- 1University Hospital, Immunology Laboratory, FRE-CNRS 3312, Saint-Etienne, France
- 2Stem Cell Laboratory, Immunology Department, Faculty of Medicine, Yeditepe University, Istanbul, Turkey
- 3Acomed Statistik, Leipzig, Germany
- 4Laboratory of Hematology, Department of Laboratory Medicine, Radboud University Medical Center, Nijmegen, Netherlands
- 5Department of Immunology-Histocompatibility, Evangelismos Hospital, Athens, Greece
- 6Institute for Medical Microbiology, Immunology and Hygiene, Technische Universität München, Munich, Germany
- 7Universitätsklinikum Knappschaftskrankenhaus Bochum, Bochum, Germany
- 8Medizinische Fakultät, Institut für Klinische Immunologie, Universität Leipzig, Leipzig, Germany
Many anticancer therapies such as antibody-based therapies, cellular therapeutics (e.g., genetically modified cells, regulators of cytokine signaling, and signal transduction), and other biologically tailored interventions strongly influence the immune system and require tools for research, diagnosis, and monitoring. In flow cytometry, in vitro diagnostic (IVD) test kits that have been compiled and validated by the manufacturer are not available for all requirements. Laboratories are therefore usually dependent on modifying commercially available assays or, most often, developing them to meet clinical needs. However, both variants must then undergo full validation to fulfill the IVD regulatory requirements. Flow cytometric immunophenotyping is a multiparametric analysis of parameters, some of which have to be repeatedly adjusted; that must be considered when developing specific antibody panels. Careful adjustments of general rules are required to meet legal and regulatory requirements in the analysis of these assays. Here, we describe the relevant regulatory framework for flow cytometry-based assays and describe methods for the introduction of new antibody combinations into routine work including development of performance specifications, validation, and statistical methodology for design and analysis of the experiments. The aim is to increase reliability, efficiency, and auditability after the introduction of in-house-developed flow cytometry assays.
Introduction
Medical routine and study laboratories are subject to a large number of regulations. Recommendations on standard practices for flow cytometry (FCM) validation procedures must comply with legal obligations, the European Regulation 2017/746 on in vitro diagnostic medical devices (EU-IVD-R), which also contains mandatory requirements for in vitro diagnostic medical devices (IVD) developed and manufactured in healthcare facilities within the European Union (1).
FCM is applied in different analytical fields that comprise assays for research use only (RUO), preclinical applications (PCA) as well as routine methods provided as medical laboratory services. Quality standards for RUO assays and PCA depend on specific rules set by authorities or research and development (R&D) institution, respectively. A common framework for research reporting is the “Minimum Information about a Flow Cytometry Experiment” (2); preclinical rules depend on the context.
Immune therapies for tumors require manifold flow cytometric support. Firstly, while detection of circulating tumor cells is still experimental (3), diagnosis of leukemias and lymphomas is well-established, and a few IVD test kits already exist. Secondly, monitoring of hematological and solid tumor response to therapy is increasingly important, especially in antibody therapies, e.g., reduction of normal of malignant B cell counts following antibody therapy (4), detection of checkpoint inhibitor receptor expression (5), or quantification of CAR-T cells following CAR-T cell therapy (6). Next, detection of adverse effects of novel therapies on lymphocyte subpopulations and their functions supports best medical practice and provides additional knowledge in novel treatments (7).
Our recommendation aims to provide guidance to fulfill legal and normative obligations of EU-IVD-R and EN ISO 15189 (ISO), respectively. Technical terms given in the following recommendations were taken from International vocabulary of metrology (VIM)—Basic and general concepts and associated terms (8). Technical terms from the EU IVD-R are preferred because of their mandatory character in cases of lack of conformity with VIM.
FCM encompasses a wide range of different methodological approaches. It is not in the scope of this article to provide detailed experimental protocols that consistently cover all FCM-based applications. Rather, our focus is on aspects that (i) address specific problems of FCM for novel diagnostic requests, (ii) are common to most FCM-based assays intended for use as a medical laboratory service, and (iii) are minimal experimental requirements that are mandatory to fulfill the above mentioned legal and normative obligations.
Legal and Regulatory Obligations
EU-IVD-R defines IVD as “…any medical device which is a reagent, reagent product, calibrator, control material, kit, instrument, apparatus, piece of equipment, software or system, whether used alone or in combination, intended by the manufacturer to be used in vitro for the examination of specimens, including blood and tissue donations, derived from the human body…” in the field of medical healthcare (1). The CE (Conformité Européenne) mark certifies that an IVD is in compliance with the European In vitro Medical Device Directive 98/79/EC. According to EU-IVD-R, the use of CE-marked IVDs is mandatory for all laboratories that perform diagnostic tests in patient care. So-called in-house tests can only be employed if no product with CE marking is available on the market that meets the appropriate level of performance, which is the case for many parameters in the field of immune oncology. Laboratories must also comply with EN ISO 15189 or, where applicable, appropriate national regulations. Minimum standards are the general safety and performance requirements according to Annex I of the EU IVD-R. Furthermore, a documented risk management system as well as the definition and evaluation of analytical or clinical performance characteristics must be maintained throughout the entire life cycle of an IVD.
ISO 15189 (9) aims to implement the quality assurance policy into medical laboratory services (10–12). This must consider biological and technical specificities encountered in some technique such as in quantitative cell analysis (cytometry) as recently discussed (13, 14).
There are numerous relationships between the requirements of the EU-IVD-R (1) and ISO 15189 (9), which are further modified by national legislation. ISO 15189 accreditation covers laboratory management and technical issues. The first part addresses general laboratory organization in detail (9). The second part addresses technical issues (Supplement I) classified under Ishikawa (Fishbone) diagram (15). Much information is common to any analysis:
• Operator authorization (ISO 15189 chapter 5.1),
• Environment (5.2),
• Instruments and reagents (5.3),
• Sampling and pre-analytics (5.4),
• Validation, metrology, or contamination (5.5), (5.6),
• Post-analytics and reporting (5.7 to 5.9), and
• Laboratory information management system (LIMS; 5.10) (9).
Additional information is highly specific to each analysis: method settings, validation, exclusion of interferences (5.5), and quality control and standardization (5.6).
ISO 15189 allows a flexible scope that is highly recommended to FCM laboratories. Flexible scope allows continuous expansion of the range of flow cytometric parameters. This depends on well-established validation procedures, followed by continuous evaluation and occasional improvements. This must be periodically supervised by audits, reports, and management reviews (14).
Various aspects of laboratory management (Quality management, LIMS, agreements, client feedback, complaints, etc.) as well as of the analytical process (measurement, “mother nature”) follow general rules of laboratory diagnostics and will not be discussed here. In contrast, manpower, material, machine, and method require serious consideration in the field of FCM for which consensual resolution is needed. Various national activities have been published to support laboratories in the validation process, for instance in Brazil (16) or Germany (17, 18).
Common Practice in Immune Oncological Flow Cytometry
Whereas, the EU-IVD-R determines the necessary properties to be validated, both general and FCM specific guidelines have been developed that provide more detailed information regarding the experimental design and statistical methods for analysis. In particular, the guidelines developed by the Clinical and Laboratory Standards Institute (CLSI “evaluation protocols”) are quite helpful (19–21). However, adaptation of the guidelines to flow cytometry is challenging.
Several attempts have been made to develop guidance for method validation experiments for flow cytometry-based assays (22, 23). Although the guidance by Selliah et al. (23) provides a wide range of experiments as well as acceptance criteria, the statistical methodology, including the rules for deriving the necessary sample sizes, do not correspond to the state of the art.
Finally, it must be mentioned that there is still inconsistency in the terms used to describe parameters to validate. For example, in the EU-IVD-R the term “analytical sensitivity” is still used although the definition of limits of detection (LoD) and quantitation (LoQ) offer a more precise description of the underlying concepts. Another example is the use of the term “accuracy.” It is differently defined in the pharmaceutical world as describes “the systematical error of a measurement” (24), while in the laboratory medicine community where accuracy encompasses both systematic and random errors. Internationally accepted white papers and protocols have been published on this topic (23, 25). The aim of our paper is to propose a reasonable but also efficient consensus strategy for introducing laboratory-developed panels and performing method validation in clinical FCM laboratories as well as to propose minimal criteria to fulfill.
What Makes FCM so Unique?
Guidance for method validation in FCM is hard to establish due to the complex nature of this technology. This includes the requirement for samples, the fact that cell characterization requires multiple parameters which can be evaluated in different combination and the high number of interacting variables in each experiment. This will become even more complicated in future when high-parameter research methods such as clustering become routine (26). There are many different clustering algorithms for evaluation of cytometry results. The Flow Cytometry Critical Assessment of Population Identification Methods (Flow-CAP) challenge has made a comparison of performance for flow cytometry clustering algorithms (27). They found that these programs are not accurate enough and too slow for routine use. While specific programs were found to be accurate, slowness rendered them impractical for routine use in clinical laboratories. New algorithms are being developed that address these problems (28).
Relevant parts of the laboratory process are shown in Box 1.
Box 1. The laboratory process.
• The pre-analytical phase. Functional assays and some differentiation markers are time- sensitive and require an analysis to be performed within a few hours of blood draw whereas some analyses can still be correctly performed within 72 h. This must be validated for each parameter that is being analyzed.
• In the analytical phase, almost all items to be reported in standard operating procedures (SOP) (including linked documents) are themselves still in need of standardization, including protocol design, international references, operator confirmation, and analytical performances as well as description of the assay principle, validation process, and supervisor authorization.
• The post-analytical phase comprises (i) the technical review of examination results as well as (ii) a plausibility check of the results prior to release. A major issue of post-analytics is to provide valid reference ranges or decision limits.
The major error sources in FCM (Box 2) are related to (i) sample quality, (ii) protocol and panel design, (iii) methods used for instrument settings, standardization, discrimination of negative or positive populations and absolute counting and (iv) data analysis and interpretation (29). Panels must be well-designed and spectral overlap must be sufficiently recognized and properly compensated (30, 31).
