- 1Perinatology Research Branch, Eunice Kennedy Shriver National Institute of Child Health and Human Development, National Institutes of Health, United States Department of Health and Human Services, Bethesda, MD, United States
- 2Perinatology Research Branch, Eunice Kennedy Shriver National Institute of Child Health and Human Development, National Institutes of Health, United States Department of Health and Human Services, Detroit, MI, United States
- 3Department of Obstetrics and Gynecology, Wayne State University School of Medicine, Detroit, MI, United States
- 4Systems Biology of Reproduction Lendulet Research Group, Institute of Enzymology, Research Centre for Natural Sciences, Hungarian Academy of Sciences, Budapest, Hungary
- 5Maternity Private Department, Kutvolgyi Clinical Block, Semmelweis University, Budapest, Hungary
- 6First Department of Pathology and Experimental Cancer Research, Semmelweis University, Budapest, Hungary
- 7Department of Obstetrics and Gynecology, University of Michigan, Ann Arbor, MI, United States
- 8Department of Epidemiology and Biostatistics, Michigan State University, East Lansing, MI, United States
- 9Center for Molecular Medicine and Genetics, Wayne State University, Detroit, MI, United States
- 10Department of Computer Science, College of Engineering, Wayne State University, Detroit, MI, United States
- 11Laboratory of Proteomics, Department of Physiology and Neurobiology, ELTE Eotvos Lorand University, Budapest, Hungary
- 12Channing Division of Network Medicine, Brigham and Women’s Hospital, Harvard University, Boston, MA, United States
- 13Zymo Research Corporation, Irvine, CA, United States
- 14Department of Obstetrics and Gynaecology, University of Debrecen, Debrecen, Hungary
- 15Institute of Biochemistry, Biological Research Centre, Hungarian Academy of Sciences, Szeged, Hungary
- 16Department of Genetics, Cell and Immunobiology, Semmelweis University, Budapest, Hungary
- 17Department of Morphology and Physiology, Semmelweis University, Budapest, Hungary
- 18TeleMarpe Ltd, Tel Aviv, Israel
- 19Department of Clinical and Translational Science, Wayne State University, Detroit, MI, United States
- 20Department of Physiology, Wayne State University School of Medicine, Detroit, MI, United States
- 21Department of Obstetrics and Gynecology, Medical University of Vienna, Vienna, Austria
- 22Department of Obstetrics and Gynecology, Soroka University Medical Center School of Medicine, Faculty of Health Sciences, Ben-Gurion University of the Negev, Beer Sheva, Israel
- 23Centre for Trophoblast Research, Department of Physiology, Development and Neuroscience, University of Cambridge, Cambridge, United Kingdom
- 24Department of Pathology, Wayne State University School of Medicine, Detroit, MI, United States
- 25Department of Pathology, Asan Medical Center, University of Ulsan, Seoul, South Korea
Preeclampsia is a disease of the mother, fetus, and placenta, and the gaps in our understanding of the complex interactions among their respective disease pathways preclude successful treatment and prevention. The placenta has a key role in the pathogenesis of the terminal pathway characterized by exaggerated maternal systemic inflammation, generalized endothelial damage, hypertension, and proteinuria. This sine qua non of preeclampsia may be triggered by distinct underlying mechanisms that occur at early stages of pregnancy and induce different phenotypes. To gain insights into these molecular pathways, we employed a systems biology approach and integrated different “omics,” clinical, placental, and functional data from patients with distinct phenotypes of preeclampsia. First trimester maternal blood proteomics uncovered an altered abundance of proteins of the renin-angiotensin and immune systems, complement, and coagulation cascades in patients with term or preterm preeclampsia. Moreover, first trimester maternal blood from preterm preeclamptic patients in vitro dysregulated trophoblastic gene expression. Placental transcriptomics of women with preterm preeclampsia identified distinct gene modules associated with maternal or fetal disease. Placental “virtual” liquid biopsy showed that the dysregulation of these disease gene modules originates during the first trimester. In vitro experiments on hub transcription factors of these gene modules demonstrated that DNA hypermethylation in the regulatory region of ZNF554 leads to gene down-regulation and impaired trophoblast invasion, while BCL6 and ARNT2 up-regulation sensitizes the trophoblast to ischemia, hallmarks of preterm preeclampsia. In summary, our data suggest that there are distinct maternal and placental disease pathways, and their interaction influences the clinical presentation of preeclampsia. The activation of maternal disease pathways can be detected in all phenotypes of preeclampsia earlier and upstream of placental dysfunction, not only downstream as described before, and distinct placental disease pathways are superimposed on these maternal pathways. This is a paradigm shift, which, in agreement with epidemiological studies, warrants for the central pathologic role of preexisting maternal diseases or perturbed maternal–fetal–placental immune interactions in preeclampsia. The description of these novel pathways in the “molecular phase” of preeclampsia and the identification of their hub molecules may enable timely molecular characterization of patients with distinct preeclampsia phenotypes.
Introduction
Preeclampsia, one of the most severe obstetrical complications affecting 5–8% of pregnant women (1–5), is a leading cause of maternal (4–15) and perinatal morbidity and mortality (6, 16–18). In addition, pathologic changes in the affected mothers and fetuses lead to a higher risk of subsequent metabolic and cardiovascular diseases later in life (8, 9, 11, 13, 19–23), further increasing healthcare costs. In spite of the severity of the problem, there is yet no early diagnosis of all forms of preeclampsia, and the current therapy is still based on the delivery of the placenta (2, 24), given the complexity of the disease and the lack of insight into the early perturbed molecular pathways.
Indeed, preeclampsia is a syndrome with heterogeneous etiology and a spectrum of phenotypes (Figure 1). It may affect women at varying gestational ages with different degrees of severity and consequences for the fetus (2, 25–31). The current classifications of preeclampsia are based upon its severity and the timing of clinical presentation, mostly dividing preeclampsia into preterm (<37 weeks) or term (≥37 weeks) and early-onset (<34 weeks) or late-onset (≥34 weeks) phenotypes (24, 26, 32–36). Preterm preeclampsia has a more severe clinical presentation and is often accompanied by fetal growth restriction compared to term preeclampsia (2, 25, 26, 29). However, severe maternal disease and fetal growth restriction may be observed in both term and preterm preeclampsia, and their presentation may not be associated with each other, suggesting that the clinical phenotype is the result of an interplay between various factors and disease pathways, also supported by observations in animal models (37).
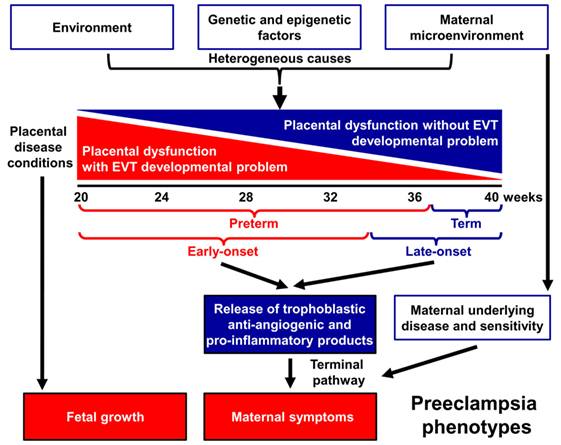
Figure 1. Pathogenesis of preeclampsia. Preeclampsia is a syndrome with heterogeneous etiology and a spectrum of phenotypes. It may appear at varying gestational ages with different degrees of severity and involvement of the fetus. Preterm, especially early-onset preeclampsia generally has a more severe clinical presentation in the mother and is more often associated with the delivery of a growth-restricted neonate than term or late-onset preeclampsia. It is a multi-stage disease with the maldevelopment and/or dysfunction of distinct trophoblast lineages of the placenta at the center of the disease. Villous and extravillous trophoblast (EVT) development and/or function may be impaired in the preclinical stage, most extensively in preterm preeclampsia associated with fetal growth restriction. The resulting abnormal maternal spiral artery remodeling, fluctuating blood-flow, and ischemic stress lead to placental histological changes and the release of harmful substances from the placenta. As a consequence, the terminal pathway of preeclampsia, an exaggerated maternal systemic inflammatory and anti-angiogenic condition, occurs. The frequency and severity of placental developmental problems continuously decrease with advancing gestational age. In term forms, other stressors than maternal vascular malperfusion and placental ischemia may trigger placental stress, trophoblastic dysfunction, and the induction of the terminal pathway. Alternatively, the maternal endothelium may have an exaggerated sensitivity to factors released from a relatively normal placenta.
A growing body of evidence offers support for the conclusion that the maldevelopment and/or dysfunction of distinct trophoblast lineages of the placenta have a central role in the pathogenesis of preeclampsia, and that the severity of the placental disease is subsequently reflected in the clinical phenotype of this syndrome. In the preclinical stage, extravillous trophoblast (EVT) development may be impaired, leading to EVT dysfunction, shallow trophoblast invasion, failure of the physiological transformation of the maternal spiral arteries, abnormal blood-flow to the placenta, and histological changes consistent with maternal vascular malperfusion (28, 30, 38–43). The frequency and severity of these lesions decrease from the preterm toward the term phenotype of preeclampsia (28, 33, 44–46) (Figure 1), mirrored by the decreasing prevalence of fetal growth restriction or the delivery of small-for-gestational age (SGA) neonates (47–53). Thus, EVT development is less frequently and extensively affected in late-onset preeclampsia, which constitutes about 90% of all cases (50, 54, 55).
In preterm preeclampsia, failure of the remodeling of the spiral arteries may lead to abnormal blood flow to the placenta and subsequently to placental structural damage and ischemic stress, villous trophoblast (VT) dysfunction and the release of detrimental placental substances (e.g. anti-angiogenic factors, pro-inflammatory cytokines, and syncytiotrophoblast debris) into the maternal circulation (40, 41, 43, 56–66). As a consequence, the terminal pathway of preeclampsia, featuring an anti-angiogenic state and exaggerated maternal systemic inflammation, occurs in most cases, and its intensity correlates with the severity of preeclampsia, which may be coupled with damage to the maternal endothelium and to the kidneys, liver, and central nervous system during the clinical phase (21, 42, 43, 64, 67–72). VT development can also be impaired in preeclampsia (73–75), especially in the preterm form associated with SGA, where VT turnover is affected together with morphometric features (73, 76).
Term preeclampsia is characterized by a lesser magnitude of maternal systemic inflammatory and anti-angiogenic states (30, 37, 55–57, 61, 62, 77–92). This phenotype may result from different stressors other than maternal vascular malperfusion and ischemia of the placenta, which include various preexisting maternal disorders, such as obesity, chronic hypertension, diabetes, and metabolic, kidney, and autoimmune diseases (25, 93, 94). These stressors may still trigger placental stress and VT dysfunction (31, 91) and induce a maternal pro-inflammatory milieu. Alternatively, maternal endothelial dysfunction may result from an exaggerated sensitivity to factors released from the placenta (21, 25, 31, 95), which increases the risk of preeclampsia upon maternal genetic predisposition for cardiovascular disease (96, 97). Indeed, preeclampsia has a genetic predisposition with high heritability of both phenotypes, and it shares common risk alleles with coronary artery disease (98–104).
In spite of extensive research efforts, our understanding of the early pathologic pathways of preeclampsia has been limited given several obstacles. First, the complexity of the disease pathways and the heterogeneity of the syndrome have not been investigated in an integrative manner in both maternal and placental compartments throughout pregnancy. Second, it has been impossible to investigate the early placental disease pathways because of the invasive nature of placental biopsy and the limited information on placental functions obtained non-invasively. Consequently, an increasing number of high-dimensional studies aiming to detect molecular signatures of preeclampsia either in the placenta or in maternal blood have mostly targeted later stages of pregnancy, at a more advanced stage of placental development and pathology (105–143). Third, animal models of preeclampsia fail to mimic early placental pathways of preeclampsia due to the anatomical and physiological uniqueness of deep placentation in humans (144–147). Fourth, in vitro studies on human placental development and trophoblast functions are hindered by the lack of self-replicating trophoblast stem cells with the ability to differentiate into both VTs and EVTs (148–150).
Here, we used a systems biology approach that integrated various omics and targeted methods to investigate both placental and maternal compartments and most aspects of preeclampsia, including placental disease; fetal and maternal outcomes; environmental, maternal, microenvironmental, and epigenetic factors; and trophoblastic functions. In the first placental study, we performed extensive investigations of the placenta at histologic, transcriptomic, epigenetic, and protein levels to target molecular pathways at the center of the disease. Molecular changes were correlated with maternal and neonatal morbidities associated with preeclampsia to uncover placental pathways affecting either maternal or fetal wellbeing. In the second, maternal study, we employed maternal blood proteomics and “virtual” liquid biopsy of the placenta to reveal blood factors of maternal or placental origin that can reflect disease conditions in early pregnancy. In the third, trophoblast study, we utilized in vitro functional assays on the trophoblast to investigate hub transcription factors at the center of placental disease gene modules and to model their in vivo involvement in placental pathways associated with maternal or placental/fetal disease (Figure 2).
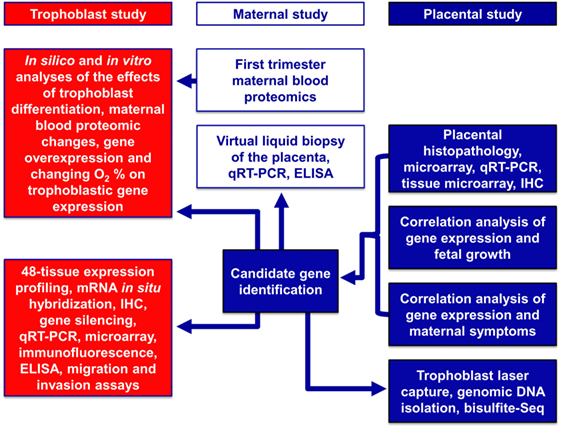
Figure 2. Flow-chart of experimental procedures. The placental study included extensive histologic, transcriptomic, epigenetic, and protein level investigations of the placenta to target the molecular pathways in the center of disease. Molecular changes were correlated with disease outcomes in both mothers and babies to uncover placental pathways affecting either maternal or fetal wellbeing. The maternal study included first trimester maternal blood proteomics and “virtual” liquid biopsy of the placenta to reveal blood factors of maternal or placental origin that can reflect disease conditions in early pregnancy. In the trophoblast study, hub transcription factors in placental gene modules separately associated with maternal or placental/fetal disease were investigated with various in vitro methods to model in vivo disease pathways.
Results
Placental Study
Alterations in the Placental Transcriptome in Preterm Preeclampsia
Given that the pathogenesis of preeclampsia has been implicated to originate from the placenta, we first aimed to investigate placental transcriptomic changes leading to placental dysfunction as well as regulatory networks involved in the pathologic pathways. The combined analysis of preterm preeclampsia cases and gestational age-matched controls (n = 17) in a Hungarian patient population (Table 1) in our placental microarray data (132) revealed 1,409 differentially expressed (DE) genes (Data S1 in Supplementary Material), which are involved in fundamental cellular processes, including blood pressure (BP) regulation, apoptosis, development, hormone secretion, metabolism, homeostasis, and signaling (Figure 3A). DE genes included 137 transcription regulatory genes and 38 predominantly placenta-expressed genes. This latter set of genes (n = 164, Data S2 in Supplementary Material), which was defined by BioGPS microarray data (n = 153) or expression data from separate studies in the lack of BioGPS data (n = 11) (151–153), was enriched among DE genes in preeclampsia [odds ratio (OR) = 3.4, p = 6.9 × 10−9]. This suggests that genes predominantly expressed by the placenta have pathologic and diagnostic significance in preeclampsia, a phenomenon indicated earlier by our targeted studies (153–155).
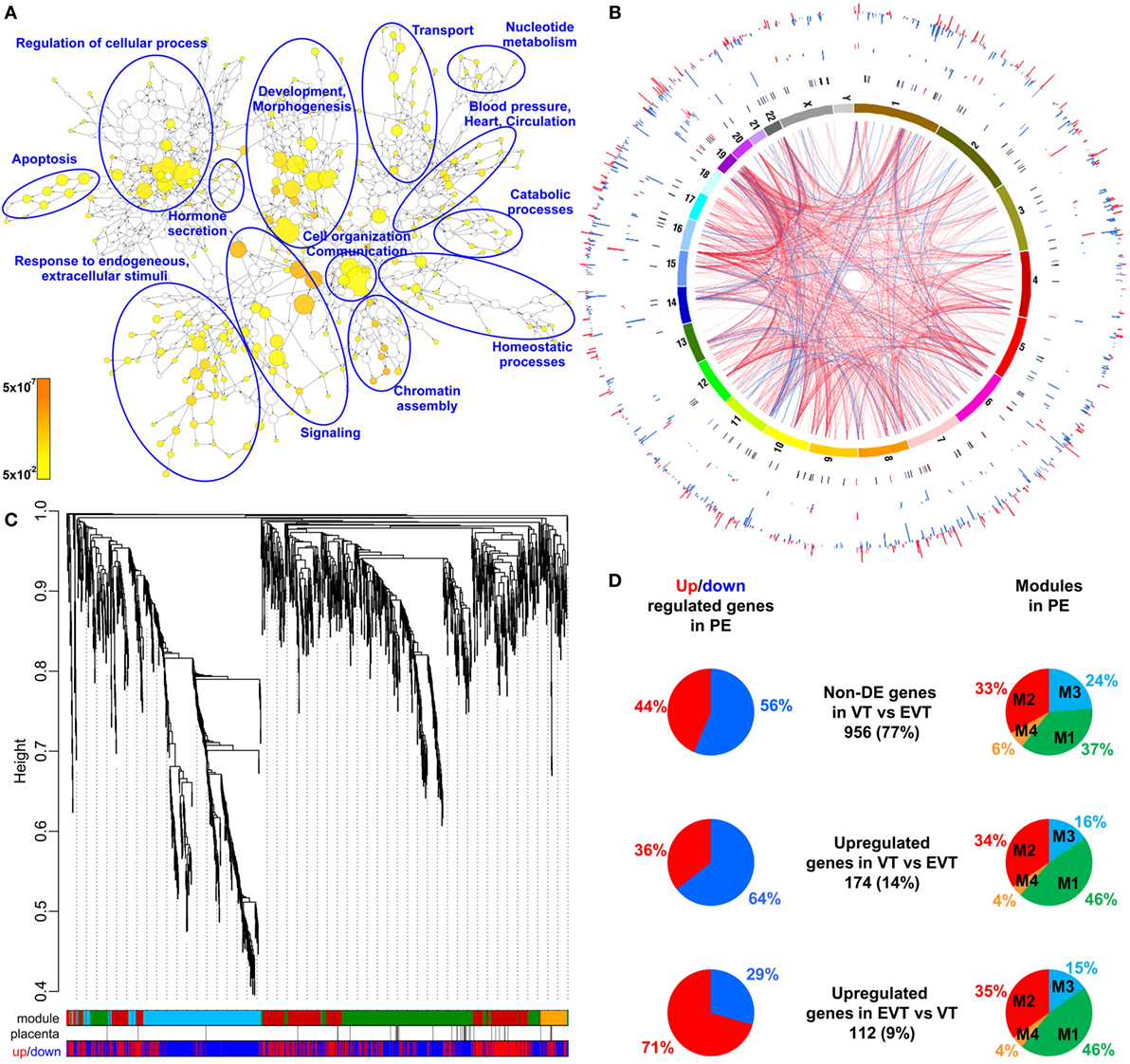
Figure 3. Placental transcriptomic changes in preterm preeclampsia. (A) The network of biological processes enriched among differentially expressed (DE) genes. Circle sizes relate to the number of genes involved in the biological processes; colors refer to p-values according to the color code. Groups of the most enriched biological processes were circled and labeled. (B) Circos visualization of DE genes in preterm preeclampsia (PE). The inner circle shows chromosomes; curved lines represent correlation (red: positive; blue: negative) between transcription regulatory genes and their targets; the second circle shows genomic location of predominantly placenta-expressed genes (black lines: non-DE; red or blue lines: up- or down-regulated); third and fourth circles show the locations of DE transcription regulatory genes and their targets, respectively with blue (down-regulated) and red (up-regulated) bars. The height of the bars represents the magnitude of expression changes. (C) Dysregulated placental gene expression was characterized by four major disease gene modules within DE genes, marked with different colors. The height plotted on the y-axis represents distance metric. Of 38 predominantly placenta-expressed genes (black vertical lines), 33 belonged to the M1 (green, n = 22) and M2 (red, n = 11) modules. These modules were enriched in predominantly placenta-expressed genes and enriched in up-regulated (M2) or down-regulated (M1) genes marked under the modules with red or blue lines, respectively. (D) Pie charts depict the distribution of three gene sets among dysregulated genes or gene modules. Genes with similar expression in villous (VT) and extravillous trophoblast (EVT) had similar distribution among up- or down-regulated genes and in modules M1 and M2. Genes with up-regulation in VT compared to EVT were predominantly down-regulated in preeclampsia and positioned in module M1. Genes with up-regulation in EVT compared to VT were predominantly up-regulated in preeclampsia and positioned also in module M1.
Subsequently, we investigated the genomic links among DE genes by searching for genomic regions associated with the observed placental transcriptomic changes. We found that Chr6 (OR = 1.54, q = 1.6 × 10−3) and Chr7 (OR = 1.42, q = 0.02) were particularly affected by these gene expression changes (Data S3 in Supplementary Material), while Chr19 (OR = 2.6, q = 0.02) was enriched in dysregulated transcription regulatory genes (Data S4 in Supplementary Material). Of interest, predominantly placenta-expressed genes were also enriched on Chr19 (OR = 2.5, q = 0.002) (Data S5 in Supplementary Material). Figure 3B shows the non-random genomic localization of DE genes in preterm preeclampsia and the pronounced gene dysregulation associated with Chr19. These results are consistent with the fact that Chr19 harbors large transcription regulatory gene families (156), and probably reflect its regulatory role in placental/trophoblastic gene expression and their dysregulation in preeclampsia.
Alterations in Biological Processes and Regulatory Networks in Preterm Preeclampsia
Next, we aimed to identify functional links among DE genes by identifying gene co-expression network modules and hub transcription regulatory genes driving differential expression in the modules. Weighted co-expression network analysis (WGCNA) was conducted among DE genes resulting in the assignment of these into four major modules, labeled as M1 (green, n = 506), M2 (red, n = 442), M3 (blue, n = 381), and M4 (orange, n = 74) (Figure 3C; Data S1 in Supplementary Material). Most predominantly placenta-expressed genes belonged to modules M1 (n = 22 genes) and M2 (n = 12). Module M1 was enriched in down-regulated genes (OR = 1.88, p = 2.59 × 10−8), while module M2 was enriched in up-regulated genes (OR = 6.47, p = 2.2 × 10−16), suggesting the presence of distinct dysregulated gene-networks. Genes with predominant VT expression (14%) were mainly down-regulated, while genes with predominant EVT expression (9%) were mainly up-regulated even though both sets had the most members in module M1 (Figure 3D). These data suggested that the functions of both VT and EVT are strongly impacted in preterm preeclampsia, albeit in different ways.
Predominantly placenta-expressed genes, down-regulated in module M1, are regulators of fetal growth (CSH1, HSD11B2) (157, 158), metabolism (ESRRG) (159), estrogen synthesis (HSD17B1) (160), and immune functions (LGALS14) (153), some of which were reported to be down-regulated in preeclampsia (30, 91, 130, 155, 161, 162). Within this module, ESRRG, POU5F1, and ZNF554 transcription regulatory genes had the highest number of significant correlations with predominantly placenta-expressed genes and, hence, deemed as hub factors (Figure S1A in Supplementary Material). Of note, these transcription factors have been implicated in the regulation of stemness and differentiation (163, 164), pointing to the possible involvement of module M1 in the dysregulation of trophoblast differentiation in preterm preeclampsia. We selected ZNF554 for functional studies, since it belongs to the KRAB zinc finger family, crucial for early embryonic development and differentiation (165), and it may regulate genes in its co-expression network involved in biological processes affected by preeclampsia, such as development, chromatin assembly, signaling, adhesion, migration, and metabolism (Figure S1B in Supplementary Material).
In module M2, FLT1, which expresses sFlt-1, the main driver of BP elevation in the terminal pathway of preeclampsia (56, 90), was up-regulated. Moreover, module M2 genes were strongly overrepresented (OR = 29.9, p = 6.54 × 10−95) among genes that had correlated expression with mean arterial pressure (MAP) (Data S6 in Supplementary Material), suggesting a key role for this module in promoting hypertension. Within this module, BCL6, BHLHE40, and ARNT2 had the highest correlation in gene expression with predominantly placenta-expressed genes, including FLT1 (Figure S1A in Supplementary Material). Of note, these transcription regulatory hub genes are involved in hypoxia response. ARNT2 heterodimerizes with hypoxia-response regulator HIF-1α that is involved in trophoblast invasion and the pathogenesis of preeclampsia (166–169), and ARNT2 is a key regulator for adaption to hypoxic conditions at high altitudes, where the incidence of preeclampsia is much higher (16%) than at low altitude (170, 171). BHLHE40 links immune and hypoxia-induced pathways (172). BCL6, a gene previously found to be up-regulated in the placenta in preterm preeclampsia, represses trophoblast differentiation and is regulated by stress-activated protein kinase signaling pathways (135, 173, 174). Our co-expression analysis revealed an enrichment of biological processes, such as differentiation, apoptosis, metabolism, signaling, and responses to stimuli including oxygen among genes co-expressed with BCL6 (Figure S1C in Supplementary Material). Since BCL6 is a key player in inflammation and oxygen-driven regulation of cell fate, we selected this gene for functional studies along with ARNT2.
The Association of Gene Modules With Clinical Parameters
Next, to validate the microarray results on a larger patient population (n = 100) comprised mostly of subjects of African-American origin presenting various phenotypes of preeclampsia (Table 2; Figure S2 in Supplementary Material), we selected 47 genes for qRT-PCR profiling of 100 placentas (Table 3), provided these genes were: (1) dysregulated genes with predominant placental and syncytiotrophoblastic expression, potentially encoding biomarkers; (2) dysregulated hub transcription regulatory genes that had a high co-expression with dysregulated, predominantly placenta-expressed genes in modules M1 and M2; and (3) non-dysregulated genes with roles in trophoblast differentiation, trophoblast-specific gene expression, or the pathogenesis of preeclampsia.
As depicted in the heatmap representing qRT-PCR data for the 100 placentas and 47 genes (Figure 4A), validation data supported microarray experiments in terms of (1) differential expression of selected genes, (2) high correlation of genes within modules M1 and M2, and (3) separate dysregulation of modules M1 and M2 from each other. Comparison between preterm preeclampsia and gestational age-matched controls revealed that qRT-PCR data validated microarray results (differential or non-differential gene expression) for 33 of 47 genes (70%), and further confirmed the differential expression of four genes that were non-dysregulated in the microarray cohort (Table 3). As expected, the extent of changes in gene expression was less pronounced in term preeclampsia (Table 3; Figure 4C; Figure S3 in Supplementary Material). As a further confirmation, there was a strong correlation between qRT-PCR data as well as tissue microarray (TMA) and immunostaining results for selected proteins in module M2 (Figures 4C–E). The extent of dysregulation was larger in preterm phenotypes of preeclampsia than in term phenotypes, in agreement with the more severe placental pathology (44). Among transcription regulatory genes of module M1, HLF had strong down-regulation only in preterm preeclampsia while ZNF554 was expressed at a lower level in all preeclampsia phenotypes (Figure S3 in Supplementary Material). Among the transcription regulatory genes in module M2, ARNT2, BCL6, and JUNB were highly expressed in preterm but not in term preeclampsia, suggesting that these might play a role only in the pathology of preterm cases (Figure 4C; Figure S3 in Supplementary Material). Overall, these data reflect the heterogeneous placental pathology and the more severely affected pathways in preterm preeclampsia.
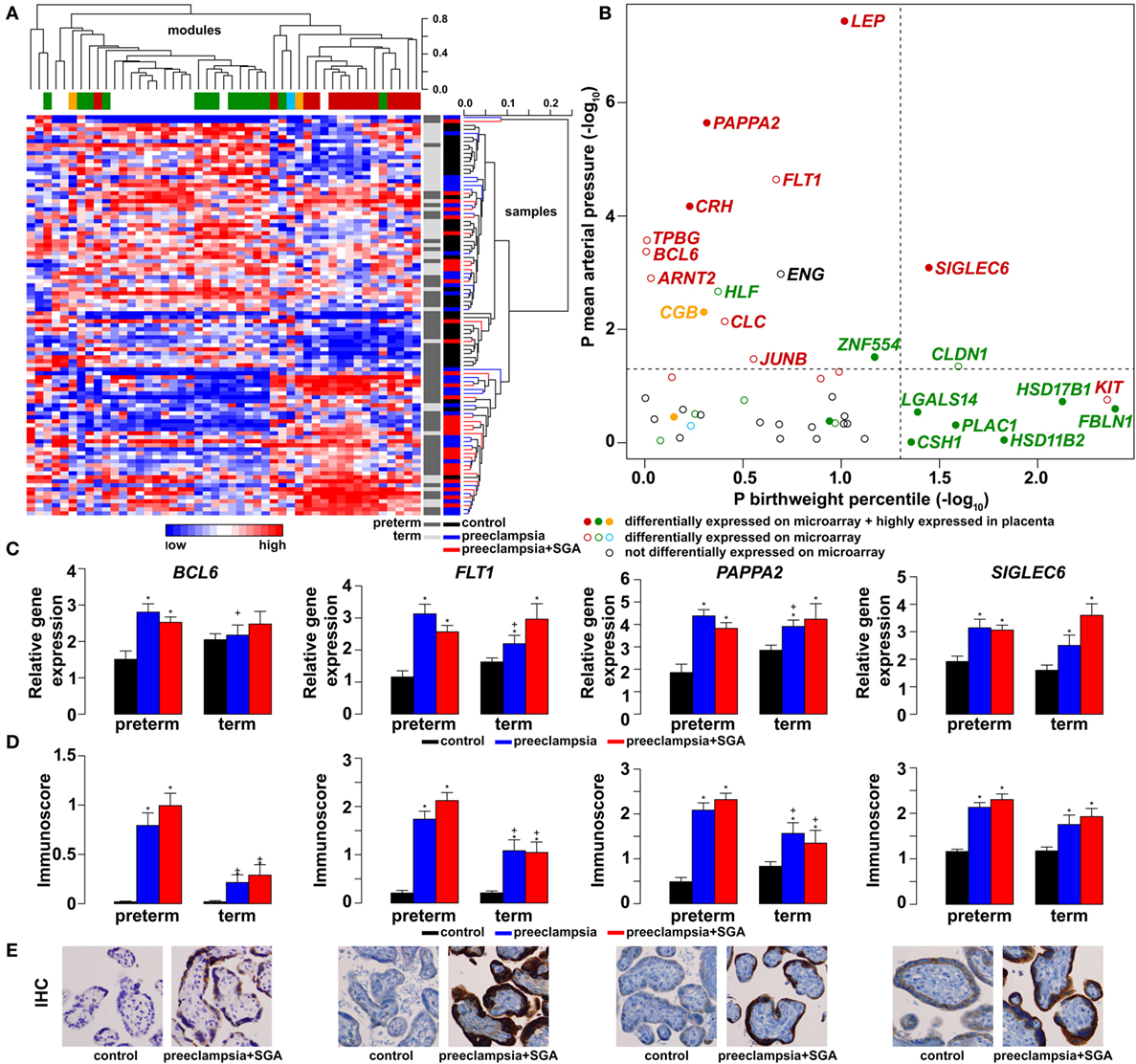
Figure 4. Gene modules associated with blood pressure (BP) and birthweight (BW). (A) Hierarchical clustering of qRT-PCR data obtained with 100 samples and 47 genes. Pearson correlation was used for similarity analysis and average method for linkage calculation. Samples were colored according to patient groups and maturity status. M1 (green) and M2 (red) module genes and 34 of 60 samples from women with preeclampsia clustered together. (B) Association of gene expression with BP and BW. The significance p-values for these coefficients were plotted for all genes, colored according to module classification (black: not changed on the microarray). Filled circles represent predominantly placenta-expressed genes and dashed lines the significance threshold at p = 0.05. Seven of 9 genes related to BW belong to module M1, while 10 of 15 genes related to BP are from module M2. Gene expression (C) and protein immunostaining (D) of selected four genes in module M2 show similar patterns in the sub-groups of preeclampsia, and semi-quantitative immunoscorings validate gene expression data. Significant differences in preterm or term preeclampsia samples compared to gestational-age matched controls are shown with “*”. When the change with preeclampsia in preterm samples was different from that in term samples, a “+” marks such an interaction. Comparisons for all genes are available in Figure S3 in Supplementary Material. (E) Representative images of the same placenta from a preterm control (left, 29 weeks) and a patient with preterm preeclampsia associated with SGA (right, 31 weeks) are shown for the immunostainings (hematoxylin counterstaining, 40× magnification).
Because of the possible involvement of these two gene modules in distinct pathologic pathways, we correlated qRT-PCR data with maternal and fetal clinico-pathological indicators to further investigate their association (Figure 4B). This analysis showed that 7 of 9 genes related to the birthweight (BW) percentile were from module M1, while 10 of 15 genes related to BP were from module M2, confirming our observations with microarray data. Therefore, we decided to also refer to these as “M1-BW” and “M2-BP” modules. Of interest, the expression of most (9/14) M1 genes was negatively associated with the “maternal vascular malperfusion” score of the placenta, while the expression of most (10/14) M2 genes was positively correlated with this parameter (Data S7 in Supplementary Material). This observation fits well with the accepted concept that maternal vascular malperfusion of the placenta leads to oxidative stress (40, 41, 63, 175) and the increased placental expression of FLT1 (63).
