis and E. coli infection (112, 113). Because IP-10 is increased in CRC samples, the negative correlation between Escherichia/Shigella spp. also can be supported.
In conclusion, our data describe a clear dissimilarity of the cellular and molecular inflammatory profile and intestinal microbiota composition between the tumor and the adjacent healthy tissue, displaying the generation of a peculiar CRC microenvironment. The infiltrating T cell features and the higher percentages of several cytokines produced in the tumor tissue document that, among all different types of immune cells involved in the complex anticancer responses, some may even encourage neoplastic progression. In addition, the distinct microbiota CRC profile may suggest that microbial communities can drive and modulate the antitumor immune response. In fact, we show—for the first time in human CRC—that Prevotella and Bacteroides species are correlated positively and negatively, respectively, with the IL-9 that has an intriguing and still debated role in tumor immunity. We are aware that other studies in humans and in animal models are needed, but the observed correlation of the cytokine signature with the GM composition confirm the presence of bidirectional crosstalk between the immune response and the host’s commensal microorganisms, which may influence cancer development.
Data Availability Statement
The datasets presented in this study can be found in online repositories. The names of the repository/repositories and accession number(s) can be found in the article/Supplementary Material.
Ethics Statement
The studies involving human participants were reviewed and approved by Comitato Etico Area Vasta Toscana Centro. The patients/participants provided their written informed consent to participate in this study.
Author Contributions
EN, ER, SB, and AA conceived and designed the study, and drafted the paper. EN, FR, and GN acquired experimental data of immune response. ER, AM, RF, GB, CF, and CC acquired experimental data of microbiota. AT and MNR were involved in enrolment and obtaining clinical data of patients. MP and FS are responsible for the statistical analysis. MR is responsible for bioinformatics’ analysis of microbiota. EN, SB, FS, MR, and AA analyzed and interpreted the data. AA, PB, and DP critically revised the paper. All authors contributed to the article and approved the submitted version.
Conflict of Interest
The authors declare that the research was conducted in the absence of any commercial or financial relationships that could be construed as a potential conflict of interest.
Acknowledgments
The authors thank all CRC patients enrolled at the Unit of Surgery, University Hospital of Careggi (AOUC), University of Florence, and the healthy controls. We also thank Regione Toscana that supported the study (The Programma Attuativo Regionale Toscana funded by FAS) N. MICpROBIMM. We thank Dr. Mascha Stroobant for the language manuscript editing.
Supplementary Material
The Supplementary Material for this article can be found online at: https://www.frontiersin.org/articles/10.3389/fimmu.2020.573158/full#supplementary-material
References
1. Siegel RL, Miller KD, Jemal A. Cancer Statistics, 2017. CA Cancer J Clin (2017) 67:7–30. doi: 10.3322/caac.21387
2. Song M, Emilsson L, Bozorg SR, Nguyen LH, Joshi AD, Staller K, et al. Risk of colorectal cancer incidence and mortality after polypectomy: a Swedish record-linkage study. Lancet Gastroenterol Hepatol (2020) 5(6):537–47. doi: 10.1016/S2468-1253(20)30009-1
3. Fridman WH, Pagès F, Sautès-Fridman C, Galon J. The immune contexture in human tumours: impact on clinical outcome. Nat Rev Cancer (2012) 12:298–306. doi: 10.1038/nrc3245
