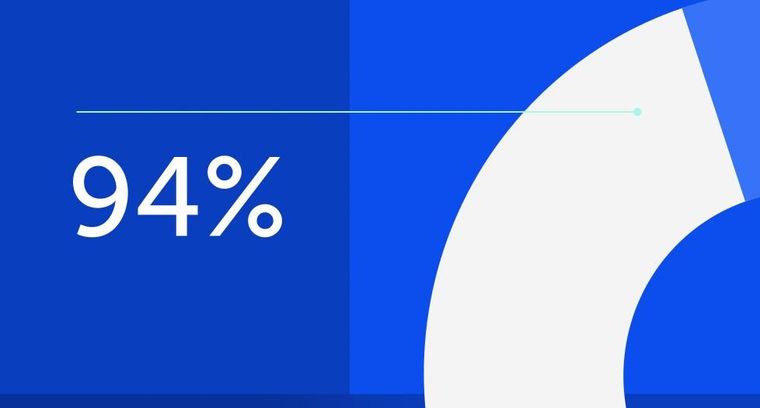
94% of researchers rate our articles as excellent or good
Learn more about the work of our research integrity team to safeguard the quality of each article we publish.
Find out more
ORIGINAL RESEARCH article
Front. Immunol., 24 January 2020
Sec. Primary Immunodeficiencies
Volume 11 - 2020 | https://doi.org/10.3389/fimmu.2020.00014
This article is part of the Research TopicNon-Infectious Complications of Primary Antibody DeficiencyView all 12 articles
The pathogenesis in the majority of patients with common variable immunodeficiency (CVID), the most common symptomatic primary immunodeficiency, remains unknown. We aimed to compare the minor and major histocompatibility complex (MHC) markers as well as polygenic scores of common genetic variants between patients with monogenic CVID and without known genetic mutation detected. Monogenic patients were identified in a CVID cohort using whole exome sequencing. Computational full-resolution MHC typing and confirmatory PCR amplicon-based high-resolution typing were performed. Exome-wide polygenic scores were developed using significantly different variants and multi-variant Mendelian randomization (MR) analyses were used to test the causality of significant genetic variants on antibody levels and susceptibility to infectious diseases. Among 83 CVID patients (44.5% females), monogenic defects were found in 40 individuals. Evaluation of the remaining CVID patients without known genetic mutation detected showed 13 and 27 significantly associated MHC-class I and II alleles, respectively. The most significant partial haplotype linked with the unsolved CVID was W*01:01:01-DMA*01:01:01-DMB*01:03:01:02-TAP1*01:01:01 (P < 0.001), where carriers had a late onset of the disease, only infection clinical phenotype, a non-familial form of CVID, post-germinal center defects and a non-progressive form of their disease. Exclusion of monogenic diseases allowed MR analyses to identify significant genetic variants associated with bacterial infections and improved discrepancies observed in MR analyses of previous GWAS studies with low pleiotropy mainly for a lower respiratory infection, bacterial infection and Streptococcal infection. This is the first study on the full-resolution of minor and major MHC typing and polygenic scores on CVID patients and showed that exclusion of monogenic forms of the disease unraveled an independent role of MHC genes and common genetic variants in the pathogenesis of CVID.
Common variable immunodeficiency (CVID) is the most common symptomatic primary immunodeficiency (PID), characterized by impairment of antibody production, recurrent infections and immune dysregulation, in particular, autoimmunity (1). Several different pathogeneses have been suggested from which monogenic diseases cover between 10 and 20% (in Western cohorts) up to 68% (in countries with a high rate of consanguinity) (2–4). Approximately 400 genes have been identified as causative defects of PID, from which half of them have been linked to impaired antibody production and CVID (2). However, additional genetic and non-genetic models have also been considered for CVID (5–7).
Minor and major histocompatibility complex (MHC) genes are the most polymorphic genomic region and specific MHC loci determine the presentation of antigens via B cells to T cells to elicit a germinal center reaction. Physiologically, both MHC class I and II molecules are critical in B cells for stimulating antibody class switching and affinity maturation (MHC class II primarily to follicular helper T cells) and supporting presentation of polysaccharides antigens (mainly via positive signals of MHC class I primarily to natural killer T-cells) (8–10). Given the high prevalence of autoimmune disorders in CVID patients, several studies have investigated the frequency of different MHC alleles in subgroups of CVID patients (11, 12). Furthermore, few reports from different ethnic CVID cohorts have also emphasized a possible contribution of the MHC, mainly class II molecules in patients with gastrointestinal autoimmunity, on the predisposition to CVID (13). In some multiplex families with co-occurrence of CVID and selective immunoglobulin A (IgA), deficiency, MHC markers have also been suggested to play a role both in inheritance and as predictors of progressive disease (14).
However, in CVID patients with a lack of identified monogenic mutations, the disease may occur due to polygenic inheritance involving many common genetic variants with a small effect. An improved methodology for calculating polygenic scores, using a larger cohort of sequenced samples and advanced algorithms, has been proposed to identify a combined impact of single nucleotide polymorphisms (SNP) that significantly increase the risk of disease (15). Mendelian randomization (MR) is an analytical method for identification of causality using polygenic variables and it has been successfully implemented for diseases conferring both monogenic and polygenic traits (16).
Hence we compared the MHC markers and polygenic predictors of CVID patients without a molecular genetic diagnosis after whole exome sequencing (WES), where computational analysis based on high-resolution MHC typing from WES data was performed for the first time. Multi-SNP MR analyses using summary-level data from WES were performed to cross-validate the causality of currently identified variants and previously suggested SNPs for antibody deficiency.
