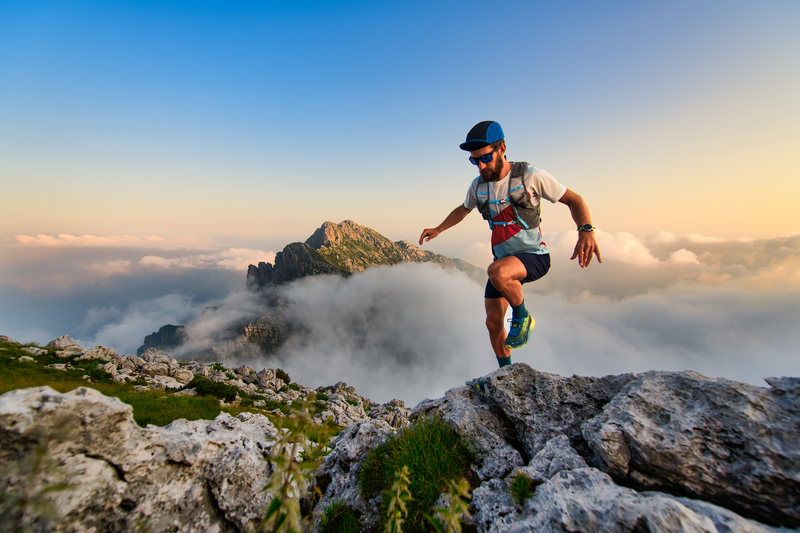
94% of researchers rate our articles as excellent or good
Learn more about the work of our research integrity team to safeguard the quality of each article we publish.
Find out more
BRIEF RESEARCH REPORT article
Front. Immunol. , 07 October 2020
Sec. Multiple Sclerosis and Neuroimmunology
Volume 11 - 2020 | https://doi.org/10.3389/fimmu.2020.02127
This article is part of the Research Topic Platelets as Players in Neuropathologies: Novel Diagnostic and Therapeutic Targets View all 10 articles
Neurodegenerative disorders such as Parkinson’s disease (PD) and Alzheimer’s disease (AD) suffer from the lack of risk-predictive circulating biomarkers, and clinical diagnosis occurs only when symptoms are evident. Among potential biomarkers, platelet parameters have been associated with both disorders. However, these associations have been scarcely investigated at the genetic level. Here, we tested genome-wide coheritability based on common genetic variants between platelet parameters and PD/AD risk, through Linkage Disequilibrium Score Regression. This revealed a significant genetic correlation between platelet distribution width (PDW), an index of platelet size variability, and PD risk (rg [SE] = 0.080 [0.034]; p = 0.019), which was confirmed by a summary-summary polygenic score analysis, where PDW explained a small but significant proportion PD risk (<1%). AD risk showed no significant correlations, although a negative trend was observed with PDW (rg [SE] =-0.088 [0.053]; p=0.096), in line with previous epidemiological reports. These findings suggest the existence of limited shared genetic bases between PDW and PD and warrant further investigations to clarify the genes involved in this relation. Additionally, they suggest that the association between platelet parameters and AD risk is more environmental in nature, prompting an investigation into which factors may influence these traits.
Common neurodegenerative disorders due to accumulation of neurotoxic protein aggregates, such as Alzheimer’s disease (AD) and Parkinson’s disease (PD), suffer from the lack of risk-predictive circulating biomarkers, and clinical diagnosis occurs only when symptoms are evident, at an advanced stage of neurodegeneration (1, 2). Therefore, it is important to identify potential biomarkers that are easy to measure and that could predict the incident risk of such diseases, e.g., circulating biomarkers (3). Among these, platelets have received increasing attention (4–6), and their link with neurodegenerative disorders has long been hypothesized (7). Indeed, platelets are considered “circulating mirrors of neurons” and share many similarities with neural cells (8). These include the molecular machinery that controls the secretory system (5), patterns of reciprocal interactions, and the metabolism of different neurotransmitters like serotonin and dopamine, but also of neurologically important proteins like the Amyloid Precursor Protein (4).
