Corrigendum: Association of LRP1B Mutation With Tumor Mutation Burden and Outcomes in Melanoma and Non-small Cell Lung Cancer Patients Treated With Immune Check-Point Blockades
- 1Clinical Epidemiology Unit, Qilu Hospital of Shandong University, Jinan, China
- 2Key Laboratory of Cancer Prevention and Therapy of Tianjin, Department of Epidemiology and Biostatistics, National Clinical Research Center for Cancer, Tianjin Medical University Cancer Institute and Hospital, Tianjin, China
- 3Key Laboratory of Cancer Prevention and Therapy, Department of Breast Cancer Pathology and Research Laboratory, National Clinical Research Center for Cancer, Tianjin Medical University Cancer Institute and Hospital, Tianjin, China
- 4Department of Respiratory Medicine, Central Hospital of Zibo, Zibo, China
- 5Institute of Pathology and Southwest Cancer Center, Southwest Hospital, Army Medical University (Third Military Medical University), Chongqing, China
- 6Key Laboratory of Tumor Immunopathology, Ministry of Education of China, Institute of Pathology and Southwest Cancer Center, Southwest Hospital, Third Military Medical University (Army Medical University), Chongqing, China
- 7Department of Epidemiology and Biostatistics, First Affiliated Hospital, Army Medical University, Chongqing, China
Background: Tumor mutation burden (TMB) have been served as the most prevalent biomarkers to predict immunotherapy response. LRP1B (low-density lipoprotein receptor-related protein 1B) is frequently mutated in melanoma, non-small cell lung cancer (NSCLC) and other tumors; however, its association with TMB and survival in patients with immunotherapy remains unknown.
Methods: We curated somatic mutation data and clinicopathologic information from 332 melanoma immunotherapy samples for discovery and 113 NSCLC samples for further corroboration. Bayesian variants non-negative matrix factorization was used to extract tumor mutational signatures. Multivariate Cox and logistic regression models were applied to adjust confounding factors. The CIBERSORT and GSEA algorithm were separately used to infer leukocyte relative abundance and significantly enriched pathways.
Results: Patients with LRP1B mutation were identified to be associated with prolonged survival in both immunotherapy cohort. Higher tumor mutation burden was found in LRP1B mutated patients, and the association remained significant after controlling for age, gender, stage, mutations in TP53 and ATR, and mutational signatures. Immune response and cell cycle regulation circuits were among the top enriched pathways in samples with LRP1B mutations.
Conclusion: Our studies suggested sequencing even a single, frequently mutated gene may provide insight into genome-wide mutational burden, and may serve as a biomarker to predict immune response.
Introduction
Immune checkpoint blockades (ICB) therapy such as anti-CTLA-4 and/or anti-PD-1 have demonstrated durable antitumor effects in treatment of multiple cancers (1–4). Tumor mutation burden was broadly considered as biomarkers associated with clinical response to ICB treatment in melanoma (5–7), non-small cell lung cancer (NSCLC) (8, 9), colorectal and gastric cancers (10). Mutations in genomic integrity associated genes, such as TP53 (11) and ATR (12), could cause genomic instability, replication stress and resulting a higher mutation rate in tumor genomes. Somatic mutation caused tumor-specific neoantigen (neopeptide fragments) that could serve as markers to identify the responders to ICB treatment (13, 14). It is also reported that neoantigen burden have a correlation with tumor mutation burden (5, 8).
The low-density lipoprotein receptor-related protein 1B (LRP1B), which encoding endocytic LDL-family receptor, is among the top 10 significantly mutated genes in human cancer (15). It has been demonstrated that LRP1B could bind to multiple extracellular ligands, including fibrinogen and apoE-carrying lipoproteins. Frequently inactivation mutation of LRP1B was observed in melanoma (16), lung cancer (17), esophagus squamous-cell carcinoma (18), head and neck squamous cancer (19, 20), gastric cacner (21), and so on. Owing to its large size (coding sequence, 16 kbp), LRP1B is often missed as a significantly mutated gene analysis, whereas its mutation still could have a functional consequence in tumorigenesis and heterogeneity.
The characteristic mutational signatures are the fingerprints of endogenous and exogenous factors that have acted over the course of tumor development and progression. Exogenous mutational signatures, such as ultraviolet radiation exposure (22, 23), tobacco smoking (9) and endogenous mutational signatures, such as APOBEC family of cytidine deaminases (24) mismatch repair defiency (10) were all contributed to higher tumor mutation burden and immune responding.
Tumor microenvironment (TME) also associate with response to ICB therapy. Baseline levels of tumor-infiltrating CD8+ T cells, CD4 T+ cells and NK cells were shown to be correlated with the likelihood of immune response (25–27). Mutations in genes involved in antigen presentation and interferon-related circuits were reported with immune (28, 29). Recently, a T cell-inflamed gene expression profile (GEP) was shown to predict response to immunotherapy (30, 31).
LRP1B is one of the most frequently mutated genes in tumor samples; however, its associations with TMB and prognosis remain unclear. In this study, we investigated whether LRP1B mutations are associated with TMB and survival prognosis in patients treated by immune check-point blockades. Findings emerged from this study may be useful for guiding immunotherapy treatment for cancer patients.
Methods
Genomic Data and Clinical Information of Melanoma and NSCLC
Somatic mutations were acquired from previous WES studies totaling 332 melanoma cases (5–7, 23, 34) and 113 NSCLC cases (8, 9, 32). Whole exome capture libraries were constructed using the Agilent SureSelect All Exon V2/V4 or 50 Mb kit. Enriched exome libraries were sequenced on a HiSeq 2000, 2500, or 4000 platform (Illumina) to generate paired-end reads (2 × 76 bp/100 bp) to a goal of 178X mean target coverage (range 32–380). The detailed sequencing information of each cohort was collected and illustrated in Supplementary Table S1. Mutations were re-annotated by oncotator against hg19 reference genome (33). Clinicopathological information including age, gender, stage, PD-L1 expression, smoke, ICB types, immune response status and survival were curated from supplemental materials of these studies (Supplementary Table S2). The predicted MHC binding affinity scores, HLA types, and clinical features were also collected from the previous studies (5, 7–9, 32, 34) (melanoma, n = 224; NSCLC, n = 113). Patients with complete or partial responses were considered to be efficacious to ICB treatment and the rest were regarded as the non-responding. Gene expression data in melanoma were available in Riaz.N and Hugo.W cohorts (6, 23). Gene expression data for NSCLC cohort were not available. Somatic mutations for samples in the TCGA datasets of SKCM (n = 467), NSLC (n = 998) were downloaded from Genome Data Commons (https://portal.gdc.cancer.gov).
Deciphering Mutational Signature Operative in the Genome
We used SignatureAnalyzer (https://software.broadinstitute.org/cancer/cga/Home) proposed by Kim et al. (35) to extract mutational signatures from the aggregated somatic mutation data in melanoma and NSCLC cohorts. This framework is based on Bayesian variant non-negative matrix factorization and it can automatically determine the optimal number of extracted mutational signatures. The SignatureAnalyzer factorized the mutation portrait matrix into two non-negative matrices W and H, where W representing mutational processes and H representing the corresponding mutational activities. The number of mutational signatures is the number of columns of matrix W. The rows of matrix A are the 96 mutational contexts (i.e., C > A, C > G, C > T, T > A, T > C, T > G, and their 5′and 3′ adjacent bases), and its columns are the samples of each cohorts (melanoma, n = 332; NSCLC, n = 113). Mutational signatures were annotated by calculating cosine similarity against 30 validated mutational signatures in the Catalog of Somatic Mutations in Cancer (COSMIC, v85) (36).
