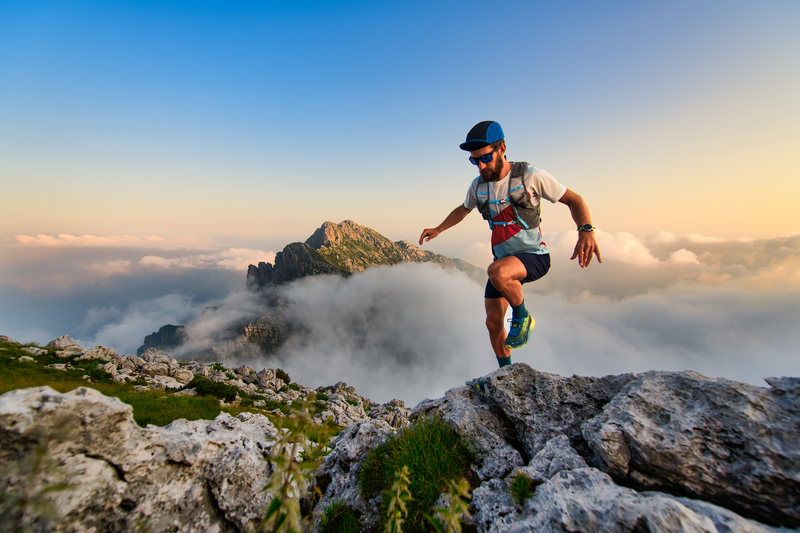
94% of researchers rate our articles as excellent or good
Learn more about the work of our research integrity team to safeguard the quality of each article we publish.
Find out more
MINI REVIEW article
Front. Immunol. , 10 July 2019
Sec. Autoimmune and Autoinflammatory Disorders
Volume 10 - 2019 | https://doi.org/10.3389/fimmu.2019.01562
This article is part of the Research Topic The Role of Extracellular Nuclear Molecules in the Pathogenesis of Autoimmune Disease View all 16 articles
Neutrophil extracellular traps (NETs) are a neutrophil defensive mechanism where chromatin is expelled together with antimicrobial proteins in response to a number of stimuli. Even though beneficial in many cases, their dysfunction has been implicated in many diseases, such as rheumatoid arthritis and cancer. Accurate quantification of NETs is of utmost importance for correctly studying their role in various diseases, especially when considering them as therapeutic targets. Unfortunately, NET quantification has a number of limitations. However, recent developments in computational methodologies for quantifying NETs have vastly improved the ability to study NETs. Methods range from using ImageJ to user friendly applications and to more sophisticated machine-learning approaches. These various methods are reviewed and discussed in this review.
Publications describing the formation of neutrophil extracellular traps (NET) have increased exponentially since their initial description in 2004 (1). Formed as a response of neutrophils to microorganisms and a host of other stimuli, NETs consist of decondensed chromatin released from the nucleus through the cytoplasm into the extracellular space (1). Nuclear and cytoplasmic components are mingled in the NETs and include antimicrobial peptides, such as myeloperoxidase (MPO), neutrophil elastase (NE) and, in certain instances, citrullinated histones (H3Cit) (1–3). NETs are believed to prevent dissemination and propagation of various pathogens (4–6). However, even though NETs might be beneficial, inappropriate function and tissue damage have been implicated in multiple pathologies i.e., pre-eclampsia (2, 7), diabetes and gestational diabetes (8–11), rheumatoid arthritis (RA) (3, 12, 13), systemic lupus erythematous (SLE) (14), community acquired pneumonia (15), sepsis (16), thrombosis (17), acute respiratory distress syndrome (18), and cancer (19, 20).
Clearly, it is evident that NETs are of considerable importance when studying innate immunity, understanding disease mechanisms or when using them as biomarkers or therapeutic targets. Thus, accurate, reproducible, high throughput and objective quantification is paramount for the study of NETs. Unfortunately, quantification is still plagued by a number of issues, such as sampling bias, insufficient objectivity, low throughput, being tedious, labor-intensive, high in cost and difficult to compare across laboratories (21–25). Luckily, recent advancements in technology allow for computational methodologies to circumvent a number of these disadvantages; being either semi or fully automated, with fully automated methods being more advantageous (25) i.e., higher in throughput, lower in cost, more sensitive and more reproducible across laboratories.