Patients with a diagnosis of CVID based on the updated clinical diagnostic criteria of the European Society for Immunodeficiencies (ESID, https://esid.org/Working-Parties/Registry-Working-Party/Diagnosis-criteria) and the American Academy of Allergy, Asthma & Immunology (AAAAI) practice parameter for the diagnosis and management of PID (17), were recruited from a cohort of antibody deficiency patients evaluated by WES (2). This cohort was designed to investigate the contribution of genetic, immunologic, and clinical factors of the disease.
Among all registered CVID patients in the Iranian national PID registry (18, 19), available individuals who were referred to the Children's Medical Center (Pediatrics Center of Excellence affiliated to Tehran University of Medical Sciences, Tehran, Iran) and completed the molecular diagnostic investigation were consecutively recruited into this study. Written informed consent for the performed evaluations was obtained from all patients and/or their parents, according to the principles of the ethics committee of the Tehran University of Medical Sciences. An evaluation document was used to summarize the demographic information of the patients, including gender, date of birth, clinical parameters and previous medical history, family history, laboratory and molecular data. A computerized database program (new registry section at, http://rcid.tums.ac.ir/) was designed for the final data collection and direct generation for statistical analysis of data.
All clinically diagnosed CVID patients were re-evaluated for fulfilling either the probable or possible diagnostic criteria, and secondary causes of antibody deficiency were ruled out. Clinical phenotyping was performed using a standard method of phenotype subdivision which has been shown to correlate with the quality of life and morbidity among patients with infections only, autoimmunity, lymphoproliferation and enteropathy (20). Based on the epidemiologic data of CVID cohorts worldwide, there are two peak ages of onset, one before the age of ten and another in the third decade of life. Therefore, we defined the early onset as disease onset before age 10 years (20).
Complete blood count, lymphocyte subpopulations, serum Ig levels, and specific antibody responses were measured as previously described (2). Immunological tests were repeated for each patient every 6-months within during routine follow-up visits after the time of diagnosis to evaluate the progression of their antibody deficiency. Patients were classified immunologically based on the main classification for B-cell subsets known as B-cell pattern classification with relevance to genetic findings (21).
Regarding genetic diagnosis, WES was performed according to the protocol described previously (22). For analysis of WES, we followed a published pipeline for prioritizing candidate variants, predicting their effect on protein, homozygosity mapping, large deletion and copy number variation (CNV) detection (2, 22). To classify a patient as a monogenic disease, the pathogenicity of the disease attributable gene variant was re-evaluated using the updated guideline for interpretation of molecular sequencing by the American College of Medical Genetics and Genomics (ACMG), considering the allele frequency in the relevant population database, computational data, immunological/multiomics functional data, familial segregation, parental data and clinical phenotyping (23). In the remaining patients not only CVID associated genes, but also all known other PID genes were normal (2), therefore we labeled them as “without known genetic mutation detected or unsolved” even though there is a possibility of yet unknown inherited disorder in a minority of them.
The WES data were used for MHC typing of both monogenic and unsolved CVID patients using the major module of Optitype (24) [>97% accuracy (25)]. This algorithm was run according to instructions using fastq files after filtering low-quality reads (base quality of <20 for more than 80% of bases) as an input. In brief, the input data were mapped to the hg38 human reference assembly, and relevant MHC reads from the Binary Alignment Map (BAM) file (chromosome 6, position 29,886,751–33,090,696) were filtered according to their quality scores. Subsequently, four-digit typing, zygosity status and full-resolution imputation were computed. Multiple predictions for an allele at a locus detected in MHC reporter were considered as ambiguous results, and only the first field information was used (26). Confirmatory PCR amplicon-based high-resolution typing was performed on the genomic DNA of the patients as described previously (14, 27).
Exome-wide association study has previously been conducted on 535,486 SNPs extracted from high-throughput sequencing data of both monogenic and unsolved CVID patients as well as 141,456 individuals at Genome Aggregation Database (gnomAD) and 2,497 individuals at Greater Middle East (GME) Variome Project. All SNPs were mapped to build relevant coordinates using liftOver. Prior to imputation, we removed variants with a genotyping rate <98%, ambiguitious SNPs, evidence of deviation from Hardy-Weinberg equilibrium in controls (p < 1 × 10−4), and minor allele frequency < 1 × 10−6. We conducted χ2 tests of association on genotypes for each cohort separately, using only variants that overlapped between patients cohort and controls. We subsequently only included in the analysis the near-independent SNPs that do not account for linkage disequilibrium (LD) and were significantly different between monogenic and unsolved patients for ease of directly comparing the results.
MR analysis was performed using the identified significant genetic variants, in order to evaluate the effect of exclusion of monogenic patients for prediction of independent common variants without confounding factors, as instrumental variables (serum Ig level) to test for causality (bacterial infections). The result of the MR model on current predictor SNPs of unsolved CVID patients was empowered by comparison of multiple genetic variants reported on previously independent studies on antibody levels using the genome-wide association (GWAS) catalog provided by the National Human Genome Research Institute (NHGRI) and the European Bioinformatics Institute (EMBL-EBI, https://www.ebi.ac.uk/gwas/). Selection of GWAS catalogs on the infectious outcomes were performed to test the causality influenced by the exposures, including ICD10 codes of: J22 Unspecified acute lower respiratory infection (UKB-a:540, n = 337,199 individuals), A49.9 Bacterial infections of unspecified site (UKB-b:1605, n = 463,010), A49.8 Other bacterial infections of unspecified site (UKB-b:1399, n = 463,010), A49.0 Staphylococcal infection, unspecified (UKB-b:3266, n = 463,010), 0410 Streptococcus infection (UKB-b:4251, n = 463,010) and A49.1 Streptococcal infection, unspecified (UKB-b:4884, n = 463,010). Recruitment of GWAS catalogs were performed in the MR-base analytical platform established by the MRC Integrative Epidemiology Unit (University of Bristol, http://app.mrbase.org).