Box 2. Error sources in flow cytometry.
• Daily instrument variation is at risk and must be measured and minimized as much as possible by tracking instrument and reagent stabilities. For clinical labs, CE-labeled cytometers should be used, and manufacturers' advice must be followed.
• Protocol outlines for sample preparation, fluorophore detection and gating strategy are often ill-defined and lack consensus.
• One analysis simultaneously identifies several cell sub-populations and provides as many results. Unlike in most diagnostic tests, one analysis does not mean one result.
• Phenotype definitions are not univocal and are constantly changing. There is no international “gold standard” for determining accuracy in terms of phenotype or absolute quantitative measurements.
• Some analyses such as leukemia typing, or functional investigation require several assays (protocols) and their interpretation require the integration of information from the multiple assays.
• Specificity of antibodies used for the detection of antigens may vary depending on the clone, conjugate, and manufacturer. In contrast, different clones can recognize the same antigen and can be certified through the Human Leukocyte Antigen determination program (32).
• There are many different typical phenotypes that need to be identified in the diagnosis of all possible diseases. Samples are frequently scarce and include bone marrow, punctates, and other biological fluids in addition to various anticoagulated blood. All these samples must be fresh for analysis. It is therefore not possible to have internal quality control (IQC) for each analysis, sample type, or pathological phenotype. However, a few IQC are commercially available, mainly for CD4+/CD8+ T cells or CD34+ stem cells. These IQC can be stored for weeks thanks to stabilizing treatment. Not all cell types could be investigated, and specific needs for immune oncology are not yet met.
• As a result of the continuously evolving landscape of biological understanding, new therapies and technological capabilities, newly optimized antibody combinations must often be incorporated into FCM assays. It is therefore important that protocols must their flexibility.
• Although samples are prepared and analyzed in parallel and several batches can be analyzed in 1 day, each sample is prepared individually with independent risks of error and variability. The analysis of one test within a batch does not depend on the whole batch as it is for microtiter-based serological immunoassays with one common standard curve. The validation of IQC inside the batch does not full guarantee the quality of each analysis. Inversely, a successful analysis on one sample, including eventually one IQC does not necessarily mean the entire batch is valid.
• For the same reasons, external quality assessment (EQA) schemes are rare (http://www.eptis.org). The majority are only available for a small number of analyses, in preserved (meaning altered) conditions. Schemes providing fresh blood samples are rare and expensive (http://www.instandev.de/en.html).
• In absence of international references, absolute counts (in cell concentration or antigen density as well) slightly differ according to the system used as shown in EQA comparisons (33).
• The risk for contamination between samples is not negligible. Samples in a batch can have extreme concentration of at least one cell subset. The sample-to-sample contamination risk depends on the organization of the sample preparation (proximity of the tubes, changes in tips or probe cleaning, and on the efficacy of the probe washing between two consecutive samples.
Types of Flow Cytometric Assays
Quantitative analyses allow the quantitation of precisely defined cell subsets, even as absolute values. Some EQA and standardization guidelines are available. They can address rare events with a need for high sensitivity (low LoQ).
Quantitation of very rare events has recently been developed for the assessment of residual disease and requires precautions to obtain good repeatability at high sensitivity. A minimum number of parameters and a minimum number of positive events to be recorded are required, which means that the sensitivity up to 0.01 or even 0.001% of leukocytes can only be achieved if at least 3 × 105 to 3 × 106 events are acquired (34). In Table 1, cell counts to be analyzed when quantifying rare cells are shown.
Most of FCM analyses are qualitative in nature. They mainly address the identification of cells, such as the diagnosis of leukemia and lymphoma, immune monitoring, or in proliferative or dysplastic disorders. Partial quantitation (%) is then determined and informative but not clinically critical. Standardization and EQC are frequently not available and IQC are rare. Measurement of precision, accuracy, or working range is not relevant.
Functional analyses usually require challenging fresh samples with different stimulants. In this case, quantitation is important but rarely standardized. Calculation of precision is done by repeating stimulations. The working range can be estimated by testing different concentrations of the stimulant. Sensitivity is estimated by the lower stimulation dose giving a significantly different readout from the negative control. Comparing positive and negative controls offers information of reproducibility of the assays and the frequency of “non-responders” observed for some assays. Measuring accuracy is generally not possible. Inter-laboratory comparison is difficult to organize as samples must be tested within 1 day. Standardization and multi-center clinical evaluations are needed.
Validation of Flow Cytometric Assays
Based on the specific characteristics of FCM mentioned above, procedures must be adapted to render method validation more efficient but realistic in daily practice. First, analytical and clinical validation must be distinguished. Clinical validation (diagnostic accuracy, e.g., sensitivity and specificity) is commonly based on clinical studies. Patient data are usually not accessible for laboratories. This is not the scope of this paper but is briefly shown in Table 2.
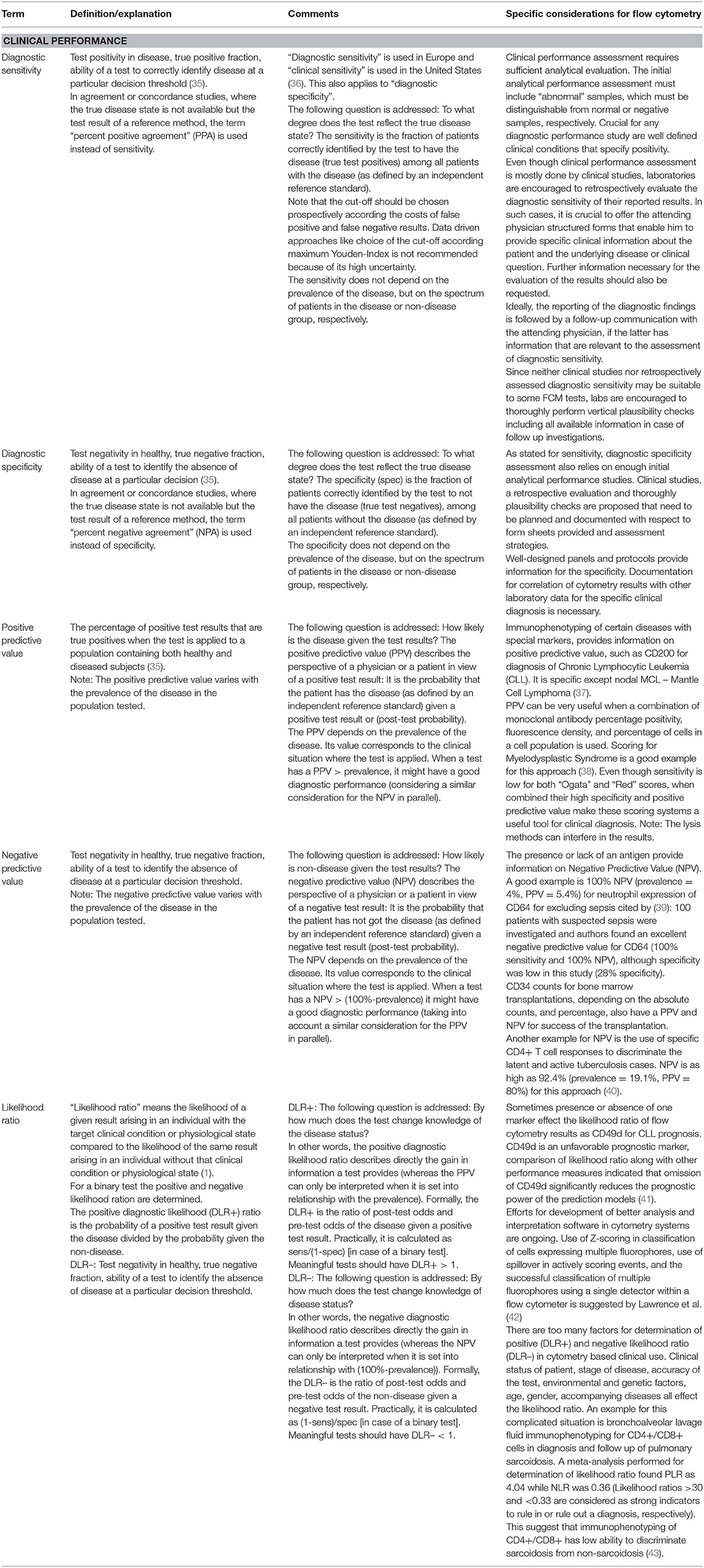
Table 2. Clinical performance characteristics given by EU-IVD-R that shall be stated by manufacturers to state “fitness for purpose” need to be maintained during the lifetime of an IVD.
Parameters for Validation
Analytical parameters for a specific assay must be determined independently in each laboratory that performs the assay. This should include, if applicable, analytical sensitivity and specificity, trueness (bias), precision, repeatability, intermediate precision, reproducibility, accuracy (resulting from trueness and precision), limits of detection, limit of quantitation, measuring range, linearity, cut-off, determination of appropriate criteria for specimen collection and handling, control of known relevant endogenous and exogeneous interference (cross-reactions), and robustness. Definitions and specifics for FCM are given in Table 3. Analytical performance characteristics given by EU-IVD-R that shall be stated by manufacturers to state “fitness for purpose” need to be maintained during the lifetime of an IVD. As commented in this table, although it should be noted that not all performance characteristics can be validated for every flow cytometric setting. And, finally, even if it would be feasible, the full method validation for each modified or novel analysis, each sample type, and each pathological issue would be outrageously expensive and time-consuming. For transparency reasons, we recommend to document which characteristics were not validated and the underlying reasons.