In spite of these interesting cues, the relation between neurodegenerative disorders and classical blood platelet parameters like mean platelet volume (MPV), platelet count (Plt), and platelet distribution width (PDW) has been scarcely investigated at the epidemiological level, also with relatively common disorders like AD and PD. Observational studies consistently revealed an inverse association of PDW with AD and other forms of mild/severe dementia (9–11), and a positive association with cognitive performance (9, 11). An association of MPV with higher PD risk (12) was also reported but was not replicated in a later study, where MPV showed an increase with PD severity (13). At the genetic level, only two studies have previously investigated the relationship of blood platelet parameters with AD (14) and PD risk (15). In a large Genome Wide Association Scan (GWAS) testing associations of common genetic variants like Single Nucleotide Polymorphisms (SNPs) and small insertions/deletions (indels) with different blood cell measures (Nmax ∼ 170,000) (14), the authors observed no evidence of a causal effect of Plt, MPV, or PDW on AD risk based on a multivariable Mendelian Randomization analysis. However, this technique may suffer from low power since it is usually based on a low number of variants (16). More recently, Nalls et al. (15) investigated genetic links of platelet parameters with PD risk through Linkage Disequilibrium (LD) Score Regression, which is a more robust approach based on hundreds of thousands of variants genome-wide (16) (see below). They reported non-significant genetic correlations with both Plt and MPV in the largest PD case-control GWAS meta-analysis carried out so far (involving ∼ 56,300 PD cases and ∼ 1.4 million controls) (15). Of note, in spite of the previous implication of PDW in neurodegenerative disorders (4, 9–11) and, more recently, in comorbid disorders like major depression (16, 17), this parameter has never been investigated with reference to PD risk at the genetic level.
Here, we tested the genetic relationship between the above mentioned platelet parameters, PD and AD risk, making use of summary statistics of large GWAS previously carried out on these traits (14, 15, 18). We first applied LD-score regression analysis to detect significant genome-wide co-heritability based on common genetic variants, and then we further investigated the significant correlations through polygenic risk association analysis (19). The aim of our investigation was twofold. First, we provided a comprehensive re-visitation of the genetic relationship between the most common neurodegenerative disorders—AD and PD—and platelet parameters commonly tested like Plt, MPV, and PDW, in a systematic and homogeneous way. Second, we provided hints into new potential biomarkers of such disorders, to drive future epidemiological, functional, and clinical studies.
We applied LD-score regression (20, 21) to summary statistics of large independent GWAS previously conducted on AD (71,880 cases and 383,378 controls) (18), PD (54,376 cases and 1,474,097 controls), and platelet parameters, namely Plt, MPV, and PDW (Nmax = 166,066) (14) (see Table S1). LD score regression models genetic correlation between two traits as a function of LD score among SNPs in 1 cM bins genome-wide, through the formula
where ρg is the genetic covariance between trait 1 and trait 2, and and represent the SNP-based heritability of the two traits (20, 21). SNP-based heritability is in turn computed as the slope of the linear function between χ2 association statistics and LD score (i.e., the sum of r2 of a given SNP with all the other SNPs in a 1 cM window), for every SNP tested genome-wide (i.e., for which the association statistics are available in a given GWAS study). For this analysis, we filtered out variants that were not SNPs (e.g., indels), strand-ambiguous SNPs, and SNPs with duplicated rs numbers or Minor Allele Frequency (MAF) ≤ 1%. Moreover, SNPs with low values of sample size were also removed, when detailed information by SNP was available in the summary statistics file (N < 321,820 and < 301,340 for the PD and the AD GWAS, respectively). Finally, we retained only common SNPs (MAF > 5%) in the HapMap 3 EUR reference panel (22)—excluding the HLA region—since these variants have good imputation quality stats (r2>0.9) in most studies (21). LD scores of these variants were derived using the 1000G phase 1 v3 EUR panel (available at https://data.broadinstitute.org/alkesgroup/LDSCORE/w_hm3.snplist.bz2). Details on the number of variants available before and after quality control (QC) for each study are reported in Table S1.
Pairwise comparisons showing significant correlations were further investigated at a more fine-grained resolution, through a summary-summary polygenic risk score (Sum-Sum PRS) analysis using PRSice v1.25 (19). This method tests genetic overlap between two traits by making use of GWAS summary statistics: a training GWAS is used to build the PRS, which is then tested as a linear predictor of another trait in an independent study (target GWAS) (23). We performed Sum-Sum PRS analysis using only SNPs with association p-values (PT) ≤ 0.05 in the training GWAS (on PDW) (14), in linkage equilibrium (r2 < 0.05) with the local top hit within a 300 kb window, and shared between the training (14) and the target GWAS (on PD risk) (15). To verify the robustness of our results, we repeated the analysis at increasing association significance thresholds in the training GWAS (with PT = 0.001, 0.05, 0.1, 0.2, 0.3, 0.4, 0.5, 0.6, 0.7, 0.8, 0.9, 1.0), as in (23). The number of SNPs meeting these criteria ranged from 2,813 (for PT ≤0.001) to 213,317 (for PT ≤1), respectively.