LRP1B Mutation With Tumor Mutation Burden
The extracted mutational signatures were stratified as binary variables (i.e., 0 and 1) in the multivariate model. The classified method is according to the previous study, which a signature was considered significant if it contributed to more than 100 substitutions or more than 25% of total mutation activities (37). As mutations in TP53 and ATR and some mutational signatures increase mutation rates in the cancer genome, we used Generalized Linear Models and Fit Proportional Hazards Regression Model to analyze associations between LRP1B mutation and TMB by including them as confounding factors. TMB was defined the number of non-synonymous alterations (SNVs or indels) using whole exome sequencing for each patient, and the median served as the cutoff value of high vs. low.
Gene set Enrichment Analysis
We partitioned 72 cases whose gene expression profiles were available into two groups according to mutation status of LRP1B. Specifically, read counts of gene expression data were normalized by calcNormFactors in R package edgeR and remove batch effect by removeBatchEffect functions in package limma. We then fed them into lmFit and eBayes functions in the R limma package, and used these statistics as input to R-function in ClusterProfile package to do gene set enrichment analysis (GSEA). The gene sets examined in GSEA of REACTOME pathways were obtained from MSigDB database (v6.2) (38).
Tumor Infiltrating Lymphocyte Cell Analysis
We curated gene expression profile of 72 pre-treatment melanoma samples from two studies of Riaz and Hugo et al. (6, 23). The relative abundance of 22 tumor infiltrating lymphocyte cells (TILs) in different LRP1B mutation status were estimated by the CIBERSORT algorithm (39), a computational approach for inferring leukocyte representation in bulk tumor transcriptomes.
T Cell-Inflamed Gene Expression (RNA) Profiling (GEP) Scores
We applied and followed the T cell-inflamed Gene expression (RNA) profiling (GEP) proposed by Ayers et al. (31) to quantify the GEP scores. The GEP was composed of 18 inflammatory genes associated with chemokine expression, cytolytic activity, antigen presentation, and adaptive immune resistance, including CD274 (PD-L1), CD276 (B7-H3), CCL5, CD27, CD8A, CMKLR1, CXCL9, CXCR6, IDO1, LAG3, NKG7, PDCD1LG2 (PDL2), PSMB10, HLA-DQA1, HLA-DRB1, HLA-E, STAT1, and TIGIT. GEP scores were calculated as a weighted mean of normalized expression values for the 18 genes.
Statistical Analyses
The statistical analyses in this study was performed with R software (version 3.2.3). The continuous variables between groups were compared by the Wilcoxon rank sum test. The association between proportion of mutated genes and immune therapy response was evaluated by Fisher's exact test. Kaplan-Meier survival analysis and Cox proportional hazards model were used to analyze the association between mutated genes and prognosis with the R package survminer and forestmodel. Association of LRP1B mutation with TMB was examined by the logistic regression by including confounding factors such age, gender, stage, PD-L1, TP53, ATR mutation and extracted mutational signatures. All comparisons were two-sided with a significance level of 0.05, and the Benjamini-Hochberg method was applied to control false discovery rate (FDR) for multiple hypothesis testing (40).
Results
Tumor Genomic Alterations in Melanoma Cohort
Of the 332 melanoma patients from previous genomic immune studies, 98 (29.5%) were recognized as immune responders. LRP1B was one of the most frequently mutated genes in the aggregated melanoma cohort, accounting for 122 of 332 patients (36.7%). The mutation plots of LRP1B in different immune response status are shown in Supplementary Figure 1. Melanoma samples with LRP1B mutations had higher mutation rate than samples without LRP1B mutation (Figure 1A). The well-known melanoma driver oncogenes (e.g., BRAF, NRAS, NF1, PREX2, ARID2, PPP6C, CCBE1, PTEN), genomic integrity maintenance and DNA replication proofreading associated genes (e.g., TP53, ATR, BRCA1/2, MRE11A) and common LRPs (i.e., LRP1, LRP2, LRP3, LRP4, LRP5, LRP6, LRP8, LRP10, and LRP12) in relation to LRP1B mutation were illustrated in waterfall plot (Figure 1B). Mutations in LRP1B, MRE11A were correlated with improved immune response (Fisher's exact test, P < 0.05; Figure 1B).
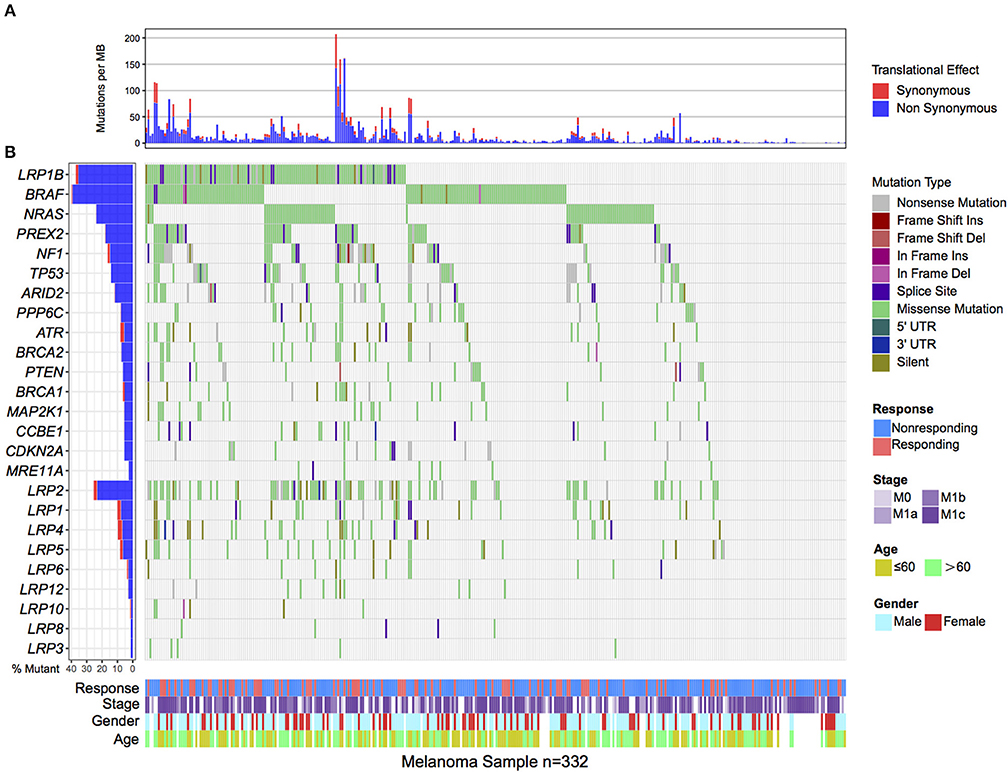
Figure 1. Mutational patterns of recurrently mutated melanoma genes, Genomic Instability associated genes and LRPs gene family in relation to LRP1B mutation in the pooled melanoma patients. (A). Mutation rates per megabase stratified by synonymous and non-synonymous mutations. (B). The left panel is mutation frequency and the middle panel depicts genes mutation patterns across each cases with different mutation types color coded differently. Clinical features of immune response status, age, gender and stage displays in bottom. Immune response related genes were highlighted in bold.
LRP1B Mutation Predictive of Immunotherapy Survival in Melanoma
In Kaplan-Meier survival analysis, the LRP1B mutation was significantly associated with a better immunotherapy survival outcome in the pooled melanoma cohort (log-rank test, P = 0.005; Figure 2A). The association between LRP1B mutation with survival remained statistically significant after taking into account age, gender, TMB status and stage (Cox proportional hazards model, HR, 0.63 [95%CI, 0.40–0.97], P = 0.037; Figure 2B). We also noticed a preferable immune response status in LRP1B mutant samples (Fisher's exact test, P = 0.008).
