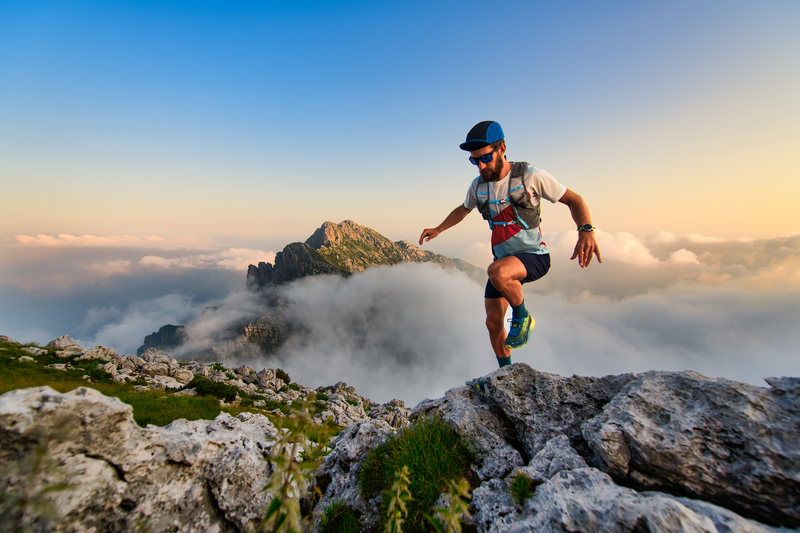
94% of researchers rate our articles as excellent or good
Learn more about the work of our research integrity team to safeguard the quality of each article we publish.
Find out more
ORIGINAL RESEARCH article
Front. Immunol. , 15 April 2020
Sec. T Cell Biology
Volume 11 - 2020 | https://doi.org/10.3389/fimmu.2020.00611
This article is part of the Research Topic Control of Regulatory T cell Stability, Plasticity and Function in Health and Disease View all 21 articles
The human T lymphocyte compartment is highly dynamic over the course of a lifetime. Of the many changes, perhaps most notable is the transition from a predominantly naïve T cell state at birth to the acquisition of antigen-experienced memory and effector subsets following environmental exposures. These phenotypic changes, including the induction of T cell exhaustion and senescence, have the potential to negatively impact efficacy of adoptive T cell therapies (ACT). When considering ACT with CD4+CD25+CD127–/lo regulatory T cells (Tregs) for the induction of immune tolerance, we previously reported ex vivo expanded umbilical cord blood (CB) Tregs remained more naïve, suppressed responder T cells equivalently, and exhibited a more diverse T cell receptor (TCR) repertoire compared to expanded adult peripheral blood (APB) Tregs. Herein, we hypothesized that upon further characterization, we would observe increased lineage heterogeneity and phenotypic diversity in APB Tregs that might negatively impact lineage stability, engraftment capacity, and the potential for Tregs to home to sites of tissue inflammation following ACT. We compared the phenotypic profiles of human Tregs isolated from CB versus the more traditional source, APB. We conducted analysis of fresh and ex vivo expanded Treg subsets at both the single cell (scRNA-seq and flow cytometry) and bulk (microarray and cytokine profiling) levels. Single cell transcriptional profiles of pre-expansion APB Tregs highlighted a cluster of cells that showed increased expression of genes associated with effector and pro-inflammatory phenotypes (CCL5, GZMK, CXCR3, LYAR, and NKG7) with low expression of Treg markers (FOXP3 and IKZF2). CB Tregs were more diverse in TCR repertoire and homogenous in phenotype, and contained fewer effector-like cells in contrast with APB Tregs. Interestingly, expression of canonical Treg markers, such as FOXP3, TIGIT, and IKZF2, were increased in CB CD4+CD127+ conventional T cells (Tconv) compared to APB Tconv, post-expansion, implying perinatal T cells may adopt a default regulatory program. Collectively, these data identify surface markers (namely CXCR3) that could be depleted to improve purity and stability of APB Tregs, and support the use of expanded CB Tregs as a potentially optimal ACT modality for the treatment of autoimmune and inflammatory diseases.
The human immune system undergoes dramatic changes over the course of a lifetime in order to maintain tissue and organism homeostasis. Highly variable cellular dynamics abound during growth and development in early life. This is particularly apparent during the nascent perinatal period, as the periphery is actively seeded with innate and adaptive immune cells that quickly gain initial exposures to foreign antigens (1). These early priming events must confer protection from pathogens, while also maintaining peripheral tolerance to microbial commensals, inert environmental antigens, and self-tissues. Interestingly, despite inconsistent immune profiles in umbilical cord blood (CB) and newborns, a recent report suggests individuals eventually converge on a common post-natal trajectory for healthy/normal immunological development (1). Efforts to define this common trajectory and the disruptions that give way to immune-mediated diseases represent an essential line of investigation. A growing body of evidence supports the notion that a breakdown in the establishment of peripheral immune tolerance is at the heart of many inflammatory and autoimmune disorders [reviewed in (2)]. While many cell types contribute to immune homeostasis, it is now appreciated that a unique subset of thymic regulatory T cells (tTreg) plays a vital role in establishing and maintaining dominant tolerance to self-antigens in the periphery (3). In fact, tTregs are so essential for maintaining immune homeostasis that loss-of-function mutations in FOXP3, the canonical transcription factor that marks the Treg cell lineage, can result in the lethal multi-organ autoimmune disease referred to as immunodysregulation polyendocrinopathy enteropathy X-linked (IPEX) syndrome [reviewed in (4)].
The identification of tTregs, and the subsequent development of methods for their ex vivo isolation and expansion from peripheral blood, has led to an explosion of research interest to harness these cells to control autoimmune diseases, inflammatory disorders, and enable tissue engraftment in the context of transplantation (5–8). The application of ex vivo expanded cells to achieve clinical outcomes is broadly referred to as adoptive cell therapy (ACT). ACT with T cells has advanced largely from pioneering work in the cancer immunotherapy space with the goal of tumor-directed immunity (9–15). These endeavors have identified critical factors determining robust clinical response and efficacy. While not comprehensive, these include key parameters of antigen-specificity of the therapeutic T cells (i.e., either polyclonal or antigen-specific) (16–18); lineage stability of the population that is used for ACT (19, 20); and the capacity of the T cells to traffic to proper sites in vivo, engraft into tissue microenvironments, and exert their context-dependent effector functions (21, 22). While the desired functions of Tregs in restoring immune regulation contrast those of effector T cells (Teff) targeting cancer, the core concepts governing specificity, stability, and functional capacity are likely to be highly analogous for the use of Tregs to treat autoimmune diseases, including type 1 diabetes (T1D).
Translating these early advances into efficacious therapies with Tregs is likely to require a more robust understanding of Treg biology. Specifically, there is a need for a more complete knowledge of the phenotypic changes that occur over the course of a human lifespan. Murine studies have demonstrated that tTregs generated during the perinatal period display a distinct receptor repertoire and are functionally different from tTregs isolated from mature mice (23). Human adult peripheral blood (APB) Tregs are comprised of a complex mixture of resting and activated subsets (24) and are known to co-opt the transcriptional profiles of the various T helper (TH) cell subsets they are tasked with suppressing (24–28). In addition, APB Tregs are reported to display lineage instability resulting in effector-like T cell phenotypes (29–31). However, the heterogeneity of Tregs in CB is generally uncharacterized.
Our prior work has demonstrated that tTregs can be isolated from human CB and expanded with exceptional purity and lineage stability (32). Here, we extend our prior studies optimizing Treg expansion protocols to further characterize the transcriptional profile and repertoire characteristics of human Tregs from CB in comparison to those isolated from APB. We employed both bulk transcriptional profiling, as well as single cell RNA sequencing (scRNA-seq) and T cell receptor (TCR) repertoire analyses to characterize CB and APB Treg populations that could be harnessed for ACT. Our novel transcriptional profiling data and repertoire analyses once again reinforce the concept of a phenotypically homogenous and lineage stable Treg population in CB when compared to APB. These studies have implications for identifying optimal cell sources for either autologous or allogeneic ACT applications. Moreover, the scRNA-seq data provide an array of novel cell surface targets that can be leveraged to further optimize Treg isolation strategies for use in Treg ACTs for the induction of immunological tolerance.
Fresh CB (processed within 24 h of birth) was obtained from LifeSouth Community Blood Center Corporate Headquarters (Gainesville, FL) into CB units containing 35 mL of citrate phosphate dextrose anticoagulant. CB units (n = 7) were delivered to the University of Florida Diabetes Institute (UFDI) and immediately processed for CB mononuclear cells (CBMCs). Leukopaks containing fresh APB(n = 6) were purchased from LifeSouth Community Blood Center (Gainesville, FL, United States). These deidentified samples were obtained under an approved IRB exempt protocol at the UFDI. APB samples were processed within 24 h for isolation of peripheral blood mononuclear cells (PBMCs). For CBMC and PBMC isolation, CB and APB samples were subjected to CD4+ enrichment with the RosetteSep® Human CD4+ T Cell Enrichment Cocktail (STEMCELL Technologies) followed by density gradient centrifugation (Ficoll-Paque PLUS, GE Healthcare) prior to fluorescence-activated cell sorting (FACS). The overall workflow for the experiments reported herein is summarized in Figure 1.
Figure 1. Single cell and bulk sample analysis workflow. We adopted a multifaceted approach to assess differences between CB and APB derived Tregs. Fresh CB Tregs, CB Tconv, APB Tregs, and APB Tconv were fluorescence activated cell sorting (FACS) isolated. Sorted CB Tregs and APB Tregs were directly analyzed by single cell RNA sequencing (scRNA-seq) on the 10x Genomics platform. We assessed single cell gene expression and T cell receptor (TCR) repertoire differences. In addition, freshly sorted CB Tregs, CB Tconv, APB Tregs, and APB Tconv were expanded in vitro for 14 days, after which we performed scRNAseq, as well as bulk transcriptional analysis by microarray, flow cytometry and cytokine secretion analysis by Luminex assay.
CD4+ T cell enriched CBMCs and PBMCs were stained with fluorescently labeled antibodies, resuspended at 2 × 107 cells/mL, and sorted on a BD FACS Aria III Cell Sorter (BD Biosciences), as previously described (32). Tregs and Tconv were sorted as CD4+CD25hiCD127lo and CD4+CD127+, respectively.
Tregs and Tconv from CB and APB were expanded as previously described (32). In brief, sorted Treg and Tconv were incubated with KT64/86 aAPCs at a 1:1 ratio in the presence of exogenous IL-2 and expanded for 14 days with restimulation using anti-CD3 anti-CD28 coated microbeads on day 9 following protocol 1 (32). Expanded CB Tregs, CB Tconv, APB Tregs, and APB Tconv were cryopreserved in CryoStor (Sigma, CS10) and later thawed for batched experiments as described below.
Following expansion, 3 × 105 CB Tregs, CB Tconv, APB Tregs, and APB Tconv were lysed in DNA/RNA lysis buffer (Zymo Research) and stored at −80°C. RNA extraction was achieved using ZR-DuetTM DNA/RNA MiniPrep (Zymo Research, Catalog No. D7001), per the manufacturer’s instructions. Quality assessment of RNA was achieved by ExperionTM Automated Electrophoresis System (BIO-RAD) using Experion RNA High Sensitivity Reagents and Experion Standard Sensitivity RNA chips following the manufacturer’s protocol. Only samples with a minimum RNA concentration of 10 ng/μL and RNA Quality Index (RQI) ≥9.4 were used.
Gene expression and V(D)J libraries were prepared from 5,000 pre- and post-expansion CB and APB Treg cells using the Chromium Single Cell 5′ Bead and Library Kit v1 and the Chromium Single Cell V(D)J Human TCR Analysis Kit (10X Genomics). Libraries were sequenced on an Illumina HiSeq instrument at a target read depth of 50,000 reads per cell.
Raw sequencing reads were processed using Cell Ranger v3.0.0 to create a raw (unfiltered) gene-barcode matrix. Briefly, Cell Ranger mkfastq was used to make fastq files from bcl files. Next, Cell Ranger count was used for aligning sequencing reads to the hg19 reference genome (refdata-cellranger-hg19-3.0.0), obtained from1 using STAR (33). For confidently mapped reads (as defined by Cell Ranger), Unique Molecular Identifier (UMI) sequences were collapsed and the number of UMI reads per gene were stored in the raw gene-barcode matrix2.