Maternal Study
Maternal Blood Reflects Gene Module Dysregulation in Preeclampsia
Next, we investigated whether placental gene module dysregulation can be detected in maternal blood and whether liquid biopsy can be used to determine when the module dysregulation occurs. To study the relationship between placental gene expression and maternal biomarker concentrations, we selected predominantly placenta-expressed genes with the most differential expression in modules M1 (CSH1) or M2 (LEP), and correlated their placental expression levels with concentrations of their secreted protein products (hPL and leptin) in maternal blood collected in both first (n = 12) and third trimesters (n = 19) in a Hungarian patient population. Positive correlations were found between placental gene expression and maternal blood protein concentrations for both biomarkers in both trimesters (Figure S4 in Supplementary Material), suggesting that certain proteins in the maternal circulation reflect the placental expression of their encoding genes throughout pregnancy.
To detect disease-associated protein signatures of placental dysfunction in maternal blood, similar to plasma DNA tissue mapping for noninvasive prenatal assessments (176, 177), we performed “virtual” liquid biopsy of the placenta in preterm preeclampsia (Figure S5 in Supplementary Material; Figure 5). We identified five genes with predominant placental expression, which have products extensively investigated in maternal blood in preterm preeclampsia or in all cases of preeclampsia during all trimesters. From data of 61 reports (35, 61, 82, 88, 126, 178–233), we built a database of 80,170 measurements in which preeclampsia data were expressed as a percentage of average levels in controls. Regarding biomarkers in module M1, we found hPL levels in preterm preeclampsia to be consistently below control levels throughout pregnancy. This is substantiated by the down-regulation of another M1 biomarker, HSD17B1, in first trimester maternal blood (160), confirming a generalized down-regulation of M1 biomarkers in early pregnancy. By contrast, the levels of biomarkers in module M2 had constant elevation in preterm preeclampsia compared to controls during gestation. When analyzing only data collected in the first trimester, prior to the onset of maternal circulation to the placenta, levels of M1 and M2 biomarkers were lower in women with preeclampsia compared to controls, while after 12 weeks of gestation, following the opening of the intervillous spaces to maternal blood flow, patients had increasing levels of M2 biomarkers in maternal blood compared to controls. These findings offer support for the conclusion that placental transcriptomic changes typical for preeclampsia in the third trimester are rooted in the first trimester.
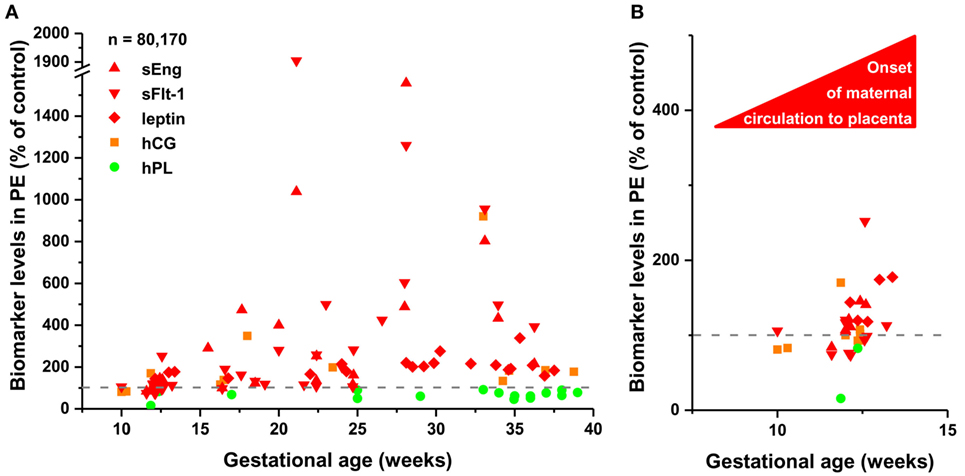
Figure 5. The timing of gene module dysregulation in preterm preeclampsia. (A) A database of 80,170 measurements published in 61 reports (35, 61, 82, 88, 126, 178–233) was built for “virtual” liquid biopsy of the placenta in preterm preeclampsia with maternal blood levels of proteins with the highest expression in the placenta among all tissues [i.e. hCG, human placental lactogen (hPL), sEng, sFlt-1, and leptin]. Levels of these biomarkers in preterm preeclampsia were expressed as the percentage of control levels (dashed line) and were represented in scatter plots by different colors reflecting gene module classification. Based on qRT-PCR data, sEng belongs to module M2 (red). hPL (M1, green module) levels in preeclampsia were constantly below control levels during gestation, while the levels of module M2 biomarkers in preeclampsia had continuous elevation compared to control levels as a function of gestational age. (B) Analysis of first trimester data revealed the lower expression of all biomarkers in preeclampsia than in controls before the onset of maternal circulation, and the increasing expression of module M2 biomarkers in preeclampsia compared to controls after 12 weeks of gestation.
Altered Maternal Serum Proteome in Preeclampsia
Next, we investigated the maternal serum proteome in early pregnancy in distinct phenotypes of preeclampsia and the potential effects of proteomic changes on the placental transcriptome. Comparing samples from women with preterm preeclampsia associated with SGA and their respective controls from an Israeli patient population (n = 10, Table 4), 19 DE protein spots were identified and investigated by mass spectrometry. According to public data, many of these proteins have a role in immune response, complement and coagulation cascades, lipid transport and metabolism, angiogenesis, BP regulation, and ion transport (Figure 6A, Data S8 in Supplementary Material). Comparing samples from women with term preeclampsia and their respective controls from this Israeli patient population (n = 10, Table 4), 14 DE protein spots could be identified (Figure S6A; Data S8 in Supplementary Material). Many of the proteins found in these spots are the same as those found in preterm preeclampsia or function in the same pathways. Of note, these pathways and the 26 differentially abundant proteins identified in term and preterm preeclampsia had mostly been implicated in a later stage of preeclampsia (43, 101, 120, 234–238). We concluded that there is a common dysregulation of the maternal serum proteome in term and preterm preeclampsia; however, the extent of changes is larger in the latter, in agreement with the more fulminant and early pathogenesis.
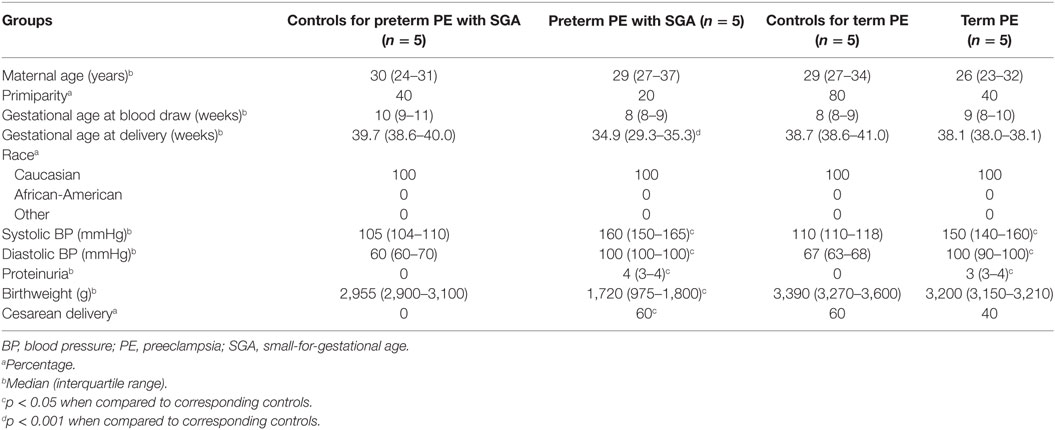
Table 4. Demographics of Israeli women included in the maternal blood two-dimensional differential in-gel electrophoresis proteomics study.
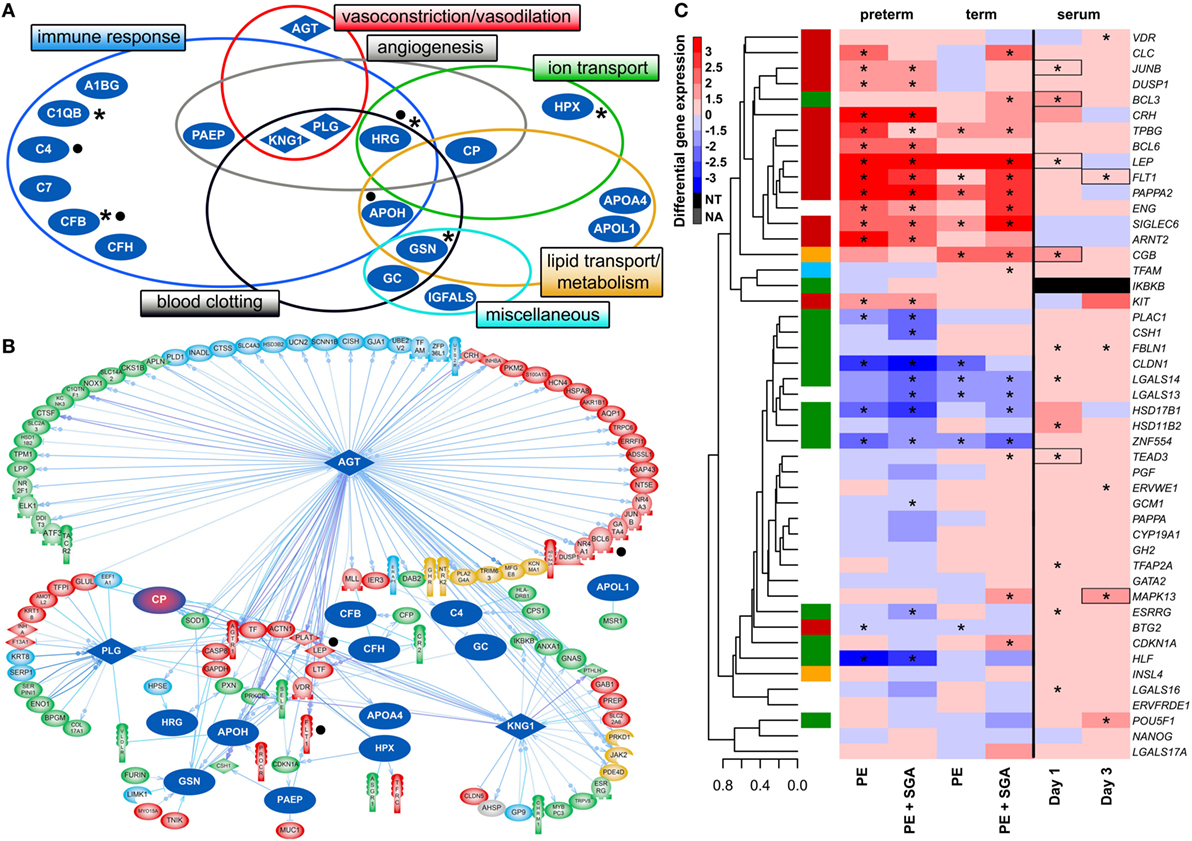
Figure 6. Maternal blood proteomic changes and their effect on differentially expressed (DE) genes in preterm preeclampsia. (A) The 19 DE maternal serum proteins in preterm preeclampsia identified by two-dimensional differential in-gel electrophoresis could be classified into functional groups relevant for preeclampsia pathophysiology. Asterisks denote proteins reported by others to have the same direction differential abundance in the first trimester in patients developing preterm preeclampsia (126, 143, 239–241). Dots denote immune proteins that multiple reaction monitoring assays identified to have differential abundance in preterm preeclampsia in the same direction. (B) The 19 DE serum proteins have connections to 121 DE placental genes, among which 48 belong to module M2. Angiotensinogen has more connections than other proteins (OR = 1.9, p = 4.9 × 10−5), and the most connections to module M2 genes (n = 35); 77 of 86 connections of angiotensinogen have a directional effect toward the gene. Dots denote genes in connection to angiotensinogen found up-regulated in villous trophoblast (VT) upon treatment with first trimester serum from preeclamptic women. (C) Hierarchical clustering tree and heatmap representing differential placental gene expression in preeclampsia as in Figure 4A. The expression of the same genes (except IKBKB) was determined in primary VTs upon treatment with first trimester maternal serum. Serum from preeclamptic compared to control women induced the up-regulation of seven placental dysregulated genes (depicted with boxes) on the first and third days of VT differentiation, among which six were up-regulated in term and three in preterm preeclampsia. Stars depict significant changes, color bar encodes signed (up or down)-fold changes. Abbreviations: NA, not expressed; NT, not examined.
Supporting our findings, five studies (126, 143, 239–241) found proteins with differential abundance in preterm preeclampsia in the same direction as our two-dimensional differential in-gel electrophoresis (2D-DIGE) study (Figure 6A). We also collected maternal blood specimens in the first trimester from a Hungarian patient population (n = 15, Table 5), and measured the concentrations of 10 immune proteins in patients with preterm preeclampsia associated with SGA and matched controls using liquid chromatography–mass spectrometry multiple reaction monitoring (MRM). In spite of the difference between the methods and ethnic background, MRM identified 4 of these 10 proteins as having differential abundance in the same direction as in the 2D-DIGE study, supporting the early pro-inflammatory changes in the maternal proteome in preterm preeclampsia (Figure 6A; Data S9 in Supplementary Material). MRM and proteomic evidence published to date supported 37% (7/19) of the proteomic changes detected by 2D-DIGE.
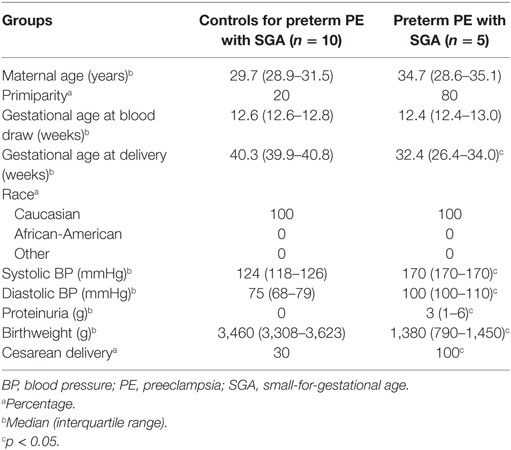
Table 5. Demographics of Hungarian women included in the maternal blood multiple reaction monitoring proteomics study.
The In Silico Effects of Altered Maternal Serum Proteome on the Placenta
To reveal whether early proteomic changes in maternal blood may affect the placental transcriptome, placental DE genes with documented connections to DE proteins in maternal serum were identified by Pathway Studio. The 121 DE placental genes with connections to DE serum proteins in preterm preeclampsia were marginally over-represented by those from module M2 (48/121, OR = 1.4, p = 0.057). Angiotensinogen had the largest number of connections to DE genes including FLT1 and LEP (n = 86, OR = 1.9, p = 4.9 × 10−5). This protein also had the largest number of connections to module M2 genes (n = 35), followed by plasminogen (n = 11) and kininogen-1 (n = 9), all involved in BP regulation (Figure 6B). These data were supported by the “renin-angiotensin signaling” as being a top pathway (p = 1.28 × 10−4) among the 35 angiotensinogen-connected DE genes (Data S10 in Supplementary Material). Similar results were obtained when analyzing connections between 116 DE placental genes and DE serum proteins in term preeclampsia (Figure S6B in Supplementary Material).
Trophoblast Study
The In Vitro Effects of an Altered Maternal Serum Proteome on the Trophoblast
Next, we aimed to study various factors implicated in the pathogenesis of preeclampsia in trophoblast models to determine whether these may drive the observed placental transcriptomic changes. Since the results noted above suggested that maternal serum proteins can influence the placental transcriptome, we first measured the effects of maternal blood from early pregnancy on trophoblastic gene expression. We treated primary VTs during differentiation with first trimester sera from women with preterm preeclampsia or normal pregnancy, and analyzed the expression of genes included in the placental validation study. Serum from preeclamptic women compared to controls induced up-regulation of seven placental DE genes on the first and third days of trophoblast differentiation, including FLT1 and LEP (Figure 6C). Among these, six genes were up-regulated in the placenta in term and three in preterm preeclampsia. These results support in silico findings and suggest that maternal serum factors can up-regulate FLT1 and may induce the terminal pathway.
The Effects of Altered VT Differentiation
Next, we examined whether disturbance in VT differentiation may be reflected in the placental gene expression signature in women with different preeclampsia phenotypes. Since there were no (n = 46) or subtle (n = 2) gestational age-dependent differences in the expression of selected target or housekeeping genes in third trimester control placentas in our microarray study (132), the comparison of placentas and trophoblasts from various gestational ages was deemed to be valid. Thus, we performed qRT-PCR profiling of primary VTs during 7 days of differentiation to reveal the dynamics in the expression of genes (Figure 7B), which we similarly profiled in the placenta (Figure 7A). We determined whether maximum expression change of selected genes during VT differentiation compared to day 0 inversely correlated with their maximum expression change in different preeclampsia phenotypes. We found that this was the case for 17 genes (Figure 7C), suggesting the delay or inhibition of VT differentiation-related expression change of these genes in preeclampsia. Among these 17 genes, 15 showed this behavior in preterm and 9 in term preeclampsia, suggesting that VT differentiation problems are more pronounced in preterm than in term preeclampsia (p = 0.057).
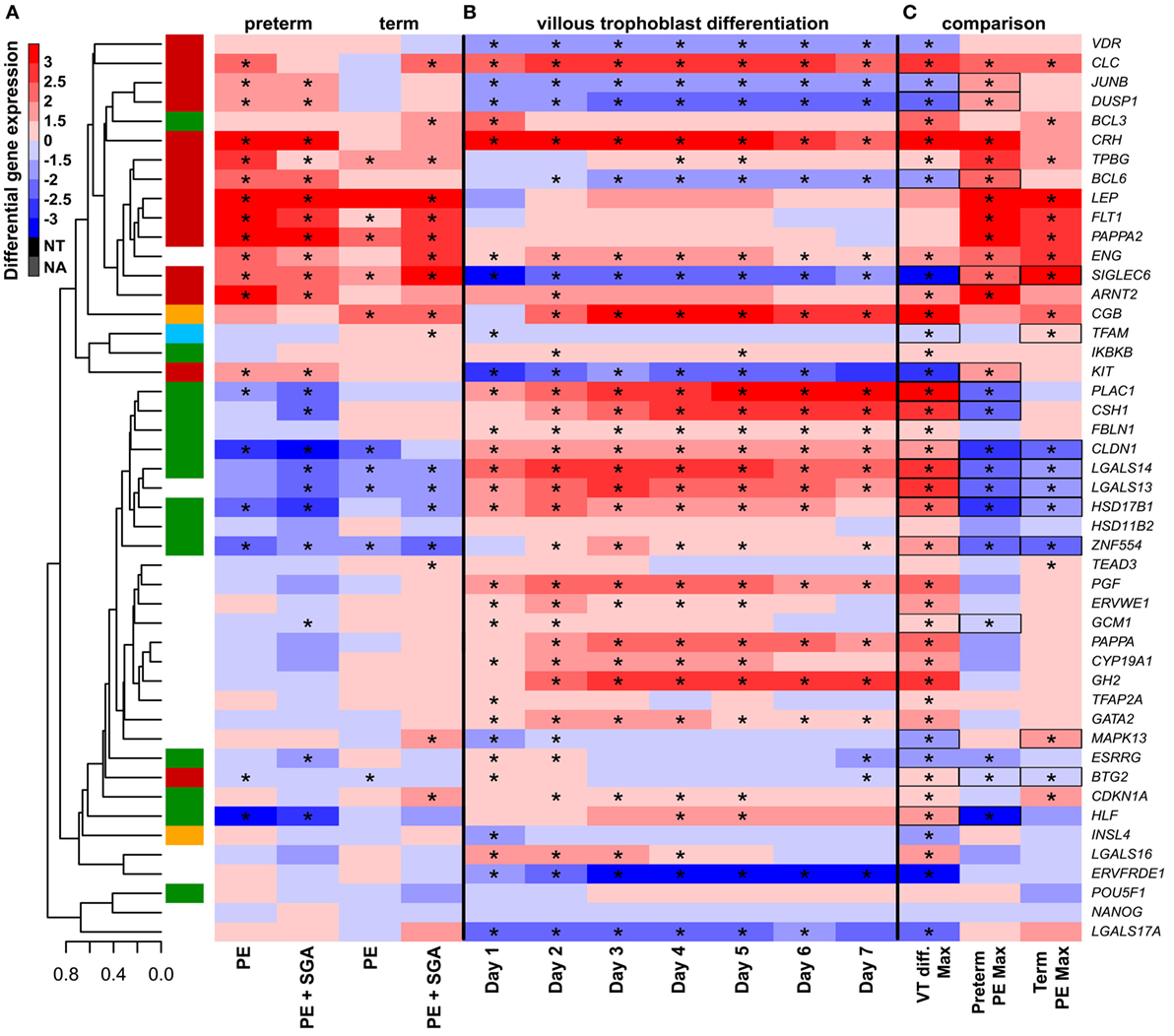
Figure 7. The effect of trophoblast differentiation on differentially expressed genes in preeclampsia. (A) Hierarchical clustering tree of expression data for 47 genes in 100 placental specimens was augmented with a heatmap representing differential gene expression in term or preterm subgroups of preeclampsia. (B) Primary villous trophoblast (VT) differentiation time series expression data for 47 genes were depicted with a heatmap representing differential gene expression in each time point (days 1–7) compared to day 0. (C) Maximum expression values in the VT differentiation time series were presented alongside with maximum expression values in the placenta in preterm or term preeclampsia. Comparative visualization revealed the opposite-direction differential regulation of 17 genes in preeclampsia compared to VT differentiation as depicted with black boxes. Among these genes, 15 had this behavior in preterm preeclampsia and 9 in term preeclampsia (p = 0.057). In (A–C), stars depict significant changes, color bar encodes signed (up or down)-fold changes. Abbreviations: NA, not expressed; NT, not examined.
The Impact of Hypoxia, Ischemia, and Overexpression of BCL6 and ARNT2 on the Trophoblast
Subsequently, we tested how other factors implicated in preeclampsia pathogenesis, namely physiologic hypoxia (2% O2) (167) or alternating hypoxic (1% O2) and normoxic (20% O2) conditions (ischemia) (40, 41, 242), in combination with the overexpression of hub transcription regulatory factors, may affect trophoblastic gene expression in a widely used BeWo cell trophoblast model. Two percent O2 induced the dysregulation of only five genes, including LEP and FLT1, from the set of genes investigated in the placenta in preterm preeclampsia (Figure 8C), while alternating O2 concentrations induced the dysregulation of only three genes (Figure 8D). Since hypoxia or ischemia alone did not induce similar transcriptomic changes in BeWo cells as seen in the placenta in preeclampsia, we examined how the overexpression of hub transcription regulatory genes in module M2 may modify the effects of these two conditions. Of note, 2% O2 combined with ARNT2 or BCL6 overexpression led to the dysregulation of a large number of genes (Figure 8C). There were 9 genes (6 in the M2 and 3 in the M1 modules) dysregulated in BeWo cells, including FLT1, ARNT2, and ZNF554, similar to preeclampsia. Alternating O2 concentrations combined with ARNT2 or BCL6 overexpression led to the dysregulation of 11 genes (5 in the M2 and 3 in the M1 modules), including LEP, FLT1, and ENG, similar to preeclampsia (Figure 8D). However, ARNT2 or BCL6 overexpression at normoxic conditions did not lead to substantial gene expression changes (Figure 8B), underlining the importance of gene–environment interactions. A permutation test showed that BCL6 overexpression in ischemia mimicked overall expression changes of module M1 and M2 genes in preterm preeclampsia, while ARNT2 overexpression in ischemia (and also in hypoxia) mimicked the up-regulation of module M2 genes in term and preterm preeclampsia (Data S11 in Supplementary Material). Since BCL6 overexpression up-regulated ARNT2 both in ischemia and hypoxia but not vice versa, we propose that BCL6 is upstream of ARNT2. The up-regulation of these two transcription regulatory genes sensitize the trophoblast to ischemia, leading to the early dysregulation of modules M1 and M2, thus promoting preterm preeclampsia (Figure S7 in Supplementary Material).
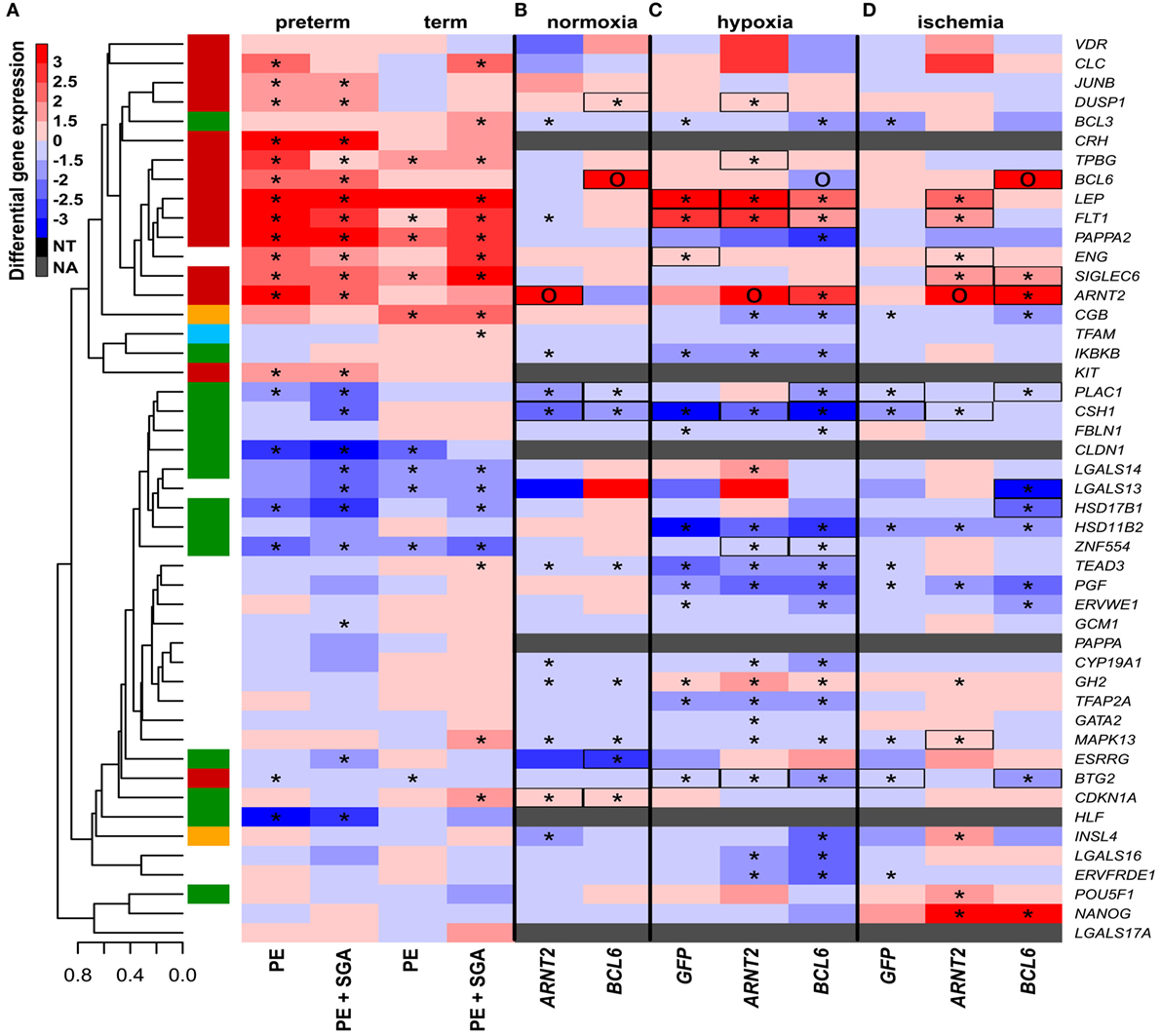
Figure 8. The effect of O2 levels, BCL6 and ARNT2 overexpression on differentially expressed genes in preeclampsia. (A) Hierarchical clustering tree and heatmap representing differential placental gene expression in preeclampsia. (B) The overexpression of ARNT2 or BCL6 in normoxic BeWo cells induced the dysregulation of three or five genes dysregulated in preeclampsia, respectively (boxed). (C) Hypoxia induced the dysregulation of five genes in BeWo cells also altered in preeclampsia. Hypoxia combined with ARNT2 or BCL6 overexpression led to the dysregulation of a large number of genes. (D) Ischemia induced the dysregulation of three genes in BeWo cells similar to preeclampsia. Ischemia combined with ARNT2 or BCL6 overexpression led to the dysregulation of 11 genes similar to preeclampsia. (C,D) Represents comparisons of gene expressions between hypoxia/ischemia vs. normoxia. In (A–D), stars depict significant changes, “O” depicts “overexpressed,” color bar encodes signed (up or down)-fold changes. Black boxes depict genes with similar expression changes in vitro as in the placenta in preeclampsia. Abbreviations: NA, not expressed; NT, not examined.
Since none of the investigated conditions could up-regulate BCL6, we wondered whether BCL6 overexpression might have an epigenetic background. Treating BeWo cells with 5-azacitidine down-regulated BCL6, supporting that its expression is regulated by DNA methylation in the trophoblast (Figure S8 in Supplementary Material). Of note, a recent study described the first intron of BCL6 to be key in its overexpression in Burkitt lymphoma via altered DNA methylation (243). The Human Reference Epigenome Mapping Project revealed a differentially methylated region (DMR) in this intron in H1 embryonic stem cells as well as trophoblastic and neuronal cells derived from H1 cells, suggesting that this DMR may be differentially methylated in the trophoblast compared to other cells. To address whether this intronic region may be affected in the trophoblast in preeclampsia, we investigated DNA methylation in this region in primary VTs compared to cord blood cells collected from the same normal pregnancies. Bisulfite sequencing showed that Chr3:187,458,083-187,458,651 and Chr3:187,460,304-187,460,374 regions contain 12 hypermethylated CpGs in the trophoblasts compared to cord blood cells (Figures S8 and S9 in Supplementary Material). Further, we tested DNA methylation in this region in 100 placentas with qRT-PCR data including patients with preeclampsia after laser capturing VTs. Three CpGs (Chr3:187,458,095, Chr3:187,458,163, and Chr3:187,458,327) were differentially methylated in preeclampsia (Figure S10 in Supplementary Material), of which CpG Chr3:187,458,163 was differentially methylated in preterm preeclampsia, suggesting that this CpG may have a role in BCL6 dysregulation in preeclampsia.
The Effects of ZNF554 Down-Regulation in VTs
Next, we were interested in how the dysregulation of module M1 genes may play a role in preeclampsia pathology. Among hub genes of this module, ZNF554 was of most interest due to the biological processes enriched in its co-expression network (Figure S1B in Supplementary Material), and also to its potential placenta- and preeclampsia-related regulation by transposable elements. This hypothesis was based on the fact that insertion of transposable elements into regulatory regions can lead to transcriptional changes, especially in the placenta (155, 244–247), and that the 5′ flanking region of ZNF554 contains many LTR10A copies which had top enrichment among module M1 genes (OR = 17.4, p = 1.27 × 10−7; Data S12 in Supplementary Material). Of note, LTR10A drives placenta-specific expression of NOS3 (248), and it may also have a similar effect on ZNF554. Indeed, ZNF554 had the highest expression in the placenta in comparison to 47 other human tissues, which mostly had negligible ZNF554 expression (Figure 9A).
