- 1Division of Veterinary Biotechnology, ICAR-Indian Veterinary Research Institute (IVRI), Bareilly, India
- 2Division of Pharmaceutics and Pharmaceutical Chemistry, The Ohio State University, Columbus, OH, United States
- 3Genomics and Computational Biology, DBT-National Institute of Animal Biotechnology, Hyderabad, India
- 4Division of Virology, ICAR-Indian Veterinary Research Institute (IVRI), Mukteswar, India
- 5Division of Animal Genetics and Breeding, ICAR-Indian Veterinary Research Institute (IVRI), Bareilly, India
- 6Division of Biological Products, ICAR-Indian Veterinary Research Institute, Bareilly, India
- 7Division of Biological Standardization, ICAR-Indian Veterinary Research Institute, Bareilly, India
- 8ICAR- Directorate of Foot and Mouth Disease, Mukteswar, India
- 9ARIS Cell, ICAR-Indian Veterinary Research Institute (IVRI), Bareilly, India
In this study, transcriptome analysis of PPRV infected PBMC subsets—T helper cells, T cytotoxic cells, monocytes, and B lymphocytes was done to delineate their role in host response. PPRV was found to infect lymphocytes and not monocytes. The established receptor for PPRV—SLAM was found downregulated in lymphocytes and non-differentially expressed in monocytes. A profound deviation in the global gene expression profile with a large number of unique upregulated genes (851) and downregulated genes (605) was observed in monocytes in comparison to lymphocytes. ISGs—ISG15, Mx1, Mx2, RSAD2, IFIT3, and IFIT5 that play a role in antiviral response and the genes for viral sensors—MDA5, LGP2, and RIG1, were found to be upregulated in lymphocytes and downregulated in monocytes. The transcription factors—IRF-7 and STAT-1 that regulate expression of most of the ISGs were found activated in lymphocytes and not in monocytes. Interferon signaling pathway and RIG1 like receptor signaling pathway were found activated in lymphocytes and not in monocytes. This contrast in gene expression profiles and signaling pathways indicated the predominant role of lymphocytes in generating the antiviral response against PPRV in goats, thus, giving us new insights into host response to PPRV.
Introduction
Peste des petits ruminants (PPR) is an acute, highly contagious viral disease with high mortality (90%) and morbidity (100%) in sheep and goats and is classified as an OIE listed disease due to its economic relevance and severity (1). It is characterized by high fever, mucous membrane erosions, discharge through eyes and nose, enteritis and pneumonia, culminating in a fatal outcome (2). The causative agent is single-stranded, negative-sense RNA virus of genus Morbillivirus and family Paramyxoviridae. An investigation into the molecular determinants of the pathogenesis of PPRV is of considerable interest to understand the host-virus interaction and to devise strategies for its eradication.
PPR virus (PPRV) is a lymphotropic and epitheliotropic cell-associated virus, which uses peripheral blood mononuclear cell (PBMCs) as a vehicle for its dissemination within the host (3, 4). SLAM/CD150 established as a receptor for PPRV, is found on activated T cells, B cells, thymocytes and dendritic cells (5). Morbilliviruses infect both T and B lymphocytes (6, 7). PBMCs play a significant role in pathogen recognition and initiation of early innate immune response. This makes them a standard model for studying host-pathogen interactions in PPRV and other related infections (8–11). We have previously identified various immune modulating transcription factors and predicted an immune signaling pathway in response to Sungri/96 live attenuated PPRV vaccine strain in vitro in PBMCs. Our group has also deciphered miRNAome of lung and spleen of PPRV infected sheep and goats, identified and validated suitable reference genes for expression studies in PPRV infected tissues and revealed the role of miRNAome of PBMCs in regulating immune processes in PPRV infected goat (12–15).
The host immune response is often complicated due to differences in how different cell types receive a signal from different classes of receptors and produce distinct effector molecules (16). The individual role of PBMC subsets in terms of their contribution to immune response against Zaire ebolavirus and inactivated seasonal influenza virus infection has been explored through RNA-Sequencing analysis (17, 18). However, RNA-Sequencing of PPRV infected PBMC subsets has not been explored to date. Transcriptome analysis of the subpopulation of highly enriched circulating leukocytes and analysis of cell-specific pathways will help in understanding leukocyte regulatory networks in PPRV infection. In this study, subsets of peripheral blood leukocytes were isolated from control (0 day) and PPRV infected (9 dpi) goats through MACS technology and subjected to RNA-Sequencing. Subsequently, transcriptome analysis combined with system-level network analyses revealed the contrast between monocytes and lymphocytes with respect to expression of genes, signaling pathways and putative upstream regulators.
Materials and Methods
Animal Experiment, Ethics Statement, and Viral Infection
Highly virulent PPRV (Izatnagar/94, accession number KR140086.1) that is being maintained at National Morbillivirus Referral Laboratory, Indian Veterinary Research Institute, Muktehswar by animal-to-animal passages (19, 20) was used in the present study. The permission to conduct the study was granted by Indian Veterinary Research Institute Animal Ethics Committee (IVRI-IAEC) under the Committee for the Purpose of Control and Supervision of Experiments on Animals (CPCSEA), India, vide letter no 387/CPSCEA. Healthy goats (n = 4) that were negative for PPRV antibody by competitive-ELISA (21) and by serum neutralization test (SNT) (22), and for PPRV antigen by s-ELISA, were inoculated with the virus as mentioned in our earlier study (23). s-ELISA for detection of virus in nasal, ocular, buccal, and rectal swabs, PPRV N gene expression by qRT-PCR in PBMC subsets, histopathology, and immunohistochemistry of tissues were used for confirmation of infection.
Isolation of T Helper Cells, T Cytotoxic Cells, B Lymphocytes, and Monocytes
Blood was collected from goats (n = 4) in heparin-coated vacutainer vials. PBMCs were isolated by using Ficol histopaque gradient method. PBMCs were strained through cell strainer of 0.40 micron. The PBMCs subsets were enriched by positive selection using indirect MACS technology (Milteny Biotech). Initially, the cell-specific surface marker FITC-conjugated primary antibodies, anti CD4+ (T helper cells, #MCA2213F), anti CD8+ (T cytotoxic cells, #MCA2216F), anti CD14+ (Monocytes, #MCA1568F), and anti CD21+ (B lymphocytes, #MCA1195F), were used. Subsequently, the cells were magnetically labeled with anti—FITC MicroBeads. Then the cell suspension was loaded on a miniMACS® column which was placed in the magnetic field of a MACS Separator. The magnetically labeled cells were retained in the column while the unlabeled cells run through. After removal of the column from the magnetic field, the magnetically retained cells were eluted as positively selected cell fraction. Cell sorting was done as per the manufacturer's protocol. Primary FITC-conjugated antibodies were titrated to determine the optimal dilution. Cells were kept on the ice and cold buffers were employed to minimize alterations in gene expression during labeling and sorting. The purity of the cells was further checked by flow cytometer. The cells were stored in RNA later for further use at −80°C.
RNA-Sequencing of the Samples
The RNA-sequencing of each subset of PBMCs was carried out following the standard procedure as described in our previous study (20). Briefly, total RNA was isolated using the RNeasy Mini kit (Qiagen GmbH, Germany). Following the quality and integrity assessment on Bioanalyzer (Agilent Technologies, Inc), RNA (100 ng) was used for library preparation with the help of NEBNext Ultra RNA Library Prep Kit for Illumina (NewEngland Biolabs Inc.). The quality of the libraries was checked on Bioanalyzer and quantity was measured using a Qubit 2.0 Fluorometer (Life Technologies) and by qPCR. Library (1.3 ml, 1.8 pM) was denatured, diluted, and loaded onto a flow cell for sequencing. FastQC (Babraham Bioinformatics) was used for quality assessment of raw sequence data and prinseq-lite software (24) was used to remove reads of low quality (mean phred score 25) and short length (<50) for downstream analysis.
Identification of Differentially Expressed Genes (DEGs)
The overview of the transcriptome analysis is given in Figure 1. Initially, the quality-filtered reads from control and infected samples (0 days and 9 dpi) were mapped to Capra hircus genome using Bowtie2 (25). The mapped reads were then assembled using RNA-Seq by expectation maximization (RSEM) (26). The counts were used for calculating DEGs by use of R packages—EBSeq, DESeq2 and edgeR. The common DEGs from the three packages were used for downstream analysis while as fold changes for the corresponding genes was taken from DESeq2.
Functional Analysis of DEGs
Using g:Profiler, the significantly expressed (p ≤ 0.05) DEGs were functionally annotated (27). The retrieved viral processes and immune system processes were chosen to find the genes associated with these processes. Further, the genes involved in immune-related functions were manually classified into antiviral interferon-stimulated genes (ISGs), chemokines, interleukins, viral sensors (PRRs), Interferons and Granzyme B, based on literature evidence and the type of associated immune process, to get comprehensive insights. Besides, 106 genes (= knowledge-based genes) were selected based on their role in host response against viruses from the earlier host-PPR virus interaction studies (8, 12) to explore variation in their expression across the PBMC subsets.
Predicted Protein-Protein Interaction(PPI) Network
The Biological General Repository for Interaction Datasets (BioGRID) is a repository of protein-protein and genetic interactions for many species. Based on the interactions available in the BioGRID database, PPI network among the selected genes is retrieved (28). Initially, the DEGs obtained from transcriptome analysis were narrowed down to differentially expressed highly connected (DEHC) genes based on immune-related functions (from g:Profiler), fold change ≥±1.5 (Up or downregulated) and degree (calculated using igraph package) ≥5 for T helper cells, T cytotoxic cells, and B lymphocytes and degree ≥10 for monocytes. The PPI networks were constructed between the DEHC-DEHC genes, and between the knowledge-based genes and DEGs. The BioGRID database contains well-defined protein-protein interactions of human than in the case of Bos taurus. Considering protein interactions are conserved across species (29), orthologs in human were queried using g: Orth in g:profiler (27). The interactions involving the DEGs were extracted using customized perl scripts and were visualized in Cytoscape 3.3.0 (30).