4. Galon J, Pagès F, Marincola FM, Angell HK, Thurin M, Lugli A, et al. Cancer classification using the Immunoscore: a worldwide task force. J Transl Med (2012) 10:205. doi: 10.1186/1479-5876-10-205
5. de Visser KE, Eichten A, Coussens LM. Paradoxical roles of the immune system during cancer development. Nat Rev Cancer (2006) 6:24–37. doi: 10.1038/nrc1782
6. Hnatyszyn A, Hryhorowicz S, Kaczmarek-Ryś M, Słomski R, Scott RJ, Pławski A. Colorectal carcinoma in the course of inflammatory bowel diseases. Hered Cancer Clin Pract (2019) 17:18. doi: 10.1186/s13053-019-0118-4
7. Jess T, Frisch M, Simonsen J. Trends in overall and cause-specific mortality among patients with inflammatory bowel disease from 1982 to 2010. Clin Gastroenterol Hepatol (2013) 11:43–8. doi: 10.1016/j.cgh.2012.09.026
8. Schreiber RD, Old LJ, Smyth MJ. Cancer immunoediting: integrating immunity’s roles in cancer suppression and promotion. Science (2011) 331:1565–70. doi: 10.1126/science.1203486
9. Tosolini M, Kirilovsky A, Mlecnik B, Fredriksen T, Mauger S, Bindea G, et al. Clinical impact of different classes of infiltrating T cytotoxic and helper cells (Th1, Th2, Treg, Th17) in patients with colorectal cancer. Cancer Res (2011) 71:1263–71. doi: 10.1158/0008-5472.CAN-10-2907
10. Clarke SL, Betts GJ, Plant A, Wright KL, El-Shanawany TM, Harrop R, et al. CD4+CD25+FOXP3+ regulatory T cells suppress anti-tumor immune responses in patients with colorectal cancer. PLoS One (2006) 1:e129. doi: 10.1371/journal.pone.0000129
11. Niccolai E, Ricci F, Russo E, Nannini G, Emmi G, Taddei A, et al. The Different Functional Distribution of “Not Effector” T Cells (Treg/Tnull) in Colorectal Cancer. Front Immunol (2017) 8:1900. doi: 10.3389/fimmu.2017.01900
12. Duchmann R, Kaiser I, Hermann E, Mayet W, Ewe K, Meyer zum büschenfelde K-H, et al. Tolerance exists towards resident intestinal flora, but is broken in active inflammatory bowel disease (IBD). Clin Exp Immunol (1995) 102:448–55. doi: 10.1111/j.1365-2249.1995.tb03836.x
13. Garrett WS. The gut microbiota and colon cancer. Science (2019) 364:1133–5. doi: 10.1126/science.aaw2367
14. Sears CL, Garrett WS. Microbes, microbiota, and colon cancer. Cell Host Microbe (2014) 15:317–28. doi: 10.1016/j.chom.2014.02.007
15. Arthur JC, Perez-Chanona E, Mühlbauer M, Tomkovich S, Uronis JM, Fan TJ, et al. Intestinal inflammation targets cancer-inducing activity of the microbiota. Science (2012) 338:120–23. doi: 10.1126/science.1224820
16. Rubinstein MR, Wang X, Liu W, Hao Y, Cai G, Han YW. Fusobacterium nucleatum promotes colorectal carcinogenesis by modulating E-cadherin/b-catenin signaling via its FadA adhesin. Cell Host Microbe (2013) 14:195–206. doi: 10.1016/j.chom.2013.07.012
17. Kostic AD, Chun E, Robertson L, Glickman JN, Gallini CA, Michaud M, et al. Fusobacterium nucleatum potentiates intestinal tumorigenesis and modulates the tumor- immune microenvironment. Cell Host Microbe (2013) 14:207–15. doi: 10.1016/j.chom.2013.07.007
18. Wu T, Cui L, Liang Z, Liu C, Liu Y, Li J, et al. Elevated serum IL-22 levels correlate with chemoresistant condition of colorectal cancer. Clin Immunol (2013) 147:38–9. doi: 10.1016/j.clim.2013.02.007
19. Gur C, Ibrahim Y, Isaacson B, Yamin R, Abed J, Gamliel M, et al. Binding of the Fap2 protein of Fusobacterium nucleatum to human inhibitory receptor TIGIT protects tumors from immune cell attack. Immunity (2015) 42:344–55. doi: 10.1016/j.immuni.2015.01.010
20. Zitvogel L, Ayyoub M, Routy B, Kroemer G. Microbiome and Anticancer Immunosurveillance. Cell (2016) 165:276–87. doi: 10.1016/j.cell.2016.03.001
21. Gagliani N, Hu B, Huber S, Elinav E, Flavell RA. The fire within: microbes inflame tumors. Cell (2014) 157:776–83. doi: 10.1016/j.cell.2014.03.006
22. Perez-Chanona E, Trinchieri G. The role of microbiota in cancer therapy. Curr Opin Immunol (2016) 39:75–81. doi: 10.1016/j.coi.2016.01.003