LD score regression analysis revealed a significant genetic correlation between PDW and PD risk (rg [Standard Error] = 0.080 [0.034]; p = 0.019; see Tables 1A, B) suggesting the existence of a genomic overlap based on common genetic variants. When we further analyzed this genetic relationship at a more fine-grained resolution, through Sum-Sum polygenic risk analysis, we observed that a modest but significant proportion of PD susceptibility (<1%) was explained by genetic variants nominally associated with PDW (at PT = 0.05: p = 7.0 × 10−4). This association was quite robust across varying p-value thresholds (PT ranging from 0.001 to 1.0; Figure 1). Overall, the evidence reported here suggests PDW as a new potential biomarker for Parkinson’s disease and is consistent with previous studies reporting positive associations between PDW and depression risk and/or symptoms, both at the epidemiological level (17, 24) and at the genetic level (16). Indeed, depression represents one of the main non-motor symptoms of PD, often presenting in its prodromal phase (25), and shows progressive patterns of microglial activation like other neurodegenerative disorders (26). Of note, previous epidemiological studies reported negative associations between PDW and the risk of cognitive impairment (9–11), which is co-morbid and partly shares biological bases with PD (27). While these two relations may appear in contrast, our knowledge of AD and PD is very limited from this point of view, and further epidemiological and clinical studies are needed to clarify the relation of PDW with the different neurodegenerative disorders, possibly through machine learning approaches including other platelet parameters and potential circulating biomarkers, to evaluate their prognostic value simultaneously. Similarly, genetic studies are warranted to identify specific genes influencing both PDW and PD risk.
Table 1 Genetic correlations of platelet parameters with (A) Parkinson’s disease and (B) Alzheimer’s disease risk, based on LD score regression analyses.
Figure 1 Summary-Summary polygenic risk score (Sum-Sum) analysis between PDW and Parkinson’s disease (PD) risk. No direction of effect could be inferred from Sum-Sum analysis, as per PRSice output (19).
By contrast, here we did not detect any significant genetic correlations between platelet parameters and AD risk (Table 1B), although PDW variability showed a trend of significance (rg [SE] = -0.088 [0.053]; p=0.096). This evidence suggests that the significant associations observed in previous epidemiological studies, which anyway showed a concordant sign (9–11), may be mainly due to shared environmental influences between platelet parameters and AD risk, and that common genetic influences are likely very limited, at least those of common variants. Indeed, a genetic link may have not been detected due to other (possibly rare or structural) genetic variants being at the basis of this. This hypothesis has been supported by recent findings for other complex traits like general cognition, educational attainment (28), and dyslexia (29), where only half of the heritability has been explained by common SNPs. Even so, this study rules out any large genetic overlap between PDW and AD risk.
In spite of the interesting findings reported here, the functional meaning of PDW and its potential usefulness as a biomarker remains to be clarified, beyond the neurodegenerative and neuropsychiatric landscape. As an index of heterogeneity of platelet size, reported associations of this marker with indices of platelet activation (30) suggest PDW might be a useful index of platelet function and procoagulant activity. This open issue, along with the modest co-heritability observed here, suggests caution in the interpretation of these findings and warrants further epidemiological, genetic, and functional studies to substantiate the potential usefulness of PDW as a new biomarker of neurodegeneration.
GWAS summary statistics analyzed in the present study are publicly available at the links reported in Table S1.
AG formulated the hypothesis, designed and performed statistical analyses. AT provided theoretical background and reviewed available literature. AG and AT wrote the manuscript, with contributions from all the co-authors. All the authors participated in discussion and interpretation of the results. All authors contributed to the article and approved the submitted version.
AG and FN were supported by Fondazione Umberto Veronesi.
The authors declare that the research was conducted in the absence of any commercial or financial relationships that could be construed as a potential conflict of interest.
The Supplementary Material for this article can be found online at: https://www.frontiersin.org/articles/10.3389/fimmu.2020.02127/full#supplementary-material
1. Selkoe DJ. Alzheimer’s disease is a synaptic failure. Science (2002) 298:789–91. doi: 10.1126/science.1074069
2. Mhyre TR, Boyd JT, Hamill RW, Maguire-Zeiss KA. Parkinson’s disease. Subcell Biochem (2012) 65:389–455. doi: 10.1007/978-94-007-5416-4_16
3. Wojsiat J, Laskowska-Kaszub K, Mietelska-Porowska A, Wojda U. Search for Alzheimer’s disease biomarkers in blood cells: Hypotheses-driven approach. Biomark Med (2017) 11:917–31. doi: 10.2217/bmm-2017-0041
4. Canobbio I, Guidetti GF, Torti M. Platelets in neurological disorders. In: Gresele P, Kleiman NS, Lopez JA, Page CP, editors. Platelets in thrombotic and non-thrombotic disorders. Cham, Switzerland: Springer International (2017). p. 513–30.