Identification of Viral Transcripts
The unmapped reads from all samples (control and infected) were mapped to PPRV reference genome (GenBank: AJ849636.2) (31) through Bowtie 2.0 for identification of viral transcripts.
Ingenuity Pathway Analysis (IPA) Analysis
QIAGEN's IPA (QIAGEN, Redwood City, USA) was used to analyze the data of T helper cells, T cytotoxic cells, B lymphocytes, and monocytes. IPA has got its own database, Ingenuity Pathways Knowledge Base (IKB) that along with the list of DEGs were used to identify the canonical pathways and the most significant biological processes. Core analysis for each dataset was performed to know activated (Z score > 2) or inactivated (Z score < −2) canonical pathways. Also, upstream regulators (transcription factors, cytokines, and other molecules) were identified.
Validation of DEGs by Quantitative Real-Time PCR (qRT-PCR)
To validate the expression of some selected genes, qRT-PCR was performed on Applied Biosystems 7500 Fast system. GAPDH was taken as the internal control as it was found to be the best suitable endogenous control in earlier studies in PPRV (12). The probe ID of selected genes used in the study for validation is given in Supplementary Table 1. Each of the samples was run in triplicates and relative expression of each gene was calculated using the 2−ΔΔCT method with control as the calibrator (32). Student's t-test was done in JMP9 (SAS Institute Inc., Cary, USA) to test the significance of difference. Differences between groups were considered significant at P ≤ 0.05.
Results
To uncover the transcriptional variation underlying the host response to PPRV at PBMCs subtype level, enriched T helper cells, T cytotoxic cells, B lymphocytes, and monocytes were obtained by using MACS technology. The purity was found to be >90% through flow cytometry (data not shown). Then, we performed the global transcriptome profiling of T helper cells, T cytotoxic cells, and B lymphocytes and monocytes at 0 day (control) and 9 dpi.
Viral Quantification by RNA-Sequencing
Following alignment with Bowtie 2, several reads in the infected T helper cells, T cytotoxic cells, and B lymphocytes mapped to PPRV reference genome but no reads from infected monocyte mapped to PPRV genome (Figure 2A). PPRV N gene expression as confirmed by qRT-PCR was found in infected T helper cells, T cytotoxic cells, and B lymphocytes, but not in infected monocytes (Figure 2B).
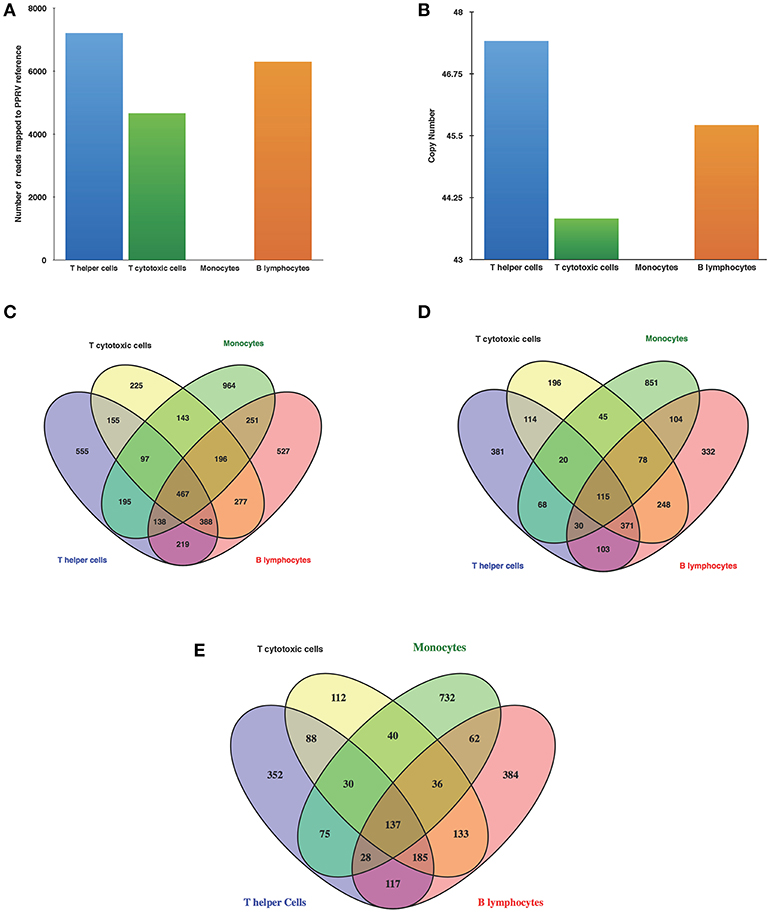
Figure 2. PPR virulent virus tropism in PBMCs subsets (A) Detection of viral reads through RNA Seq (B) N gene expression by q-RT-PCR of infected goats at 9 dpi. Venn diagrams representing unique/common (C) DEGs among cells (D) Upregulated genes among cells (E) Downregulated genes among cells.
Identification of DEGs—Transcriptome Analysis of Isolated Cell Subpopulations Divulges Unique Gene Expression Patterns
The number of DEGs in T helper cells were 2,214 (1,202 upregulated, 1,012 downregulated), T cytotoxic cells−1,948 (1,187 upregulated, 761 downregulated), monocytes−2,451 (1,311 upregulated, 1,140 downregulated), and B lymphocytes−2,463 (1,381 upregulated, 1,082 downregulated). The number of upregulated genes was larger than the numbers of downregulated genes in all the cells. Furthermore, Venn diagrams were generated to examine the overlapping mRNA profiles between cells. This revealed 467 genes being common all subsets and 555, 225, 964, and 527 genes being unique in T helper cells, T cytotoxic cells, monocytes, and B lymphocytes, respectively (Figure 2C). Upon comparison of upregulated and downregulated genes 115 and 137 genes were common, respectively. The number of unique upregulated genes were 381, 196, 851, and 332 in T helper cells, T cytotoxic cells, monocytes, and B lymphocytes, respectively (Figure 2D) whereas number of unique downregulated genes were 352, 112, 732, and 384 in T helper cells, T cytotoxic cells, monocytes, and B lymphocytes, respectively (Figure 2E). Based on fold change values, top 20 DEGs showing marked upregulation and downregulation were enlisted in Supplementary Tables 2, 3, respectively. The list of top 20 upregulated and downregulated genes in monocytes were found to be unique with no overlap across other subsets.
Transcriptome Landscape of DEGs Like Antiviral Interferon-Stimulated Genes (ISGs), Chemokines, Interleukins, Viral Sensors (PRRs), Signaling Lymphocytic Activation Molecule (SLAM), Interferons, Granzyme B
ISGs highly expressed in lymphocytes were, ISG15, ISG20, IRF7, IFIT1, IFIT3, IFIT5, MX1, MX2, RELA, RSAD2, DDIT4, EIF2AK2, IFI6, OAS1X, PML, and RTP4. Monocytes showed a contrasting ISGs profile with most of the genes downregulated (Supplementary Figure 1). Among the chemokines and their receptors, CXCL10 was upregulated in lymphocytes and not differentially expressed in monocytes and CXCR4 was upregulated in lymphocytes as well as monocytes (Supplementary Figure 2A). More number of interleukins and their receptors were differentially expressed in lymphocytes than in monocytes. IL-1β was upregulated in lymphocytes and not differentially expressed in monocytes. IL-18 and receptor IL1RN were topmost upregulated interleukins in lymphocytes and monocytes, respectively (Supplementary Figure 2B). Among Viral RNA sensors, DDX58 and IFIH1 were found to be upregulated in lymphocytes and downregulated in monocytes. The differential expression of DDX58 across subsets in comparison to TLR/NLR expression indicated the predominant role of RIG-I-like receptors under PPRV infection (Supplementary Figure 3). IFN α and IFN β were not differentially expressed in lymphocytes and monocytes. However, IFN receptors IFNAR1 was downregulated in lymphocytes and monocytes while IFN Gamma receptors IFNGR1 and IFNGR2 were upregulated B lymphocytes (Supplementary Figure 3). SLAM was found to be downregulated in T helper cells (Log2 fold change −2.130), T cytotoxic cells (Log2 fold change −1.185), and B lymphocytes (Log2 fold change −2.204). On the contrary, it was not found to be differentially expressed in monocytes. Further, granzyme B (GZB) was found to be non-differentially expressed in lymphocytes and monocytes.
DEGs Involved in Pathways in the Viral Process, Viral Life Cycle, Viral Genome Replication etc.
Among the DEGs, several genes were found to be involved in viral process, viral life cycle, viral genome replication, regulation of viral process, regulation of viral life cycle, regulation of viral genome replication, viral gene expression, and defense response to virus. The number of genes involved in above-mentioned processes was more in lymphocytes than in monocytes. Among these genes ISG15, ISG20, IFIT3, MX2, IFIT1, IFIT2, IFIT5,OAS1X, MX1, DHX58, DDX58, HSPB1, DDIT4, RSAD2, EIF2AK2, NPC2, C19ORF66, ADAR, and DDX3X were found to be upregulated in lymphocytes and downregulated in monocytes. Whereas, as CXCL10, HMOX1, TMPRSS2, TNF, ICAM1, C1QBP, LEF1, EIF3D, and SNW1 were found to be upregulated in lymphocytes and not differentially expressed in monocytes (Supplementary Figure 4).