Statistical analysis was performed using SPSS (version 21.0.0, SPSS, Chicago, Illinois) and R statistical systems (version 3.4.1.; R Foundation for Statistical Computing, Vienna, Austria) software to compare clinical and immunological parameters between patients with an identified genetic defect and patients with no genetic diagnosis. The one-sample Kolmogorov-Smirnov test was applied to estimate whether data distribution was normal. Parametric and non-parametric analyses were performed based on the finding of this evaluation. Regarding MR, we used the proxy SNPs method instead of LD tagging with minimum LD values of 0.8 and minor allele frequency of (MAF) threshold of aligning palindromes as 0.3. Several MR methods with different sensitivities were applied including Wald ratio, MR Egger, weighted median, and inverse variance weighted algorithms. Forest plot and funnel plot were used to illustrate causality effects and horizontal pleiotropy, respectively. A P-value of <5 × 10−8 was considered for multiple testing and selection of significant SNPs and P-value <0.05 was assumed for comparisons of monogenic and unsolved CVID patients as statistically significant.
Among all genetically evaluated CVID patients, 83 patients agreed to participate in this study (Table 1) and monogenic defects were found and confirmed in 40 individuals (2). The remaining 43 “idiopathic” CVID patients were labeled as an unsolved patient. The studied patients (46 males, 37 females) from 71 unrelated kindreds were mainly children and adolescents at the time of the study (43 patients were <18 years old) and parental consanguinity was recorded in 64 patients. The median age of the patients at the onset of symptoms was 3 years (range 0.5–36 years; early-onset manifestation in 79.5%) and the median diagnostic delay (the gap between the onset of the symptoms and diagnosis of CVID) was 4 years (range 0.4–39 years). Of note, 11 patients were from multiplex families (classified as familial cases, 36% with an unsolved disease) and 7 cases progressed to CVID from another form of antibody deficiency during the course of the disease (IgA deficiency and IgG subclass deficiency, 14.2% with an unsolved disease). A summary of the clinical and immunologic phenotype of the studied patients is provided in Table 1. There was a significant difference among patients with or without monogenic disorders regarding the age of onset and progressive form of CVID, while parental consanguinity and familial cases were comparable.
We first investigated the frequency of MHC class I and II alleles in the CVID patients. Among the 83 patients, high-resolution WES-based typing of class I revealed the highest diversity in MHC-B with 84 unique alleles (mainly B*35, 31 alleles out of total 166 alleles:18.6%). However, the CVID cohort had a restricted MHC-H repertoire with only 4 unique alleles (mainly H*02, 113 alleles: 68.0%, Tables S1–S8). Evaluation of MHC class II showed that the most diverse locus was MHC-DPB1 with 40 unique alleles (mainly DPB1*463:01:01, 23 alleles: 13.8%), in contrast to three unique alleles for MHC-DRB4 (mainly DRB4*01:03:01, 135 alleles: 81.3%, Tables S9–S23). The most significant increases in the proportions of class I in the unsolved patient cohort were observed in B*39 (p = 0.02), B*50:01:01:01 (p = 0.02), and E*01:08N (p = 0.02, Table 2, Figures 1A,B). Moreover, susceptibility class II regions for unsolved CVID were most significantly associated with DQA1*01:04:01 (p < 0.001), DQB1*03:01:01 (p = 0.002), DPA1*01:03:01:04 (p = 0.002), and TAP1*01:01:01:01(p = 0.002, Table 3, Figures 1A,C). There were no significant differences in the frequency of alleles of MHC–H, –G –DRB3, and –DRB4 between monogenic and unsolved CVID patients (Tables S6, S7, S20, S21, Figure 1).
Table 2. Significantly different MHC-class I alleles associated with monogenic and unsolved CVID patients.
Figure 1. (A) Schematic presentation of association of MHC status in the pathogenesis of common variable immunodeficiency based on the role of MHC in antigen presentation within germinal center B cells. (B) MHC class I (C) and MHC class II risk and protective alleles for unsolved CVID compared to monogenic-CVID patients.
Table 3. Significantly different MHC-class II alleles associated with monogenic and non-monogenic CVID patients.
Regression model analysis using the identified significant MHC alleles suggested a combination of B*35, DMA*01:02, TAP1*06:01, MICB*002:01, DQA1*01:04:01, and DQB1*03:01:01 as the best fit model to predict an unsolved form of CVID (p = 4.5 × 10−6, Table S24). In the second model, we tested for an association with a significant haplotype in patients with unsolved CVID. In this model, the W*01:01:01-DMA*01:01:01-DMB*01:03:01:02-TAP1*01:01:01 (p < 0.001), was the most significant associated haplotype with an increased unsolved CVID odds (Table 4). This haplotype was exclusively identified in 11 patients without a genetic diagnosis. The majority of these patients had a late onset (n = 9, 81.8%) and none of them were of a familial CVID or progressive form of the disease. Infections only phenotype (n = 10, 90.9%) and post-germinal center defects (n = 8, 72.7%) were the main clinical and immunologic phenotypes in these patients.
Table 4. Distribution of MHC haplotypes among unsolved CVID patients vs. monogenic CVID patients (significant haplotypes with frequency ≥5% are shown).