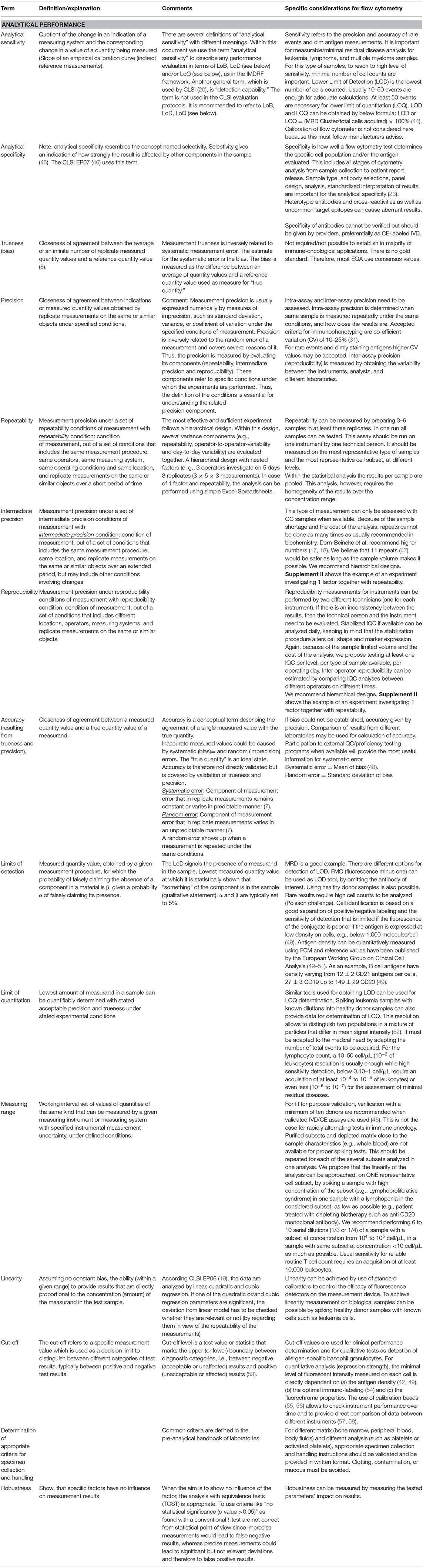
Table 3. Analytical performance characteristics given by EU-IVD-R that shall be stated by manufacturers to state “fitness for purpose” need to be maintained during the lifetime of an IVD.
Performance Targets (Table 4)
For a validation, we must define acceptance criteria in advance as part of the validation plan. Performance targets must enable the reviewer of the validation data to state whether the determined performance capability is adequate for the intended use or not. In some cases, the assessment may lead to the conclusion that further investigation is necessary or that restrictions exist for the analytical procedure that need to be considered in routine diagnostics.
There are only few international recommendations for tolerated variability in flow cytometric diagnostics. As a rare example, references are proposed in Westgard data base for CD4+ T cells counts although no technical conditions are defined such as system used, internal standards, or even units that are critical in Quality Assurance of the technique as discussed before (15, 59).
Experimental Set-Up
The design of validation experiments must follow general rules but can be adapted if necessary. Especially, very often the small number of samples, the limited time in which the samples can be processed, and the small volume accessible are limiting factors. The best options to overcome this are multi-sample or multi-center approaches. The aspect of sample size as an important part of experimental design is mentioned below.
Statistics for Validation Experiments
There have been strong efforts to improve the quality of statistical approaches in design and analysis of method validation experiments in the last years. There are four principle features of statistical methodology which should be considered (Box 3).
Box 3. Four principle features of statistical methodology
1) Stringent use of prospectively defined acceptance criteria, which are used as limits in later statistical tests.
2) Any result (statistical term: estimate) should be reported together with its uncertainty, typically expressed as a confidence interval (CI). Within the framework of statistical analyses, the location of the CI is considered in comparison to the acceptance criteria. If the confidence interval does not overlap with the acceptance limits, the validity is proven. It should be noted that conclusions can only be drawn in this direction: if an acceptance criterion is within confidence interval, no conclusion is possible.
3) We therefore recommend the application of equivalence tests: often, the aim is to show a difference of zero, e.g., in experiments evaluating robustness or selectivity, where the results of distorted measurements should be equivalent to results of an undistorted control experiment. After establishing acceptance criteria prospectively, the CI of the difference of distorted and undistorted measurement results should be within acceptance criteria around zero (Figure 1). The related statistical test is the TOST approach (two one sided t-tests, see Supplement III for details) (60).
4) Finally, sample sizes should be determined by power calculations. Statistical tests differ in their robustness to small numbers of cases. The user should know and estimate the behavior of the algorithms used. Procedures that are more reliable for small case numbers should be preferred. An example is given for robustness in Table 5. The sample sizes required for sufficient test power should be known before validation. The resulting test power should be included in the evaluation, especially if the sample size is smaller. Practically, the sample size is determined using software, formulas, statistically derived recommendations as CLSI-guidelines (19– 21) and tabulations (see Table 5 for TOST in this paper). We cannot recommend oversimplified so-called practical approaches (“ <5 replicates were found adequate to validate assay imprecision levels below the 5–10% CV” (61). Here, simulations (62) performed on common spreadsheet software or R could be helpful, Figure 2 shows such considerations for uncertainty of standard deviations one could achieve in simple repeat experiments when 3, 5, 10, 20, and 50 replicates are used.
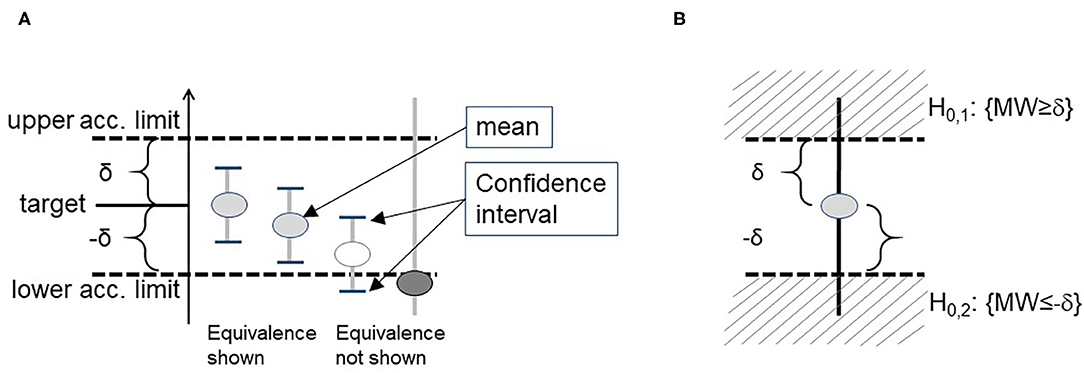
Figure 1. Demonstration of a statistically proof using confidence intervals (A). When this problem is formulated as a statistical test, it refers to the two 1-sided test approach (TOST) (B).
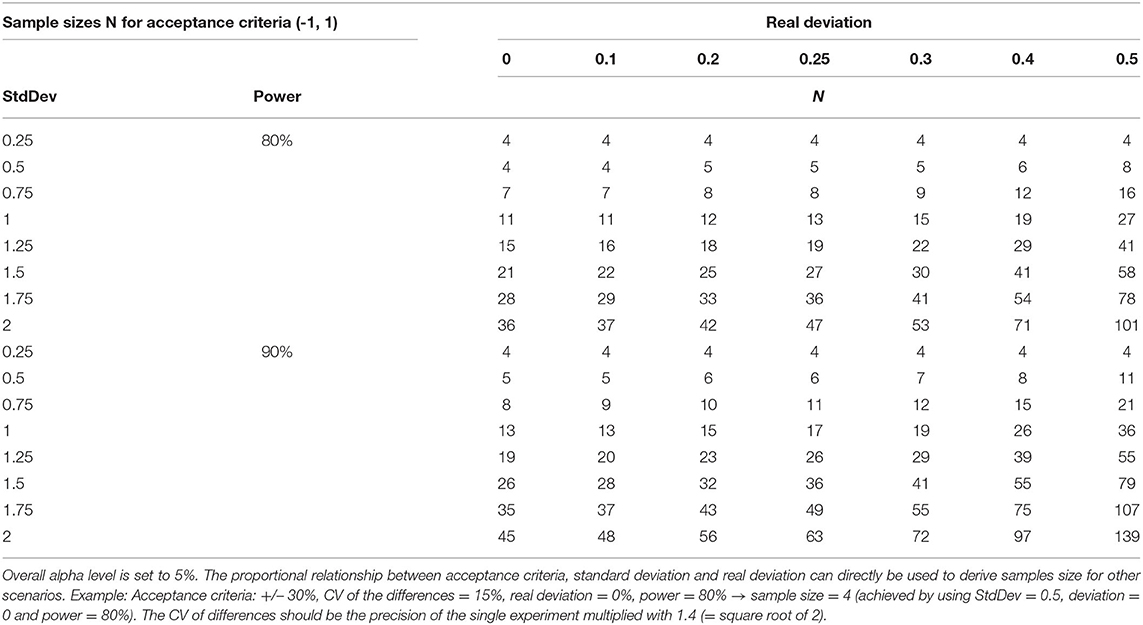
Table 5. Sample sizes necessary to demonstrate equivalence via TOST in a paired design when acceptance criteria cover the range (−1, 1), in dependence on standard deviation of the pairwise differences, real deviation, and power.