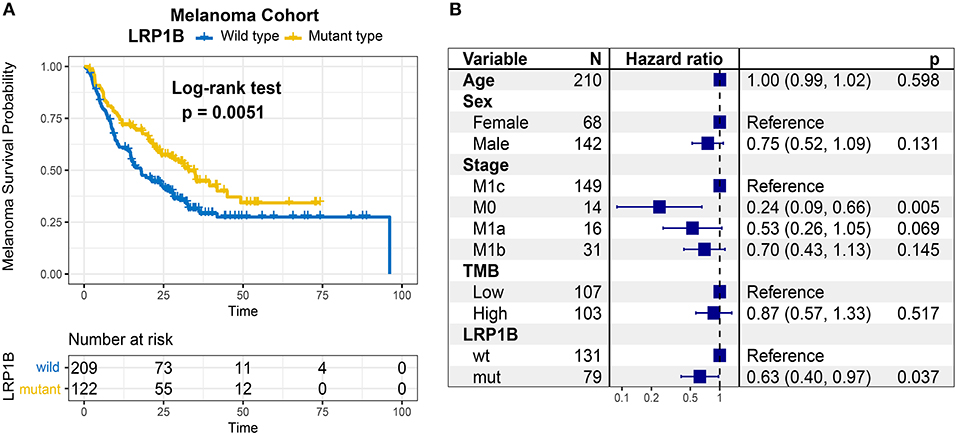
Figure 2. Association of LRP1B Mutation With Prognosis in melanoma immunotherapy Cohort. (A) Kaplan-Meier survival analysis classified by LRP1B mutation status. (B) Multivariate Cox regression analysis of LRP1B mutations with age, gender, TMB status, TNM stage were taken into account.
LRP1B Mutation Associated With TMB
Melanoma samples with LRP1B mutations had a significantly higher tumor mutation burden and neoantigen burden by Wilcoxon rank sum test (log2 TMB, 9.5 vs. 7.3, P < 0.001; log2 NB, 9.2 vs. 6.9, P < 0.001; Figures 3A,B). We also observed the parallel result in TCGA melanoma cohort (log2 TMB, 9.2 vs. 7.7; Wilcoxon rank sum test, P < 0.001; Supplementary Figure 2). Tumor mutation burden is largely attributed to genomic instability, which is prevalent in melanoma. In these samples, we extracted 6 mutational signatures (Supplementary Figures 3A,B), including signatures related to genomic instability. The numbers of somatic mutations attributed to each mutational signature varied considerably in each sample. Underlying associations with these mutational signatures included failure of DNA double-strand break-repair by homologous recombination (signature 3, 8,745 of 207,310, 4.2%), defective DNA mismatch repair (signature 26, 3,050 of 207,310, 1.5%), alkylating agent treatment (signature 11, 30,835 of 207,310, 14.9%) and ultraviolet light exposure (signature 7, 157,250 of 207,310, 75.9%). Samples with signature 7 and signature 11 had greater TMB compared with samples without these features (Supplementary Figure 3C). The mutational activity attributable to each mutational signature in each melanoma sample and variation were shown in Supplementary Table S3.
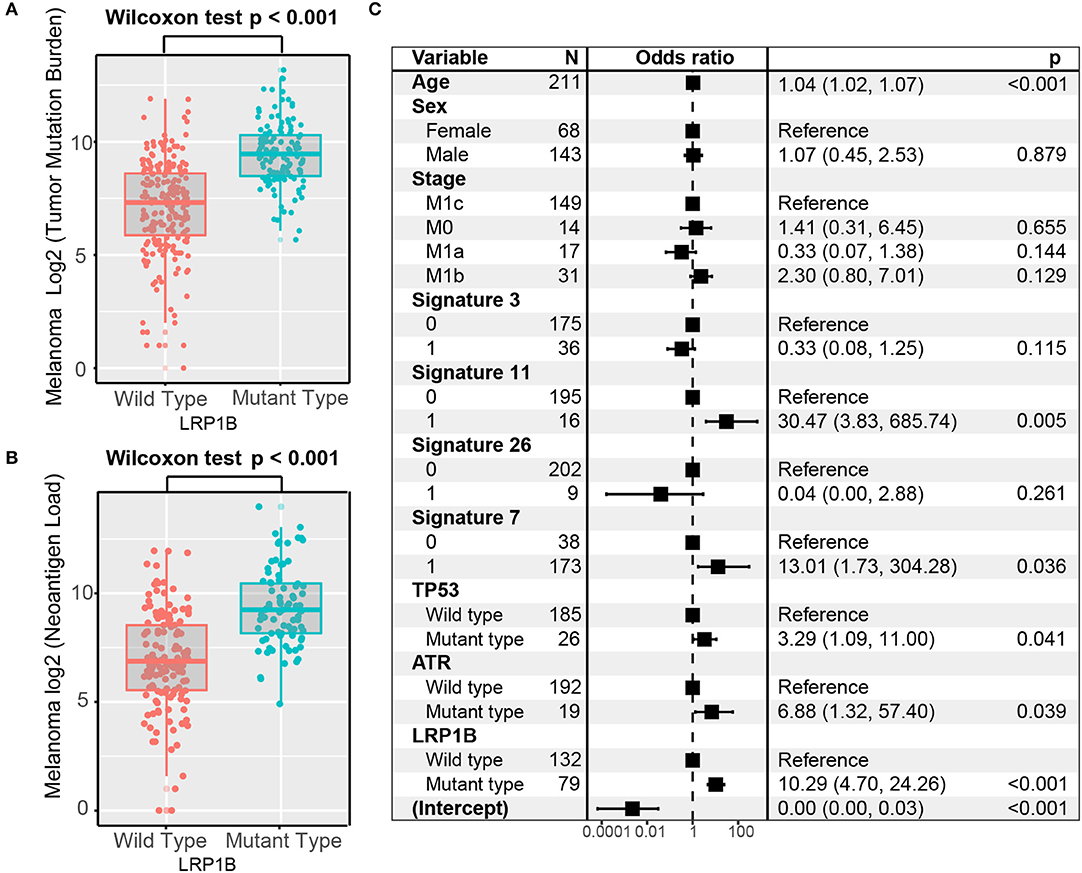
Figure 3. Association of LRP1B Mutation With Higher Tumor Mutation Burden in pooled melanoma cohort. Tumor mutation burden (A) and neoantigen burden (B) of melanoma stratified by LRP1B mutation status. (C) Multivariate Logistic regression analysis of LRP1B mutation with TMB after adjusted for age, sex, TNM stage, mutational signatures, and mutations in TP53 and ATR. Square data markers indicate estimated hazard ratios. Error bars represent 95% CIs.
To rule out the possibility that associations between LRP1B mutations and TMB were affected by these confounding factors, we included mutation associated signatures (3, 7, 11, 26) and mutations in TP53 and ATR in the multivariate model. Associations between LRP1B mutations and TMB remained statistically significant after adjustment (logistic regression model, OR, 10.29; 95% CI, 4.70–24.26, P < 0.001; Figure 3C).
Further Corroboration of LRP1B Mutations in the NSCLC Cohort
As LRP1B mutation was common in lung cancer, we aggregated whole exome sequencing data from three studies of NSCLC immunotherapy to corroborate the results. LRP1B was also frequently mutated (35 of 113 patients [31.0%]) in the pooled NSCLC cohort. The mutation plots of LRP1B in NSCLC of different immune response status are shown in Supplementary Figure 4. Mutation in LRP1B was significantly associated with a better survival prognostic (log-rank test, P = 0.031; Figure 4A) even after taking into account age, gender, smoke, TMB and PD-L1 expression status (Cox proportional hazards model, HR, 0.54 [95%CI, 0.30–0.96], P = 0.035; Figure 4B).