Exome-wide significant results between monogenic and unsolved CVID patients and variants with the strongest association compared to healthy individuals were selected for MR analysis. The distribution of significant variants supported a polygenic etiology of unsolved CVID (Figure 2A). Correlation matrix and principal component analysis (PCA) of selected variants showed two distinct sets of SNPs, discriminating monogenic and unsolved CVID patients (Figures 2B,C). Subsequently, the selected variants were incorporated into a MR model considering them as directly linked to antibody deficiency (Figure 2D) and compared with previously suggested variants found in GWAS studies of antibody deficient patients without a genetic evaluation (Table S25). Table 5 and Table S26 summarize the MR estimates from each method of the causal effect of the exposures (variants of the current study and previous GWAS studies) on the susceptibility to infectious diseases as an outcome. The Wald ratio effects reported in the previous GWAS studies, neglecting the exclusion of monogenic diseases, showed only a significant negative correlation of IgG levels on streptococcal infection (p = 0.002) and IgA levels on bacterial infection (p = 0.04). Although our MR analysis approach also only provide a link between unsolved CVID with bacterial infections, all the estimated coefficients for other infectious diseases were directly associated with these SNPs, whereas several discrepancies were have been observed in MR analyses of previous GWAS studies (Table S26).
Figure 2. (A) Manhattan plot of exome-wide significant results of 535,486 SNPs between monogenic and unsolved CVID patients supporting a polygenic etiology of unsolved CVID where variants with a genotyping rate <98%, ambiguitious SNPs, evidence of deviation from Hardy-Weinberg equilibrium in controls (p < 1 × 10−4), and minor allele frequency < 1 × 10−6 were removed. Only the near-independent SNPs that do not account for linkage disequilibrium and were significantly different between monogenic and unsolved patients were included for the next step. (B) Principal component analysis (PCA) and (C) correlation matrix of significant variants discriminating monogenic and non-monogenic CVID patients. (D) MR model generated for selected variants were inputed to a considering them as direct linked with exposure (antibody deficiency) and outcome (infectious diseases). (E) Polygenic score of unsolved CVID disease was increased when the testing dataset had a lower percentage of MHC risk alleles.
Table 5. MR estimates from each method of the causal effect of the exposures (variants of current studies and GWAS studies) on the infectious diseases as outcome.
To evaluate the consistency of the causal estimate of all SNPs observed, the variability in the estimates obtained for each SNP was calculated and heterogeneity was only significant in association with IgA level and lower respiratory infections in previous GWAS studies (MR Egger, p = 0.0089 and inverse variance weighted, p = 0.016, Table S27) but none in our suggested model (Table S28). Forest plots showed that the causal effect of every single SNP in previously published GWAS studies had a non-unified prediction on outcome in line with formal MR estimates of heterogeneity as shown in Figures S1, S2. However, the symmetric influence of SNPs selected by the exclusion of monogenic CVID on the outcome was observed in our dataset for all infectious diseases evaluated, except unspecific bacterial infections (Figure 3). Asymmetry and larger spread of βIV in the Funnal plots also suggests a higher heterogeneity and presence of horizontal pleiotropy due to the absence of exclusion of monogenic disease in previous GWAS studies (Figures S3, S4), whereas a homogenous βIV value was observed for lower respiratory infection, bacterial infection, and Streptococcal infection in currently identified SNPs (Figure S5). Of note, the polygenic score of unsolved CVID disease was increased when the testing dataset had a lower percentage of MHC risk factor (Figure 2E).
Figure 3. Forest plots depicting causal effect of every single SNP in this cohort by exclusion of monogenic CVID patients suggesting a unified prediction on outcome for all infectious diseases including (A) lower respiratory infection, (B) staphylococcal infection, (C) bacterial infections, (E) Streptococcus infection, (F) Streptococcal infection, unspecific, except unspecific bacterial infections (D).
In this study, we demonstrated the importance of exclusion of monogenic CVID on the evaluation of the effects of MHC alleles and polygenic allele scores in MR analyses in the remaining patients. Since the late 1970s, when the association of MHC locus was initially determined as a principal genetic factor in antibody deficient patients (28, 29), the debate still is ongoing about the direct (the presentation of antigens by MHC) or indirect (association with the adjacent gene within the same region) role of these markers on the pathogenesis of these diseases. However, recently, several monogenic diseases originating from genes outside chromosome 6, the genomic location of MHC, have been discovered to underlie these disorders (2–4, 30–33). For the first time, we used this patient group as a control which may deconfound the effect of other genetic etiologies and may identify the difference in MHC markers between patients with and without monogenic defects. Moreover, using high-resolution MHC typing we also investigated both minor and major histocompatibility complex genes which have not been performed hitherto.
The distribution of major histocompatibility alleles in the current study, compared to our previous investigation on a mixed CVID patient population using low-throughput PCR-based molecular DNA typing, provides reproducible data on MHC class II (with proportional increase in DQB1*0201, DQA1*0103, and DRB1*15 alleles) (11), indicating the advantage of next-generation sequencing by generating data on a larger patients sample with a high resolution of the MHC typing. The findings of the current investigation also conferred that despite some observed significant differences between mixed CVID populations and healthy controls (DRB1*4, DRB1*11, DRB1*07), the design of previous studies has led to false positivity in six alleles and false negativity in 14 alleles associated with MHC class II of unsolved CVID (11). Therefore, subtracting the monogenic patients is an essential factor that should be considered when evaluating whether inheritance of a particular MHC haplotype is associated with CVID development.