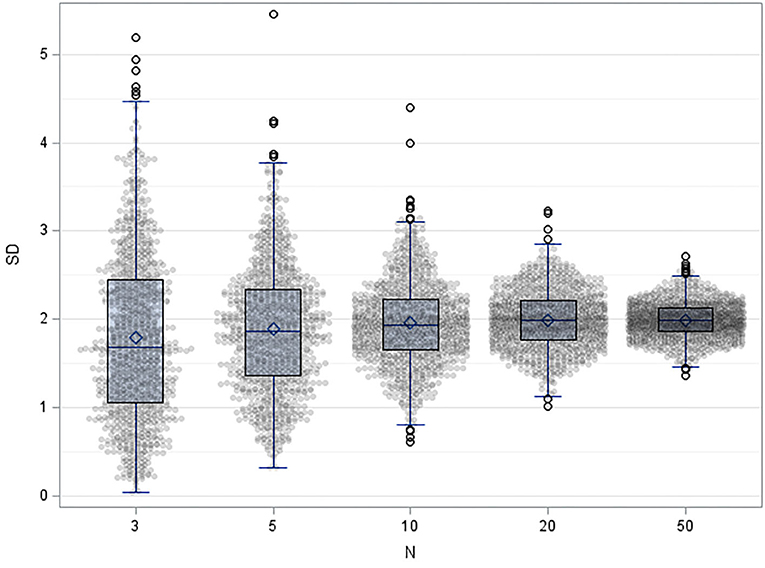
Figure 2. Result of 1,000 simulation of results of repeatability experiment when 3, 5, 10, 20, and 50 replicates are used, with mean=10 and standard deviation =2, shown as dot-plots with overlying Box-whisker plots.
In addition to statistical methodology for analysis of validation experiments, the following practical aspects of analysis should be discussed:
• Deviation from normal distribution: Statistical tests determining deviations from normal distribution are not useful for demonstrating a lack of normal distribution. One can apply visual inspection of histograms (no outliers, symmetrical gauss-shaped distribution, or QQ-plot presenting a straight line). Moreover, one can use the fact, that replicates of a measurement are very often normally distributed. Finally, a transformation of data could be useful (see below).
• Outliers or better “aberrant values”: Statistical methods could help to identify whether an aberrant value is an outlier, however, the decision whether the outlier has to be incorporated in the data is not a statistical task, since an imperfection of the method, e.g., to handle matrix effects, could be the reason. Rules how to handle outliers must be defined in advance. An easy way to enlighten the situation is to perform the measurements in duplicates and in a random order: when both replicates are aberrant values although they were processed on different positions in the work flow, they cannot regarded as outliers but to be real values. When only one of the replicates is aberrant, it might be an outlier which can be handled according the internal SOP how to handle outliers.
• Counting data like single cells or particles, especially in the low range (1 … ~ 20) follow the Poisson distribution. This distribution has some specific properties in that large imprecision is just given by the distribution and cannot be improved by experimental efforts. It is out of the scope of this report to address the specific approaches necessary for Poisson-distributed data, see (63–65) for further reading. Note that square root transformation of count data is helpful within statistical analysis (66) in the same sense as log-transformation is often applied.
• In case of low sample sizes one can statistically average (other term: pool) the results over the samples. An example are precision analyses: If only a small number of replicates are available per sample, a pooled precision can be calculated as the square root of the sum of squared standard deviations (or by specific methods related to variance components). We refer also to the next chapter, §4, and to Supplement II). However, homogeneity of the variances (standard deviations do not systematically depend on concentration) is a prerequisite for the pooling and—if not given—could be achieved by appropriate transformation of data (ln, square root).
• ln-transformation: In case of natural log (ln)-transformation, the standard deviations obtained for ln-transformed data can directly be read as CV in the originally scaled data (for instance: SD=0.1 in ln-transformed data CV=10%in originally scaled data, valid up to 30% CV).
Evaluation of the Results
Validation is successful when the acceptance criteria are met. If these performance criteria are not met, this may be for the following reasons: (1) the estimated target value is outside of the criteria, (2) uncertainty of the target value is too high and does not allow a decision, or (3) representative samples are absent in the experiment (e.g., missing positive specimen). Whereas, in case 1 the method itself must be modified, in both latter cases, an extension of the validation process can be indicated. A common approach is a two-step clearance procedure with an extended sample collection phase that increases the sample size by continuously evaluating the results of measured patient samples and accompanying data on quality assurance. In such cases, the completion of the validation process should be declared preliminary and clear instructions should be given on the measures still to be taken. The reservations resulting from a preliminary clearance status should be formulated and reported to the customers.
Our Proposal for the Introduction of Laboratory Developed Tests in Accredited Laboratories
Considering all difficulties in the accreditation process of FCM analysis and all discussions in dedicated meetings, we propose a reasonable and pragmatic solution (Table 4). We also include the consideration that the majority of samples with pathological phenotypes are rare or only available in small volumes and cannot be tested too many times for repeatability and reproducibility.
1. New antibodies are often only available in research-only vials. They are not always labeled with the desired fluorochrome. To check the specific binding, it has proven to be best to use two different or differently labeled antibodies in the validation phase. In addition, Full Fluorescence Minus One control (FMO) must be used to ensure that there is no spill-over into other channels.
2. The reagent quality is guaranteed by the manufacturer, but some alteration can appear during the delivery from the provider to the laboratory according to the conditions. The basic requirement is a stable measuring instrument, which is ensured by daily checking with fluorescent beads. Furthermore, fluorescence intensity of novel antibody batched should be checked with antibody binding standard beads. It would be a huge endeavor to check each single vial before doing analysis but daily checks of the fluorescence intensity of control blood is a good way to validate not only the reagent quality but also the labeling process and the state of the sample. The proper labeling can be easily checked by using a pre-recorded template where each cell populations should fit into the gates positioned at the usual place. So, it is critical to validate each analysis with checking all dot plots graphs.
3. The premix stability must be compared to freshly mixed antibodies on a fresh sample or following IQC. Because labeling intensity may gradually decrease with time, not only population phenotypes but also median fluorescence intensity should be compared.
4. Cells are analyzed from different sample types. The analyses are similar to each other within prespecified acceptance criteria regarding the sample type excepting some minor adaptations for the sample preparation. We recommend doing the method validation on one of the most representative type of samples such as peripheral blood or bone marrow aspirate. Sample types which are explicitly unsuitable for the considered test, but which may arrive in the laboratory should be specified and the reasons leading to the rejection of the order should be described.
5. Several cell subsets are analyzed in one analysis (one analysis, several results). However, each subset cannot be fully tested individually. As all subsets are exposed to the same preparation and same risks of errors, we propose to consider that the performances observed for two representative subsets and one type of sample can be used as reference for Quality Assurance for the analysis of the other cell subsets and sample types. The selected sample type should correspond to the most frequently occurring ones. Subsets chosen should be of clinical relevance. Expected values should cover a wide measurement range or at least include both low and high measurement signals.
6. The effect of transportation and storage on sample stability must be tested typically on 10 samples for the acceptable storage duration (2–3 days, dependent on target cells). Again, TOST approaches are helpful for the analysis: the mean of deviations due to a possible instability should be within predefined limits around zero. Modern approaches include using a regression analysis and setting the confidence band of the regression line into relationship with prespecified acceptance criteria (67).
7. Carry-over can be evaluated by measuring consecutively 3 times the sample with the highest content (e.g., Lymphoproliferative disorder) and 3 times the sample with the lowest content (e.g., depleted sample in biotherapy) the day they are both available. The high values should be at least 100 times higher than the lower content. As the risk does not depend on the subset identification, it can be extrapolated to all other subsets. perform the experiment in at least 3 cycles and use non-inferiority testing (= one sided equivalence test) for statistical analysis (68).
8. Bias estimation/method comparison: When two or more instruments are used independently or as backup in case of instrument malfunction, assays should be performed repeatedly on both machines for comparison. In clinical FCM, number of repeats is often limited by the number of samples required for valid results, therefore alternative procedures must be found. Statisticians commonly recommend performing at least 30 assays on both systems and the CLSI EP 9 guidance (69) recommends using 40 samples for the laboratory and 100 samples for the manufacturer. When the TOST is used for analysis of difference plots (Supplement III), sample sizes provided in table 5 can be used. For analysis Bland-Altman plots (70, 71) as well as specific regression methods like Passing-Bablok regression (72) or Deming regression are recommended (73). Note that simple ordinal linear regression as well as the correlation coefficient r2–although often used—are not appropriate (74, 75). Especially the r2 does not detect proportional and constant biases, e.g., one could achieve a r2=1 even when one method measures the double of the other method. For analysis the TOST or similar approaches are helpful. In the Bland-Altman plot the CI of the mean of sample-wise differences should be within predefined limits around zero. When regression methods are applied, the CI of the slope should be within predefined limits around 1 and the intercept within predefined limits around zero, or the CI of biases calculated from the regression line vs. line of equality at specific concentrations (typically 3 values within the measurement range) should be within predefined limits.
9. Precision: The most effective way to estimate several components of variability follows a hierarchical design with nested factors (e.g., 3 operators investigate on 5 days 5 replicates (3 × 5 × 5 measurements) (21). Within this design, several variance components (e.g., repeatability, operator-to-operator-variability, and day-to-day variability) are evaluated together (Supplement II). Especially repeatability is pooled over several experimental units. In case of one parameter and repeatability, the analysis can be performed using simple spreadsheet-software like MS Excel. It is also possible to pool the results over several samples and use fewer replicates within the factors, however, homogeneity of variances must be achieved for the analysis then, eg. by transformation of the measurement values (ln, square root). One should note that the CI-approach (which would use the one-sided upper confidence limit here) is not common in precision evaluations in the laboratory medicine community. It was shown that the level of variability was mainly related to the size of the population. Accordingly, Tosato et al. (76) described a CV of 2% for large T cell populations, 5.5% for B cells, and 12.5% for NK cells in 10 independent measurements of an IQC for clearly defined markers (Immuno-Trol Cell Control; Beckman Coulter).
10. In the absence of any international standard to validate EQA samples, accuracy can often be approached only by inter-laboratory comparisons in EQA. The targeted accuracy (EQC bias) should be below 15%.
11. Calculation of measurement uncertainty combines reproducibility and accuracy. Because of the rarity of EQA, we propose to use IQC for this calculation. When investigating measurement uncertainty, it must be considered that the various cytometric stains used are not independent variables. This influences the propagation of errors in a positive way (25).
12. As discussed, the determination of the complete working range is not possible. We propose that the linearity of the analysis can be approached, on ONE representative cell subset, by spiking a representative cell line into one sample with a low count in the considered subset. We recommend performing 10 serial dilutions. The usual sensitivity for reliable routine T cell count requires an acquisition of at least 10 000 leukocytes.