23. He Y, Dong L, Cao Y, Bi Y, Liu G. IL-9 and Th9 Cells in Tumor Immunity. Adv Exp Med Biol (2020) 1240:35–46. doi: 10.1007/978-3-030-38315-2_3
24. Coussens LM, Zitvogel L, Palucka AK. Neutralizing tumor-promoting chronic inflammation: a magic bullet? Science (2013) 339:286–91. doi: 10.1126/science.1232227
25. Atarashi K, Nishimura J, Shima T, Umesaki Y, Yamamoto M, Onoue M, et al. ATP drives lamina propria TH17 cell differentiation. Nature (2008) 455:808–12. doi: 10.1038/nature07240
26. Grivennikov SI, Wang K, Mucida D, Stewart CA, Schnabl B, Jauch D, et al. Adenoma-linked barrier defects and microbial products drive IL-23/IL-17-mediated tumour growth. Nature (2012) 491:254–8. doi: 10.1038/nature11465
27. Humblin E, Thibaudin M, Chalmin F, Derangère V, Limagne E, Richard C, et al. IRF8-dependent molecular complexes control the Th9 transcriptional program. Nat Commun (2017) 8:2085. doi: 10.1038/s41467-017-01070-w
28. Singh N, Gurav A, Sivaprakasam S, Brady E, Padia R, Shi H, et al. Activation of Gpr109a, receptor for niacin and the commensal metabolite butyrate, suppresses colonic inflammation and carcinogenesis. Immunity (2014) 40:128–39. doi: 10.1016/j.immuni.2013.12.007
29. Furusawa Y, Obata Y, Fukuda S, Endo TA, Nakato G, Takahashi D, et al. Commensal microbe-derived butyrate induces the differentiation of colonic regulatory T cells. Nature (2013) 504:446–50. doi: 10.1038/nature12721
30. Pagliai G, Russo E, Niccolai E, Dinu M, Di Pilato V, Magrini A, et al. Influence of a 3-month low-calorie Mediterranean diet compared to the vegetarian diet on human gut microbiota and SCFA: the CARDIVEG Study. Eur J Nutr (2019) 59(5):2011–24. doi: 10.1007/s00394-019-02050-0
31. Albanese D, Fontana P, De Filippo C, Cavalieri D, Donati C. MICCA: a complete and accurate software for taxonomic profiling of metagenomic data. Sci Rep (2015) 5:9743. doi: 10.1038/srep09743
32. McMurdie PJ, Holmes S. phyloseq: An R package for reproducible interactive analysis and graphics of microbiome census data. PLoS One (2013) 8:e61217. doi: 10.1371/journal.pone.0061217
33. Love MI, Huber W, Anders S. Moderated estimation of fold change and dispersion for RNA-seq data with DESeq2. Genome Biol (2014) 15:550. doi: 10.1186/s13059-014-0550-8
34. Willis A, Bunge J. Estimating diversity via frequency ratios. Biometrics (2015) 71:1042–9. doi: 10.1111/biom.12332
35. Willis A. Species richness estimation with high diversity but spurious singletons. (2016+). arXiv Available at: https://adw96.github.io/breakaway/.
36. Oksanen J, Blanchet G, Friendly M, Kindt R, Legendre P, McGlinn P, et al. vegan: Community Ecology Package. R package version 2.2-1 (2015). Available at: http://CRAN.R-project.org/package=vegan.
37. Good IJ. The population frequencies of species and the estimation of population parameters. Biometrika (1953) 40:237–64. doi: 10.1093/biomet/40.3-4.237
38. Pielou EC. The measurement of diversity in different types of biological collections. J Theor Biol (1966) 13:131–44. doi: 10.1016/0022-5193(66)90013-0
39. Fan J, Lv J. Sure independence screening for ultrahigh dimensional feature space. J R Stat Soc B (2008) 70:849–911. doi: 10.1111/j.1467-9868.2008.00674.x
40. Maier MJ. DirichletReg: Dirichlet regression for compositional data in R. Research Report Series / Department of Statistics and Mathematics, 125. (Vienna: WU Vienna University of Economics and Business) (2014). Available at: http://dirichletreg.r-forge.r-project.org/.
41. Pedone M, Stingo FC. Soft thresholding Bayesian variable selection for compositional data analysis. SIS2019: Smart Statistics for Smart Applications. in: Arbia G, Peluso S, Pini A, Rivellini G Book of Short Papers SIS2019. Pearson Italia: Milano. (2019) 1200.