In a study designed by Waldrep et al. (12) to test for the possibility of synergy (epistasis) between a mutant transmembrane activator and calcium-modulator and cyclophilin-ligand interactor (TACI) and genes located near the MHC class I locus, they stratified patients based on the variants identified in the TNFRSF13B gene. Although the strength of the study was not sufficient and only evaluated the polymorphic region of the TNFRSF13B gene (exons 3 and 4), their preliminary data support the hypothesis that the overall pattern of MHC alleles in individuals with a mutated TACI allele is different than in individuals with idiopathic CVID. This notion is consistent with the observation in our study by excluding all monogenic disorders underlying CVID and evaluation of both MHC class I and II alleles.
Most of the previous works on genetic susceptibility factors in CVID patients have only focused on MHC class II due to its known role in antibody class switching and affinity maturation. Using a limited number of CVID patients, we showed previously that MHC haplotypes, including DRB1*04-DQB1*03:01-DQA1*03:01 and DRB1*01:01-DQB1*03:01-DQA1*05:05 confer susceptibility to CVID, while DRB1*07-DQA1*02:01 constitutes a protective haplotype (11). A restricted diversity of MHC class II, in particular, MHC-DR, has also been reported previously in patients with a familial form of CVID with first degree relatives showing IgA deficiency (DRB1*03:01-DQB1*02:01 and DRB1*04) (34–36).
Both MHC class I and II markers in CVID patients could predict the clinical presentation and immunologic profile including enteropathy and autoimmunity (DQ*02:05 and DQ*8) (13), chronic inflammation (A*29) (37), severe infectious complication (A*11 and B*44) (38, 39), progressive disease (A*24, DQB1*03:01 and DQA1*05:01) (40, 41), and number of marginal zone-like B cells and switched memory B cells (B*8 and B*44) (42). However, MHC class I and other minor histocompatibility variants in this region have not been evaluated in most previous CVID studies. In a few reports, the effect of an increased proportion of A*24, B*14, A*02-B*40, A*02-B*044, A*03-B*07, A*01-B*08:01, and B*44-C*16, and a reduced proportion of B*62 and C*7 on the function of NK cells in CVID patients have been demonstrated (43–46). One previous study has also suggested that the CVID risk is increased in patients where Killer cell immunoglobulin-like receptors (KIR)/MHC class I combinations facilitate NK cell activation (B*44-KIR3DS1 and C*16-KIR2DL3) (46).
With a full resolution MHC typing, we identified a hitherto not recognized, novel MHC haplotype (W*01:01:01-DMA*01:01:01-DMB*01:03:01:02-TAP1*01:01:01), which is associated with unsolved CVID in patients with a late onset of symptoms, present a non-progressive form of the disease with an infections only phenotype and post-germinal center defects. Of note, the proportion of some specific MHC markers was also increased in the monogenic CVID patients which could suggest the deprivation of non-monogenic patients from those MHC alleles or it may be due to linkage of MHC markers with monogenic disorders. However, the latter is less likely since the 40 patients included in this study with the monogenic disease have 26 different genetic problems (2).
We also performed a simulation study on common genetic variants with significant differences between monogenic and unsolved CVID patients as an instrument in MR and compared them to previous GWAS studies. The data supports the notion that variants included in our model satisfy the assumptions of Ig production and bacterial infections as instrumental variables. Since the observed SNPs were consistent for prediction of infectious diseases, the cohort sample bias was not significant. Although the effect of sample size and usage of these unweighted allele scores may influence the predictivity and significance of the genetic model, where exclusion of monogenic forms of CVID unified the effect direction on all evaluated outcomes. Although imprecisely weighted allele scores would not bias our observed estimates, we suggest further cross-validation with other CVID cohorts before integration of weights in the MR data analysis to prevent reduction of power.
Since the number of SNPs used for instruments do not influence the effect size, we have included all significant markers discriminating between monogenic and unsolved CVIDs. It has been previously recommended that variants should be selected on the basis of scientific knowledge rather than statistical testing. However, in a majority of previous MR studies, all variants which can be reasonably assumed to be valid instruments have been considered during analysis to improve the precision of the causal estimate. However, we also performed a pathway analysis for instrumented SNPs representing their function in the immune system, particularly O-glycosylation of proteins and TNFR1-induced proapoptotic signaling, which are important for immunoglobulin production (Table S29).
We have also demonstrated that the use of multiple genetic variants in the context of MR has a significant impact on the strength of predictive MHC markers, with slight reductions in power of polygenic scores in CVID carriers of significant MHC markers. These findings support the notion of two separate mechanisms of MHC and polygenic variants in individuals with unsolved CVID. MHC haplotype is a genetic susceptibility factor for CVID which has been identified as a modifying factor for clinical presentation and immunologic phenotype. However, with current findings, we suggest MHC typing and polygenic evaluation of unsolved CVID patients should be integrated into the flowcharts of genetic screening (2). Moreover, with finding population-specific MHC and SNP predictors we can identify the at-risk asymptomatic individuals within the families of CVID patients and follow them up to improve the prognosis of the disease.
Considering the limitation of the current data of using multiple genetic variants, allele scores in particular, and missing data leading to reduced sample sizes for analysis, future studies in multiple variant setting in the whole genomic level and with higher samples size of monogenic and unsolved patients (from a similar population) could help imputation as it has been shown to be effective against any reduction in power due to missing data. The generalization of discovered MHC and SNP markers in unsolved patients should be performed with caution considering the genetic variations of the cohort population (mainly early-onset, higher rate of consanguinity and lower delay in diagnosis may be due to earlier and severe presentation), however, the methodology presented in the current study would be a commonly recommended approach after performing next-generation sequencing. Although familial cases were slightly higher in a group of patients with identified genetic defects, we cannot conclude at least from our data that multiple cases in a family suggest absolutely the monogenic form of CVID, the fact which is consistent with data from several Western cohorts of patients with accumulation of IgAD and CVID in a family without defining underlying genetic defect but similar MHC markers.