13. Definition of limit of quantitation (LoQ) must be adapted to the medical need by adapting the number of total events to be acquired. For the lymphocyte count, a 10–50 cell/μL (10e-3 of leukocytes) resolution is usually enough while high sensitivity detection, below 0.10-1 cell/μL require an acquisition of at least 10e-4 to 10e-5 of leukocytes) or even less (10e-6 to 10e-7) for the assessment of minimal residual disease.
14. Robustness, specificity: When measurements of distorted and not-distorted samples must be compared, it is the aim to show a missing difference. As introduced and explained above, the TOST can be used to show the equivalence. Depending on the design, paired or unpaired measurements must be regarded, whereby a paired design is more powerful. Beside other software, free of cost MS Excel-tools are available (https://www.acomed-statistik.de/en-gb/statistical-tools-download.html#TOST). The sample size depends on width of interval included by acceptance criteria, the expected real difference and its standard deviation as well as on the assumed α (typically 5% and β errors (typically 10–20%). The following Table 5 provides sample sizes for a paired design (all samples are measured under both conditions; the difference of both results is evaluated in analysis). Supplement III provides an example.
15. Reference ranges can be preliminarily calculated from 31 to 35 assays, however CLSI guideline EP28 (77) recommends 120 to 135 healthy donors. The CLSI recommendation refers to a non-parametric estimation of percentiles. Lower sample sizes require the application of complex parametric methods (78). As the reliability of reference ranges is limited if the proposed sample size used, the 90% confidence interval of both lower and upper reference interval limits should be calculated and critically reviewed (10, 11). By doing this, an inappropriate sample size becomes obvious. Even in case of recommended sample sizes the CI are surprisingly wide. More accurate determination specific to the population to be tested (e.g., babies/children, elderly over age 75, or gender) cannot be measured in each lab for practical, economical, and ethical reasons and can be taken from international data available although they are rarely standardized (79–83). Here, quantile regression for age groups is superior but not realistic for most laboratories. A simplified proposal has been described by Özcürümez et al. (84). For complex phenotypes, subset identification regarding antibody combination and gating strategy must be clearly described in the SOP. Gating strategy must be double-checked repeatedly. A simple tool is the control of the quality of the sample in FSC/SSC plots and each single labeling vs. SSC that gives information on the quality and specificity of the immunostaining (85–88).
16. As accreditation is a continuous process, we propose method validation should be repeated periodically. If established, an IQC program should be done every operating day. Precision, working range, and contamination should be checked repeatedly every 1 or 2 years. Normal ranges should be verified every 10 years.
Document Hierarchy
All method descriptions and characteristics must be reported in detail and continuously updated in the accreditation records, SOP, and LIMS. These reports must be easy to read and in a fixed layout.
Because of protocol flexibility and frequent evolution in FCM, details on the method description must be frequently updated. Typical examples would be:
• Removing or replacing an antibody or one clone or
• Adding a washing and red blood cell lysing step, if incomplete lysis was occasionally observed in some samples.
If the same information is cited at different positions along the accreditation forms or in the LIMS, there is a very high risk for discordance. Redundancy severely impairs readability and makes document maintenance risky and error-prone and consequently should be avoided as much as possible.
Lots of facts are common to several assays, e.g., environment, the instrument characteristics, the method principle, procedures on standardization, sample preparation, samples/reagents management, security, and risks. Results of different sub-populations are frequently complementary subsets of some parent populations. Several combinations of antibodies (panels) can have common features. As an example, a panel for diagnosis of leukemia can require 6–8 assays with a common backbone. Multiple results are produced and should then be considered together for interpretation. An accreditation report must combine multiple results (one analysis—several results) or possibly multiple assays as a panel (several analyses—one result), in the same file and preferentially lists of information are presented in a table for readability.
For efficacy and safety reasons, we propose organizing the documents on 4 different levels (Figure 3):
1. Any common information must be gathered (“factorized”) in a common “generic” accreditation form as much as possible.
2. The specificities (reagents, method, performances) must be detailed in analysis-specific forms: One analysis “one analysis—several results” or “several analyses—one result” in one common accreditation form
3. The technical specificities required for daily practice at the bench and interpretation (gating strategy, reagents specificities, etc.) must be specified in the analysis-specific SOP.
4. The information necessary for interpretation and a report with the results (reference values, LoQ, units, etc.) must be collected in the LIMS.
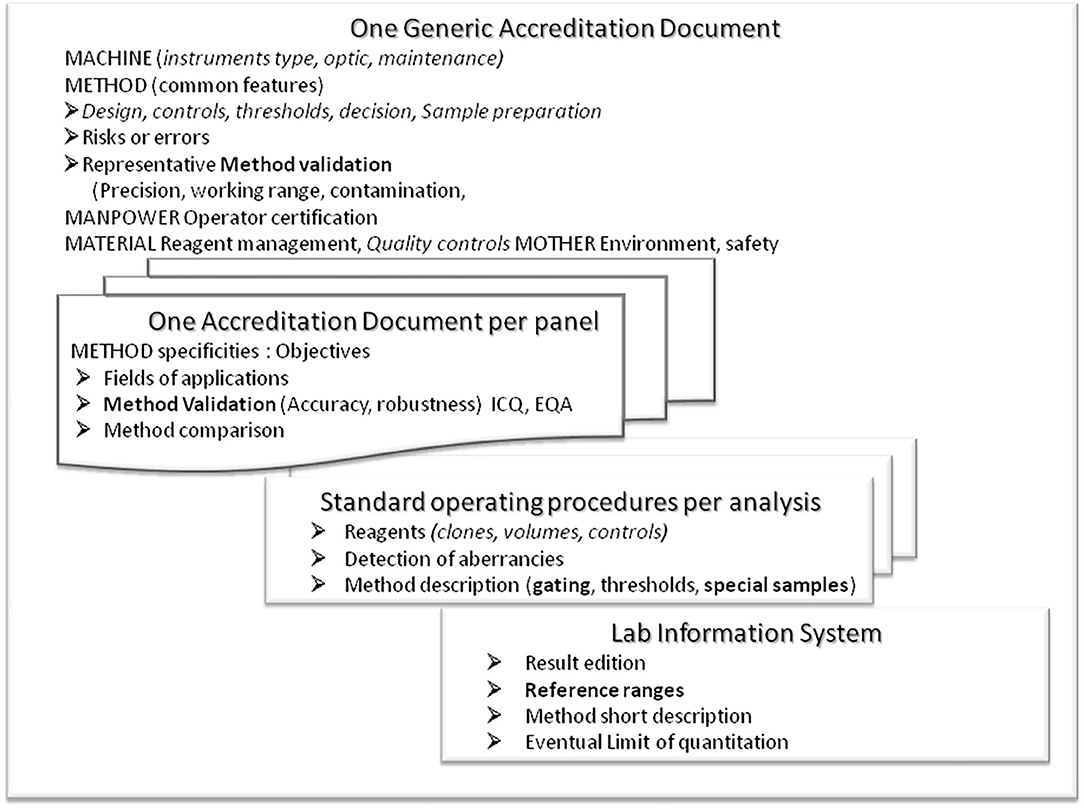
Figure 3. Presentation of the structure proposed for the accreditation documents. A generic form is to record and report all common information (including environment, material, management, manpower) and method characteristics that cannot be tested for each panel. Then specific forms should be written individually per panel (several parameters, several assays). Technical details (antibodies, clones, conjugates, gating strategy, risks of error, and guidelines for interpretation) should be presented in an easy-to-update SOP. Results with technical and reference information should be managed by the laboratory informatics system to be published for correct interpretation. Any redundancy should be avoided for safety and management reasons.
The generic description must mention all common critical points; operators and supervisors (education, training, CPD/CME, information), environment (storing requirement; work space ergonomics, hygiene, air quality, humidity, room temperature), measurement principles, material management (reagents, standards and samples; conditioning, storing, transportation, label/identity, acceptability/rejection, registration, tracking); instrument characteristics including cytometer and accessory instruments, optical bench, instructions, daily checks for fluidic and optical stability, principles for settings, spectral overlap compensations, standardization of signal detection, check-up, maintenance. Some common components of method validation can also be gathered in this generic form such as sample preparation including process for immuno-labeling, washes, red blood cell lysis, fixation, storing, calibration, absolute counting strategy; units, standards, data acquisition, interpretation; reference to peer recommendations (ICSH), quality control management, risks of error, result validation, recording, transfer, and reporting. Part of the method characteristics is also common. Risks of Error (RoE, caused by pipetting errors of antibodies or internal standards, incomplete lysis of red blood cells, clots, centrifugation, cell loss), and effects on fluorochromes (between fluorochromes, energy transfer, steric hindrance, matrix effects such as bile salts or antibodies to fluorochromes), their detection (minimal count of cells, correct cell location in dot plots) and their prevention and correction must be listed. Most RoE are common to all FCM analyses and thus should be detailed in the generic form rather than in the panel-specific information. Lists of technical parameters/materials (antibodies, fluorescence dyes, clones, provider, concentration) must be presented in tables that are easier to read instead of text and attachments.
The analysis-specific records must include the specificities for the environmental conditions and method (lysis, washing steps, internal standards, dyes, templates, expected normal, and aberrant populations) and should be conceived according to clinical relevance (awareness for doublets or dead cells relevant, relevance of percentages of absolute values, delta check, limit of detection). If required, these forms can also merge data from different analyses like non-stimulated and stimulated cells or different panels for the distribution of T cell clonotypes. These analyses are usually closely related, sharing many features (sample type, incubation steps, lysis, washing buffers, centrifuge, incubation). Each detail that can be changed or adapted frequently should not be included here like reagent lots, pipetting, volumes respective cell numbers of cells, additional washing steps, rare sample types), but in the SOP. These specific forms (per analysis) should also contain as much as possible information on analysis characteristics. Some assays validation could be approached from a related analysis (working range, linearity, limit of quantitation) that cannot be done for all analysis but can be extrapolated from other analyses and described in the generic form (like absolute count linearity, limits of detection, or contamination. This is also true for common errors (like pipetting, reagents quality, centrifugation, red blood cell lysis, cell separation procedures, washing).