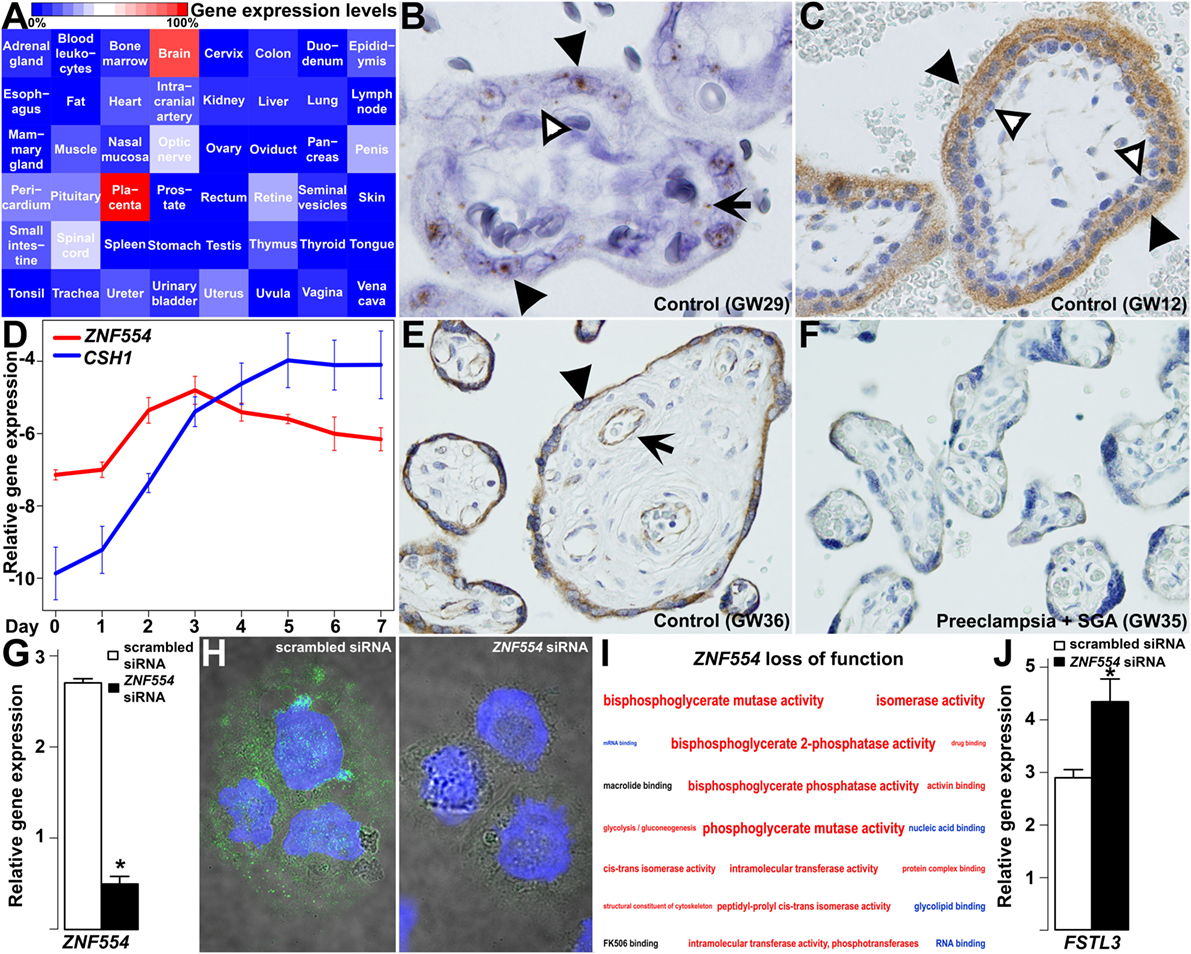
Figure 9. ZNF554 down-regulation in the villous trophoblast (VT) in preeclampsia. (A) Tissue qRT-PCR array revealed the highest ZNF554 expression in the placenta among 48 human tissues. Color code depicts gene expression levels relative to that of the placenta (100%). (B) In situ hybridization of a third trimester control placenta (GW29) and (C) immunohistochemistry of a first trimester placenta (GW12) shows mainly syncytiotrophoblastic ZNF554 expression (hematoxylin counterstaining, 1,400 and 400× magnifications, respectively). Black or white arrowheads depict syncytiotrophoblast or cytotrophoblast, while black arrow depicts fetal endothelium, respectively. (D) qRT-PCR revealed that ZNF554 expression is up-regulated during VT differentiation in parallel with CSH1. (E,F) ZNF554 immunopositivity was faint in the syncytiotrophoblast in preeclampsia [(F), GW35] compared to gestational-age matched controls [(E), GW36] (hematoxylin counterstaining, 400× magnifications). Arrow and arrowhead depict syncytiotrophoblast and villous endothelium, respectively. (G) ZNF554 mRNA expression was 74% lower in ZNF554-silenced BeWo cells compared to controls used for the microarrays (p = 5.24 × 10−6). (H) Nuclear and cytoplasmic ZNF554 immunofluorescence decreased in BeWo cells treated with ZNF554 siRNA compared to control cells (3,500× magnifications). (I) Molecular functions and one Kyoto Encyclopedia of Genes and Genomes (KEGG) pathway (glycolysis/gluconeogenesis) affected in ZNF554-silenced BeWo cells. Colors denote the proportions of up- or down-regulated genes (red: >0.5 up-regulated; blue:>0.5 down-regulated; black: 0.5–0.5 up- and down-regulated). Letter sizes represent the minus log10 of p-values of the given functions or pathway. (J) qRT-PCR validated FSTL3 up-regulation (2.7-fold, p < 0.001) in BeWo cells upon ZNF554 knock-down.
Subsequent in situ hybridization (Figure 9B) and immunostaining (Figure 9C) of first and third trimester placentas of women with a normal pregnancy showed dominant ZNF554 expression in the syncytiotrophoblast but not in the villous cytotrophoblast. Thus, we investigated ZNF554 expression during VT differentiation, in which it was up-regulated similar to CSH1 (Figure 9D), supporting that ZNF554 expression is developmentally regulated in VTs. Of interest, ZNF554 immunostaining was faint in the syncytiotrophoblast in preeclampsia compared to controls (Figures 9E,F). To characterize the loss of syncytiotrophoblastic ZNF554 function, we silenced ZNF554 in BeWo cells. At 74% ZNF554 knock-down (p = 5.24 × 10−6) (Figure 9G), decreased nuclear and cytoplasmic ZNF554 immunostaining was found (Figure 9H). Microarray analyses of ZNF554-silenced cells revealed 123 DE genes (Data S13 in Supplementary Material) including 9 DE placental genes in preeclampsia, and the dysregulation of the “glycolysis/gluconeogenesis” pathway (OR = 7.8, q = 0.06) as well as 18 molecular functions including “RNA binding” (down) and “activin binding” (up) (Figure 9I; Data S14 in Supplementary Material). The up-regulation of FSTL3 was confirmed by qRT-PCR (2.7-fold, p < 0.001) (Figure 9J). FSTL3 encodes a secreted glycoprotein that inactivates activin and other TGFβ ligands (249). It is involved in the regulation of EVT invasion (250, 251) and its placental up-regulation is associated with low BW in preeclampsia (252). This finding confirms that the dysregulation of ZNF554 may have key downstream effects on the pathogenesis of preeclampsia.
The Effects of ZNF554 Down-Regulation in EVTs
We supposed that ZNF554 may also affect EVTs, since its expression in EVTs was detected in first and third trimester maternal decidua (Figures 10A–D), and ZNF554-positive intraluminal and endovascular trophoblasts were found in the wall of transformed spiral arteries (Figure 10B). In preterm preeclampsia, ZNF554 immunostaining of EVTs was weaker than in controls (Figures 10C,D). To characterize the loss of ZNF554 function in EVTs, we silenced ZNF554 in trophoblastic HTR8/SVneo cells (253). At 87% knock-down (p < 0.001) (Figure 10E), we observed decreased nuclear and cytoplasmic ZNF554 immunostaining (Figure 10F). Microarray analysis of ZNF554-silenced cells showed 185 DE genes (Data S15 in Supplementary Material) including 18 DE placental genes in preeclampsia. Gene ontology (GO) analysis revealed 16 molecular functions dysregulated, including “cyclin-dependent protein kinase regulator activity,” “metalloendopeptidase inhibitor activity,” and “insulin-like growth factor binding.” The 67 enriched biological processes included “regulation of growth,” “smooth muscle cell migration,” “smooth muscle cell-matrix adhesion,” and “response to oxygen levels,” all relevant to trophoblast invasion and placental pathology of preeclampsia (Data S16 in Supplementary Material).
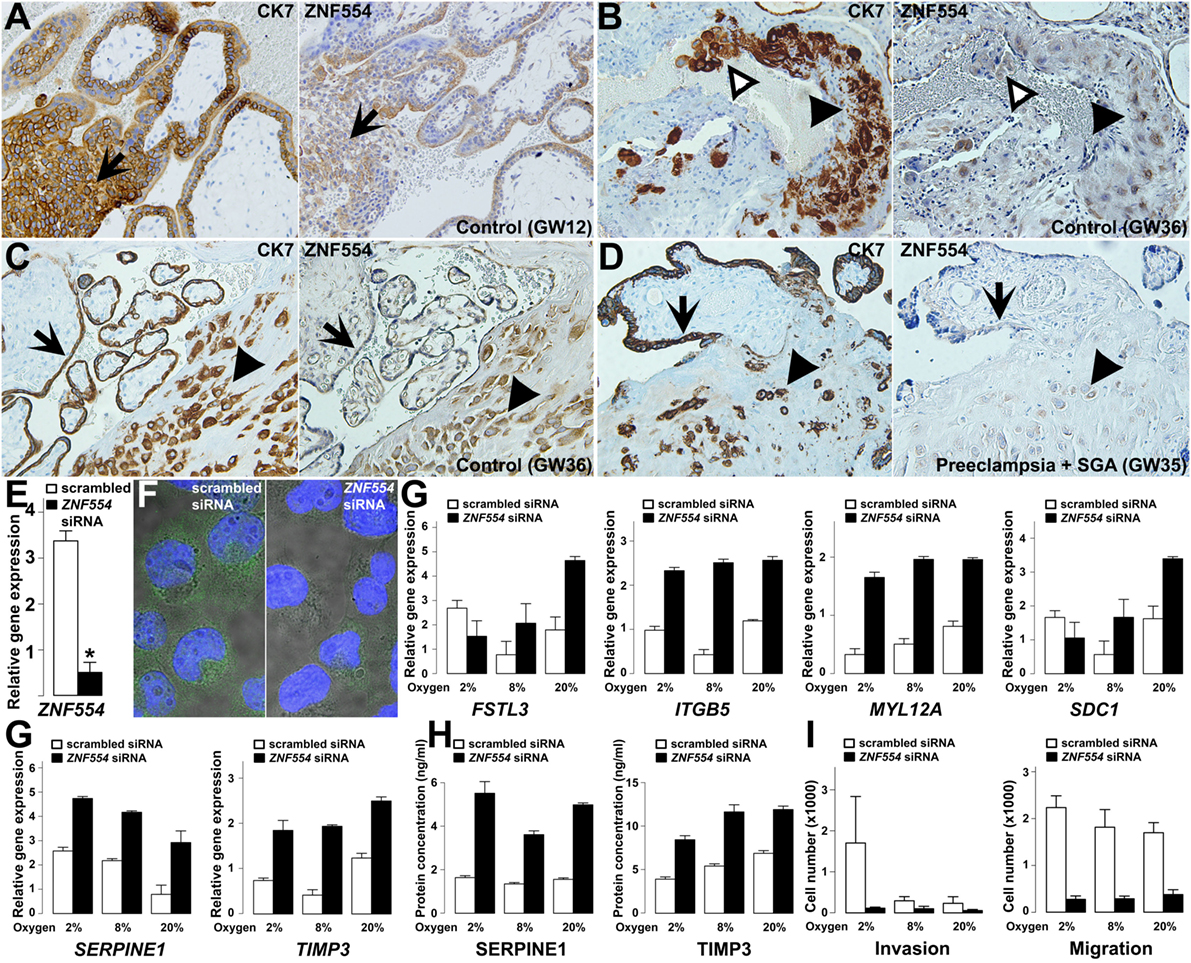
Figure 10. ZNF554 down-regulation in extravillous trophoblasts (EVTs) in preeclampsia. (A) EVTs in trophoblastic columns and (B) endovascular and intraluminal trophoblasts in the myometrium were immunostained for cytokeratin-7 (left) and ZNF554 (right). Arrows depict the direction of trophoblast invasion, black and white arrowheads point to EVTs in the wall and lumen of the spiral artery, respectively (serial sections, hematoxylin counterstaining, 200× magnifications). ZNF554 immunostaining in EVTs (arrowheads) and syncytiotrophoblast (arrows) was weaker in (D) preeclampsia than in (C) controls (serial sections, hematoxylin counterstaining, 200× magnifications). (E) ZNF554 mRNA expression decreased to 13% upon knock-down (p < 0.001), (F) ZNF554 immunofluorescence was weaker in the nucleus and cytoplasm of ZNF554-silenced (left) than of control (right) HTR8/SVneo cells (3,500× magnifications). (G) The dysregulation of selected genes upon ZNF554 knock-down was confirmed by qRT-PCR. (H) Plasminogen activator inhibitor-1 (PAI-1 / SERPINE1) and TIMP-3 proteins were increasingly secreted from ZNF554-silenced cells. (I) ZNF554-silenced cells had remarkably decreased invasive (left) and migratory (right) characteristics. O2 concentrations are shown below the bars.
qRT-PCR confirmed the dysregulation of eight DE genes. Two genes (CDKN1A, STK40) are involved in the regulation of cell proliferation and differentiation (254, 255), and proliferation assays showed that ZNF554 knock-down decreased cell proliferation in HTR8/SVneo cells slightly after 48 h (−14%, p = 0.02) (Figure S11 in Supplementary Material). Six genes (FSTL3, ITGB5, MYL12A, SDC1, SERPINE1, and TIMP3) encode proteins involved in cell adhesion, migration, invasion, and angiogenesis (Figure 10G). Since EVTs move through an environment with changing O2 levels, we used O2 concentrations for conditions relevant for endovascular (8%) and interstitial (2%) trophoblast invasion besides standard cell cultures (20%). The effect of ZNF554 knock-down was significant regardless of O2 levels on four genes (ITGB5, MYL12A, SERPINE1, and TIMP3), while there was an interaction between O2 levels and ZNF554 silencing on two genes (FSTL3 and SDC1) (Figure 10G).
The up-regulation of SERPINE1 (PAI-1) and tissue inhibitor of metalloproteinases-3 (TIMP-3) was also confirmed at the protein level in supernatants of ZNF554-silenced cells (Figure 10H). Both proteins have an inhibitory function on trophoblast migration and invasion (250, 256, 257), and TIMP-3 is the major tissue metalloproteinase inhibitor at the maternal–fetal interface, which is up-regulated in preeclampsia (106, 258, 259). These results suggested that ZNF554-silenced cells have reduced migratory and invasive functions. Indeed, functional assays revealed that ZNF554 silencing had a strong inhibitory effect on trophoblast migration (p = 1.9 × 10−10) regardless of the O2 concentration, and also on invasion (p < 0.001), especially at 2% O2 concentration (Figure 10I). These data corroborated that ZNF554 supports trophoblast invasion via modulating a set of key genes that are involved in this process.
DNA Methylation-Mediated Trophoblastic ZNF554 Down-Regulation in Preeclampsia
We wondered whether placental ZNF554 down-regulation might have an epigenetic background, given that the ZNF554 flanking region contains several transposable elements, including several Alus, which are generally hypomethylated in germ cells and the placenta (260, 261), while their hypermethylation leading to altered gene expression may be detected in preeclampsia (262, 263). Thus, the down-regulation of ZNF554 expression in the placenta of patients with preeclampsia may also be reflected in the DNA methylation of the transposable elements in its 5′ flanking region.
The treatment of BeWo cells with 5-azacitidine increased ZNF554 expression, showing the role of DNA methylation in trophoblastic ZNF554 regulation (Figure 11A). The subsequent search in the Human Reference Epigenome Mapping Project data revealed a DMR located in the AluY, which was hypomethylated in H1 embryonic stem cells and H1-derived trophoblasts compared to H1-derived neuronal cells (Figure 11B). This was of interest, since AluY is a retrotransposon evolved recently in primates, and its differential DNA methylation supports the expression of other gene transcripts in the placenta compared to somatic tissues (264). These data prompted us to investigate the DNA methylation in this genomic region in primary VTs and cord blood cells collected from the same normal pregnancies. In fact, bisulfite sequencing showed that the AluY, similarly to the AluSq2, is heavily methylated in cord blood cells compared to the hypomethylated trophoblast, suggesting its importance in the developmental regulation of ZNF554 expression (Figure 11B; Figure S12 in Supplementary Material).
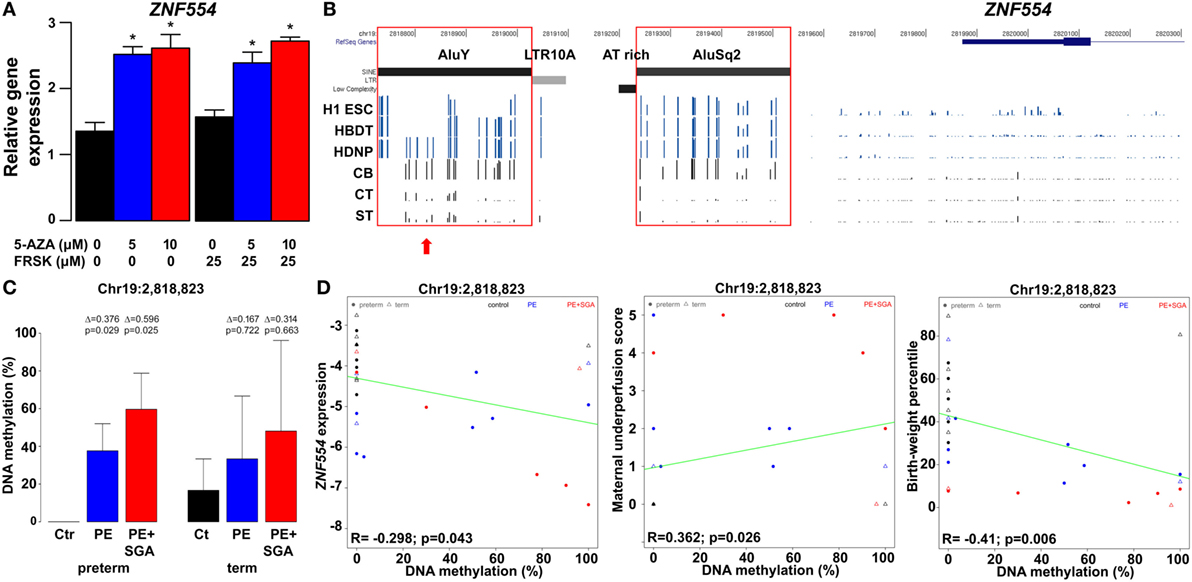
Figure 11. The effect of trophoblastic DNA methylation on ZNF554 expression and clinical parameters in preeclampsia. (A) Increased ZNF554 expression in BeWo cells upon treatment with 5-azacitidine (5-AZA) irrespective of forskolin (FRSK) co-treatment. (B) Upper three lanes: whole genome bisulfite sequencing data of ZNF554 5′ flanking region from the Human Reference Epigenome Mapping Project. H1 ESC, H1 embryonic stem cell; HBDT, H1 BMP4-derived trophoblast; and HDNP, H1-derived neuronal progenitor. Lower three lanes: bisulfite sequencing data from this study. Abbreviations: CB, cord blood cell; CT, cytotrophoblast; ST, syncytiotrophoblast. Red boxes: AluY and AluSq2 are heavily methylated in cord blood cells and hypomethylated in trophoblasts. Red arrow: CpG Chr19:2,818,823. (C) CpG Chr19:2,818,823 was differentially methylated in trophoblasts in preterm preeclampsia ± SGA. (D) Correlation was observed between Chr19:2,818,823 CpG methylation and ZNF554 expression, maternal vascular malperfusion score of the placenta, and birthweight percentile.
Further, we tested DNA methylation in this region in 100 placentas with qRT-PCR data, including patients with preeclampsia after laser capture of the VTs. Bisulfite sequencing of the trophoblastic DNA revealed four CpGs on AluY (Chr19:2,818,823, Chr19:2,818,864, Chr19:2,818,868, and Chr19:2,818,876) hypomethylated in controls but hypermethylated in preterm preeclampsia, with highest methylation in cases associated with SGA (Figure 11C; Figure S13 in Supplementary Material). Importantly, we found correlations between Chr19:2,818,823 CpG methylation and ZNF554 expression (R = −0.30, p = 0.04), maternal vascular underperfusion score of the placenta (R = 0.36, p = 0.03), and BW percentile (R = −0.41, p < 0.01) (Figure 11D). These data collectively provide evidence that the hypermethylation of certain CpGs in AluY in the trophoblast may result in low ZNF554 expression, impaired trophoblast invasion, preeclampsia, and fetal growth restriction.
Discussion
The placenta has a key role in the pathogenesis of the terminal pathway of preeclampsia, which may be triggered by discrete disease pathways at early stages of pregnancy, leading to the development of different preeclampsia phenotypes. In this study, an integrated systems biology approach was employed to gain insights into these complex pathways, given that this strategy offered the ultimate analytic solution to investigate and understand the complex disease pathways of the syndrome of preeclampsia (265–268). We incorporated “omics,” clinical, placental, and functional data from patients with distinct phenotypes of preeclampsia. We employed molecular network-based approaches to identify networks and modules of genes or proteins that are perturbed in the placenta and the maternal circulation of women with preeclampsia.
Our placental transcriptomics study identified 1,409 DE genes involved in many biological processes (e.g. BP regulation, apoptosis, development, hormone secretion, metabolism, and signaling) that were previously implicated in the pathogenesis of preterm preeclampsia by other placental transcriptomics studies (105, 110, 111, 113, 114, 116, 117, 122, 123, 125, 127, 128, 130, 131, 134, 137, 269–272). Despite the differences in patient populations, design, or methodologies between these studies, many DE genes on our list have also been found by other groups. Indeed, from the 40-gene meta-signature that characterized the significant intersection of DE genes from independent placental gene signatures in preeclampsia in the meta-analysis of Kleinrouweler et al. (135), our microarray and qRT-PCR studies found 26 (65%) to be DE in preterm preeclampsia. Of note, 16 of these 26 genes belong to module M2, while only six to the M1 module. This supports our observation that the dysregulation of module M2 is associated with BP elevation, the maternal disease condition required for patient inclusion into these studies. The weaker involvement of module M1 genes in the meta-signature may reflect the heterogeneity of preeclampsia transcriptomics studies regarding fetal (growth restriction) and placental disease conditions. Our microarray study was homogeneous for preterm preeclampsia cases with low BW and placental disease, while only a couple of other studies had this rigor. To overcome the inconsistency of smaller placental transcriptomics studies, Leavey et al. (140, 142) employed advanced bioinformatics methods to aggregate microarray datasets across multiple platforms to generate large datasets of patient samples. Unsupervised clustering of these datasets revealed three distinct molecular subclasses of preeclampsia. Among these, the “canonical” subclass, which is associated with the differential expression of our module M2 genes, was characteristic for preterm preeclampsia and consistent with stress response to poor oxygenation, further supporting our findings. However, our discovery on the two major dysregulated placental disease gene modules and their hub transcription regulatory genes, separately associated with maternal or fetal disease pathways, are novel (Figure 12).
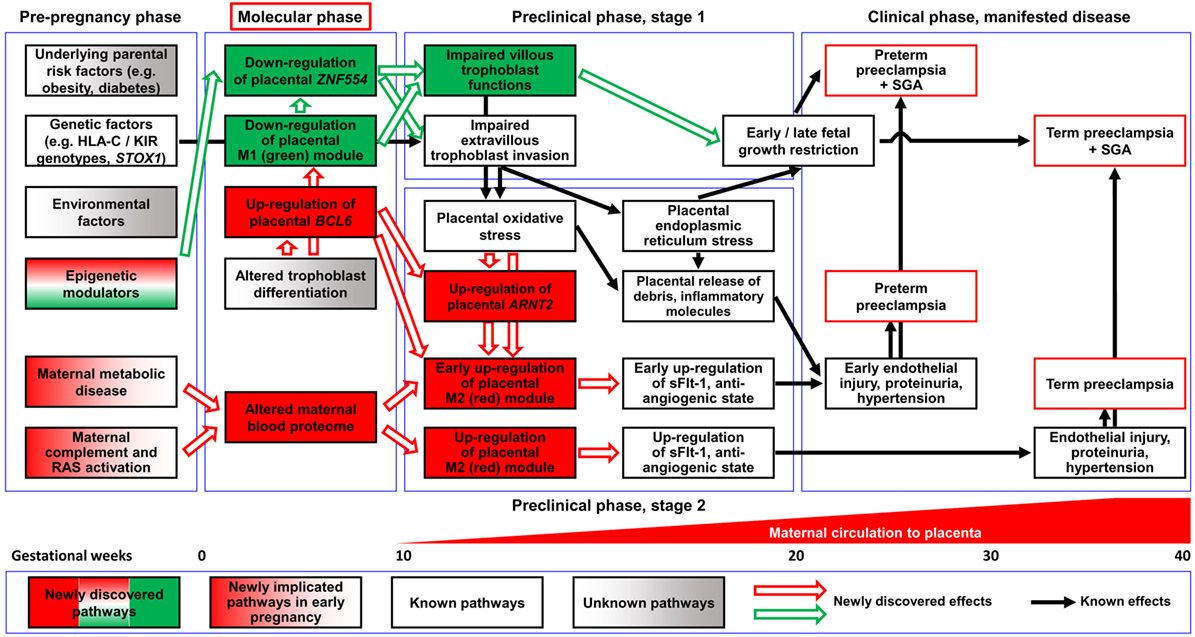
Figure 12. Pathologic pathways in preeclampsia. Maternal pathways: alterations in the maternal blood proteome, including systemic inflammatory changes, can be observed in both preterm and term preeclampsia before the maternal circulation of the placenta has been established, supporting the observation that maternal factors have a key role in triggering early disease pathways. Later, these alterations can induce trophoblastic functional changes leading to the up-regulation of module M2 genes, the overproduction of sFlt-1, and an anti-angiogenic state through a trajectory that does not necessarily affect fetal growth. Placental pathways: altered differentiation of the trophoblast leads to the dysregulation of module M1 genes and hub factors in module M2. The down-regulation of ZNF554 and module M1 genes involved in the regulation of fetal growth and metabolism imply impaired villous trophoblast (VT) functions besides abnormal extravillous trophoblast (EVT) invasion. The up-regulation of the BCL6-ARNT2 pathway sensitizes the trophoblast to ischemia and increases FLT1 expression after the maternal circulation to the placenta has been established. These changes are observed only in preterm preeclampsia, suggesting that this placental pathway promotes the early development of preeclampsia. The interplay of these molecular pathways leads to the complex pathogenesis of preeclampsia. Abbreviations: RAS, renin-angiotensin system; SGA, small-for-gestational age.
Functional assays on hub transcription factors of these two disease gene modules demonstrated that ZNF554 (M1) down-regulation leads to impaired trophoblast invasion, while BCL6 and ARNT2 (M2) overexpression sensitizes the trophoblast to ischemia, which are hallmarks in the pathogenesis of preterm preeclampsia. In the “ZNF554” pathway, hypermethylation of AluY in the ZNF554 5′ flanking region inhibits gene expression, leading to impaired trophoblast invasion, placental vascular malperfusion, and low BW. In the “BCL6-ARNT2” pathway, which is activated only in preterm preeclampsia, ischemic stress of the trophoblast coupled with BCL6 and ARNT2 overexpression increases FLT1 expression. This then eventually promotes the anti-angiogenic state, hypertension (37, 56, 57, 61, 62, 83), and the early onset of this syndrome (Figure S7 in Supplementary Material).
The perturbed placental disease gene modules in preterm preeclampsia can be detected by liquid biopsy in maternal blood in the early stages of pregnancy. Indeed, we detected the down-regulation of M1 and M2 disease gene module biomarkers in these patients during the first trimester. Of interest, the up-regulation of module M2 biomarkers can be detected after the establishment of maternal circulation in the intervillous space. These findings support the observation that placental transcriptomic changes, typical for preterm preeclampsia observed in the third trimester, are rooted in the first trimester. The positive correlation of gene expression in module M1 with BW and in module M2 with BP suggests that M1 genes may be biomarkers for placental and fetal growth and development while M2 genes can serve as biomarkers for placental stress.
First trimester maternal blood proteomics uncovered the altered abundance of proteins of the renin–angiotensin and immune systems as well as complement and coagulation cascades in patients who subsequently developed both preterm and term preeclampsia. The same proteins and pathways were found to be dysregulated in maternal blood in later stages of preeclampsia by other proteomics studies (108, 115, 118–120, 126, 129, 133, 136, 138, 139, 143); however, ours is the first revealing their dysregulation at an earlier stage of pregnancy when there is no or minimal direct connection between the placenta and the maternal circulation (273).
From these dysregulated maternal serum proteins, in silico analysis pointed to candidates, which may drive trophoblastic transcriptomic changes, and corroborated earlier findings on angiotensinogen/angiotensin II in driving hypertension indirectly through FLT1 up-regulation in addition to its direct effects (91, 274). Moreover, in vitro functional assays revealed that altered maternal serum proteome in the first trimester can affect the trophoblastic transcriptome and up-regulate FLT1. This is in agreement with reports indicating that maternal blood factors in preeclampsia can induce trophoblastic soluble endoglin overexpression and the development of preeclampsia-like symptoms in mice (85, 275).
Remarkably, most of the dysregulated maternal serum proteins in the first trimester in both preterm (11 of 19) and term (7 of 14) preeclampsia are implicated in immune functions (Figure 6; Figure S6 in Supplementary Material), suggesting a critical role for immune pathways and inflammation in the early pathogenesis of both phenotypes of preeclampsia. This is consistent with clinical, epidemiological, and immunological evidence showing that: (1) preeclampsia has multiple risk factors (e.g. dyslipidemia, hypertension, insulin resistance, and obesity) characterized by heightened inflammation (29, 276); (2) the combination of inhibitory decidual NK (dNK) cell killer immunoglobulin-like receptor and the fetal HLA-C2 genotype increases the susceptibility to preeclampsia due to the loss of activating interactions between trophoblasts and dNK cells at early stages of placentation (168, 277–283); (3) an altered local immune regulation and a shift toward the pro-inflammatory macrophage phenotype promotes a pro-inflammatory milieu in the maternal decidua in preeclampsia (284, 285); (4) an imbalance between Th1/Th2/Th17/Treg cells in preeclampsia leads to failure of maternal–fetal tolerance mechanisms (286–289); and (5) complement system activation in preeclampsia leads to the activation of innate immune cells and placental damage (101, 234, 236, 237, 290–292). The role of inflammation in early preeclampsia disease pathways is also supported by in vivo studies showing that bacterial endotoxin administration to pregnant rats induces placentation defects and symptoms consistent with preeclampsia (293–298).
Overall, our data show that there are distinct maternal and placental disease pathways, and their interaction influences the clinical presentation of preeclampsia. The activation of maternal disease pathways can be detected in both preterm and term preeclampsia earlier and upstream of placental dysfunction, not only downstream as described before (43), and distinct placental disease pathways are superimposed on these maternal pathways. This is a paradigm shift in our understanding of preeclampsia, which in agreement with epidemiological studies (25, 31) warrants for the central pathologic role of preexisting maternal diseases or perturbed maternal–fetal–placental immune interactions in preeclampsia.
The superimposed placental disease pathways differ between preterm and term preeclampsia. For preterm preeclampsia, our functional data suggest that placental disease pathways are partly originated from altered trophoblast differentiation, which is followed by trophoblastic stress, induced by perturbed maternal blood proteome factors and/or ischemia after the onset of maternal circulation to the placenta. Our data are consistent with recent views indicating that defects in trophoblast proliferation, differentiation, invasion, and plugging are associated with defective decidualization (299), decidual inflammation (300), and the disturbance in endometrial-trophoblast dialog during the peri-conception (301) period. Abnormal trophoblast invasion and plugging will subsequently lead to the aberrant onset of maternal circulation (273) and malperfusion, causing placental oxidative stress in preterm preeclampsia (175, 273, 302). On the other hand, maternal disease pathways induce mainly placental dysfunction without maldevelopment in term preeclampsia. This is substantiated by the differences observed in the maternal proteome, placental transcriptome, and trophoblastic DNA methylation between term and preterm preeclampsia in our study. Moreover, this is also consistent with the major differences between these two preeclampsia phenotypes in etiology (31), placental histopathology (28, 33, 44–46), and stress levels (303) as well as clinical presentation (2, 25, 26, 29, 304).
Our findings are very timely in the light of recent clinical research showing that the administration of aspirin before 16 weeks of gestation to pregnant women at risk for preeclampsia prevents the preterm phenotype of this syndrome (24, 305–309). Thus, the anti-inflammatory and anti-platelet actions of aspirin (308–311) may ameliorate the early pro-inflammatory disease pathways leading to placental maldevelopment in preterm preeclampsia. Based on our discovery of these novel disease pathways and their hub molecules, we propose a “molecular phase” of preeclampsia (Figure 12), where early pathologic events can already be detected by maternal blood biomarkers, offering non-invasive diagnostics of maternal and placental disease pathways. Biomarkers of these disease pathways may open new venues for the molecular characterization of patients destined to develop preeclampsia, using multi-biomarker profiles that support preventive approaches for patients with distinct preeclampsia phenotypes.
Materials and Methods
Placental Study
Placental Microarray Study
Study Groups and Clinical Definitions
Placental tissue and maternal blood samples were collected from Caucasian women at the First Department of Obstetrics and Gynaecology, Semmelweis University in Budapest, Hungary as described previously (132). Pregnancies were dated according to ultrasound scans between 8 and 12 weeks of gestation. Patients with multiple pregnancies or fetuses having congenital or chromosomal abnormalities were excluded. The collection and investigation of human clinical samples were approved by the Health Science Board of Hungary. Written informed consent was obtained from women prior to sample collection, and the experiments conformed to the principles set out in the World Medical Association Declaration of Helsinki and the Department of Health and Human Services Belmont Report. Specimens and data were stored anonymously.
Women were enrolled in the following groups: (1) preterm severe preeclampsia, with or without HELLP (hemolysis, elevated liver enzymes, low platelets) syndrome (n = 12) and (2) preterm controls (n = 5) (Table 1). Preeclampsia was defined according to the criteria set by the American College of Obstetricians and Gynecologists (1), and subdivided into preterm (<37 weeks) or term (≥37 weeks) groups. Severe preeclampsia was defined according to Sibai et al. (2). Preterm controls had no medical complications, clinical or histological signs of chorioamnionitis, and delivered neonates with a BW appropriate-for-gestational age (AGA) (312). SGA was defined as neonatal BW below the 10th percentile for gestational age. Cesarean delivery was performed in all cases due to severe symptoms as well as in all controls due to previous Cesarean delivery or malpresentation.