DEGs Selected Through a Knowledge-Based Approach
Out of these 106 knowledge-based genes, 71, 72, 72, and 80 were differentially expressed in T helper cells, T cytotoxic cells, monocytes, and B lymphocytes, respectively. Most of the genes were found to be downregulated in monocytes but upregulated in lymphocytes. The innate antiviral genes—ISGs; ISG15, ISG20, DDX58, DHX58, IRF3, IRF7, IFIT1, IFIT3, IFIT5, MX1, MX2, and RSAD2 were downregulated in monocytes indicating a dampened antiviral response of monocytes (Supplementary Figure 5).
Predicted PPI Network of DEGs
DEGs regulating the immune processes were selected through functional analysis. The number of immune-related genes with cut off fold change ≥±1.5 and degree ≥5 were 269, 250, 70, 356 in T helper cells, T cytotoxic cells, monocytes, and B lymphocytes, respectively, and were designated as differentially expressed highly connected (DEHC) genes. As the number of interactions was higher in monocytes, the cut off was increased to degree ≥10 reducing the number of DEHC genes to 194. The PPI networks were constructed between immune DEHC genes; and between the genes selected through knowledge-based approach (106 genes) and DEGs, which revealed common hubs (Supplementary Figures 6–9). In the PPI networks, hubs explain the functional and structural importance of a network. The genes, which act as hubs in PPI networks in different cells are mentioned in the Supplementary Tables 4, 5. ISG15, PML, MYC, and MAP3K5 genes were identified as common hubs in PPI network of immune DEHC-DEHC genes and ISG15, STAT1, and BCL6 genes were identified as common hubs in PPI networks of selected genes and DEGs across all the subsets. The network hub genes (except MAP3K5) were downregulated in monocytes and upregulated in lymphocytes.
Pathway Analysis by IPA
Core Analysis of T Helper Cells, T Cytotoxic Cells, B Lymphocytes, and Monocytes
Core analysis for each dataset was performed to know activated (Z score > 2) or inactivated (Z score < −2) canonical pathways. Moreover, the focus was on the apoptosis, cellular immune response, humoral immune response, cytokine signaling, and pathogen influenced signaling ingenuity canonical pathways.
T helper cells
Canonical pathways associated with T helper cells in infected goats at 9 dpi are represented in Figure 3A. The canonical pathway TNFR1 signaling was found to have the highest ratio of genes involved vis-a-vis the genes in the database. The top activated pathways based on Z score were TNFR1 signaling, MIF regulation of innate immunity and the role of RIG1 like receptors in antiviral immunity. The pathways—CD28 signaling in T Helper Cells, iCOS-iCOSL signaling in T helper cells, calcium-induced T lymphocyte apoptosis, the role of NFAT in the regulation of the immune response, Fc gamma receptor-mediated phagocytosis in macrophages and monocytes and PKC theta signaling in T Lymphocytes were found to be inactivated.
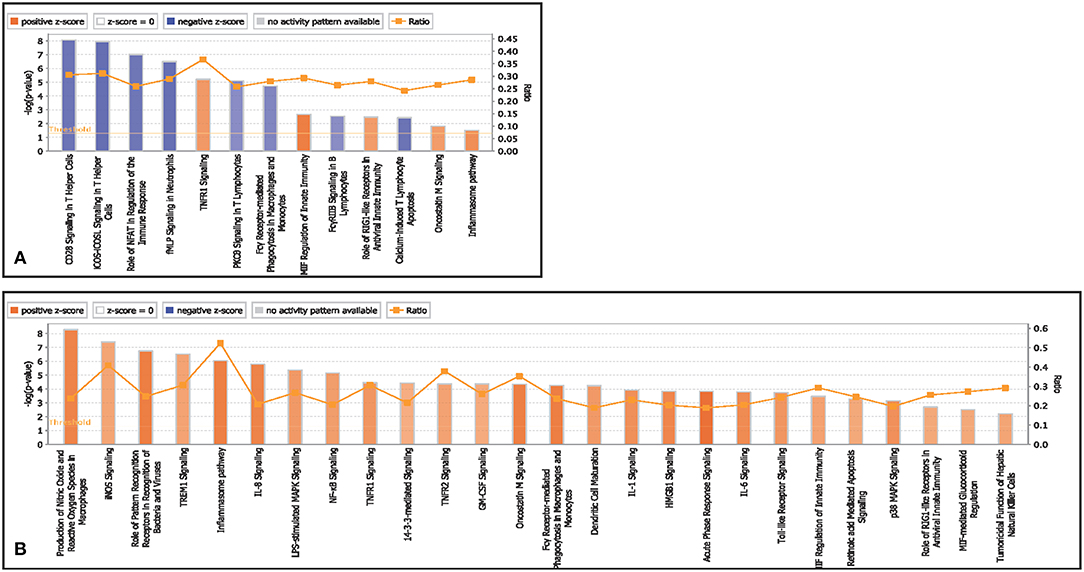
Figure 3. Canonical pathways activated/inactivated in (A) T helper cells (B) T cytotoxic cells of infected goats at 9 dpi generated in core analysis of Ingenuity pathway analysis tool. Orange color pathways are activated (>2) and blue color pathways are inactivated (<2). Height of the bar graphs indicates -log (p-value) and line graph showing the ratio of list genes found in each pathway over the total number of genes in that pathway.
T cytotoxic cells
Canonical pathways associated with T cytotoxic cells in infected goats at 9 dpi are represented in Figure 3B. The top activated pathways based on Z score were production of NO and ROS in macrophages, iNOs signaling, role of PRRs in recognition of pathogens, inflammasome pathway, IL-8 pathway, Oncostatin M signaling, and acute phase response signaling. The canonical pathway inflammasome pathway TNFR1 signaling was found to have the highest ratio of genes involved vis-a-vis the genes in the database.
Monocytes
Canonical pathways associated with monocytes in infected goats at 9 dpi are represented in Figure 4A. The top activated pathways based on Z score were IL-8 signaling, LPS stimulated MAPK signaling, 41B signaling in T lymphocytes, and IL-1 signaling. Interferon signaling was the only pathway to be inactivated. The canonical pathway inflammasome pathway was found to have the highest ratio of genes involved vis-a-vis the genes in the database.
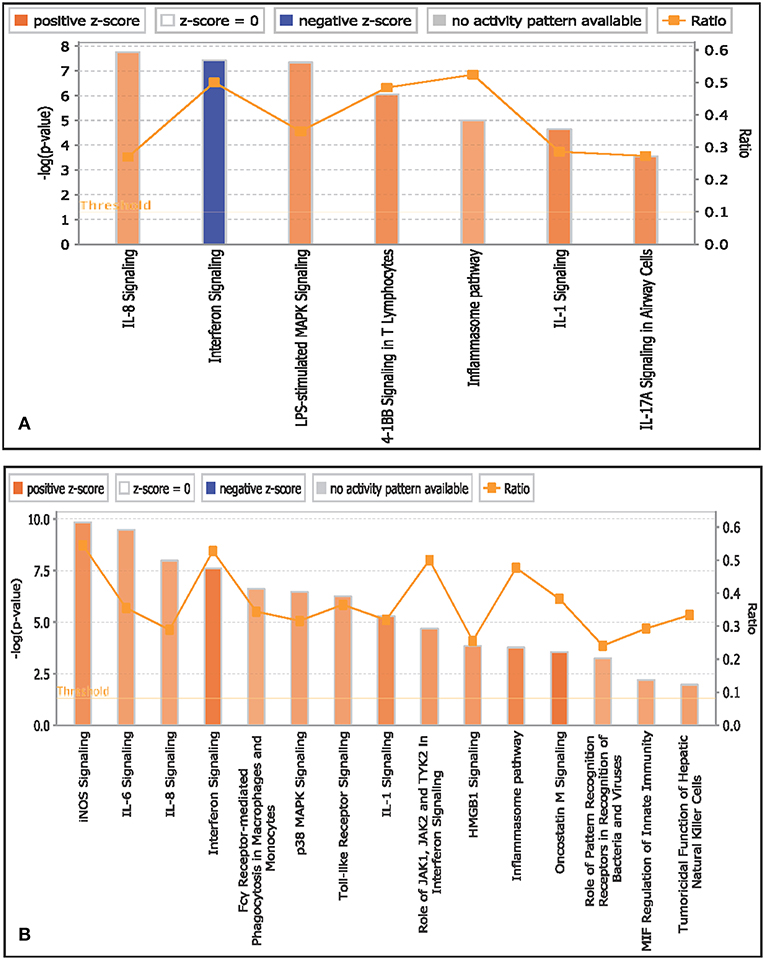
Figure 4. Canonical pathways activated/inactivated in (A) monocytes and (B) B lymphocytes of infected goats at 9 dpi generated in core analysis of Ingenuity pathway analysis tool. Orange color pathways are activated (>2) and blue color pathways are inactivated (<2). Height of the bar graphs indicates -log (p-value) and line graph show the ratio of list genes found in each pathway over the total number of genes in that pathway.
B lymphocytes
Canonical pathways associated with B lymphocytes in infected goats at 9 dpi are represented in Figure 4B. The top activated pathways based on Z score were interferon signaling, oncostatin M signaling, IL-1 signaling, inflammasome pathway, IL-1 signaling, acute phase response signaling, iNOS signaling, IL-6 signaling, p38 MAPK signaling, Toll-like receptor signaling, IL-8 signaling, MIF-mediated glucocorticoid regulation, and MIF regulation of innate immunity. The canonical pathways iNOS signaling and interferon signaling were found to have the highest ratio of genes involved vis-a-vis the genes in the database.
