- 1Department of Neurology, University of California, San Francisco, San Francisco, CA, United States
- 2Division of Biomedical Informatics and Personalized Medicine, Department of Immunology, University of Colorado, Denver, CO, United States
The killer-cell immunoglobulin-like receptor (KIR) region comprises a fast-evolving family of genes that encode receptors for natural killer (NK) cells and have crucial role in host defense. Evolution of KIR was examined in the context of the human genome. Gene-content diversity and single nucleotide polymorphisms (SNP) in the KIR genes and flanking regions were compared to >660,000 genome-wide SNPs in over 800 individuals from 52 populations of the human genome diversity panel (HGDP). KIR allelic diversity was further examined using next generation sequencing in a subset of 56 individuals. We identified the SNP rs587560 located in KIR3DL3 as a marker of KIR2DL2 and KIR2DL3 and, consequently, Cen A and Cen B haplotypes. We also show that combinations of two KIR2DL4 SNPs (rs35656676 and rs592645) distinguish KIR3DL1 from KIR3DS1 and also define the major KIR3DL1 high- and low-expressing alleles lineages. Comparing the diversity of the SNPs within the KIR region to remainder of the genome, we observed a high diversity for the centromeric KIR region consistent with balancing selection (p < 0.01); in contrast, centromeric KIR diversity is significantly reduced in East Asian populations (p < 0.01), indicating purifying selection. By analyzing SNP haplotypes in a region spanning ~500 kb that includes the KIR cluster, we observed evidence of strong positive selection in Africa for high-expressing KIR3DL1 alleles, favored over the low-expressing alleles (p < 0.01). In sharp contrast, the strong positive selection (p < 0.01) that we also observed in the telomeric KIR region in Oceanic populations tracked with a high frequency of KIR3DS1. In addition, we demonstrated that worldwide frequency of high-expression KIR3DL1 alleles was correlated with virus with virus (r = 0.64, p < 10−6) and protozoa (r = 0.69, p < 10−6) loads, which points to selection globally on KIR3DL1 high-expressing alleles attributable to pathogen exposure.
Introduction
Due to their pivotal role in the immune response, much attention has been given recently to variation in the highly polymorphic killer cell immunoglobulin-like receptors (KIR), expressed on the surface of natural killer (NK) (1) cells and a subset of T cells (2). The KIR gene family co-evolves with the genes that encode the human leukocyte antigen (HLA) class I molecules, the ligands for most KIR molecules (3–5). KIR transduce inhibitory and/or activating signals that regulate NK cell activation, and specific KIR and HLA combinations have been associated with numerous diseases, including autoimmunity, cancer and infection (6–10). In addition, KIR-HLA combinations also impact reproduction and placentation (11–14).
The unusual structural polymorphism of the KIR region, yielding variable presence or absence for most of the KIR genes (and thus numerous observed gene-content haplotypes) (15) combined with pronounced allelic variation at each locus- and their demonstrated importance for human survival (16, 17) make them intriguing targets for disease association and evolutionary studies. The 15 KIR loci were formed by multiple duplication events and unequal crossovers (18), evolving relatively rapidly compared to other genomic regions (19–21). As a consequence, KIR genes share substantial sequence similarity with one another, which together with their structural polymorphism, impose technical barriers to their study, particularly at allelic level (22).
KIR gene-content haplotypes are generally described as belonging to two groups, A and B (23, 24), with the A haplotype being relatively conserved in terms of gene-content configuration and represented mostly by inhibitory genes; in contrast, the B group has significant variation in haplotypes that include different combinations of inhibitory and activating KIR. Although a large number of KIR haplotypes have been reported, the most common haplotypes are formed by combinations of four centromeric (CenA, CenB1, CenB2, and CenB3) and two telomeric (TelA and TelB) configurations of KIR genes (25, 26). The framework genes are those that are present in almost all haplotypes and flank the centromeric and telomeric regions of the KIR haplotypes. Flanking the centromeric segment of the region are KIR3DL3 and KIR3DP1, while KIR2DL4 and KIR3DL2 flank the telomeric portion.
Here, we analyzed publicly available data for over 660,000 single nucleotide polymorphisms (SNPs) in the context of KIR diversity in 52 populations from the well-established panel of samples from the Human Genome Diversity Project—Centre d'Etude du Polymorphisme Humain (HGDP-CEPH) (27). The HGDP-CEPH panel is a worldwide collection of population-based samples that have been analyzed with respect to hundreds of thousands of genetic variants, including presence and absence of all KIR genes (26). We observed compelling evidence of selection shaping the diversity of the KIR region in a population-specific manner. In particular, we found strong signals for positive selection in Africans favoring members of the KIR3DL1 allelic lineage that is expressed at highest levels on the surface of NK cells.
Methods
Data Collection
We analyzed publicly available SNP and sequencing data for 817 individuals from 52 populations from the HGDP panel. The first subset of samples was genotyped by Illumina SNP microarray (San Diego, California, USA) and was comprised of 805 individuals from 50 populations (subset 1, Table 1), from which we analyzed a total of 660,918 SNPs extracted from two sources: 143,945 SNPs from the UCLA Medical Center Illumina Immunochip22 HGDP Dataset 15 (ftp://ftp.cephb.fr/hgdp_supp15/) and 516,973 from the Stanford HGDP SNP Genotyping Dataset 2 (http://www.hagsc.org/hgdp/files.html). The other subset (subset 2, Table 1) was comprised of 56 individuals that had been previously sequenced for the whole genome or whole exome (28, 29), in which we applied our custom bioinformatics pipeline (30) to determine KIR allelic genotyping at high-resolution. We analyzed the SNP and sequence data in the context of KIR gene content that was previously genotyped for the HGDP panel by analyzing the amplicons generated by polymerase chain reaction with specific sequence primers (PCR-SSP) using matrix-assisted laser desorption-ionization time-of-flight (MALDI-TOF).
Data Analysis
PLINK version 1.07 (31) was used for all manipulation of SNP data. We only included SNPs whose genotypic distributions did not deviate from Hardy-Weinberg equilibrium (p > 0.01) and with minor allele frequency (MAF) > 0.01. A total of 62 SNPs were extracted from the KIR region (GRCh38.p12, chr19:54727369-54865755). After quality control and merging of the two platforms, 660,918 unique SNPs were available for analysis. To compare the diversity of the SNPs within the KIR region to the distribution of SNPs across the whole genome, we generated distributions for heterozygosity of the SNPs in the KIR and genome-wide regions using the “ecdf” function in the R (32) stats package, and applied the Kolmogorov–Smirnov test using the “ks.test” function, a nonparametric test that quantifies the distance between the empirical distribution functions of two datasets (33), to examine differences in the distributions (34). To examine the association of SNPs with the presence of specific variable KIR loci, we first visualized linkage disequilibrium (LD) between the SNPs and the KIR loci using Haploview (35) to identify SNPs marking loci of interest, and subsequently manually sorted and examined their associations in Microsoft Excel. Similarly, we analyzed whether specific SNPs were associated with KIR alleles and allelic lineages, and manually calculated LD values.