Quality control was performed for scRNA-seq data from pre- and post-expansion APB and CB Tregs (Supplementary Figure S1). Barcodes associated with droplets containing cells were distinguished from ambient RNA droplets using the emptyDrops algorithm implemented in the DropletUtils R/Bioconductor package (34). Briefly, each barcode is tested for deviations from the estimated ambient RNA profile as defined by barcodes with 100 UMIs or less. Barcodes with a false discovery rate adjusted p-value < 0.01 were retained after this initial filter. A second filter based on the inflection point in the UMI rank versus total UMI curve was used for more stringent identification of cellular barcodes (Supplementary Figure S1A). Next, we filtered on commonly used quality control measures, such as the total number of UMIs per cell (library size), the number of genes expressed, and the percentage of mitochondrial reads per cell to identify cells with low RNA content, possible doublets, and presumably dead or damaged cells. Cells with a total UMI count or number of genes expressed greater than or less than three median absolute deviations (MADs) from the median were removed. Additionally, cells with a percentage of mitochondrial reads greater than three MADs from the median were removed. This filtering was implemented using the isOutlier function in the scran R/Bioconductor package (35) (Supplementary Figures S1B,C). Additionally, cells with more than one unique TRB chain and two unique TRA chains as defined by the concatenated V-gene, complementarity determining region 3 (CDR3) sequence, and J-gene were excluded as presumed doublets (Supplementary Figure S1D). 4320 cells from the APB and 4842 cells for the CB pre-expansion samples, and 4403 cells from post-expansion APB and 3842 cells from post-expansion CB Treg passed these filters and were used in downstream analyses. To remove variation in the number of molecules detected per cell, residuals from regularized negative binomial regression with library size as a covariate was used as described in (36) and implemented in the SCTransform function in Seurat v3.1 (37). Briefly, a negative binomial regression model is fit for each gene with the number of molecules per cell as a covariate and the read-count of the cell as the dependent variable. This method selects stable model parameters that are robust to overfitting by pooling parameter estimates across genes with similar abundances.
The cord blood and adult peripheral blood datasets were integrated as detailed in (38) and implemented in Seurat. Briefly, canonical correlation analysis (CCA) was performed to identify shared sources of variation across the datasets, and mutual nearest neighbors in the CCA space were identified to produce anchors between datasets. For pre-expansion datasets, highly variable genes accounting for the majority of the heterogeneity within each sample were identified by ranking genes based on variance of the residuals from the regularized negative binomial regression model described above, again as described in (36) and implemented in the SCTransform function in the Seurat R package (37, 39). For post-expansion datasets, the corresponding variable features from the pre-expansion state were used, as the variable features post-expansion were dominated by cell-cycle driven expansion-related genes that were not of primary interest. Using these features, anchors between the datasets which correspond to similar cells across datasets were identified using the FindIntegrationAnchors function, and this was used as input into the IntegrateData function to generate an integrated dataset. For dimensionality reduction, expression values for each gene in the integrated dataset were scaled to have a mean of zero and standard deviation of one using the ScaleData function, and principal component analysis (PCA) was run on this matrix using the RunPCA function in Seurat (37, 39). For visualization, Uniform Manifold Approximation and Projection (UMAP), a common dimensionality reduction method in scRNA-seq, plots were created based on the top 20 principal components using the RunUMAP function in Seurat.
Cells were clustered into groups of similar transcriptomic profiles using graph-based clustering on the first 20 principal components of the integrated dataset. Briefly, a shared nearest neighbors graph was created based on the Jaccard similarity of the sets of the 20-nearest neighbors for each cell, as implemented in FindNeighbors function in Seurat (37, 39). Clusters were then identified by partitioning this graph using the Louvain community detection algorithm with a resolution of 0.4, as implemented in the FindClusters function in Seurat (37). Clusters sizes and the relationship between clusters at different resolutions were analyzed to determine this value (Supplementary Figures S5, S6) (40). DE genes across clusters were identified by comparing each individual cluster with the remaining pooled clusters for each sample using the Wilcoxon rank sum test implemented in the wilcoxauc function in the presto R package (41). P-values for each cluster from each sample were then combined using Wilkinson’s method as implemented in the minimump function in the metap package (42) to identify conserved markers across datasets.
Clonotypes were assigned to cells based on unique paired TRA-TRB V-gene/CDR3/J-gene sequences. Only cells with one β-chain and one α-chain were assigned clonotypes to prevent artificial inflation of clone counts due to reduced information about the sequence. Evenness profiles were calculated as initially described (43). Briefly, for sample-level analysis, clonotypes were tabulated, and frequency vectors for each clonotype within a sample were calculated. Evenness profiles based on the exponential of Hill diversity were computed for α in the range 0–10, with step size 0.2, where and , where αE is the evenness for a given α and αD is the Hill diversity for a given α, and f is the frequency vector. When α = 1, while Hill diversity is not defined, it tends to Shannon entropy (43). This resulted in a 51-dimensional evenness profile for each sample. A large range of α was used to capture differences in clonal expansion across the clonal frequency distribution, as the majority of the clonotypes were single occurrence, and an increased α results in higher frequency clones being given a greater weight. The same procedure was followed for the cluster-level analysis, except each cluster from each sample is treated as an independent sample. Overall, the evenness profile is a low-dimensional vector containing the majority of the information contained in a clonal frequency distribution (44).
Post-expansion Treg transcript analysis was performed as previously described (45). Briefly, mRNA was reverse transcribed and amplified. Resulting cDNA was fragmented and labeled using the GeneChip WT Plus Kit and subsequently, hybridized onto the Clariom S Human Array (Thermo Scientific), following the manufacturer’s procedures. Arrays were scanned with the GeneChip Scanner 3000 7G using AGCC software and subsequently normalized using RMA as implemented in Partek 6.6. GEO Accession #: GSE137301.
The R/Bioconductor package limma (46) was used for differential expression of genes using a linear model using the lmFit function with a model matrix with no intercept and fixed effect for treatment (e.g., CB Treg, APB Treg, CB Tconv, and APB Tconv), blocking on donor, and specifying an inter-donor correlation using duplicateCorrelation, effectively treating donor as a random effect. Contrasts were specified using makeContrasts, and the contrasts were fit using contrasts.fit. Moderated t-statistics were then computed using the empirical Bayes moderation as implemented in the eBayes function.
APB and CB Treg DNA was assayed using the Absolute Human Telomere Length Quantification qPCR Assay Kit (ScienCell) according to the manufacturer’s instructions, with the exception of the qPCR master mix, for which we used Syber Select Master Mix (Applied Biosystems). Briefly, DNA were isolated using the DNEasy Blood and Tissue Kit (Qiagen), quantified using a Qubit Fluorometer (Thermo Fisher), after which 5 ng was input into the assay per subject. Data were acquired on a Roche LightCycler480 instrument, exported into Excel and analyzed in GraphPad PRISM v8.
Expanded cryopreserved Tregs and Tconv from CB and APB were thawed in RPMI complete media and stimulated with phorbol myristate acetate (PMA; 10 ng/mL) and ionomycin (500 nM) for 4 h at 37°C with the addition of Golgistop (BD Biosciences; 0.66 μl/mL). Cells were stained for surface and intracellular markers to assess differentiation and effector markers, chemokine receptors and activation status (Supplementary Table S1). Data were collected on an LSRFortessa (BD Biosciences) and analyzed using FlowJo software (Tree Star, Inc). For each marker, the percentage of cells positive for the marker was modeled with a mixed effects model using the lmer function in the lme4 package (47) with treatment (e.g., CB Treg, APB Treg, CB Tconv, and APB Tconv) as a fixed effect and donor as a random effect. Pairwise contrasts for treatment were computed using the emmeans function in the emmeans package (48). For supplementary experiments, expanded cryopreserved APB and CB Tregs or CBMC and PBMC were thawed in RPMI complete media and restimulated with αCD3/28-coated microbeads at a 1:1 ratio (milltenyi) or soluble αCD3 (2 μg/mL, clone HIT3a, BD Biosciences) and αCD28 (1 μg/mL, clone 28.2, BD Biosciences), respectively, for 48 h at 37°C with the addition of Golgistop (BD Biosciences; 0.66 μl/mL) for the last 4 h. Data were collected on a Cytek Aurora and analyzed as above with statistics computed and data plotted using Graphpad PRISM software v8, as indicated in figure legends.
Isolated expanded CB Tregs, CB Tconv, APB Tregs, and APB Tconv were stimulated in a 96-well plate with PMA (10 ng/mL) and ionomycin (500 nM) for 4 h at 37°C. IL-2, IL-10, IL-12 (p40), IL-12 (p70), IL-19, IL-20, IL-22, IL-26, IL-27 (p28), IL-28A, IL-29, and IL-35 were detected in the supernatant using the Bio-Plex Pro Human Treg Cytokine 12-Plex Panel (Bio-RadR) according to the manufacturer’s procedures with the following modification. Standards were diluted in standard Diluent HB as opposed to culture medium to generate a seven-point curve. For each cytokine, log10 (concentration) was modeled with a mixed effects model using the lmer function in the lme4 package (47) with treatment (e.g., CB Treg, APB Treg, CB Tconv, and APB Tconv) as a fixed effect and donor as a random effect. Pairwise contrasts for treatment were computed using the emmeans function in the emmeans package (48).
Data were visualized using the following R packages: ggplot2 (49), ComplexHeatmap (50), scanalysis (51), ggexp (52), and clustree (40). Flow cytometry data were analyzed in FlowJo software (Tree Star, Inc.) and raw data were exported to GraphPad PRISM v8 or R for statistical analysis.
An R package with runner scripts to reproduce all analyses and figures in this manuscript are available at https://github.com/keshav-motwani/tregPaper (53).
We sought to identify differences in the composition of native (i.e., unexpanded) CB and APB Tregs at the single cell level that might contribute to non-Treg contaminants in a post-expansion cell product for use in ACT. After identification of 4842 high quality cells in CB (n = 1 subject) and 4320 in APB (n = 1 subject), datasets were normalized for cell-specific biases related to sequencing depth using the residuals of regularized negative binomial regression as described in (36). To enable direct comparisons between APB and CB, the datasets were integrated by identifying anchors between similar cells across datasets (38). We performed graph-based clustering on the top 20 principal components (PCs) of the integrated data, identifying a total of 6 clusters which are overlaid on a reduced dimensional representation of the first 20 PCs using UMAP (54) (Figure 2A). From visual inspection of the first two UMAP components (i.e., UMAP1 and UMAP2; Figure 2A), cells in clusters C01-C05 are largely clumped together, but cluster C06 is more of an outlier (Figure 2A), and it is more pronounced in the APB sample (∼3% of cells in APB versus ∼1% in CB) (Figure 2B). To understand the underlying biology in each of the 6 subpopulations, we computed differentially expressed genes between each cluster and the rest of the cells in the dataset. For C06 in particular, the top five differentially expressed (DE) genes (ranked on p-value) were CCL5, GZMK, CXCR3, LYAR, and NKG7 (Supplementary Table S2), as shown in the UMAP plots colored by relative expression (Figure 2C). CXCR3, CCL5, and NKG7 have all been associated previously with TH1 migratory capacity (55, 56) and phenotype (57), while the expression of GZMK and LYAR likely indicate a cytotoxic and activated population (58, 59). Figure 2C also depicts the canonical Treg markers, FOXP3 and IKZF2, which were highly expressed in the majority of APB and CB Treg clusters but only lowly expressed in C06. Figure 2D shows the expression of these seven genes across all clusters where notably, there is a decrease in FOXP3 and IKZF2 expression and an increase in the C06 DE genes (CCL5, GZMK, CXCR3, LYAR, and NKG7). These trends are much more prominent in APB as compared to CB, due to the greater number of contaminant cells in APB. Moreover, there is greater co-expression of FOXP3 and IKZF2 with these five “contaminant cell” genes in CB compared to APB (Figure 2E). This could potentially indicate that contaminants expressing these TH1-associated genes are present in both CB and APB, but the TH1-like contaminants in CB still retain a Treg phenotype while in APB, they lose their regulatory phenotype and adopt an effector-like program. Further examination of the top 50 DE genes in C6 (Supplementary Table S2, ranked on combined p-value) shows upregulation of additional TH1-associated genes including BHLHE40 (60), IFNG (61), and TBX21 (62); TH17-related genes including KLRB1, which encodes CD161 (63), and TGFB1 (64); as well as IL12RB2 (65), shown to be expressed highly in TH1/17 cells, which collectively suggests this contaminant population to belong to the recently characterized TH1/17 subset (66). These data indicate that the CB Treg transcriptomic profile is more homogenous as a lineage as compared to APB Tregs, which contain non-Treg TH1/17 contaminants with cytotoxic and pro-inflammatory potential.