Comparison Analysis of Canonical Pathways Among T Helper Cells, T Cytotoxic Cells, B Lymphocytes, and Monocytes
Comparison of canonical pathways in T helper cells, T cytotoxic cells, monocytes, and B lymphocytes at 9 dpi in goats is represented in Supplementary Figure 10. Inflammasome pathway was activated in all cells. T cytotoxic cells had more number of activated pathways. MIF regulation of innate immunity, acute phase response signaling, acute phase response signaling, and Oncostatin M signaling were the topmost activated pathways in T helper cells, T cytotoxic cells, monocytes, and B lymphocytes, respectively.
Canonical pathways interferon signaling, Oncostatin M signaling, role of RIG 1 like receptors in antiviral immunity and complement system showed contrasting gene expression profiles in monocytes in comparison to lymphocytes. Interferon Signaling was found to be inactivated in monocytes (Z score −2.66) in comparison to T helper cells (Z score 0.77), T cytotoxic cells (Z score 1.88), and B lymphocytes (Z score 3.44). Genes in the interferon signaling network GIP2 (ISG15), IFIT1, IFIT3, MX1, IFI6, IFITM1, IFI35, STAT1, and STAT2 were significantly downregulated in monocytes. However, all these genes were upregulated in T helper cells, T cytotoxic cells, and B lymphocytes with varying degree of expression (Figure 5). Oncostatin M Signaling was found to be activated in T helper cells, T cytotoxic cells, and B lymphocytes. OSM was not differentially expressed and STAT1 and CH13L1 were downregulated in monocytes in contrast to their upregulation in T helper cells, T cytotoxic cells, and B lymphocytes (Figure 6). Role of RIG1-like Receptors in antiviral innate immunity was having lowest z score in monocytes (−0.83) in comparison to T helper cells (2.12), T cytotoxic cells (2.12), and B lymphocytes (1.89). A contrast in the expression of the genes involved in this pathway was also found in monocytes and lymphocytes (Figure 7). Complement system was found to be having negative z score in monocytes (−1.88) and a positive score in T helper cells (1.66), T cytotoxic cells (1.13), and B lymphocytes (0.37). Genes involved in the complement—C1q, C3b, C3a, and C3 were upregulated in lymphocytes but downregulated in monocytes. C1QBP was upregulated in lymphocytes but not significantly expressed in monocytes (Figure 8).
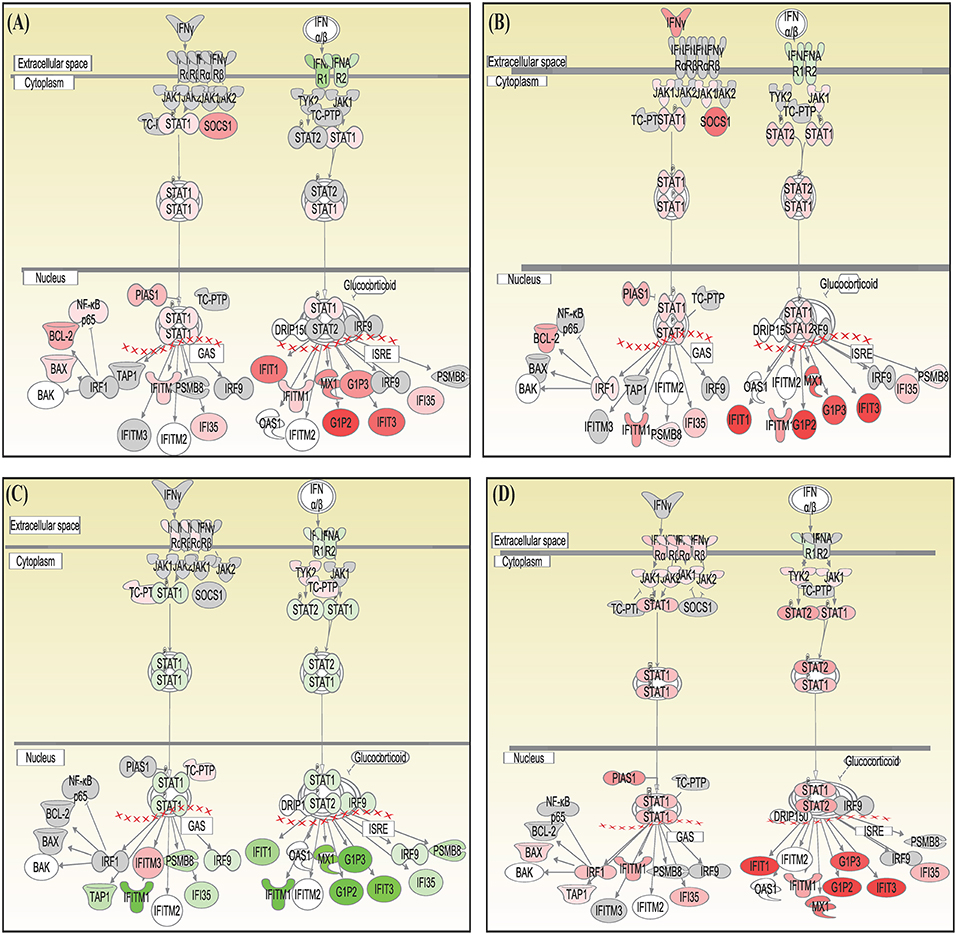
Figure 5. Canonical pathways generated in Ingenuity Pathway Analysis of interferon signaling pathway of DEGs in (A) T helper cells, (B) T cytotoxic cells, (C) monocytes, and (D) B lymphocytes of infected goats at 9 dpi. Genes that were upregulated are shown in red and downregulated in green. The intensity of red and green corresponds to an increase and decrease, respectively, in Log2 fold change. Genes in gray were not significantly differentially expressed and those in white are not present in the dataset but have been incorporated in the network through the relationship with other molecules by IPA. Symbol shape indicates gene function.
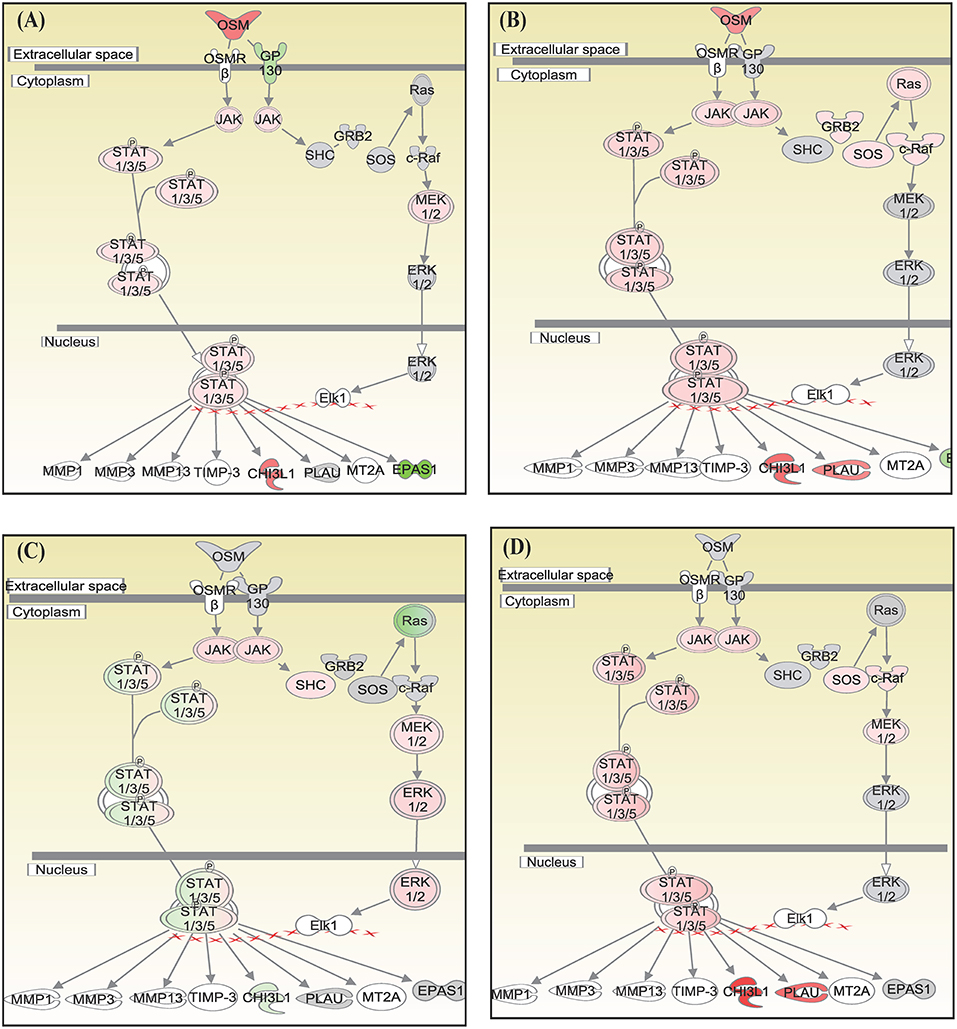
Figure 6. Canonical pathways generated in Ingenuity Pathway Analysis of Oncostatin M signaling pathway of DEGs in (A) T helper cells, (B) T cytotoxic cells, (C) monocytes, and (D) B lymphocytes of infected goats at 9 dpi. Genes that were upregulated are shown in red and downregulated in green. The intensity of red and green corresponds to an increase and decrease, respectively, in Log2 fold change. Genes in gray were not significantly differentially expressed and those in white are not present in the dataset but have been incorporated in the network through the relationship with other molecules by IPA. Symbol shape indicates gene function.