For analysis of the extended haplotype homozygosity (EHH) (36), we analyzed 402 SNPs located within a range of 237,688 bp upstream and 121,621 bp downstream the KIR region, totaling 497,695 bp (GRCh38.p12, chr19:54489681-54987376). We used the R package rehh (37) after phasing the SNPs using the FastPHASE package (38) to generate bifurcation and EHH plots.
To perform correlation analysis between KIR frequencies and pathogen load, we used previously published data quantifying pathogen load in each HGDP population (39) using the “cor” function in the R base package. Labels for populations were randomly permuted 10,000 times and the correlations recalculated to obtain an empirical distribution for the correlation coefficient to obtain p-values.
Results
Diversity of Centromeric and Telomeric KIR Regions Varies Between Geographic Groups
Whereas, KIR diversity has been examined to-date in numerous population studies (40), without the context of genome-wide data it is difficult to disentangle whether observed diversity is a feature of population demographics, or rather indicative of a history of selection on the region. To understand how diversity within the KIR region compares to genome-wide diversity within populations, we examined genotypic data from over 660,000 SNPs in 805 individuals from 50 populations (Table 1) of the human genome diversity panel (HGDP). A total of 62 SNPs were located within the KIR gene cluster, from which 29 were in the centromeric region (KIR3DL3 ~ KIR3DP1) and 33 in the telomeric region (KIR2DL4 ~ KIR3DL2). Populations were grouped according to geographic region for analysis (Table 1). We compared the distribution of heterozygosity (He) of the variants within the KIR telomeric and centromeric regions to that for the variants across the genome in each geographic group. We observed a significantly reduced (p < 0.01) diversity of the centromeric KIR SNPs in comparison to the genome-wide diversity in East Asians, as well as increased centromeric diversity in Oceania (Figure 1A). For the telomeric region, we observed reduced diversity in Africa and Oceania compared to genome-wide (p < 0.01; Figure 1B).
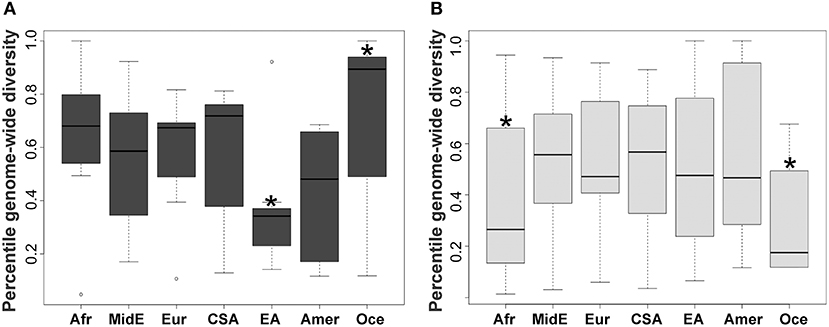
Figure 1. Distinct diversity patterns of centromeric and telomeric KIR regions. (A) Diversity of centromeric KIR SNPs relative to the distribution of genome-wide SNPs. (B) Diversity of telomeric KIR SNPs relative to the distribution of genome-wide SNPs. Horizontal bar represents mean. Asterisks mark statistical significance (p = 0.01) for Kolmogorov–Smirnov test. Afr, Africa; MidE, Middle East; Eur, Europe; CSA, Central and South Asia; EA, East Asia; Amer, America; Oce, Oceania.
KIR3DL3 Intronic Variant rs587560 Distinguishes CenA and CenB Haplotypes and a Pair of KIR2DL4 SNPs Defines the Major KIR3DL1S1 Allelic Lineages
KIR2DL2 and KIR2DL3 had been previously treated as two separate genes but are now known to be major allelic groups of the same locus, with specific haplotypic associations. Because these allele groups have been associated with numerous diseases (41–43) as well as outcome in hematopoietic stem cell transplant (HSCT) (44), there is interest in identifying markers that distinguish them. Synthesizing the SNP data with data for KIR gene-content for the HGDP panel (26), we observed that the allele rs587560C is in strong linkage disequilibrium (LD) with (and essentially marks the presence of) KIR2DL3 (Cen-A haplotypes), while the allele rs587560G is in linkage disequilibrium with KIR2DL2 (Cen-B1, Cen-B2 and Cen-B3 haplotypes; D' = 0.92; Figure 2).
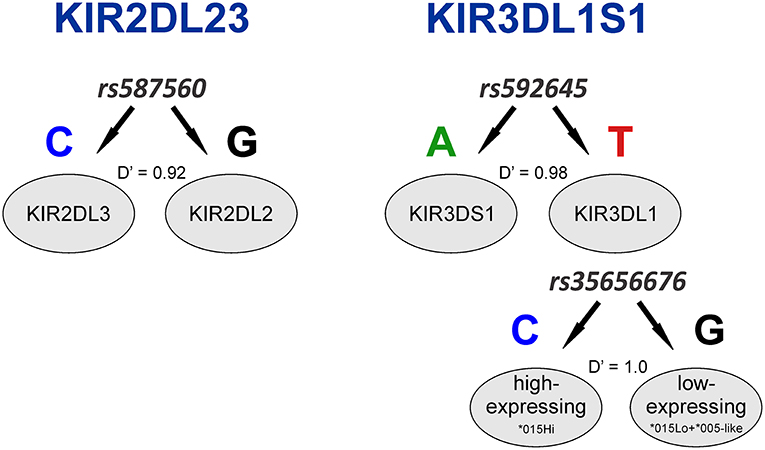
Figure 2. Three SNPs define KIR2DL23 and KIR3DL1S1 allelic lineages. The SNP rs587560 (KIR3DL3 intron 5) marks KIR2DL23 allelic lineages while the pair of SNPs rs592645 (KIR2DL4 intron 4) and rs35656676 (KIR2DL4 5' UTR) defines the major KIR3DL1S1 lineages.
KIR3DL1S1, also formerly described as two genes (KIR3DL1 and KIR3DS1), is possibly the most well-characterized KIR, with almost 200 known alleles (45) that form three major ancient lineages. The KIR3DL1*015 lineage is comprised of the alleles coding inhibitory receptors with the highest cell surface expression, with the exception of the low-expressing *007-like subgroup. These two forms of KIR3DL1*015 are referred here as *015Hi and *015Lo. The KIR3DL1*005 lineage is comprised of alleles encoding low-expressing inhibitory receptors, termed *005-like. Finally, the KIR3DS1 lineage encodes activating receptors (46). We observed that the SNP rs592645 (located in KIR2DL4 intron 4) is a marker for the KIR3DL1 and KIR3DS1 allelic lineages. The variant rs592645A is in strong LD with the presence of KIR3DS1 whereas the allele rs592645T marks KIR3DL1 (D' = 0.98). Using high-resolution allelic genotyping for a subset of individuals (Table 1), we also analyzed the SNP data in the context of KIR3DL1S1 alleles (Supplemental Table 1). We found that the variant rs35656676C, located in KIR2DL4 5' UTR, marks KIR3DL1 high-expressing alleles (*015Hi) and rs35656676G marks KIR3DL1 low-expressing alleles (*005-like and *015Lo) (D' = 1.0; Figure 2).