42. Contasta I, Berghella AM, Pellegrini P, Adorno D. Passage from normal mucosa to adenoma and colon cancer: alteration of normal sCD30 mechanisms regulating TH1/TH2 cell functions. Cancer Biother Radiopharm (2003) 18:549–57. doi: 10.1089/108497803322287628
43. Cui G, Florholmen J. Polarization of cytokine profile from Th1 into Th2 along colorectal adenoma-carcinoma sequence: implications for the biotherapeutic target? Inflamm Allergy Drug Targets (2008) 7:94–7. doi: 10.2174/187152808785107589
44. Knochelmann HM, Dwyer CJ, Bailey SR, Amaya SM, Elston DM, Mazza-McCrann JM, et al. When worlds collide: Th17 and Treg cells in cancer and autoimmunity. Cell Mol Immunol (2018) 15:458–69. doi: 10.1038/s41423-018-0004-4
45. Kuen DS, Kim BS, Chung Y. IL-17-Producing Cells in Tumor Immunity: Friends or Foes? Immune Netw (2020) 20:e6. doi: 10.4110/in.2020.20.e6
46. Kryczek I, Banerjee M, Cheng P, Vatan L, Szeliga W, Wei S, et al. Phenotype, distribution, generation, and functional and clinical relevance of Th17 cells in the human tumor environments. Blood (2009) 114:1141–9. doi: 10.1182/blood-2009-03-208249
47. Lee MH, Tung-Chieh Chang J, Liao CT, Chen YS, Kuo ML, Shen C-R, et al. Interleukin 17 and peripheral IL-17-expressing T cells are negatively correlated with the overall survival of head and neck cancer patients. Oncotarget (2018) 9:9825–37. doi: 10.18632/oncotarget.23934
48. Doulabi H, Rastin M, Shabahangh H, Maddah G, Abdollahi A, Nosratabadi R, et al. Analysis of Th22, Th17 and CD4+ cells co-producing IL-17/IL-22 at different stages of human colon cancer. BioMed Pharmacother (2018) 103:1101–6. doi: 10.1016/j.biopha.2018.04.147
49. Dunne MR, Ryan C, Nolan B, Tosetto M, Geraghty R, Winter DC, et al. Enrichment of Inflammatory IL-17 and TNF-α Secreting CD4+ T Cells within Colorectal Tumors despite the Presence of Elevated CD39+ T Regulatory Cells and Increased Expression of the Immune Checkpoint Molecule, PD-1. Front Oncol (2016) 6:50. doi: 10.3389/fonc.2016.00050
50. Kuang DM, Peng C, Zhao Q, Wu Y, Zhu LY, Wang J, et al. Tumor-activated monocytes promote expansion of IL-17-producing CD8+ T cells in hepatocellular carcinoma patients. J Immunol (2010) 185:1544–9. doi: 10.4049/jimmunol.0904094
51. Pastille E, Wasmer M, Adamczyk A, Vu VP, Mager LF, Phuong NNT, et al. The IL-33/ST2 pathway shapes the regulatory T cell phenotype to promote intestinal cancer. Mucosal Immunol (2019) 12:990–1003. doi: 10.1038/s41385-019-0176-y
52. Zahran AM, Nafady-Hego H, Mansor SG, Abbas WA, Abdel-Malek MO, Mekky MA, et al. Increased frequency and FOXP3 expression of human CD8+CD25High+ T lymphocytes and its relation to CD4 regulatory T cells in patients with hepatocellular carcinoma. Hum Immunol (2019) 80:510–6. doi: 10.1016/j.humimm.2019.03.014
53. Gai XD, Li C, Song Y, Lei Y-M, Yang B-X. In situ analysis of FOXP3+ regulatory T cells and myeloid dendritic cells in human colorectal cancer tissue and tumor-draining lymph node. BioMed Rep (2013) 1:207–12. doi: 10.3892/br.2012.35
54. Girardin A, McCall J, Black MA, Edwards F, Phillips V, Taylor ES, et al. Inflammatory and regulatory T cells contribute to a unique immune microenvironment in tumor tissue of colorectal cancer patients. Int J Cancer (2013) 132:1842–50. doi: 10.1002/ijc.27855
55. Chaput N, Louafi S, Bardier A, Charlotte F, Vaillant JC, Ménégaux F, et al. Identification of CD8+CD25+Foxp3+ suppressive T cells in colorectal cancer tissue. Gut (2009) 58:520–9. doi: 10.1136/gut.2008.158824