Although the exclusion of monogenic disorders in a newly clinically diagnosed CVID patient is a first mandatory step toward evaluation of other pathogenic mechanisms, detection of the causative genomic element is a challenging task in the MHC region in idiopathic patients due to its complexity and density of genes. Based on current data both MHC alleles and their adjacent genes are involved directly or indirectly in the etiology of some of unsolved CVID patients. Therefore, full sequencing of the MHC region in large populations of CVID patients is recommended. For patients with monogenic diseases, MHC typing may also unravel some markers for variation of the clinical presentation in patients with the same mutation or as a predictive marker for morbidity and mortality. However, to prove this we need a global effort to access a significant amount of patients with unique gene defects or better with a unique mutation within a specific gene. Moreover, the current findings indicate the probability of poly-genic etiology in idiopathic CVID patients. However, before extrapolating the polygenic scores observed in these patients, application of instrumental variable methods with genetic instruments to estimate the causal effect of reduction of immunoglobulin levels (rather than other defects in immune or non-immune system) on the infection diseases should be evaluated further with future observational data.
All datasets generated for this study are included in the article/Supplementary Material.
The studies involving human participants were reviewed and approved by the Karolinska Institutet and Tehran University of Medical Sciences. Written informed consent to participate in this study was provided by the participants and/or their legal guardian/next of kin.
HA designed the project, collected the clinical data, interpreted the analysis, and wrote the paper. CL performed the bioinformatic analysis and analyzed and interpreted the data. AA collected the clinical materials and followed up the patients. LH designed the project, analyzed and interpreted the data, and wrote the paper.
The authors declare that the research was conducted in the absence of any commercial or financial relationships that could be construed as a potential conflict of interest.
The handling editor declared a past co-authorship with the authors HA, LH, and CL.
We thank the families who participated in the study and made this research possible. This study was supported by the Swedish Research Council, the Jonas Söderquist scholarship, and the Ruth and Richard Julin Foundation.
The Supplementary Material for this article can be found online at: https://www.frontiersin.org/articles/10.3389/fimmu.2020.00014/full#supplementary-material
1. Park MA, Li JT, Hagan JB, Maddox DE, Abraham RS. Common variable immunodeficiency: a new look at an old disease. Lancet. (2008) 372:489–502. doi: 10.1016/S0140-6736(08)61199-X
2. Abolhassani H, Aghamohammadi A, Fang M, Rezaei N, Jiang C, Liu X, et al. Clinical implications of systematic phenotyping and exome sequencing in patients with primary antibody deficiency. Genet Med. (2019) 21:243–51. doi: 10.1038/s41436-018-0012-x
3. Bogaert DJ, Dullaers M, Lambrecht BN, Vermaelen KY, De Baere E, Haerynck F. Genes associated with common variable immunodeficiency: one diagnosis to rule them all? J Med Genet. (2016) 53:575–90. doi: 10.1136/jmedgenet-2015-103690
4. Stray-Pedersen A, Sorte HS, Samarakoon P, Gambin T, Chinn IK, Coban Akdemir ZH, et al. Primary immunodeficiency diseases: genomic approaches delineate heterogeneous Mendelian disorders. J Allergy Clin Immunol. (2017) 139:232–45. doi: 10.1016/j.jaci.2016.05.042
5. Jolles S. The variable in common variable immunodeficiency: a disease of complex phenotypes. J Allergy Clin Immunol Pract. (2013) 1:545–56; quiz 57. doi: 10.1016/j.jaip.2013.09.015
6. Abolhassani H, Parvaneh N, Rezaei N, Hammarström L, Aghamohammadi A. Genetic defects in B-cell development and their clinical consequences. J Invest Allergol Clin Immunol. (2014) 24:6–22; quiz 2 p following
7. Kienzler AK, Hargreaves CE, Patel SY. The role of genomics in common variable immunodeficiency disorders. Clin Exp Immunol. (2017) 188:326–32. doi: 10.1111/cei.12947
8. Mills DM, Cambier JC. B lymphocyte activation during cognate interactions with CD4+ T lymphocytes: molecular dynamics and immunologic consequences. Semin Immunol. (2003) 15:325–9. doi: 10.1016/j.smim.2003.09.004
9. Bai L, Deng S, Reboulet R, Mathew R, Teyton L, Savage PB, et al. Natural killer T (NKT)-B-cell interactions promote prolonged antibody responses and long-term memory to pneumococcal capsular polysaccharides. Proc Natl Acad Sci USA. (2013) 110:16097–102. doi: 10.1073/pnas.1303218110
10. Lang ML. How do natural killer T cells help B cells? Expert Rev Vaccines. (2009) 8:1109–21. doi: 10.1586/erv.09.56
11. Amanzadeh A, Amirzargar AA, Mohseni N, Arjang Z, Aghamohammadi A, Shokrgozar MA, et al. Association of HLA-DRB1, DQA1 and DQB1 alleles and haplotypes with common variable immunodeficiency in Iranian patients. Avicenna J Med Biotechnol. (2012) 4:103–12.