Placental Tissue and Maternal Blood Collection
Placental tissue specimens were processed immediately after delivery as described previously (132). For the microarray study, 1 × 1 cm villous tissue samples were excised from central cotyledons close to the umbilical cord to reduce the possible bias due to regional differences in gene expression (313, 314). These tissue blocks were then dissected from the choriodecidua on dry ice, snap-frozen, and stored at −80oC. For histopathologic evaluations, five representative tissue blocks were taken from each placenta to include central and peripheral cotyledons and the maternal side of the placenta with the fetal membranes. These blocks were embedded in paraffin after fixation in 10% neutral-buffered formalin (FFPE). Maternal blood samples were obtained at the time of admission into the delivery room; aliquots of maternal sera and plasma were stored at −80oC.
Histopathologic Evaluation of the Placentas
Placental specimens were examined according to a standard protocol, describing the topography and size of macroscopic lesions. Four micrometer sections were cut from the five FFPE blocks and mounted on SuperFrost/Plus slides (Fisherbrand, UK). After deparaffinization, slides were rehydrated, stained with hematoxylin and eosin, and examined in 10 randomly chosen microscopic fields using bright-field light microscopy by a pathologist blinded to the clinical information. Macroscopic and microscopic lesions were defined according to published criteria (315–317).
Placental Total RNA Isolation and Microarray Experiments
Tissues were homogenized using a ThermoSavant FastPrep FP120 Homogenizer (Thermo Scientific, Wilmington, DE, USA) with Lysing MatrixD (MP Biomedicals, Illkirch, France). Total RNA was isolated using RNeasy Fibrous Tissue Mini Kit (QIAGEN GmbH, Hilden, Germany), quantified with NanoDrop 1000 (Thermo Scientific), and assessed by Agilent 2100 Bioanalyzer (Matriks AS, Oslo, Norway). Total RNAs were labeled, and Cy3-RNAs were fragmented and hybridized to the Whole Human Genome Oligo Microarray G4112A (Agilent Technologies, Santa Clara, CA, USA) on an Agilent scanner, and processed with Agilent Feature Extraction software v9.5 according to the manufacturer’s guidelines.
Data Analysis
Demographics data were compared by the Fisher’s exact test and Mann–Whitney test using SPSS version 12.0 (SPSS Inc., Chicago, IL, USA). Microarray data analysis was performed using the R statistical language and environment1 following the MIAME guidelines and methodologies described previously (318). Microarray expression intensities were background corrected using the “minimum” method in the “backgroundCorrect” function of the “limma” package (319). After log2 transformation, data were quantile-normalized. From the 41,093 probesets on the array, 93 were removed before differential expression analysis, lacking annotation in the array definition file (Agilent Technologies). Subsequently, an expression filter was applied to retain probesets with intensity greater than log2(50) in at least two samples, yielding a final matrix of 30,027 probesets (15,939 unique genes). Differential gene expression was assessed using linear models including adjustment for batch effects, with coefficient evaluation via moderated t-tests. P-values were adjusted using the false discovery rate (FDR) method and Benjamini–Hochberg correction to compute q-values. Since there were no differences among patient groups in maternal age or ethnic background, we did not adjust for these parameters. Target gene Entrez IDs for the probesets were determined using the R package “hgu4112a.db.” For probesets without annotation in the package, Entrez IDs were taken from the array definition file (Agilent Technologies). Probesets remaining un-annotated (without Entrez ID and/or gene symbol) were removed from further analysis. Probesets were defined as DE (n = 1,409) if they had a q ≤ 0.2 and a fold-change ≥1.5 (Data S1 in Supplementary Material).
From the DE genes in preeclampsia, those encoding for proteins with functions in transcription regulation (n = 137) were identified using the Metacore (GeneGo Inc., Saint Joseph, MI, USA) and GeneCards v32 databases.
We downloaded the human U133A/GNF1H microarray data on 79 human tissues, cells, and cell lines from the Symatlas/BioGPS database (151) to identify human genes with predominant placental expression. A probeset was defined as having predominant placental expression if its placental expression was (1) ≥1,000 fluorescence units; (2) six times higher than the median value in 78 other tissue and cell sources; and (3) two times higher than its expression in the tissue with the second highest expression. The resulting 215 probesets corresponded to 153 unique genes. Eleven additional genes that were not present on the microarray platform used by Symatlas/BioGPS (Affymetrix, Santa Clara, CA, USA) were added to this list based on previously published evidence of placenta-specific expression (151–153) (Data S2 in Supplementary Material). Of the 164 predominantly placenta-expressed genes, 157 were present in our placental microarray data. These genes were tested for enrichment in DE genes compared to all genes on the array (1,409 of 15,939) using the Fisher’s exact test.
The expression levels of DE genes in EVT compared to VT lineages were analyzed by retrieving published microarray datasets (320–322) and reanalyzing expression data. Raw Affymetrix GeneChip Human Genome U133A 2.0 Array data from Bilban et al. (320) and Tilburgs et al. (322) was downloaded from GEO (GSE9773) and ArrayExpress (E-MATB-3217) respectively, and was processed with the “affy” (323) and “limma” (324) packages of Bioconductor.3 After RMA normalization, log-fold changes were calculated. Processed Illumina Human HT-12 V3 BeadArray data from Apps et al. (321) was downloaded from ArrayExpress (E-MATB-429), and then log-fold changes were calculated.
Chromosomal locations for all genes tested on the Agilent array were obtained from the R package “org.Hs.eg.db.” Of the 15,939 unique and 1,409 DE genes on the array, 15,935 and 1,408 could be assigned to chromosomes, respectively. Mapping the microarray probesets on the Affymetrix human U133A/GNF1H chips to ENTREZ identifiers was performed using the Bioconductor “hgu133a.db” and “hgfocus.db” packages (325, 326). Chromosomal locations of the resulting list of genes were obtained from the package “org.Hs.eg.db” and from the National Center for Biotechnology Information for the 11 additional genes (327). Enrichment analyses for chromosomes among predominantly placenta-expressed genes, DE genes and DE genes encoding for transcriptional regulators (Data S3–S5 in Supplementary Material) were tested by the Fisher’s exact test. Chromosomal locations of these genes were visualized by Circos (328).
Weighted gene co-expression network analysis (329, 330) was applied on the 1,409 DE genes across 17 samples to identify distinct regulatory modules and prioritize candidate genes for qRT-PCR validation. A gene pair-wise similarity (absolute Pearson correlation) matrix was first computed, then soft-thresholded by raising it to the power of 10 (chosen based on the scale-free topology criterion) to obtain an adjacency matrix. The topology overlap matrix (TOM) was then derived from the adjacency matrix. The topology overlap (331) measures the node interconnectedness within a network and was generalized to WGCNA (329). This measure defines the similarity between the two genes based on both correlations within themselves and outside other genes. Gene distance matrix was defined as 1-TOM and used for average linkage hierarchical clustering. A hybrid dynamic tree-cutting method (332) was applied to obtain modules (tree clusters).
Gene modules identified with this approach were further tested for enrichment in DE genes using the Fisher’s exact test. Transcription regulatory genes expressed at high levels (average log2 intensity >9) and co-expressed (absolute Pearson coefficient >0.7) with the most genes among DE genes were treated as candidates for hub genes in the module. Hub genes then were selected based on the number and strength of their Pearson co-expression partners as well as their and their networks’ biological activities. The networks of biological processes enriched among genes co-expressed with hub factors modules were created by BINGO and visualized with Cytoscape.
Enrichment analysis of transposable elements present in the 10,000 bp upstream region of DE genes was performed separately for the M1 (green) and the M2 (red) modules in preeclampsia versus all genes present on the microarray using the Fisher’s exact test. The locations of transposable elements and their families and classes were obtained from the “RepeatMasker” table of the UCSC Table Browser.4 P-values <0.05 were considered significant.
Placental Validation Study
Study Groups and Clinical Definitions
Third trimester placentas (n = 100) collected predominantly from African-American women were retrieved from the Bank of Biological Specimens of the Perinatology Research Branch (Detroit, MI, USA). Pregnancies were dated according to ultrasound scans between 8 and 12 weeks. Patients with multiple pregnancies or fetuses having congenital or chromosomal abnormalities were excluded. The use of biological specimens and clinical data for research purposes was approved by the Wayne State University Human Investigation Committee and the Institutional Review Board of the Eunice Kennedy Shriver National Institute of Child Health and Human Development (NICHD, NIH, DHHS). Written informed consent was obtained from women prior to sample collection, and the experiments conformed to the principles set out in the World Medical Association Declaration of Helsinki and the Department of Health and Human Services Belmont Report. Specimens and data were stored anonymously.
The following homogenous patient groups were selected from a cohort: (1) preterm severe preeclampsia (n = 20); (2) preterm severe preeclampsia associated with SGA (n = 20); (3) preterm controls (n = 20); (4) term severe preeclampsia (n = 10); (5) term severe preeclampsia associated with SGA (n = 10); and (6) term controls (n = 20). Term controls, consisting of normal pregnant women with (n = 10) or without (n = 10) labor, and preterm controls with preterm labor and delivery had no medical complications or clinical or histological signs of chorioamnionitis, and delivered an AGA neonate (333) (Table 2; Figure S2 in Supplementary Material).
Labor was defined by the presence of regular uterine contractions at a frequency of at least two contractions every 10 min with cervical changes resulting in delivery (334). Preeclampsia was defined according to the criteria set by the American College of Obstetricians and Gynecologists (1) and was subdivided into preterm (<37 weeks) or term (≥37 weeks) groups. Severe preeclampsia was defined according to Sibai et al. (2). SGA was defined as neonatal BW below the 10th percentile for gestational age (333). Cesarean delivery was performed in cases due to severe symptoms and in controls due to previous Cesarean delivery or malpresentation.
Placental Tissue Collection
Third trimester placental tissue specimens (n = 100) were processed immediately after delivery. For histopathologic evaluations, representative tissue blocks were taken from each placenta to include central and peripheral cotyledons, maternal side of the placenta, fetal membranes, and the umbilical cord. These blocks were embedded in paraffin after fixation in 10% neutral-buffered formalin (FFPE). Villous tissue blocks from central cotyledons were selected for TMA. For gene expression studies, systematic random sampling (335) was used to obtain villous tissues to reduce the possible bias due to regional gene expression differences (313, 314). Excised tissue blocks were homogenized and mixed in TRIzol reagent (Life Technologies), snap-frozen with liquid N2, and stored at −80oC.
Histopathologic Evaluation of the Placentas
Placental specimens (n = 100) were examined according to a standard protocol, describing the topography and size of macroscopic lesions. Five µm sections were cut from the representative FFPE tissue blocks, stained with hematoxylin and eosin, and examined using bright-field light microscopy by two anatomic pathologists blinded to the clinical information. Histopathologic changes of the placenta were defined according to published criteria proposed by the Perinatal Section of the Society for Pediatric Pathology (336). “Maternal vascular malperfusion score” was calculated by summing the number of pathologic lesions consistent with this lesion category (46, 336) present in a given placenta.
Placental Total RNA Isolation and qRT-PCR
Total RNA was isolated from snap-frozen third trimester placental villous tissues (n = 100) with TRIzol reagent (Life Technologies) and RNeasy kit (QIAGEN, Valencia, CA, USA) according to the manufacturers’ recommendations. The 28S/18S ratios and the RNA integrity numbers were assessed using an Agilent Bioanalyzer 2100, and RNA concentrations were measured with NanoDrop 1000. Total RNA (500 ng) was reverse transcribed with the High Capacity cDNA Reverse Transcription Kit using random hexamers (Applied Biosystems, Foster City, CA, USA). TaqMan Assays (Applied Biosystems; Table 3, Data S17 in Supplementary Material) were used for high-throughput gene expression profiling on the Biomark qRT-PCR system (Fluidigm, San Francisco, CA, USA) according to the manufacturers’ instructions.
Placental TMA Construction, Immunohistochemistry, and Immunoscoring
Tissue microarrays were constructed from central tissue blocks of third trimester FFPE placentas (n = 100) as described earlier (337). Briefly, three 20 × 35 mm recipient blocks were made of Paraplast X-Tra tissue embedding media (Fisher Scientific, Pittsburgh, PA, USA). One mm diameter cores from tissue blocks were transferred in triplicate into recipient paraffin blocks using an automated tissue arrayer (Beecher Instruments, Inc., Silver Spring, MD, USA). Five µm sections cut from TMAs were placed on silanized slides and stained with antibodies and reagents (Data S18 in Supplementary Material) either on Ventana Discovery or Leica BOND-MAX autostainers.
Tissue microarray immunostainings were semi-quantitatively scored by three examiners blinded to the clinical information with an immunoreactive score modified from a previously published one (154). Immunostaining intensity was graded as follows: 0 = negative, 1 = weak, 2 = intermediate, and 3 = strong. All villi in a random field of each of the three cores were evaluated by all examiners, and scores within each core were averaged to represent the target protein quantity of that core. Thus, each placenta had three scores corresponding to the three cores examined.
Data Analysis
Demographics data were compared by the Fisher’s exact test and Mann–Whitney test using SPSS version 12.0 (SPSS). All other data were analyzed in the R statistical environment (see text footnote 1).
Placental qRT-PCR. Data were analyzed using the ΔΔCt method. Data were first normalized to the reference gene (RPLP0), and the batch effect was adjusted through calibrator samples. Log2 mRNA relative concentrations were obtained for each sample as −ΔCt(gene) = Ct(RPLPO) − Ct(gene). The surrogate gene expression values (−ΔCtgene) were used to perform a hierarchical clustering with 1-Pearson correlation distance and average linkage. Between-group comparisons (in which groups were predefined based on the clinical characteristics of the patients) were performed by fitting a linear model on −ΔCt values, using the group variable indicator and the maturity status of the fetus (term vs. preterm) as covariates while allowing for an interaction effect (Figure 4; Figure S3 in Supplementary Material).
Histopathology. The association between qRT-PCR gene expression and the “maternal vascular malperfusion” score was tested using a linear model. P-values of <0.05 were considered significant.
TMA Immunoscoring. Group comparisons using immunoscores were conducted in the same way as for the qRT-PCR data (Figure 4).
Correlation of Clinical Parameters and Placental Gene Expression
Data Analysis
To reveal whether the expression of any gene on the microarray was correlated with MAP while controlling for BW, a linear model (y~MAP + BW + Batch) was fit for every gene on the array, in which y represents gene expression, and the dependent variables represent MAP, BW, and batch, respectively. A moderated t-test was used to obtain p-values, which then were adjusted using the FDR method and Benjamini–Hochberg correction for multiple testing. Significance was determined using a q ≤ 0.2. Gene modules were also tested for the enrichment in genes with their expression correlated with MAP using the Fisher’s exact test (Data S6 in Supplementary Material).
In the qRT-PCR validation study, we extended our analysis to include all 100 patients to test for the association between gene expression and mean arterial BP as well as BW percentile while adjusting for gestational age. All variables were treated as continuous. P-values of <0.05 were considered significant (Figure 4).
Genomic DNA Methylation Analysis of the Trophoblast
Laser Capture Microdissection
Fifteen µm sections were cut from snap-frozen placentas that were also used for qRT-PCR expression profiling (n = 100) on 2 µm Glass Foiled PEN slides (Leica Microsystems). The trophoblast layer of 300–350 villi in each specimen was laser captured by a Leica DM6000B microscope (Leica Microsystems) into 0.5 ml microcentrifuge tubes. The captured material was digested with Proteinase K (PicoPure DNA Extraction Kit, Applied Biosystems) at 56°C by overnight incubation. Digestions were stopped at 95°C, and samples were stored at −70°C until DNA isolation.
Genomic DNA Isolation
Genomic DNA was isolated from laser captured VTs (n = 100), from primary VTs (n = 3) collected for functional studies described below, and from respective umbilical cord blood leukocytes (n = 3) taken from the same pregnancies. The EZ1 Advanced Nucleic Acid Isolation System using EZ1 DNA Tissue and EZ1 DNA Blood Kits (QIAGEN) were utilized for DNA isolation, and DNA samples were quantified with Quantifiler Human DNA Quantification Kit (Applied Biosystems) according to the manufacturers’ instructions.
Primer Design and Validation
The methylation status of CpGs in human H1 embryonic stem cells, H1-derived trophoblast cultured cells, and H1-derived neuronal progenitor cultured cells obtained by whole-genome shotgun bisulfite sequencing (University of California, San Diego, USA; UCSD Human Reference Epigenome Mapping Project) were visualized by the Epigenome Browser5 and used for the selection of regions of interest. Primer design, targeted amplification, and sequencing were conducted using the targeted sequencing service protocol of Zymo Research Corporation. For targeted bisulfite sequencing, 30 primer pairs were designed and validated. Primers were synthesized by Integrated DNA Technologies, Inc., (Coralville, IA, USA) and underwent quality control, which included duplicate testing for specific amplification of 1 ng bisulfite DNA using bisulfite converted human DNA. Quality control criteria included robust and specific amplification (Cp values <40 cycles and CV <10% for duplicates) of the bisulfite primers on a LightCycler 480 real-time qRT-PCR instrument (Roche Diagnostics Corp. Indianapolis, IN, USA).
Bisulfite Conversion, Multiplex Amplification, Bar-Coding and Adapterization PCR, and Next-Generation Sequencing
Genomic DNA samples from laser captured VTs, primary VTs, and umbilical cord blood cells as well as control samples were subjected to sodium bisulfite treatment using the EZ DNA Methylation-Direct Kit (Zymo Research Corporation). For non-methylated control, human DNA was extracted and purified with Quick-gDNA Miniprep Kit (Zymo Research Corporation) from the HCT116 cell line (American Type Culture Collection, Manassas, VA, USA), which is double knock-out for both DNA methyltransferases DNMT1 (−/−) and DNMT3b (−/−), and thus contains a low level (<5%) of DNA methylation. For methylated control, human DNA was purified similarly from the HCT116 cell line and was enzymatically methylated at all cytosine positions comprising CG dinucleotides by CpG Methylase (Zymo Research Corporation).
Bisulfite-treated samples and 30 validated primer pairs were subjected to targeted amplification on the 48.48 Access Array System (Fluidigm), using the targeted sequencing service protocol of Zymo Research Corporation. Fluidigm’s protocols were used for sample loading, harvesting, and pooling, 1:100 dilution of amplicon pools for each sample, and for amplification using barcoded adapter-linkers (Fluidigm). Reactions were cleaned up using the DNA Clean and Concentrator-5 (Zymo Research Corporation), and products were normalized by concentration and pooled. The sequencing library was denatured, diluted, and sequenced with a 150-base paired-end run on the MiSeq Benchtop Sequencer (Illumina) according to Illumina’s protocols.
Sequence Alignment and Data Analysis
Sequence reads from bisulfite-treated libraries were identified using standard Illumina base-calling software, and then analyzed using a Zymo Research Corporation proprietary analysis pipeline. Residual cytosines (Cs) in each read were first converted to thymines (Ts), with each such conversion noted for subsequent analysis. Reads were aligned by Bismark, a Bowtie-based alignment tool for bisulfite converted reads.6 The number of mismatches in the induced alignment was then counted between the unconverted read and reference, ignoring cases in which a T in the unconverted read was matched to a C in the unconverted reference. For a given read, only the best scored alignment was kept. If there were more than one best read, then only one was kept arbitrarily. The methylation level of each sampled cytosine was estimated as the number of reads reporting a C, divided by the total number of reads reporting a C or T. CpGs with coverage of less than four reads were removed from the analysis. The developed sensitive and robust bisulfite sequencing assays yielded a median total sequencing read of 533 (range: 30–1,725) per CpG in the trophoblast-fetal blood cell comparison and a median total sequencing read of 136 (range: 4–2,609) per CpG in the clinical sample comparison.
Multiple sequencing counts (total and methylated) were summed for each sample at each CpG site, and samples with a total count <4 were dropped from the analysis. The mean methylation ratio in each group was computed for genomic visualization. In the comparison of methylation levels between trophoblasts and cord blood cells, a group sample size of two was considered as a minimum. In order to fit the count data, we used a generalized linear model of Poisson distribution with log link. When all samples in any of the two groups being compared had zero methylation counts, the maximum likelihood estimation of the Poisson model went to infinity. In such cases, the Student’s t-test was used alternatively. P-values and the group difference in methylation ratios were included above the bar plots in Figures S9 and S12 in the Supplementary Material. For comparisons of methylation levels between the clinical groups, only comparisons with a minimum group sample size of four were considered, and the Wilcoxon rank-sum test was used. P-values and the group difference in methylation ratios were included above the bar plots in Figure 11 as well as Figures S10 and S13 in the Supplementary Material. Differential methylation was considered to be mild, moderate, or strong when the p-value was <0.05 and the difference in methylation ratios was ≥0.125, ≥0.25, or ≥0.5, respectively. The correlation between methylation levels on each CpG in clinical samples and various demographical, clinical, or histopathological variables were evaluated by Kendall’s tau statistics. Correlation coefficients and p-values were plotted in the scatter plots. A Kendall’s tau p < 0.05 was considered significant.
Maternal Study
“Virtual” Liquid Biopsy of the Placenta in Preterm Preeclampsia
Sample Collection
First trimester placentas and maternal blood samples were collected from healthy Caucasian women undergoing termination of pregnancy (n = 12), and processed at the Maternity Clinic and Semmelweis University in Budapest, Hungary. Villous tissues were dissected from the choriodecidua on dry ice and stored at −80oC. Aliquots of maternal sera and plasma were stored at −80oC.
Pregnancies were dated according to ultrasound scans between 5 and 13 weeks of gestation. Patients with multiple pregnancies were excluded. The collection and investigation of human clinical samples were approved by the Health Science Board of Hungary. Written informed consent was obtained from women prior to sample collection, and the experiments conformed to the principles set out in the World Medical Association Declaration of Helsinki and the Department of Health and Human Services Belmont Report. Specimens and data were stored anonymously.
Placental Total RNA Isolation and qRT-PCR
Total RNA was isolated from snap-frozen first trimester placental villous tissues (n = 12) with Direct-zol RNA MiniPrep Kit (Zymo Research Corporation) according to the manufacturer’s recommendations. The 28S/18S ratios and the RNA integrity numbers were assessed using an Agilent Bioanalyzer 2100 (Agilent Technologies), and RNA concentrations were measured with NanoDrop 1000. Total RNA (500 ng) was reverse transcribed with the qScript cDNA Synthesis Kit (Quanta Biosciences, Gaithersburg, MD, USA). TaqMan Assays (Applied Biosystems; Data S17 in Supplementary Material) were used for expression profiling on the StepOnePlus Real-Time PCR System (Applied Biosystems).
Enzyme-Linked Immunosorbent Assays
Concentrations of leptin and human placental lactogen (hPL) in first (n = 12) and third (n = 19) trimester maternal blood samples were measured with sensitive and specific immunoassays (Leptin ELISA Kit, Abnova, Taipei City, Taiwan; Human Placental Lactogen ELISA Kit, Alpco, Salem, NH, USA) according to the manufacturers’ instructions. Standard curves were generated, and sample assay values were extrapolated. The sensitivities of the assays were <4.4 ng/ml (leptin) and <550 ng/ml (hPL).
Correlation Analysis of Placental Gene Expressions and Maternal Plasma Protein Concentrations
Placental gene expression was measured with either microarray on third trimester samples or qRT-PCR on first trimester samples as described above. Maternal plasma protein concentrations were measured with the above-described immunoassays on respective blood samples taken from the same patients on the day of either delivery or termination of pregnancy. Correlations between placental gene expression and maternal plasma concentrations of gene product proteins were calculated with the Pearson method and visualized on scatter plots (Figure S4 in Supplementary Material).
Publication Search, Database Build, and “Virtual” Liquid Biopsy of the Placenta
To detect disease-associated protein signatures of placental dysfunction in maternal blood, similar to plasma DNA tissue mapping for noninvasive prenatal, cancer, and transplantation assessments (176), we performed “virtual” liquid biopsy of the placenta. Briefly, human microarray data on 79 human tissues and cells were downloaded from the BioGPS database, which was used for the generation of placenta enrichment scores (placental expression/mean expression in 78 other tissues and cells). Five genes (CGB3, CSH1, ENG, FLT1, and LEP) with enrichment scores between 1.4 and 1,490 were selected based on a literature search due to the extensive investigations of their gene products in maternal blood in preeclampsia (Figure S5 in Supplementary Material). Next, an extensive PubMed search was conducted for first trimester maternal blood protein measurements of these five gene products in patients who developed preeclampsia later in pregnancy. Altogether, 61 scientific reports were identified that met the inclusion criteria, which contained data for 80,170 measurements (35, 61, 82, 88, 126, 178–233). These reports were used to build a database for the “virtual” liquid biopsy. In this database, biomarker levels in preterm preeclampsia were expressed as the percentage of control levels as a function of gestational age. Then, the correlations of control percentage values with gestational age were evaluated using the Pearson method. Scatterplots were used to visualize data (Figure S5 in Supplementary Material; Figure 5).
Maternal Serum Proteomics Discovery Study
Study Groups, Clinical Definitions, and Sample Collection
Women were enrolled in a prospective, longitudinal, and multicenter study (196) in prenatal community clinics of the Maccabi Healthcare Services, Israel. Pregnancies were dated according to the last menstrual period and verified by first trimester ultrasound. Patients with multiple pregnancies or fetuses having congenital or chromosomal abnormalities were excluded. The collection and investigation of human clinical samples were approved by the Maccabi Institutional Review Board. Written informed consent was obtained from women prior to sample collection, and the experiments conformed to the principles set out in the World Medical Association Declaration of Helsinki and the Department of Health and Human Services Belmont Report. Specimens and data were stored anonymously.
Preeclampsia was defined according to the criteria set by the International Society for the Study of Hypertension in Pregnancy (338) and was subdivided into preterm (<37 weeks) or term (≥37 weeks) groups. Severe preeclampsia was defined by Sibai et al. (2). SGA was defined as neonatal BW below the 10th percentile for gestational age. Healthy controls had no medical or obstetric complications and delivered a neonate with a BW appropriate for gestational age.
Peripheral blood samples were obtained by venipuncture in the first trimester from women who subsequently developed preterm severe preeclampsia (n = 5) and term severe preeclampsia (n = 5) as well as healthy controls (n = 10) matched for gestational age at blood draw (Table 4). Blood samples were allowed to clot and were then centrifuged at 10,000 × g for 10 min to separate and collect sera. Serum samples were stored in aliquots at −20oC in the Maccabi Central Laboratory until shipped on dry ice to Hungary.
Sample Preparations, Immunodepletion of High-Abundance Serum Proteins
Sera were immunodepleted at Biosystems International Ltd., (Debrecen, Hungary) for 14 highly abundant serum proteins on an Agilent 1100 HPLC system using Multiple Affinity Removal LC Column-Human 14 (Agilent Technologies) according to the manufacturer’s protocol. To improve the resolution of 2D gels, immunodepleted serum samples were lyophilized, delipidated, and salt-depleted at the Proteomics Laboratory of the Eotvos Lorand University (Budapest, Hungary) (339). The delipidated and salt-depleted plasma protein samples were dissolved in lysis buffer, and their pH was adjusted to 8.0.
Fluorescent Labeling and 2D-DIGE
Protein concentrations of the immunodepleted, desalted, and delipidated serum samples were between 2 and 4 µg/µl as determined with PlusOne 2D Quant Kit (GE Healthcare, Little Chalfont, United Kingdom). Samples were equalized for protein content, and then 5 µg of each protein sample was labeled with a CyDye DIGE Fluor Labeling kit for Scarce Samples (saturation dye) (GE Healthcare) according to the manufacturer’s instructions. Individual samples from cases (n = 2 × 5) and controls (n = 2 × 5) were labeled with Cy5. An internal standard reference sample was pooled from equal amounts (2.5 µg) of all individual samples in this experimental set and was labeled with Cy3. Then, 5 µg of each Cy5-labeled individual sample was merged with 5 µg of the Cy3-labeled reference sample, and these 20 mixtures were run in 2 × 10 gels simultaneously. Briefly, labeled proteins were dissolved in isoelectric focusing (IEF) buffer and were rehydrated passively onto 24 cm immobilized non-linear pH gradient (IPG) strips (pH 3–10, GE Healthcare) for at least 14 h at room temperature. After rehydration, the IPG strips were subjected to the first dimension of IEF for 24 h to attain a total of 80 kVh. Focused proteins were reduced by equilibrating with a buffer containing 1% mercaptoethanol for 20 min. After reduction, IPG strips were loaded onto 10% polyacrylamide gels (24 × 20 cm) and SDS-PAGE was conducted at 12W/gel in the second dimension. Then, gels were scanned in a Typhoon TRIO + scanner (GE Healthcare) using appropriate lasers and filters with the photomultiplier tube biased at 600V. Images in different channels were overlaid using selected colors, and the differences were visualized using Image Quant software (GE Healthcare). Differential protein expression analysis was performed using the differential in-gel analysis and biological variance analysis (BVA) modules of the DeCyder 6.0 software package (GE Healthcare).
Identification of DE Protein Spots
The internal standard reference sample representative of every protein present in all experiments was loaded equally in all gels and thus provided an average image for the normalization of individual samples. The determination of the relative abundance of the fluorescent signal between internal standards across all gels provided standardization between the gels, removing experimental variations and reducing gel-to-gel variations. According to the standard proteomic protocol, the threshold for differential expression was set at 1.05-fold minimum fold-change (340). A p-value was determined for each protein spot using the Student’s t-test by the BVA module of the DeCyder software. A p-value of <0.05 was considered statistically significant.
Sample Preparation, Fluorescent Labeling, and Preparative 2D-DIGE
The density of spots in the case of Colloidal Coomassie Blue labeling depends only on the concentration of protein in the sample; however, the density of the spots in the case of saturation dye labeling also depends on the number of cysteines of the labeled proteins because the saturation dye labeling method labels all available cysteines on each protein. This results in the same pattern with different density among samples on the analytical and the preparative gels, rendering identification more difficult. To eliminate this problem for the exact identification of proteins in spots of interest, preparative 2D gel electrophoresis was performed using CyDye saturation fluorescent labeling and Colloidal Coomassie Blue labeling in the same gel. A total of 800 µg of proteins per each of the two gels was run. Following electrophoresis, gels were scanned in a Typhoon TRIO + scanner as described above, the significantly altered spots were matched among the “master” analytical and the fluorescent preparative gel images using the BVA module of the DeCyder 6.0 software package. The resolved protein spots were visualized by the Colloidal Coomassie Blue G-250 staining protocol. Individual spots of interest were excised from the gels based on the comparison of the matched images.
In-Gel Digestion, Liquid Chromatography–Tandem Mass Spectrometry (LC–MS/MS)
The excised protein spots were analyzed at the Proteomics Research Group of the Biological Research Center of the Hungarian Academy of Sciences (Szeged, Hungary); the detailed protocol is described in http://ms-facility.ucsf.edu/ingel.html. Briefly, salts, SDS, and Coomassie brilliant blue dye were washed out, disulfide bridges were reduced with dithiothreitol, and then free sulfhydryls were alkylated with iodoacetamide. Digestion with side-chain protected porcine trypsin (Promega, Madison, WI, USA) proceeded at 37°C for 4 h. The resulting peptides were extracted from the gel using 1% formic acid in 50% acetonitrile; then the samples were dried down and dissolved in 0.1% formic acid.
Samples were analyzed on an Eldex nanoHPLC system online coupled to a 3D ion trap tandem mass spectrometer (LCQ Fleet, Thermo Scientific) in “triple play” data-dependent acquisition mode, where MS acquisitions were followed by CID analyses on computer-selected multiply charged ions. HPLC conditions included in-line trapping onto a nanoACQUITY UPLC trapping column (Symmetry, C18 5 µm, 180 µm × 20 mm; and 15 µl/min with 3% solvent B) followed by a linear gradient of solvent B (5–60% in 35 min, flow rate: 300 nl/min, where solvent A was 0.1% formic acid in water and solvent B was 0.1% formic acid in acetonitrile) on a Waters Atlantis C18 Column (3 µm, 75 µm × 100 mm).
Database Search and Data Interpretation
Raw data files were converted into Mascot generic files (*.mgf) with the Mascot Distiller software v2.1.1.0 (Matrix Science Inc, London, UK). The resulting peak lists were searched against a human subdatabase of the non-redundant protein database of the NCBI (NCBInr, Bethesda, MD, USA) in MS/MS ion search mode on an in-house Mascot server v2.2.04 using Mascot Daemon software v2.2.2 (Matrix Science Inc). Monoisotopic masses with peptide mass tolerance of ±0.6 Da and fragment mass tolerance of ±1 Da were submitted. Trypsin with up to two missed cleavages was specified as an enzyme. Carbamidomethylation of cysteines was set as fixed modification, and acetylation of protein N-termini, methionine oxidation, and pyroglutamic acid formation from peptide N-terminal glutamine residues were permitted as variable modifications. Acceptance criterion was set to at least two significant (peptide score >40, p < 0.05) individual peptides per protein. Localization of identified peptides in the core protein sequences was visually analyzed in order to identify potential protein split products.