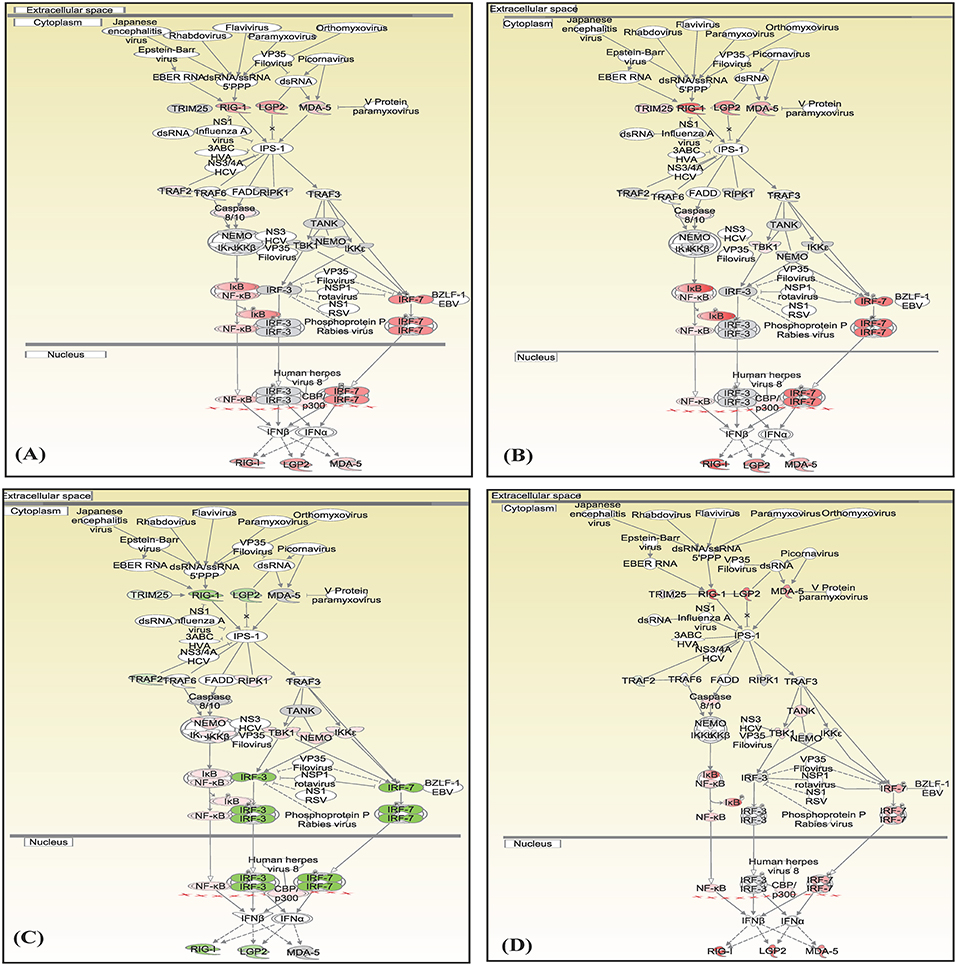
Figure 7. Canonical pathways generated in Ingenuity Pathway Analysis of the role of RIG 1 like receptors in antiviral immunity pathway of DEGs in (A) T helper cells, (B) T cytotoxic cells, (C) monocytes, and (D) B lymphocytes of infected goats at 9 dpi. Genes that were upregulated are shown in red and downregulated in green. The intensity of red and green corresponds to an increase and decrease, respectively, in Log2 fold change. Genes in gray were not significantly differentially expressed and those in white are not present in the dataset but have been incorporated in the network through the relationship with other molecules by IPA. Symbol shape indicates gene function.
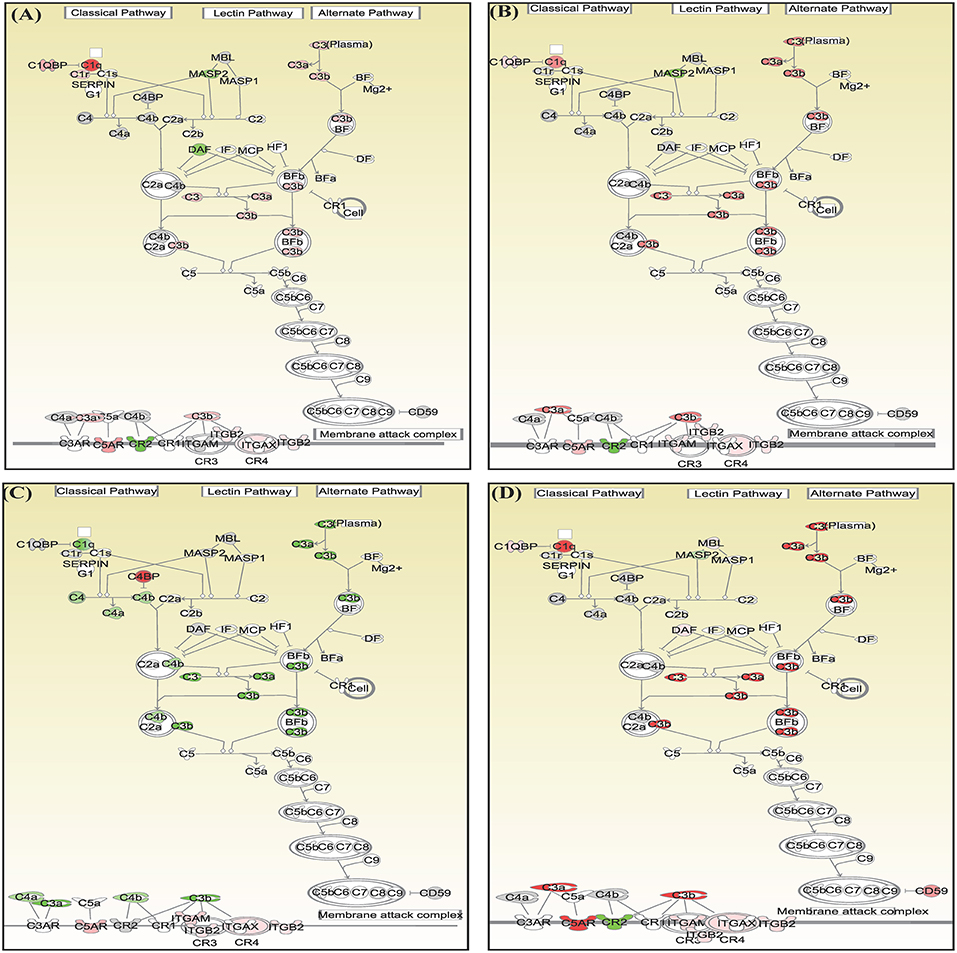
Figure 8. Canonical pathways generated in Ingenuity Pathway Analysis of role of complement system pathway of DEGs in (A) T helper cells, (B) T cytotoxic cells, (C) monocytes, and (D) B lymphocytes of infected goats at 9 dpi. Genes that were upregulated are shown in red and downregulated in green. The intensity of red and green corresponds to an increase and decrease, respectively, in Log2 fold change. Genes in gray were not significantly differentially expressed and those in white are not present in the dataset but have been incorporated in the network through the relationship with other molecules by IPA. Symbol shape indicates gene function.
Upstream Regulators, That Regulate DEGs in T Helper Cells, T Cytotoxic Cells, B Lymphocytes, and Monocytes
The number of TFs (activated/inactivated) differentially expressed and governing the DEGs in T helper cells, T cytotoxic cells, monocytes, and B lymphocytes were 21, 24, 10, and 30, respectively. The top four upregulated TFs in T helper cells were KLF4, IRF7, XBP1, and MYC; in T cytotoxic cells were KLF4, ETS2, NFKB1A, and IRF7; in monocytes were EGR1, PPP1R13L, and STAT3 and in B Lymphocytes were MXD1, KLF4, NFKBIA, and BCL6 (Supplementary Figure 11).
Master regulator, IRF7—transcription factor and STAT1—a transcription regulator, were found to be activated in T helper cells, T cytotoxic cells, and B lymphocytes and inactivated in monocytes. The genes governed by the IRF7 and STAT1 were mostly ISGs including IFIT3, MX1, and ISG15 (Supplementary Figures 12, 13). Also, KLF4 was found to be in the list of top 4 upregulated transcription factors in T helper cells (z score 2.62), T cytotoxic cells (z score −2.72), and B lymphocytes (z score −3.42). However, KLF4 was not found involved in monocytes (Supplementary Figure 14). Further, the comparative analysis of other upstream regulators (enzyme, GPCR, growth factors, ion channels, kinases, ligand-dependent nucleic acid receptors, peptidases, phosphates, translation regulators, transmembrane receptors, transporters) revealed DDX58, EIF2AK2, S100A8, S100A9, C3, ICAM1, and PARP9 genes to be downregulated and IFIH1, SAMSN1, EIF4E, and TNFAIP3 genes not to be differentially expressed in monocytes. On the contrary, these genes were upregulated in T helper cells, T cytotoxic cells, and B lymphocytes. The genes involved in EIF2AK2 and DDX58 network were mostly ISGs (Supplementary Figures 15, 16).
The contrasting features between the monocytes and the lymphocytes are clearly given in the graphical abstract (Figure 9).
DEGs Were Validated by qRT-PCR
Key genes identified from RNA sequencing data—DDX58, HERC5, IFIT3, IRF7, ISG15, and MX1 at 9 dpi were validated by qRT-PCR. The expression of all the validated genes was in concordance with RNA sequencing results (Figure 10).