5. Goubau C, Buyse GM, Di Michele M, Van Geet C, Freson K. Regulated granule trafficking in platelets and neurons: A common molecular machinery. Eur J Paediatr Neurol (2013) 17:117–25. doi: 10.1016/j.ejpn.2012.08.005
6. Espinosa-Parrilla Y, Gonzalez-Billault C, Fuentes E, Palomo I, Alarcón M. Decoding the role of platelets and related microRNAs in aging and neurodegenerative disorders. Front Aging Neurosci (2019) 10:1–18. doi: 10.3389/fnagi.2019.00151
7. de Gaetano G. Blood platelets as a pharmacological model of serotoninergic synaptosomes. In: de Gaetano G, Garattini S, editors. Platelets: A Multidisciplinary Approach. New York: Raven Press (1978). p. 373–84.
8. Canobbio I. Blood platelets: Circulating mirrors of neurons? Res Pract Thromb Haemost (2019) 3:564–5. doi: 10.1002/rth2.12254
9. Wang RT, Jin D, Li Y. Liang Q cheng. Decreased mean platelet volume and platelet distribution width are associated with mild cognitive impairment and Alzheimer’s disease. J Psychiatr Res (2013) 47:644–9. doi: 10.1016/j.jpsychires.2013.01.014
10. Chen SH, Bu XL, WS J, Shen LL, Wang J, Zhuang ZQ, et al. Altered peripheral profile of blood cells in Alzheimer disease. Med (United States) (2017) 96:1–7. doi: 10.1097/MD.0000000000006843
11. Liang QC, Jin D, Li Y, Wang RT. Mean platelet volume and platelet distribution width in vascular dementia and Alzheimer’s disease. Platelets (2014) 25:433–8. doi: 10.3109/09537104.2013.831064
12. Koçer A, Yaman A, Niftaliyev E, Dürüyen H, Eryilmaz M, Koçer E. Assessment of platelet indices in patients with neurodegenerative diseases: Mean platelet volume was increased in patients with Parkinson’s disease. Curr Gerontol Geriatr Res (2013) 2013:986254. doi: 10.1155/2013/986254
13. GEYİK S, YİĞİTER R, AKGÜL GP, ELÇİ MA, EKMEKYAPAR FIRAT Y. The Relationship Between Parkinson ‘ s Disease and Mean Platelet Volume. Park Hast ve Hareket Bozuklukları Derg (2016) 19:31–4. doi: 10.5606/phhb.dergisi.2016.07
14. Astle WJ, Elding H, Jiang T, Allen D, Ruklisa D, Mann AL, et al. The Allelic Landscape of Human Blood Cell Trait Variation and Links to Common Complex Disease. Cell (2016) 167:1415–1429.e19. doi: 10.1016/j.cell.2016.10.042
15. Nalls MA, Blauwendraat C, Vallerga CL, Heilbron K, Bandres-Ciga S, Chang D, et al. Identification of novel risk loci, causal insights, and heritable risk for Parkinson’s disease: a meta-analysis of genome-wide association studies. Lancet Neurol (2019) 18: 1091–102. doi: 10.1016/S1474-4422(19)30320-5
16. Gialluisi A, Izzi B, Di Castelnuovo A, Cerletti C, Donati MB, de Gaetano G, et al. Revisiting the link between platelets and depression through genetic epidemiology: new insights from platelet distribution width. Haematologica (2019) 105(5). doi: 10.3324/haematol.2019.222513. haematol.2019.222513.