Figure 2. Single cell gene expression profiles of unexpanded APB Tregs and unexpanded CB Tregs show the presence of a TH1/TH17-like contaminant cluster in APB. (A) UMAP plots for APB Tregs (n = 1, top) and CB Tregs (n = 1, bottom) colored by the assigned cluster number (C1-C6) shows the presence of a subpopulation (C06) that is not along the main trajectory and appears more prevalent in APB. (B) Absolute number of cells belonging to each cluster and relative abundances for each cluster within unexpanded APB Tregs (left) and unexpanded CB Tregs (right) show differences in cluster composition. Notably, C06 was comprised of 117 cells (3%) of APB Tregs versus 42 cells (1%) of CB Tregs. (C) For APB Tregs (left) and CB Tregs (right), UMAP plots are colored by expression of the five most differentially expressed genes in C06 (CCL5, GZMK, CXCR3, LYAR, and NKG7) as well as two canonical Treg genes (FOXP3 and IKZF2). Numbers in the top right of each plot indicate the minimum and maximum expression for that sample. Points are colored based on the expression of the gene, with gray being the lowest value and dark red the highest value for that feature across both samples. (D) Visualizing expression of CCL5, GZMK, CXCR3, LYAR, NKG7, FOXP3, and IKZF2 shows increases in “contaminant” gene expression in C06 and decreases in canonical Treg gene expression within this cluster from APB Tregs (left) and CB Tregs (right). The number of cells with nonzero expression of each feature in each cluster is annotated at the top of each plot. (E) For all clusters, pairwise scatterplots between canonical Treg genes FOXP3 (left) and IKZF2 (right) and the identified “contaminant” genes (CCL5, GZMK, CXCR3, LYAR, and NKG7) shows increased co-expression in CB Tregs (lower) compared to APB Tregs (upper). The annotated number represents the number of cells with nonzero expression for both genes. (D,E) For each data point, the color corresponds to the cluster number as presented in (A).
Immune tolerance is initiated by tTregs that seed the periphery in early life, as reviewed previously (1, 23, 67). We and others have shown from bulk TRB immunosequencing that Tregs generally express a diverse repertoire of TCRs (32, 68–71). Here, we extended these studies to include paired TRA and TRB receptor analysis in unexpanded APB and CB Tregs. To understand how clonal expansion of cells related to their phenotype as represented on the UMAP plots, we compared expanded CDR3 sequences spanning the V and J genes for TCR-α and TCR-β chains (i.e., TRAV, TRAJ, TRBV, TRBJ) wherein every clone with a single occurrence was presented in gray while expanded clones with two or more occurrences were assigned a unique color (Figure 3A). Clonal expansion was observed in clusters 1, 2, 3, 4 (C01, C02, C03, and C04, respectively), and C06. To further characterize clonal expansion in each sample and the extent within each cluster we assessed receptor evenness profiles, which reflect the frequency vectors’ distance from a uniform distribution and serve as a normalized diversity metric (Figure 3B) (43, 44). Overall, APB Tregs showed reduced diversity compared to CB Tregs (Figure 3B). This likely reflects TCR enrichments over time, presumably from chronic antigen exposures and selective pressures in the periphery. To determine if these differences were due to the influence of specific clusters, we compared the receptor evenness of each cluster between APB and CB (Figure 3C). Clusters 1 (C01) and 2 (C02) were moderately expanded in CB alone (Figure 3C, red and green), and were found to express genes related to Treg development, stability, and migratory capacity [JUNB (72), DUSP2 (73), and ITGB1 (74)], while the latter expressed genes associated with Treg activation and suppressive function [IDI1 (75), FCRL3 (76), and ID3 (77)]. APB Treg cluster 3 (C03) demonstrated reduced receptor evenness in both APB and CB (Figure 3C, purple), and expressed genes related to T cell activation and memory phenotypes, namely S100A4, S100A10, DUSP4, LGALS1, and LGALS3 (Supplementary Table S2) (78–81), as well as class II HLA and co-stimulatory molecules TNFRSF4 and TNFRSF18 (82, 83) and PRDM1 (BLIMP-1) (Supplementary Table S2), likely representing a population of activated memory Tregs (84). C04 was also expanded in APB alone (Figure 3C, brown), and possessed an eTreg phenotype, with expression of CCR4 (86) (Supplementary Table S2). Lastly, C06 (Figure 3C, orange), which is discussed extensively above, was expanded in CB, though the co-expression of TH1-associated genes with FOXP3 and IKZF2, which encodes Helios (Figure 2), suggests this population to potentially represent differentiated Tregs capable of suppressing the TH1 effector lineage, as opposed to a TH1 contaminant (87). Hence, among clusters exhibiting clonal expansion, the majority appeared to retain a regulatory identity with the exception of C06 within APB. Moreover, we were able to identify a cluster with a similar phenotype to C06 within expanded CB and APB Treg (C08, Supplementary Figure S2, and Supplementary Table S3), which expressed KLRB1 and IFNG, as well as CD40LG (88), cytotoxic molecules GZMA and GZMB (58), and additional TH17-associated genes CCR6 (89) and RORC (90), indicating that the pre-expansion C06 cluster phenotype may still be relevant after expansion. Notably, we found post-expansion CB Treg to exhibit increased receptor evenness as compared to APB Treg (Supplementary Figure S3). Overlap between pre- and post-expansion contaminant cluster gene signatures is shown in Supplementary Figure S4. Additionally, both pre- and post-expansion, the contaminant cluster was a distinct cluster that was robust to changes in cluster resolution (Supplementary Figures S5, S6). Lastly, to understand the reactivities of the Tregs in APB and CB, we defined CDR3β sequence specificities using the manually curated catalog of pathology-associated T cell receptor sequences (McPAS-TCR) (91), matching the observed sequence composition (CDR3B) to sequences associated with putatively annotated reactivities and pathological conditions. Interestingly, we observed a greater total number of CB Treg sequences corresponding to known predicted targets as compared to APB Tregs, and though there are comparable distributions of predicted reactivities, we observed an increased number of sequences with autoreactive specificity in CB Tregs (Figure 3D and Supplementary Figure S4D). These data suggest that even in the polyclonal state, CB Treg may be optimal for broad tissue engraftment with more clones expressing TCRs reactive to autoantigens when compared to Tregs derived from APB.
Figure 3. T cell receptor (TCR) profiling from scRNA-seq data identifies clonally expanded subpopulations in pre-expanded Tregs. (A) UMAP plot colored by clonotypes with single occurrences (gray) and multiple occurrences (each clonotype distinctly colored) identifies clusters with expansion (UMAP Components 1 and 2 are the same as those presented in Figure 2). (B) Alpha value (i.e., clonotype frequency) versus evenness value (43) for APB Tregs (orange) and CB Tregs (blue) shows that along the range of the frequency distribution, APB Tregs show decreased evenness at all alpha values, thus exhibiting greater clonal expansion than CB Tregs. (C) When APB Tregs (n = 1, left) and CB Tregs (n = 1, right) are split by cluster (C01-C06, as defined in Figure 2), APB C03 and APB C04 contain expanded subpopulations, while CB C01, C02, C03, and C06 are also moderately expanded. (D) Putative antigen specificity composition of TCRs in each sample show an increased number of clones mapping to known reactivities, including autoimmune clones, in CB Tregs (right) compared to APB Tregs (left).
Achieving clinically effective Treg numbers in ACT often requires cell expansion (92). Moreover, it is essential that Tregs maintain a regulatory identity and the capacity for cycling and activation post-expansion. We previously determined CB Treg to maintain a naïve phenotype post-expansion (32) which is substantiated by our observation of increased telomere length in expanded CB Treg (CB mean: 383.4 ± 16 kb, APB mean: 258.2 ± 11 kb, Supplementary Figure S7). However, there is a paucity of data examining transcriptomic differences between expanded CB and APB Tregs. Therefore, we sought to address this by characterizing the transcriptome of CB and APB derived Tregs and Tconv by microarray after a 14 day in vitro expansion period. As expected (32, 93), among the top 30 DE genes between APB Treg and APB Tconv were the canonical Treg transcription factors FOXP3 and IKZF2, along with various negative regulators and functional molecules, namely TIGIT and TNFRSF9 (88), while APB Tconv preferentially expressed pro-inflammatory and cytotoxic mediators such as GZMA, IL-7R, GZMB, GNLY, and IL18RAP (Figure 4A and Supplementary Table S4). Differences in expression of canonical Treg genes were less robust between CB Tconv and CB Tregs (Figure 4A and Supplementary Table S4). Nevertheless, in CB Treg versus CB Tconv, we observed higher expression of LGALS3 and LGMN, which enhance FOXP3 expression (94), as well as HES1, which promotes TGF-β signaling (95) (Figure 4A and Supplementary Table S4). Moreover, in CB Tconv versus APB Tconv, we observed increased IKZF2, TIGIT, TNFRSF9 and SOX4, the latter of which is induced by TGF-β signaling (96) (Figure 4A and Supplementary Table S4), supporting the notion that CB Tconv may adopt a more regulatory phenotype than APB Tconv. Interestingly, relative to CB Tregs, APB Tregs expressed higher levels of GBP1 and STAT1 (Figure 4A and Supplementary Table S4), previously shown to be involved in IFN-γ signaling (97) and to serve as a driver of TH1 differentiation (98), respectively. In addition, compared to APB Tregs, CB Tregs were enriched for markers promoting homing to the gut [GPR55 (99)], adhesion and migration through the basal lamina [DST (100)], and stem-cell and recent thymic emigrant phenotypes [TCF4 (101) and THEMIS (102)] (Figure 4A and Supplementary Table S4). To summarize these data, heatmaps of a selection of differentially expressed genes between Treg and Tconv show that both CB and APB Tregs highly expressed canonical Treg genes (e.g., FOXP3, IKZF2, CTLA4, and TIGIT), while APB Tconv largely lacked expression of these genes (Figure 4B). Interestingly, CB Tconv expressed some markers typically attributed to a Treg phenotype, namely TIGIT, IKZF2, and FOXP3 (Figure 4B), again suggesting a more immunoregulatory phenotype than their APB Tconv counterparts or the selective expansion of Treg following the initial cell isolation. Hence, bulk transcriptomic profiling of CB Tregs supports their lineage stability and retention of a suppressive phenotype post-expansion.
Figure 4. Bulk gene-expression profiles from expanded cells show increased regulatory phenotype in both Tregs and Tconv from CB compared to APB. (A) Volcano plots showing log2 (Fold Change) versus -log10 (adjusted p-value) annotated with differentially expressed genes (155) colored by downregulation (blue) and upregulation (red). (B) Heatmap of canonical Treg and Tconv genes shows clustering by cell type (Treg (n = 13) versus Tconv (n = 13) and also sample origin (APB (n = 14) versus CB (n = 12).