56. Purwar R, Schlapbach C, Xiao S, Kang HS, Elyaman W, Jiang X, et al. Robust tumor immunity to melanoma mediated by interleukin-9-producing T cells. Nat Med (2012) 18:1248–53. doi: 10.1038/nm.2856
57. Lu Y, Hong S, Li H, Park J, Hong B, Wang L, et al. Th9 cells promote antitumor immune responses in vivo. J Clin Invest (2012) 122:4160–71. doi: 10.1172/JCI65459
58. Chen N, Wang X. Role of IL-9 and STATs in hematological malignancies (review). Oncol Lett (2014) 7:602–10. doi: 10.3892/ol.2013.1761
59. Yamaguchi M, Okamura S, Yamaji T, Iwasaki M, Tsugane S, Shetty V, et al. Plasma cytokine levels and the presence of colorectal cancer. PLoS One (2019) 14:e0213602. doi: 10.1371/journal.pone.0213602
60. Song M, Sasazuki S, Camargo MC, Shimazu T, Charvat H, Yamaji T, et al. Circulating inflammatory markers and colorectal cancer risk: A prospective case-cohort study in Japan. Int J Cancer (2018) 143:2767–76. doi: 10.1002/ijc.31821
61. Krzystek-Korpacka M, Zawadzki M, Kapturkiewicz B, Lewandowska P, Bednarz-Misa I, Gorska S, et al. Subsite heterogeneity in the profiles of circulating cytokines in colorectal cancer. Cytokine (2018) 110:435–41. doi: 10.1016/j.cyto.2018.05.015
62. Kantola T, Klintrup K, Väyrynen J, Vornanen J, Bloigu R, Karhu T, et al. Stage-dependent alterations of the serum cytokine pattern in colorectal carcinoma. Br J Cancer (2012) 107:1729–36. doi: 10.1038/bjc.2012.456
63. Wang D, Dubois RN, Richmond A. The role of chemokines in intestinal inflammation and cancer. Curr Opin Pharmacol (2009) 9:688–96. doi: 10.1016/j.coph.2009.08.003
64. Bie Y, Ge W, Yang Z, Cheng X, Zhao Z, Li S, et al. The Crucial Role of CXCL8 and Its Receptors in Colorectal Liver Metastasis. Dis Markers (2019) 2019:8023460. doi: 10.1155/2019/8023460
65. Allen F, Bobanga ID, Rauhe P, Barkauskas D, Teich N, Tong C, et al. CCL3 augments tumor rejection and enhances CD8+ T cell infiltration through NK and CD103+ dendritic cell recruitment via IFNγ. Oncoimmunology (2017) 7:e1393598. doi: 10.1080/2162402X.2017.1393598
66. De la Fuente López M, Landskron G, Parada D, Dubois-Camacho K, Simian D, Martinez M, et al. The relationship between chemokines CCL2, CCL3, and CCL4 with the tumor microenvironment and tumor-associated macrophage markers in colorectal cancer. Tumour Biol (2018) 40(11):1010428318810059. doi: 10.1177/1010428318810059
67. Dimberg J, Skarstedt M, Löfgren S, Zar N, Matussek A. Protein expression and gene polymorphism of CXCL10 in patients with colorectal cancer. BioMed Rep (2014) 2:340–3. doi: 10.3892/br.2014.255
68. Jin WJ, Xu JM, Xu WL, Gu DH, Li PW. Diagnostic value of interleukin-8 in colorectal cancer: a case-control study and meta-analysis. World J Gastroenterol (2014) 20:16334–42. doi: 10.3748/wjg.v20.i43.16334
69. Zeng J, Tang ZH, Liu S, Guo SS. Clinicopathological significance of overexpression of interleukin-6 in colorectal cancer. World J Gastroenterol (2017) 23:1780–6. doi: 10.3748/wjg.v23.i10.1780
70. Wu D, Wu P, Huang Q, Liu Y, Ye J, Huang J. Interleukin-17: a promoter in colorectal cancer progression. Clin Dev Immunol (2013) 2013:436307. doi: 10.1155/2013/436307
71. Zhao P, Zhang Z. TNF-α promotes colon cancer cell migration and invasion by upregulating TROP-2. Oncol Lett (2018) 15:3820–7. doi: 10.3892/ol.2018.7735
72. Kakourou A, Koutsioumpa C, Lopez DS, Hoffman-Bolton J, Bradwin G, Rifai N, et al. Interleukin-6 and risk of colorectal cancer: results from the CLUE II cohort and a meta-analysis of prospective studies. Cancer Causes Control (2015) 26:1449–60. doi: 10.1007/s10552-015-0641-1