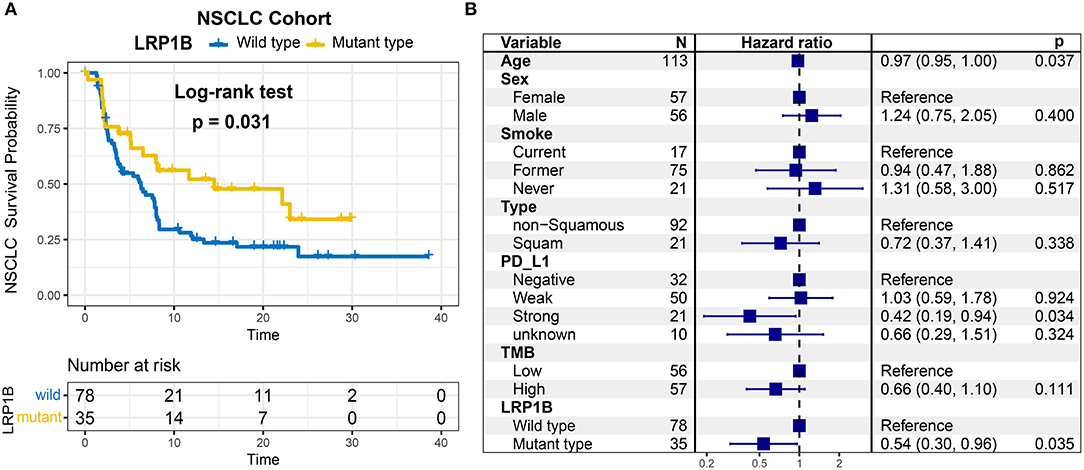
Figure 4. LRP1B mutation is associated with immunotherapy survival outcome of NSCLC patients. (A) Kaplan-Meier survival analysis stratified by LRP1B mutation. (B) Multivariate Cox regression analysis of LRP1B mutation by taking into account confounding factors, such as age, gender, PD-L1 expression, TMB, smoke status, histology type.
The significantly higher tumor mutation burden and neoantigen burden were observed in aggregated NSCLC samples with LRP1B mutations by Wilcoxon rank sum test (log2 TMB, 8.3 vs. 7.1, P < 0.001; log2 NB, 8.7 vs. 7.4, P < 0.001) (Figures 5A,B). The similar results was also observed in TCGA NSCLC cohort (log2 TMB, 8.3 vs. 7.4; Wilcoxon rank sum test, P < 0.001, Supplementary Figure 5). The extracted mutational signatures in NSCLC samples included signature 4 (tobacco smoking), signature 2 (APOBEC family of cytidine deaminases), signature 6 (DNA mismatch repair), signature 7 (UV exposure) and signature 16 (etiology unknown) (Supplementary Figures 6A,B). The mutational activity attributable to each mutational signature in NSCLC sample were shown in Supplementary Table S4. NSCLC samples with signature 2 and signature 4 had greater TMB compared with samples without these features (Supplementary Figure 6C). Associations of LRP1B mutations with the lung cancer driver oncogenes (KRAS, STK11, EGFR, ROS1, ALK, PTEN, CCBE1), maintaining genomic integrity genes (TP53, ATR, POLE), and LRPs gene family are shown in the middle panel of Supplementary Figure 7. The association of LRP1B mutations with higher TMB remained statistically significant after controlling for age, gender, smoke, PD-L1 expression, mutational signatures (etiology known), and mutations in TP53 and ATR in the multivariate model (Logistic regression model, OR, 6.26 [95%CI, 2.05–22.19], P < 0.001; Figure 5C).
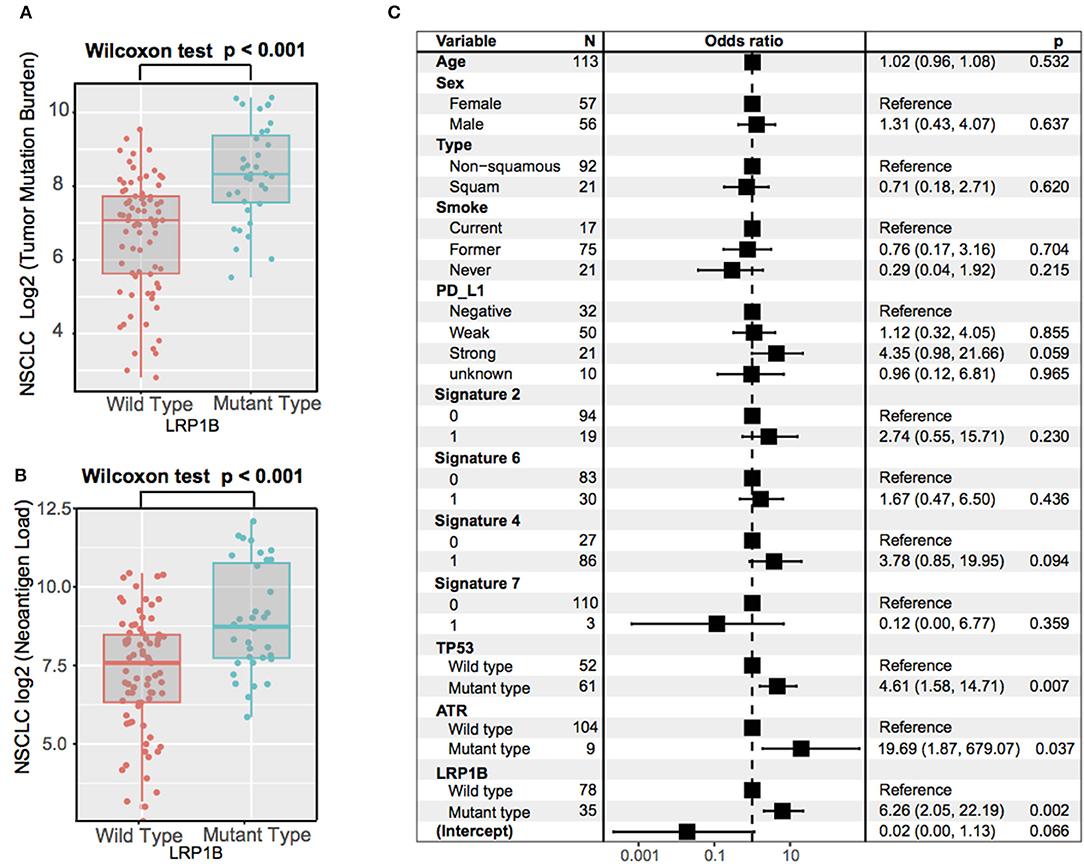
Figure 5. Association of LRP1B Mutation With Higher Tumor Mutation Burden in aggregated NSCLC cohort. Tumor mutation burden (A) and neoantigen burden (B) of NSCLC stratified by LRP1B mutation status. (C) Multivariate Logistic regression analysis of LRP1B mutation with TMB after adjusted for age, sex, PD-L1, smoke status, mutational signatures, and mutations in TP53 and ATR. Square data markers indicate estimated hazard ratios. Error bars represent 95% CIs.
We also analysis the association between binding affinity (IC50) of candidate neoantigen and clinical benefit, and observed a significantly lower value in immune responder group (median binding affinity, melanoma: 149 vs. 174, p < 0.001; NSCLC: 163 vs. 169, p = 0.006). Besides, a decreased predicated binding affinity (IC50) were also detected in LRP1B mutant samples, although these difference was not statistically significant (median binding affinity, melanoma: 166 vs. 170, p = 0.168; NSCLC: 164 vs. 167, p = 0.392). This is also the case in predicated neoantigen peptides which caused by LRP1B mutation (median, melanoma: 181 vs. 199, p = 0.293; NSCLC: 124 vs. 167, p = 0.079).