12. Waldrep ML, Zhuang Y, Schroeder HW Jr. Analysis of TACI mutations in CVID & RESPI patients who have inherited HLA B*44 or HLA*B8. BMC Med Genet. (2009) 10:100. doi: 10.1186/1471-2350-10-100
13. Venhoff N, Emmerich F, Neagu M, Salzer U, Koehn C, Driever S, et al. The role of HLA DQ2 and DQ8 in dissecting celiac-like disease in common variable immunodeficiency. J Clin Immunol. (2013) 33:909–16. doi: 10.1007/s10875-013-9892-3
14. Aghamohammadi A, Mohammadi J, Parvaneh N, Rezaei N, Moin M, Espanol T, et al. Progression of selective IgA deficiency to common variable immunodeficiency. Int Archiv Allergy Immunol. (2008) 147:87–92. doi: 10.1159/000135694
15. Torkamani A, Wineinger NE, Topol EJ. The personal and clinical utility of polygenic risk scores. Nat Rev Genet. (2018) 19:581–90. doi: 10.1038/s41576-018-0018-x
16. Emdin CA, Khera AV, Kathiresan S. Mendelian randomization. JAMA. (2017) 318:1925–6. doi: 10.1001/jama.2017.17219
17. Bonilla FA, Bernstein IL, Khan DA, Ballas ZK, Chinen J, Frank MM, et al. Practice parameter for the diagnosis and management of primary immunodeficiency. Ann Allergy Asthma Immunol. (2005) 94:S1–63. doi: 10.1016/S1081-1206(10)61142-8
18. Aghamohammadi A, Mohammadinejad P, Abolhassani H, Mirminachi B, Movahedi M, Gharagozlou M, et al. Primary immunodeficiency disorders in Iran: update and new insights from the third report of the national registry. J Clin Immunol. (2014) 34:478–90. doi: 10.1007/s10875-014-0001-z
19. Abolhassani H, Kiaee F, Tavakol M, Chavoshzadeh Z, Mahdaviani SA, Momen T, et al. Fourth update on the Iranian National Registry of primary immunodeficiencies: integration of molecular diagnosis. J Clin Immunol. (2018) 38:816–32. doi: 10.1007/s10875-018-0556-1
20. Chapel H, Cunningham-Rundles C. Update in understanding common variable immunodeficiency disorders (CVIDs) and the management of patients with these conditions. Br J Haematol. (2009) 145:709–27. doi: 10.1111/j.1365-2141.2009.07669.x
21. Driessen GJ, van Zelm MC, van Hagen PM, Hartwig NG, Trip M, Warris A, et al. B-cell replication history and somatic hypermutation status identify distinct pathophysiologic backgrounds in common variable immunodeficiency. Blood. (2011) 118:6814–23. doi: 10.1182/blood-2011-06-361881
22. Fang M, Abolhassani H, Lim CK, Zhang J, Hammarström L. Next generation sequencing data analysis in primary immunodeficiency disorders–future directions. J Clin Immunol. (2016) 36:68–75. doi: 10.1007/s10875-016-0260-y
23. Richards S, Aziz N, Bale S, Bick D, Das S, Gastier-Foster J, et al. Standards and guidelines for the interpretation of sequence variants: a joint consensus recommendation of the American College of Medical Genetics and Genomics and the Association for Molecular Pathology. Genet Med. (2015) 17:405–24. doi: 10.1038/gim.2015.30
24. Szolek A, Schubert B, Mohr C, Sturm M, Feldhahn M, Kohlbacher O. OptiType: precision HLA typing from next-generation sequencing data. Bioinformatics. (2014) 30:3310–6. doi: 10.1093/bioinformatics/btu548
25. Kiyotani K, Mai TH, Nakamura Y. Comparison of exome-based HLA class I genotyping tools: identification of platform-specific genotyping errors. J Hum Genet. (2017) 62:397–405. doi: 10.1038/jhg.2016.141
26. Xie C, Yeo ZX, Wong M, Piper J, Long T, Kirkness EF, et al. Fast and accurate HLA typing from short-read next-generation sequence data with xHLA. Proc Natl Acad Sci USA. (2017) 114:8059–64. doi: 10.1073/pnas.1707945114
27. Ferreira RC, Pan-Hammarström Q, Graham RR, Fontán G, Lee AT, Ortmann W, et al. High-density SNP mapping of the HLA region identifies multiple independent susceptibility loci associated with selective IgA deficiency. PLoS Genet. (2012) 8:e1002476. doi: 10.1371/journal.pgen.1002476
28. Volanakis JE, Zhu ZB, Schaffer FM, Macon KJ, Palermos J, Barger BO, et al. Major histocompatibility complex class III genes and susceptibility to immunoglobulin A deficiency and common variable immunodeficiency. J Clin Invest. (1992) 89:1914–22. doi: 10.1172/JCI115797
29. Van Thiel DH, Smith WI Jr, Rabin BS, Fisher SE, Lester R. A syndrome of immunoglobulin A deficiency, diabetes mellitus, malabsorption, a common HLA haplotype. Immunologic and genetic studies of forty-three family members. Ann Intern Med. (1977) 86:10–9. doi: 10.7326/0003-4819-86-1-10
30. Abolhassani H, Aghamohammadi A, Hammarström L. Monogenic mutations associated with IgA deficiency. Expert Rev Clin Immunol. (2016) 12:1321–35. doi: 10.1080/1744666X.2016.1198696
31. Maffucci P, Filion CA, Boisson B, Itan Y, Shang L, Casanova JL, et al. Genetic diagnosis using whole exome sequencing in common variable immunodeficiency. Front Immunol. (2016) 7:220. doi: 10.3389/fimmu.2016.00220
32. van Schouwenburg PA, Davenport EE, Kienzler AK, Marwah I, Wright B, Lucas M, et al. Application of whole genome and RNA sequencing to investigate the genomic landscape of common variable immunodeficiency disorders. Clin Immunol. (2015) 160:301–14. doi: 10.1016/j.clim.2015.05.020