17. Gialluisi A, Bonaccio M, Di Castelnuovo A, Costanzo S, De Curtis A, Sarchiapone M, et al. Lifestyle and biological factors influence the relationship between mental health and low-grade inflammation. Brain Behav Immun (2020) 85:4–13. doi: 10.1016/J.BBI.2019.04.041
18. Jansen IE, Savage JE, Watanabe K, Bryois J, Williams DM, Steinberg S, et al. Genome-wide meta-analysis identifies new loci and functional pathways influencing Alzheimer’s disease risk. Nat Genet (2019) 51:404–13. doi: 10.1038/s41588-018-0311-9
19. Euesden J, Lewis CM, O’Reilly PF. PRSice: Polygenic Risk Score software. Bioinformatics (2015) 31:1466–8. doi: 10.1093/bioinformatics/btu848
20. Bulik-Sullivan B, Loh PR, Finucane HK, Ripke S, Yang J, Patterson N, et al. LD score regression distinguishes confounding from polygenicity in genome-wide association studies. Nat Genet (2015) 47:291–5. doi: 10.1038/ng.3211
21. Bulik-Sullivan B, Finucane HK, Anttila V, Gusev A, Day FR, Loh PR, et al. An atlas of genetic correlations across human diseases and traits. Nat Genet (2015) 47:1236–41. doi: 10.1038/ng.3406
22. The International HapMap C. Integrating common and rare genetic variation in diverse human populations. Nature (2010) 467:52. doi: 10.1038/nature09298
23. Gialluisi A, Andlauer TFM, Mirza-Schreiber N, Moll K, Becker J, Hoffmann P, et al. Genome-wide association scan identifies new variants associated with a cognitive predictor of dyslexia. Transl Psychiatry (2019) 9:77. doi: 10.1038/s41398-019-0402-0
24. Aleksovski B, Neceva V, Vujović V, Manusheva N, Rendevski V, Novotni A, et al. SSRI-reduced platelet reactivity in non-responding patients with life-long Recurrent Depressive Disorder: Detection and involved mechanisms. Thromb Res (2018) 165:24–32. doi: 10.1016/j.thromres.2018.03.006
25. Kusters CDJ, Paul KC, Guella I, Bronstein JM, Sinsheimer JS, Farrer MJ, et al. Dopamine receptors and BDNF-haplotypes predict dyskinesia in Parkinson’s disease. Park Relat Disord (2018) 47:39–44. doi: 10.1016/j.parkreldis.2017.11.339
26. Setiawan E, Attwells S, Wilson AA, Mizrahi R, Rusjan PM, Miler L, et al. Association of translocator protein total distribution volume with duration of untreated major depressive disorder: a cross-sectional study. Lancet Psychiatry (2018) 5:339–47. doi: 10.1016/S2215-0366(18)30048-8
27. Aarsland D, Creese B, Politis M, Chaudhuri KR, Ffytche DH, Weintraub D, et al. Cognitive decline in Parkinson disease. Nat Rev Neurol (2017) 13:217–31. doi: 10.1038/nrneurol.2017.27
28. Hill WD, Arslan RC, Xia C, Luciano M, Amador C, Navarro P, et al. Genomic analysis of family data reveals additional genetic effects on intelligence and personality. Mol Psychiatry (2018) 23:2347–62. doi: 10.1038/s41380-017-0005-1
29. Gialluisi A, Andlauer TMF, Mirza-Schreiber N, Moll K, Becker J, Hoffman P, et al. Genome Wide Association Study reveals new insights into the heritability and genetic correlates of developmental dyslexia. Transl Psychiatry (2020) 9:77. doi: 10.1038/s41398-019-0402-0
Keywords: neurodegenerative disorders, Parkinson disease, Alzheimer disease, platelets, genetics, platelet distribution width, genetic correlation
Citation: Tirozzi A, Izzi B, Noro F, Marotta A, Gianfagna F, Hoylaerts MF, Cerletti C, Donati MB, de Gaetano G, Iacoviello L and Gialluisi A (2020) Assessing Genetic Overlap Between Platelet Parameters and Neurodegenerative Disorders. Front. Immunol. 11:02127. doi: 10.3389/fimmu.2020.02127
Received: 31 March 2020; Accepted: 05 August 2020;
Published: 07 October 2020.
Edited by:
Samuel C. Wassmer, University of London, United KingdomReviewed by:
Souvarish Sarkar, Brigham and Women’s Hospital and Harvard Medical School, United StatesCopyright © 2020 Tirozzi, Izzi, Noro, Marotta, Gianfagna, Hoylaerts, Cerletti, Donati, de Gaetano, Iacoviello and Gialluisi. This is an open-access article distributed under the terms of the Creative Commons Attribution License (CC BY). The use, distribution or reproduction in other forums is permitted, provided the original author(s) and the copyright owner(s) are credited and that the original publication in this journal is cited, in accordance with accepted academic practice. No use, distribution or reproduction is permitted which does not comply with these terms.
*Correspondence: Licia Iacoviello, bGljaWEuaWFjb3ZpZWxsb0Btb2xpLXNhbmkub3Jn
Disclaimer: All claims expressed in this article are solely those of the authors and do not necessarily represent those of their affiliated organizations, or those of the publisher, the editors and the reviewers. Any product that may be evaluated in this article or claim that may be made by its manufacturer is not guaranteed or endorsed by the publisher.
Research integrity at Frontiers
Learn more about the work of our research integrity team to safeguard the quality of each article we publish.