Next, we expanded CB and APB derived Tregs and Tconv, restimulated with PMA/ionomycin, and examined their phenotype and cytokine production by flow cytometry and Luminex assay, respectively. We found cytokine production and phenotypic profiles to be mostly similar for expanded CB Tregs versus APB Tregs (Figure 5 and Figure 6, blue and orange) and for expanded CB Tconv versus APB Tconv (Figure 5 and Figure 6, green and red) with the most dramatic differences observed between Tregs and Tconv, regardless of the source (CB or APB). Expectedly, CB and APB Treg produced limited pro-inflammatory and effector cytokines (Figures 5A–H) relative to Tconv (103–108), and though we observed no differences in the production of immunosuppressive or effector Treg-associated cytokines (109, 110) between the two subsets (Figures 5I–K), we did observe APB Treg to produce increased IL-2 relative to CB Treg (Figure 5L). This could be indicative of non-Treg contaminants, consistent with known Treg reliance on exogenous IL-2 (111). Phenotypically, we found CB Tregs to be more activated than APB Treg, as evidenced by an increased frequency of CB Tregs expressing the costimulatory molecule CD226 (Figure 6A). Moreover, CB Treg possessed increased proportions of cells expressing CD73 and CD95L (Fas Ligand (FasL) (Figures 6B,C), while CD279 (PD-1) was not significantly different between APB and CB Tregs (Figure 6D). This suggests CB Treg to have increased capacity for functional suppression via conversion of extracellular ATP to adenosine [CD73 (112)] and activation induced cell death [AICD; FasL (113)], without succumbing to Treg exhaustion [PD-1 (114)] (Supplementary Figure S8). CD28+ cells were more frequent among CB Tregs and APB Tregs as compared to CB Tconv and APB Tconv (Figure 6E); this was not surprising given that CD28 signaling is essential for Treg development (115), promotes lineage stability and anti-inflammatory cytokine production (116). CB Tregs and APB Tregs both displayed an increased frequency of cells expressing the activation marker HLA-DR as compared to APB Tconv and CB Tconv (Figure 6F). Moreover, compared to APB Tconv, CB Tregs, APB Tregs and CB Tconv displayed increased percentages of cells expressing the suppressive and activation marker, TIGIT (Figure 6G), in agreement with our post-expansion bulk sequencing data (Figure 4). Compared to APB Tconv, Tregs derived from either APB or CB were enriched for cells expressing CD95, a memory Treg marker (Figure 6H) (117). CB Tregs, APB Tregs, and CB Tconv also contained a greater percentage of cells positive for the chemokine receptors CD197 and CD194 as compared to APB Tconv (Figures 6I,J), potentially reflective of increased homing potential to secondary lymphoid organs and the skin, respectively (118, 119). Importantly, compared to Tconv, CB and APB Treg possess a reduced percentage of cells expressing CD183 (CXCR3), a TH1-related chemokine receptor (Figure 6K). Moreover, we found CB Treg to possess fewer FOXP3+HELIOS– cells pre- and post-expansion, with an increase in CXCR3 (gMFI and percent positive) noted on this subset within APB (Supplementary Figures S9, S10). Finally, we assessed CD49b expression as a marker of Tr1 cells, which have been shown to exhibit similarity to TH1 cells and to express CXCR3 (120). Tr1 differentiation has been reported to occur in the presence of pro-inflammatory cytokines (121, 122), and while these cells have the potential to be potent suppressors, they lose this capability in the absence of IL-10 while still retaining a cytotoxic program (123), thus making their inclusion in an ACT product a potential risk. The frequencies of CD49b+ cells were low and did not differ between CB Tregs versus APB Tregs (Figure 6L), indicating a lack of Tr1 differentiation (124). Cumulatively, these data when considered in addition to the transcriptional profiles suggest that CB Tregs retained a highly activated status, suppressive phenotype, and distinct homing capacity when compared APB Tregs.
Figure 5. Expanded APB Tregs and CB Tregs have similar cytokine production profiles. Expanded CB Tregs (blue, n = 4), APB Tregs (orange, n = 8), CB Tconv (green, n = 3), and APB Tconv (red, n = 3) were activated with PMA/ionomycin and cytokine production detected by Luminex assay. CB Tregs and APB Tregs displayed limited differences in cytokine production profile, as there were no differences in production of (A) IL-20, (B) IL-22, (C) IL-26, (D) IL-27, (E) IL-12p40, (F) IL-12p70, (G) IL-28, or (H) IL-29. APB and CB Tregs produce similar amounts of immunosuppressive cytokines (I) IL-19, (J) IL-35, and (K) IL-10, while APB Tregs produced significantly more (L) IL-2 relative to CB Tregs. When comparing CB Tconv versus APB Tconv, (A–J,L) cytokine production was similar except APB Tconv produced significantly more (K) IL28A/IFNg2 as compared to CB Tconv. Data were analyzed in R with a mixed effects model using the lmer function in the lme4 package, as noted in Materials and Methods. *p < 0.05, **p < 0.01, ***p < 0.001, ****p < 0.0001.
Figure 6. Flow cytometric analysis shows increased activation and suppressive phenotype of CB Tregs compared to APB Tregs. CB Tregs and CB Tconv (n = 7), and APB Tregs and APB Tconv (n = 6) were assessed for differences in phenotype post-expansion. Frequencies of (A) CD226, (B) CD73, and (C) CD95L positive cells were increased in CB Tregs versus APB Tregs. CB Tregs and APB Tregs possessed similar frequencies of (D) CD279+ (PD-1+) cells, while CD279+ cells were more prevalent among CB Tconv and APB Tconv. CB Tregs and APB Tregs also possessed similar proportions of cells positive for (E) CD28, (F) HLA-DR, (G) TIGIT, (H) CD95, (I) CD197 and (J) CD194, with Tregs overall displaying an increased proportion of cells positive for these as compared to Tconv. CB Tconv and APB Tconv displayed an increased proportion of cells expressing (K) CD183 (CXCR3) and (L) CD49b as compared to Tregs. Data were analyzed in R with a mixed effects model using the lmer function in the lme4 package, as noted in Materials and Methods. *p < 0.05, **p < 0.01, ***p < 0.001, ****p < 0.0001.
Tregs, when used in the context of ACT, are expected to function as “living drugs” and exert their suppressive functions via numerous mechanisms including expression of negative surface regulators, IL-2 sequestration, and the production of immunoregulatory cytokines as well as other suppressive mediators (125). Importantly, these cells have the potential to traffic to relevant sites of inflammation, and are capable of bystander suppression and infectious tolerance (126). It is precisely this combination of therapeutic properties that has generated great interest in harnessing Tregs for the establishment of long-term tolerance in situations of autoimmunity and/or transplantation. In fact, many of these concepts have been repeatedly demonstrated in animal models of disease but have not, to date, been broadly translated into effective therapies in humans. We would speculate that translation is hampered both by practical considerations (e.g., cost and feasibility of large-scale production) as well as incomplete knowledge of the optimal cellular properties needed to maximize Treg specificity and function in humans.
We previously demonstrated the potential to isolate and expand previously cryopreserved CB-derived Tregs with increased lineage stability relative to APB (32). Given that it is now possible to store CB to create large population biobanks, it is now feasible to consider options for both autologous and HLA-matched allogeneic CB sourcing for ACT applications. Additionally, the utilization of banked CB units avoids the necessity for large blood draws or leukapheresis procedures to obtain sufficient Treg quantities for expansion. This would be highly desirable in pediatric and autoimmune subjects, many of whom may exhibit lymphopenia, increased inflammatory cell populations as potential contaminants, or express multiple genetic susceptibility alleles that may negatively affect Treg function (127–129).
In an effort to better understand how CB compare to APB Tregs, we conducted scRNA-seq of pre- and post-expansion Tregs (Figure 1). This analysis demonstrated an enriched cell cluster (herein referred to as C06) in APB defined by a gene expression profile associated with the TH1 (CCL5, CXCR3, BHLHE40, NKG7, IFNG, TBX21) and TH17 (KLRB1, TGFB1, IL12RB2, CCR6, RORC) lineages, as well as activation and cytotoxicity (GZMK, LYAR), indicating this population may belong to the recently characterized TH1/17 lineage (66). Interestingly, functional Treg markers (FOXP3, IKZF2) were downregulated in this cluster amongst APB but not CB Tregs, supporting the notion that CB Tregs are a more homogenous population with increased lineage stability and reduced Teff contaminants. Enrichment of cells expressing pro-inflammatory genes, namely IFNG, could have negative implications for Treg plasticity and function. Indeed, IFNγ+ Tregs have been documented in healthy donors but are enriched in various autoimmune conditions including multiple sclerosis (130) and T1D (31), and have impaired suppressive capacity (131). Moreover, CCL5 and CXCR3 have been shown to be induced following IFNγ signaling (132, 133) and to influence the trafficking of GvHD-promoting proinflammatory T cells (134, 135); hence, we propose that the cellular phenotype of C06 present in APB Treg should be further investigated for its potential to negatively impact the success of Treg ACT.
An important consideration in the development of Treg therapies is antigen specificity, as autoreactive thymocytes with lower to moderate affinity TCRs are thought to preferentially differentiate to the Treg lineage in an autoimmune regulator (AIRE)-dependent manner (136). This bias toward self-reactivity represents a critical paradigm in the suppression of autoreactive Teff in the periphery. However, achieving antigen-specific Tregs in doses sufficient for clinical translation has been hampered by the low frequency of these cells in circulation. We previously demonstrated increased TCR β-chain repertoire diversity in CB Tregs (32). In this study, we expand on those bulk sequences to investigate paired gene expression and TCR profiles within the 6 identified APB and CB Treg clusters (Figure 2). We show APB Tregs to demonstrate increased clonal expansion as compared to CB Treg. We were able to define by gene expression the most expanded cluster in APB (C03), which expressed genes indicative of an activated memory phenotype (S100A4, DUSP4, S100A10, LGALS1, LGALS3). In contrast, the clusters that were moderately expanded in CB represent activated, functional Tregs expressing markers conferring adhesive and migratory potential, namely C01 (JUNB, DUSP2, and ITGB1), C02 (IDI1, FCRL3, ID3), and C06. Importantly, while C06 was shown to function as a contaminant in APB, increased co-expression of the cluster-defining genes (CCL5, GZMK, CXCR3, LYAR, and NKG7) with FOXP3 and IKZF2 in CB compared to APB suggests C06 CB Tregs to retain a regulatory phenotype more so than C06 in APB Tregs (Figure 2). We also show an increased number of CB TCRs map to putatively annotated autoreactive sequences, relative to TCRs from APB. Hence, polyclonal CB Tregs might provide a more comprehensive repertoire from which to seed the periphery. Indeed, a recent study has demonstrated the capacity to expand proinsulin (PI)-reactive Tregs from CB with increased yield compared to peripheral blood from subjects with T1D (137). The resultant pool of PI-specific Tregs was found to maintain lineage stability and suppressive function. These results coupled with our data support the notion that CB Tregs represent a population with increased phenotypic homogeneity alongside increased receptor diversity compared to APB Treg, thus serving as an ideal candidate for ACT in autoimmune diseases. Additionally, the potential to generate TCR redirected or chimeric antigen receptor (CAR) Treg has become a topic of great interest in the immunotherapy space (138–140). Our data suggest that the phenotypic stability and homogeneity of CB Tregs relative to APB might ameliorate concerns over lineage stability with TCR or CAR-directed Treg therapies.
We next examined the bulk transcriptomic profiles of CB and APB Tconv and Treg subsets after a 14-day expansion period (Figure 4). As expected, APB Tregs displayed increased expression of immunoregulatory markers (FOXP3, IKZF2, TIGIT, TNFRSF9) as compared to APB Tconv. In APB Tconv, we observed upregulation of GZMA, GZMB, GNLY, IL7R, and IL18RAP, which promote pro-inflammatory signaling (141–143) and have been associated with reduced suppressive capacity (144) as well as autoimmune susceptibility (145). In fact, a number of these genes have been implicated in the progression of autoimmune diseases, including T1D (146), hence avoiding cellular contaminants that express them is paramount to the development of an effective therapy with low risk of exacerbating the underlying pathology. While CB Tregs upregulate genes that promote regulatory function relative to CB Tconv (LGALS3, LGMN, and HES1), our data also support a more immunoregulatory phenotype in CB Tconv versus APB Tconv, as evidenced by upregulation of IKZF2, SOX4, TIGIT, and TNFRSF9. This observation suggests that, even in naïve CD4+ Tconv subsets, the default developmental program during the perinatal period may preferentially induce a regulatory gene expression profile. Furthermore, we observed increased expression of GBP1 and STAT1 by APB Tregs as compared to CB Tregs, likely signifying a more pro-inflammatory phenotype (147) and potentially, reduced suppressive capacity (148). In contrast, CB Tregs were enriched in markers that promote homing to the gut and activation and migratory potential (GPR55, DST) as well as stem-cell and recent thymic emigrant phenotypes (TCF4, THEMIS). Interestingly, single nucleotide polymorphisms (SNPs) within the chromosome region containing THEMIS have recently been associated with younger age at T1D diagnosis (149), suggesting that alterations in this gene may contribute to aberrant thymocyte selection and thereby, autoimmunity. Collectively, our data imply that expanded CB Tregs may better maintain their ability to traffic to sites of inflammation without the acquisition of an ex-Treg phenotype observed to be enriched in APB Tregs in T1D (28).