The SOP must detail all technical specificities, the method principles, specific reagents (references, isotypes, clones, providers, fluorochromes, and conjugated antibodies), concentrations (based on titration or manufacturer recommendations), calibration, specific requirements on sample preparation, acquisition parameters (delay, number of events to acquire), and expiration date. As phenotype definition is critical, each subset should be clearly described (antibody, gating strategy, population hierarchy) and be referred to peer literature when available. FSC/SSC plots provide valuable information on the sample quality and debris. Doublets and dead cells must be excluded from analysis. This is easily done for dead cells because a live/dead staining such as 7-Aminoactinomycin D or aggregation of dead cells helps to exclude them. Doublet exclusion can be done by gating scatter height vs. area. Population overlap (e.g., lymphocytes and monocytes) must be avoided by gating strategies such as Boolean gates. Backgating and use of color codes are good tools to check the quality of the gating. The template with typical results including dot plots, level of fluorescence intensity expected, and most common and atypical types (sub-populations) should be described. It is recommended that the template include “alert gates” for unexpected combinations to provide a signal in case of improbable phenotypes.
LIMS should include all information needed to interpret the results. Subset definitions, LoQ, reference values must be listed in the data management system (LIMS).
As discussed, operator competence in FCM directly relates to quality assurance. Different projects supporting education and certification at an international standard are under development by various international societies: ESCCA, ICCS, or ISAC. The educational sessions (courses, congresses, etc.) visited by staff members should be clearly described and competence should be tested. All documents must be archived.
Educational Sources
FCM technique is rarely formally taught in general biological fields and even less in diagnosis. Only a few countries grant certificates or have study programs in this specific technique like the French University Certificate on Cytometry. The International Society on Analytical Cytometry (ISAC) proposes an internationally recognized qualification in basic cytometry (International Cytometry Certification Exam (http://cytometrycertification.org/) with continuous follow up. The International Federation of Clinical Chemistry and Laboratory Medicine (IFCC) offers courses and schools, organized by the working group flow cytometry WG-FC (http://www.ifcc.org/). The European Society for Clinical Cell Analysis (ESCCA) promotes continuous education and training in annual international schools and courses as well as professional development and evaluation on specific topics. In 2017, ESCCA has initiated an examination for their members to become an ESCCA-certified cytometrist. ESCCA European cytometry certification includes two levels of certification, one for cytometry operators and one for cytometry specialists (http://www.escca.eu).
Conclusion
We propose a “generic” accreditation method for all common steps (instrument settings, protocol design, and data analysis and decision strategy), a detailed description of each method (protocol, RoE), and quantitative validation of a few representative methods. More detailed and frequently updated data such as reagent characteristics, gating strategy, typical results, and reference data must be described in the SOP and, in part, also in the LIMS. The flow cytometry technique is entering a mature state with better-defined methodology for instrument settings, protocol design, standardization, and data analysis and interpretation. Nonetheless, because of its large scope and flexibility and for economic reasons, FCM accreditation procedures must be pragmatic, feasible, and efficient. Our proposal also defines several premises for further harmonization of the processes connected with the validation of FCM assays. In a next step, for instance, the community of laboratories that frequently perform such validation routines could now compile a collection of sample records and may develop “best practice” templates for the evaluation of validation data.
Data Availability Statement
All datasets generated for this study are included in the article/Supplementary Material.
Author Contributions
CL, GY, TK, FP, KP, MS, MÖ, and US wrote parts of this manuscript, double-checked the submitted draft, and agree to be accountable for the content of the work.
Funding
The author(s) acknowledge support from the German Research Foundation (DFG) and Universität Leipzig within the program of Open Access Publishing.
Conflict of Interest
TK is the owner of Acomed Statistik.
The remaining authors declare that the research was conducted in the absence of any commercial or financial relationships that could be construed as a potential conflict of interest.
Acknowledgments
The authors would like to thank the scientific community supporting them. CL, FP, GY, and US are members of the European Society of Clinical Cell Analysis (ESCCA) working group on Primary Immunodeficiencies. CL, KP, MS, and US are members of the IFCC working group on flow cytometry. MÖ, TK, and US contribute to the working group Entscheidungslimits/Richtwerte of the DGKL. MÖ and US are consultants of accreditation bodies. The authors acknowledge support from the German Research Foundation (DFG) and Leipzig University within the program of Open Access Publishing.
Supplementary Material
The Supplementary Material for this article can be found online at: https://www.frontiersin.org/articles/10.3389/fimmu.2020.02169/full#supplementary-material
Abbreviations
CAR-T, Chimeric antigen receptor transduced T lymphocytes; CE, Conformité Européenne; CI, Confidence interval; CLSI, Clinical and Laboratory Standards Institute; CME, continuing medical education; CPD, continuing professional development; CV, coefficient of variation; DLR, diagnostic likelihood ratio; EQA, external quality assessment; EQC, external quality control; ESCCA, European Society for Clinical Cell Analysis; EU-IVD-R, European Regulation on in vitro diagnostic medical devices; FCM, flow cytometry; FMO, fluorescence minus one; FSC, forward scatter; ICCS, International Clinical Cytometry Society; ICSH, International Committee for Standardization of Hematology; IMDRF, International medical device regulators forum; IQC, internal quality control; ISAC, International Society for Advancement of Cytometry; IVD, in vitro diagnostic medical devices; LDT, laboratory developed tests; LIMS, laboratory information management systems; LoB, limit of blank; LoD, limits of detection; LoQ, limit of quantification; MRD, minimal residual disease; QQ-plot, quantile-quantile-plot; RoE, risks of error; SD, standard deviation; SOP, standard operating procedure; SSC, side scatter; TOST, test of one-sided significance; VIM, International vocabulary of metrology.
References
1. European Union. Regulation (EU) 2017/746 of the European Parliament and of the Council of 5 April 2017 on in vitro diagnostic medical devices and repealing Directive 98/79/EC and Commission Decision 2010/227/EU. In: L 117/176. (ed.) European Union. Official Journal of the European Union (2017).
2. MIFlowCyt. Minimum information about a flow cytometry experiment (MIFlowCyt) checklist (Numbered in accordance with MIFlowCyt 1.0 document). Cytometry A. (2010) 77:813. doi: 10.1002/cyto.a.20941
3. Bhagwat N, Dulmage K, Pletcher CH, Wang L, Demuth W, Sen M, et al. An integrated flow cytometry-based platform for isolation and molecular characterization of circulating tumor single cells and clusters. Sci Rep. (2018) 8:5035. doi: 10.1038/s41598-018-23217-5
4. Maloney DG, Grillo-Lopez AJ, White CA, Bodkin D, Schilder RJ, Neidhart JA, et al. IDEC-C2B8 (Rituximab) anti-CD20 monoclonal antibody therapy in patients with relapsed low-grade non-Hodgkin's lymphoma. Blood. (1997) 90:2188–95. doi: 10.1182/blood.V90.6.2188
5. Darvin P, Toor SM, Sasidharan Nair V, Elkord E. Immune checkpoint inhibitors: recent progress and potential biomarkers. Exp Mol Med. (2018) 50:165. doi: 10.1038/s12276-018-0191-1
6. De Oliveira SN, Wang J, Ryan C, Morrison SL, Kohn DB, Hollis RP. A CD19/Fc fusion protein for detection of anti-CD19 chimeric antigen receptors. J Transl Med. (2013) 11:23. doi: 10.1186/1479-5876-11-23
7. Hegde PS, Karanikas V, Evers S. The where, the when, and the how of immune monitoring for cancer immunotherapies in the era of checkpoint Inhibition. Clin Cancer Res. (2016) 22:1865–74. doi: 10.1158/1078-0432.CCR-15-1507
8. VIM. Technical Terms Given in the Following Recommendations Were Taken From International Vocabulary of Metrology – Basic and General Concepts and Associated Terms. JCGM (2012).
9. ISO. ISO 15189-2012 Medical Laboratories - Requirements for Quality and Competence. Geneva: ISO (2012).
10. Keeney M, Barnett D, Gratama JW. Impact of standardization on clinical cell analysis by flow cytometry. J Biol Regul Homeost Agents. (2004) 18:305–12.
11. Guzel O, Guner EI. ISO 15189 accreditation: requirements for quality and competence of medical laboratories, experience of a laboratory I. Clin Biochem. (2009) 42:274–8. doi: 10.1016/j.clinbiochem.2008.09.011
12. Thelen MH, Vanstapel FJ, Kroupis C, Vukasovic I, Boursier G, Barrett E, et al. Flexible scope for ISO 15189 accreditation: a guidance prepared by the European Federation of Clinical Chemistry and Laboratory Medicine (EFLM) Working Group Accreditation and ISO/CEN standards (WG-A/ISO). Clin Chem Lab Med. (2015) 53:1173–80. doi: 10.1515/cclm-2015-0257
13. Barnett D, Louzao R, Gambell P, De J, Oldaker T, Hanson CA, et al. Validation of cell-based fluorescence assays: practice guidelines from the ICSH and ICCS - part IV - postanalytic considerations. Cytometry B Clin Cytom. (2013) 84:309–14. doi: 10.1002/cyto.b.21107
14. Sack U, Barnett D, Demirel GY, Fossat C, Fricke S, Kafassi N, et al. Accreditation of flow cytometry in Europe. Cytometry B Clin Cytom. (2013) 84:135–42. doi: 10.1002/cyto.b.21079
15. Westgard JO, Barry PL, Tomar RH. Implementing total quality management (TQM) in health-care laboratories. Clin Lab Manage Rev. (1991) 5:353–5.