73. Nagasaki T, Hara M, Nakanishi H, Takahashi H, Sato M, Takeyama H, et al. Interleukin-6 released by colon cancer-associated fibroblasts is critical for tumour angiogenesis: anti-interleukin-6 receptor antibody suppressed angiogenesis and inhibited tumour-stroma interaction. Br J Cancer (2014) 110:469–78. doi: 10.1038/bjc.2013.748
74. Galizia G, Orditura M, Romano C, Lieto E, Castellano P, Pelosio L, et al. Prognostic significance of circulating IL-10 and IL-6 serum levels in colon cancer patients undergoing surgery. Clin Immunol (2002) 102:169–78. doi: 10.1006/clim.2001.5163
75. Al Obeed OA, Alkhayal KA, Al Sheikh A, Zubaidi AM, Vaali-Mohammed MA, Boushey R, et al. Increased expression of tumor necrosis factor-α is associated with advanced colorectal cancer stages. World J Gastroenterol (2014) 20:18390–6. doi: 10.3748/wjg.v20.i48.18390
76. Razaghi A, Owens L, Heimann K. Review of the recombinant human interferon gamma as an immunotherapeutic: Impacts of production platforms and glycosylation. J Biotechnol (2016) 240:48–60. doi: 10.1016/j.jbiotec.2016.10.022
77. Mager LF, Wasmer MH, Rau TT, Krebs P. Cytokine-Induced Modulation of Colorectal Cancer. Front Oncol (2016) 6:96. doi: 10.3389/fonc.2016.00096
78. Kusnierczyk H, Pajtasz-Piasecka E, Koten J-W, Bijleveld C, Krawczyk K, Den Otter W. Further development of local il-2 therapy of cancer: multiple versus single il-2 treatment of transplanted murine colon carcinoma. Cancer Immunol Immunother (2004) 53:445–52. doi: 10.1007/s00262-003-0490-8
79. Wang Y, Wang M, Li Y. Anti-colorectal cancer effect of interleukin-2 and interferon-β fusion gene driven by carcinoembryonic antigen promoter. Onco Targets Ther (2016) 9:3259–67. doi: 10.2147/OTT.S97444
80. Wang J, Sun M, Zhao H, Huang Y, Li D, Mao D, et al. IL-9 Exerts Antitumor Effects in Colon Cancer and Transforms the Tumor Microenvironment In Vivo. Technol Cancer Res Treat (2019) 18:1533033819857737. doi: 10.1177/1533033819857737
81. Huang Y, Cao Y, Zhang S, Gao F. Association between low expression levels of interleukin-9 and colon cancer progression. Exp Ther Med (2015) 10:942–6. doi: 10.3892/etm.2015.2588
82. Tian L, Li Y, Chang R, Zhang P, Zhang J, Huo L. Lentiviral vector-mediated IL-9 overexpression stimulates cell proliferation by targeting c-myc and cyclin D1 in colitis-associated cancer. Oncol Lett (2019) 17:175–82. doi: 10.3892/ol.2018.9567
83. Gagnière J, Raisch J, Veziant J, Barnich N, Bonnet R, Buc E, et al. Gut microbiota imbalance and colorectal cancer. World J Gastroenterol (2016) 22:501–18. doi: 10.3748/wjg.v22.i2.501
84. Tilg H, Adolph TE, Gerner RR, Moschen AR. The Intestinal Microbiota in Colorectal Cancer. Cancer Cell (2018) 33:954–64. doi: 10.1016/j.ccell.2018.03.004
85. Wong SH, Yu J. Gut microbiota in colorectal cancer: mechanisms of action and clinical applications. Nat Rev Gastroenterol Hepatol (2019) 16:690–704. doi: 10.1038/s41575-019-0209-8
86. Russo E, Bacci G, Chiellini C, Fagorzi C, Niccolai E, Taddei A, et al. Preliminary Comparison of Oral and Intestinal Human Microbiota in Patients with Colorectal Cancer: A Pilot Study. Front Microbiol (2018) 8:2699. doi: 10.3389/fmicb.2017.02699
87. Flemer B, Lynch DB, Brown JM, Jeffery IB, Ryan FJ, Claesson MJ, et al. Tumour-associated and non-tumour-associated microbiota in colorectal cancer. Gut (2017) 66:633–43. doi: 10.1136/gutjnl-2015-309595