Biological functions of the altered serum proteins were retrieved from Pathway Studio 9.0 software (Ariadne Genomics Inc., Rockville, MD, USA), and from the open-access GO database7 (Figure 6; Figure S6 and Data S8 in Supplementary Material). To elucidate possible interactions between the altered serum proteins and placental genes in the microarray data, bioinformatics analysis was performed using the same software. Molecular networks between the changed serum proteins in preterm (n = 19) or term preeclampsia (n = 14) and DE placental genes annotated in the GO database (n = 1,142) were built separately with a non-linear literature processing search engine, and the resulting connections were manually validated by reading full-text publications (Figure 6; Figure S6 in Supplementary Material). The Fisher’s exact test was used to test for the enrichment of the connections between the altered serum proteins and (1) DE genes in individual modules, taking the connections between the proteins and DE genes in all modules as a background; and (2) DE placental genes, taking the connections between the proteins and all genes tested on the array as a background. To reveal the pathways enriched among the DE genes connected to angiotensinogen, the Ingenuity Pathway Analysis software (QIAGEN, Redwood City, Redwood City, CA, USA) was used, which utilizes Fisher’s exact test and Benjamini–Hochberg correction for multiple testing for the analyses. Statistical significance was set at q < 0.01 (Data S10 in Supplementary Material).
Maternal Serum Proteomics Validation Pilot Study
Study Groups, Clinical Definitions, and Sample Collection
Caucasian women were enrolled in a prospective study at the Department of Obstetrics and Gynaecology of the University of Debrecen and at the Andras Josa Teaching Hospital in Nyiregyhaza, Hungary. Pregnancies were dated according to ultrasound scans between 8 and 14 weeks of gestation. Patients with multiple pregnancies or fetuses having congenital or chromosomal abnormalities were excluded. The collection and investigation of human clinical samples were approved by the Regional Ethics Committee of the University of Debrecen. Written informed consent was obtained from women prior to sample collection, and the experiments conformed to the principles set out in the World Medical Association Declaration of Helsinki and the Department of Health and Human Services Belmont Report. Specimens and data were stored anonymously.
Women were included in the following groups: (1) preterm severe preeclampsia with SGA (n = 5) and (2) controls (n = 10). Women were matched for gestational age at blood draw (Table 5). Preeclampsia was defined according to the criteria set by the American College of Obstetricians and Gynecologists (1) and was subdivided into preterm (<37 weeks) or term (≥37 weeks) groups. Severe preeclampsia was defined according to Sibai et al. (2). SGA was defined as neonatal BW below the 10th percentile for gestational age. Healthy controls had no medical or obstetric complications and delivered a neonate with a BW appropriate for gestational age.
Peripheral blood samples were obtained by venipuncture in the first trimester. Plasma samples were separated by double centrifugation for 10 min. Samples were stored in aliquots at −80oC.
Sample Preparations
All solvents were HPLC-grade from Sigma-Aldrich (St. Louis, MO, USA) and all chemicals, where not stated otherwise, were obtained from Sigma-Aldrich. Frozen plasma samples were thawed and denatured with denaturing buffer (Biognosys AG; Schlieren, Switzerland). Samples were alkylated using alkylation solution (Biognosys). Subsequently, samples were digested overnight with sequencing grade modified trypsin (Promega; Madison, WI, USA) at a protein:protease ratio of 50:1. C18 cleanup for mass spectrometry was carried out according to the manufacturer’s instructions using C18 Micro Spin columns (Nest Group Inc.; Southborough, MA, USA). Peptides were dried down to complete dryness using a SpeedVac system. Dried peptides were redissolved with LC solvent A (1% acetonitrile in water with 0.1% formic acid). Final peptide concentrations were determined for all samples by 280 nm measurement (SpectrostarNANO, BMG Labtech, Offenburg, Germany). Samples were spiked with PlasmaDive™ (Biognosys) reference peptides mix at known concentrations.
LC–MRM Measurements and Data Analysis
Peptides (1 µg per sample, corresponding to injection of 0.0259 µl of initial plasma sample) were injected to a self-packed C18column [75 µm inner diameter and 10 cm column length, New Objective (Woburn, MA, USA); column material was Magic AQ, 3 µm particle size, and 200Å pore size from Michrom] on a ThermoScientific EASY-nLC1000 nano-liquid chromatography system. LC–MRM assays were measured on a ThermoScientific TSQ Vantage triple quadrupole mass spectrometer equipped with a standard nano-electrospray source. The LC gradient for LC–MRM was 6–40% solvent B (85% acetonitrile in water with 0.1% formic acid) for 30 min followed by 40–94% solvent B for 2 min and 94% solvent B for 8 min (total gradient length was 40 min). A subset of Biognosys’ PlasmaDive™ MRM Panel of 10 peptides representing 10 proteins (Data S9 in Supplementary Material) was used for the measurements of 10 altered proteins involved in immune responses. For the quantification of the peptides across samples, the TSQ Vantage was operated in scheduled MRM mode with an acquisition window length of 5 min. The LC eluent was electrosprayed at 1.9 kV and Q1/Q3 were operated at unit resolution (0.7 Da). Signal processing and data analysis were carried out using SpectroDive™ 8.0—Biognosys’ software for multiplexed MRM/PRM data analysis based on mProphet (341). A q-value filter of 1% was applied.
Because of the small sample size of this pilot study, statistical simulations were carried out to predict power and significance in the extended validation study by multiple permutation testing. The probability of significant differences between the two groups was estimated by a paired t-test and the Mann–Whitney test. For both, p < 0.05 was taken as criteria to count successful simulations. The number of successful simulations was calculated for different sample sizes (n = 10 or 100) and repeats of simulations (n = 10, 100, or 1,000). The simulated significance level at p < 0.05 was accepted if the number of successful simulations was >25% (Figure 6; Data S9 in Supplementary Material).
Publication Search
An extensive PubMed search was conducted for first trimester maternal blood protein measurements of the DE proteins found by 2D-DIGE. Altogether five scientific reports were identified that met the inclusion criteria (126, 143, 239–241). Figure 6 depicts biomarkers with the same direction differential abundance in preterm preeclampsia in published data as in 2D-DIGE assays.
Trophoblast Study
In Vitro Modeling of Placental Disease Pathways
Database Search and Data Analysis
To build optimal in vitro cellular models of trophoblastic disease, an extensive PubMed search was first conducted for similar assays. Since no human trophoblastic stems cells were yet available that would enable a natural proliferative trophoblastic pool with differentiation potential into villous or extravillous lineages, choriocarcinoma-derived trophoblastic cell lines or immortalized EVTs were mostly used for such purposes. Our search revealed BeWo cells (342) and HTR8/SVneo cells (253) as the increasingly most accepted cell model systems based on 1,500 published articles.
Primary VT Differentiation
For in vitro trophoblast experiments, placentas (n = 6) were collected prospectively at the Perinatology Research Branch (NICHD, NIH, DHHS) from normal pregnant women at term who delivered an AGA neonate with Cesarean section. Cytotrophoblasts were isolated from these placentas by the modified method of Kliman et al. (343). Briefly, 100 g villous tissues were cut, rinsed in PBS, and sequentially digested with Trypsin (0.25%; Life Technologies, Grand Island, NY, USA) and DNAse I (60 U/ml; Sigma-Aldrich) for 90 min at 37oC. Dispersed cells were filtered through 100 µm Falcon nylon mesh cell strainers (BD Biosciences, San Jose, CA, USA), and then erythrocytes were lysed with 5 ml NH4Cl solution (Stemcell Technologies, Vancouver, BC, Canada). Washed and resuspended cells were layered over 20–50% Percoll gradients and centrifuged for 20 min at 1,200 × g. Trophoblast containing bands were collected and non-trophoblastic cells were excluded by negative selection using anti-CD9 (20 µg/ml) and anti-CD14 (20 µg/ml) mouse monoclonal antibodies (R&D Systems, Minneapolis, MN, USA), MACS anti-mouse IgG microbeads, and MS columns (Miltenyi Biotec, Auburn, CA, USA). Then, primary VTs were plated on a collagen-coated 12-well plate (BD Biosciences; 3 × 106 cells/well) in Iscove’s modified Dulbecco’s medium (IMDM; Life Technologies) supplemented with 10% fetal bovine serum (FBS) and 1% penicillin/streptomycin (P/S). To test the effect of trophoblast differentiation on selected genes’ expression, primary trophoblasts were kept in IMDM containing 5% non-pregnant human serum (SeraCare, Milford, MA, USA) and 1% P/S. The medium was replenished every 24 h, and cells were harvested for total RNA every 24 h between days 1 and 7.
The Effect of Maternal Serum on Trophoblastic Gene Expression
Villous trophoblasts were isolated from normal term placentas and plated as described above. To test the effect of preeclampsia serum on VTs at two time points of differentiation, VTs were kept in IMDM containing 1% P/S and 10% first trimester maternal sera from control or preeclamptic women. Medium was replenished after 48 h, and cells were harvested for total RNA either 24 or 72 h after the start of the human serum treatment. All experiments were run in triplicate.
The Effect of Oxygen Levels, BCL6, and ARNT2 Overexpression on Trophoblastic Gene Expression
BeWo cells (American Type Culture Collection) were incubated in a T-25 flask or 6-well plate with Ham’s F12-K medium (Life Technologies) supplemented with 10% FBS and 1% P/S in a humidified incubator (5% CO2, 20% O2) at 37oC until reaching 50–80% confluence. To test the effect of ARNT2 or BCL6 overexpression on gene expression, cells were transiently transfected with ARNT2, BCL6, or control (GFP) vectors. Briefly, 4 µg expression plasmid (OriGene Technologies, Inc., Rockville, MD, USA) and 12 µl FuGENE HD transfection reagent (Promega) were mixed with 180 µl Ham’s F12-K medium (10% FBS, 1% P/S), incubated at RT for 15 min and added to cell cultures with 1.8 ml medium in each well of 6-well plate. Twenty-four hours after transfection, cells were split into three treatment groups and kept under either normoxic (20% O2), hypoxic (2% O2), or ischemic (1% and 20% O2 alternating for 6 h) conditions in an Oxycycler C42 (BioSpherix, Lacona, NY, USA) for 48 h before cell harvest. This study setup followed the generally accepted view in reproductive sciences that ~2% O2 concentration represents physiologic hypoxia at the implantation site and that placental development occurs under physiologic hypoxia in the first trimester (242, 344).
Total RNA Isolation and qRT-PCR
Total RNA was isolated from primary VTs on days 0–7 of differentiation and from BeWo cell cultures with TRIzol reagent (Life Technologies) and RNeasy kit (QIAGEN) according to the manufacturers’ recommendations. The 28S/18S ratios and the RNA integrity numbers were assessed using an Agilent Bioanalyzer 2100; RNA concentrations were measured with NanoDrop 1000. Total RNA (500 ng) was reverse transcribed with High Capacity cDNA Reverse Transcription Kit using random hexamers (Applied Biosystems). TaqMan Assays (Applied Biosystems; Data S17 in Supplementary Material) were used for high-throughput gene expression profiling on the Biomark qRT-PCR system (Fluidigm) according to the manufacturers’ instructions.
Data Analysis
qRT-PCR. Data were analyzed using the ΔΔCt method. Data were first normalized to the reference gene (RPLP0), and log2 mRNA relative concentrations were obtained for each sample as −ΔCt(gene) = Ct(RPLPO) − Ct(gene).
Primary Trophoblast Differentiation. The overall changes in gene expression during the 7 days of differentiation were analyzed by comparing the mean expressions on a given day versus Day 0. The highest fold change for a given gene was defined as the maximum of the daily expression differences during the 7-day time-period. Significant differences were defined by a paired t-test (p < 0.05) (Figure 7).
Serum Treatment of Primary Trophoblast. Gene expression data were analyzed using the Student’s t-test to compare the effect of preeclampsia serum with the effect of control serum on gene expression at Days 1 and 3 of trophoblast differentiation. P-values of <0.05 were considered significant (Figure 6).
BeWo Cell Transfections. Gene expression data were analyzed to compare the effect of ARNT2 or BCL6 overexpression with the effect of control vector overexpression on gene expression in normoxic conditions using a one-way ANOVA model. The same model was used to assess the differential effect of ARNT2, BCL6, or GFP overexpression on gene expression in hypoxic or ischemic conditions vs. normoxia. P-values of <0.05 were considered significant (Figure 8).
A permutation test was used to measure the statistical significance of the matching between differential gene expression patterns in in vitro and in vivo conditions. Genes were discretized into three states, i.e. up-regulated (UP), down-regulated (DN), or unchanged (NS). For each gene in the two conditions, a score of 1 was assigned for a perfect match of UP/UP or DN/DN, 0 for a neutral match of NS/NS, −1 for a perfect mismatch of UP/DN or DN/UP, and −0.5 for all other patterns. The matching score for any pair of conditions was computed as the sum of all scores for each individual gene. The significance of the scores was assessed via a permutation on the class labels. Permutations were exhaustive when feasible, otherwise limited to a random sample of 5,000 (Data S11 in Supplementary Material).
Evaluation of Placental/Trophoblastic Expression and Function of ZNF554
Tissue qRT-PCR Array Expression Profiling
TaqMan assays for ZNF554 and RPLP0 (Data S17 in Supplementary Material) were run in triplicate for expression profiling of the Human Major Tissue qPCR Array (OriGene Technologies) that contains cDNAs from 48 different pooled tissues.
mRNA In Situ Hybridization
In situ hybridization on third trimester FFPE placental tissues (n = 6) retrieved from the Bank of Biological Specimens of the Perinatology Research Branch was carried out using the RNAscope 2.0 FFPE Assay–Brown (Advanced Cell Diagnostics, Hayward, CA, USA) on a HybEZ Hybridization System (Figure 9). Briefly, tissue sections were incubated with ZNF554 target probe (Cat.No.: 423831, Advanced Cell Diagnostics) for 2 h at 40°C. After rinsing with 1× Wash Buffer, slides underwent a six-step amplification procedure at 40°C and were washed with 1× Wash Buffer between amplification steps. Chromogenic detection was performed using a 1:1 mixture of Brown-A and Brown-B solutions. Slides were counterstained with hematoxylin, dehydrated in graded ethanol, and mounted in xylene.
Immunohistochemistry
Third trimester placentas were retrieved from the Bank of Biological Specimens of the Perinatology Research Branch. First trimester placentas were collected from healthy Caucasian women undergoing termination of pregnancy and processed at the Maternity Clinic and Semmelweis University in Budapest, Hungary as described above. Five µm sections of first and third trimester FFPE placental tissues (n = 15) were placed on silanized slides and stained using anti-ZNF554 or anti-cytokeratin-7 antibodies as well as reagents listed in Data S18 (Supplementary Material) either on Ventana Discovery (Ventana Medical Systems, Inc, Tucson, AZ, USA) or Leica BOND-MAX (Leica Microsystems, Wetzlar, Germany) autostainers (Figures 9 and 10).
Primary VT Differentiation
The experimental procedures were embedded in the study on VT differentiation as described above (Figure 9).
BeWo Cell Cultures
BeWo cells were incubated in a T-25 flask or 6-well plate with Ham’s F12-K medium (Life Technologies) supplemented with 10% FBS and 1% P/S in a humidified incubator (5% CO2, 20% O2) at 37oC until reaching 50–80% confluence.
To test the effect of ZNF554 knockdown on gene expression, cells were treated either with 100 nM ZNF554 siRNA (Ambion-Life Technologies, Foster City, CA, USA) or 100 nM scrambled (control) siRNA (Ambion) using X-tremeGENE siRNA transfection reagent (Roche, Mississauga, ON, Canada), and incubated at 37oC in 2 ml serum-free Opti-MEM (Gibco-Life Technologies) medium. After 6 h, the medium was replaced with 2 ml Ham’s F12-K medium supplemented with 10% FBS. After 48 h, cells were collected for RNA isolation, microarray, and qRT-PCR as well as confocal microscopy (Figure 9).
To test the effect of DNA methylation on gene expression, BeWo cells were treated with 5 or 10 µM 5-azacitidine (Sigma-Aldrich), and control cells with DMSO. This experiment was also performed when both 5-azacitidine-treated and control cells received 25 µM forskolin (Sigma-Aldrich) to induce syncytialization. After 24 h incubation, cells were harvested for RNA isolation and qRT-PCR. The experiment was performed in six replicates (Figure 11; Figure S8 in Supplementary Material).
HTR8/SVneo Cell Cultures
HTR8/SVneo EVT cells (kindly provided by Dr. Charles H. Graham, Queen’s University, Kingston, Ontario, Canada) were incubated in a 6-well plate with RPMI-1640 medium (Gibco-Life Technologies) supplemented with 10% FBS and 1% P/S in a humidified incubator (5% CO2, 20% O2) at 37oC until reaching 50% confluence.
To test the effect of ZNF554 knockdown on gene expression and functions, cells were treated either with 100 nM ZNF554 siRNA or 100 nM scrambled (control) siRNA as described for BeWo cells. After 6 h, the medium was replaced with 2 ml RPMI-1640 medium (Gibco-Life Technologies) supplemented with 10% FBS. On the following day, cells were kept in various O2 concentrations (2, 8, or 20%) in an Oxycycler C42. Cells were collected for functional assays after 24 h, while cells were collected for RNA isolation, microarray, qRT-PCR, or confocal microscopy and their supernatants for ELISA after 48 h. Cell cultures were used for cell proliferation assays after 0, 24, and 48 h.
Total RNA Isolation, Microarray, and qRT-PCR
Total RNA was isolated from BeWo and HTR8/SVneo cell cultures with TRIzol reagent (Life Technologies) and RNeasy kit (QIAGEN, Valencia, CA, USA) according to the manufacturers’ recommendations. The 28S/18S ratios and the RNA integrity numbers were assessed using an Agilent Bioanalyzer 2100; RNA concentrations were measured with NanoDrop 1000. DNase-treated RNA from BeWo and HTR8/SVneo cells (500 ng) was amplified and biotin-labeled with the Illumina TotalPrep RNA Amplification Kit (Ambion-Life Technologies). Labeled cRNAs were hybridized to a HumanHT-12v4 Expression BeadChip (Illumina, Inc., San Diego, CA). BeadChips were imaged using a BeadArray Reader (Illumina, Inc.), and raw data were obtained with BeadStudio Software V.3.4.0 (Illumina, Inc.). Total RNA (500 ng) was also reverse transcribed with High Capacity cDNA Reverse Transcription Kit using random hexamers (Applied Biosystems, Foster City, CA, USA). TaqMan Assays (Applied Biosystems; Data S17 in Supplementary Material) were used for high-throughput gene expression profiling on the Biomark qRT-PCR system (Fluidigm, San Francisco, CA, USA) according to the manufacturers’ instructions.
Confocal Microscopy
ZNF554 knock-down and control BeWo and HTR8/SVneo cells cultured in 6-well plate were detached with 0.05% Trypsin-EDTA (Life Technologies), washed, and resuspended in PBS, and then 4 × 104 cells were cytospined to Superfrost Plus slides (Fisher Scientific). Then, cells were fixed with 4% paraformaldehyde (Electron Microscopy Sciences, Hatfield, PA, USA), blocked with Protein Block (Dako North America, Inc., Carpinteria, CA, USA), and immunostained with anti-ZNF554 mouse polyclonal antibody (1:100 dilution, overnight; Abnova, Taipei City, Taiwan) and an AlexaFluor-488 goat anti-mouse antibody (1:1,000 dilution; Life Technologies). Cells were mounted with ProLong Gold antifade reagent with 4’,6-diamidino-2-phenylindole (DAPI; Life Technologies), followed by confocal microscopy using a Leica TCS SP5 MP spectral confocal system (Leica Microsystems) (Figures 9 and 10).
Enzyme-Linked Immunosorbent Assays
Concentrations of human plasminogen activator inhibitor-1 (PAI-1) and TIMP-3 in HTR8/SVneo cell culture supernatants were measured with sensitive and specific immunoassays (Human PAI-1 ELISA Kit, Invitrogen; TIMP-3 Human ELISA Kit, Abcam Inc., Cambridge, MA, USA) according to the manufacturers’ instructions. Standard curves were generated, and sample assay values were extrapolated. The sensitivities of the assays were <30 pg/mL (PAI-1) and <2 pg/ml (TIMP-3) (Figure 10).
Cell Proliferation Assays
Cell cultures of control and ZNF554 siRNA treated HTR8/SVneo cells were assayed using the CellTiter 96 Aqueous Non-radioactive Cell Proliferation Assay (Promega) according to the manufacturer’s instructions (Figure S11 in Supplementary Material).
Migration Assay
The migratory capacity of HTR8/SVneo cells was examined with 8μm-pore transwell inserts (Corning, NY, USA) inserted in a 12-well plate described previously (345). After transfection with ZNF554 or scrambled siRNAs for 24 h, 5 × 105 HTR8/SVneo cells were plated in the upper chambers in a serum-free RPMI-1640 medium, whereas the lower chambers contained an RPMI-1640 medium supplemented with 10% FBS. After incubation for 36 h in 2, 8, or 20% O2 concentrations, cells on the upper side of the membranes were removed by cotton swab, and the inserts were fixed in methanol for 10 min at RT and washed once with PBS. Then, the membranes were cut out and mounted on Superfrost Plus slides (Fisher Scientific) with ProLong Gold antifade reagent with DAPI. Comprehensive images of each membrane were taken using a Leica TCS SP5 MP spectral confocal system. The number of invaded cells was quantified using Image-Pro Premier v9.0.2 (Media Cybernetics, Inc., Rockville, MD, USA). The experiment was performed in six replicates (Figure 10).
Matrigel Invasion Assay
The invasiveness of HTR8/SVneo cells was examined with a Matrigel invasion assay using 8 μm-pore cell culture inserts (BD Biosciences) pre-coated with Matrigel (125 ng/ml; BD Biosciences) and inserted in a 24-well plate described previously (345). After transfection with ZNF554 or scrambled siRNAs for 24 h, 2 × 105 cells were plated in the upper chambers in a serum-free RPMI-1640 medium, whereas an RPMI-1640 medium supplemented with 10% FBS was added to the lower chambers. After incubation for 48 h in 2, 8, or 20% O2 concentrations, cells on the Matrigel side of the membranes were removed by cotton swab, and the membranes were processed as in the migration assay. Comprehensive images taken using the Leica TC5 SP5 spectral confocal system were quantified using Image-Pro Plus 6.2 (Media Cybernetics, Inc.). The experiment was performed in triplicate (Figure 10).
Data Analysis
Tissue qRT-PCR Array. The expression of ZNF554 relative to RPLP0 in the placenta was compared to 47 other human tissues using the Student’s t-test. P-values of <0.05 were considered significant (Figure 9).
The Student’s t-test was used to evaluate ZNF554 knock-down efficiency and the effect of ZNF554 knock-down on gene expression in BeWo and HTR8/SVneo cells (Figures 9 and 10).
BeWo and HTR8/SVneo Cell Microarray. Data were analyzed using the Bioconductor packages in R (346) following the MIAME guidelines and methodologies described previously (318). Raw microarray gene expression data were normalized by a quantile normalization approach. A moderated t-test was used to select DE genes using a cutoff of >1.5 fold-change and q < 0.1. GO analysis and pathway analysis using the Kyoto Encyclopedia of Genes and Genomes (KEGG) pathway database were also performed.
HTR8/SVneo Cell qRT-PCR, Immunoassay, Cell Proliferation, Migration, and Invasion. qRT-PCR data were analyzed using the ΔΔCt method relative to RPLP0 expression. The Student’s t-test was used to evaluate ZNF554 knock-down efficiency in HTR8/SVneo cells and the effect of ZNF554 knock-down on gene expression and cell proliferation. A linear model was built to quantify the effects of ZNF554 knock-down and various O2 concentrations on the gene expression and protein secretion of HTR8/SVneo cells as well as on their migratory and invasive capacity. O2 concentration was treated as a continuous variable, and the interaction between ZNF554 knock-down and O2 concentrations was retained in the model when the coefficient was significant. P-values of <0.05 were considered significant (Figure 10).
Data Availability
All relevant data are within the paper and its Supplementary Material files. MIAME compliant microarray data are available from the Gene Expression Omnibus (GEO) under accession numbers GSE65866, GSE65940, and GSE66273.
Ethics Statement
The collection and use of human biological specimens and clinical data for research purposes were approved by the Health Science Board of Hungary, the Institutional Review Board of the Eunice Kennedy Shriver National Institute of Child Health and Human Development (NICHD, NIH, DHHS), the Wayne State University Human Investigation Committee, the Maccabi Institutional Review Board, and the Regional Ethics Committee of the University of Debrecen. Written informed consent was obtained from women prior to sample collection, and the experiments conformed to the principles set out in the World Medical Association Declaration of Helsinki and the Department of Health and Human Services Belmont Report. Specimens and data were stored anonymously.
Author Contributions
NGT conceptualized study and designed research. NGT, KAK, YX, KJ, RJL, EH-G, ZsD, AS, KE, SzSz, VT, HE-A, CL, AB, GSz, SL, and ZD performed research. NGT, RR, ALT, ZX, LO, OT, HM, SD, SSH, THC, CJK, and ZP contributed new reagents/analytic tools/clinical specimens. NGT, RR, ALT, KAK, YX, ZX, KJ, GB, ZsG, JP, THC, BAGy, AD, ASz, ZsD, GSz, IK, AF, MKr, MKn, OE, GJB, CJK, GJ, and ZP analyzed and interpreted data. All authors contributed to manuscript writing and approved the paper.
Conflict of Interest Statement
Part of the data was submitted by NGT, RR, ALT, KAK, RJL, THC, HM, MK, GJ, and ZP as patent applications in 2012 and 2014 to describe biomarkers for preeclampsia. Other authors declare no conflict of interest.
Acknowledgments
We thank Brad Baker, Ryan Cantarella, Po Jen Chiang, Stella DeWar, Sandy Field, Hong Meng, Olesya Plazyo, Russ Price, Theodore Price, Gerardo Rodriguez, Dayna Sheldon, Sivasakthy Sivalogan, Rona Wang (Perinatology Research Branch), Matthew Hess, Daniel Lott, Tara Reinholz (Wayne State University), Katalin Karaszi, Barbara Kocsis-Deak, Edit Zabolai (Semmelweis University), and Istvan Kurucz (Biosystems International) for their assistance, Gergely Szakacs, Professor Peter Zavodszky (Hungarian Academy of Sciences), Petronella Hupuczi (Maternity Private Department), Professor Sinuhe Hahn, Simona Rossi (University of Basel), Professor Douglas Ruden (Wayne State University) and Geza Ambrus-Aikelin (Jecure Therapeutics) for helpful discussions, Maureen McGerty and Sara Tipton (Wayne State University) for critical reading of the manuscript.
Funding
This research was supported by: the Perinatology Research Branch, Division of Obstetrics and Maternal-Fetal Medicine, Division of Intramural Research, Eunice Kennedy Shriver National Institute of Child Health and Human Development, National Institutes of Health, US Department of Health and Human Services (NICHD/NIH/DHHS); Federal funds from NICHD/NIH/DHHS under Contract No. HHSN275201300006C; European Union FP6 grant Pregenesys-037244; Hungarian Academy of Sciences Momentum grant LP2014-7/2014; Hungarian National Research, Development and Innovation Fund grant FIEK_16-1-2016-0005; Hungarian National Science Fund grant OTKA K124862; and Zymo Research Corporation. The funders had no role in study design, data collection and analysis, decision to publish, or preparation of the manuscript.
Supplementary Material
The Supplementary Material for this article can be found online at https://www.frontiersin.org/articles/10.3389/fimmu.2018.01661/full#supplementary-material.
Figure S1. Hub transcription regulatory genes in the M1 and M2 modules and their interaction network. (A) Co-expression matrix of transcription regulatory genes and predominantly placenta-expressed genes in the M1 and M2 modules. Within the M1 (green) module, ESRRG, POU5F1, and ZNF554 transcription regulatory genes correlated most strongly with predominantly placenta-expressed genes. ESRRG and ZNF554 had the most correlation with CSH1 and HSD11B2, genes strongly implicated in fetal growth. Within the M2 (red) module, BCL6, BHLHE40, and ARNT2 transcription regulatory genes were correlated most strongly with predominantly placenta-expressed genes. BCL6 and ARNT2 had the most correlation with FLT1. Heatmap represents Pearson coefficients. (B,C) The networks of biological processes enriched among genes dysregulated in preeclampsia and co-expressed with hub factors in M1 (ZNF554) and M2 (BCL6) modules were visualized with the BINGO module of Cytoscape. Sizes of the circles relate to the number of genes involved in the biological processes and colors refer to p-values. The groups of most enriched biological processes were manually circled and labeled. The color code depicts p-values.
Figure S2. Clinical characteristics in the transcriptomic validation study groups. Blood pressure, birth weight, and gestational age data from the 100 pregnant women included in the validation study show that preeclampsia phenotypes are heterogeneous, underlining the complex pathways of disease in preeclampsia.
Figure S3. Placental gene expression changes in various phenotypes of preeclampsia detected by qRT-PCR. Data represent gene expressions relative to RPLP0 measured across 100 placentas. In each bar plot (mean ± SE), the left and right panels show significant differences (“*”) in preterm and term preeclampsia associated with or without SGA samples compared to gestational age-matched controls, respectively. Changes in preterm preeclampsia samples significantly different to changes in term preeclampsia samples are indicated by “+”.
Figure S4. The correlation between placental gene expression and maternal plasma protein concentration. Placental LEP and CSH1 gene expression was either measured with microarrays in the third trimester or with qRT-PCR in the first trimester. Maternal plasma leptin and serum human placental lactogen protein concentrations were measured with ELISA. Third trimester placental microarray data were correlated with ELISA data from maternal blood samples collected at the time of delivery from the same patients. qRT-PCR data from placentas taken from first trimester terminations were correlated with ELISA data from blood samples collected at the time of the procedure from the same patients. Correlations were investigated with the Pearson method and visualized on scatter plots. The two investigated genes’ expression and their protein products’ concentrations correlated both in the first and third trimesters.
Figure S5. The timing of gene module dysregulation in preterm preeclampsia. (A) Human microarray data on 79 human tissues and cells downloaded from the BioGPS database was used for the generation of placenta enrichment scores (placental expression/mean expression in 78 other tissues and cells). Five genes with scores between 1.4 and 1,490 were selected based on literature search due to the extensive investigations of their gene products in maternal blood in preeclampsia. Colors depict gene module involvement. (B–F) The 80,170 measurements for five gene products published in 61 scientific reports (35, 61, 82, 88, 126, 178–233) were used for the virtual liquid biopsy of the placenta in preterm preeclampsia. Biomarker levels in preterm preeclampsia were expressed as the percentage of control levels (dotted lines) throughout pregnancy. Percentage values were represented in the scatter plots by different colors reflecting gene module classification. Based on qRT-PCR data, sEng belongs to M2 (red) module. The number of measurements, the Pearson correlation values for biomarker levels, and gestational age as well as corresponding p-values are depicted for each biomarker.
Figure S6. Maternal blood proteomic changes in term preeclampsia and their effect on differentially expressed (DE) genes in the placenta. (A) The 14 DE maternal serum proteins in term preeclampsia belong to six functional groups. (B) These 14 proteins have connections with 116 DE placental genes, among which 46 belong to the M2 (red) module. Angiotensinogen has more connections than other proteins (OR = 2.5, p = 1.6 × 10−8) and the most with M2 (red) module genes (n = 35). Seventy seven of 86 connections of angiotensinogen have a directional effect toward the gene.
Figure S7. Summary of functional experiments on module M2. Epigenetic changes to the trophoblast and abnormal trophoblast differentiation lead to a general down-regulation of gene expression and the up-regulation of hub factors in module M2 (e.g. BCL6). After placental circulation has been established and placental ischemic stress occurs, the up-regulation of BCL6 sensitizes the trophoblast to ischemia by inducing ARNT2 up-regulation and downstream increase of expression of FLT1, ENG, LEP, leading to the placental release of pro-inflammatory and anti-angiogenic gene products. This pathway is only observed in preterm preeclampsia, suggesting that the dysregulation of this placental pathway promotes the early development of preeclampsia. The alterations in maternal blood proteome can induce trophoblastic functional changes leading to the up-regulation of module M2 genes, the overproduction of sFlt-1 and an anti-angiogenic state through a trajectory that does not necessarily affect fetal growth.
Figure S8. DNA methylation regulates BCL6 expression in the trophoblast. (A) Decreased BCL6 expression was observed in BeWo cells upon treatment with 5-azacitidine (5-AZA) irrespective of Forskolin (FRSK) co-treatment. (B) Upper three lanes: whole genome bisulfite sequencing data of BCL6 first intron from the Human Reference Epigenome Mapping Project. H1 ESC; H1 embryonic stem cell; HBDT, H1 BMP4-derived trophoblast; and HDNP, H1-derived neuronal progenitor. Lower three lanes: bisulfite sequencing data in this study. Abbreviations: CB, cord blood cell; CT, cytotrophoblast; ST, syncytiotrophoblast. Red box: differentially methylated region; red arrow: CpG Chr3:187458163.