In examining Treg surface phenotype, we showed both APB and CB Tregs to express more markers of Treg activation/suppression than Tconv, namely TIGIT, HLA-DR, and CD28. Indeed, TIGIT+ Tregs have been shown to be more activated, to express early activation molecules such as CD69 and checkpoint molecules such as PD-1, and to suppress CD8 T cell and NK cell responses (150). Similarly, HLA-DR+ Tregs have been identified as a highly suppressive population, which is depleted in patients with acute post-transplant rejection (151). PD-1 expression was reduced amongst both APB and CB Tregs as compared to Tconv. While PD-1 expression has been shown to facilitate Treg activation and suppression of Teff responses through interaction with PD-L1 (152), high levels of PD-1 expression have been associated with T cell exhaustion (153). Indeed, Tregs expressing high levels of PD-1 have been shown to exhibit functional impairments, such as reduced suppressive capacity and increased IFNγ secretion (154). We recapitulated our previous observations in Figure 4 that show CB Tconv exhibit an immunoregulatory phenotype, with increased TIGIT as compared to APB Tconv. Finally, increased CD226 expression concomitant TIGIT in CB Tregs indicates increased activation, a finding consistent with increased cell yield following expansion cultures (32), while increased CD73 and CD95L expression is indicative of a more broadly suppressive population.
Our data suggest altered transcriptional profiles and suppressive properties of CB Treg relative to APB; however, we acknowledge a number of limitations in our current study. First, our analyses include comparisons across different donors due to longitudinal sample limitations and availability. It is possible that some of the observed differences in gene expression and phenotype are driven by genetic background of donors, and not a function of CB versus APB Tregs. We also acknowledge that some of our observations could relate to the relative imbalance in naïve and memory Treg subsets in CB and APB. Despite these limitations, we expect that the epigenetic profile of CB and APB may differ dramatically, even among the naïve Treg subset. Thus, studies are ongoing to examine the single cell differential chromatin accessibility profiles of CB and APB Tregs through the single cell Assay for Transposase Accessible Chromatin with sequencing (scATAC-seq). These data may also help to uncover the molecular basis for the more regulatory phenotype we observed in CB Tconv.
Our data suggest that modifications of the standard Treg sorting protocol to exclude cells expressing the surface marker CXCR3 present in our APB “contaminant” population as well as targeting/suppressing genes encoding pro-inflammatory markers and transcription factors (BHLHE40, GBP1) may result in a purer Treg population for use in ACT. Cumulatively, our observations suggest that APB-derived Tregs contain subsets of more differentiated and expanded Tregs as well as contaminants capable of producing cytotoxic molecules and proinflammatory cytokines, which could compromise the success of Treg ACT. In contrast, the CB Treg transcriptomic profile was more homogenous, supporting an undifferentiated regulatory phenotype, and reflects a predisposition for increased cell cycling and proliferation. In sum, clinical CB biobanks may serve as an important source material as the field continues to explore advanced cellular therapies, including the potential for highly specialized and gene and receptor edited cell products to induce durable immune tolerance.
The datasets generated for this study can be found in the GEO repository, with the accession numbers GSE137301 and GSE147794.
KM and LP researched and analyzed the data and wrote the manuscript. WV, AE, HS, and ML researched and analyzed the data and reviewed/edited the manuscript. HB analyzed the data and contributed to discussion. AP reviewed/edited the manuscript and contributed to discussion. MB and DP researched the data, contributed to discussion, and reviewed/edited the manuscript. RB analyzed the data and reviewed/edited the manuscript. JL reviewed/edited the manuscript. MH conceived of the study and reviewed/edited the manuscript. TB conceived of the study and wrote the manuscript.
These studies were supported by grants from the JDRF (2-PDF-2016-207-A-N to DP) and the National Institutes of Health (P01 AI42288 and R01 DK106191 to TB). LP was supported by a T32 training grant (5T32DK108736-03). Additional programmatic support was provided by the McJunkin Family Foundation, an unrestricted grant from Cord Blood Registry, and The Leona M. and Harry B. Helmsley Charitable Trust.
The authors declare that the research was conducted in the absence of any commercial or financial relationships that could be construed as a potential conflict of interest.
The Supplementary Material for this article can be found online at: https://www.frontiersin.org/articles/10.3389/fimmu.2020.00611/full#supplementary-material
1. Olin A, Henckel E, Chen Y, Lakshmikanth T, Pou C, Mikes J, et al. Stereotypic immune system development in newborn children. Cell. (2018) 174:1277–92.e14. doi: 10.1016/j.cell.2018.06.045
2. Theofilopoulos AN, Kono DH, Baccala R. The multiple pathways to autoimmunity. Nat Immunol. (2017) 18:716–24. doi: 10.1038/ni.3731
3. Legoux FP, Lim JB, Cauley AW, Dikiy S, Ertelt J, Mariani TJ, et al. CD4+ T cell tolerance to tissue-restricted self antigens is mediated by antigen-specific regulatory T cells rather than deletion. Immunity. (2015) 43:896–908. doi: 10.1016/j.immuni.2015.10.011
4. Bacchetta R, Barzaghi F, Roncarolo MG. From IPEX syndrome to FOXP3 mutation: a lesson on immune dysregulation. Ann N Y Acad Sci. (2018) 1417:5–22. doi: 10.1111/nyas.13011
5. Marshall GP, Cserny J, Perry DJ, Yeh W, Seay HR, Elsayed AG, et al. Clinical applications of regulatory T cells in adoptive cell therapies. Cell Gene Therapy Insights. (2018) 4:405–29. doi: 10.18609/cgti.2018.042
6. Boardman DA, Philippeos C, Fruhwirth GO, Ibrahim MA, Hannen RF, Cooper D, et al. Expression of a chimeric antigen receptor specific for donor HLA class I enhances the potency of human regulatory T cells in preventing human skin transplant rejection. Am J Transplant. (2017) 17:931–43. doi: 10.1111/ajt.14185
7. Gliwinski M, Iwaszkiewicz-Grzes D, Trzonkowski P. Cell-based therapies with T regulatory cells. BioDrugs. (2017) 31:335–47. doi: 10.1007/s40259-017-0228-3
8. Ramlal R, Hildebrandt GC. Advances in the use of regulatory T-cells for the prevention and therapy of graft-vs.-host disease. Biomedicines. (2017) 5:23. doi: 10.3390/biomedicines5020023
9. DeSelm CJ, Tano ZE, Varghese AM, Adusumilli PS. CAR T-cell therapy for pancreatic cancer. J Surg Oncol. (2017) 9:2166. doi: 10.1002/jso.24627
10. Scarfo I, Maus MV. Current approaches to increase CAR T cell potency in solid tumors: targeting the tumor microenvironment. J Immunother Cancer. (2017) 5:28. doi: 10.1186/s40425-017-0230-9
11. Busch DH, Frassle SP, Sommermeyer D, Buchholz VR, Riddell SR. Role of memory T cell subsets for adoptive immunotherapy. Semin Immunol. (2016) 28:28–34. doi: 10.1016/j.smim.2016.02.001
12. Hinrichs CS, Rosenberg SA. Exploiting the curative potential of adoptive T-cell therapy for cancer. Immunol Rev. (2014) 257:56–71. doi: 10.1111/imr.12132
13. Jaspers JE, Brentjens RJ. Development of CAR T cells designed to improve antitumor efficacy and safety. Pharmacol Ther. (2017) 178:83–91. doi: 10.1016/j.pharmthera.2017.03.012
14. Porter DL, Hwang WT, Frey NV, Lacey SF, Shaw PA, Loren AW, et al. Chimeric antigen receptor T cells persist and induce sustained remissions in relapsed refractory chronic lymphocytic leukemia. Sci Transl Med. (2015) 7:303ra139. doi: 10.1126/scitranslmed.aac5415
15. Davila ML, Riviere I, Wang X, Bartido S, Park J, Curran K, et al. Efficacy and toxicity management of 19-28z CAR T cell therapy in B cell acute lymphoblastic leukemia. Sci Transl Med. (2014) 6:224ra25. doi: 10.1126/scitranslmed.3008226
16. Tsuji T, Yasukawa M, Matsuzaki J, Ohkuri T, Chamoto K, Wakita D, et al. Generation of tumor-specific, HLA class I-restricted human Th1 and Tc1 cells by cell engineering with tumor peptide-specific T-cell receptor genes. Blood. (2005) 106:470–6. doi: 10.1182/blood-2004-09-3663
17. Bernhard H, Neudorfer J, Gebhard K, Conrad H, Hermann C, Nahrig J, et al. Adoptive transfer of autologous, HER2-specific, cytotoxic T lymphocytes for the treatment of HER2-overexpressing breast cancer. Cancer Immunol Immunother. (2008) 57:271–80. doi: 10.1007/s00262-007-0355-7
18. Besser MJ, Shapira-Frommer R, Itzhaki O, Treves AJ, Zippel DB, Levy D, et al. Adoptive transfer of tumor-infiltrating lymphocytes in patients with metastatic melanoma: intent-to-treat analysis and efficacy after failure to prior immunotherapies. Clin Cancer Res. (2013) 19:4792–800. doi: 10.1158/1078-0432.CCR-13-0380
19. Morrot A, da Fonseca LM, Salustiano EJ, Gentile LB, Conde L, Filardy AA, et al. Metabolic symbiosis and immunomodulation: how tumor cell-derived lactate may disturb innate and adaptive immune responses. Front Oncol. (2018) 8:81. doi: 10.3389/fonc.2018.00081
20. Ligtenberg MA, Mougiakakos D, Mukhopadhyay M, Witt K, Lladser A, Chmielewski M, et al. Coexpressed catalase protects chimeric antigen receptor-redirected T cells as well as bystander cells from oxidative stress-induced loss of antitumor activity. J Immunol. (2016) 196:759–66. doi: 10.4049/jimmunol.1401710
21. Guedan S, Posey AD Jr., Shaw C, Wing A, Da T, Patel PR, et al. Enhancing CAR T cell persistence through ICOS and 4-1BB costimulation. JCI Insight. (2018) 3:e96976. doi: 10.1172/jci.insight.96976
22. Spitzer MH, Carmi Y, Reticker-Flynn NE, Kwek SS, Madhireddy D, Martins MM, et al. Systemic immunity is required for effective cancer immunotherapy. Cell. (2017) 168:487–502.e15. doi: 10.1016/j.cell.2016.12.022
23. Yang S, Fujikado N, Kolodin D, Benoist C, Mathis D. Immune tolerance. regulatory T cells generated early in life play a distinct role in maintaining self-tolerance. Science. (2015) 348:589–94. doi: 10.1126/science.aaa7017