For this review we discuss the different methods for NET sample preparation followed by various computational solutions available for NET quantification. These solutions are only applicable for samples prepared for in vitro and in situ quantification of NETs. In vivo detection and quantification of NETs is important and it must be noted that quantification is usually done using in situ methods. NETs can also be detected and quantified in vivo by analysing serum or plasma for specific NET markers (12, 15, 26–29), however, since these do not involve computational methodologies for more automated quantification, they are not discussed in this review.
All available techniques used to visualize NETs for quantification have comprehensibly been reviewed by de Buhr and Köckritz-Blickwede (30). Table 1 provides a complete overview of these methods including their advantages and disadvantages. Methods include SYTOX/PicoGreen (fluorescence reader or fluorescence microscopy) (1, 31, 32, 40, 41), immunolabelling (immunofluorescence microscopy [IFM] (22, 31–39), microscopy imaging flow cytometry [MIFC] (21), flow cytometry [FACS] (42), and electron microscopy (SEM and TEM) (31, 43). The most widely published and accepted techniques are SYTOX and IFM (24, 30) and thus, are the easiest to implement and with the best quantitative computational methodologies available.
Table 1. Summary of the main NET visualization techniques used for quantification of NETs and their advantages or disadvantages.
SYTOX does not pass through intact cell membranes and detects NETs by staining extracellular DNA (51, 52). Its use has a number of advantages i.e., low cost and easy implementation. However, a major disadvantage is the susceptibility to false positives due to apoptosis or necrosis of neutrophils (24, 30, 53). Thus, quantification of NETs by SYTOX should always be supplemented with IFM i.e., specific labelling for NET markers, such as MPO and H3Cit (24, 30, 53). This is standard practice for in vitro detection of NETs and for most computational methodologies developed for these techniques.
FACS and MIFC (immunolabelling for MPO, NE, or H3Cit) also allow for robust, rapid, specific and sensitive detection of NETs in suspension (21, 30, 42). However, detection of neutrophils that have already undergone NETosis is not possible and thus cannot completely replace IFM (30). In addition, MIFC has an advantage over FACS since the technique combines FACS data as well as imaging for single cells (21, 30). Both FACS and MIFC are more challenging to implement compared to SYTOX and IFM based methods, because they are slightly more technical in nature.
As pointed out by de Buhr and Köckritz-Blickwede (30), an important consideration is the detection of NETs in in vivo tissue sections i.e., in situ detection. Since NETs are mainly quantified in vitro using neutrophils from peripheral blood, or ELISA based methods using serum (12, 15, 26–29), detection of NETs in localised tissue holds great importance, as was determined in placenta (7), intestine (1), kidney (27), lung (48), intracoronary material (49), and skin (50). It is possible in certain conditions that NETosis might be completely missed if not investigated in situ. Immunolabelling for NET specific markers on tissue sections is well-published and automated methods for their detection exist.
No automated methods for detection of NETs using SEM and TEM are available to our knowledge.
Table 2 compares the advantages and disadvantages of all the computational metholdogies discussed in this review for easy comparison.
Table 2. Advantages and disadvantages of the main computational methodologies available to quantify NETs in vitro and in situ.
Two methods for semi-automated quantification for NETs stained with SYTOX exist i.e., DNA Area NETosis Analysis (DANA) (23) and another using 3-dimensional confocal scanning laser microscopy (3D-CSLM) (46). DANA involves the use of a fluorescence microscope, ImageJ macros and a Java based programme with a batch processing option. Easy to follow YouTube tutorials for DANA also exist (45). Quantifying NETs by 3D-CSLM requires skilled confocal operators. No easy to follow protocols for quantification using ImageJ exist, which could make it more difficult to implement.