Significantly Enriched Pathways and Immunocytes Associated With LRP1B Mutations
As LRP1B play an important role in immunotherapy outcomes, we investigate the potential mechanism behind LRP1B mutation and immune response. Gene set enrichment analysis (GSEA) on Reactome gene sets revealed enrichment of genes involved in Cell Cycle Mitotic and Antigen Processing and Presentation pathways were significantly altered in samples with LRP1B mutations (p.adj < 0.001, Figure 6A, Supplementary Figure 6B). Nevertheless, Complement Cascade, Formation of Tubulin Folding were enriched in wild-type group (p.adj < 0.001, Supplementary Figure 8). Besides, T cell-inflamed gene expression profile (GEP) were also analyzed and found a higher scores in LRP1B mutational groups (GEP scores, 1.14 vs. 1.03, Wilcoxon rank sum test, P = 0.019; Figure 6B).
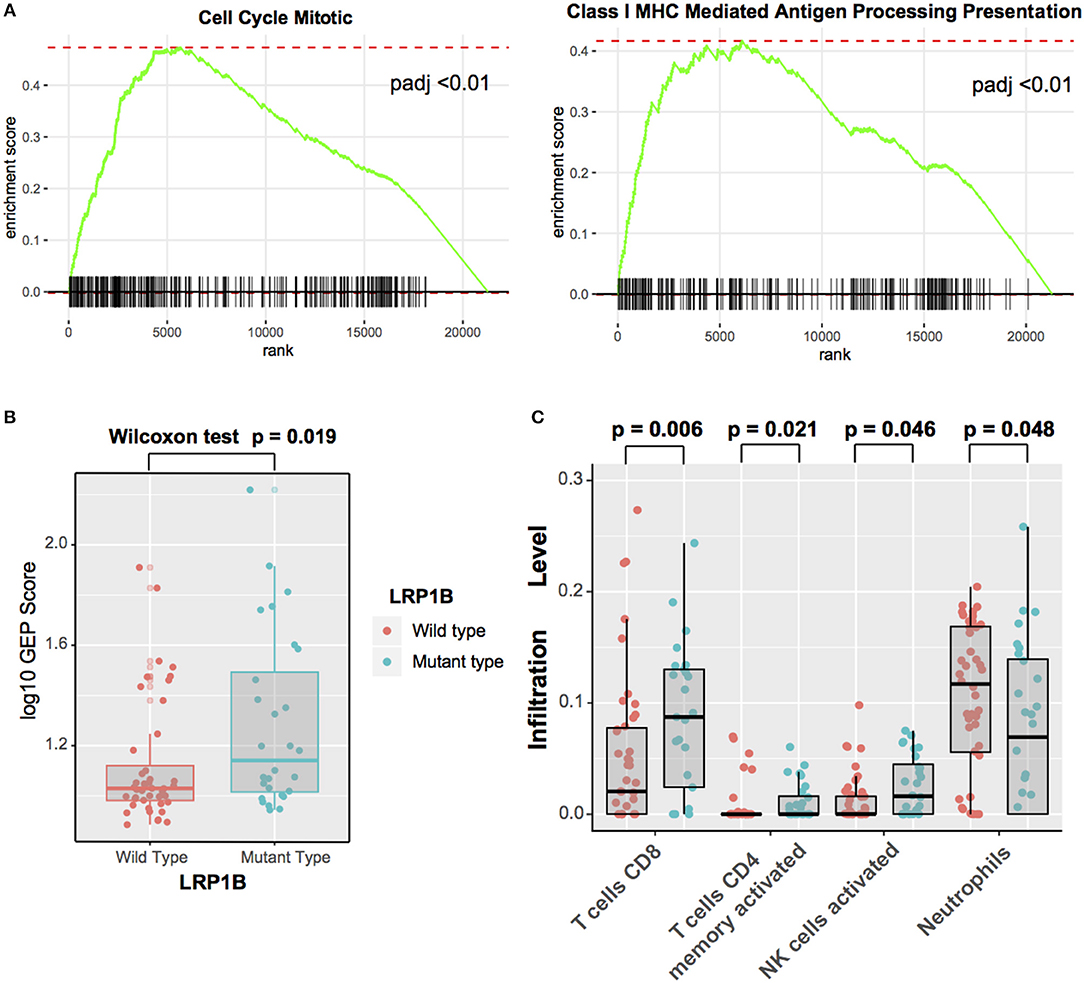
Figure 6. Significantly Enriched Pathways and Immunocytes Associated With LRP1B Mutations (A) Top enriched Reactome pathways in LRP1B mutant group vs. LRP1B wild-type group. (B) T cell-inflamed GEP with LRP1B mutation was assessed in melanoma. (C) Relative abundance of tumor infiltrating leukocytes in LRP1B mutant vs. LRP1B wild-type samples.
Moreover, we evaluated (with use of CIBER algorithm) the abundance of tumor-infiltrating lymphocyte cells in melanoma microenvironment using the gene expression data. We found that CD8+ T cells, activated CD4 memory T cells, activated NK cells, were more enriched in LRP1B mutant type group, nevertheless, Neurtrophils was enriched in wild-type group (Figure 6C).
Discussion
We analyzed LRP1B mutation with immune response and outcome in 332 samples from the melanoma immunotherapy cohort and 113 samples from the NSCLC immunotherapy cohort for further corroboration. LRP1B was frequently mutated in melanoma and NSCLC, and its mutation was associated with higher TMB and better survival outcome. The association of LRP1B mutation with TMB was independent of a significant presence of mutational signatures and of mutations in TP53 and ATR. Samples with LRP1B mutations were characterized by upregulation of signaling pathways involved in immune system, antigen processing and presentation and cell cycle check-points. Tumor-infiltrating immune cells results manifested LRP1B mutated samples were infiltrated in CD8+ T cells, NK cells and activated CD4 memory T cells, which supported the previous observations that such leucocytes and pathways present predominately in the tumor microenvironment of immune responder and promote the immune response (27, 41).
Besides melanoma and non-small cell lung cancer, LRP1B was also frequently mutated in multiple types of human cancer. Several observations favor LRP1B being a bona fide driver mutation in many tumors, including a very high frequency of homozygous deletions (42), and frequent point mutation in esophagus (18), oral (20), liver (43), colon (44), gastric (21), breast (45), thyroid (46), and pancreatic cancer (47). Recently, LRP1B has been implicated in antigen presentation and as a regulator of inflammation and progression in cancer (47, 48). Owing to its large size, LRP1B was often excluded from lists of significantly mutated genes. Another common ignored gene was MUC16, but Li et al. found MUC16 mutation was strongly associated with tumor mutation load and prognostic outcome in gastric cancer patients, and may benefits for patients with immunotherapy (49). The mechanisms underlying the association between TMB and LRP1B is not entirely clear. A leading hypothesis suggests that the large size (coding sequence,16 kbp) and gene location near the FRA2F fragile site (50) may contribute to a higher tumor mutation rate.
The classical role of LRPs is to modulate the clearance of a numerous of extracellular ligands from the pericellular microenvironment (51, 52). In recent years, LRPs emerged as an important regulator of the inflammatory response. LRP1 was supposed to modulate the microglial immune response via regulation of c-Jun N-terminal kinase (JNK) and NF-κB signaling pathways (53). Overexpression of LRP1 is associated with worsened prognosis and suppressive tumor immunity in renal clear-cell carcinoma (54). Deletion of LRP5 and LRP6 in dendritic cells markedly delayed tumor growth and enhanced host antitumor immunity by blocking the Wnt pathway (55). Two SNPs in atopy-related immunologic candidate genes LRP1B were associated with pancreatic cancer risk, even after adjustment for multiple comparisons (47).