Strong Positive Selection for KIR3DL1S1 Allelic Lineages in Africa and Oceania
Having identified KIR2DL4 SNPs as markers for KIR3DL1S1 allelic lineages, we sought to examine them in the context of extended haplotypes to detect signatures of selection. We calculated the extended haplotype homozygosity (EHH) across nearly 500 kb flanking the KIR region using rs592645 and rs35656676 as focal SNPs. EHH detects the transmission of an extended haplotype without recombination, examining the probability of two randomly chosen chromosomes carrying a core of alleles in homozygosis (for the interval from the core region to the focal SNP) being identical by descent (36). We identified the ancient and derived alleles from the Database of Single Nucleotide Polymorphisms (dbSNP) (47) and generated bifurcation and EHH plots to visualize the range and frequency of extended haplotypes for each allele. The observed patterns point to a history of strong, recent positive selection favoring the derived alleles rs592645 and rs35656676 (Figure 3). Specifically, the high frequency of a conserved haplotype linked to the derived allele suggests that recent positive selection has acted to increase the frequency of the haplotype on which it originated, more rapidly than it could be broken down by recombination (36).
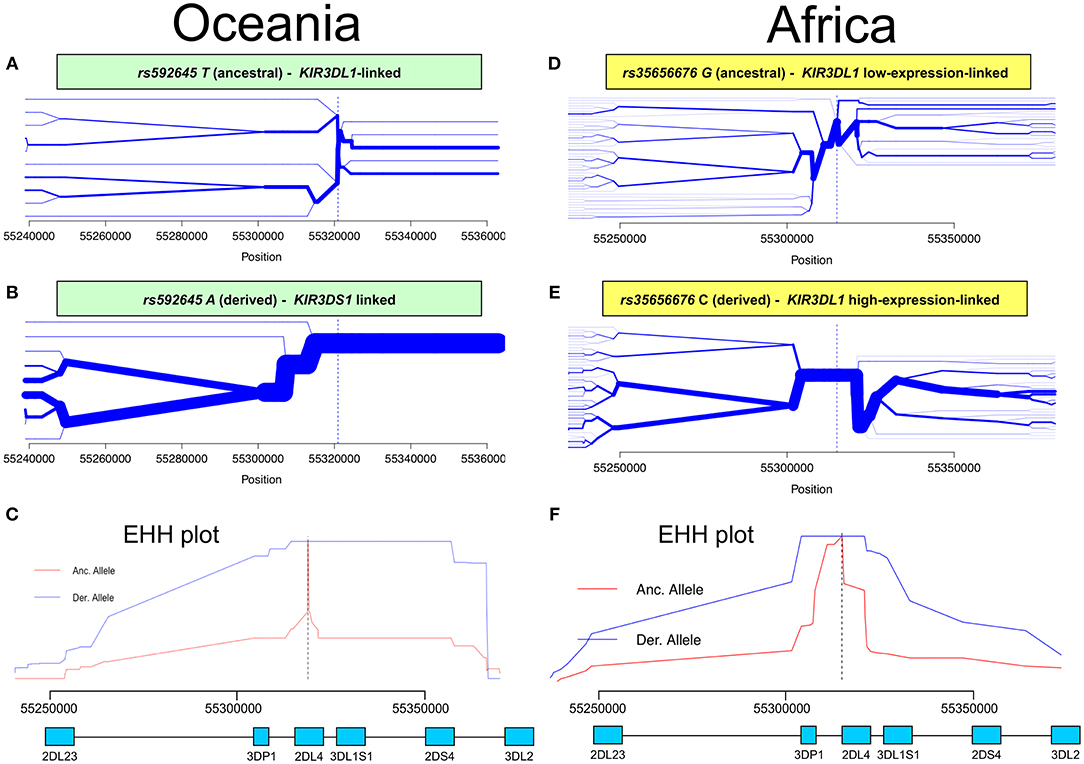
Figure 3. Extended haplotype homozygosity (EHH) analysis points to ongoing positive selection in Oceania and Africa. Haplotype bifurcation diagrams for ancestral (A) and derived (B) alleles of rs592645 showing conserved haplotype associated with the derived allele, but not with the ancestral. The thickness of the line denotes haplotype frequency. (C). EHH plot for rs592645 showing decay of haplotype homozygosity in which the derived allele A is under selection and sweeping to fixation. Haplotype bifurcation diagrams for ancestral (D) and derived (E) alleles of rs35656676 showing conserved haplotype associated with the derived allele, but not with the ancestral. (F) EHH plot for rs35656676 showing decay of haplotype homozygosity in which the derived allele C is under selection and sweeping to fixation.
Worldwide Population Frequencies of KIR3DL1 High-Expressing Alleles Correlate With Pathogen Load
We used the patterns of LD observed for the SNPs linked to KIR3DL1S1 to impute the frequencies of the high- and low-expressing KIR3DL1 lineages and KIR3DS1 in 50 populations from the HGDP for whom SNP data were available (Figure 4). The lowest frequencies of KIR3DS1 were observed in African populations (frequencies ranging from 0 to 0.10) and the highest in Oceanic populations (0.64 to 0.77), similar to what has been previously observed in worldwide populations (48, 49). The high-expressing KIR3DL1 allele lineages were generally observed in higher frequencies in East Asian, Amerindian and African populations. As validation of this approach, the inferred frequencies of KIR3DS1 in our study were compared to the previous frequencies as described in Hollenbach et al. (26), and we observed a strong correlation for these results (r = 0.89, p < 10−7; Supplementary Figure 1). In addition, the relatively high frequencies of KIR3DS1 in Oceanic and Amerindian populations, as well as high frequency of low-expressing alleles in Europeans, are consistent with previous population genetics studies (3, 22, 46, 50, 51).