For 3D-CSLM, NETs are quantified based on SYTOX green area corrected to PKH26 area (binds to membranes indicating neutrophils). Using this approach, Kraaij et al. (46) successfully detected NETs in neutrophils exposed to RA and SLE serum using 3D-CSLM. Immune complexes produce lower and more subtle NETs (54) and 3D-CSLM together with ImageJ were successful in their quantification, making it a highly sensitive semi-automated technique (46). For DANA, NET-like structures are quantified on a per cell, per image and per sample basis. DANA can also sufficiently exclude overlapping cells and fragments, which might be recognized as false positives (23). These characteristics of DANA are not possible using 3D-CSLM and ImageJ. Rebernick et al. (23) were also successful in detecting spontaneous NETs in RA neutrophils using DANA.
Rebernick et al. (23) went further to show that DANA detected a similar amount of NETs compared between to two individual readers and reduced the time for analysis from 7–10 to 1.5 h. The authors were also able to detect NETs in DAPI stained murine cells, indicating robustness for the program.
Since only SYTOX is used, time required for pipetting is significantly reduced. However, in order to confirm results from the assay, IFM of specific NET markers is likely needed (24, 30, 53). Both methods do provide unintentional bias between sample quantification, and eliminate inter-individual variability. For DANA, reproducibility of results across laboratories is also likely achievable due to its robust nature. It must be noted that in our experience, DANA still requires a large amount of human input for optimization of the program and large datasets with many different individual donors can still be time-consuming to analyse.
A more fully automated and high-throughput way to quantify NETs involves quantification of extracellular DNA using SYTOX green in a plate assay. However, this technique is known for being susceptible to false positives (24, 30) since NETs are not quantified based on morphology, but rather RFU. Even though this method is considered to be unbiased, non-visualization of NETs and non-specific staining of DNA prevents differentiation of necrosis and NETosis, and blocking of staining can occur due to the presence of cationic peptides (30).
For NET quantification using IFM, one semi-automated method (37) and two fully automated methods exist (22, 25). For the semi-automated method, NETs are quantified based on morphological and spatial distribution using ImageJ (37). Fully automated methods for NET quantification include using a supervised machine-learning algorithm (regression model) trained on visually annotated images (22) or NETQUANT, a MATLAB application that quantifies NETs based on a number of criteria i.e., increases in cell surface area of single cells, deformation of DNA circularity, increase in DNA:NET bound protein ratio (25).
In our experience, NETQUANT is the most user friendly and easiest to implement with the user interface being extremely easy to use (25). The machine-learning method of Coelho et al. (22) is more technically challenging since knowledge in Python is required, even though a guide on GitHub exists (47). Furthermore, since the algorithm was trained using PMA stimulated neutrophils, new training would be required for new conditions to be investigated since NETs differ by stimuli (55), whereas for NETQUANT, metadata from images is used allowing the app to adapt to different conditions and thus be really robust. The semi-automated method requires multiple steps involving ImageJ, such as segmentation, thresholding, and particle analysis to quantify NETs, making it more difficult to implement compared to NETQUANT. These additional steps could also risk sampling bias or reduce reproducibility across laboratories.
Another advantage of NETQUANT is the inclusion of the watershed algorithm (56). This allows the app to differentiate NETs in contact with each other, a feature not available in other methods. Other methods would segment clumps of neutrophils or NETs as one and not individually. The batch processing option of NETQUANT also allows for image analysis of large datasets within minutes, providing detailed single-cell data and thus allowing for more advanced post-analysis of NET formation.
All methods were successful in NET detection in varying conditions, such as PMA stimulation, cytokine induction and even in the presence of pathogens. Coelho et al. (22) and Mohanty et al. (25) went one step further and showed that their methodologies correlated well to the detection of NETs comparing two individual experts.
Currently, NETQUANT appears to be the most unbiased and uses the most stringent, biologically relevant NET definition criteria that can be applied rapidly over many different datasets.