Recent studies also revealed LRP1B may as a tumor suppressor in common chemotherpay with deletion mutation of LRP1B causing a chemoresistance and poor outcome (56, 57). In TCGA database, patients with LRP1B mutation have no benefits on conventional chemotherapy in melanoma and NSCLC (Supplementary Figure 9), but displayed preferable clinical outcome in immune check-point blockades therapy, suggested the molecular marker prediction of selectivity and specificity in drug treatment.
The main limitation is using the public dataset from different cohort which are somewhat heterogeneous in data processing and patient population. Although we utilized multiple datasets in different tumor type for analysis, but lack of the independent dataset for validation in the same tumor types. The analyzing tools used in sequencing data may have been different between these studies and may introduce bias in the final mutation lists. Besides, the number of samples with expression data in the cohort was limited, which limits the ability to adjust statistical power. As a result, association data between mutational patterns and gene expression, including analysis in immune cell infiltration and oncogenic pathways need further validation and studies.
Our studies suggested that sequencing even a single, frequently mutated gene may provide insight into genome-wide tumor mutational burden and could be served as a biomarker to predict immune response. The mechanisms through which LRP1B induce high mutations rate in tumors are still unknown and require future investigation. An investigation of a possible role of LRP1B in response to immune check-point blockade treatment in other cancer types (HNSC, ESCC, STAD, etc.) is warranted to study. The full implication of LRP1B in immunotherapy prognostic and monitoring remains elusive and requires in-depth studies.
Data Availability
All relevant data and materials within this work are made available in this manuscript and TCGA databases.
Ethics Statement
All studies have been approved by the Institutional Research Board.
Author Contributions
All authors reviewed the manuscript and agreed to submission. HC, QW, and XW designed the project. HC, QW, MM, WC, and YY performed administrative, technical, or material support. HC and XW performed statistical analysis. HC and WC wrote the manuscript. XW and MM revised the paper.
Funding
This work was supported by grants from the Natural Science Foundation of China (81802508 to MM). Top talent training program of the first affiliated hospital of PLA Army Medical University (SWH2018BJKJ-12 to XW).
Conflict of Interest Statement
The authors declare that the research was conducted in the absence of any commercial or financial relationships that could be construed as a potential conflict of interest.
Acknowledgments
The authors thanks Prof. Xiangchun Li from Tianjin Cancer Institute for protocols and valuable suggestions, and thanks Meng Yang, Qinghua Wang, Luyang Liu, and Chao Sheng for unselfish help and discussions. HC thanks WC for her patience, care and support, and would like spent the rest lives with her.
Supplementary Material
The Supplementary Material for this article can be found online at: https://www.frontiersin.org/articles/10.3389/fimmu.2019.01113/full#supplementary-material
Supplementary Figure 1. Lollipop plot shows the distribution of LRP1B mutations in melanoma responder cohort and non-responder cohort.
Supplementary Figure 2. Tumor mutation burden(TMB) associated with LRP1B mutation status in TCGA skin melanoma database.
Supplementary Figure 3. Mutational signatures extracted from the aggregated melanoma dataset. (A) The mutational activities of corresponding extracted mutational signatures (Signature 1, 3, 7, 11, 18, 26, named as COSMIC signature). (B) The mutational activities of corresponding mutational signatures showed in pie chart. (C) The mutational signature 7 and 11 in melanoma cohort was associated with higher tumor mutation burden.
Supplementary Figure 4. Lollipop plot shows the distribution of LRP1B mutations in non-small cell lung cancer responder and non-responder cohort.
Supplementary Figure 5. Tumor mutation burden(TMB) associated with LRP1B mutation status in TCGA non-small cell lung cancer database.
Supplementary Figure 6. Mutational signatures extracted from the aggregated NSCLC dataset. (A) The mutational activities of corresponding extracted mutational signatures (Signature 2, 4, 6, 7, 16, 26, and unmatched, named as COSMIC signature). (B) The mutational activities of corresponding mutational signatures showed in pie chart. (C) The mutational signature 2 and 4 in NSCLC cohort was associated with higher tumor mutation burden.
Supplementary Figure 7. Mutational patterns of recurrently mutated lung cancer genes, Genomic Instability associated genes and LRPs gene family in relation to LRP1B mutation in the pooled NSCLC patients. (A). Mutation rates per megabase stratified by synonymous and non-synonymous mutations. (B). The left panel is mutation frequency and the middle panel depicts genes mutation patterns across each cases with different mutation types color coded differently. Clinical features of immune response status, age, gender and stage displays in bottom. Immune response related genes were highlighted in bold.
Supplementary Figure 8. GSEA was performed on RNA-seq from pretreatment tumors in the melanoma cohorts by using the hallmark gene sets. Enrichment plots show increased enrichment of the immune-related gene set and decreased expression of Complement Cascade gene set in the LRP1B mutant samples.
Supplementary Figure 9. Kaplan-Meier survival analysis stratified by LRP1B mutation status in melanoma and NSCLC cohort from TCGA database.
Supplementary Table 1. Detailed sequencing information of each cohort.
Supplementary Table 2. Clinical and genome characteristics of each cancer patient.
Supplementary Table 3. Mutational signatures and activities in melanoma patients.
Supplementary Table 4. Mutational signatures and activities in NSCLC patients.
Abbreviations
LRP1B, Low-density lipoprotein receptor-related protein 1B; ICB, immune check-point blockade; CTLA-4, Cytotoxic T-lymphocyte protein 4; PD-1, Programmed cell death protein 1; NSCLC, non-small cell lung cancer; TMB, tumor mutation burden; NB, neoantigene burden; HR, hazards ratio; OR, odds ratio; TCGA, The Cancer Genome Atlas; COSMIC, Catalog of Somatic Mutations in Cancer; GSEA, gene set enrichment analysis; LRPs, Low-density lipoprotein receptor-related protein families; SKCM, cutaneous melanoma; ESCC, esophagus squamous-cell carcinoma.