Figure S9. DNA methylation levels at individual CpGs in BCL6 in the trophoblast and umbilical cord blood cells. DNA methylation levels (0–100%) at individual CpGs in BCL6 in umbilical cord blood cells (CB), cytotrophoblasts (CT), and differentiated syncytiotrophoblasts (ST) are depicted in the bar plots that represent means and SEs. Umbilical cord blood cells and cytotrophoblasts were obtained from the same fetuses. The genomic coordinates of the CpGs, the group differences (CB vs. CT; CT vs. ST) in mean DNA methylation levels and the p-values are shown above the bar plots. The number of samples analyzed with methylation reads above the threshold are shown below the bar plots (only comparisons with a group sample size of minimum two were considered). Differential methylation was claimed to be mild, moderate, or strong when the p-value was <0.05 and the difference in methylation level was ≥0.125, ≥0.25, or ≥0.5, respectively.
Figure S10. DNA methylation levels at individual CpGs in BCL6 in the trophoblast in controls and in cases of preeclampsia. DNA methylation levels (0–100%) at individual CpGs in BCL6 in laser captured trophoblasts are depicted in the bar plots that represent means and SEs. The genomic coordinates of the CpGs, the group differences (compared preterm or term controls) in DNA methylation levels and the p-values are shown above the bar plots. The number of samples analyzed with methylation reads above the threshold are shown below the bar plots (only comparisons with a group sample size of minimum four were considered). Differential methylation was claimed to be mild, moderate, or strong when the p-value was <0.05 and the difference in methylation level was ≥0.125, ≥0.25, or ≥0.5, respectively. Preterm (left) and term (right) groups of patients were analyzed separately. Abbreviations: PE, preeclampsia; PE + SGA, preeclampsia associated with small-for-gestational age.
Figure S11. The effect of ZNF554 knock-down on cell proliferation in HTR8/SVneo extravillous trophoblastic cells. (A) Cell proliferation assays showed that ZNF554 knock-down slightly but significantly decreased (−14%, p = 0.02) cell proliferation rate in HTR8/SVneo extravillous trophoblastic cells after 48 h. Y-axis depicts viable cell number, X-axis shows incubation time. (B) The differential expression of CDKN1A (cyclin-dependent kinase inhibitor 1A) and STK40 (serine/threonine kinase 40), genes involved in the regulation of cell cycle, upon ZNF554 knock-down was confirmed by qRT-PCR.
Figure S12. DNA methylation levels at individual CpGs in ZNF554 in the trophoblast and umbilical cord blood cells. DNA methylation levels (0–100%) at individual CpGs in ZNF554 in umbilical cord blood cells (CB), cytotrophoblasts (CT), and differentiated syncytiotrophoblasts (ST) are depicted in the bar plots that represent means and SEs. Umbilical cord blood cells and CT were obtained from the same fetuses. The genomic coordinates of the CpGs, the group differences (CB vs. CT; CT vs. ST) in mean methylation levels and the p-values are shown above the bar plots. The number of samples analyzed with methylation reads above the threshold are shown below the bar plots (only comparisons with a group sample size of minimum two were considered). Differential methylation was claimed to be mild, moderate, or strong when the p-value was <0.05 and the difference in methylation level was ≥0.125, ≥0.25, or ≥0.5, respectively.
Figure S13. DNA methylation levels at individual CpGs in ZNF554 in the trophoblast in controls and in cases of preeclampsia. DNA methylation levels (0–100%) at individual CpGs in ZNF554 in laser captured trophoblasts are depicted in the bar plots that represent means and SEs. The genomic coordinates of the CpGs, the group differences (compared preterm or term controls) in methylation levels, and the p-values are shown above the bar plots. The number of samples analyzed with methylation reads above the threshold are shown below the bar plots (only comparisons with a group sample size of minimum four were considered). Differential methylation was claimed to be mild, moderate, or strong when the p-value was <0.05 and the difference in methylation level was ≥0.125, ≥0.25, or ≥0.5, respectively. Preterm (left) and term (right) groups of patients were analyzed separately. Abbreviations: PE, preeclampsia; PE + SGA, preeclampsia associated with SGA.
Data S1. Genes differentially expressed in the placenta in preterm preeclampsia.
Data S2. Predominantly placenta-expressed genes.
Data S3. The enrichment of differentially expressed genes on chromosomes.
Data S4. The enrichment of differentially expressed transcription regulatory genes on chromosomes.
Data S5. The enrichment of predominantly placenta-expressed genes on chromosomes.
Data S6. Genes associated with blood pressure.
Data S7. The association of gene expression with placental pathology.
Data S8. Maternal blood proteomic changes in preeclampsia—two-dimensional differential in-gel electrophoresis.
Data S9. Maternal blood proteomic changes in preeclampsia—multiple reaction monitoring.
Data S10. Placental pathways enriched among the differentially expressed genes connected to angiotensinogen.
Data S11. Permutation test of functional experiments.
Data S12. Enrichment of transposable elements in genes within the M1 and M2 gene modules.
Data S13. Genes differentially expressed in ZNF554-silenced BeWo cells.
Data S14. Enrichment analysis of ZNF554-silenced BeWo cells.
Data S15. Genes differentially expressed in ZNF554-silenced HTR8/SVneo cells.
Data S16. Enrichment analysis of ZNF554-silenced HTR8/SVneo cells.
Data S17. TaqMan assays.
Data S18. Immunostaining conditions and antibodies.
Footnotes
References
1. ACOG Committee on Practice Bulletins–Obstetrics. ACOG practice bulletin. Diagnosis and management of preeclampsia and eclampsia. Number 33, January 2002. Obstet Gynecol (2002) 99(1):159–67.
2. Sibai B, Dekker G, Kupferminc M. Pre-eclampsia. Lancet (2005) 365(9461):785–99. doi:10.1016/S0140-6736(05)71003-5
3. Wallis AB, Saftlas AF, Hsia J, Atrash HK. Secular trends in the rates of preeclampsia, eclampsia, and gestational hypertension, United States, 1987-2004. Am J Hypertens (2008) 21(5):521–6. doi:10.1038/ajh.2008.20
4. Kuklina EV, Ayala C, Callaghan WM. Hypertensive disorders and severe obstetric morbidity in the United States. Obstet Gynecol (2009) 113(6):1299–306. doi:10.1097/AOG.0b013e3181a45b25
5. Hutcheon JA, Lisonkova S, Joseph KS. Epidemiology of pre-eclampsia and the other hypertensive disorders of pregnancy. Best Pract Res Clin Obstet Gynaecol (2011) 25(4):391–403. doi:10.1016/j.bpobgyn.2011.01.006
6. Paruk F, Moodley J. Maternal and neonatal outcome in early- and late-onset pre-eclampsia. Semin Neonatol (2000) 5(3):197–207. doi:10.1053/siny.2000.0023
7. MacKay AP, Berg CJ, Atrash HK. Pregnancy-related mortality from preeclampsia and eclampsia. Obstet Gynecol (2001) 97(4):533–8. doi:10.1097/00006250-200104000-00011
8. Smith GC, Pell JP, Walsh D. Pregnancy complications and maternal risk of ischaemic heart disease: a retrospective cohort study of 129,290 births. Lancet (2001) 357(9273):2002–6. doi:10.1016/S0140-6736(00)05112-6
9. Bellamy L, Casas JP, Hingorani AD, Williams DJ. Pre-eclampsia and risk of cardiovascular disease and cancer in later life: systematic review and meta-analysis. BMJ (2007) 335(7627):974. doi:10.1136/bmj.39335.385301.BE
10. Berg CJ, Mackay AP, Qin C, Callaghan WM. Overview of maternal morbidity during hospitalization for labor and delivery in the United States: 1993-1997 and 2001-2005. Obstet Gynecol (2009) 113(5):1075–81. doi:10.1097/AOG.0b013e3181a09fc0
11. Stekkinger E, Zandstra M, Peeters LL, Spaanderman ME. Early-onset preeclampsia and the prevalence of postpartum metabolic syndrome. Obstet Gynecol (2009) 114(5):1076–84. doi:10.1097/AOG.0b013e3181b7b242
12. Schutte JM, Steegers EA, Schuitemaker NW, Santema JG, de Boer K, Pel M, et al. Rise in maternal mortality in the Netherlands. BJOG (2010) 117(4):399–406. doi:10.1111/j.1471-0528.2009.02382.x
13. Adams T, Yeh C, Bennett-Kunzier N, Kinzler WL. Long-term maternal morbidity and mortality associated with ischemic placental disease. Semin Perinatol (2014) 38(3):146–50. doi:10.1053/j.semperi.2014.03.003
14. Lisonkova S, Sabr Y, Mayer C, Young C, Skoll A, Joseph KS. Maternal morbidity associated with early-onset and late-onset preeclampsia. Obstet Gynecol (2014) 124(4):771–81. doi:10.1097/AOG.0000000000000472
15. Ozimek JA, Eddins RM, Greene N, Karagyozyan D, Pak S, Wong M, et al. Opportunities for improvement in care among women with severe maternal morbidity. Am J Obstet Gynecol (2016) 215(4):e1–6. doi:10.1016/j.ajog.2016.05.022
16. Hnat MD, Sibai BM, Caritis S, Hauth J, Lindheimer MD, MacPherson C, et al. Perinatal outcome in women with recurrent preeclampsia compared with women who develop preeclampsia as nulliparas. Am J Obstet Gynecol (2002) 186(3):422–6. doi:10.1067/mob.2002.120280
17. Madazli R, Yuksel MA, Imamoglu M, Tuten A, Oncul M, Aydin B, et al. Comparison of clinical and perinatal outcomes in early- and late-onset preeclampsia. Arch Gynecol Obstet (2014) 290(1):53–7. doi:10.1007/s00404-014-3176-x
18. Sarno L, Maruotti GM, Saccone G, Sirico A, Mazzarelli LL, Martinelli P. Pregnancy outcome in proteinuria-onset and hypertension-onset preeclampsia. Hypertens Pregnancy (2015) 34(3):284–90. doi:10.3109/10641955.2015.1015731
19. Barker DJ. Fetal nutrition and cardiovascular disease in later life. Br Med Bull (1997) 53(1):96–108. doi:10.1093/oxfordjournals.bmb.a011609
20. Irgens HU, Reisaeter L, Irgens LM, Lie RT. Long term mortality of mothers and fathers after pre-eclampsia: population based cohort study. BMJ (2001) 323(7323):1213–7. doi:10.1136/bmj.323.7323.1213
21. Powe CE, Levine RJ, Karumanchi SA. Preeclampsia, a disease of the maternal endothelium: the role of antiangiogenic factors and implications for later cardiovascular disease. Circulation (2011) 123(24):2856–69. doi:10.1161/CIRCULATIONAHA.109.853127
22. Clifton VL, Stark MJ, Osei-Kumah A, Hodyl NA. The feto-placental unit, pregnancy pathology and impact on long term maternal health. Placenta (2012) 33(Suppl):S37–41. doi:10.1016/j.placenta.2011.11.005
23. Chen CW, Jaffe IZ, Karumanchi SA. Pre-eclampsia and cardiovascular disease. Cardiovasc Res (2014) 101(4):579–86. doi:10.1093/cvr/cvu018
24. Chaiworapongsa T, Chaemsaithong P, Korzeniewski SJ, Yeo L, Romero R. Pre-eclampsia part 2: prediction, prevention and management. Nat Rev Nephrol (2014) 10(9):531–40. doi:10.1038/nrneph.2014.103
25. Ness RB, Roberts JM. Heterogeneous causes constituting the single syndrome of preeclampsia: a hypothesis and its implications. Am J Obstet Gynecol (1996) 175(5):1365–70. doi:10.1016/S0002-9378(96)70056-X
26. von Dadelszen P, Magee LA, Roberts JM. Subclassification of preeclampsia. Hypertens Pregnancy (2003) 22(2):143–8. doi:10.1081/PRG-120021060
27. Di Renzo GC. The great obstetrical syndromes. J Matern Fetal Neonatal Med (2009) 22(8):633–5. doi:10.1080/14767050902866804
28. Brosens I, Pijnenborg R, Vercruysse L, Romero R. The “great obstetrical syndromes” are associated with disorders of deep placentation. Am J Obstet Gynecol (2011) 204(3):193–201. doi:10.1016/j.ajog.2010.08.009
29. Than NG, Vaisbuch E, Kim CJ, Mazaki-Tovi S, Erez O, Yeo L, et al. Early-Onset Preeclampsia and HELLP Syndrome: an Overview. In: Preedy VR, editor. Handbook of Growth and Growth Monitoring in Health and Disease. New York: Springer (2012). p. 1867–1891.
30. Chaiworapongsa T, Chaemsaithong P, Yeo L, Romero R. Pre-eclampsia part 1: current understanding of its pathophysiology. Nat Rev Nephrol (2014) 10(8):466–80. doi:10.1038/nrneph.2014.102
31. Myatt L, Roberts JM. Preeclampsia: syndrome or disease? Curr Hypertens Rep (2015) 17(11):83. doi:10.1007/s11906-015-0595-4
32. Vatten LJ, Skjaerven R. Is pre-eclampsia more than one disease? BJOG (2004) 111(4):298–302. doi:10.1111/j.1471-0528.2004.00071.x
33. Sebire NJ, Goldin RD, Regan L. Term preeclampsia is associated with minimal histopathological placental features regardless of clinical severity. J Obstet Gynaecol (2005) 25(2):117–8. doi:10.1080/014436105400041396
34. Valensise H, Vasapollo B, Gagliardi G, Novelli GP. Early and late preeclampsia: two different maternal hemodynamic states in the latent phase of the disease. Hypertension (2008) 52(5):873–80. doi:10.1161/HYPERTENSIONAHA.108.117358
35. Akolekar R, Syngelaki A, Sarquis R, Zvanca M, Nicolaides KH. Prediction of early, intermediate and late pre-eclampsia from maternal factors, biophysical and biochemical markers at 11-13 weeks. Prenat Diagn (2011) 31(1):66–74. doi:10.1002/pd.2660
36. Tranquilli AL, Brown MA, Zeeman GG, Dekker G, Sibai BM. The definition of severe and early-onset preeclampsia. Statements from the International Society for the Study of Hypertension in Pregnancy (ISSHP). Pregnancy Hypertens (2013) 3(1):44–7. doi:10.1016/j.preghy.2012.11.001
37. Szalai G, Romero R, Chaiworapongsa T, Xu Y, Wang B, Ahn H, et al. Full-length human placental sFlt-1-e15a isoform induces distinct maternal phenotypes of preeclampsia in mice. PLoS One (2015) 10:e0119547. doi:10.1371/journal.pone.0119547
38. Brosens IA, Robertson WB, Dixon HG. The role of the spiral arteries in the pathogenesis of preeclampsia. Obstet Gynecol Annu (1972) 1:177–91.
39. Burton GJ, Jauniaux E. Placental oxidative stress: from miscarriage to preeclampsia. J Soc Gynecol Investig (2004) 11(6):342–52. doi:10.1016/j.jsgi.2004.03.003
40. Burton GJ, Woods AW, Jauniaux E, Kingdom JC. Rheological and physiological consequences of conversion of the maternal spiral arteries for uteroplacental blood flow during human pregnancy. Placenta (2009) 30(6):473–82. doi:10.1016/j.placenta.2009.02.009
41. Burton GJ, Yung HW, Cindrova-Davies T, Charnock-Jones DS. Placental endoplasmic reticulum stress and oxidative stress in the pathophysiology of unexplained intrauterine growth restriction and early onset preeclampsia. Placenta (2009) 30(Suppl A):S43–8. doi:10.1016/j.placenta.2008.11.003
42. Roberts JM, Hubel CA. The two stage model of preeclampsia: variations on the theme. Placenta (2009) 30(Suppl A):S32–7. doi:10.1016/j.placenta.2008.11.009
43. Redman CW, Sargent IL. Immunology of pre-eclampsia. Am J Reprod Immunol (2010) 63(6):534–43. doi:10.1111/j.1600-0897.2010.00831.x
44. Moldenhauer JS, Stanek J, Warshak C, Khoury J, Sibai B. The frequency and severity of placental findings in women with preeclampsia are gestational age dependent. Am J Obstet Gynecol (2003) 189(4):1173–7. doi:10.1067/S0002-9378(03)00576-3
45. van der Merwe JL, Hall DR, Wright C, Schubert P, Grove D. Are early and late preeclampsia distinct subclasses of the disease – what does the placenta reveal? Hypertens Pregnancy (2010) 29(4):457–67. doi:10.3109/10641950903572282
46. Ogge G, Chaiworapongsa T, Romero R, Hussein Y, Kusanovic JP, Yeo L, et al. Placental lesions associated with maternal underperfusion are more frequent in early-onset than in late-onset preeclampsia. J Perinat Med (2011) 39(6):641–52. doi:10.1515/JPM.2011.098
47. Odegard RA, Vatten LJ, Nilsen ST, Salvesen KA, Austgulen R. Preeclampsia and fetal growth. Obstet Gynecol (2000) 96(6):950–5. doi:10.1097/00006250-200012000-00016
48. Yu CK, Khouri O, Onwudiwe N, Spiliopoulos Y, Nicolaides KH. Fetal medicine foundation second-trimester screening G. Prediction of pre-eclampsia by uterine artery Doppler imaging: relationship to gestational age at delivery and small-for-gestational age. Ultrasound Obstet Gynecol (2008) 31(3):310–3. doi:10.1002/uog.5252
49. Eskild A, Romundstad PR, Vatten LJ. Placental weight and birthweight: does the association differ between pregnancies with and without preeclampsia? Am J Obstet Gynecol (2009) 201(6):e1–5. doi:10.1016/j.ajog.2009.06.003
50. Lisonkova S, Joseph KS. Incidence of preeclampsia: risk factors and outcomes associated with early- versus late-onset disease. Am J Obstet Gynecol (2013) 209(6):e1–12. doi:10.1016/j.ajog.2013.08.019
51. Espinoza J, Lee W, Martin SR, Belfort MA. Customized growth curves for identification of large-for-gestational age neonates in pre-eclamptic women. Ultrasound Obstet Gynecol (2014) 43(2):165–9. doi:10.1002/uog.12518
52. Rasmussen S, Irgens LM, Espinoza J. Maternal obesity and excess of fetal growth in pre-eclampsia. BJOG (2014) 121(11):1351–7. doi:10.1111/1471-0528.12677
53. Verlohren S, Melchiorre K, Khalil A, Thilaganathan B. Uterine artery Doppler, birth weight and timing of onset of pre-eclampsia: providing insights into the dual etiology of late-onset pre-eclampsia. Ultrasound Obstet Gynecol (2014) 44(3):293–8. doi:10.1002/uog.13310
54. Kenneth L, Hall DR, Gebhardt S, Grove D. Late onset preeclampsia is not an innocuous condition. Hypertens Pregnancy (2010) 29(3):262–70. doi:10.3109/10641950902777697
55. Soto E, Romero R, Kusanovic JP, Ogge G, Hussein Y, Yeo L, et al. Late-onset preeclampsia is associated with an imbalance of angiogenic and anti-angiogenic factors in patients with and without placental lesions consistent with maternal underperfusion. J Matern Fetal Neonatal Med (2012) 25(5):498–507. doi:10.3109/14767058.2011.591461
56. Maynard SE, Min JY, Merchan J, Lim KH, Li J, Mondal S, et al. Excess placental soluble fms-like tyrosine kinase 1 (sFlt1) may contribute to endothelial dysfunction, hypertension, and proteinuria in preeclampsia. J Clin Invest (2003) 111(5):649–58. doi:10.1172/JCI17189
57. Chaiworapongsa T, Romero R, Espinoza J, Bujold E, Kim YM, Goncalves LF, et al. Evidence supporting a role for blockade of the vascular endothelial growth factor system in the pathophysiology of preeclampsia. Young Investigator Award. Am J Obstet Gynecol (2004) 190(6):1541–7. doi:10.1016/j.ajog.2004.03.043
58. Matthiesen L, Berg G, Ernerudh J, Ekerfelt C, Jonsson Y, Sharma S. Immunology of preeclampsia. Chem Immunol Allergy (2005) 89:49–61. doi:10.1159/000087912
59. Redman CW, Sargent IL. Latest advances in understanding preeclampsia. Science (2005) 308(5728):1592–4. doi:10.1126/science.1111726
60. Goswami D, Tannetta DS, Magee LA, Fuchisawa A, Redman CW, Sargent IL, et al. Excess syncytiotrophoblast microparticle shedding is a feature of early-onset pre-eclampsia, but not normotensive intrauterine growth restriction. Placenta (2006) 27(1):56–61. doi:10.1016/j.placenta.2004.11.007
61. Levine RJ, Lam C, Qian C, Yu KF, Maynard SE, Sachs BP, et al. Soluble endoglin and other circulating antiangiogenic factors in preeclampsia. N Engl J Med (2006) 355(10):992–1005. doi:10.1056/NEJMoa055352
62. Venkatesha S, Toporsian M, Lam C, Hanai J, Mammoto T, Kim YM, et al. Soluble endoglin contributes to the pathogenesis of preeclampsia. Nat Med (2006) 12(6):642–9. doi:10.1038/nm1429
63. Cindrova-Davies T. Gabor Than Award lecture 2008: pre-eclampsia - from placental oxidative stress to maternal endothelial dysfunction. Placenta (2009) 30(Suppl A):S55–65. doi:10.1016/j.placenta.2008.11.020
64. Gupta AK, Hasler P, Holzgreve W, Gebhardt S, Hahn S. Induction of neutrophil extracellular DNA lattices by placental microparticles and IL-8 and their presence in preeclampsia. Hum Immunol (2005) 66(11):1146–54. doi:10.1016/j.humimm.2005.11.003
65. Lai Z, Kalkunte S, Sharma S. A critical role of interleukin-10 in modulating hypoxia-induced preeclampsia-like disease in mice. Hypertension (2011) 57(3):505–14. doi:10.1161/HYPERTENSIONAHA.110.163329
66. Kumar A, Begum N, Prasad S, Agarwal S, Sharma S. IL-10, TNF-alpha & IFN-gamma: potential early biomarkers for preeclampsia. Cell Immunol (2013) 283(1–2):70–4. doi:10.1016/j.cellimm.2013.06.012
67. Roberts JM, Taylor RN, Musci TJ, Rodgers GM, Hubel CA, McLaughlin MK. Preeclampsia: an endothelial cell disorder. Am J Obstet Gynecol (1989) 161(5):1200–4. doi:10.1016/0002-9378(89)90665-0
68. Maynard S, Epstein FH, Karumanchi SA. Preeclampsia and angiogenic imbalance. Annu Rev Med (2008) 59:61–78. doi:10.1146/annurev.med.59.110106.214058
69. Vaisbuch E, Whitty JE, Hassan SS, Romero R, Kusanovic JP, Cotton DB, et al. Circulating angiogenic and antiangiogenic factors in women with eclampsia. Am J Obstet Gynecol (2011) 204(2):e1–9. doi:10.1016/j.ajog.2010.08.049
70. Hahn S, Giaglis S, Hoesli I, Hasler P. Neutrophil NETs in reproduction: from infertility to preeclampsia and the possibility of fetal loss. Front Immunol (2012) 3:362.:362. doi:10.3389/fimmu.2012.00362
71. Stoikou M, Grimolizzi F, Giaglis S, Schafer G, van Breda SV, Hoesli IM, et al. Gestational diabetes mellitus is associated with altered neutrophil activity. Front Immunol (2017) 8:702. doi:10.3389/fimmu.2017.00702
72. Bereczki D Jr. Pregnancy and acute ischemic stroke. Orv Hetil (2016) 157(20):763–6. doi:10.1556/650.2016.30421
73. Myatt L. Role of placenta in preeclampsia. Endocrine (2002) 19(1):103–11. doi:10.1385/ENDO:19:1:103
74. Roberts JM, Escudero C. The placenta in preeclampsia. Pregnancy Hypertens (2012) 2(2):72–83. doi:10.1016/j.preghy.2012.01.001
75. Sibley CP. Treating the dysfunctional placenta. J Endocrinol (2017) 234(2):R81–97. doi:10.1530/JOE-17-0185
76. Mayhew TM. A stereological perspective on placental morphology in normal and complicated pregnancies. J Anat (2009) 215(1):77–90. doi:10.1111/j.1469-7580.2008.00994.x
77. Levine RJ, Maynard SE, Qian C, Lim KH, England LJ, Yu KF, et al. Circulating angiogenic factors and the risk of preeclampsia. N Engl J Med (2004) 350(7):672–83. doi:10.1056/NEJMoa031884
78. Chaiworapongsa T, Romero R, Kim YM, Kim GJ, Kim MR, Espinoza J, et al. Plasma soluble vascular endothelial growth factor receptor-1 concentration is elevated prior to the clinical diagnosis of pre-eclampsia. J Matern Fetal Neonatal Med (2005) 17(1):3–18. doi:10.1080/14767050400028816
79. Maynard SE, Venkatesha S, Thadhani R, Karumanchi SA. Soluble Fms-like tyrosine kinase 1 and endothelial dysfunction in the pathogenesis of preeclampsia. Pediatr Res (2005) 57(5 Pt 2):1R–7R. doi:10.1203/01.PDR.0000159567.85157.B7
80. Vatten LJ, Eskild A, Nilsen TI, Jeansson S, Jenum PA, Staff AC. Changes in circulating level of angiogenic factors from the first to second trimester as predictors of preeclampsia. Am J Obstet Gynecol (2007) 196(3):e1–6. doi:10.1016/j.ajog.2006.10.909
81. Wikstrom AK, Larsson A, Eriksson UJ, Nash P, Norden-Lindeberg S, Olovsson M. Placental growth factor and soluble FMS-like tyrosine kinase-1 in early-onset and late-onset preeclampsia. Obstet Gynecol (2007) 109(6):1368–74. doi:10.1097/01.AOG.0000264552.85436.a1
82. Crispi F, Llurba E, Dominguez C, Martin-Gallan P, Cabero L, Gratacos E. Predictive value of angiogenic factors and uterine artery Doppler for early- versus late-onset pre-eclampsia and intrauterine growth restriction. Ultrasound Obstet Gynecol (2008) 31(3):303–9. doi:10.1002/uog.5184
83. Romero R, Nien JK, Espinoza J, Todem D, Fu W, Chung H, et al. A longitudinal study of angiogenic (placental growth factor) and anti-angiogenic (soluble endoglin and soluble vascular endothelial growth factor receptor-1) factors in normal pregnancy and patients destined to develop preeclampsia and deliver a small for gestational age neonate. J Matern Fetal Neonatal Med (2008) 21(1):9–23. doi:10.1080/14767050701830480
84. Chaiworapongsa T, Romero R, Kusanovic JP, Mittal P, Kim SK, Gotsch F, et al. Plasma soluble endoglin concentration in pre-eclampsia is associated with an increased impedance to flow in the maternal and fetal circulations. Ultrasound Obstet Gynecol (2010) 35(2):155–62. doi:10.1002/uog.7491
85. Kalkunte S, Boij R, Norris W, Friedman J, Lai Z, Kurtis J, et al. Sera from preeclampsia patients elicit symptoms of human disease in mice and provide a basis for an in vitro predictive assay. Am J Pathol (2010) 177(5):2387–98. doi:10.2353/ajpath.2010.100475
86. Agarwal I, Karumanchi SA. Preeclampsia and the anti-angiogenic state. Pregnancy Hypertens (2011) 1(1):17–21. doi:10.1016/j.preghy.2010.10.007
87. Kumasawa K, Ikawa M, Kidoya H, Hasuwa H, Saito-Fujita T, Morioka Y, et al. Pravastatin induces placental growth factor (PGF) and ameliorates preeclampsia in a mouse model. Proc Natl Acad Sci U S A (2011) 108(4):1451–5. doi:10.1073/pnas.1011293108
88. Myatt L, Clifton RG, Roberts JM, Spong CY, Wapner RJ, Thorp JM Jr., et al. Can changes in angiogenic biomarkers between the first and second trimesters of pregnancy predict development of pre-eclampsia in a low-risk nulliparous patient population? BJOG (2013) 120(10):1183–91. doi:10.1111/1471-0528.12128
89. Allen RE, Rogozinska E, Cleverly K, Aquilina J, Thangaratinam S. Abnormal blood biomarkers in early pregnancy are associated with preeclampsia: a meta-analysis. Eur J Obstet Gynecol Reprod Biol (2014) 182:194–201. doi:10.1016/j.ejogrb.2014.09.027
90. Szalai G, Xu Y, Romero R, Chaiworapongsa T, Xu Z, Chiang PJ, et al. In vivo experiments reveal the good, the bad and the ugly faces of sFlt-1 in pregnancy. PLoS One (2014) 9(11):e110867. doi:10.1371/journal.pone.0110867
91. Redman CW, Sargent IL, Staff AC. IFPA senior award lecture: making sense of pre-eclampsia – Two placental causes of preeclampsia? Placenta (2014) 35(Suppl):S20–5. doi:10.1016/j.placenta.2013.12.008
92. Hahn S, Lapaire O, Than NG. Biomarker development for presymptomatic molecular diagnosis of preeclampsia: feasible, useful or even unnecessary? Expert Rev Mol Diagn (2015) 15:617–29. doi:10.1586/14737159.2015.1025757
93. Eastabrook G, Brown M, Sargent I. The origins and end-organ consequence of pre-eclampsia. Best Pract Res Clin Obstet Gynaecol (2011) 25(4):435–47. doi:10.1016/j.bpobgyn.2011.01.005
94. Vaisbuch E, Mazaki-Tovi S. Preeclampsia, portliness, and perturbation of adipose tissue function-are we beginning to connect the Dots? Am J Hypertens (2017) 30(6):559–60. doi:10.1093/ajh/hpx040
95. Scioscia M, Karumanchi SA, Goldman-Wohl D, Robillard PY. Endothelial dysfunction and metabolic syndrome in preeclampsia: an alternative viewpoint. J Reprod Immunol (2015) 108:42–7. doi:10.1016/j.jri.2015.01.009
96. Kupferminc MJ, Eldor A, Steinman N, Many A, Bar-Am A, Jaffa A, et al. Increased frequency of genetic thrombophilia in women with complications of pregnancy. N Engl J Med (1999) 340(1):9–13. doi:10.1056/NEJM199901073400102
97. van Dijk M, Oudejans C. (Epi)genetics of pregnancy-associated diseases. Front Genet (2013) 4:180. doi:10.3389/fgene.2013.00180
98. Valenzuela FJ, Perez-Sepulveda A, Torres MJ, Correa P, Repetto GM, Illanes SE. Pathogenesis of preeclampsia: the genetic component. J Pregnancy. (2012) 2012:632732. doi:10.1155/2012/632732
99. McGinnis R, Steinthorsdottir V, Williams NO, Thorleifsson G, Shooter S, Hjartardottir S, et al. Variants in the fetal genome near FLT1 are associated with risk of preeclampsia. Nat Genet (2017) 49(8):1255–60. doi:10.1038/ng.3895
100. Majander KK, Villa PM, Kivinen K, Kere J, Laivuori H. A follow-up linkage study of finnish pre-eclampsia families identifies a new fetal susceptibility locus on chromosome 18. Eur J Hum Genet (2013) 21(9):1024–6. doi:10.1038/ejhg.2013.6
101. Lokki AI, Kaartokallio T, Holmberg V, Onkamo P, Koskinen LLE, Saavalainen P, et al. Analysis of complement C3 gene reveals susceptibility to severe preeclampsia. Front Immunol (2017) 8:589. doi:10.3389/fimmu.2017.00589
102. Lokki AI, Daly E, Triebwasser M, Kurki MI, Roberson EDO, Happola P, et al. Protective low-frequency variants for preeclampsia in the Fms related tyrosine kinase 1 gene in the finnish population. Hypertension (2017) 70(2):365–71. doi:10.1161/HYPERTENSIONAHA.117.09406
103. Johnson MP, Brennecke SP, East CE, Goring HH, Kent JW Jr., Dyer TD, et al. Genome-wide association scan identifies a risk locus for preeclampsia on 2q14, near the inhibin, beta B gene. PLoS One (2012) 7(3):e33666. doi:10.1371/journal.pone.0033666
104. Johnson MP, Brennecke SP, East CE, Dyer TD, Roten LT, Proffitt JM, et al. Genetic dissection of the pre-eclampsia susceptibility locus on chromosome 2q22 reveals shared novel risk factors for cardiovascular disease. Mol Hum Reprod (2013) 19(7):423–37. doi:10.1093/molehr/gat011