24. Miyara M, Yoshioka Y, Kitoh A, Shima T, Wing K, Niwa A, et al. Functional delineation and differentiation dynamics of human CD4+ T cells expressing the FoxP3 transcription factor. Immunity. (2009) 30:899–911. doi: 10.1016/j.immuni.2009.03.019
25. Mohr A, Malhotra R, Mayer G, Gorochov G, Miyara M. Human FOXP3(+) T regulatory cell heterogeneity. Clin Transl Immunology. (2018) 7:e1005. doi: 10.1002/cti2.1005
26. Wing JB, Tanaka A, Sakaguchi S. Human FOXP3(+) regulatory T cell heterogeneity and function in autoimmunity and cancer. Immunity. (2019) 50:302–16. doi: 10.1016/j.immuni.2019.01.020
27. Levine AG, Mendoza A, Hemmers S, Moltedo B, Niec RE, Schizas M, et al. Stability and function of regulatory T cells expressing the transcription factor T-bet. Nature. (2017) 546:421–5. doi: 10.1038/nature22360
28. Duhen T, Duhen R, Lanzavecchia A, Sallusto F, Campbell DJ. Functionally distinct subsets of human FOXP3+ Treg cells that phenotypically mirror effector Th cells. Blood. (2012) 119:4430–40. doi: 10.1182/blood-2011-11-392324
29. Zhou X, Bailey-Bucktrout SL, Jeker LT, Penaranda C, Martinez-Llordella M, Ashby M, et al. Instability of the transcription factor Foxp3 leads to the generation of pathogenic memory T cells in vivo. Nat Immunol. (2009) 10:1000–7. doi: 10.1038/ni.1774
30. Zhou X, Bailey-Bucktrout S, Jeker LT, Bluestone JA. Plasticity of CD4(+) FoxP3(+) T cells. Curr Opin Immunol. (2009) 21:281–5. doi: 10.1016/j.coi.2009.05.007
31. McClymont SA, Putnam AL, Lee MR, Esensten JH, Liu W, Hulme MA, et al. Plasticity of human regulatory T cells in healthy subjects and patients with type 1 diabetes. J Immunol. (2011) 186:3918–26. doi: 10.4049/jimmunol.1003099
32. Seay HR, Putnam AL, Cserny J, Posgai AL, Rosenau EH, Wingard JR, et al. Expansion of human tregs from cryopreserved umbilical cord blood for gmp-compliant autologous adoptive cell transfer therapy. Mol Ther Methods Clin Dev. (2017) 4:178–91. doi: 10.1016/j.omtm.2016.12.003
33. Dobin A, Davis CA, Schlesinger F, Drenkow J, Zaleski C, Jha S, et al. STAR: ultrafast universal RNA-seq aligner. Bioinformatics. (2013) 29:15–21. doi: 10.1093/bioinformatics/bts635
34. Lun ATL, Riesenfeld S, Andrews T, Dao TP, Gomes T, Atlas J, et al. EmptyDrops: distinguishing cells from empty droplets in droplet-based single-cell RNA sequencing data. Genome Biol. (2019) 20:63. doi: 10.1186/s13059-019-1662-y
35. Lun AT, McCarthy DJ, Marioni JC. A step-by-step workflow for low-level analysis of single-cell RNA-seq data with Bioconductor. F1000Res. (2016) 5:2122. doi: 10.12688/f1000research.9501.2
36. Hafemeister C, Satija R. Normalization and variance stabilization of single-cell RNA-seq data using regularized negative binomial regression. Genome Biol. (2019) 20:296. doi: 10.1186/s13059-019-1874-1
37. Butler A, Hoffman P, Smibert P, Papalexi E, Satija R. Integrating single-cell transcriptomic data across different conditions, technologies, and species. Nat Biotechnol. (2018) 36:411–20. doi: 10.1038/nbt.4096
38. Stuart T, Butler A, Hoffman P, Hafemeister C, Papalexi E, Mauck WM III, et al. Comprehensive integration of single-cell data. Cell. (2019) 177:1888–902.e21. doi: 10.1016/j.cell.2019.05.031
39. Haghverdi L, Buettner F, Theis FJ. Diffusion maps for high-dimensional single-cell analysis of differentiation data. Bioinformatics. (2015) 31:2989–98. doi: 10.1093/bioinformatics/btv325
40. Zappia L, Oshlack A. Clustering trees: a visualization for evaluating clusterings at multiple resolutions. Gigascience. (2018) 7:giy083. doi: 10.1093/gigascience/giy083
41. Korsunsky I, Nathan A, Millard N, Raychaudhuri S. Presto scales Wilcoxon and auROC analyses to millions of observations. BioRxiv [Preprint]. (2019): doi: 10.1101/653253
43. Greiff V, Bhat P, Cook SC, Menzel U, Kang W, Reddy ST. A bioinformatic framework for immune repertoire diversity profiling enables detection of immunological status. Genome Med. (2015) 7:49. doi: 10.1186/s13073-015-0169-8
44. Brown AJ, Snapkov I, Akbar R, Pavloviæ M, Miho E, Sandve GK, et al. Augmenting adaptive immunity: progress and challenges in the quantitative engineering and analysis of adaptive immune receptor repertoires. Mol Syst Des Eng. (2019) 4:701–36. doi: 10.1039/C9ME00071B
45. Fuhrman CA, Yeh WI, Seay HR, Saikumar Lakshmi P, Chopra G, Zhang L, et al. Divergent phenotypes of human regulatory T cells expressing the receptors TIGIT and CD226. J Immunol. (2015) 195:145–55. doi: 10.4049/jimmunol.1402381
46. Ritchie ME, Phipson B, Wu D, Hu Y, Law CW, Shi W, et al. Limma powers differential expression analyses for RNA-sequencing and microarray studies. Nucleic Acids Res. (2015) 43:e47. doi: 10.1093/nar/gkv007
47. Bates D, Machler M, Bolker BM, Walker SC. Fitting linear mixed-effects models using lme4. J Stat Softw. (2015) 67:1–48.
48. Lenth R. emmeans: Estimated Marginal Means, aka Least-Squares Means. R Package Version 1.3.3. (2019).
50. Gu Z, Eils R, Schlesner M. Complex heatmaps reveal patterns and correlations in multidimensional genomic data. Bioinformatics. (2016) 32:2847–9. doi: 10.1093/bioinformatics/btw313
51. Motwani K. scanalysis. GitHub Repository. (2020). Available online at: https://github.com/keshav-motwani/scanalysis (accessed March 2, 2020).
52. Motwani K. ggexp. GitHub Repository. (2020). Available online at: https://github.com/keshav-motwani/ggexp (accessed March 2, 2020).
53. Motwani K. Treg paper. GitHub Repository. (2020). Available online at: https://github.com/keshav-motwani/tregPaper (accessed March 2, 2020).
54. Becht E, McInnes L, Healy J, Dutertre CA, Kwok IWH, Ng LG, et al. Dimensionality reduction for visualizing single-cell data using UMAP. Nat Biotechnol. (2018) 37:38–44. doi: 10.1038/nbt.4314
55. Shadidi KR, Aarvak T, Henriksen JE, Natvig JB, Thompson KM. The chemokines CCL5, CCL2 and CXCL12 play significant roles in the migration of Th1 cells into rheumatoid synovial tissue. Scand J Immunol. (2003) 57:192–8.
56. Wadwa M, Klopfleisch R, Adamczyk A, Frede A, Pastille E, Mahnke K, et al. IL-10 downregulates CXCR3 expression on Th1 cells and interferes with their migration to intestinal inflammatory sites. Mucosal Immunol. (2016) 9:1263–77. doi: 10.1038/mi.2015.132
57. Hahtola S, Tuomela S, Elo L, Hakkinen T, Karenko L, Nedoszytko B, et al. Th1 response and cytotoxicity genes are down-regulated in cutaneous T-cell lymphoma. Clin Cancer Res. (2006) 12:4812–21. doi: 10.1158/1078-0432.CCR-06-0532
58. Riaz T, Sollid LM, Olsen I, de Souza GA. Quantitative proteomics of gut-derived Th1 and Th1/Th17 clones reveal the presence of CD28+ NKG2D- Th1 cytotoxic CD4+ T cells. Mol Cell Proteomics. (2016) 15:1007–16. doi: 10.1074/mcp.M115.050138
59. Wu Y, Liu M, Li Z, Wu XB, Wang Y, Wang Y, et al. LYAR promotes colorectal cancer cell mobility by activating galectin-1 expression. Oncotarget. (2015) 6:32890–901. doi: 10.18632/oncotarget.5335
60. Yu F, Sharma S, Jankovic D, Gurram RK, Su P, Hu G, et al. The transcription factor Bhlhe40 is a switch of inflammatory versus antiinflammatory Th1 cell fate determination. J Exp Med. (2018) 215:1813–21. doi: 10.1084/jem.20170155
61. Halim L, Romano M, McGregor R, Correa I, Pavlidis P, Grageda N, et al. An atlas of human regulatory T helper-like cells reveals features of Th2-like tregs that support a tumorigenic environment. Cell Rep. (2017) 20:757–70. doi: 10.1016/j.celrep.2017.06.079
62. Kanhere A, Hertweck A, Bhatia U, Gokmen MR, Perucha E, Jackson I, et al. T-bet and GATA3 orchestrate Th1 and Th2 differentiation through lineage-specific targeting of distal regulatory elements. Nat Commun. (2012) 3:1268. doi: 10.1038/ncomms2260
63. Cosmi L, De Palma R, Santarlasci V, Maggi L, Capone M, Frosali F, et al. Human interleukin 17-producing cells originate from a CD161+CD4+ T cell precursor. J Exp Med. (2008) 205:1903–16. doi: 10.1084/jem.20080397
64. Gutcher I, Donkor MK, Ma Q, Rudensky AY, Flavell RA, Li MO. Autocrine transforming growth factor-beta1 promotes in vivo Th17 cell differentiation. Immunity. (2011) 34:396–408. doi: 10.1016/j.immuni.2011.03.005
65. Duhen T, Campbell DJ. IL-1beta promotes the differentiation of polyfunctional human CCR6+CXCR3+ Th1/17 cells that are specific for pathogenic and commensal microbes. J Immunol. (2014) 193:120–9. doi: 10.4049/jimmunol.1302734
66. Cohen CJ, Crome SQ, MacDonald KG, Dai EL, Mager DL, Levings MK. Human Th1 and Th17 cells exhibit epigenetic stability at signature cytokine and transcription factor loci. J Immunol. (2011) 187:5615–26. doi: 10.4049/jimmunol.1101058
67. Pohar J, Simon Q, Fillatreau S. Antigen-specificity in the thymic development and peripheral activity of CD4(+)FOXP3(+) T regulatory cells. Front Immunol. (2018) 9:1701. doi: 10.3389/fimmu.2018.01701
68. Seay HR, Yusko E, Rothweiler SJ, Zhang L, Posgai AL, Campbell-Thompson M, et al. Tissue distribution and clonal diversity of the T and B cell repertoire in type 1 diabetes. JCI Insight. (2016) 1:e88242. doi: 10.1172/jci.insight.88242
69. Wang C, Sanders CM, Yang Q, Schroeder HW Jr., Wang E, Babrzadeh F, et al. High throughput sequencing reveals a complex pattern of dynamic interrelationships among human T cell subsets. Proc Natl Acad Sci USA. (2010) 107:1518–23. doi: 10.1073/pnas.0913939107
70. Kasow KA, Chen X, Knowles J, Wichlan D, Handgretinger R, Riberdy JM. Human CD4+CD25+ regulatory T cells share equally complex and comparable repertoires with CD4+CD25- counterparts. J Immunol. (2004) 172:6123–8. doi: 10.4049/jimmunol.172.10.6123
71. Ritvo PG, Saadawi A, Barennes P, Quiniou V, Chaara W, El Soufi K, et al. High-resolution repertoire analysis reveals a major bystander activation of Tfh and Tfr cells. Proc Natl Acad Sci USA. (2018) 115:9604–9. doi: 10.1073/pnas.1808594115
72. Katagiri T, Yamazaki S, Fukui Y, Aoki K, Yagita H, Nishina T, et al. JunB plays a crucial role in development of regulatory T cells by promoting IL-2 signaling. Mucosal Immunol. (2019) 12:1104–17. doi: 10.1038/s41385-019-0182-0