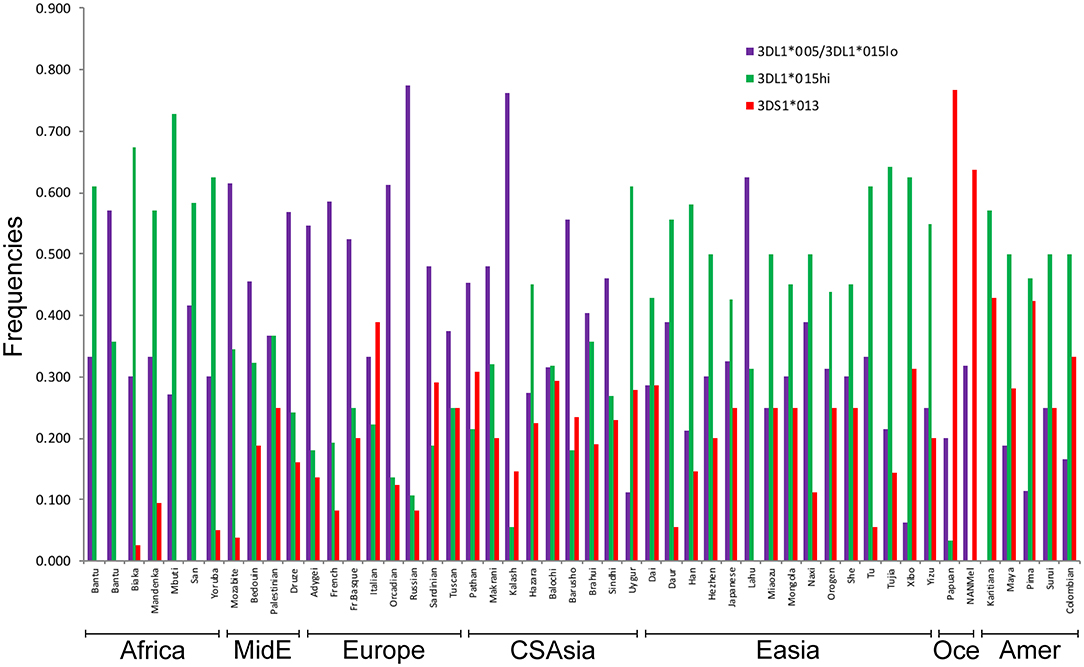
Figure 4. Inferred worldwide frequencies of KIR3DL1S1 allelic lineages. The inference was based on the pair of SNPs rs592645 and rs35656676, which mark the KIR3DS1 (D' = 0.98) and KIR3DL1 high- and low-expressing lineages (D' = 1.0), respectively. MidE, Middle East; CSAsia, Central and South Asia; EAsia, East Asia; Amer, America; Oce, Oceania.
The inferred frequencies of high-expressing KIR3DL1 alleles were then compared to previously published data quantifying pathogen diversity (given as the number of different species for a given pathogen type) in all HGDP populations (39). We found a strong and significant positive correlation between the worldwide frequencies of the high-expressing KIR3DL1 allele lineage with virus (r = 0.64, p < 10−6; Figure 5A) and protozoa (r = 0.69, p < 10−6; Figure 5B) loads.
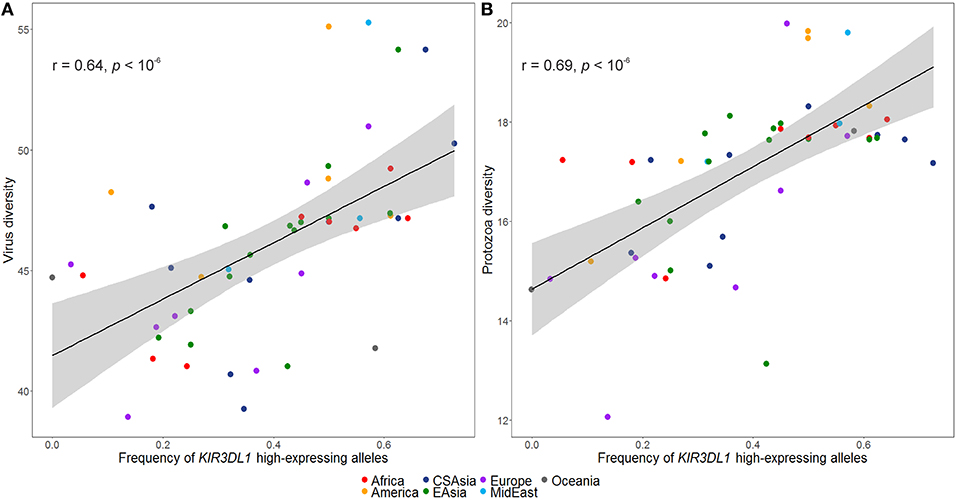
Figure 5. Worldwide frequencies of KIR3DL1 high-expressing alleles correlate with virus and protozoa diversity. (A) Virus load correlates with frequencies of KIR3DL1 high-expressing alleles. (B) Protozoa load correlates with frequencies of KIR3DL1 high-expressing alleles. Each dot represents a population; the shaded area represents the 95% confidence interval for the regression line.
Discussion
The complexity of polymorphism at the KIR cluster has served as a barrier to analysis in the context of whole genome diversity. Here, by leveraging publicly available data for of 650,000 SNPs in one of the most well-characterized sample collections of global populations, we present the first analysis of diversity and patterns of selection in the KIR region in comparison to diversity across the human genome. We merged these data with previously reported KIR gene-content genotyping data and derived high-resolution allelic genotyping for a subset of samples in which whole-exome or -genome sequencing data were available. We found that strong LD in the region allows exploitation of specific SNPs to mark both the presence of KIR haplotypes and allelic lineages of KIR loci and previously reported to be associated with disease and transplant outcome, as well as being under natural selection. For instance, the presence of KIR2DL2 or KIR2DL3 allelic lineages can be determined with 92% of accuracy by simply analyzing the SNP rs587560 in KIR3DL3. By way of comparison, Vukcevic et al. (52) previously reported accuracy of 98% by imputing KIR2DL2 and KIR2DL3 in European populations based on several tag SNPs extracted from the Illumina Immunochip (52). It is important to note, however, that although our sample is small, our results apply to many non-European populations. Although both KIR2DL2 and KIR2DL3 molecules are inhibitory and share 96% of their amino acid sequence, it has been shown that the interaction of KIR2DL2 with HLA-C1 is stronger than the interaction with KIR2DL3 (53), and each have been variably associated with a number of diseases (42, 43). Additionally, KIR2DL2 also marks the presence of Cen B haplotypes, which carry more activating genes than the Cen A haplotypes and have been reported to be favorable for HSCT outcome when present in donors3. We also showed that a pair of KIR2DL4 SNPs, rs592645 and rs35656676, define the three main KIR3DL1S1 allelic lineages. The distinction between KIR3DS1, and KIR3DL1 high- and low-expressing alleles are particularly relevant for NK cell education and disease studies, as it has been previously shown that low expression of KIR3DL1 limits the reactive potential of educated NK cells (54–56). Therefore, it is notable that these functionally relevant SNPs may serve as a proxy for these lineages in studies in which genotyping KIR at high-resolution is not possible.
Consistent with purifying selection, we observed lower levels of diversity in the telomeric KIR region in comparison to the entire genome in African and Oceanic populations relative to genome-wide diversity. In contrast, the diversity of the KIR centromeric region in Oceania was significantly higher in comparison to genome-wide, which could be explained by balancing selection (57). In contrast, and highlighting the considerable fluctuation of selection pressures, East Asian populations exhibit signatures of ongoing purifying selection on the centromeric region, consistent with previous work (58). Lack of signs of selection in Amerindians is in accordance with previous conclusions that demographic factors have generally a stronger effect in shaping KIR polymorphism in these isolated populations than natural selection (51, 59).