88. Wirbel J, Pyl PT, Kartal E, Zych K, Kashani A, Milanese A, et al. Meta-analysis of fecal metagenomes reveals global microbial signatures that are specific for colorectal cancer. Nat Med (2019) 25(4):679–89. doi: 10.1038/s41591-019-0406-6
89. Yang J, McDowell A, Kim EK, Seo H, Lee WH, Moon CM, et al. Development of a colorectal cancer diagnostic model and dietary risk assessment through gut microbiome analysis. Exp Mol Med (2019) 51:1–15. doi: 10.1038/s12276-019-0313-4
90. Liu W, Zhang R, Shu R, Yu J, Li H, Long H, et al. Study of the Relationship between Microbiome and Colorectal Cancer Susceptibility Using 16SrRNA Sequencing. BioMed Res Int (2020) 7828392:1–17. doi: 10.1155/2020/7828392
91. Kostic AD, Gevers D, Pedamallu CS, Michaud M, Duke F, Earl AM, et al. Genomic analysis identifies association of Fusobacterium with colorectal carcinoma. Genome Res (2012) 22:292–8. doi: 10.1101/gr.126573.111
92. Yang Y, Weng W, Peng J, Hong L, Yang L, Toiyama Y, et al. Fusobacterium nucleatum increases proliferation of colorectal cancer cells and tumor development in mice by activating toll-like receptor 4 signaling to nuclear factor-kappaB, and up-regulating expression of microRNA-21. Gastroenterology (2017) 152:851–66. doi: 10.1053/j.gastro.2016.11.018
93. Park C, Han D, Oh Y, Lee AR, Lee YR, Eun CS. Role of Fusobacteria in the serrated pathway of colorectal carcinogenesis. Sci Rep (2016) 6:25271. doi: 10.1038/srep25271
94. Shin NR, Whon TW, Bae JW. Proteobacteria: microbial signature of dysbiosis in gut microbiota. Trends Biotechnol (2015) 33:496–503. doi: 10.1016/j.tibtech.2015.06.011
95. Mori G, Rampelli S, Orena BS, Rengucci G, De Maio G, Barbieri G, et al. Shifts of Faecal Microbiota During Sporadic Colorectal Carcinogenesis. Sci Rep (2018) 8:10329. doi: 10.1038/s41598-018-28671-9
96. Weir TL, Manter DK, Sheflin AM, Barnett BA, Heuberger AL, Ryan EP. Stool microbiome and metabolome differences between colorectal cancer patients and healthy adults. PLoS One (2013) 8:e70803. doi: 10.1371/journal.pone.0070803
97. Sun J, Kato I. Gut microbiota, inflammation and colorectal cancer. Genes Dis (2016) 3:130–43. doi: 10.1016/j.gendis.2016.03.004
98. Sobhani I, Tap J, Roudot-Thoraval F, Roperch JP, Letulle S, Langella P, et al. Microbial dysbiosis in colorectal cancer (CRC) patients. PLoS One (2011) 201(6):e16393. doi: 10.1371/journal.pone.0016393
99. Sakkal S, Miller S, Apostolopoulos V, Nurgali K. Eosinophils in Cancer: Favourable or Unfavourable? Curr Med Chem (2016) 23:650–66. doi: 10.2174/0929867323666160119094313
100. Loktionov A. Eosinophils in the gastrointestinal tract and their role in the pathogenesis of major colorectal disorders. World J Gastroenterol (2019) 25:3503–26. doi: 10.3748/wjg.v25.i27.3503
101. Fernández-Aceñero MJ, Galindo-Gallego M, Sanz J, Aljama A. Prognostic influence of tumor-associated eosinophilic infiltrate in colorectal carcinoma. Cancer (2000) 88:1544–8. doi: 10.1002/(SICI)1097-0142(20000401)88:7<1544::AID-CNCR7>3.0.CO;2-S
102. Prizment AE, Vierkant RA, Smyrk TC, Tillmans LS, Lee JJ, Sriramarao P, et al. Tumor eosinophil infiltration and improved survival of colorectal cancer patients: Iowa Women’s Health Study. Mod Pathol (2016) 29:516–27. doi: 10.1038/modpathol.2016.42
103. Harbaum L, Pollheimer MJ, Kornprat P, Lindtner RA, Bokemeyer C, Langner C. Peritumoral eosinophils predict recurrence in colorectal cancer. Mod Pathol (2015) 28:403–13. doi: 10.1038/modpathol.2014.104
104. Carretero R, Sektioglu IM, Garbi N, Salgado OC, Beckhove P, Hämmerling GJ. Eosinophils orchestrate cancer rejection by normalizing tumor vessels and enhancing infiltration of CD8(+) T cells. Nat Immunol (2015) 16:609–17. doi: 10.1038/ni.3159