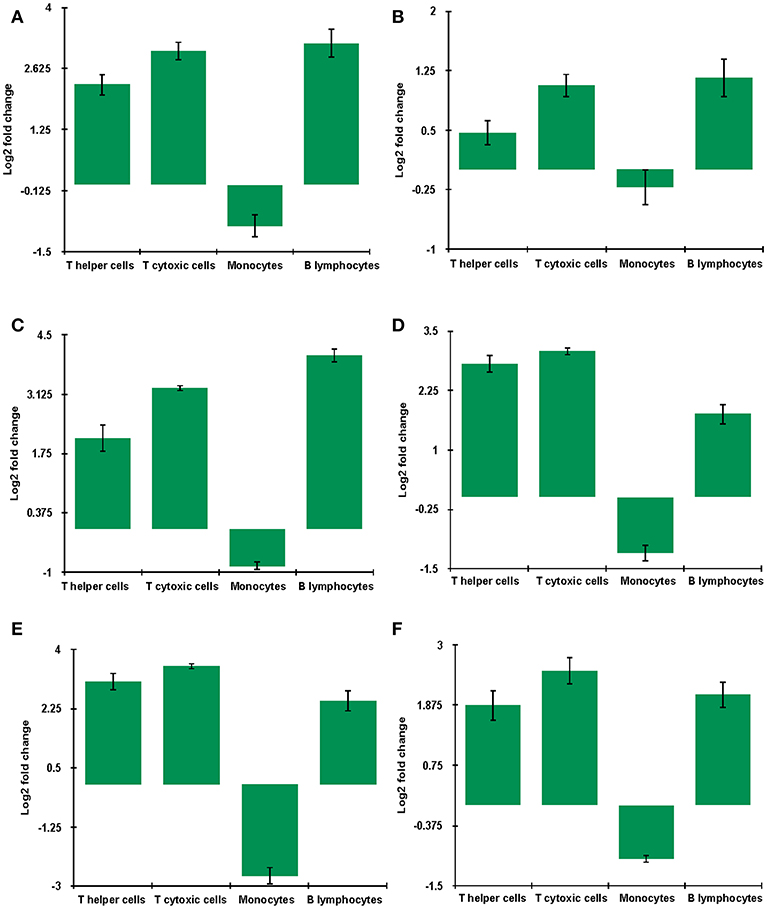
Figure 10. mRNA levels of genes (A) DDX58 (B) HERC5 (C) IFIT3 (D) IRF7 (E) ISG15 (F) MX1 in unvaccinated-infected goats at 9 dpi were validated using quantitative qRT-PCR with GAPDH as a reference gene for normalization. Log2 fold change calculated by delta-delta Ct method with control as the calibrator is represented along with the standard error of the difference.
Discussion
Transcriptome analysis of subsets of PBMCs and analysis of cell-specific pathways facilitate in understanding the regulatory networks involved under PPRV infection. Virulent PPRV has been detected in PBMCs (4, 9, 33–36). No experimental evidence is available regarding the immune cell types that are infected with virulent PPRV. Further, due to the fact that each of the cell types has a program inherited for generating a distinct immune response, the analysis of each cell type under PPRV infection attains significance (17). We, therefore, conducted transcriptome analysis at 0 days (control) and 9 dpi (a time that corresponds to the peak of viremia) of each subset of PBMCs to uncover the genes involved in host response and identify distinct transcriptional alterations in T helper cells, T cytotoxic cells, monocytes, and B lymphocytes.
In our study, expression of N gene in PPRV was detected in infected goats at 9 dpi in lymphocytes (T helper cells, T cytotoxic cells, and B lymphocytes) and not in monocytes which is in concordance with the alignment of reads with PPRV genome. Moreover, maximum viral transcripts were found in T helper cells in goats. These results indicate that the PPRV virulent virus probably replicates in lymphocytes and not in monocytes. The closely related viruses, Canine distemper virus (CDV) and Measles virus (MV) are reported to infect and replicate in T cells and not in monocytes (37–39). SLAM, the established receptor for PPRV, was found to be downregulated in T helper, T cytotoxic, and B lymphocytes and was not found to be differentially expressed in monocytes of the PRRV infected goats. The absence of any viral transcript in monocytes is consistent with the lack of differential expression of SLAM. The downregulation of SLAM expression with increased viral replication at 9 dpi in lymphocytes, may be due to superinfection exclusion phenomenon as observed in many viruses of human and veterinary importance (40). Superinfection exclusion is a phenomenon in which a pre-existing or established viral infection prevents the entry of same virus or a closely related virus. In our earlier PPRV host-pathogen interaction studies, SLAM was found to be downregulated in PBMCs (9).
Global transcriptome of monocytes indicated profound deviation from the lymphocytes as evident from a large number of unique upregulated genes (851) and downregulated genes (605) under PPRV infection. Differential expression analysis of immune cell types in trivalent inactivated influenza vaccine-induced immune response also showed unique transcriptomic expression profiles as well as changing biological networks (17). Among the genes differentially expressed under PPRV infection, there was predominant dysregulation in ISGs across all subtypes. Most of the ISGs and viral sensors were found to be upregulated in lymphocyte subtypes and downregulated in monocytes.
DEGs that were found to be involved in viral process, viral life cycle, and viral genome replication were mostly ISGs and few viral sensors, chemokines, and interleukins in all the subsets. RIG1-like Receptor signaling pathway as predicted through IPA analysis was least active in monocytes. The upregulated viral sensors in lymphocytes, MDA5 (IFIH1), and R1G-1 (DDX58) activate NFKB and IRF3/7 (41–43) and LGP2, inhibits paramyxovirus-induced activation of IFN genes (44). This indicates that a powerful sensing mechanism to induce effector antiviral molecules exists in PPRV infected lymphocytes, and not in monocytes. Further, ISGs—ISG15, Mx1, Mx2, RSAD2, IFIT3, and IFIT5 have a protective effect against various RNA viruses (8, 9, 45–57). The upregulation of ISGs in lymphocytes and downregulation in monocytes suggests a predominant role of lymphocytes and poor involvement of monocytes in anti-viral response against PPRV.
However, the positive regulators of ISGs (58), type I interferons (IFN-α/β) were not differentially expressed in lymphocytes or monocytes. The upstream regulator analysis in lymphocytes revealed activation of transcription factors, IRF-7, and STAT-1 that regulate most of the ISGs under PPRV infection. Thus, it expected that ISGs are transcriptionally induced more by IRF7 and STAT1 than by stimulation of interferons in PPRV infected lymphocytes. The presence of STAT1, which plays a vital role in interferon type I (IFN-α/β) and type II (IFN-γ) signaling (59) and the upregulation of ISGs substantiate for the activation of Interferon signaling pathway in B lymphocytes, T helper cells, and T cytotoxic cells under PPRV infection. On the contrary, inactivation of Interferon signaling pathway, IRF-7, and STAT-1 along with downregulation of ISGs was observed in monocytes.
IFN-α/β are mostly secreted by Plasmacytoid dendritic cells (pDCs) (60). Any kind of perturbance in their expression is appreciable in pDCs than in T or B cells. Type I interferons (IFN-α/β) were not differentially expressed in both lymphocytes or monocytes meaning that their expression is undeterred in B and T cells under PPRV infection. It is likely that PPRV is inhibiting the expression of IFN-α/β in pDCs and not in T or B cells. However, this warrants further studies.
Chemokine-CXCL10 that was upregulated in the T helper cells, T cytotoxic cells, and B lymphocytes and not differentially expressed in monocytes have been reported to have chemoattractant (61). IL-1β that was found upregulated in lymphocytes and not differentially expressed in monocytes, is a potent inflammatory cytokine involved in the recruitment of immune and inflammatory cells into the site of infection and influences the development of adaptive immune responses (62). This suggests the role of lymphocytes in the recruitment of immune cells and induction of apoptosis in PPRV infected cells. Further, Complement factors- C1QA, C1QBP, and C1QC were upregulated in lymphocytes and downregulated in monocytes indicating strong virus opsonization by lymphocytes. C1q (C1QA, C1QB, C1QC) stimulates the hemagglutination and neutralization activity (63).
Our data suggest that important cell type-specific information is gained through transcriptome analysis of PBMCs subsets than from PBMCs as a whole. The presence of PPRV; downregulation of SLAM receptor; upregulation of viral sensors—MDA5 and DDX58; activation of upstream regulators—IRF7 and STAT1; activation of interferon signaling pathway and; upregulation of ISGs in lymphocytes with a contrast in monocytes indicated the predominant role of lymphocytes in generating the antiviral response against PPRV in goats.
Data Availability
The datasets for this study were deposited in GEO (GSE132429).
Ethics Statement
The study was carried out after obtaining permission from Indian Veterinary Research Institute Animal Ethics Committee (IVRI—IAEC) under the Committee for the Purpose of Control and Supervision of Experiments on Animals (CPCSEA), India. The protocols were approved vide letter no 387/CPSCEA.
Author Contributions
RS, BPM, and RG conceived and designed the research. KR and DM performed the vaccine testing experiment. YS maintained the server for analysis. SW, ARS, DC and SS conducted the wet lab work. SW, RK, ARS, AP, VS, SK, and RG analyzed the data. SW, RG, RK, WM, APS, NH, AT, BS, and BM helped in manuscript drafting and editing. RS, BPM, and RG proofread the manuscript.
Conflict of Interest Statement
The authors declare that the research was conducted in the absence of any commercial or financial relationships that could be construed as a potential conflict of interest.
The reviewer NS and handling editor declared their shared affiliation.