16. Correia RP, Bortolucci ACA, Lopes ACW, Sandes AF, Azambuja APD, Viana MA, et al. Recommendations for quality assurance in multiparametric flow cytometry: first consensus of the Brazilian Group of Flow Cytometry (GBCFLUX). J Brasileiro de Patologia e Medicina Laboratorial 51, 389–396. doi: 10.5935/1676-2444.20150061
17. Nebe CT, Dorn-Beineke A, Braun P, Daniel V, Ilieva Z, Kuling G, et al. Imprecision and quality control in immunophenotyping of lymphocyte subsets in peripheral blood. J Lab Med. (2013) 37:233–50. doi: 10.1515/labmed-2013-0052
18. Dorn-Beineke A, Sack U. Quality control and validation in clinical flow cytometry. Lab J Lab Med. (2016) 40:65–79. doi: 10.1515/labmed-2016-0016
19. CLSI. Evaluation of the Linearity of a Quantitative Measurement Procedure: A statistical Approach; Approved Guideline. Wayne, PA: Clinical and Laboratory Standards Institute (2003).
20. CLSI. Evaluation of Detection Capability for Clinical Laboratory Measurement Procedures; Approved Guideline. 2nd ed. Wayne, PA: Clinical and Laboratory Standards Institute (2012).
21. CLSI. Evaluation of Precision of Quantitative Measurement Procedures; Approved Guideline. 3rd ed. Wayne, PA: Clinical and Laboratory Standards Institute (2018).
22. ICSH/ICCS. Validation of cell-based fluorescence assays: practice guidelines from the International Council for Standardization of Haematology and International Clinical Cytometry Society. Cytometry B Clin Cytom. (2013) 84:281. doi: 10.1002/cyto.b.21103
23. Selliah N, Eck S, Green C, Oldaker T, Stewart J, Vitaliti A, et al. Flow cytometry method validation protocols. Curr Protoc Cytom. (2019) 87:e53. doi: 10.1002/cpcy.53
24. USP. Statistical Tools for Procedure Validation. (2018). Available online at: https://www.uspnf.com.
25. Cossarizza A, Chang HD, Radbruch A, Acs A, Adam D, Adam-Klages S, et al. Guidelines for the use of flow cytometry and cell sorting in immunological studies (second edition). Eur J Immunol. (2019) 49:1457–973. doi: 10.1002/eji.201970107
26. Lacombe F, Lechevalier N, Vial JP, Bene MC. An R-derived FlowSOM process to analyze unsupervised clustering of normal and malignant human bone marrow classical flow cytometry data. Cytometry A. (2019) 95:1191–7. doi: 10.1002/cyto.a.23897
27. Aghaeepour N, Finak G, Flow CPC, Consortium D, Hoos H. Critical assessment of automated flow cytometry data analysis techniques. Nat Methods. (2013) 10:228–38. doi: 10.1038/nmeth.2365
28. Ye X, Ho JWK. Ultrafast clustering of single-cell flow cytometry data using FlowGrid. BMC Syst Biol. (2019) 13:35. doi: 10.1186/s12918-019-0690-2
29. Burel JG, Qian Y, Lindestam Arlehamn C, Weiskopf D, Zapardiel-Gonzalo J, Taplitz R, et al. An integrated workflow to assess technical and biological variability of cell population frequencies in human peripheral blood by flow cytometry. J Immunol. (2017) 198:1748–58. doi: 10.4049/jimmunol.1601750
30. Wood BL, Arroz M, Barnett D, Digiuseppe J, Greig B, Kussick SJ, et al. 2006 Bethesda International Consensus recommendations on the immunophenotypic analysis of hematolymphoid neoplasia by flow cytometry: optimal reagents and reporting for the flow cytometric diagnosis of hematopoietic neoplasia. Cytometry B Clin Cytom. (2007) 72(Suppl 1):S14–22. doi: 10.1002/cyto.b.20363
31. Wood B, Jevremovic D, Bene MC, Yan M, Jacobs P, Litwin V, et al. Validation of cell-based fluorescence assays: practice guidelines from the ICSH and ICCS - part V - assay performance criteria. Cytometry B Clin Cytom. (2013) 84:315–23. doi: 10.1002/cyto.b.21108
32. Larjo A, Eveleigh R, Kilpelainen E, Kwan T, Pastinen T, Koskela S, et al. Accuracy of programs for the determination of human leukocyte antigen alleles from next-generation sequencing data. Front Immunol. (2017) 8:1815. doi: 10.3389/fimmu.2017.01815
33. Levering WH, Van Wieringen WN, Kraan J, Van Beers WA, Sintnicolaas K, Van Rhenen DJ, et al. Flow cytometric lymphocyte subset enumeration: 10 years of external quality assessment in the Benelux countries. Cytometry B Clin Cytom. (2008) 74:79–90. doi: 10.1002/cyto.b.20370
34. Arroz M, Came N, Lin P, Chen W, Yuan C, Lagoo A, et al. Consensus guidelines on plasma cell myeloma minimal residual disease analysis and reporting. Cytometry B Clin Cytom. (2016) 90:31–9. doi: 10.1002/cyto.b.21228
35. CLSI. Validation, Verification, and Quality Assurance of Automated Hematology Analyzers; Approved Standard. Wayne, PA: Clinical and Laboratory Standards Institute (2010).
36. CLSI. Harmonized Terminology Database. Wayne, PA: Clinical and Laboratory Standards Institute (2020).
37. Sorigue M, Magnano L, Miljkovic MD, Nieto-Moragas J, Santos-Gomez M, Villamor N, et al. Positive predictive value of CD200 positivity in the differential diagnosis of chronic lymphocytic leukemia. Cytometry B Clin Cytom. (2019). doi: 10.1002/cyto.b.21849. [Epub ahead of print].
38. Bento LC, Correia RP, Pitangueiras Mangueira CL, De Souza Barroso R, Rocha FA, Bacal NS, et al. The use of flow cytometry in myelodysplastic syndromes: a review. Front Oncol. (2017) 7:270. doi: 10.3389/fonc.2017.00270
39. Mahmoodpoor A, Paknezhad S, Shadvar K, Hamishehkar H, Movassaghpour AA, Sanaie S, et al. Flow cytometry of CD64, HLA-DR, CD25, and TLRs for diagnosis and prognosis of sepsis in critically ill patients admitted to the intensive care unit: a review article. Anesth Pain Med. (2018) 8:e83128. doi: 10.5812/aapm.83128
40. Harari A, Rozot V, Bellutti Enders F, Perreau M, Stalder JM, Nicod LP, et al. Dominant TNF-alpha+ Mycobacterium tuberculosis-specific CD4+ T cell responses discriminate between latent infection and active disease. Nat Med. (2011) 17:372–6. doi: 10.1038/nm.2299
41. Bulian P, Shanafelt TD, Fegan C, Zucchetto A, Cro L, Nuckel H, et al. CD49d is the strongest flow cytometry-based predictor of overall survival in chronic lymphocytic leukemia. J Clin Oncol. (2014) 32:897–904. doi: 10.1200/JCO.2013.50.8515
42. Lawrence JG, Butela K, Atzinger A. A likelihood approach to classifying fluorescent events collected by multicolor flow cytometry. J Microbiol Methods. (2013) 94:1–12. doi: 10.1016/j.mimet.2013.04.001
43. Shen Y, Pang C, Wu Y, Li D, Wan C, Liao Z, et al. Diagnostic performance of bronchoalveolar lavage fluid CD4/CD8 ratio for sarcoidosis: a meta-analysis. EBioMed. (2016) 8:302–8. doi: 10.1016/j.ebiom.2016.04.024
44. Fuda F, Chen W. Minimal/measurable residual disease detection in acute leukemias by multiparameter flow cytometry. Curr Hematol Malig Rep. (2018) 13:455–66. doi: 10.1007/s11899-018-0479-1
45. Kellner R, Mermet JM, Otto M, Widmer HM. Analytical Chemistry: A Modern Approach to Analytical Science. Weinheim: Wiley and VCH (1998).
46. CLSI. Interference Testing in Clinical Chemistry. 3rd ed. Wayne, PA: Clinical and Laboratory Standards Institute (2018).
47. Lambert C, Sarrat A, Bienvenu F, Brabant S, Nicaise-Roland P, Alyanakian MA, et al. The importance of EN ISO 15189 accreditation of allergen-specific IgE determination for reliable in vitro allergy diagnosis. Allergy. (2015) 70:180–6. doi: 10.1111/all.12546
48. Mandy FF, Nicholson JKA, McDougal JS. Guidelines for performing single-platform absolute CD4+ T-cell determinations with CD45 gating for persons infected with human immunodeficiency virus. MMWR. (2003) 52, 1–13.
49. Bikoue A, George F, Poncelet P, Mutin M, Janossy G, Sampol J. Quantitative analysis of leukocyte membrane antigen expression: normal adult values. Cytometry. (1996) 26:137–47. doi: 10.1002/(SICI)1097-0320(19960615)26:2<137::AID-CYTO7>3.0.CO;2-D
50. Gratama JW, D'Hautcourt JL, Mandy F, Rothe G, Barnett D, Janossy G, et al. Flow cytometric quantitation of immunofluorescence intensity: problems and perspectives. European Working Group on Clinical Cell Analysis. Cytometry. (1998) 33:166–78. doi: 10.1002/(SICI)1097-0320(19981001)33:2<166::AID-CYTO11>3.0.CO;2-S
51. Rossmann ED, Lenkei R, Lundin J, Mellstedt H, Osterborg A. Performance of calibration standards for antigen quantitation with flow cytometry in chronic lymphocytic leukemia. Cytometry B Clin Cytom. (2007) 72:450–7. doi: 10.1002/cyto.b.20359
52. Tangri S, Vall H, Kaplan D, Hoffman B, Purvis N, Porwit A, et al. Validation of cell-based fluorescence assays: practice guidelines from the ICSH and ICCS - part III - analytical issues. Cytometry B Clin Cytom. (2013) 84:291–308. doi: 10.1002/cyto.b.21106
53. CLSI. Assessment of the Diagnostic Accuracy of Laboratory Tests Using Receiver Operating Characteristic Curves. Wayne, PA: Clinical and Laboratory Standards Institute (2011).