105. Reimer T, Koczan D, Gerber B, Richter D, Thiesen HJ, Friese K. Microarray analysis of differentially expressed genes in placental tissue of pre-eclampsia: up-regulation of obesity-related genes. Mol Hum Reprod (2002) 8(7):674–80. doi:10.1093/molehr/8.7.674
106. Pang ZJ, Xing FQ. Expression profile of trophoblast invasion-associated genes in the pre-eclamptic placenta. Br J Biomed Sci (2003) 60(2):97–101. doi:10.1080/09674845.2003.11783682
107. Tsoi SC, Cale JM, Bird IM, Kay HH. cDNA microarray analysis of gene expression profiles in human placenta: up-regulation of the transcript encoding muscle subunit of glycogen phosphorylase in preeclampsia. J Soc Gynecol Investig (2003) 10(8):496–502. doi:10.1016/S1071-5576(03)00154-0
108. Watanabe H, Hamada H, Yamada N, Sohda S, Yamakawa-Kobayashi K, Yoshikawa H, et al. Proteome analysis reveals elevated serum levels of clusterin in patients with preeclampsia. Proteomics (2004) 4(2):537–43. doi:10.1002/pmic.200300565
109. Gack S, Marme A, Marme F, Wrobel G, Vonderstrass B, Bastert G, et al. Preeclampsia: increased expression of soluble ADAM 12. J Mol Med (Berl). (2005) 83(11):887–96. doi:10.1007/s00109-005-0714-9
110. Heikkila A, Tuomisto T, Hakkinen SK, Keski-Nisula L, Heinonen S, Yla-Herttuala S. Tumor suppressor and growth regulatory genes are overexpressed in severe early-onset preeclampsia – an array study on case-specific human preeclamptic placental tissue. Acta Obstet Gynecol Scand (2005) 84(7):679–89. doi:10.1111/j.0001-6349.2005.00814.x
111. Soleymanlou N, Jurisica I, Nevo O, Ietta F, Zhang X, Zamudio S, et al. Molecular evidence of placental hypoxia in preeclampsia. J Clin Endocrinol Metab (2005) 90(7):4299–308. doi:10.1210/jc.2005-0078
112. Vaiman D, Mondon F, Garces-Duran A, Mignot TM, Robert B, Rebourcet R, et al. Hypoxia-activated genes from early placenta are elevated in preeclampsia, but not in intra-uterine growth retardation. BMC Genomics (2005) 6:111. doi:10.1186/1471-2164-6-111
113. Hansson SR, Chen Y, Brodszki J, Chen M, Hernandez-Andrade E, Inman JM, et al. Gene expression profiling of human placentas from preeclamptic and normotensive pregnancies. Mol Hum Reprod (2006) 12(3):169–79. doi:10.1093/molehr/gal011
114. Zhou R, Zhu Q, Wang Y, Ren Y, Zhang L, Zhou Y. Genomewide oligonucleotide microarray analysis on placentae of pre-eclamptic pregnancies. Gynecol Obstet Invest (2006) 62(2):108–14. doi:10.1159/000092857
115. Herse F, Dechend R, Harsem NK, Wallukat G, Janke J, Qadri F, et al. Dysregulation of the circulating and tissue-based renin-angiotensin system in preeclampsia. Hypertension (2007) 49(3):604–11. doi:10.1161/01.HYP.0000257797.49289.71
116. Nishizawa H, Pryor-Koishi K, Kato T, Kowa H, Kurahashi H, Udagawa Y. Microarray analysis of differentially expressed fetal genes in placental tissue derived from early and late onset severe pre-eclampsia. Placenta (2007) 28(5–6):487–97. doi:10.1016/j.placenta.2006.05.010
117. Enquobahrie DA, Meller M, Rice K, Psaty BM, Siscovick DS, Williams MA. Differential placental gene expression in preeclampsia. Am J Obstet Gynecol (2008) 199(5):566–511. doi:10.1016/j.ajog.2008.04.020
118. Blankley RT, Gaskell SJ, Whetton AD, Dive C, Baker PN, Myers JE. A proof-of-principle gel-free proteomics strategy for the identification of predictive biomarkers for the onset of pre-eclampsia. BJOG (2009) 116(11):1473–80. doi:10.1111/j.1471-0528.2009.02283.x
119. Blumenstein M, Prakash R, Cooper GJ, North RA. Aberrant processing of plasma vitronectin and high-molecular-weight kininogen precedes the onset of preeclampsia. Reprod Sci (2009) 16(12):1144–52. doi:10.1177/1933719109342756
120. Blumenstein M, McMaster MT, Black MA, Wu S, Prakash R, Cooney J, et al. A proteomic approach identifies early pregnancy biomarkers for preeclampsia: novel linkages between a predisposition to preeclampsia and cardiovascular disease. Proteomics (2009) 9(11):2929–45. doi:10.1002/pmic.200800625
121. Cox B, Kotlyar M, Evangelou AI, Ignatchenko V, Ignatchenko A, Whiteley K, et al. Comparative systems biology of human and mouse as a tool to guide the modeling of human placental pathology. Mol Syst Biol (2009) 5:279. doi:10.1038/msb.2009.37
122. Sitras V, Paulssen RH, Gronaas H, Leirvik J, Hanssen TA, Vartun A, et al. Differential placental gene expression in severe preeclampsia. Placenta (2009) 30(5):424–33. doi:10.1016/j.placenta.2009.01.012
123. Winn VD, Gormley M, Paquet AC, Kjaer-Sorensen K, Kramer A, Rumer KK, et al. Severe preeclampsia-related changes in gene expression at the maternal-fetal interface include sialic acid-binding immunoglobulin-like lectin-6 and pappalysin-2. Endocrinology (2009) 150(1):452–62. doi:10.1210/en.2008-0990
124. Centlow M, Hansson SR, Welinder C. Differential proteome analysis of the preeclamptic placenta using optimized protein extraction. J Biomed Biotechnol (2010) 2010:458748. doi:10.1155/2010/458748
125. Hoegh AM, Borup R, Nielsen FC, Sorensen S, Hviid TV. Gene expression profiling of placentas affected by pre-eclampsia. J Biomed Biotechnol (2010) 2010:787545. doi:10.1155/2010/787545
126. Rasanen J, Girsen A, Lu X, Lapidus JA, Standley M, Reddy A, et al. Comprehensive maternal serum proteomic profiles of preclinical and clinical preeclampsia. J Proteome Res (2010) 9(8):4274–81. doi:10.1021/pr100198m
127. Centlow M, Wingren C, Borrebaeck C, Brownstein MJ, Hansson SR. Differential gene expression analysis of placentas with increased vascular resistance and pre-eclampsia using whole-genome microarrays. J Pregnancy (2011) 2011:472354. doi:10.1155/2011/472354
128. Kang JH, Song H, Yoon JA, Park DY, Kim SH, Lee KJ, et al. Preeclampsia leads to dysregulation of various signaling pathways in placenta. J Hypertens (2011) 29(5):928–36. doi:10.1097/HJH.0b013e328344a82c
129. Liu C, Zhang N, Yu H, Chen Y, Liang Y, Deng H, et al. Proteomic analysis of human serum for finding pathogenic factors and potential biomarkers in preeclampsia. Placenta (2011) 32(2):168–74. doi:10.1016/j.placenta.2010.11.007
130. Nishizawa H, Ota S, Suzuki M, Kato T, Sekiya T, Kurahashi H, et al. Comparative gene expression profiling of placentas from patients with severe pre-eclampsia and unexplained fetal growth restriction. Reprod Biol Endocrinol (2011) 9:107. doi:10.1186/1477-7827-9-107
131. Tsai S, Hardison NE, James AH, Motsinger-Reif AA, Bischoff SR, Thames BH, et al. Transcriptional profiling of human placentas from pregnancies complicated by preeclampsia reveals disregulation of sialic acid acetylesterase and immune signalling pathways. Placenta (2011) 32(2):175–82. doi:10.1016/j.placenta.2010.11.014
132. Varkonyi T, Nagy B, Fule T, Tarca AL, Karaszi K, Schonleber J, et al. Microarray profiling reveals that placental transcriptomes of early-onset HELLP syndrome and preeclampsia are similar. Placenta (2011) 32(Suppl):S21–9. doi:10.1016/j.placenta.2010.04.014
133. Kolla V, Jeno P, Moes S, Lapaire O, Hoesli I, Hahn S. Quantitative proteomic (iTRAQ) analysis of 1st trimester maternal plasma samples in pregnancies at risk for preeclampsia. J Biomed Biotechnol (2012) 2012:305964. doi:10.1155/2012/305964
134. Lapaire O, Grill S, Lalevee S, Kolla V, Hosli I, Hahn S. Microarray screening for novel preeclampsia biomarker candidates. Fetal Diagn Ther (2012) 31(3):147–53. doi:10.1159/000337325
135. Kleinrouweler CE, van Uitert M, Moerland PD, Ris-Stalpers C, van der Post JA, Afink GB. Differentially expressed genes in the pre-eclamptic placenta: a systematic review and meta-analysis. PLoS One (2013) 8(7):e68991. doi:10.1371/journal.pone.0068991
136. Liu LY, Yang T, Ji J, Wen Q, Morgan AA, Jin B, et al. Integrating multiple ‘omics’ analyses identifies serological protein biomarkers for preeclampsia. BMC Med (2013) 11:236. doi:10.1186/1741-7015-11-236
137. Moslehi R, Mills JL, Signore C, Kumar A, Ambroggio X, Dzutsev A. Integrative transcriptome analysis reveals dysregulation of canonical cancer molecular pathways in placenta leading to preeclampsia. Sci Rep (2013) 3:2407. doi:10.1038/srep02407
138. Myers JE, Tuytten R, Thomas G, Laroy W, Kas K, Vanpoucke G, et al. Integrated proteomics pipeline yields novel biomarkers for predicting preeclampsia. Hypertension (2013) 61(6):1281–8. doi:10.1161/HYPERTENSIONAHA.113.01168
139. Wen Q, Liu LY, Yang T, Alev C, Wu S, Stevenson DK, et al. Peptidomic identification of serum peptides diagnosing preeclampsia. PLoS One (2013) 8(6):e65571. doi:10.1371/journal.pone.0065571
140. Leavey K, Bainbridge SA, Cox BJ. Large scale aggregate microarray analysis reveals three distinct molecular subclasses of human preeclampsia. PLoS One (2015) 10(2):e0116508. doi:10.1371/journal.pone.0116508
141. van Uitert M, Moerland PD, Enquobahrie DA, Laivuori H, van der Post JA, Ris-Stalpers C, et al. Meta-analysis of placental transcriptome data identifies a novel molecular pathway related to preeclampsia. PLoS One (2015) 10(7):e0132468. doi:10.1371/journal.pone.0132468
142. Leavey K, Benton SJ, Grynspan D, Kingdom JC, Bainbridge SA, Cox BJ. Unsupervised placental gene expression profiling identifies clinically relevant subclasses of human preeclampsia. Hypertension (2016) 68(1):137–47. doi:10.1161/HYPERTENSIONAHA.116.07293
143. Kolialexi A, Tsangaris GT, Sifakis S, Gourgiotis D, Katsafadou A, Lykoudi A, et al. Plasma biomarkers for the identification of women at risk for early-onset preeclampsia. Expert Rev Proteomics (2017) 14(3):269–76. doi:10.1080/14789450.2017.1291345
144. Wildman DE, Chen C, Erez O, Grossman LI, Goodman M, Romero R. Evolution of the mammalian placenta revealed by phylogenetic analysis. Proc Natl Acad Sci U S A (2006) 103(9):3203–8. doi:10.1073/pnas.0511344103
145. Carter AM. Comparative studies of placentation and immunology in non-human primates suggest a scenario for the evolution of deep trophoblast invasion and an explanation for human pregnancy disorders. Reproduction (2011) 141:391–6. doi:10.1530/REP-10-0530
146. Carter AM, Pijnenborg R. Evolution of invasive placentation with special reference to non-human primates. Best Pract Res Clin Obstet Gynaecol (2011) 25:249–57. doi:10.1016/j.bpobgyn.2010.10.010
147. McGowen MR, Erez O, Romero R, Wildman DE. The evolution of embryo implantation. Int J Dev Biol (2014) 58(2–4):155–61. doi:10.1387/ijdb.140020dw
148. Horii M, Li Y, Wakeland AK, Pizzo DP, Nelson KK, Sabatini K, et al. Human pluripotent stem cells as a model of trophoblast differentiation in both normal development and disease. Proc Natl Acad Sci U S A (2016) 113(27):E3882–91. doi:10.1073/pnas.1604747113
149. Yabe S, Alexenko AP, Amita M, Yang Y, Schust DJ, Sadovsky Y, et al. Comparison of syncytiotrophoblast generated from human embryonic stem cells and from term placentas. Proc Natl Acad Sci U S A (2016) 113(19):E2598–607. doi:10.1073/pnas.1601630113
150. Chang CW, Parast MM. Human trophoblast stem cells: real or not real? Placenta (2017) 60(Suppl 1):S57–60. doi:10.1016/j.placenta.2017.01.003
151. Su AI, Wiltshire T, Batalov S, Lapp H, Ching KA, Block D, et al. A gene atlas of the mouse and human protein-encoding transcriptomes. Proc Natl Acad Sci U S A (2004) 101(16):6062–7. doi:10.1073/pnas.0400782101
152. Rawn SM, Cross JC. The evolution, regulation, and function of placenta-specific genes. Annu Rev Cell Dev Biol (2008) 24:159–81. doi:10.1146/annurev.cellbio.24.110707.175418
153. Than NG, Romero R, Goodman M, Weckle A, Xing J, Dong Z, et al. A primate subfamily of galectins expressed at the maternal-fetal interface that promote immune cell death. Proc Natl Acad Sci U S A (2009) 106(24):9731–6. doi:10.1073/pnas.0903568106
154. Than NG, Abdul RO, Magenheim R, Nagy B, Fule T, Hargitai B, et al. Placental protein 13 (galectin-13) has decreased placental expression but increased shedding and maternal serum concentrations in patients presenting with preterm pre-eclampsia and HELLP syndrome. Virchows Arch (2008) 453(4):387–400. doi:10.1007/s00428-008-0658-x
155. Than NG, Romero R, Xu Y, Erez O, Xu Z, Bhatti G, et al. Evolutionary origins of the placental expression of chromosome 19 cluster galectins and their complex dysregulation in preeclampsia. Placenta (2014) 35(11):855–65. doi:10.1016/j.placenta.2014.07.015
156. Grimwood J, Gordon LA, Olsen A, Terry A, Schmutz J, Lamerdin J, et al. The DNA sequence and biology of human chromosome 19. Nature (2004) 428(6982):529–35. doi:10.1038/nature02399
157. Murphy VE, Zakar T, Smith R, Giles WB, Gibson PG, Clifton VL. Reduced 11beta-hydroxysteroid dehydrogenase type 2 activity is associated with decreased birth weight centile in pregnancies complicated by asthma. J Clin Endocrinol Metab (2002) 87(4):1660–8. doi:10.1210/jc.87.4.1660
158. Mannik J, Vaas P, Rull K, Teesalu P, Rebane T, Laan M. Differential expression profile of growth hormone/chorionic somatomammotropin genes in placenta of small- and large-for-gestational-age newborns. J Clin Endocrinol Metab (2010) 95(5):2433–42. doi:10.1210/jc.2010-0023
159. Poidatz D, Dos Santos E, Brule A, De Mazancourt P, Dieudonne MN. Estrogen-related receptor gamma modulates energy metabolism target genes in human trophoblast. Placenta (2012) 33(9):688–95. doi:10.1016/j.placenta.2012.06.002
160. Ohkuchi A, Ishibashi O, Hirashima C, Takahashi K, Matsubara S, Takizawa T, et al. Plasma level of hydroxysteroid (17-beta) dehydrogenase 1 in the second trimester is an independent risk factor for predicting preeclampsia after adjusting for the effects of mean blood pressure, bilateral notching and plasma level of soluble fms-like tyrosine kinase 1/placental growth factor ratio. Hypertens Res (2012) 35(12):1152–8. doi:10.1038/hr.2012.109
161. Hertig A, Liere P, Chabbert-Buffet N, Fort J, Pianos A, Eychenne B, et al. Steroid profiling in preeclamptic women: evidence for aromatase deficiency. Am J Obstet Gynecol (2010) 203(5):477–9. doi:10.1016/j.ajog.2010.06.011
162. Ishibashi O, Ohkuchi A, Ali MM, Kurashina R, Luo SS, Ishikawa T, et al. Hydroxysteroid (17-beta) dehydrogenase 1 is dysregulated by miR-210 and miR-518c that are aberrantly expressed in preeclamptic placentas: a novel marker for predicting preeclampsia. Hypertension (2012) 59(2):265–73. doi:10.1161/HYPERTENSIONAHA.111.180232
163. Kumar P, Mendelson CR. Estrogen-related receptor gamma (ERRgamma) mediates oxygen-dependent induction of aromatase (CYP19) gene expression during human trophoblast differentiation. Mol Endocrinol (2011) 25(9):1513–26. doi:10.1210/me.2011-1012
164. Gupta R, Ezashi T, Roberts RM. Squelching of ETS2 transactivation by POU5F1 silences the human chorionic gonadotropin CGA subunit gene in human choriocarcinoma and embryonic stem cells. Mol Endocrinol (2012) 26(5):859–72. doi:10.1210/me.2011-1146
165. Groner AC, Meylan S, Ciuffi A, Zangger N, Ambrosini G, Denervaud N, et al. KRAB-zinc finger proteins and KAP1 can mediate long-range transcriptional repression through heterochromatin spreading. PLoS Genet (2010) 6(3):e1000869. doi:10.1371/journal.pgen.1000869
166. Maltepe E, Keith B, Arsham AM, Brorson JR, Simon MC. The role of ARNT2 in tumor angiogenesis and the neural response to hypoxia. Biochem Biophys Res Commun (2000) 273(1):231–8. doi:10.1006/bbrc.2000.2928
167. Nevo O, Soleymanlou N, Wu Y, Xu J, Kingdom J, Many A, et al. Increased expression of sFlt-1 in in vivo and in vitro models of human placental hypoxia is mediated by HIF-1. Am J Physiol Regul Integr Comp Physiol (2006) 291(4):R1085–93. doi:10.1152/ajpregu.00794.2005
168. Rosario GX, Konno T, Soares MJ. Maternal hypoxia activates endovascular trophoblast cell invasion. Dev Biol (2008) 314(2):362–75. doi:10.1016/j.ydbio.2007.12.007
169. Chakraborty D, Cui W, Rosario GX, Scott RL, Dhakal P, Renaud SJ, et al. HIF-KDM3A-MMP12 regulatory circuit ensures trophoblast plasticity and placental adaptations to hypoxia. Proc Natl Acad Sci U S A (2016) 113(46):E7212–21. doi:10.1073/pnas.1612626113
170. Palmer SK, Moore LG, Young D, Cregger B, Berman JC, Zamudio S. Altered blood pressure course during normal pregnancy and increased preeclampsia at high altitude (3100 meters) in Colorado. Am J Obstet Gynecol (1999) 180(5):1161–8. doi:10.1016/S0002-9378(99)70611-3
171. Bigham AW, Lee FS. Human high-altitude adaptation: forward genetics meets the HIF pathway. Genes Dev (2014) 28(20):2189–204. doi:10.1101/gad.250167.114
172. Ivanov SV, Salnikow K, Ivanova AV, Bai L, Lerman MI. Hypoxic repression of STAT1 and its downstream genes by a pVHL/HIF-1 target DEC1/STRA13. Oncogene (2007) 26(6):802–12. doi:10.1038/sj.onc.1209842
173. Moriyama M, Yamochi T, Semba K, Akiyama T, Mori S. BCL-6 is phosphorylated at multiple sites in its serine- and proline-clustered region by mitogen-activated protein kinase (MAPK) in vivo. Oncogene (1997) 14(20):2465–74. doi:10.1038/sj.onc.1201084
174. Muschol-Steinmetz C, Jasmer B, Kreis NN, Steinhauser K, Ritter A, Rolle U, et al. B-cell lymphoma 6 promotes proliferation and survival of trophoblastic cells. Cell Cycle (2016) 15(6):827–39. doi:10.1080/15384101.2016.1149273
175. Hansson SR, Naav A, Erlandsson L. Oxidative stress in preeclampsia and the role of free fetal hemoglobin. Front Physiol (2014) 5:516. doi:10.3389/fphys.2014.00516
176. Sun K, Jiang P, Chan KC, Wong J, Cheng YK, Liang RH, et al. Plasma DNA tissue mapping by genome-wide methylation sequencing for noninvasive prenatal, cancer, and transplantation assessments. Proc Natl Acad Sci U S A (2015) 112(40):E5503–12. doi:10.1073/pnas.1508736112
177. Nagy B, Csanadi Z, Poka R. The importance of “free” nucleic acids in the non-invasive diagnostics. Orv Hetil (2016) 157(48):1900–9. doi:10.1556/650.2016.30621
178. Lindberg BS, Nilsson BA. Human placental lactogen (HPL) levels in abnormal pregnancies. J Obstet Gynaecol Br Commonw (1973) 80(12):1046–53. doi:10.1111/j.1471-0528.1973.tb02978.x
179. Ergüler E, Özel N, Ergüler G, Kücükoglu E. Human placental lactogen levels in preeclampsia-eclampsia. Gaizan Üniv Tip Fakült Derg (1993) 4:237–41.
180. Luckas M, Hawe J, Meekins J, Neilson J, Walkinshaw S. Second trimester serum free beta human chorionic gonadotrophin levels as a predictor of pre-eclampsia. Acta Obstet Gynecol Scand (1998) 77(4):381–4. doi:10.1034/j.1600-0412.1998.770404.x
181. Mise H, Sagawa N, Matsumoto T, Yura S, Nanno H, Itoh H, et al. Augmented placental production of leptin in preeclampsia: possible involvement of placental hypoxia. J Clin Endocrinol Metab (1998) 83(9):3225–9. doi:10.1210/jc.83.9.3225
182. Gökdeniz R, Ariguloglu E, Bazoglu N, Balat Ö. Elevated serum b-hCG levels in severe preeclampsia. Turk J Med Sci (2000) 30:43–5.
183. Bartha JL, Romero-Carmona R, Escobar-Llompart M, Comino-Delgado R. The relationships between leptin and inflammatory cytokines in women with pre-eclampsia. BJOG (2001) 108(12):1272–6. doi:10.1111/j.1471-0528.2001.00284.x
184. Bersinger NA, Odegard RA. Second- and third-trimester serum levels of placental proteins in preeclampsia and small-for-gestational age pregnancies. Acta Obstet Gynecol Scand (2004) 83(1):37–45. doi:10.1111/j.1600-0412.2004.00277.x
185. Ning Y, Williams MA, Muy-Rivera M, Leisenring WM, Luthy DA. Relationship of maternal plasma leptin and risk of pre-eclampsia: a prospective study. J Matern Fetal Neonatal Med (2004) 15(3):186–92. doi:10.1080/14767050410001668293
186. Kharfi A, Giguere Y, De Grandpre P, Moutquin JM, Forest JC. Human chorionic gonadotropin (hCG) may be a marker of systemic oxidative stress in normotensive and preeclamptic term pregnancies. Clin Biochem (2005) 38(8):717–21. doi:10.1016/j.clinbiochem.2005.04.011
187. Spencer K, Yu CK, Cowans NJ, Otigbah C, Nicolaides KH. Prediction of pregnancy complications by first-trimester maternal serum PAPP-A and free beta-hCG and with second-trimester uterine artery Doppler. Prenat Diagn (2005) 25(10):949–53. doi:10.1002/pd.1251
188. Spencer K, Yu CK, Savvidou M, Papageorghiou AT, Nicolaides KH. Prediction of pre-eclampsia by uterine artery Doppler ultrasonography and maternal serum pregnancy-associated plasma protein-A, free beta-human chorionic gonadotropin, activin A and inhibin A at 22 + 0 to 24 + 6 weeks’ gestation. Ultrasound Obstet Gynecol (2006) 27(6):658–63. doi:10.1002/uog.2676
189. Banzola I, Farina A, Concu M, Sekizawa A, Purwosunu Y, Strada I, et al. Performance of a panel of maternal serum markers in predicting preeclampsia at 11-15 weeks’ gestation. Prenat Diagn (2007) 27(11):1005–10. doi:10.1002/pd.1821
190. Ouyang Y, Chen H, Chen H. Reduced plasma adiponectin and elevated leptin in pre-eclampsia. Int J Gynaecol Obstet (2007) 98(2):110–4. doi:10.1016/j.ijgo.2007.04.021
191. Rana S, Karumanchi SA, Levine RJ, Venkatesha S, Rauh-Hain JA, Tamez H, et al. Sequential changes in antiangiogenic factors in early pregnancy and risk of developing preeclampsia. Hypertension (2007) 50(1):137–42. doi:10.1161/HYPERTENSIONAHA.107.087700
192. Zwahlen M, Gerber S, Bersinger NA. First trimester markers for pre-eclampsia: placental vs. non-placental protein serum levels. Gynecol Obstet Invest (2007) 63(1):15–21. doi:10.1159/000094672
193. De Vivo A, Baviera G, Giordano D, Todarello G, Corrado F, D’Anna R. Endoglin, PlGF and sFlt-1 as markers for predicting pre-eclampsia. Acta Obstet Gynecol Scand (2008) 87(8):837–42. doi:10.1080/00016340802253759
194. Diab AE, El-Behery MM, Ebrahiem MA, Shehata AE. Angiogenic factors for the prediction of pre-eclampsia in women with abnormal midtrimester uterine artery Doppler velocimetry. Int J Gynaecol Obstet (2008) 102(2):146–51. doi:10.1016/j.ijgo.2008.02.016
195. Erez O, Romero R, Espinoza J, Fu W, Todem D, Kusanovic JP, et al. The change in concentrations of angiogenic and anti-angiogenic factors in maternal plasma between the first and second trimesters in risk assessment for the subsequent development of preeclampsia and small-for-gestational age. J Matern Fetal Neonatal Med (2008) 21(5):279–87. doi:10.1080/14767050802034545
196. Gonen R, Shahar R, Grimpel YI, Chefetz I, Sammar M, Meiri H, et al. Placental protein 13 as an early marker for pre-eclampsia: a prospective longitudinal study. BJOG (2008) 115(12):1465–72. doi:10.1111/j.1471-0528.2008.01902.x
197. Kang JH, Farina A, Park JH, Kim SH, Kim JY, Rizzo N, et al. Down syndrome biochemical markers and screening for preeclampsia at first and second trimester: correlation with the week of onset and the severity. Prenat Diagn (2008) 28(8):704–9. doi:10.1002/pd.1997
198. Stepan H, Geipel A, Schwarz F, Kramer T, Wessel N, Faber R. Circulatory soluble endoglin and its predictive value for preeclampsia in second-trimester pregnancies with abnormal uterine perfusion. Am J Obstet Gynecol (2008) 198(2):175–6. doi:10.1016/j.ajog.2007.08.052
199. Durkovic J, Mandic B. Human placental lactogen in the third trimester of pregnancy. J Med Biochem (2009) 28(2):97–100. doi:10.2478/v10011-009-0003-1
200. El-Baradie SM, Mahmoud M, Makhlouf HH. Elevated serum levels of interleukin-15, interleukin-16, and human chorionic gonadotropin in women with preeclampsia. J Obstet Gynaecol Can (2009) 31(2):142–8. doi:10.1016/S1701-2163(16)34098-1
201. Kim YN, Lee DS, Jeong DH, Sung MS, Kim KT. The relationship of the level of circulating antiangiogenic factors to the clinical manifestations of preeclampsia. Prenat Diagn (2009) 29(5):464–70. doi:10.1002/pd.2203
202. Kusanovic JP, Romero R, Chaiworapongsa T, Erez O, Mittal P, Vaisbuch E, et al. A prospective cohort study of the value of maternal plasma concentrations of angiogenic and anti-angiogenic factors in early pregnancy and midtrimester in the identification of patients destined to develop preeclampsia. J Matern Fetal Neonatal Med (2009) 22(11):1021–38. doi:10.3109/14767050902994754
203. Stepan H, Ebert T, Schrey S, Reisenbuchler C, Bluher M, Stumvoll M, et al. Preliminary report: Serum levels of retinol-binding protein 4 in preeclampsia. Metabolism (2009) 58(3):275–7. doi:10.1016/j.metabol.2008.10.001
204. Foidart JM, Munaut C, Chantraine F, Akolekar R, Nicolaides KH. Maternal plasma soluble endoglin at 11-13 weeks’ gestation in pre-eclampsia. Ultrasound Obstet Gynecol (2010) 35(6):680–7. doi:10.1002/uog.7621
205. Gaber K, Hamdy E, Hanafy A. Soluble endoglin as a new marker for prediction of pre-eclampsia in early pregnancy. Middle East Fertil Soc J (2010) 15(1):42–6. doi:10.1016/j.mefs.2010.03.009
206. Masuyama H, Segawa T, Sumida Y, Masumoto A, Inoue S, Akahori Y, et al. Different profiles of circulating angiogenic factors and adipocytokines between early- and late-onset pre-eclampsia. BJOG (2010) 117(3):314–20. doi:10.1111/j.1471-0528.2009.02453.x
207. Papastefanou I, Samolis S, Panagopoulos P, Tagia M, Bale C, Kouskoukis A, et al. Correlation between maternal first trimester plasma leptin levels and birth weight among normotensive and preeclamptic women. J Matern Fetal Neonatal Med (2010) 23(12):1435–43. doi:10.3109/14767051003678283
208. Stepan H, Kralisch S, Klostermann K, Schrey S, Reisenbuchler C, Verlohren M, et al. Preliminary report: circulating levels of the adipokine vaspin in gestational diabetes mellitus and preeclampsia. Metabolism (2010) 59(7):1054–6. doi:10.1016/j.metabol.2009.11.001
209. Wortelboer EJ, Koster MP, Cuckle HS, Stoutenbeek PH, Schielen PC, Visser GH. First-trimester placental protein 13 and placental growth factor: markers for identification of women destined to develop early-onset pre-eclampsia. BJOG (2010) 117(11):1384–9. doi:10.1111/j.1471-0528.2010.02690.x
210. Dayal M, Gupta P, Manju V, Ghosh UK, Bhargava A. Role of second trimester maternal serum markers as predictor of preeclampsia. J Obstet Gynecol India (2011) 61(1):38–41.
211. Stepan H, Philipp A, Roth I, Kralisch S, Jank A, Schaarschmidt W, et al. Serum levels of the adipokine chemerin are increased in preeclampsia during and 6 months after pregnancy. Regul Pept (2011) 168(1–3):69–72. doi:10.1016/j.regpep.2011.03.005
212. Youssef A, Righetti F, Morano D, Rizzo N, Farina A. Uterine artery Doppler and biochemical markers (PAPP-A, PIGF, sFlt-1, P-selectin, NGAL) at 11 + 0 to 13 + 6 weeks in the prediction of late (> 34 weeks) pre-eclampsia. Prenat Diagn (2011) 31(12):1141–6. doi:10.1002/pd.2848
213. Nanda S, Akolekar R, Acosta IC, Wierzbicka D, Nicolaides KH. Maternal serum leptin at 11-13 weeks gestation in normal and pathological pregnancies. Metabolic Synd. (2012) 1:113. doi:10.4172/2167-0943.1000113
214. Perni U, Sison C, Sharma V, Helseth G, Hawfield A, Suthanthiran M, et al. Angiogenic factors in superimposed preeclampsia: a longitudinal study of women with chronic hypertension during pregnancy. Hypertension (2012) 59(3):740–6. doi:10.1161/HYPERTENSIONAHA.111.181735
215. Diguisto C, Le Gouge A, Piver E, Giraudeau B, Perrotin F. Second-trimester uterine artery Doppler, PlGF, sFlt-1, sEndoglin, and lipid-related markers for predicting preeclampsia in a high-risk population. Prenat Diagn (2013) 33(11):1070–4. doi:10.1002/pd.4198
216. Hassan MF, Rund NM, Salama AH. An elevated maternal plasma soluble fms-Like tyrosine kinase-1 to placental growth factor ratio at midtrimester is a useful predictor for preeclampsia. Obstet Gynecol Int (2013) 2013:202346. doi:10.1155/2013/202346
217. Keikkala E, Vuorela P, Laivuori H, Romppanen J, Heinonen S, Stenman UH. First trimester hyperglycosylated human chorionic gonadotrophin in serum - a marker of early-onset preeclampsia. Placenta (2013) 34(11):1059–65. doi:10.1016/j.placenta.2013.08.006
218. Stepan H, Richter J, Kley K, Kralisch S, Jank A, Schaarschmidt W, et al. Serum levels of growth arrest specific protein 6 are increased in preeclampsia. Regul Pept (2013) 182:7–11. doi:10.1016/j.regpep.2012.12.013
219. Suri S, Muttukrishna S, Jauniaux E. 2D-Ultrasound and endocrinologic evaluation of placentation in early pregnancy and its relationship to fetal birthweight in normal pregnancies and pre-eclampsia. Placenta (2013) 34(9):745–50. doi:10.1016/j.placenta.2013.05.003
220. Villa PM, Hamalainen E, Maki A, Raikkonen K, Pesonen AK, Taipale P, et al. Vasoactive agents for the prediction of early- and late-onset preeclampsia in a high-risk cohort. BMC Pregnancy Childbirth (2013) 13:110. doi:10.1186/1471-2393-13-110
221. Begum Z, Ara I, Tanira S, Keya K. The association between serum beta-human chorionic gonadotropin and preeclampsia. J Dhaka Med Coll. (2014) 23(1):89–93.