73. Lu D, Liu L, Ji X, Gao Y, Chen X, Liu Y, et al. The phosphatase DUSP2 controls the activity of the transcription activator STAT3 and regulates TH17 differentiation. Nat Immunol. (2015) 16:1263–73. doi: 10.1038/ni.3278
74. Klann JE, Kim SH, Remedios KA, He Z, Metz PJ, Lopez J, et al. Integrin activation controls regulatory T cell-mediated peripheral tolerance. J Immunol. (2018) 200:4012–23. doi: 10.4049/jimmunol.1800112
75. Galgani M, De Rosa V, La Cava A, Matarese G. Role of metabolism in the immunobiology of regulatory T cells. J Immunol. (2016) 197:2567–75. doi: 10.4049/jimmunol.1600242
76. Bin Dhuban K, d’Hennezel E, Nashi E, Bar-Or A, Rieder S, Shevach EM, et al. Coexpression of TIGIT and FCRL3 identifies Helios+ human memory regulatory T cells. J Immunol. (2015) 194:3687–96. doi: 10.4049/jimmunol.1401803
77. Rauch KS, Hils M, Lupar E, Minguet S, Sigvardsson M, Rottenberg ME, et al. Id3 maintains Foxp3 expression in regulatory T cells by controlling a transcriptional network of E47, Spi-B, and SOCS3. Cell Rep. (2016) 17:2827–36. doi: 10.1016/j.celrep.2016.11.045
78. Weatherly K, Bettonville M, Torres D, Kohler A, Goriely S, Braun MY. Functional profile of S100A4-deficient T cells. Immun Inflamm Dis. (2015) 3:431–44. doi: 10.1002/iid3.85
79. Yu M, Li G, Lee WW, Yuan M, Cui D, Weyand CM, et al. Signal inhibition by the dual-specific phosphatase 4 impairs T cell-dependent B-cell responses with age. Proc Natl Acad Sci USA. (2012) 109:E879–88. doi: 10.1073/pnas.1109797109
80. van Aalderen MC, van den Biggelaar M, Remmerswaal EBM, van Alphen FPJ, Meijer AB, Ten Berge IJM, et al. Label-free analysis of CD8(+) T Cell subset proteomes supports a progressive differentiation model of human-virus-specific T cells. Cell Rep. (2017) 19:1068–79. doi: 10.1016/j.celrep.2017.04.014
81. Weng NP, Araki Y, Subedi K. The molecular basis of the memory T cell response: differential gene expression and its epigenetic regulation. Nat Rev Immunol. (2012) 12:306–15. doi: 10.1038/nri3173
82. Ronchetti S, Ricci E, Petrillo MG, Cari L, Migliorati G, Nocentini G, et al. Glucocorticoid-induced tumour necrosis factor receptor-related protein: a key marker of functional regulatory T cells. J Immunol Res. (2015) 2015:171520. doi: 10.1155/2015/171520
83. Nagar M, Jacob-Hirsch J, Vernitsky H, Berkun Y, Ben-Horin S, Amariglio N, et al. TNF activates a NF-kappaB-regulated cellular program in human CD45RA- regulatory T cells that modulates their suppressive function. J Immunol. (2010) 184:3570–81. doi: 10.4049/jimmunol.0902070
84. Bankoti R, Ogawa C, Nguyen T, Emadi L, Couse M, Salehi S, et al. Differential regulation of Effector and Regulatory T cell function by Blimp1. Sci Rep. (2017) 7:12078. doi: 10.1038/s41598-017-12171-3
85. Cuadrado E, van den Biggelaar M, de Kivit S, Chen YY, Slot M, Doubal I, et al. Proteomic analyses of human regulatory T cells reveal adaptations in signaling pathways that protect cellular identity. Immunity. (2018) 48:1046–59.e6. doi: 10.1016/j.immuni.2018.04.008
86. Sugiyama D, Nishikawa H, Maeda Y, Nishioka M, Tanemura A, Katayama I, et al. Anti-CCR4 mAb selectively depletes effector-type FoxP3+CD4+ regulatory T cells, evoking antitumor immune responses in humans. Proc Natl Acad Sci USA. (2013) 110:17945–50. doi: 10.1073/pnas.1316796110
87. Zheng Y, Chaudhry A, Kas A, deRoos P, Kim JM, Chu TT, et al. Regulatory T-cell suppressor program co-opts transcription factor IRF4 to control T(H)2 responses. Nature. (2009) 458:351–6. doi: 10.1038/nature07674
88. Nowak A, Lock D, Bacher P, Hohnstein T, Vogt K, Gottfreund J, et al. CD137+CD154- expression as a regulatory T cell (Treg)-specific activation signature for identification and sorting of stable human tregs from in vitro expansion cultures. Front Immunol. (2018) 9:199. doi: 10.3389/fimmu.2018.00199
89. Wang C, Kang SG, Lee J, Sun Z, Kim CH. The roles of CCR6 in migration of Th17 cells and regulation of effector T-cell balance in the gut. Mucosal Immunol. (2009) 2:173–83. doi: 10.1038/mi.2008.84
90. Castro G, Liu X, Ngo K, De Leon-Tabaldo A, Zhao S, Luna-Roman R, et al. RORgammat and RORalpha signature genes in human Th17 cells. PLoS One. (2017) 12:e0181868. doi: 10.1371/journal.pone.0181868
91. Tickotsky N, Sagiv T, Prilusky J, Shifrut E, Friedman N. McPAS-TCR: a manually curated catalogue of pathology-associated T cell receptor sequences. Bioinformatics. (2017) 33:2924–9. doi: 10.1093/bioinformatics/btx286
92. Riley JL, June CH, Blazar BR. Human T regulatory cell therapy: take a billion or so and call me in the morning. Immunity. (2009) 30:656–65. doi: 10.1016/j.immuni.2009.04.006
93. Bhairavabhotla R, Kim YC, Glass DD, Escobar TM, Patel MC, Zahr R, et al. Transcriptome profiling of human FoxP3+ regulatory T cells. Hum Immunol. (2016) 77:201–13. doi: 10.1016/j.humimm.2015.12.004
94. Probst-Kepper M, Geffers R, Kroger A, Viegas N, Erck C, Hecht HJ, et al. GARP: a key receptor controlling FOXP3 in human regulatory T cells. J Cell Mol Med. (2009) 13:3343–57. doi: 10.1111/j.1582-4934.2009.00782.x
95. Ostroukhova M, Qi Z, Oriss TB, Dixon-McCarthy B, Ray P, Ray A. Treg-mediated immunosuppression involves activation of the Notch-HES1 axis by membrane-bound TGF-beta. J Clin Invest. (2006) 116:996–1004. doi: 10.1172/JCI26490
96. Kuwahara M, Yamashita M, Shinoda K, Tofukuji S, Onodera A, Shinnakasu R, et al. The transcription factor Sox4 is a downstream target of signaling by the cytokine TGF-beta and suppresses T(H)2 differentiation. Nat Immunol. (2012) 13:778–86. doi: 10.1038/ni.2362
97. Ostler N, Britzen-Laurent N, Liebl A, Naschberger E, Lochnit G, Ostler M, et al. Gamma interferon-induced guanylate binding protein 1 is a novel actin cytoskeleton remodeling factor. Mol Cell Biol. (2014) 34:196–209. doi: 10.1128/MCB.00664-13
98. Ma H, Lu C, Ziegler J, Liu A, Sepulveda A, Okada H, et al. Absence of Stat1 in donor CD4(+) T cells promotes the expansion of Tregs and reduces graft-versus-host disease in mice. J Clin Invest. (2011) 121:2554–69. doi: 10.1172/JCI43706
99. Stancic A, Jandl K, Hasenohrl C, Reichmann F, Marsche G, Schuligoi R, et al. The GPR55 antagonist CID16020046 protects against intestinal inflammation. Neurogastroenterol Motil. (2015) 27:1432–45. doi: 10.1111/nmo.12639
100. Kunzli K, Favre B, Chofflon M, Borradori L. One gene but different proteins and diseases: the complexity of dystonin and bullous pemphigoid antigen 1. Exp Dermatol. (2016) 25:10–6. doi: 10.1111/exd.12877
101. Lu R, Qu Y, Ge J, Zhang L, Su Z, Pflugfelder SC, et al. Transcription factor TCF4 maintains the properties of human corneal epithelial stem cells. Stem Cells. (2012) 30:753–61. doi: 10.1002/stem.1032
102. Paster W, Brockmeyer C, Fu G, Simister PC, de Wet B, Martinez-Riano A, et al. GRB2-mediated recruitment of THEMIS to LAT is essential for thymocyte development. J Immunol. (2013) 190:3749–56. doi: 10.4049/jimmunol.1203389
103. Rich BE, Kupper TS. Cytokines: IL-20 – a new effector in skin inflammation. Curr Biol. (2001) 11:R531–4.
104. Parks OB, Pociask DA, Hodzic Z, Kolls JK, Good M. Interleukin-22 signaling in the regulation of intestinal health and disease. Front Cell Dev Biol. (2015) 3:85. doi: 10.3389/fcell.2015.00085
105. Larochette V, Miot C, Poli C, Beaumont E, Roingeard P, Fickenscher H, et al. IL-26, a cytokine with roles in extracellular DNA-induced inflammation and microbial defense. Front Immunol. (2019) 10:204. doi: 10.3389/fimmu.2019.00204
106. Hibbert L, Pflanz S, De Waal Malefyt R, Kastelein RA. IL-27 and IFN-alpha signal via Stat1 and Stat3 and induce T-Bet and IL-12Rbeta2 in naive T cells. J Interferon Cytokine Res. (2003) 23:513–22. doi: 10.1089/10799900360708632
107. Athie-Morales V, Smits HH, Cantrell DA, Hilkens CM. Sustained IL-12 signaling is required for Th1 development. J Immunol. (2004) 172:61–9. doi: 10.4049/jimmunol.172.1.61
108. Dolganiuc A, Kodys K, Marshall C, Saha B, Zhang S, Bala S, et al. Type III interferons, IL-28 and IL-29, are increased in chronic HCV infection and induce myeloid dendritic cell-mediated FoxP3+ regulatory T cells. PLoS One. (2012) 7:e44915. doi: 10.1371/journal.pone.0044915
109. Canto E, Garcia Planella E, Zamora-Atenza C, Nieto JC, Gordillo J, Ortiz MA, et al. Interleukin-19 impairment in active Crohn’s disease patients. PLoS One. (2014) 9:e93910. doi: 10.1371/journal.pone.0093910
110. Wei X, Zhang J, Gu Q, Huang M, Zhang W, Guo J, et al. Reciprocal expression of IL-35 and IL-10 defines two distinct effector treg subsets that are required for maintenance of immune tolerance. Cell Rep. (2017) 21:1853–69. doi: 10.1016/j.celrep.2017.10.090
111. de la Rosa M, Rutz S, Dorninger H, Scheffold A. Interleukin-2 is essential for CD4+CD25+ regulatory T cell function. Eur J Immunol. (2004) 34:2480–8. doi: 10.1002/eji.200425274
112. Alonso R, Flament H, Lemoine S, Sedlik C, Bottasso E, Peguillet I, et al. Induction of anergic or regulatory tumor-specific CD4(+) T cells in the tumor-draining lymph node. Nat Commun. (2018) 9:2113. doi: 10.1038/s41467-018-04524-x
113. Le Gallo M, Poissonnier A, Blanco P, Legembre P. CD95/Fas, non-apoptotic signaling pathways, and kinases. Front Immunol. (2017) 8:1216. doi: 10.3389/fimmu.2017.01216
114. Woods DM, Ramakrishnan R, Laino AS, Berglund A, Walton K, Betts BC, et al. Decreased suppression and increased phosphorylated STAT3 in regulatory T cells are associated with benefit from adjuvant PD-1 blockade in resected metastatic melanoma. Clin Cancer Res. (2018) 24:6236–47. doi: 10.1158/1078-0432.CCR-18-1100
115. Zhang R, Huynh A, Whitcher G, Chang J, Maltzman JS, Turka LA. An obligate cell-intrinsic function for CD28 in Tregs. J Clin Invest. (2013) 123:580–93. doi: 10.1172/JCI65013
116. He X, Smeets RL, van Rijssen E, Boots AM, Joosten I, Koenen HJ. Single CD28 stimulation induces stable and polyclonal expansion of human regulatory T cells. Sci Rep. (2017) 7:43003. doi: 10.1038/srep43003
117. Banham AH, Powrie FM, Suri-Payer E. FOXP3+ regulatory T cells: current controversies and future perspectives. Eur J Immunol. (2006) 36:2832–6. doi: 10.1002/eji.200636459