33. de Valles-Ibáñez G, Esteve-Solé A, Piquer M, González-Navarro EA, Hernandez-Rodriguez J, Laayouni H, et al. Evaluating the genetics of common variable immunodeficiency: monogenetic model and beyond. Front Immunol. (2018) 9:636. doi: 10.3389/fimmu.2018.00636
34. Bonilla FA, Barlan I, Chapel H, Costa-Carvalho BT, Cunningham-Rundles C, de la Morena MT, et al. International Consensus Document (ICON): common variable immunodeficiency disorders. J Allergy Clin Immunol Pract. (2016) 4:38–59. doi: 10.1016/j.jaip.2015.07.025
35. Olerup O, Smith CI, Björkander J, Hammarström L. Shared HLA class II-associated genetic susceptibility and resistance, related to the HLA-DQB1 gene, in IgA deficiency and common variable immunodeficiency. Proc Natl Acad Sci USA. (1992) 89:10653–7. doi: 10.1073/pnas.89.22.10653
36. De La Concha EG, Fernandez-Arquero M, Martinez A, Vidal F, Vigil P, Conejero L, et al. HLA class II homozygosity confers susceptibility to common variable immunodeficiency (CVID). Clin Exp Immunol. (1999) 116:516–20. doi: 10.1046/j.1365-2249.1999.00926.x
37. Coussa RG, Antaki F, Lederer DE. HLA-A29 negative Birdshot-like chorioretinopathy associated with common variable immunodeficiency. Am J Ophthalmol Case Rep. (2018) 10:18–24. doi: 10.1016/j.ajoc.2018.01.024
38. Ginori A, Barone A, Bennett D, Butorano MA, Mastrogiulio MG, Fossi A, et al. Diffuse panbronchiolitis in a patient with common variable immunodeficiency: a casual association or a pathogenetic correlation? Diagn Pathol. (2014) 9:12. doi: 10.1186/1746-1596-9-12
39. Johnston DT, Mehaffey G, Thomas J, Young KR, Wiener H, Li J, et al. Increased frequency of HLA-B44 in recurrent sinopulmonary infections (RESPI). Clin Immunol. (2006) 119:346–50. doi: 10.1016/j.clim.2006.02.001
40. Cheraghi T, Aghamohammadi A, Mirminachi B, Keihanian T, Hedayat E, Abolhassani H, et al. Prediction of the evolution of common variable immunodeficiency: HLA typing for patients with selective IgA deficiency. J Invest Allergol Clin Immunol. (2014) 24:198–200.
41. Barton JC, Bertoli LF, Barton JC. Comparisons of CVID and IgGSD: referring physicians, autoimmune conditions, pneumovax reactivity, immunoglobulin levels, blood lymphocyte subsets, and HLA-A and -B typing in 432 adult index patients. J Immunol Res. (2014) 2014:542706. doi: 10.1155/2014/542706
42. Giannobile JV, Kapoor P, Brown EE, Schroeder HW Jr. CVID patients with HLAB8 or B44 have higher numbers of class switched memory B cell numbers than CVID patients without B8 or B44. J Allergy Clin Immunol. (2009) 123:S11. doi: 10.1016/j.jaci.2008.12.055
43. Barton JC, Bertoli LF, Acton RT. HLA-A and -B alleles and haplotypes in 240 index patients with common variable immunodeficiency and selective IgG subclass deficiency in central Alabama. BMC Med Genet. (2003) 4:3. doi: 10.1186/1471-2350-4-3
44. Aghamohammadi A, Farhoudi A, Hosseini Nik H, Khazali S, Pourpak Z, Khosravi F, et al. Human leukocyte class I and II antigens in Iranian patients with common variable immunodeficiency. Acta Med Iran. (2004) 42:272–6. Available online at: http://acta.tums.ac.ir/index.php/acta/article/view/2734
45. Kartal O, Musabak U, Yesillik S, Sagkan RI, Pekel A, Demirel F, et al. Killer-cell immunoglobulin-like receptor and human leukocyte antigen-C genes in common variable immunodeficiency. Wien Klin Wochenschr. (2016) 128:822–6. doi: 10.1007/s00508-015-0769-8
Keywords: primary immunodeficiency, antibody deficiency, common variable immunodeficiency (CVID), whole exome sequencing, full-resolution MHC typing, Mendelian randomization
Citation: Abolhassani H, Lim CK, Aghamohammadi A and Hammarström L (2020) Histocompatibility Complex Status and Mendelian Randomization Analysis in Unsolved Antibody Deficiency. Front. Immunol. 11:14. doi: 10.3389/fimmu.2020.00014
Received: 08 June 2019; Accepted: 06 January 2020;
Published: 24 January 2020.
Edited by:
Antonio Condino-Neto, University of São Paulo, BrazilReviewed by:
Tomohiro Morio, Tokyo Medical and Dental University, JapanCopyright © 2020 Abolhassani, Lim, Aghamohammadi and Hammarström. This is an open-access article distributed under the terms of the Creative Commons Attribution License (CC BY). The use, distribution or reproduction in other forums is permitted, provided the original author(s) and the copyright owner(s) are credited and that the original publication in this journal is cited, in accordance with accepted academic practice. No use, distribution or reproduction is permitted which does not comply with these terms.
*Correspondence: Lennart Hammarström, bGVubmFydC5oYW1tYXJzdHJvbUBraS5zZQ==
Disclaimer: All claims expressed in this article are solely those of the authors and do not necessarily represent those of their affiliated organizations, or those of the publisher, the editors and the reviewers. Any product that may be evaluated in this article or claim that may be made by its manufacturer is not guaranteed or endorsed by the publisher.
Research integrity at Frontiers
Learn more about the work of our research integrity team to safeguard the quality of each article we publish.