Apart from using the software provided for MIFC (IDEAS, considered to be semi-automatic, with batch processing possible) (21), only one fully automated methodology for NET quantification using MIFC data exists (48). The method developed by Ginley et al. (48) is a supervised machine learning algorithm for NET detection (chromatin staining only) using MIFC data. With a support vector machine (SVM), it provided a more well-rounded performance than an alternative convolutional neural network (CNN) approach. This was due to the amount of training data required. Since the algorithm only considered cells stimulated with PMA, additional training for different conditions would be necessary. Moreover, similar to Coelho et al. (22), the technical nature of the protocol can make it difficult to implement for persons lacking knowledge in informatics.
The same authors as above (48) used an unsupervised learning method on confocal images obtained from thin sections of lung tissue in a murine fungal pneumonia model stained for DNA, MPO and histone H1. The percent pixels of H1, present in decondensed nuclei colocalised with MPO, was the classification criteria. Applying deep CNN to this co-localisation data, a supervised approach can be applied. The pixel wise sensitivity/specificity was 0.99/0.98 for NET detection on 14 images using the unsupervised learning method. Their supervised CNN method uses object patches that had an object-wise holdout sensitivity/specificity of 0.86/0.90 on 631 object patches (from two images). This is the most automated method for NET detection in tissue sections. Unfortunately, as with other machine-learning methodologies, it can be challenging to implement.
Santos et al. (49) developed a semi-automated method for NET detection in paraffin-embedded intracoronary thrombus aspirate samples. Using confocal microscopy, NETs in the sections are detected by staining for DNA, MPO, and H3Cit. Thus, the method is highly specific and easier to implement than that of machine-learning algorithms proposed by Ginley et al. (48). Naturally, analysis is slightly more tedious and slower than the fully automated methods of Ginley et al. (48). A disadvantage is the requirement for specific analysis software i.e., SF SOFTWARE VERSION 2.6.07266 (LEICA). Since the method is largely based on co-localisation, development of methods using Imaris might provide more robust methods for cross-laboratory application.
NETs were also generated in vivo using a Mycobacterium tuberculosis guinea pig model and quantified in situ using semi-automated methods (50). Using ImageJ, the authors quantify NETs based on pixel density per area. Tissue sections were stained using Hoechst. Thus, NET quantification was based on an increase in the observed DNA area. As mentioned, this is not specific to NET formation which requires additional staining for NET markers, such as MPO, elastase etc. Thus, the authors went further to prove that the increase in DNA area is colocalised with certain NET markers. A more accurate method involving the quantification of NETs based on specific markers, such as MPO would prove to be more accurate i.e., that of Santos et al. (49).
Imaging of NETs can be a tedious task subject to sampling bias. Fortunately, a large number of groups are working towards high quality and easy to implement software packages that allow for high throughput and accurate quantification of NETs. This further will allow for reduction in sampling bias and allow for better reproducibility across laboratories.
SvB conceptualized and wrote the manuscript. LV prepared the table. CN assisted with references. SR, SH, and PH read and revised the manuscript.
SvB was 50% funded by a research grant from Celgene, USA.
The authors declare that this study received partial funding from Celgene, USA. The funder had no role in the design of the mini review, decision to publish or preparation of the manuscript.
The authors declare that the research was conducted in the absence of any commercial or financial relationships that could be construed as a potential conflict of interest.