As this approach analyzes the variation of a specific genomic region in comparison to the whole genome in the same individuals, the differences that we observed are not likely to be explained by demographic or stochastic factors. Likewise, our results bolster previous findings that showed high diversity and balancing selection shaping the KIR centromeric region in African populations whereas low diversity was observed in the telomeric region in these populations(46).
We applied a robust method to detect signs of positive selection within the KIR telomeric region. The EHH method relies on the relationship between the frequency of an allele and the extent of linkage disequilibrium in neighboring positions. Under the neutral theory of molecular evolution, a novel variant will take a long time to reach high frequency in a population (60). Meanwhile, as a consequence of recombination, the LD between the novel variant and those in the adjacent genomic region decays significantly with time. However, in the case of strong positive selection, the frequency of an allele may increase more rapidly than the haplotype decays under recombination, resulting in large extended haplotypes at high frequencies. The patterns visualized in the bifurcation plots for rs592645 and rs35656676 (Figure 3) are consistent with positive selection on the derived alleles of these SNPs (36). In Oceania, there is evidence for positive selection on rs592645A, which marks the activating KIR3DS1 allelic lineages. It has been suggested that high frequencies of activating KIR are particularly relevant for populations with an extensive history of migration (61). Specifically, the presence of KIR3DS1 has been associated with susceptibility to several diseases (62–67), while the A haplotype (predominantly inhibitory) has been associated with protection against viral infections (68–70). The populations from the Pacific islands suffered historical mass mortality due to infectious diseases between the 16th to 19th centuries. Epidemics of smallpox, bacterial dysenteries and measles devastated the isolated populations from those islands, killing from one quarter to half of the entire population (71–77). A model proposed by Parham and Moffett (78) suggests that when populations are exposed to epidemic infections, there is a positive selection of A haplotypes. However, as the B haplotype (generally more activating than A haplotypes) are associated with successful reproduction, there is selection toward the B haplotype in subsequent generations of those who survive an epidemic. Thus, this model may explain the signature of positive selection in the Oceanic populations, which migrated to Oceania 40–50 thousand years ago, followed by episodes of epidemic infectious diseases and population expansion as well as long-term isolation from the rest of the world (79).
Our analysis was also consistent with patterns of positive selection observed in the KIR telomeric region in Africa. We showed evidence of positive selection toward the high-expressing KIR3DL1 allelic lineage (marked by the allele rs35656676C) in African populations. These results corroborate previous work concluding that exposure to pathogens led to positive selection of more inhibitory forms of KIR3DL1 in Africans (46). Moreover, high-expressing KIR3DL1 alleles have been associated with lower human immunodeficiency virus (HIV) viral load and slower progression to acquired immunodeficiency syndrome (AIDS) (9). In addition, expression levels of KIR3DL1S1 play an important role in NK cell activation against HIV infected cells (54) and are critical in educating NK cells to be primed for attack (55). It is therefore tempting to speculate that extensive exposure to pathogens might be underlying positive selection for high-expressing KIR3DL1 alleles in Africa. Likewise, malaria is a protozoan disease that has strongly impacted human evolution, particularly in Africa (80, 81), and its susceptibility has also been associated with the presence of KIR3DL1 (82, 83). This notion of ongoing pathogen-driven positive selection in Africa is supported by the strong positive correlation that we observed between the frequencies of KIR3DL1 high-expressing alleles with virus and protozoa loads. While the role of NK cells in viral control is well-understood, a possible mechanism underlying the correlation of KIR expression with protozoa load is not immediately clear; however, as with some viral infection, it could be related to immune evasion in protozoans via downregulation of HLA class I (84). At the same time, we also observed a strong correlation between virus and protozoa loads (r = 0.76, p < 10−6). Therefore, an alternative explanation is that selection on KIR is driven by viral diversity, and the association of KIR with protozoa load is simply a consequence of protozoan diversity tracking closely with viral diversity. Despite this limitation in providing mechanistic explanations, our results suggest that KIR3DL1S1 pathogen-driven selection is a global phenomenon.
In conclusion, we observed geographically variable and fluctuating diversity of the centromeric and telomeric KIR regions among populations as well as population-specific signatures of selection. These fluctuations are likely the consequence of rapidly evolving genes that are strongly impacted by local pressures. Our findings point to the continued importance of studying KIR diversity and evolution across worldwide populations to improve our understanding of how this unique and complex system may contribute to human health and survival.
Data Availability
Publicly available datasets were analyzed in this study. This data can be found here: ftp://ftp.cephb.fr/hgdp_supp15.
Author Contributions
DA and JH drafted the manuscript. JH, PN, DA, RD analyzed the data. All authors discussed the results and contributed to the final manuscript.
Funding
This work was funded by NIH grant U19NS095774.
Conflict of Interest Statement
The authors declare that the research was conducted in the absence of any commercial or financial relationships that could be construed as a potential conflict of interest.
Acknowledgments
DA acknowledges the National Council for Scientific and Technological Development (CNPq) in Brazil for their support. Special thanks to Chao Zhao for help with the figures.
Supplementary Material
The Supplementary Material for this article can be found online at: https://www.frontiersin.org/articles/10.3389/fimmu.2019.00989/full#supplementary-material
Supplementary Figure 1. Frequencies of inferred KIR3DS1 frequencies correlated with observed frequencies. Each dot represents a population.
Supplementary Table 1. rs35656676, rs592645 and KIR3DL1S1 genotypes.
References
1. Colonna M, Moretta A, Vély F, Vivier E. A high-resolution view of NK-cell receptors: structure and function. Immunol Today. (2000) 21:428–31.