54. Davis BH, Dasgupta A, Kussick S, Han JY, Estrellado A, Group IIW. Validation of cell-based fluorescence assays: practice guidelines from the ICSH and ICCS - part II - preanalytical issues. Cytometry B Clin Cytom. (2013) 84:286–90. doi: 10.1002/cyto.b.21105
55. Schwartz A, Fernandez Repollet E, Vogt R, Gratama JW. Standardizing flow cytometry: construction of a standardized fluorescence calibration plot using matching spectral calibrators. Cytometry. (1996) 26:22–31. doi: 10.1002/(SICI)1097-0320(19960315)26:1<22::AID-CYTO4>3.0.CO;2-I
56. Tarnok A. Flow and image cytometry side by side for the new frontiers in quantitative single-cell analysis. Cytometry A. (2009) 75:169–71. doi: 10.1002/cyto.a.20709
57. Gratama JW, Kraan J, Van Den Beemd R, Hooibrink B, Van Bockstaele DR, Hooijkaas H. Analysis of variation in results of flow cytometric lymphocyte immunophenotyping in a multicenter study. Cytometry. (1997) 30:166–77. doi: 10.1002/(SICI)1097-0320(19970815)30:4<166::AID-CYTO2>3.0.CO;2-I
58. Schwartz A, Marti GE, Poon R, Gratama JW, Fernandez-Repollet E. Standardizing flow cytometry: a classification system of fluorescence standards used for flow cytometry. Cytometry. (1998) 33:106–14. doi: 10.1002/(SICI)1097-0320(19981001)33:2<106::AID-CYTO4>3.0.CO;2-H
59. Westgard JO. Desirable Specifications for Total Error, Imprecision, and Bias, Derived From Intra- and Inter-Individual Biologic Variation. (2019). Available online at: ((https://www.westgard.com/biodatabase1.htm)).
60. Schuirmann DJ. A comparison of the two one-sided tests procedure and the power approach for assessing the equivalence of average bioavailability. J Pharmacokinet Biopharm. (1987) 15:657–80. doi: 10.1007/BF01068419
61. Davis BH, McLaren CE, Carcio AJ, Wong L, Hedley BD, Keeney M, et al. Determination of optimal replicate number for validation of imprecision using fluorescence cell-based assays: proposed practical method. Cytometry B Clin Cytom. (2013) 84:329–37. doi: 10.1002/cytob.21116
63. Tibbe AG, Miller MC, Terstappen LW. Statistical considerations for enumeration of circulating tumor cells. Cytometry A. (2007) 71:154–62. doi: 10.1002/cyto.a.20369
64. Allan AL, Keeney M. Circulating tumor cell analysis: technical and statistical considerations for application to the clinic. J Oncol. (2010) 2010:426218. doi: 10.1155/2010/426218
65. Cummings J, Morris K, Zhou C, Sloane R, Lancashire M, Morris D, et al. Method validation of circulating tumour cell enumeration at low cell counts. BMC Cancer. (2013) 13:415. doi: 10.1186/1471-2407-13-415
67. Holland M, Kraght P, Akbas N, Budd J, Klee G. Improved statistical methods for evaluation of stability of in vitro diagnostic reagents. Statist Biopharmaceut Res. (2017) 9:272–8. doi: 10.1080/19466315.2017.1305287
68. Keller T, Brinkmann T. Proposed guidance for carryover studies, based on elementary equivalence testing techniques. Clin Lab. (2014) 60:1153–61. doi: 10.7754/Clin.Lab.2013.130818
69. CLSI. Measurement Procedure Comparison and Bias Estimation Using Patient Samples. 3rd ed. Wayne, PA: Clinical and Laboratory Standards Institute (2018).
70. Bland JM, Altman DG. Statistical methods for assessing agreement between two methods of clinical measurement. Lancet. (1986) 1:307–10. doi: 10.1016/S0140-6736(86)90837-8
71. Barnhart HX, Haber MJ, Lin LI. An overview on assessing agreement with continuous measurements. J Biopharm Stat. (2007) 17:529–69. doi: 10.1080/10543400701376480
72. Passing H, Bablok W. A new biometrical procedure for testing the equality of measurements from two different analytical methods. Application of linear regression procedures for method comparison studies in clinical chemistry, Part I. J Clin Chem Clin Biochem. (1983) 21:709–20. doi: 10.1515/cclm.1983.21.11.709
73. Haeckel R, Wosniok W, Klauke R. Comparison of ordinary linear regression, orthogonal regression, standardized principal component analysis, Deming and Passing-Bablok approach for method validation in laboratory medicine. Laboratoriumsmedizin. (2013) 37:147–63. doi: 10.1515/labmed-2013-0003
74. Hollis S. Analysis of method comparison studies. Ann Clin Biochem. (1996) 33 (Pt 1):1–4. doi: 10.1177/000456329603300101
75. Carstensen B. Comparing Clinical Measurement Methods. A Practical Guide. Chichester: Wiley (2010). doi: 10.1002/9780470683019
76. Tosato F, Bernardi D, Sanzari MC, Pantano G, Plebani M. Biological variability of lymphocyte subsets of human adults' blood. Clin Chim Acta. (2013) 424:159–63. doi: 10.1016/j.cca.2013.06.001
77. CLSI. Defining, Establishing, and Verifying Reference Intervals in the Clinical Laboratory. Wayne, PA: Clinical and Laboratory Standards Institute (2008).
79. Shearer WT, Rosenblatt HM, Gelman RS, Oyomopito R, Plaeger S, Stiehm ER, et al. Lymphocyte subsets in healthy children from birth through 18 years of age: the Pediatric AIDS Clinical Trials Group P1009 study. J Allergy Clin Immunol. (2003) 112:973–80. doi: 10.1016/j.jaci.2003.07.003
80. Sack U, Gerling F, Tarnok A. Age-related lymphocyte subset changes in the peripheral blood of healthy children - A meta-study. Transf Med Hemother. (2007) 34:176–81. doi: 10.1159/000101357
81. Koenig M, Huenecke S, Salzmann-Manrique E, Esser R, Quaritsch R, Steinhilber D, et al. Multivariate analyses of immune reconstitution in children after allo-SCT: risk-estimation based on age-matched leukocyte sub-populations. Bone Marrow Transplant. (2010) 45:613–21. doi: 10.1038/bmt.2009.204
82. Smet J, Mascart F, Schandene L. Are the reference values of B cell subpopulations used in adults for classification of common variable immunodeficiencies appropriate for children? Clin Immunol. (2011) 138:266–73. doi: 10.1016/j.clim.2010.12.001
83. Duchamp M, Sterlin D, Diabate A, Uring-Lambert B, Guerin-El Khourouj V, Le Mauff B, et al. B-cell subpopulations in children: National reference values. Immun Inflamm Dis. (2014) 2:131–40. doi: 10.1002/iid3.26
84. Özcürümez Mustafa K, Haeckel R, Gurr E, Streichert T, Sack U. Determination and verification of reference interval limits in clinical chemistry. Recommendations for laboratories on behalf of the Working Group Guide Limits of the DGKL with respect to ISO Standard 15189 and the Guideline of the German Medical Association on Quality Assurance in Medical Laboratory Examinations (Rili-BAEK). J Lab Med. (2019) 43:127–33. doi: 10.1515/labmed-2018-0500
85. Zheng SG, Wang JH, Koss MN, Quismorio F, Gray JD, Horwitz DA. CD4+ and CD8+ regulatory T cells generated ex vivo with IL-2 and TGF-beta suppress a stimulatory graft-versus-host disease with a lupus-like syndrome. J Immunol. (2004) 172:1531–9. doi: 10.4049/jimmunol.172.3.1531
86. Donnenberg AD, Donnenberg VS. Understanding clinical flow cytometry. In: M. R. G. O'Gorman, and A. D. Donnenberg, editors. Handbook of Human Immunology, 2nd Edn. Boca Raton, FL; London; New York, NY: Taylor and Francis (2008). 181–219. doi: 10.1201/9781420003710.ch6
87. Araujo JA, Mesquita D, De Melo Cruvinel W, Salmazi KI, Kallas EG, Andrade LE. Th17 cells and CD4(+) multifunctional T cells in patients with systemic lupus erythematosus. Rev Bras Reumatol Engl Ed. (2016) 56:28–36. doi: 10.1016/j.rbre.2015.10.003
Keywords: flow cytometry, procedures, accreditation, quality control, laboratory diagnostics, validation
Citation: Lambert C, Yanikkaya Demirel G, Keller T, Preijers F, Psarra K, Schiemann M, Özçürümez M and Sack U (2020) Flow Cytometric Analyses of Lymphocyte Markers in Immune Oncology: A Comprehensive Guidance for Validation Practice According to Laws and Standards. Front. Immunol. 11:2169. doi: 10.3389/fimmu.2020.02169
Received: 30 January 2020; Accepted: 10 August 2020;
Published: 17 September 2020.
Edited by:
Barbara Rolfe, The University of Queensland, AustraliaReviewed by:
Helen Marie McGuire, The University of Sydney, AustraliaSabine Ivison, The University of British Columbia, Canada
Alfonso Blanco, University College Dublin, Ireland
Copyright © 2020 Lambert, Yanikkaya Demirel, Keller, Preijers, Psarra, Schiemann, Özçürümez and Sack. This is an open-access article distributed under the terms of the Creative Commons Attribution License (CC BY). The use, distribution or reproduction in other forums is permitted, provided the original author(s) and the copyright owner(s) are credited and that the original publication in this journal is cited, in accordance with accepted academic practice. No use, distribution or reproduction is permitted which does not comply with these terms.
*Correspondence: Ulrich Sack, ulrich.sack@medizin.uni-leipzig.de