1. Brinkmann V, Reichard U, Goosmann C, Fauler B, Uhlemann Y, Weiss DS, et al. Neutrophil extracellular traps kill bacteria. Science. (2004) 303:1532–5. doi: 10.1126/science.1092385
2. Gupta A, Hasler P, Gebhardt S, Holzgreve W, Hahn S. Occurrence of neutrophil extracellular DNA traps (NETs) in pre-eclampsia: a link with elevated levels of cell-free DNA? Ann N Y Acad Sci. (2006) 1075:118–22. doi: 10.1196/annals.1368.015
3. Khandpur R, Carmona-Rivera C, Vivekanandan-Giri A, Gizinski A, Yalavarthi S, Knight JS, et al. NETs are a source of citrullinated autoantigens and stimulate inflammatory responses in rheumatoid arthritis. Sci Transl Med. (2013) 5:178ra40. doi: 10.1126/scitranslmed.3005580
4. Delgado-Rizo V, Martinez-Guzman MA, Iniguez-Gutierrez L, Garcia-Orozco A, Alvarado-Navarro A, Fafutis-Morris M. Neutrophil extracellular traps and its implications in inflammation: an overview. Front Immunol. (2017) 8:81. doi: 10.3389/fimmu.2017.00081
5. Papayannopoulos V, Zychlinsky A. NETs: a new strategy for using old weapons. Trends Immunol. (2009) 30:513–21. doi: 10.1016/j.it.2009.07.011
6. Yang H, Biermann MH, Brauner JM, Liu Y, Zhao Y, Herrmann M. New insights into neutrophil extracellular traps: mechanisms of formation and role in inflammation. Front Immunol. (2016) 7:302. doi: 10.3389/fimmu.2016.00302
7. Gupta AK, Hasler P, Holzgreve W, Gebhardt S, Hahn S. Induction of neutrophil extracellular DNA lattices by placental microparticles and IL-8 and their presence in preeclampsia. Hum Immunol. (2005) 66:1146–54. doi: 10.1016/j.humimm.2005.11.003
8. Stoikou M, Grimolizzi F, Giaglis S, Schafer G, van Breda SV, Hoesli IM, et al. Gestational diabetes mellitus is associated with altered neutrophil activity. Front Immunol. (2017) 8:702. doi: 10.3389/fimmu.2017.00702
9. Carestia A, Frechtel G, Cerrone G, Linari MA, Gonzalez CD, Casais P, et al. NETosis before and after hyperglycemic control in type 2 diabetes mellitus patients. PLoS ONE. (2016) 11:e168647. doi: 10.1371/journal.pone.0168647
10. Menegazzo L, Ciciliot S, Poncina N, Mazzucato M, Persano M, Bonora B, et al. NETosis is induced by high glucose and associated with type 2 diabetes. Acta Diabetol. (2015) 52:497–503. doi: 10.1007/s00592-014-0676-x
11. Wong SL, Demers M, Martinod K, Gallant M, Wang YM, Goldfine AB, et al. Diabetes primes neutrophils to undergo NETosis, which impairs wound healing. Nat Med. (2015) 21:815–9. doi: 10.1038/nm.3887
12. Sur Chowdhury C, Giaglis S, Walker UA, Buser A, Hahn S, Hasler P. Enhanced neutrophil extracellular trap generation in rheumatoid arthritis: analysis of underlying signal transduction pathways and potential diagnostic utility. Arthritis Res Ther. (2014) 16:R122. doi: 10.1186/ar4579
13. Wang W, Peng W, Ning X. Increased levels of neutrophil extracellular trap remnants in the serum of patients with rheumatoid arthritis. Int J Rheum Dis. (2018) 21:415–21. doi: 10.1111/1756-185X.13226
14. Kaplan MJ, Radic M. Neutrophil extracellular traps: double-edged swords of innate immunity. J Immunol. (2012) 189:2689–95. doi: 10.4049/jimmunol.1201719
15. Ebrahimi F, Giaglis S, Hahn S, Blum CA, Baumgartner C, Kutz A, et al. Markers of neutrophil extracellular traps predict adverse outcome in community-acquired pneumonia: secondary analysis of a randomised controlled trial. Eur Respir J. (2018) 51:1701389. doi: 10.1183/13993003.01389-2017
16. Camicia G, Pozner R, de Larranaga G. Neutrophil extracellular traps in sepsis. Shock. (2014) 42:286–94. doi: 10.1097/SHK.0000000000000221