118. Campbell JJ, Murphy KE, Kunkel EJ, Brightling CE, Soler D, Shen Z, et al. CCR7 expression and memory T cell diversity in humans. J Immunol. (2001) 166:877–84. doi: 10.4049/jimmunol.166.2.877
119. Yoshie O, Matsushima K. CCR4 and its ligands: from bench to bedside. Int Immunol. (2015) 27:11–20. doi: 10.1093/intimm/dxu079
120. Kunicki MA, Amaya Hernandez LC, Davis KL, Bacchetta R, Roncarolo MG. Identity and diversity of human peripheral Th and T regulatory cells defined by single-cell mass cytometry. J Immunol. (2018) 200:336–46. doi: 10.4049/jimmunol.1701025
121. Levings MK, Sangregorio R, Galbiati F, Squadrone S, de Waal Malefyt R, Roncarolo MG. IFN-alpha and IL-10 induce the differentiation of human type 1 T regulatory cells. J Immunol. (2001) 166:5530–9. doi: 10.4049/jimmunol.166.9.5530
122. Le Buanec H, Gougeon ML, Mathian A, Lebon P, Dupont JM, Peltre G, et al. IFN-alpha and CD46 stimulation are associated with active lupus and skew natural T regulatory cell differentiation to type 1 regulatory T (Tr1) cells. Proc Natl Acad Sci USA. (2011) 108:18995–9000. doi: 10.1073/pnas.1113301108
123. Brockmann L, Gagliani N, Steglich B, Giannou AD, Kempski J, Pelczar P, et al. IL-10 receptor signaling is essential for TR1 cell function in vivo. J Immunol. (2017) 198:1130–41. doi: 10.4049/jimmunol.1601045
124. Gagliani N, Magnani CF, Huber S, Gianolini ME, Pala M, Licona-Limon P, et al. Coexpression of CD49b and LAG-3 identifies human and mouse T regulatory type 1 cells. Nat Med. (2013) 19:739–46. doi: 10.1038/nm.3179
125. Bluestone JA, Tang Q. Treg cells-the next frontier of cell therapy. Science. (2018) 362:154–5. doi: 10.1126/science.aau2688
126. Sakaguchi S, Wing K, Onishi Y, Prieto-Martin P, Yamaguchi T. Regulatory T cells: how do they suppress immune responses? Int Immunol. (2009) 21:1105–11. doi: 10.1093/intimm/dxp095
127. Garg G, Tyler JR, Yang JH, Cutler AJ, Downes K, Pekalski M, et al. Type 1 diabetes-associated IL2RA variation lowers IL-2 signaling and contributes to diminished CD4+CD25+ regulatory T cell function. J Immunol. (2012) 188:4644–53. doi: 10.4049/jimmunol.1100272
128. Svensson MN, Doody KM, Schmiedel BJ, Bhattacharyya S, Panwar B, Wiede F, et al. Reduced expression of phosphatase PTPN2 promotes pathogenic conversion of Tregs in autoimmunity. J Clin Invest. (2019) 129:1193–210. doi: 10.1172/JCI123267
129. Liu J, Zhang H. -1722T/C polymorphism (rs733618) of CTLA-4 significantly associated with systemic lupus erythematosus (SLE): a comprehensive meta-analysis. Hum Immunol. (2013) 74:341–7. doi: 10.1016/j.humimm.2012.12.009
130. Sumida T, Lincoln MR, Ukeje CM, Rodriguez DM, Akazawa H, Noda T, et al. Activated beta-catenin in Foxp3(+) regulatory T cells links inflammatory environments to autoimmunity. Nat Immunol. (2018) 19:1391–402. doi: 10.1038/s41590-018-0236-6
131. Kitz A, de Marcken M, Gautron AS, Mitrovic M, Hafler DA, Dominguez-Villar M. AKT isoforms modulate Th1-like Treg generation and function in human autoimmune disease. EMBO Rep. (2016) 17:1169–83. doi: 10.15252/embr.201541905
132. Groom JR, Luster AD. CXCR3 in T cell function. Exp Cell Res. (2011) 317:620–31. doi: 10.1016/j.yexcr.2010.12.017
133. Liu J, Guan X, Ma X. Interferon regulatory factor 1 is an essential and direct transcriptional activator for interferon {gamma}-induced RANTES/CCl5 expression in macrophages. J Biol Chem. (2005) 280:24347–55. doi: 10.1074/jbc.M500973200
134. Choi J, Ziga ED, Ritchey J, Collins L, Prior JL, Cooper ML, et al. IFNgammaR signaling mediates alloreactive T-cell trafficking and GVHD. Blood. (2012) 120:4093–103. doi: 10.1182/blood-2012-01-403196
135. Koga S, Kapoor A, Novick AC, Toma H, Fairchild RL. RANTES is produced by CD8+ T cells during acute rejection of skin grafts. Transplant Proc. (2000) 32:796–7. doi: 10.1016/s0041-1345(00)00986-6
136. Malchow S, Leventhal DS, Lee V, Nishi S, Socci ND, Savage PA. Aire enforces immune tolerance by directing autoreactive t cells into the regulatory T cell lineage. Immunity. (2016) 44:1102–13. doi: 10.1016/j.immuni.2016.02.009
137. Paul M, Dayal D, Bhansali A, Dhaliwal L, Sachdeva N. In vitro assessment of cord blood-derived proinsulin-specific regulatory T cells for cellular therapy in type 1 diabetes. Cytotherapy. (2018) 20:1355–70. doi: 10.1016/j.jcyt.2018.09.004
138. Brusko TM, Koya RC, Zhu S, Lee MR, Putnam AL, McClymont SA, et al. Human antigen-specific regulatory T cells generated by T cell receptor gene transfer. PLoS One. (2010) 5:e11726. doi: 10.1371/journal.pone.0011726
139. Yeh WI, Seay HR, Newby B, Posgai AL, Moniz FB, Michels A, et al. Avidity and bystander suppressive capacity of human regulatory T cells expressing de novo autoreactive T-cell receptors in type 1 diabetes. Front Immunol. (2017) 8:1313. doi: 10.3389/fimmu.2017.01313
140. Boroughs AC, Larson RC, Choi BD, Bouffard AA, Riley LS, Schiferle E, et al. Chimeric antigen receptor costimulation domains modulate human regulatory T cell function. JCI Insight. (2019) 5:e126194. doi: 10.1172/jci.insight.126194
141. Metkar SS, Menaa C, Pardo J, Wang B, Wallich R, Freudenberg M, et al. Human and mouse granzyme a induce a proinflammatory cytokine response. Immunity. (2008) 29:720–33. doi: 10.1016/j.immuni.2008.08.014
142. Afonina IS, Tynan GA, Logue SE, Cullen SP, Bots M, Luthi AU, et al. Granzyme B-dependent proteolysis acts as a switch to enhance the proinflammatory activity of IL-1alpha. Mol Cell. (2011) 44:265–78. doi: 10.1016/j.molcel.2011.07.037
143. Tewary P, Yang D, de la Rosa G, Li Y, Finn MW, Krensky AM, et al. Granulysin activates antigen-presenting cells through TLR4 and acts as an immune alarmin. Blood. (2010) 116:3465–74. doi: 10.1182/blood-2010-03-273953
144. Liu W, Putnam AL, Xu-Yu Z, Szot GL, Lee MR, Zhu S, et al. CD127 expression inversely correlates with FoxP3 and suppressive function of human CD4+ T reg cells. J Exp Med. (2006) 203:1701–11. doi: 10.1084/jem.20060772
145. Vandenbroeck K. Cytokine gene polymorphisms and human autoimmune disease in the era of genome-wide association studies. J Interferon Cytokine Res. (2012) 32:139–51. doi: 10.1089/jir.2011.0103
146. Panarina M, Kisand K, Alnek K, Heilman K, Peet A, Uibo R. Interferon and interferon-inducible gene activation in patients with type 1 diabetes. Scand J Immunol. (2014) 80:283–92. doi: 10.1111/sji.12204
147. Wei J, Duramad O, Perng OA, Reiner SL, Liu YJ, Qin FX. Antagonistic nature of T helper 1/2 developmental programs in opposing peripheral induction of Foxp3+ regulatory T cells. Proc Natl Acad Sci USA. (2007) 104:18169–74. doi: 10.1073/pnas.0703642104
148. Koguchi Y, Buenafe AC, Thauland TJ, Gardell JL, Bivins-Smith ER, Jacoby DB, et al. Preformed CD40L is stored in Th1, Th2, Th17, and T follicular helper cells as well as CD4+ 8- thymocytes and invariant NKT cells but not in Treg cells. PLoS One. (2012) 7:e31296. doi: 10.1371/journal.pone.0031296
149. Inshaw JRJ, Walker NM, Wallace C, Bottolo L, Todd JA. The chromosome 6q22.33 region is associated with age at diagnosis of type 1 diabetes and disease risk in those diagnosed under 5 years of age. Diabetologia. (2018) 61:147–57. doi: 10.1007/s00125-017-4440-y
150. Yang ZZ, Kim HJ, Jalali S, Wu H, Price-Troska T, Ansell SM. Tigit expression defines a subset of activated Treg cells with prognostic relevance in follicular lymphoma. Blood. (2018) 132:1590. doi: 10.1182/blood-2018-99-115328
151. Schaier M, Seissler N, Becker LE, Schaefer SM, Schmitt E, Meuer S, et al. The extent of HLA-DR expression on HLA-DR(+) Tregs allows the identification of patients with clinically relevant borderline rejection. Transpl Int. (2013) 26:290–9. doi: 10.1111/tri.12032
152. Gianchecchi E, Fierabracci A. Inhibitory receptors and pathways of lymphocytes: the role of PD-1 in Treg development and their involvement in autoimmunity onset and cancer progression. Front Immunol. (2018) 9:2374. doi: 10.3389/fimmu.2018.02374
154. Lowther DE, Goods BA, Lucca LE, Lerner BA, Raddassi K, van Dijk D, et al. PD-1 marks dysfunctional regulatory T cells in malignant gliomas. JCI Insight. (2016) 1:e85935. doi: 10.1172/jci.insight.85935
Keywords: cord blood, peripheral blood, regulatory T cells, Tregs, adoptive cell therapy, scRNA-seq, immunosequencing
Citation: Motwani K, Peters LD, Vliegen WH, El-sayed AG, Seay HR, Lopez MC, Baker HV, Posgai AL, Brusko MA, Perry DJ, Bacher R, Larkin J, Haller MJ and Brusko TM (2020) Human Regulatory T Cells From Umbilical Cord Blood Display Increased Repertoire Diversity and Lineage Stability Relative to Adult Peripheral Blood. Front. Immunol. 11:611. doi: 10.3389/fimmu.2020.00611
Received: 14 September 2019; Accepted: 17 March 2020;
Published: 15 April 2020.
Edited by:
Lucy S. K. Walker, University College London, United KingdomReviewed by:
Markus Kleinewietfeld, VIB-UGent Center for Inflammation Research (IRC), BelgiumCopyright © 2020 Motwani, Peters, Vliegen, El-sayed, Seay, Lopez, Baker, Posgai, Brusko, Perry, Bacher, Larkin, Haller and Brusko. This is an open-access article distributed under the terms of the Creative Commons Attribution License (CC BY). The use, distribution or reproduction in other forums is permitted, provided the original author(s) and the copyright owner(s) are credited and that the original publication in this journal is cited, in accordance with accepted academic practice. No use, distribution or reproduction is permitted which does not comply with these terms.
*Correspondence: Todd M. Brusko, dGJydXNrb0B1ZmwuZWR1
†These authors have contributed equally to this work and share first authorship
‡Present address: Howard R. Seay, FlowJo, LLC, Ashland, OR, United States
Disclaimer: All claims expressed in this article are solely those of the authors and do not necessarily represent those of their affiliated organizations, or those of the publisher, the editors and the reviewers. Any product that may be evaluated in this article or claim that may be made by its manufacturer is not guaranteed or endorsed by the publisher.
Research integrity at Frontiers
Learn more about the work of our research integrity team to safeguard the quality of each article we publish.