2. Björkström NK, Béziat V, Cichocki F, Liu LL, Levine J, Larsson S, et al. CD8 T cells express randomly selected KIRs with distinct specificities compared with NK cells. Blood. (2012) 120:3455–65. doi: 10.1182/blood-2012-03-416867
3. Single RM, Martin MP, Gao X, Meyer D, Yeager M, Kidd JR, et al. Global diversity and evidence for coevolution of KIR and HLA. Nat Genet. (2007) 39:1114–19. doi: 10.1038/ng2077
4. Augusto DG, Petzl-Erler ML. KIR and HLA under pressure: evidences of coevolution across worldwide populations. Hum Genet. (2015) 134:929–40. doi: 10.1007/s00439-015-1579-9
5. Norman PJ, Hollenbach JA, Nemat-Gorgani N, Guethlein LA, Hilton HG, Pando MJ, et al. Co-evolution of human leukocyte antigen (HLA) class I ligands with killer-cell immunoglobulin-like receptors (KIR) in a genetically diverse population of sub-Saharan Africans. PLoS Genet. (2013) 9:e1003938. doi: 10.1371/journal.pgen.1003938
6. Augusto DG, Lobo-Alves SC, Melo MF, Pereira NF, Petzl-Erler ML. Activating KIR and HLA Bw4 ligands are associated to decreased susceptibility to pemphigus foliaceus, an autoimmune blistering skin disease. PLoS ONE. (2012) 7:e39991. doi: 10.1371/journal.pone.0039991
7. Kulkarni S, Martin MP, Carrington M. KIR genotyping by multiplex PCR-SSP. Methods Mol Biol. (2010) 612:365–75. doi: 10.1007/978-1-60761-362-6_25
8. Augusto DG. The impact of KIR polymorphism on the risk of developing cancer: not as strong as imagined? Front Genet. (2016) 7:121. doi: 10.3389/fgene.2016.00121
9. Martin MP, Qi Y, Gao X, Yamada E, Martin JN, Pereyra F, et al. Innate partnership of HLA-B and KIR3DL1 subtypes against HIV-1. Nat Genet. (2007) 39:733–40. doi: 10.1038/ng2035
10. Augusto DG, O'Connor GM, Lobo-Alves SC, Bass S, Martin MP, Carrington M, et al. Pemphigus is associated with KIR3DL2 expression levels and provides evidence that KIR3DL2 may bind HLA-A3 and A11 in vivo. Eur J Immunol. (2015) 45:2052–60. doi: 10.1002/eji.201445324
11. Xiong S, Sharkey AM, Kennedy PR, Gardner L, Farrell LE, Chazara O, Bauer J, et al. Maternal uterine NK cell-activating receptor KIR2DS1 enhances placentation. J. Clin. Invest. (2013) 123:4264–72. doi: 10.1172/JCI68991
12. Nakimuli A, Chazara O, Hiby SE, Farrell L, Tukwasibwe S, Jayaraman J, et al. A KIR B centromeric region present in Africans but not Europeans protects pregnant women from pre-eclampsia. Proc Natl Acad Sci USA. (2015) 112:845–50. doi: 10.1073/pnas.1413453112
13. Hiby SE, Walker JJ, O'shaughnessy KM, Redman CWG, Carrington M, Trowsdale J, et al. Combinations of maternal KIR and fetal HLA-C genes influence the risk of preeclampsia and reproductive success. J Exp Med. (2004) 200:957–65. doi: 10.1084/jem.20041214
14. Trowsdale J, Moffett A. NK receptor interactions with MHC class I molecules in pregnancy. Semin Immunol. (2008) 20:317–20. doi: 10.1016/j.smim.2008.06.002
15. Parham P. Immunogenetics of killer cell immunoglobulin-like receptors. Mol Immunol. (2005) 42:459–62. doi: 10.1016/j.molimm.2004.07.027
16. Parham P. MHC class I molecules and KIRs in human history, health and survival. Nat Rev Immunol. (2005) 5:201–14. doi: 10.1038/nri1570
17. Bashirova AA, Thomas R, Carrington M. HLA/KIR restraint of HIV: surviving the fittest. Annu Rev Immunol. (2011) 29:295–317. doi: 10.1146/annurev-immunol-031210-101332
18. Martin MP, Bashirova A, Traherne J, Trowsdale J, Carrington M. Cutting edge: expansion of the KIR locus by unequal crossing over. J Immunol. (2003) 171:2192–5. doi: 10.4049/jimmunol.171.5.2192
19. Khakoo SI, Rajalingam R, Shum BP, Weidenbach K, Flodin L, Muir DG, et al. Rapid evolution of NK cell receptor systems demonstrated by comparison of chimpanzees and humans. Immunity. (2000) 12:687–98. doi: 10.1016/S1074-7613(00)80219-8
20. Hao L, Nei M. Rapid expansion of killer cell immunoglobulin-like receptor genes in primates and their coevolution with MHC Class I genes. Gene. (2005) 347:149–59. doi: 10.1016/j.gene.2004.12.012
21. Vilches C, Parham P. KIR: diverse, rapidly evolving receptors of innate and adaptive immunity. Annu Rev Immunol. (2002) 20:217–51. doi: 10.1146/annurev.immunol.20.092501.134942
22. Misra MK, Augusto DG, Martin GM, Nemat-Gorgani N, Sauter J, Hofmann JA, et al. Report from the Killer-cell Immunoglobulin-like Receptors (KIR) component of the 17th International HLA and Immunogenetics Workshop. Hum Immunol. (2018) 79:825–33. doi: 10.1016/j.humimm.2018.10.003
23. Hsu KC, Liu X-R, Selvakumar A, Mickelson E, O'Reilly RJ, Dupont B. Killer Ig-like receptor haplotype analysis by gene content: evidence for genomic diversity with a minimum of six basic framework haplotypes, each with multiple subsets. J Immunol. (2002) 169:5118–29. doi: 10.4049/jimmunol.169.9.5118
24. Uhrberg M, Parham P, Wernet P. Definition of gene content for nine common group B haplotypes of the Caucasoid population: KIR haplotypes contain between seven and eleven KIR genes. Immunogenetics. (2002) 54:221–9. doi: 10.1007/s00251-002-0463-7
25. Pyo C-W, Guethlein LA, Vu Q, Wang R, Abi-Rached L, Norman PJ, et al. Different patterns of evolution in the centromeric and telomeric regions of group A and B haplotypes of the human killer cell Ig-like receptor locus. PLoS ONE. (2010) 5:e15115. doi: 10.1371/journal.pone.0015115
26. Hollenbach JA, Nocedal I, Ladner MB, Single RM, Trachtenberg EA. Killer cell immunoglobulin-like receptor (KIR) gene content variation in the HGDP-CEPH populations. Immunogenetics. (2012) 64:719–37. doi: 10.1007/s00251-012-0629-x
27. Cann HM, de Toma C, Cazes L, Legrand M-F, Morel V, Piouffre L, et al. A human genome diversity cell line panel. Science. (2002) 296:261–2. doi: 10.1126/science.296.5566.261b
28. Meyer M, Kircher M, Gansauge M-T, Li H, Racimo F, Mallick S, et al. A high-coverage genome sequence from an archaic Denisovan individual. Science. (2012) 338:222–6. doi: 10.1126/science.1224344
29. Henn BM, Botigué LR, Peischl S, Dupanloup I, Lipatov M, Maples BK, et al. Distance from sub-Saharan Africa predicts mutational load in diverse human genomes. Proc Natl Acad Sci USA. (2016) 113:E440–9. doi: 10.1073/pnas.1510805112
30. Norman PJ, Hollenbach JA, Nemat-Gorgani N, Marin WM, Norberg SJ, Ashouri E, et al. Defining KIR and HLA Class I genotypes at highest resolution via high-throughput sequencing. Am J Hum Genet. (2016) 99:375–91. doi: 10.1016/j.ajhg.2016.06.023
31. Purcell S, Neale B, Todd-Brown K, Thomas L, Ferreira MAR, Bender D, et al. PLINK: a tool set for whole-genome association and population-based linkage analyses. Am J Hum Genet. (2007) 81:559–575. doi: 10.1086/519795
32. Bennett MJ, Hugen DL. The R Language for Statistical Computing. In: Financial Analytics with R Building a Laptop Laboratory for Data Science. Cambridge: Cambridge University Press, 7–22.