17. Fuchs TA, Brill A, Duerschmied D, Schatzberg D, Monestier M, Myers DD Jr, et al. Extracellular DNA traps promote thrombosis. Proc Natl Acad Sci USA. (2010) 107:15880–5. doi: 10.1073/pnas.1005743107
18. Porto BN, Stein RT. Neutrophil extracellular traps in pulmonary diseases: too much of a good thing? Front Immunol. (2016) 7:311. doi: 10.3389/fimmu.2016.00311
19. Cools-Lartigue J, Spicer J, Najmeh S, Ferri L. Neutrophil extracellular traps in cancer progression. Cell Mol Life Sci. (2014) 71:4179–94. doi: 10.1007/s00018-014-1683-3
20. Albrengues J, Shields MA, Ng D, Park CG, Ambrico A, Poindexter ME, et al. Neutrophil extracellular traps produced during inflammation awaken dormant cancer cells in mice. Science. (2018) 361:eaao4227. doi: 10.1126/science.aao4227
21. Zhao W, Fogg DK, Kaplan MJ. A novel image-based quantitative method for the characterization of NETosis. J Immunol Methods. (2015) 423:104–10. doi: 10.1016/j.jim.2015.04.027
22. Coelho LP, Pato C, Friaes A, Neumann A, von Kockritz-Blickwede M, Ramirez M, et al. Automatic determination of NET (neutrophil extracellular traps) coverage in fluorescent microscopy images. Bioinformatics. (2015) 31:2364–70. doi: 10.1093/bioinformatics/btv156
23. Rebernick R, Fahmy L, Glover C, Bawadekar M, Shim D, Holmes CL, et al. DNA Area and NETosis Analysis (DANA): a high-throughput method to quantify neutrophil extracellular traps in fluorescent microscope images. Biol Proced Online. (2018) 20:7. doi: 10.1186/s12575-018-0072-y
24. Carmona-Rivera C, Kaplan MJ. Induction and quantification of NETosis. Curr Protoc Immunol. (2016) 115:14.41.1–14. doi: 10.1002/cpim.16
25. Mohanty T, Sorensen OE, Nordenfelt P. NETQUANT: automated quantification of neutrophil extracellular traps. Front Immunol. (2017) 8:1999. doi: 10.3389/fimmu.2017.01999
26. Giaglis S, Stoikou M, Sur Chowdhury C, Schaefer G, Grimolizzi F, Rossi SW, et al. Multimodal regulation of NET formation in pregnancy: progesterone antagonizes the Pro-NETotic effect of estrogen and G-CSF. Front Immunol. (2016) 7:565. doi: 10.3389/fimmu.2016.00565
27. Kessenbrock K, Krumbholz M, Schonermarck U, Back W, Gross WL, Werb Z, et al. Netting neutrophils in autoimmune small-vessel vasculitis. Nat Med. (2009) 15:623–5. doi: 10.1038/nm.1959
28. Thalin C, Daleskog M, Goransson SP, Schatzberg D, Lasselin J, Laska AC, et al. Validation of an enzyme-linked immunosorbent assay for the quantification of citrullinated histone H3 as a marker for neutrophil extracellular traps in human plasma. Immunol Res. (2017) 65:706–12. doi: 10.1007/s12026-017-8905-3
29. Perez-Sanchez C, Ruiz-Limon P, Aguirre MA, Jimenez-Gomez Y, Arias-de la Rosa I, Abalos-Aguilera MC, et al. Diagnostic potential of NETosis-derived products for disease activity, atherosclerosis and therapeutic effectiveness in rheumatoid arthritis patients. J Autoimmun. (2017) 82:31–40. doi: 10.1016/j.jaut.2017.04.007
30. de Buhr N, von Kockritz-Blickwede M. How neutrophil extracellular traps become visible. J Immunol Res. (2016) 2016:4604713. doi: 10.1155/2016/4604713
31. von Kockritz-Blickwede M, Chow O, Ghochani M, Nizet V. Visualization and functional evaluation of phagocyte extracellular traps. Method Microbiol. (2010) 37:139–60. doi: 10.1016/S0580-9517(10)37007-3
32. Neumann A, Vollger L, Berends ET, Molhoek EM, Stapels DA, Midon M, et al. Novel role of the antimicrobial peptide LL-37 in the protection of neutrophil extracellular traps against degradation by bacterial nucleases. J Innate Immun. (2014) 6:860–8. doi: 10.1159/000363699