222. Camejo MI, Casart YC. Relation between human chorionic gonadotropin and thyroid hormones in preeclampsia. Open Access Library J (2014) 1:e652. doi:10.4236/oalib.1100652
223. Kulmala L, Phupong V. Combination of plasma-soluble fms-like tyrosine kinase 1 and uterine artery Doppler for the prediction of preeclampsia in cases of elderly gravida. Hypertens Res (2014) 37(6):538–42. doi:10.1038/hr.2014.27
224. Salimi S, Farajian-Mashhadi F, Naghavi A, Mokhtari M, Shahrakipour M, Saravani M, et al. Different profile of serum leptin between early onset and late onset preeclampsia. Dis Markers (2014) 2014:628476. doi:10.1155/2014/628476
225. Tobinaga CM, Torloni MR, Gueuvoghlanian-Silva BY, Pendeloski KP, Akita PA, Sass N, et al. Angiogenic factors and uterine Doppler velocimetry in early- and late-onset preeclampsia. Acta Obstet Gynecol Scand (2014) 93(5):469–76. doi:10.1111/aogs.12366
226. Crovetto F, Figueras F, Triunfo S, Crispi F, Rodriguez-Sureda V, Dominguez C, et al. First trimester screening for early and late preeclampsia based on maternal characteristics, biophysical parameters, and angiogenic factors. Prenat Diagn (2015) 35(2):183–91. doi:10.1002/pd.4519
227. Kalinderis M, Papanikolaou A, Kalinderi K, Vyzantiadis TA, Ioakimidou A, Tarlatzis BC. Serum levels of leptin and IP-10 in preeclampsia compared to controls. Arch Gynecol Obstet (2015) 292(2):343–7. doi:10.1007/s00404-015-3659-4
228. Rahman S, Zahoor AS. Serum leptin levels as a marker for severity of pre-eclampsia. KJMS. (2015) 8(1):85–8.
229. Taylor BD, Ness RB, Olsen J, Hougaard DM, Skogstrand K, Roberts JM, et al. Serum leptin measured in early pregnancy is higher in women with preeclampsia compared with normotensive pregnant women. Hypertension (2015) 65(3):594–9. doi:10.1161/HYPERTENSIONAHA.114.03979
230. Karampas GA, Eleftheriades MI, Panoulis KC, Rizou MD, Haliassos AD, Metallinou DK, et al. Prediction of pre-eclampsia combining NGAL and other biochemical markers with Doppler in the first and/or second trimester of pregnancy. A pilot study. Eur J Obstet Gynecol Reprod Biol (2016) 205:153–7. doi:10.1016/j.ejogrb.2016.08.034
231. Mohamed FA, El-Salam MA, Al-Nori MA, Asser T, Ismail Y. Uterine artery diastolic notch and serum leptin assay for prediction of pre-eclampsia. Med J Cairo Univ (2016) 84(2):251–6.
232. Poveda NE, Garces MF, Ruiz-Linares CE, Varon D, Valderrama S, Sanchez E, et al. Serum adipsin levels throughout normal pregnancy and preeclampsia. Sci Rep (2016) 6:20073. doi:10.1038/srep20073
233. Song Y, Gao J, Qu Y, Wang S, Wang X, Liu J. Serum levels of leptin, adiponectin and resistin in relation to clinical characteristics in normal pregnancy and preeclampsia. Clin Chim Acta (2016) 458:133–7. doi:10.1016/j.cca.2016.04.036
234. Rampersad R, Barton A, Sadovsky Y, Nelson DM. The C5b-9 membrane attack complex of complement activation localizes to villous trophoblast injury in vivo and modulates human trophoblast function in vitro. Placenta (2008) 29(10):855–61. doi:10.1016/j.placenta.2008.07.008
235. Zhou CC, Zhang Y, Irani RA, Zhang H, Mi T, Popek EJ, et al. Angiotensin receptor agonistic autoantibodies induce pre-eclampsia in pregnant mice. Nat Med (2008) 14(8):855–62. doi:10.1038/nm.1856
236. Girardi G, Prohaszka Z, Bulla R, Tedesco F, Scherjon S. Complement activation in animal and human pregnancies as a model for immunological recognition. Mol Immunol (2011) 48(14):1621–30. doi:10.1016/j.molimm.2011.04.011
237. Lokki AI, Heikkinen-Eloranta J, Jarva H, Saisto T, Lokki ML, Laivuori H, et al. Complement activation and regulation in preeclamptic placenta. Front Immunol (2014) 5:312. doi:10.3389/fimmu.2014.00312
238. Kouser L, Madhukaran SP, Shastri A, Saraon A, Ferluga J, Al-Mozaini M, et al. Emerging and novel functions of complement protein C1q. Front Immunol (2015) 6:317. doi:10.3389/fimmu.2015.00317
239. Bolin M, Wikstrom AK, Wiberg-Itzel E, Sundstrom-Poromaa I, Axelsson O, Thilaganathan B, et al. Prediction of preterm preeclampsia by combining histidine-rich glycoprotein and uterine artery Doppler in early pregnancy. Pregnancy Hypertens (2011) 1(3–4):282–3. doi:10.1016/j.preghy.2011.08.081
240. Tannetta DS, Redman CW, Sargent IL. Investigation of the actin scavenging system in pre-eclampsia. Eur J Obstet Gynecol Reprod Biol (2014) 172:32–5. doi:10.1016/j.ejogrb.2013.10.022
241. Anderson UD, Gram M, Ranstam J, Thilaganathan B, Kerstrom B, Hansson SR. Fetal hemoglobin, alpha1-microglobulin and hemopexin are potential predictive first trimester biomarkers for preeclampsia. Pregnancy Hypertens (2016) 6(2):103–9. doi:10.1016/j.preghy.2016.02.003
242. Burton GJ. Oxygen, the Janus gas; its effects on human placental development and function. J Anat (2009) 215(1):27–35. doi:10.1111/j.1469-7580.2008.00978.x
243. Lai AY, Fatemi M, Dhasarathy A, Malone C, Sobol SE, Geigerman C, et al. DNA methylation prevents CTCF-mediated silencing of the oncogene BCL6 in B cell lymphomas. J Exp Med (2010) 207(9):1939–50. doi:10.1084/jem.20100204
244. Lynch VJ, Leclerc RD, May G, Wagner GP. Transposon-mediated rewiring of gene regulatory networks contributed to the evolution of pregnancy in mammals. Nat Genet (2011) 43(11):1154–9. doi:10.1038/ng.917
245. Emera D, Wagner GP. Transposable element recruitments in the mammalian placenta: impacts and mechanisms. Brief Funct Genomics (2012) 11(4):267–76. doi:10.1093/bfgp/els013
246. Chuong EB, Rumi MA, Soares MJ, Baker JC. Endogenous retroviruses function as species-specific enhancer elements in the placenta. Nat Genet (2013) 45(3):325–9. doi:10.1038/ng.2553
247. Lynch VJ, Nnamani MC, Kapusta A, Brayer K, Plaza SL, Mazur EC, et al. Ancient transposable elements transformed the uterine regulatory landscape and transcriptome during the evolution of mammalian pregnancy. Cell Rep (2015) 10(4):551–61. doi:10.1016/j.celrep.2014.12.052
248. Huh JW, Ha HS, Kim DS, Kim HS. Placenta-restricted expression of LTR-derived NOS3. Placenta (2008) 29(7):602–8. doi:10.1016/j.placenta.2008.04.002
249. Schneyer A, Schoen A, Quigg A, Sidis Y. Differential binding and neutralization of activins A and B by follistatin and follistatin like-3 (FSTL-3/FSRP/FLRG). Endocrinology (2003) 144(5):1671–4. doi:10.1210/en.2002-0203
250. Knofler M, Pollheimer J. Human placental trophoblast invasion and differentiation: a particular focus on Wnt signaling. Front Genet (2013) 4:190. doi:10.3389/fgene.2013.00190
251. Li Y, Klausen C, Cheng JC, Zhu H, Leung PC. Activin A, B, and AB increase human trophoblast cell invasion by up-regulating N-cadherin. J Clin Endocrinol Metab (2014) 99(11):E2216–25. doi:10.1210/jc.2014-2118
252. Pryor-Koishi K, Nishizawa H, Kato T, Kogo H, Murakami T, Tsuchida K, et al. Overproduction of the follistatin-related gene protein in the placenta and maternal serum of women with pre-eclampsia. BJOG (2007) 114(9):1128–37. doi:10.1111/j.1471-0528.2007.01425.x
253. Graham CH, Hawley TS, Hawley RG, MacDougall JR, Kerbel RS, Khoo N, et al. Establishment and characterization of first trimester human trophoblast cells with extended lifespan. Exp Cell Res (1993) 206(2):204–11. doi:10.1006/excr.1993.1139
254. Steinman RA. Cell cycle regulators and hematopoiesis. Oncogene (2002) 21(21):3403–13. doi:10.1038/sj.onc.1205325
255. Zhang J, Zhang J, Zhao C, Shen R, Guo X, Li C, et al. Analysis of transcription factor Stk40 expression and function during mouse pre-implantation embryonic development. Mol Med Rep (2014) 9(2):535–40. doi:10.3892/mmr.2013.1828
256. Huber AV, Saleh L, Bauer S, Husslein P, Knofler M. TNFalpha-mediated induction of PAI-1 restricts invasion of HTR-8/SVneo trophoblast cells. Placenta (2006) 27(2–3):127–36. doi:10.1016/j.placenta.2005.02.012
257. Prutsch N, Fock V, Haslinger P, Haider S, Fiala C, Pollheimer J, et al. The role of interleukin-1beta in human trophoblast motility. Placenta (2012) 33(9):696–703. doi:10.1016/j.placenta.2012.05.008
258. Bass KE, Li H, Hawkes SP, Howard E, Bullen E, Vu TK, et al. Tissue inhibitor of metalloproteinase-3 expression is upregulated during human cytotrophoblast invasion in vitro. Dev Genet (1997) 21(1):61–7. doi:10.1002/(SICI)1520-6408(1997)21:1<61::AID-DVG7>3.0.CO;2-6
259. Giudice LC. Genes associated with embryonic attachment and implantation and the role of progesterone. J Reprod Med (1999) 44(2 Suppl):165–71.
260. Hellmann-Blumberg U, Hintz MF, Gatewood JM, Schmid CW. Developmental differences in methylation of human Alu repeats. Mol Cell Biol (1993) 13(8):4523–30. doi:10.1128/MCB.13.8.4523
261. Price EM, Cotton AM, Penaherrera MS, McFadden DE, Kobor MS, Robinson W. Different measures of “genome-wide” DNA methylation exhibit unique properties in placental and somatic tissues. Epigenetics (2012) 7(6):652–63. doi:10.4161/epi.20221
262. Gimenez J, Montgiraud C, Oriol G, Pichon JP, Ruel K, Tsatsaris V, et al. Comparative methylation of ERVWE1/syncytin-1 and other human endogenous retrovirus LTRs in placenta tissues. DNA Res (2009) 16(4):195–211. doi:10.1093/dnares/dsp011
263. Li F, Karlsson H. Expression and regulation of human endogenous retrovirus W elements. APMIS (2016) 124(1–2):52–66. doi:10.1111/apm.12478
264. Macaulay EC, Weeks RJ, Andrews S, Morison IM. Hypomethylation of functional retrotransposon-derived genes in the human placenta. Mamm Genome (2011) 22(11–12):722–35. doi:10.1007/s00335-011-9355-1
265. Wang K, Lee I, Carlson G, Hood L, Galas D. Systems biology and the discovery of diagnostic biomarkers. Dis Markers (2010) 28(4):199–207. doi:10.3233/DMA-2010-0697
266. Loscalzo J, Barabasi AL. Systems biology and the future of medicine. Syst Biol Med. (2011) 3(6):619–27. doi:10.1002/wsbm.144
267. Vidal M, Cusick ME, Barabasi AL. Interactome networks and human disease. Cell (2011) 144(6):986–98. doi:10.1016/j.cell.2011.02.016
268. Hood L, Balling R, Auffray C. Revolutionizing medicine in the 21st century through systems approaches. Biotechnol J (2012) 7(8):992–1001. doi:10.1002/biot.201100306
269. Junus K, Centlow M, Wikstrom AK, Larsson I, Hansson SR, Olovsson M. Gene expression profiling of placentae from women with early- and late-onset pre-eclampsia: down-regulation of the angiogenesis-related genes ACVRL1 and EGFL7 in early-onset disease. Mol Hum Reprod (2012) 18(3):146–55. doi:10.1093/molehr/gar067
270. Song Y, Liu J, Huang S, Zhang L. Analysis of differentially expressed genes in placental tissues of preeclampsia patients using microarray combined with the Connectivity Map database. Placenta (2013) 34(12):1190–5. doi:10.1016/j.placenta.2013.09.013
271. Louwen F, Muschol-Steinmetz C, Friemel A, Kampf AK, Tottel E, Reinhard J, et al. Targeted gene analysis: increased B-cell lymphoma 6 in preeclamptic placentas. Hum Pathol (2014) 45(6):1234–42. doi:10.1016/j.humpath.2014.02.002
272. Kaartokallio T, Cervera A, Kyllonen A, Laivuori K, Kere J, Laivuori H, et al. Gene expression profiling of pre-eclamptic placentae by RNA sequencing. Sci Rep (2015) 5:14107. doi:10.1038/srep14107
273. Jauniaux E, Watson AL, Hempstock J, Bao YP, Skepper JN, Burton GJ. Onset of maternal arterial blood flow and placental oxidative stress. A possible factor in human early pregnancy failure. Am J Pathol (2000) 157(6):2111–22. doi:10.1016/S0002-9440(10)64849-3
274. Xiong Y, Liebermann DA, Holtzman EJ, Jeronis S, Hoffman B, Geifman-Holtzman O. Preeclampsia-associated stresses activate Gadd45a signaling and sFlt-1 in placental explants. J Cell Physiol (2013) 228(2):362–70. doi:10.1002/jcp.24139
275. Aoki Y, Yamamoto T, Fumihisa C, Nakamura A, Asanuma A, Suzuki M. Effect on the production of soluble endoglin from human choriocarcinoma cells by preeclampsia sera. Am J Reprod Immunol (2012) 67(5):413–20. doi:10.1111/j.1600-0897.2011.01086.x
276. Savoia C, Schiffrin EL. Inflammation in hypertension. Curr Opin Nephrol Hypertens (2006) 15(2):152–8. doi:10.1097/01.mnh.0000203189.57513.76
277. Hanna J, Wald O, Goldman-Wohl D, Prus D, Markel G, Gazit R, et al. CXCL12 expression by invasive trophoblasts induces the specific migration of CD16- human natural killer cells. Blood (2003) 102(5):1569–77. doi:10.1182/blood-2003-02-0517
278. Hanna J, Goldman-Wohl D, Hamani Y, Avraham I, Greenfield C, Natanson-Yaron S, et al. Decidual NK cells regulate key developmental processes at the human fetal-maternal interface. Nat Med (2006) 12(9):1065–74. doi:10.1038/nm1452
279. Moffett A, Loke C. Immunology of placentation in eutherian mammals. Nat Rev Immunol (2006) 6(8):584–94. doi:10.1038/nri1897
280. Sargent IL, Borzychowski AM, Redman CW. NK cells and human pregnancy – an inflammatory view. Trends Immunol (2006) 27(9):399–404. doi:10.1016/j.it.2006.06.009
281. Moffett A, Hiby SE. How does the maternal immune system contribute to the development of pre-eclampsia? Placenta (2007) 28(Suppl A):S51–6. doi:10.1016/j.placenta.2006.11.008
282. Chakraborty D, Rumi MA, Soares MJ. NK cells, hypoxia and trophoblast cell differentiation. Cell Cycle (2012) 11(13):2427–30. doi:10.4161/cc.20542
283. Xiong S, Sharkey AM, Kennedy PR, Gardner L, Farrell LE, Chazara O, et al. Maternal uterine NK cell-activating receptor KIR2DS1 enhances placentation. J Clin Invest (2013) 123(10):4264–72. doi:10.1172/JCI68991
284. Kliman HJ, Sammar M, Grimpel YI, Lynch SK, Milano KM, Pick E, et al. Placental protein 13 and decidual zones of necrosis: an immunologic diversion that may be linked to preeclampsia. Reprod Sci (2012) 19(1):16–30. doi:10.1177/1933719111424445
285. Faas MM, Spaans F, De Vos P. Monocytes and macrophages in pregnancy and pre-eclampsia. Front Immunol (2014) 5:298. doi:10.3389/fimmu.2014.00298
286. Saito S, Sakai M. Th1/Th2 balance in preeclampsia. J Reprod Immunol (2003) 59(2):161–73. doi:10.1016/S0165-0378(03)00045-7
287. Saito S, Sakai M, Sasaki Y, Nakashima A, Shiozaki A. Inadequate tolerance induction may induce pre-eclampsia. J Reprod Immunol (2007) 76(1–2):30–9. doi:10.1016/j.jri.2007.08.002
288. Sasaki Y, Darmochwal-Kolarz D, Suzuki D, Sakai M, Ito M, Shima T, et al. Proportion of peripheral blood and decidual CD4(+) CD25(bright) regulatory T cells in pre-eclampsia. Clin Exp Immunol (2007) 149(1):139–45. doi:10.1111/j.1365-2249.2007.03397.x
289. Saito S. Th17 cells and regulatory T cells: new light on pathophysiology of preeclampsia. Immunol Cell Biol (2010) 88(6):615–7. doi:10.1038/icb.2010.68
290. Ahmed A, Singh J, Khan Y, Seshan SV, Girardi G. A new mouse model to explore therapies for preeclampsia. PLoS One (2010) 5(10):e13663. doi:10.1371/journal.pone.0013663
291. Singh J, Ahmed A, Girardi G. Role of complement component C1q in the onset of preeclampsia in mice. Hypertension (2011) 58(4):716–24. doi:10.1161/HYPERTENSIONAHA.111.175919
292. Girardi G. Complement activation, a threat to pregnancy. Semin Immunopathol (2018) 40(1):103–11. doi:10.1007/s00281-017-0645-x
293. Faas MM, Schuiling GA, Baller JF, Visscher CA, Bakker WW. A new animal model for human preeclampsia: ultra-low-dose endotoxin infusion in pregnant rats. Am J Obstet Gynecol (1994) 171(1):158–64. doi:10.1016/0002-9378(94)90463-4
294. Santner-Nanan B, Peek MJ, Khanam R, Richarts L, Zhu E, Fazekas de St Groth B, et al. Systemic increase in the ratio between Foxp3+ and IL-17-producing CD4+ T cells in healthy pregnancy but not in preeclampsia. J Immunol (2009) 183(11):7023–30. doi:10.4049/jimmunol.0901154
295. Lin F, Zeng P, Xu Z, Ye D, Yu X, Wang N, et al. Treatment of Lipoxin A(4) and its analogue on low-dose endotoxin induced preeclampsia in rat and possible mechanisms. Reprod Toxicol (2012) 34(4):677–85. doi:10.1016/j.reprotox.2012.09.009
296. Cotechini T, Komisarenko M, Sperou A, Macdonald-Goodfellow S, Adams MA, Graham CH. Inflammation in rat pregnancy inhibits spiral artery remodeling leading to fetal growth restriction and features of preeclampsia. J Exp Med (2014) 211(1):165–79. doi:10.1084/jem.20130295
297. Huang Q, Liu L, Hu B, Di X, Brennecke SP, Liu H. Decreased seizure threshold in an eclampsia-like model induced in pregnant rats with lipopolysaccharide and pentylenetetrazol treatments. PLoS One (2014) 9(2):e89333. doi:10.1371/journal.pone.0089333
298. Xue P, Zheng M, Gong P, Lin C, Zhou J, Li Y, et al. Single administration of ultra-low-dose lipopolysaccharide in rat early pregnancy induces TLR4 activation in the placenta contributing to preeclampsia. PLoS One (2015) 10(4):e0124001. doi:10.1371/journal.pone.0124001
299. Garrido-Gomez T, Dominguez F, Quinonero A, Diaz-Gimeno P, Kapidzic M, Gormley M, et al. Defective decidualization during and after severe preeclampsia reveals a possible maternal contribution to the etiology. Proc Natl Acad Sci U S A (2017) 114(40):E8468–77. doi:10.1073/pnas.1706546114
300. Staff AC, Redman CW. IFPA award in placentology lecture: preeclampsia, the decidual battleground and future maternal cardiovascular disease. Placenta (2014) 35(Suppl):S26–31. doi:10.1016/j.placenta.2013.12.003
301. Ruane PT, Berneau SC, Koeck R, Watts J, Kimber SJ, Brison DR, et al. Apposition to endometrial epithelial cells activates mouse blastocysts for implantation. Mol Hum Reprod (2017) 23(9):617–27. doi:10.1093/molehr/gax043
302. Burton GJ, Jauniaux E. The cytotrophoblastic shell and complications of pregnancy. Placenta (2017) 60:134–9. doi:10.1016/j.placenta.2017.06.007
303. Yung HW, Atkinson D, Campion-Smith T, Olovsson M, Charnock-Jones DS, Burton GJ. Differential activation of placental unfolded protein response pathways implies heterogeneity in causation of early- and late-onset pre-eclampsia. J Pathol (2014) 234(2):262–76. doi:10.1002/path.4394
304. Sonek J, Krantz D, Carmichael J, Downing C, Jessup K, Haidar Z, et al. First-trimester screening for early and late preeclampsia using maternal characteristics, biomarkers, and estimated placental volume. Am J Obstet Gynecol (2018) 218(1):e1–13. doi:10.1016/j.ajog.2017.10.024
305. Sibai BM, Caritis SN, Thom E, Klebanoff M, McNellis D, Rocco L, et al. Prevention of preeclampsia with low-dose aspirin in healthy, nulliparous pregnant women. The National Institute of Child Health and Human Development Network of Maternal-Fetal Medicine Units. N Engl J Med (1993) 329(17):1213–8. doi:10.1056/NEJM199310213291701
306. Bujold E, Roberge S, Lacasse Y, Bureau M, Audibert F, Marcoux S, et al. Prevention of preeclampsia and intrauterine growth restriction with aspirin started in early pregnancy: a meta-analysis. Obstet Gynecol (2010) 116(2 Pt 1):402–14. doi:10.1097/AOG.0b013e3181e9322a
307. Roberge S, Giguere Y, Villa P, Nicolaides K, Vainio M, Forest JC, et al. Early administration of low-dose aspirin for the prevention of severe and mild preeclampsia: a systematic review and meta-analysis. Am J Perinatol (2012) 29(7):551–6. doi:10.1055/s-0032-1310527
308. Roberge S, Villa P, Nicolaides K, Giguere Y, Vainio M, Bakthi A, et al. Early administration of low-dose aspirin for the prevention of preterm and term preeclampsia: a systematic review and meta-analysis. Fetal Diagn Ther (2012) 31(3):141–6. doi:10.1159/000336662
309. Rolnik DL, Wright D, Poon LC, O’Gorman N, Syngelaki A, de Paco Matallana C, et al. Aspirin versus placebo in pregnancies at high risk for preterm preeclampsia. N Engl J Med (2017) 377(7):613–22. doi:10.1056/NEJMoa1704559
310. Atallah A, Lecarpentier E, Goffinet F, Doret-Dion M, Gaucherand P, Tsatsaris V. Aspirin for prevention of preeclampsia. Drugs (2017) 77(17):1819–31. doi:10.1007/s40265-017-0823-0
311. Cadavid AP. Aspirin: the mechanism of action revisited in the context of pregnancy complications. Front Immunol (2017) 8:261. doi:10.3389/fimmu.2017.00261
312. Papp C, Szabo G, Toth-Pal E, Papp Z. Fetal growth rate and its variations 1988/89. Orv Hetil (1991) 132(34):1865–70.
313. Wyatt SM, Kraus FT, Roh CR, Elchalal U, Nelson DM, Sadovsky Y. The correlation between sampling site and gene expression in the term human placenta. Placenta (2005) 26(5):372–9. doi:10.1016/j.placenta.2004.07.003
314. Burton GJ, Sebire NJ, Myatt L, Tannetta D, Wang YL, Sadovsky Y, et al. Optimising sample collection for placental research. Placenta (2014) 35(1):9–22. doi:10.1016/j.placenta.2013.11.005
315. Langston C, Kaplan C, Macpherson T, Manci E, Peevy K, Clark B, et al. Practice guideline for examination of the placenta: developed by the Placental Pathology Practice Guideline Development Task Force of the College of American Pathologists. Arch Pathol Lab Med (1997) 121(5):449–76.
316. Hargitai B, Marton T, Cox PM. Best practice no 178. Examination of the human placenta. J Clin Pathol (2004) 57(8):785–92. doi:10.1136/jcp.2003.014217
317. Redline RW, Boyd T, Campbell V, Hyde S, Kaplan C, Khong TY, et al. Maternal vascular underperfusion: nosology and reproducibility of placental reaction patterns. Pediatr Dev Pathol (2004) 7(3):237–49. doi:10.1007/s10024-003-8083-2
318. Tarca AL, Romero R, Draghici S. Analysis of microarray experiments of gene expression profiling. Am J Obstet Gynecol (2006) 195(2):373–88. doi:10.1016/j.ajog.2006.07.001
319. Smyth GK. Limma: linear models for microarray data. In: Gentleman R, Carey V, Dudoit S, Irizarry R, Huber W editors. Bioinformatics and Computational Biology Solutions using R and Bioconductor. New York: Springer (2005). p. 397–420.
320. Bilban M, Haslinger P, Prast J, Klinglmuller F, Woelfel T, Haider S, et al. Identification of novel trophoblast invasion-related genes: heme oxygenase-1 controls motility via peroxisome proliferator-activated receptor gamma. Endocrinology (2009) 150(2):1000–13. doi:10.1210/en.2008-0456
321. Apps R, Sharkey A, Gardner L, Male V, Trotter M, Miller N, et al. Genome-wide expression profile of first trimester villous and extravillous human trophoblast cells. Placenta (2011) 32(1):33–43. doi:10.1016/j.placenta.2010.10.010
322. Tilburgs T, Crespo AC, van der Zwan A, Rybalov B, Raj T, Stranger B, et al. Human HLA-G+ extravillous trophoblasts: Immune-activating cells that interact with decidual leukocytes. Proc Natl Acad Sci U S A (2015) 112(23):7219–24. doi:10.1073/pnas.1507977112
323. Gautier L, Cope L, Bolstad BM, Irizarry RA. affy – analysis of affymetrix GeneChip data at the probe level. Bioinformatics (2004) 20(3):307–15. doi:10.1093/bioinformatics/btg405
324. Ritchie ME, Phipson B, Wu D, Hu Y, Law CW, Shi W, et al. limma powers differential expression analyses for RNA-sequencing and microarray studies. Nucleic Acids Res (2015) 43(7):e47. doi:10.1093/nar/gkv007
325. Carlson S, Falcon S, Pages H, Li N. hgfocus.db: Affymetrix Human Genome Focus Array annotation data (chip hgfocus). R package version 2.2.11. (2005).
326. Carlson S, Falcon S, Pages H, Li N. hgu133a.db: Affymetrix Human Genome U133 Set annotation data (chip hgu133a). R package version 2.2.11. (2005).
327. Carlson S, Falcon S, Pages H, Li N. org.Hs.eg.db: Genome wide annotation for Human. R package version 2.2.11. (2005).
328. Krzywinski M, Schein J, Birol I, Connors J, Gascoyne R, Horsman D, et al. Circos: an information aesthetic for comparative genomics. Genome Res (2009) 19(9):1639–45. doi:10.1101/gr.092759.109
329. Zhang B, Horvath S. A general framework for weighted gene co-expression network analysis. Stat Appl Genet Mol Biol (2005) 4:Article17. doi:10.2202/1544-6115.1128
330. Langfelder P, Horvath S. WGCNA: an R package for weighted correlation network analysis. BMC Bioinformatics (2008) 9:559. doi:10.1186/1471-2105-9-559
331. Ravasz E, Somera AL, Mongru DA, Oltvai ZN, Barabasi AL. Hierarchical organization of modularity in metabolic networks. Science (2002) 297(5586):1551–5. doi:10.1126/science.1073374
332. Langfelder P, Zhang B, Horvath S. Defining clusters from a hierarchical cluster tree: the dynamic tree cut package for R. Bioinformatics (2008) 24(5):719–20. doi:10.1093/bioinformatics/btm563
333. Alexander GR, Himes JH, Kaufman RB, Mor J, Kogan M. A United States national reference for fetal growth. Obstet Gynecol (1996) 87(2):163–8. doi:10.1016/0029-7844(95)00386-X
334. Than NG, Kim SS, Abbas A, Han YM, Hotra J, Tarca AL, et al. Chorioamnionitis and increased galectin-1 expression in PPROM – an anti-inflammatory response in the fetal membranes? Am J Reprod Immunol (2008) 60(4):298–311. doi:10.1111/j.1600-0897.2008.00624.x
335. Mayhew TM. Taking tissue samples from the placenta: an illustration of principles and strategies. Placenta (2008) 29(1):1–14. doi:10.1016/j.placenta.2007.05.010
336. Redline RW, Heller D, Keating S, Kingdom J. Placental diagnostic criteria and clinical correlation – a workshop report. Placenta (2005) 26(Suppl A):S114–7. doi:10.1016/j.placenta.2005.02.009
337. Richani K, Romero R, Kim YM, Cushenberry E, Soto E, Han YM, et al. Tissue microarray: an effective high-throughput method to study the placenta for clinical and research purposes. J Matern Fetal Neonatal Med (2006) 19(8):509–15. doi:10.1080/14767050600852718
338. Brown MA, Lindheimer MD, de Swiet M, van Assche A, Moutquin JM. The classification and diagnosis of the hypertensive disorders of pregnancy: statement from the International Society for the Study of Hypertension in Pregnancy (ISSHP). Hypertens Pregnancy (2001) 20(1):IX–XIV. doi:10.1081/PRG-100104165
339. Santos-Gonzalez M, Gomez DC, Navas P, Villalba JM. Modifications of plasma proteome in long-lived rats fed on a coenzyme Q10-supplemented diet. Exp Gerontol (2007) 42(8):798–806. doi:10.1016/j.exger.2007.04.013
340. Marouga R, David S, Hawkins E. The development of the DIGE system: 2D fluorescence difference gel analysis technology. Anal Bioanal Chem (2005) 382(3):669–78. doi:10.1007/s00216-005-3126-3
341. Reiter L, Rinner O, Picotti P, Huttenhain R, Beck M, Brusniak MY, et al. mProphet: automated data processing and statistical validation for large-scale SRM experiments. Nat Methods (2011) 8(5):430–5. doi:10.1038/nmeth.1584
342. Pattillo RA, Gey GO, Delfs E, Mattingly RF. Human hormone production in vitro. Science (1968) 159(3822):1467–9. doi:10.1126/science.159.3822.1467
343. Kliman HJ, Nestler JE, Sermasi E, Sanger JM, Strauss JF III. Purification, characterization, and in vitro differentiation of cytotrophoblasts from human term placentae. Endocrinology (1986) 118(4):1567–82. doi:10.1210/endo-118-4-1567
344. Mohyeldin A, Garzon-Muvdi T, Quinones-Hinojosa A. Oxygen in stem cell biology: a critical component of the stem cell niche. Cell Stem Cell (2010) 7(2):150–61. doi:10.1016/j.stem.2010.07.007
345. Fu J, Lv X, Lin H, Wu L, Wang R, Zhou Z, et al. Ubiquitin ligase cullin 7 induces epithelial-mesenchymal transition in human choriocarcinoma cells. J Biol Chem (2010) 285(14):10870–9. doi:10.1074/jbc.M109.004200
Keywords: inflammation, ischemia, liquid biopsy, omics, placenta, pregnancy, systems biology, trophoblast invasion
Citation: Than NG, Romero R, Tarca AL, Kekesi KA, Xu Y, Xu Z, Juhasz K, Bhatti G, Leavitt RJ, Gelencser Z, Palhalmi J, Chung TH, Gyorffy BA, Orosz L, Demeter A, Szecsi A, Hunyadi-Gulyas E, Darula Z, Simor A, Eder K, Szabo S, Topping V, El-Azzamy H, LaJeunesse C, Balogh A, Szalai G, Land S, Torok O, Dong Z, Kovalszky I, Falus A, Meiri H, Draghici S, Hassan SS, Chaiworapongsa T, Krispin M, Knöfler M, Erez O, Burton GJ, Kim CJ, Juhasz G and Papp Z (2018) Integrated Systems Biology Approach Identifies Novel Maternal and Placental Pathways of Preeclampsia. Front. Immunol. 9:1661. doi: 10.3389/fimmu.2018.01661
Received: 28 February 2018; Accepted: 04 July 2018;
Published: 08 August 2018
Edited by:
Herman Waldmann, University of Oxford, United KingdomReviewed by:
Phillip E. Melton, Curtin University, AustraliaAngelo A. Manfredi, Università Vita-Salute San Raffaele, Italy
Copyright: © 2018 Than, Romero, Tarca, Kekesi, Xu, Xu, Juhasz, Bhatti, Leavitt, Gelencser, Palhalmi, Chung, Gyorffy, Orosz, Demeter, Szecsi, Hunyadi-Gulyas, Darula, Simor, Eder, Szabo, Topping, El-Azzamy, LaJeunesse, Balogh, Szalai, Land, Torok, Dong, Kovalszky, Falus, Meiri, Draghici, Hassan, Chaiworapongsa, Krispin, Knöfler, Erez, Burton, Kim, Juhasz and Papp. This is an open-access article distributed under the terms of the Creative Commons Attribution License (CC BY). The use, distribution or reproduction in other forums is permitted, provided the original author(s) and the copyright owner(s) are credited and that the original publication in this journal is cited, in accordance with accepted academic practice. No use, distribution or reproduction is permitted which does not comply with these terms.
*Correspondence: Nandor Gabor Than, dGhhbi5nYWJvciYjeDAwMDQwO3R0ay5tdGEuaHU=;
Roberto Romero, cHJiY2hpZWZzdGFmZiYjeDAwMDQwO21lZC53YXluZS5lZHU=;
Zoltan Papp, cHpvcnZvc2loZXRpbGFwJiN4MDAwNDA7bWF0ZXJuaXR5Lmh1