References
1. Borghaei H, Paz-Ares L, Horn L, Spigel DR, Steins M, Ready NE, et al. Nivolumab versus docetaxel in advanced nonsquamous non-small-cell lung cancer. N Engl J Med. (2015) 373:1627–39. doi: 10.1056/NEJMoa1507643
2. Escudier B, Motzer RJ, Sharma P, Wagstaff J, Plimack ER, Hammers HJ, et al. Treatment beyond progression in patients with advanced renal cell carcinoma treated with nivolumab in checkmate 025. Eur Urol. (2017) 72:368–76. doi: 10.1016/j.eururo.2017.03.037
3. Wolchok JD, Chiarion-Sileni V, Gonzalez R, Rutkowski P, Grob JJ, Cowey CL, et al. Overall survival with combined nivolumab and ipilimumab in advanced melanoma. N Engl J Med. (2017) 377:1345–56. doi: 10.1056/NEJMoa1709684
4. Ferris RL, Blumenschein G Jr, Fayette J, Guigay J, Colevas AD, Licitra L, et al. Nivolumab for recurrent squamous-cell carcinoma of the head and neck. N Engl J Med. (2016) 375:1856–67. doi: 10.1056/NEJMoa1602252
5. Van Allen EM, Miao D, Schilling B, Shukla SA, Blank C, Zimmer L, et al. Genomic correlates of response to CTLA-4 blockade in metastatic melanoma. Science. (2015) 350:207–11. doi: 10.1126/science.aad0095
6. Hugo W, Zaretsky JM, Sun L, Song C, Moreno BH, Hu-Lieskovan S, et al. Genomic and transcriptomic features of response to anti-PD-1 therapy in metastatic melanoma. Cell. (2016) 165:35–44. doi: 10.1016/j.cell.2016.02.065
7. Snyder A, Makarov V, Merghoub T, Yuan J, Zaretsky JM, Desrichard A, et al. Genetic basis for clinical response to CTLA-4 blockade in melanoma. N Engl J Med. (2014) 371:2189–99. doi: 10.1056/NEJMoa1406498
8. Hellmann MD, Nathanson T, Rizvi H, Creelan BC, Sanchez-Vega F, Ahuja A, et al. Genomic features of response to combination immunotherapy in patients with advanced non-small-cell lung cancer. Cancer Cell. (2018) 33:843–52 e4. doi: 10.1016/j.ccell.2018.03.018
9. Rizvi NA, Hellmann MD, Snyder A, Kvistborg P, Makarov V, Havel JJ, et al. Cancer immunology. mutational landscape determines sensitivity to PD-1 blockade in non-small cell lung cancer. Science. (2015) 348:124–8. doi: 10.1126/science.aaa1348
10. Le DT, Durham JN, Smith KN, Wang H, Bartlett BR, Aulakh LK, et al. Mismatch repair deficiency predicts response of solid tumors to PD-1 blockade. Science. (2017) 357:409–13. doi: 10.1126/science.aan6733
11. Stracquadanio G, Wang X, Wallace MD, Grawenda AM, Zhang P, Hewitt J, et al. The importance of p53 pathway genetics in inherited and somatic cancer genomes. Nat Rev Cancer. (2016) 16:251–65. doi: 10.1038/nrc.2016.15
12. Saldivar JC, Cortez D, Cimprich KA. The essential kinase ATR: ensuring faithful duplication of a challenging genome. Nat Rev Mol Cell Biol. (2017) 18:622–36. doi: 10.1038/nrm.2017.67
13. Brown SD, Warren RL, Gibb EA, Martin SD, Spinelli JJ, Nelson BH, et al. Neo-antigens predicted by tumor genome meta-analysis correlate with increased patient survival. Genome Res. (2014) 24:743–50. doi: 10.1101/gr.165985.113
14. Luksza M, Riaz N, Makarov V, Balachandran VP, Hellmann MD, Solovyov A, et al. A neoantigen fitness model predicts tumour response to checkpoint blockade immunotherapy. Nature. (2017) 551:517–20. doi: 10.1038/nature24473
15. Beroukhim R, Mermel CH, Porter D, Wei G, Raychaudhuri S, Donovan J, et al. The landscape of somatic copy-number alteration across human cancers. Nature. (2010) 463:899–905. doi: 10.1038/nature08822
16. Nikolaev SI, Rimoldi D, Iseli C, Valsesia A, Robyr D, Gehrig C, et al. Exome sequencing identifies recurrent somatic MAP2K1 and MAP2K2 mutations in melanoma. Nat Genet. (2011) 44:133–9. doi: 10.1038/ng.1026
17. Ding L, Getz G, Wheeler DA, Mardis ER, McLellan MD, Cibulskis K, et al. Somatic mutations affect key pathways in lung adenocarcinoma. Nature. (2008) 455:1069–75. doi: 10.1038/nature07423
18. Chang J, Tan W, Ling Z, Xi R, Shao M, Chen M, et al. Genomic analysis of oesophageal squamous-cell carcinoma identifies alcohol drinking-related mutation signature and genomic alterations. Nat Commun. (2017) 8:15290. doi: 10.1038/ncomms15290
19. Ali SM, Yao M, Yao J, Wang J, Cheng Y, Schrock AB, et al. Comprehensive genomic profiling of different subtypes of nasopharyngeal carcinoma reveals similarities and differences to guide targeted therapy. Cancer. (2017) 123:3628–37. doi: 10.1002/cncr.30781
20. Nakagawa T, Pimkhaokham A, Suzuki E, Omura K, Inazawa J, Imoto I. Genetic or epigenetic silencing of low density lipoprotein receptor-related protein 1B expression in oral squamous cell carcinoma. Cancer Sci. (2006) 97:1070–4. doi: 10.1111/j.1349-7006.2006.00283.x
21. Takeda H, Rust AG, Ward JM, Yew CC, Jenkins NA, Copeland NG. Sleeping beauty transposon mutagenesis identifies genes that cooperate with mutant Smad4 in gastric cancer development. Proc Natl Acad Sci USA. (2016) 113:E2057–65. doi: 10.1073/pnas.1603223113
22. Johnson DB, Frampton GM, Rioth MJ, Yusko E, Xu Y, Guo X, et al. Targeted next generation sequencing identifies markers of response to PD-1 blockade. Cancer Immunol Res. (2016) 4:959–67. doi: 10.1158/2326-6066.CIR-16-0143
23. Riaz N, Havel JJ, Makarov V, Desrichard A, Urba WJ, Sims JS, et al. Tumor and microenvironment evolution during immunotherapy with nivolumab. Cell. (2017) 171:934–49 e15. doi: 10.1016/j.cell.2017.09.028
24. Wang S, Jia M, He Z, Liu XS. APOBEC3B and APOBEC mutational signature as potential predictive markers for immunotherapy response in non-small cell lung cancer. Oncogene. (2018) 37:3924–36. doi: 10.1038/s41388-018-0245-9
25. Topalian SL, Taube JM, Anders RA, Pardoll DM. Mechanism-driven biomarkers to guide immune checkpoint blockade in cancer therapy. Nat Rev Cancer. (2016) 16:275–87. doi: 10.1038/nrc.2016.36
26. Borst J, Ahrends T, Babala N, Melief CJM, Kastenmuller W. CD4(+) T cell help in cancer immunology and immunotherapy. Nat Rev Immunol. (2018) 18:635–47. doi: 10.1038/s41577-018-0044-0
27. Hsu J, Hodgins JJ, Marathe M, Nicolai CJ, Bourgeois-Daigneault MC, Trevino TN, et al. Contribution of NK cells to immunotherapy mediated by PD-1/PD-L1 blockade. J Clin Invest. (2018) 128:4654–68. doi: 10.1172/JCI99317
28. Benci JL, Xu B, Qiu Y, Wu TJ, Dada H, Twyman-Saint Victor C, et al. Tumor interferon signaling regulates a multigenic resistance program to immune checkpoint blockade. Cell. (2016) 167:1540–54 e12. doi: 10.1016/j.cell.2016.11.022
29. Pang B, Neefjes J. Coupled for cross-presentation in tumor immunotherapy. Sci Transl Med. (2010) 2:44ps40. doi: 10.1126/scitranslmed.3001245
30. Cristescu R, Mogg R, Ayers M, Albright A, Murphy E, Yearley J, et al. Pan-tumor genomic biomarkers for PD-1 checkpoint blockade-based immunotherapy. Science. (2018) 362:6411. doi: 10.1126/science.aar3593
31. Ayers M, Lunceford J, Nebozhyn M, Murphy E, Loboda A, Kaufman DR, et al. IFN-gamma-related mRNA profile predicts clinical response to PD-1 blockade. J Clin Invest. (2017) 127:2930–40. doi: 10.1172/JCI91190