Acknowledgments
This study was supported in part by Centre for Agricultural Bioinformatics (ICAR-IASRI) (CABin/100644/16103/ 801/10133) and SubDIC (BTISnet), ICAR-IVRI. We also thank Department of Biotechnology, Govt of India for providing fellowship and contingency for (DBT Fellow No. DBT/2014/IVRI/171), ARS (DBT Fellow No. DBT/2014/IVRI/170) and RK (DBT Fellow No. DBT/2017/IVRI/768).
Supplementary Material
The Supplementary Material for this article can be found online at: https://www.frontiersin.org/articles/10.3389/fimmu.2019.01463/full#supplementary-material
References
1. Singh RK, Balamurugan V, Bhanuprakash V, Sen A, Saravanan P, Yadav MP. Possible control and eradication of peste des petits ruminants from India: technical aspects. Vet Ital. (2009) 45:449–62.
2. Shaila M, Purushothaman V, Bhavasar D, Venugopal K, Venkatesan R. Peste des petits ruminants of sheep in India. Vet Rec. (1989) 125:602.
3. Birch J, Juleff N, Heaton MP, Kalbfleisch T, Kijas J, Bailey D. Characterization of ovine Nectin-4, a novel peste des petits ruminants virus receptor. J Virol. (2013) 87:4756–61. doi: 10.1128/JVI.02792-12
4. Pope RA, Parida S, Bailey D, Brownlie J, Barrett T, Banyard AC. Early events following experimental infection with peste-des-petits ruminants virus suggest immune cell targeting. PLoS ONE. (2013) 8:e55830. doi: 10.1371/journal.pone.0055830
5. Pawar R, Dhinakar GR, Balachandran C. Relationship between the level of signaling lymphocyte activation molecule mRNA and replication of Peste-des-petits-ruminants virus in peripheral blood mononuclear cells of host animals. Acta Virol. (2008) 52:231–6.
6. Hamalainen H, Meissner S, Lahesmaa R. Signaling lymphocytic activation molecule (SLAM) is differentially expressed in human Th1 and Th2 cells. J Immunol Methods. (2000) 242:9–19. doi: 10.1016/S0022-1759(00)00200-3
7. Erlenhoefer C, Wurzer WJ, Löffler S, Schneider-Schaulies S, ter Meulen V, Schneider-Schaulies J. CD150 (SLAM) is a receptor for measles virus but is not involved in viral contact-mediated proliferation inhibition. J Virol. (2001) 75:4499–505. doi: 10.1128/JVI.75.10.4499-4505.2001
8. Manjunath S, Mishra BP, Mishra B, Sahoo AP, Tiwari AK, Rajak KK, et al. Comparative and temporal transcriptome analysis of peste des petits ruminants virus infected goat peripheral blood mononuclear cells. Virus Res. (2017) 229:28–40. doi: 10.1016/j.virusres.2016.12.014
9. Manjunath S, Mishra B, Mishra BP, Saxena S, Mondal P, Sahu AR, et al. Identification of suitable reference gene in goat peripheral blood mononuclear cells (PBMCs) infected with peste des petits ruminants virus (PPRV). Livestock Sci. (2015) 181:150–5. doi: 10.1016/j.livsci.2015.09.010
10. Iwasa T, Suga S, Qi L, Komada Y. Apoptosis of human peripheral blood mononuclear cells by wild-type measles virus infection is induced by interaction of hemagglutinin protein and cellular receptor, SLAM via caspase-dependent pathway. Microbiol Immunol. (2010) 54:405–16. doi: 10.1111/j.1348-0421.2010.00231.x
11. Bolt G, Berg K, Blixenkrone-Møller M. Measles virus-induced modulation of host-cell gene expression. J Gen Virol. (2002) 83:1157–65. doi: 10.1099/0022-1317-83-5-1157
12. Manjunath S, Kumar GR, Mishra BP, Mishra B, Sahoo AP, Joshi CG, et al. Genomic analysis of host–Peste des petits ruminants vaccine viral transcriptome uncovers transcription factors modulating immune regulatory pathways. Vet Res. (2015) 46:15. doi: 10.1186/s13567-015-0153-8
13. Pandey A, Sahu AR, Wani SA, Saxena S, Kanchan S, Sah V, et al. Modulation of host miRNAs transcriptome in lung and spleen of peste des petits ruminants virus infected sheep and goats. Front Microbiol. (2017) 8:1146. doi: 10.3389/fmicb.2017.01146
14. Khanduri A, Sahu AR, Wani SA, Khan RIN, Pandey A, Saxena S, et al. Dysregulated miRNAome and proteome of PPRV infected goat PBMCs reveal a coordinated immune response. Front Immunol. (2018) 9:2631. doi: 10.3389/fimmu.2018.02631
15. Sahu AR, Wani SA, Saxena S, Rajak KK, Chaudhary D, Sahoo AP, et al. Selection and validation of suitable reference genes for qPCR gene expression analysis in goats and sheep under Peste des petits ruminants virus (PPRV), lineage IV infection. Sci Rep. (2018) 8:15969. doi: 10.1038/s41598-018-34236-7
16. Subramanian N, Torabi-Parizi P, Gottschalk RA, Germain RN, Dutta B. Network representations of immune system complexity. Wiley Interdisc Rev. (2015) 7:13–38. doi: 10.1002/wsbm.1288
17. Hoek KL, Samir P, Howard LM, Niu X, Prasad N, Galassie A, et al. A cell-based systems biology assessment of human blood to monitor immune responses after influenza vaccination. PLoS ONE. (2015) 10:e0118528. doi: 10.1371/journal.pone.0118528
18. Menicucci AR, Versteeg K, Woolsey C, Mire CE, Geisbert JB, Cross RW, et al. Transcriptome analysis of circulating immune cell subsets highlight the role of monocytes in Zaire Ebola virus makona pathogenesis. Front Immunol. (2017) 8:1372. doi: 10.3389/fimmu.2017.01372
19. Sreenivasa B, Dhar P, Singh R, Bandyopadhyay S editors. Development of peste des petits ruminants (PPR) challenge virus from a field isolate. In: XIV Annual Conference and National Seminar on Management of Viral Diseases With Emphasis on Global Trade and WTO Regime of Indian Virological Society (2002).
20. Sahu AR, Wani SA, Saminathan M, Rajak KK, Sahoo AP, Pandey A, et al. Genome sequencing of an Indian peste des petits ruminants virus isolate, Izatnagar/94, and its implications for virus diversity, divergence and phylogeography. Arch Virol. (2017) 162:1677–93. doi: 10.1007/s00705-017-3288-2
21. Singh R, Sreenivasa B, Dhar P, Shah L, Bandyopadhyay S. Development of a monoclonal antibody based competitive-ELISA for detection and titration of antibodies to peste des petits ruminants (PPR) virus. Vet Microbiol. (2004) 98:3–15. doi: 10.1016/j.vetmic.2003.07.007
22. Raj GD, Nachimuthu K, Nainar AM. A simplified objective method for quantification of peste des petits ruminants virus or neutralizing antibody. J Virol Methods. (2000) 89:89–95. doi: 10.1016/S0166-0934(00)00206-8
23. Wani SA, Sahu AR, Saxena S, Rajak KK, Saminathan M, Sahoo AP, et al. Expression kinetics of ISG15, IRF3, IFNγ, IL10, IL2 and IL4 genes vis-a-vis virus shedding, tissue tropism and antibody dynamics in PPRV vaccinated, challenged, infected sheep and goats. Microb Pathog. (2018) 117:206–18. doi: 10.1016/j.micpath.2018.02.027
24. Schmieder R, Edwards R. Quality control and preprocessing of metagenomic datasets. Bioinformatics. (2011) 27:863–4. doi: 10.1093/bioinformatics/btr026
25. Bickhart DM, Rosen BD, Koren S, Sayre BL, Hastie AR, Chan S, et al. Single-molecule sequencing and chromatin conformation capture enable de novo reference assembly of the domestic goat genome. Nat Genet. (2017) 49:643. doi: 10.1038/ng.3802
26. Li B, Dewey CN. RSEM: accurate transcript quantification from RNA-Seq data with or without a reference genome. BMC Bioinformatics. (2011) 12:323. doi: 10.1186/1471-2105-12-323
27. Reimand J, Arak T, Vilo J. g: Profiler—a web server for functional interpretation of gene lists (2011 update). Nucleic Acids Res. (2011) 39(Suppl. 2):W307–15. doi: 10.1093/nar/gkr378
28. Stark C, Breitkreutz B-J, Reguly T, Boucher L, Breitkreutz A, Tyers M. BioGRID: a general repository for interaction datasets. Nucleic Acids Res. (2006) 34(Suppl. 1):D535–9. doi: 10.1093/nar/gkj109
29. Yu H, Luscombe NM, Lu HX, Zhu X, Xia Y, Han J-DJ, et al. Annotation transfer between genomes: protein–protein interologs and protein–DNA regulogs. Genome Res. (2004) 14:1107–18. doi: 10.1101/gr.1774904
30. Shannon P, Markiel A, Ozier O, Baliga NS, Wang JT, Ramage D, et al. Cytoscape: a software environment for integrated models of biomolecular interaction networks. Genome Res. (2003) 13:2498–504. doi: 10.1101/gr.1239303
31. Langmead B, Salzberg SL. Fast gapped-read alignment with Bowtie 2. Nat Methods. (2012) 9:357. doi: 10.1038/nmeth.1923
32. Schmittgen TD, Livak KJ. Analyzing real-time PCR data by the comparative C T method. Nat Protoc. (2008) 3:1101. doi: 10.1038/nprot.2008.73
33. Patel A, Rajak KK, Balamurugan V, Sen A, Sudhakar SB, Bhanuprakash V, et al. Cytokines expression profile and kinetics of Peste des petits ruminants virus antigen and antibody in infected and vaccinated goats. Virol Sin. (2012) 27:265–71. doi: 10.1007/s12250-012-3240-2
34. Mondal B, Sreenivasa B, Dhar P, Singh R, Bandyopadhyay S. Apoptosis induced by peste des petits ruminants virus in goat peripheral blood mononuclear cells. Virus Res. (2001) 73:113–9. doi: 10.1016/S0168-1702(00)00214-8
35. Dhanasekaran S, Biswas M, Vignesh AR, Ramya R, Raj GD, Tirumurugaan KG, et al. Toll-like receptor responses to Peste des petits ruminants virus in goats and water buffalo. PLoS ONE. (2014) 9:e111609. doi: 10.1371/journal.pone.0111609
36. Nizamani ZA, Servan de Almeida R, Albina E, Parveen F, Libeau G. In vitro study of lymphotropic and immunomodulatory properties of the peste des petits ruminants virus (pprv). J Anim Plant Sci. (2014) 24:1380–7.