105. Mateen S, Moin S, Shahzad S, Khan AQ. Level of inflammatory cytokines in rheumatoid arthritis patients: Correlation with 25-hydroxy vitamin D and reactive oxygen species. PLoS One (2017) 12:e0178879. doi: 10.1371/journal.pone.0178879
106. Scher JU, Sczesnak A, Longman RS, Segata N, Ubeda C, Bielski C, et al. Expansion of intestinal prevotella copri correlates with enhanced susceptibility to arthritis. Elife (2013) 2:e01202. doi: 10.7554/eLife.01202
107. de Aquino SG, Abdollahi-Roodsaz S, Koenders MI, van de Loo FA, Pruijn GJ, Marijnissen RJ, et al. Periodontal pathogens directly promote autoimmune experimental arthritis by inducing a TLR2- and IL-1-driven Th17 response. J Immunol (2014) 192:4103–11. doi: 10.4049/jimmunol.1301970
108. Marietta EV, Murray JA, Luckey DH, Jeraldo PR, Lamba A, Patel R, et al. Suppression of Inflammatory Arthritis by Human Gut-Derived Prevotella histicola in Humanized Mice. Arthritis Rheumatol (2016) 68:2878–88. doi: 10.1002/art.39785
109. Campisciano G, Gheit T, De Seta F, Cason C, Zanotta N, Delbue S, et al. Oncogenic Virome Benefits from the Different Vaginal Microbiome-Immune Axes. Microorganisms (2019) 7:414. doi: 10.3390/microorganisms7100414
110. Round JL, Lee SM, Li J, Tran G, Jabri B, Chatila TA, et al. The toll-like receptor 2 pathway establishes colonization by a commensal of the human microbiota. Science (2011) 332:974–7. doi: 10.1126/science.1206095
111. Vaahtovuo J, Munukka E, Korkeamäki M, Luukkainen R, Toivanen P. Fecal microbiota in early rheumatoid arthritis. J Rheumatol (2008) 35:1500–05.
112. Casrouge A, Bisiaux A, Stephen L, Schmolz M, Mapes J, Pfister C, et al. Discrimination of agonist and antagonist forms of CXCL10 in biological samples. Clin Exp Immunol (2012) 167:137–48. doi: 10.1111/j.1365-2249.2011.04488.x
Keywords: cytokines, colorectal cancer, T cells, immune response, gut microbiota
Citation: Niccolai E, Russo E, Baldi S, Ricci F, Nannini G, Pedone M, Stingo FC, Taddei A, Ringressi MN, Bechi P, Mengoni A, Fani R, Bacci G, Fagorzi C, Chiellini C, Prisco D, Ramazzotti M and Amedei A (2021) Significant and Conflicting Correlation of IL-9 With Prevotella and Bacteroides in Human Colorectal Cancer. Front. Immunol. 11:573158. doi: 10.3389/fimmu.2020.573158
Received: 29 September 2020; Accepted: 19 November 2020;
Published: 08 January 2021.
Edited by:
Marcello Chieppa, National Institute of Gastroenterology S. de Bellis Research Hospital (IRCCS), ItalyReviewed by:
Giulio Verna, National Institute of Gastroenterology S. de Bellis Research Hospital (IRCCS), ItalySama Rezasoltani, Shahid Beheshti University of Medical Sciences, Iran
Copyright © 2021 Niccolai, Russo, Baldi, Ricci, Nannini, Pedone, Stingo, Taddei, Ringressi, Bechi, Mengoni, Fani, Bacci, Fagorzi, Chiellini, Prisco, Ramazzotti and Amedei. This is an open-access article distributed under the terms of the Creative Commons Attribution License (CC BY). The use, distribution or reproduction in other forums is permitted, provided the original author(s) and the copyright owner(s) are credited and that the original publication in this journal is cited, in accordance with accepted academic practice. No use, distribution or reproduction is permitted which does not comply with these terms.
*Correspondence: Amedeo Amedei, YWFtZWRlaUB1bmlmaS5pdA==
†Present address: Carolina Chiellini, Department of Agriculture Food and Environment, University of Pisa, Pisa, Italy