33. Saitoh T, Komano J, Saitoh Y, Misawa T, Takahama M, Kozaki T, et al. Neutrophil extracellular traps mediate a host defense response to human immunodeficiency virus-1. Cell Host Microbe. (2012) 12:109–16. doi: 10.1016/j.chom.2012.05.015
34. Narasaraju T, Yang E, Samy RP, Ng HH, Poh WP, Liew AA, et al. Excessive neutrophils and neutrophil extracellular traps contribute to acute lung injury of influenza pneumonitis. Am J Pathol. (2011) 179:199–210. doi: 10.1016/j.ajpath.2011.03.013
35. Urban CF, Reichard U, Brinkmann V, Zychlinsky A. Neutrophil extracellular traps capture and kill Candida albicans yeast and hyphal forms. Cell Microbiol. (2006) 8:668–76. doi: 10.1111/j.1462-5822.2005.00659.x
36. Fuchs TA, Abed U, Goosmann C, Hurwitz R, Schulze I, Wahn V, et al. Novel cell death program leads to neutrophil extracellular traps. J Cell Biol. (2007) 176:231–41. doi: 10.1083/jcb.200606027
37. Brinkmann V, Goosmann C, Kuhn LI, Zychlinsky A. Automatic quantification of in vitro NET formation. Front Immunol. (2012) 3:413. doi: 10.3389/fimmu.2012.00413
38. de Buhr N, Stehr M, Neumann A, Naim HY, Valentin-Weigand P, von Kockritz-Blickwede M, et al. Identification of a novel DNase of Streptococcus suis (EndAsuis) important for neutrophil extracellular trap degradation during exponential growth. Microbiology. (2015) 161(Pt 4):838–50. doi: 10.1099/mic.0.000040
39. de Buhr N, Neumann A, Jerjomiceva N, von Kockritz-Blickwede M, Baums CG. Streptococcus suis DNase SsnA contributes to degradation of neutrophil extracellular traps (NETs) and evasion of NET-mediated antimicrobial activity. Microbiology. (2014) 160(Pt 2):385–95. doi: 10.1099/mic.0.072199-0
40. Seper A, Hosseinzadeh A, Gorkiewicz G, Lichtenegger S, Roier S, Leitner DR, et al. Vibrio cholerae evades neutrophil extracellular traps by the activity of two extracellular nucleases. PLoS Pathog. (2013) 9:e1003614. doi: 10.1371/journal.ppat.1003614
41. Pilsczek FH, Salina D, Poon KK, Fahey C, Yipp BG, Sibley CD, et al. A novel mechanism of rapid nuclear neutrophil extracellular trap formation in response to Staphylococcus aureus. J Immunol. (2010) 185:7413–25. doi: 10.4049/jimmunol.1000675
42. Gavillet M, Martinod K, Renella R, Harris C, Shapiro NI, Wagner DD, et al. Flow cytometric assay for direct quantification of neutrophil extracellular traps in blood samples. Am J Hematol. (2015) 90:1155–8. doi: 10.1002/ajh.24185
43. Krautgartner WD, Vitkov L. Visualization of neutrophil extracellular traps in TEM. Micron. (2008) 39:367–72. doi: 10.1016/j.micron.2007.03.007
44. Onouchi T, Shiogama K, Matsui T, Mizutani Y, Sakurai K, Inada K, et al. Visualization of neutrophil extracellular traps and fibrin meshwork in human fibrinopurulent inflammatory lesions: II. Ultrastructural study. Acta Histochem Cytochem. (2016) 49:117–23. doi: 10.1267/ahc.16016
45. Shelef MA. DANA Software Request. Available online at http://www.medicine.wisc.edu/rheumatology/shelef-dana-software-request (accessed February 5, 2018).
46. Kraaij T, Tengstrom FC, Kamerling SW, Pusey CD, Scherer HU, Toes RE, et al. A novel method for high-throughput detection and quantification of neutrophil extracellular traps reveals ROS-independent NET release with immune complexes. Autoimmun Rev. (2016) 15:577–84. doi: 10.1016/j.autrev.2016.02.018
47. Coelho LP. Determination of NET Content. Available online at: https://github.com/luispedro/Coelho2015_NetsDetermination (accessed February 5, 2018).