37. Von Messling V, Svitek N, Cattaneo R. Receptor (SLAM [CD150]) recognition and the V protein sustain swift lymphocyte-based invasion of mucosal tissue and lymphatic organs by a morbillivirus. J Virol. (2006) 80:6084–92. doi: 10.1128/JVI.00357-06
38. Von Messling V, Milosevic D, Cattaneo R. Tropism illuminated: lymphocyte-based pathways blazed by lethal morbillivirus through the host immune system. Proc Natl Acad Sci USA. (2004) 101:14216–21. doi: 10.1073/pnas.0403597101
39. De Swart RL, Ludlow M, De Witte L, Yanagi Y, Van Amerongen G, McQuaid S, et al. Predominant infection of CD150+ lymphocytes and dendritic cells during measles virus infection of macaques. PLoS Pathog. (2007) 3:e178. doi: 10.1371/journal.ppat.0030178
40. Folimonova SY. Superinfection exclusion is an active virus-controlled function that requires a specific viral protein. J Virol. (2012) 86:5554–61. doi: 10.1128/JVI.00310-12
41. Seth RB, Sun L, Ea C-K, Chen ZJ. Identification and characterization of MAVS, a mitochondrial antiviral signaling protein that activates NF-κB and IRF3. Cell. (2005) 122:669–82. doi: 10.1016/j.cell.2005.08.012
42. Onoguchi K, Yoneyama M, Fujita T. Retinoic acid-inducible gene-I-like receptors. J Interf Cytokine Res. (2011) 31:27–31. doi: 10.1089/jir.2010.0057
43. Loo Y-M, Gale M Jr. Immune signaling by RIG-I-like receptors. Immunity. (2011) 34:680–92. doi: 10.1016/j.immuni.2011.05.003
44. Childs K, Randall R, Goodbourn S. Paramyxovirus V proteins interact with the RNA Helicase LGP2 to inhibit RIG-I-dependent interferon induction. J Virol. (2012) 86:3411–21. doi: 10.1128/JVI.06405-11
45. Schneider WM, Chevillotte MD, Rice CM. Interferon-stimulated genes: a complex web of host defenses. Annu Rev Immunol. (2014) 32:513–45. doi: 10.1146/annurev-immunol-032713-120231
46. Vanderven HA, Petkau K, Ryan-Jean KE, Aldridge JR Jr, Webster RG, Magor KE. Avian influenza rapidly induces antiviral genes in duck lung and intestine. Mol Immunol. (2012) 51:316–24. doi: 10.1016/j.molimm.2012.03.034
47. Braun BA, Marcovitz A, Camp JG, Jia R, Bejerano G. Mx1 and Mx2 key antiviral proteins are surprisingly lost in toothed whales. Proc Natl Acad Sci USA. (2015) 112:8036–40. doi: 10.1073/pnas.1501844112
48. Espert L, Degols G, Lin Y-L, Vincent T, Benkirane M, Mechti N. Interferon-induced exonuclease ISG20 exhibits an antiviral activity against human immunodeficiency virus type 1. J Gen Virol. (2005) 86:2221–9. doi: 10.1099/vir.0.81074-0
49. Espert L, Degols G, Gongora C, Blondel D, Williams BR, Silverman RH, et al. ISG20, a new interferon-induced RNase specific for single-stranded RNA, defines an alternative antiviral pathway against RNA genomic viruses. J Biol Chem. (2003) 278:16151–8. doi: 10.1074/jbc.M209628200
50. Zhou Z, Wang N, Woodson SE, Dong Q, Wang J, Liang Y, et al. Antiviral activities of ISG20 in positive-strand RNA virus infections. Virology. (2011) 409:175–88. doi: 10.1016/j.virol.2010.10.008
51. Hsiang T-Y, Zhao C, Krug RM. Interferon-induced ISG15 conjugation inhibits influenza A virus gene expression and replication in human cells. J Virol. (2009) 83:5971–7. doi: 10.1128/JVI.01667-08
52. Lenschow DJ, Giannakopoulos NV, Gunn LJ, Johnston C, O'Guin AK, Schmidt RE, et al. Identification of interferon-stimulated gene 15 as an antiviral molecule during Sindbis virus infection in vivo. J Virol. (2005) 79:13974–83. doi: 10.1128/JVI.79.22.13974-13983.2005
53. Okumura A, Pitha PM, Harty RN. ISG15 inhibits Ebola VP40 VLP budding in an L-domain-dependent manner by blocking Nedd4 ligase activity. Proc Natl Acad Sci USA. (2008) 105:3974–9. doi: 10.1073/pnas.0710629105
54. Durfee LA, Lyon N, Seo K, Huibregtse JM. The ISG15 conjugation system broadly targets newly synthesized proteins: implications for the antiviral function of ISG15. Mol Cell. (2010) 38:722–32. doi: 10.1016/j.molcel.2010.05.002
55. Dai J, Pan W, Wang P. ISG15 facilitates cellular antiviral response to dengue and west nile virus infection in vitro. Virol J. (2011) 8:468. doi: 10.1186/1743-422X-8-468
56. Werneke SW, Schilte C, Rohatgi A, Monte KJ, Michault A, Arenzana-Seisdedos F, et al. ISG15 is critical in the control of Chikungunya virus infection independent of UbE1L mediated conjugation. PLoS Pathog. (2011) 7:e1002322. doi: 10.1371/journal.ppat.1002322
57. Zhao C, Collins MN, Hsiang T-Y, Krug RM. Interferon-induced ISG15 pathway: an ongoing virus–host battle. Trends Microbiol. (2013) 21:181–6. doi: 10.1016/j.tim.2013.01.005
58. Lazear HM, Lancaster A, Wilkins C, Suthar MS, Huang A, Vick SC, et al. IRF-3, IRF-5, and IRF-7 coordinately regulate the type I IFN response in myeloid dendritic cells downstream of MAVS signaling. PLoS Pathog. (2013) 9:e1003118. doi: 10.1371/journal.ppat.1003118
59. Wang J, Basagoudanavar SH, Wang X, Hopewell E, Albrecht R, García-Sastre A, et al. NF-κB RelA subunit is crucial for early IFN-β expression and resistance to RNA virus replication. J Immunol. (2010) 185:1720–9. doi: 10.4049/jimmunol.1000114
60. Manz MG. Plasmacytoid dendritic cells: origin matters. Nat Immunol. (2018) 19:652. doi: 10.1038/s41590-018-0143-x
61. Park S-J, Kumar M, Kwon HI, Seong R-K, Han K, Song JM, et al. Dynamic changes in host gene expression associated with H5N8 avian influenza virus infection in mice. Sci Rep. (2015) 5:16512. doi: 10.1038/srep16512
62. Dinarello CA. Anti-inflammatory agents: present and future. Cell. (2010) 140:935–50. doi: 10.1016/j.cell.2010.02.043
Keywords: lymphocytes, monocytes, RNA sequencing, PPRV, goat, host-pathogen interaction
Citation: Wani SA, Sahu AR, Khan RIN, Pandey A, Saxena S, Hosamani N, Malla WA, Chaudhary D, Kanchan S, Sah V, Rajak KK, Muthuchelvan D, Mishra B, Tiwari AK, Sahoo AP, Sajjanar B, Singh YP, Gandham RK, Mishra BP and Singh RK (2019) Contrasting Gene Expression Profiles of Monocytes and Lymphocytes From Peste-Des-Petits-Ruminants Virus Infected Goats. Front. Immunol. 10:1463. doi: 10.3389/fimmu.2019.01463
Received: 31 January 2019; Accepted: 10 June 2019;
Published: 05 July 2019.
Edited by:
Javier Dominguez, Instituto Nacional de Investigación y Tecnología Agraria y Alimentaria (INIA), SpainReviewed by:
Noemi Sevilla, Instituto Nacional de Investigación y Tecnología Agraria y Alimentaria (INIA), SpainHarry D. Dawson, Agricultural Research Service (USDA), United States
Copyright © 2019 Wani, Sahu, Khan, Pandey, Saxena, Hosamani, Malla, Chaudhary, Kanchan, Sah, Rajak, Muthuchelvan, Mishra, Tiwari, Sahoo, Sajjanar, Singh, Gandham, Mishra and Singh. This is an open-access article distributed under the terms of the Creative Commons Attribution License (CC BY). The use, distribution or reproduction in other forums is permitted, provided the original author(s) and the copyright owner(s) are credited and that the original publication in this journal is cited, in accordance with accepted academic practice. No use, distribution or reproduction is permitted which does not comply with these terms.
*Correspondence: Ravi Kumar Gandham, gandham71@gmail.com; Bishnu Prasad Mishra, bpmishra_1@hotmail.com; Raj Kumar Singh, rks_virology@rediffmail.com