33. Simard R, L'Ecuyer P. Computing the two-sided kolmogorov-smirnov distribution. J Stat Softw. (2011) 39:1–18. doi: 10.18637/jss.v039.i11
35. Barrett JC, Fry B, Maller J, Daly MJ. Haploview: analysis and visualization of LD and haplotype maps. Bioinformatics. (2005) 21:263–265. doi: 10.1093/bioinformatics/bth457
36. Sabeti PC, Reich DE, Higgins JM, Levine HZP, Richter DJ, Schaffner SF, et al. Detecting recent positive selection in the human genome from haplotype structure. Nature. (2002) 419:832–7. doi: 10.1038/nature01140
37. Gautier M, Vitalis R. rehh: an R package to detect footprints of selection in genome-wide SNP data from haplotype structure. Bioinformatics. (2012) 28:1176–77. doi: 10.1093/bioinformatics/bts115
38. Scheet P, Stephens M. A fast and flexible statistical model for large-scale population genotype data: applications to inferring missing genotypes and haplotypic phase. Am J Hum Genet. (2006) 78:629–44. doi: 10.1086/502802
39. Fumagalli M, Sironi M, Pozzoli U, Ferrer-Admetlla A, Ferrer-Admettla A, Pattini L, et al. Signatures of environmental genetic adaptation pinpoint pathogens as the main selective pressure through human evolution. PLoS Genet. (2011) 7:e1002355. doi: 10.1371/journal.pgen.1002355
40. Gonzalez-Galarza FF, Takeshita LYC, Santos EJM, Kempson F, Maia MHT, da Silva ALS, et al. Allele frequency net 2015 update: new features for HLA epitopes, KIR and disease and HLA adverse drug reaction associations. Nucleic Acids Res. (2015) 43:D784–8. doi: 10.1093/nar/gku1166
41. Hollenbach JA, Ladner MB, Saeteurn K, Taylor KD, Mei L, Haritunians T, et al. Susceptibility to Crohn's disease is mediated by KIR2DL2/KIR2DL3 heterozygosity and the HLA-C ligand. Immunogenetics. (2009) 61:663–671. doi: 10.1007/s00251-009-0396-5
42. Kulkarni S, Martin MP, Carrington M. The yin and yang of HLA and KIR in human disease. Semin Immunol. (2008) 20:343–52. doi: 10.1016/j.smim.2008.06.003
43. Khakoo SI, Carrington M. KIR and disease: a model system or system of models? Immunol Rev. (2006) 214:186–201. doi: 10.1111/j.1600-065X.2006.00459.x
44. Cooley S, Trachtenberg E, Bergemann TL, Saeteurn K, Klein J, Le CT, et al. Donors with group B KIR haplotypes improve relapse-free survival after unrelated hematopoietic cell transplantation for acute myelogenous leukemia. Blood. (2009) 113:726–32. doi: 10.1182/blood-2008-07-171926
45. Robinson J, Mistry K, McWilliam H, Lopez R, Marsh SGE. IPD–the Immuno polymorphism database. Nucleic Acids Res. (2010) 38:D863–9. doi: 10.1093/nar/gkp879
46. Norman PJ, Abi-Rached L, Gendzekhadze K, Korbel D, Gleimer M, Rowley D, et al. Unusual selection on the KIR3DL1/S1 natural killer cell receptor in Africans. Nat Genet. (2007) 39:1092–1099. doi: 10.1038/ng2111
47. Sherry ST, Ward MH, Kholodov M, Baker J, Phan L, Smigielski EM, et al. dbSNP: the NCBI database of genetic variation. Nucleic Acids Res. (2001) 29:308–311. doi: 10.1093/nar/29.1.308
48. Hollenbach JA, Augusto DG, Alaez C, Bubnova L, Fae I, Fischer G, et al. 16(th) IHIW: population global distribution of killer immunoglobulin-like receptor (KIR) and ligands. Int J Immunogenet. (2013) 40:39–45. doi: 10.1111/iji.12028
49. Hollenbach JA, Meenagh A, Sleator C, Alaez C, Bengoche M, Canossi A, et al. Report From the Killer Immunoglobulin-Like Receptor (KIR) Anthropology Component of the 15th International Histocompatibility Workshop: Worldwide Variation In the KIR Loci and Further Evidence For The Co-Evolution of KIR and HLA. John Wiley & Sons, Ltd, 9–17.(2010)
50. Velickovic M, Velickovic Z, Panigoro R, Dunckley H. Diversity of killer cell immunoglobulin-like receptor genes in Indonesian populations of Sumatra, Sulawesi and Moluccas Islands. Tissue Antigen. (2010) 76:325–30. doi: 10.1111/j.1399-0039.2010.01525.x
51. Augusto DG, Piovezan BZ, Tsuneto LT, Callegari-Jacques SM, Petzl-Erler ML. KIR gene content in amerindians indicates influence of demographic factors. PLoS ONE. (2013) 8:e56755. doi: 10.1371/journal.pone.0056755
52. Vukcevic D, Traherne JA, Næss S, Ellinghaus E, Kamatani Y, Dilthey A, et al. Imputation of KIR Types from SNP Variation Data. Am J Hum Genet. (2015) 97:593–607. doi: 10.1016/j.ajhg.2015.09.005
53. Moesta AK, Norman PJ, Yawata M, Yawata N, Gleimer M, Parham P. Synergistic polymorphism at two positions distal to the ligand-binding site makes KIR2DL2 a stronger receptor for HLA-C than KIR2DL3. J. Immunol. (2008) 180:3969–3979. doi: 10.4049/jimmunol.180.6.3969
54. Boudreau JE, Mulrooney TJ, Le Luduec J-B, Barker E, Hsu KC. KIR3DL1 and HLA-B Density and binding calibrate nk education and response to HIV. J Immunol. (2016) 196:3398–3410. doi: 10.4049/jimmunol.1502469
55. Saunders PM, Pymm P, Pietra G, Hughes VA, Hitchen C, O'Connor GM, et al. Killer cell immunoglobulin-like receptor 3DL1 polymorphism defines distinct hierarchies of HLA class I recognition. J Exp Med. (2016) 213:791–807. doi: 10.1084/jem.20152023
56. Boulet S, Kleyman M, Kim JY, Kamya P, Sharafi S, Simic N, et al. A combined genotype of KIR3DL1 high expressing alleles and HLA-B*57 is associated with a reduced risk of HIV infection. AIDS. (2008) 22:1487–1491. doi: 10.1097/QAD.0b013e3282ffde7e
57. Charlesworth D. Balancing selection and its effects on sequences in nearby genome regions. PLoS Genet. (2006) 2:e64. doi: 10.1371/journal.pgen.0020064
58. Yawata M, Yawata N, Draghi M, Little A-M, Partheniou F, Parham P. Roles for HLA and KIR polymorphisms in natural killer cell repertoire selection and modulation of effector function. J Exp Med. (2006) 203:633–45. doi: 10.1084/jem.20051884
59. Augusto DG, Hollenbach JA, Petzl-Erler ML. A deep look at KIR-HLA in Amerindians: Comprehensive meta-analysis reveals limited diversity of KIR haplotypes. Human Immunol. (2015) 76:272–80. doi: 10.1016/j.humimm.2015.01.025