32. Anagnostou V, Smith KN, Forde PM, Niknafs N, Bhattacharya R, White J, et al. Evolution of neoantigen landscape during immune checkpoint blockade in non-small cell lung cancer. Cancer Discov. (2017) 7:264–76. doi: 10.1158/2159-8290.CD-16-0828
33. Ramos AH, Lichtenstein L, Gupta M, Lawrence MS, Pugh TJ, Saksena G, et al. Oncotator: cancer variant annotation tool. Hum Mutat. (2015) 36:E2423–9. doi: 10.1002/humu.22771
34. Roh W, Chen PL, Reuben A, Spencer CN, Prieto PA, Miller JP, et al. Integrated molecular analysis of tumor biopsies on sequential CTLA-4 and PD-1 blockade reveals markers of response and resistance. Sci Transl Med. (2017) 9:eaah3560. doi: 10.1126/scitranslmed.aah3560
35. Kim J, Mouw KW, Polak P, Braunstein LZ, Kamburov A, Kwiatkowski DJ, et al. Somatic ERCC2 mutations are associated with a distinct genomic signature in urothelial tumors. Nat Genet. (2016) 48:600–6. doi: 10.1038/ng.3557
36. Alexandrov LB, Nik-Zainal S, Wedge DC, Campbell PJ, Stratton MR. Deciphering signatures of mutational processes operative in human cancer. Cell Rep. (2013) 3:246–59. doi: 10.1016/j.celrep.2012.12.008
37. Alexandrov LB, Nik-Zainal S, Wedge DC, Aparicio SA, Behjati S, Biankin AV, et al. Signatures of mutational processes in human cancer. Nature. (2013) 500:415–21. doi: 10.1038/nature12477
38. Subramanian A, Tamayo P, Mootha VK, Mukherjee S, Ebert BL, Gillette MA, et al. Gene set enrichment analysis: a knowledge-based approach for interpreting genome-wide expression profiles. Proc Natl Acad Sci USA. (2005) 102:15545–50. doi: 10.1073/pnas.0506580102
39. Newman AM, Liu CL, Green MR, Gentles AJ, Feng W, Xu Y, et al. Robust enumeration of cell subsets from tissue expression profiles. Nat Methods. (2015) 12:453–7. doi: 10.1038/nmeth.3337
40. Love MI, Huber W, Anders S. Moderated estimation of fold change and dispersion for RNA-seq data with DESeq2. Genome Biol. (2014) 15:550. doi: 10.1186/s13059-014-0550-8
41. Daud AI, Loo K, Pauli ML, Sanchez-Rodriguez R, Sandoval PM, Taravati K, et al. Tumor immune profiling predicts response to anti-PD-1 therapy in human melanoma. J Clin Invest. (2016) 126:3447–52. doi: 10.1172/JCI87324
42. Bignell GR, Greenman CD, Davies H, Butler AP, Edkins S, Andrews JM, et al. Signatures of mutation and selection in the cancer genome. Nature. (2010) 463:893–8. doi: 10.1038/nature08768
43. Ding D, Lou X, Hua D, Yu W, Li L, Wang J, et al. Recurrent targeted genes of hepatitis B virus in the liver cancer genomes identified by a next-generation sequencing-based approach. PLoS Genet. (2012) 8:e1003065. doi: 10.1371/journal.pgen.1003065
44. Wang Z, Sun P, Gao C, Chen J, Li J, Chen Z, et al. Down-regulation of LRP1B in colon cancer promoted the growth and migration of cancer cells. Exp Cell Res. (2017) 357:1–8. doi: 10.1016/j.yexcr.2017.04.010
45. Asano Y, Takeuchi T, Okubo H, Saigo C, Kito Y, Iwata Y, et al. Nuclear localization of LDL receptor-related protein 1B in mammary gland carcinogenesis. J Mol Med (Berl). 97:257–68. doi: 10.1007/s00109-018-01732-2
46. Prazeres H, Torres J, Rodrigues F, Pinto M, Pastoriza MC, Gomes D, et al. (2011) Chromosomal, epigenetic and microRNA-mediated inactivation of LRP1B, a modulator of the extracellular environment of thyroid cancer cells. Oncogene. (2019) 30:1302–17. doi: 10.1038/onc.2010.512
47. Cotterchio M, Lowcock E, Bider-Canfield Z, Lemire M, Greenwood C, Gallinger S, et al. Association between variants in atopy-related immunologic candidate genes and pancreatic cancer risk. PLoS ONE. (2015) 10:e0125273. doi: 10.1371/journal.pone.0125273
48. Gonias SL, Campana WM. LDL receptor-related protein-1: a regulator of inflammation in atherosclerosis, cancer, and injury to the nervous system. Am J Pathol. (2014) 184:18–27. doi: 10.1016/j.ajpath.2013.08.029
49. Li X, Pasche B, Zhang W, Chen K. Association of MUC16 mutation with tumor mutation load and outcomes in patients with gastric cancer. JAMA Oncol. (2018) 4:1691–8. doi: 10.1001/jamaoncol.2018.2805
50. Smith DI, Zhu Y, McAvoy S, Kuhn R. Common fragile sites, extremely large genes, neural development and cancer. Cancer Lett. (2006) 232:48–57. doi: 10.1016/j.canlet.2005.06.049
51. Herz J, Strickland DK. LRP: a multifunctional scavenger and signaling receptor. J Clin Invest. (2001) 108:779–84. doi: 10.1172/JCI13992
52. May P, Woldt E, Matz RL, Boucher P. The LDL receptor-related protein (LRP) family: an old family of proteins with new physiological functions. Ann Med. (2007) 39:219–28. doi: 10.1080/07853890701214881
53. Yang L, Liu CC, Zheng H, Kanekiyo T, Atagi Y, Jia L, et al. LRP1 modulates the microglial immune response via regulation of JNK and NF-kappaB signaling pathways. J Neuroinflamm. (2016) 13:304. doi: 10.1186/s12974-016-0772-7
54. Feng C, Ding G, Ding Q, Wen H. Overexpression of low density lipoprotein receptor-related protein 1 (LRP1) is associated with worsened prognosis and decreased cancer immunity in clear-cell renal cell carcinoma. Biochem Biophys Res Commun. (2018) 503:1537–43. doi: 10.1016/j.bbrc.2018.07.076
55. Hong Y, Manoharan I, Suryawanshi A, Shanmugam A, Swafford D, Ahmad S, et al. Deletion of LRP5 and LRP6 in dendritic cells enhances antitumor immunity. Oncoimmunology. (2016) 5:e1115941. doi: 10.1080/2162402X.2015.1115941
56. Tabouret E, Labussiere M, Alentorn A, Schmitt Y, Marie Y, Sanson M. LRP1B deletion is associated with poor outcome for glioblastoma patients. J Neurol Sci. (2015) 358:440–3. doi: 10.1016/j.jns.2015.09.345
Keywords: LRP1B, melanoma, NSCLC, tumor mutation burden, immunotherapy, mutation signatures
Citation: Chen H, Chong W, Wu Q, Yao Y, Mao M and Wang X (2019) Association of LRP1B Mutation With Tumor Mutation Burden and Outcomes in Melanoma and Non-small Cell Lung Cancer Patients Treated With Immune Check-Point Blockades. Front. Immunol. 10:1113. doi: 10.3389/fimmu.2019.01113
Received: 20 February 2019; Accepted: 01 May 2019;
Published: 21 May 2019.
Edited by:
Francesco Acquati, University of Insubria, ItalyReviewed by:
Koichi Takahashi, University of Texas MD Anderson Cancer Center, United StatesManel Juan, Hospital Clínic de Barcelona, Spain
Copyright © 2019 Chen, Chong, Wu, Yao, Mao and Wang. This is an open-access article distributed under the terms of the Creative Commons Attribution License (CC BY). The use, distribution or reproduction in other forums is permitted, provided the original author(s) and the copyright owner(s) are credited and that the original publication in this journal is cited, in accordance with accepted academic practice. No use, distribution or reproduction is permitted which does not comply with these terms.
*Correspondence: Min Mao, mmarine510704@hotmail.com
Xin Wang, wangxinmarine@126.com
†These authors have contributed equally to this work