48. Ginley BG, Emmons T, Lutnick B, Urban CF, Segal BH, Sarder P. Computational detection and quantification of human and mouse neutrophil extracellular traps in flow cytometry and confocal microscopy. Sci Rep. (2017) 7:17755. doi: 10.1038/s41598-017-18099-y
49. Santos A, Martin P, Blasco A, Solano J, Cozar B, Garcia D, et al. NETs detection and quantification in paraffin embedded samples using confocal microscopy. Micron. (2018) 114:1–7. doi: 10.1016/j.micron.2018.07.002
50. Filio-Rodriguez G, Estrada-Garcia I, Arce-Paredes P, Moreno-Altamirano MM, Islas-Trujillo S, Ponce-Regalado MD, et al. In vivo induction of neutrophil extracellular traps by Mycobacterium tuberculosis in a guinea pig model. Innate Immun. (2017) 23:625–37. doi: 10.1177/1753425917732406
51. Metzler KD, Fuchs TA, Nauseef WM, Reumaux D, Roesler J, Schulze I, et al. Myeloperoxidase is required for neutrophil extracellular trap formation: implications for innate immunity. Blood. (2011) 117:953–9. doi: 10.1182/blood-2010-06-290171
52. Papayannopoulos V, Metzler KD, Hakkim A, Zychlinsky A. Neutrophil elastase and myeloperoxidase regulate the formation of neutrophil extracellular traps. J Cell Biol. (2010) 191:677–91. doi: 10.1083/jcb.201006052
53. Gonzalez AS, Bardoel BW, Harbort CJ, Zychlinsky A. Induction and quantification of neutrophil extracellular traps. Methods Mol Biol. (2014) 1124:307–18. doi: 10.1007/978-1-62703-845-4_20
54. Behnen M, Leschczyk C, Moller S, Batel T, Klinger M, Solbach W, et al. Immobilized immune complexes induce neutrophil extracellular trap release by human neutrophil granulocytes via Fc gamma RIIIB and Mac-1. J Immunol. (2014) 193:1954–65. doi: 10.4049/jimmunol.1400478
55. Kenny EF, Herzig A, Kruger R, Muth A, Mondal S, Thompson PR, et al. Diverse stimuli engage different neutrophil extracellular trap pathways. Elife. (2017) 6:e24437. doi: 10.7554/eLife.24437
Keywords: neutrophil extracellular traps, myeloperoxidase, neutrophil elastase, citrullinated histone, machine-learning
Citation: van Breda SV, Vokalova L, Neugebauer C, Rossi SW, Hahn S and Hasler P (2019) Computational Methodologies for the in vitro and in situ Quantification of Neutrophil Extracellular Traps. Front. Immunol. 10:1562. doi: 10.3389/fimmu.2019.01562
Received: 14 December 2018; Accepted: 24 June 2019;
Published: 10 July 2019.
Edited by:
Edit I. Buzás, Semmelweis University, HungaryReviewed by:
Samer Tohme, University of Pittsburgh, United StatesCopyright © 2019 van Breda, Vokalova, Neugebauer, Rossi, Hahn and Hasler. This is an open-access article distributed under the terms of the Creative Commons Attribution License (CC BY). The use, distribution or reproduction in other forums is permitted, provided the original author(s) and the copyright owner(s) are credited and that the original publication in this journal is cited, in accordance with accepted academic practice. No use, distribution or reproduction is permitted which does not comply with these terms.
*Correspondence: Shane V. van Breda, c2hhbmV2b250ZWxpbi52YW5icmVkYUB1bmliYXMuY2g=
Disclaimer: All claims expressed in this article are solely those of the authors and do not necessarily represent those of their affiliated organizations, or those of the publisher, the editors and the reviewers. Any product that may be evaluated in this article or claim that may be made by its manufacturer is not guaranteed or endorsed by the publisher.
Research integrity at Frontiers
Learn more about the work of our research integrity team to safeguard the quality of each article we publish.