61. Rajalingam R, Pavithra-Arulvani R, Du Z, Vidhyalakshmi A, Middleton D, Reed EF, et al. Distinct diversity of KIR genes in three southern Indian populations: comparison with world populations revealed a link between KIR gene content and pre-historic human migrations. Immunogenetics. (2008) 60:207–217. doi: 10.1007/s00251-008-0286-2
62. Martin MP, Gao X, Lee J-H, Nelson GW, Detels R, Goedert JJ, et al. Epistatic interaction between KIR3DS1 and HLA-B delays the progression to AIDS. Nat Genet. (2002) 31:429–434. doi: 10.1038/ng934
63. Qi Y, Martin MP, Gao X, Jacobson L, Goedert JJ, Buchbinder S, et al. KIR/HLA pleiotropism: protection against both HIV and opportunistic infections. PLoS Pathog. (2006) 2:e79. doi: 10.1371/journal.ppat.0020079
64. Boulet S, Sharafi S, Simic N, Bruneau J, Routy J-P, Tsoukas CM, et al. Increased proportion of KIR3DS1 homozygotes in HIV-exposed uninfected individuals. AIDS. (2008) 22:595–599. doi: 10.1097/QAD.0b013e3282f56b23
65. Zhi-ming L, Yu-lian J, Zhao-lei F, Chun-xiao W, Zhen-fang D, Bing-chang Z, et al. Polymorphisms of killer cell immunoglobulin-like receptor gene: possible association with susceptibility to or clearance of hepatitis B virus infection in Chinese Han population. Croat Med J. (2007) 48:800–6. doi: 10.3325/cmj.2007.6.800
66. Paladino N, Flores AC, Marcos CY, Fainboim H, Theiler G, Arruvito L, et al. Increased frequencies of activating natural killer receptors are associated with liver injury in individuals who do not eliminate hepatitis C virus. Tissue Antigen. (2007) 69 (Suppl) 1:109–111. doi: 10.1111/j.1399-0039.2006.762_7.x
67. Shahsavar F, Mousavi T, Azargon A, Entezami K. Association of KIR3DS1+HLA-B Bw4Ile80 combination with susceptibility to tuberculosis in Lur population of Iran. Iran J Immunol. (2012) 9:39–47.
68. Wauquier N, Padilla C, Becquart P, Leroy E, Vieillard V. Association of KIR2DS1 and KIR2DS3 with fatal outcome in Ebola virus infection. Immunogenetics. (2010) 62:767–771. doi: 10.1007/s00251-010-0480-x
69. Khakoo SI, Thio CL, Martin MP, Brooks CR, Gao X, Astemborski J, et al. HLA and NK cell inhibitory receptor genes in resolving hepatitis C virus infection. Science. (2004) 305:872–874. doi: 10.1126/science.1097670
70. Dring MM, Morrison MH, McSharry BP, Guinan KJ, Hagan R, Irish HCV, Research Consortium, et al. Innate immune genes synergize to predict increased risk of chronic disease in hepatitis C virus infection. Proc Natl Acad Sci USA. (2011) 108:5736–5741. doi: 10.1073/pnas.1016358108
71. Shanks GD, Lee S-E, Howard A, Brundage JF. Extreme mortality after first introduction of measles virus to the polynesian island of Rotuma, 1911. Am J Epidemiol. (2011) 173:1211–1222. doi: 10.1093/aje/kwq504
72. Schlomowitz R. Mortality and the pacific labour trade. J Pac Hist. (1987) 22:34–55. doi: 10.1080/00223348708572550
73. Shanks GD. Lethality of first contact dysentery epidemics on Pacific Islands. Am J Trop Med Hyg. (2016) 95:273–277. doi: 10.4269/ajtmh.16-0169
75. Epidemic dysentery in the fiji islands. Epidemic dysentery in the Fiji Islands. Lancet. (1912) 179:1420–21. doi: 10.1016/S0140-6736(01)68040-1
76. Adels BR, Gajdusek DC. Survey of measles patters in New Guinea, Micronesia and Australia. With a report of new virgin soil epidemics and the demonstration of susceptible primitive populations by serology. Am J Hyg. (1963) 77:317–43.
77. Penman BS, Gupta S, Shanks GD. Rapid mortality transition of Pacific Islands in the 19th century. Epidemiol Infect. (2017) 145:1–11. doi: 10.1017/S0950268816001989
78. Parham P, Moffett A. Variable NK cell receptors and their MHC class I ligands in immunity, reproduction and human evolution. Nat Rev Immunol. (2013) 13:133–144. doi: 10.1038/nri3370
79. Kayser M. The human genetic history of Oceania: near and remote views of dispersal. Curr Biol. (2010) 20:R194–201. doi: 10.1016/j.cub.2009.12.004
80. Barreiro LB, Quintana-Murci L. From evolutionary genetics to human immunology: how selection shapes host defence genes. Nat Rev Genet. (2010) 11:17–30. doi: 10.1038/nrg2698
81. Kwiatkowski DP. How malaria has affected the human genome and what human genetics can teach us about malaria. Am J Hum Genet. (2005) 77:171–192. doi: 10.1086/432519
82. Lourembam SD, Sawian CE, Baruah S. Differential association of KIR gene loci to risk of malaria in ethnic groups of Assam, Northeast India. Infect Genet Evol. (2011) 11:1921–1928. doi: 10.1016/j.meegid.2011.08.017
83. Taniguchi M, Kawabata M. KIR3DL1/S1 genotypes and KIR2DS4 allelic variants in the AB KIR genotypes are associated with Plasmodium-positive individuals in malaria infection. Immunogenetics. (2009) 61:717–730. doi: 10.1007/s00251-009-0401-z
Keywords: killer cell immunoglobulin-like receptors, evolution, human populations, diversity, pathogens, imputation
Citation: Augusto DG, Norman PJ, Dandekar R and Hollenbach JA (2019) Fluctuating and Geographically Specific Selection Characterize Rapid Evolution of the Human KIR Region. Front. Immunol. 10:989. doi: 10.3389/fimmu.2019.00989
Received: 15 February 2019; Accepted: 16 April 2019;
Published: 17 May 2019.
Edited by:
Lutz Walter, Deutsches Primatenzentrum, GermanyReviewed by:
Salim Iqbal Khakoo, University of Southampton, United KingdomMarkus Uhrberg, Heinrich Heine Universität Düsseldorf, Germany
Copyright © 2019 Augusto, Norman, Dandekar and Hollenbach. This is an open-access article distributed under the terms of the Creative Commons Attribution License (CC BY). The use, distribution or reproduction in other forums is permitted, provided the original author(s) and the copyright owner(s) are credited and that the original publication in this journal is cited, in accordance with accepted academic practice. No use, distribution or reproduction is permitted which does not comply with these terms.
*Correspondence: Jill A. Hollenbach, amlsbC5ob2xsZW5iYWNoQHVjc2YuZWR1