- 1Centre for Immunology and Infection, Department of Biology, Hull York Medical School, York, UK
- 2Department of Electronics, University of York, York, UK
- 3York Computational Immunology Laboratory, University of York, York, UK
The molecular and cellular processes driving the formation of secondary lymphoid tissues have been extensively studied using a combination of mouse knockouts, lineage-specific reporter mice, gene expression analysis, immunohistochemistry, and flow cytometry. However, the mechanisms driving the formation and function of tertiary lymphoid tissue (TLT) experimental techniques have proven to be more enigmatic and controversial due to differences between experimental models and human disease pathology. Systems-based approaches including data-driven biological network analysis (gene interaction network, metabolic pathway network, cell–cell signaling, and cascade networks) and mechanistic modeling afford a novel perspective from which to understand TLT formation and identify mechanisms that may lead to the resolution of tissue pathology. In this perspective, we make the case for applying model-driven experimentation using two case studies, which combined simulations with experiments to identify mechanisms driving lymphoid tissue formation and function, and then discuss potential applications of this experimental paradigm to identify novel therapeutic targets for TLT pathology.
Formation and Function of Secondary and Tertiary Immune Microenvironments
Lymphoid tissues are responsible for the orchestration of functional immune responses. This is achieved through the development and maintenance of niches that support the retention, activation, and proliferation of adaptive immune cells in response to antigenic stimulation. Adult lymphoid tissue architecture is organized by an underlying network of stromal cells that produce extracellular matrix (e.g., collagens) and provide survival (e.g., BAFF, IL-7), migratory (CCL19/21, CXCL13), and immune activation [the storage and presentation of immune complexes by follicular dendritic cell (FDC)] signals (1). Distinct stromal subsets with unique secretion profiles (chemokines, other cytokines, and survival factors) develop in response to signaling from lymphocytes with a key role for TNF superfamily receptors; this stromal–lymphocyte cross talk ensures the correct cell type is stimulated (or regulated) at the right time and place. Sustained cross talk between mesenchymal stroma and lymphocyte subsets is a core feature of lymphoid tissue formation and maintenance and occurs irrespective of the tissue type or anatomical location.
Formation of lymphoid tissues can occur by different cellular and molecular mechanisms. During fetal development, secondary lymphoid tissues form in a process dependent on the RAR-related orphan receptor gamma transcription factor expressing lymphoid tissue inducer cells (LTi) responding to localized chemotactic gradients leading to the formation of lymph nodes (LN) and Peyer’s patches (PPs) in a lymphotoxin β (LTβ)-dependent process (2). Localized mesenchyme, lymphoid tissue organizer (LTo) cells differentiate into adult marginal reticular cells, fibroblastic reticular cells, and FDCs (3). Likewise, in the adult, innate lymphoid cells type 3, the adult equivalent of LTi cells, have a key role in regulating cryptopatches that can mature into isolated lymphoid follicles (4). These specialized lymphoid structures contain predominantly B cells and often contain germinal center (GC) reactions.
In humans, tertiary lymphoid tissues (TLTs) are found in inflammatory immune responses associated with chronic pathology from hip joint replacements, keloids, tissues in autoimmune disease (e.g., the salivary gland in Sjogren’s syndrome, multiple sclerosis, and rheumatoid arthritis) to solid tumors and follicular lymphomas in the bone marrow (5–9). Although the role of specific cell types has been controversial, there is an emerging paradigm of a multistep process where localized inflammation induces stromal cell activation in a lymphocyte independent process, leading to localized microenvironments permissive for T and B cells entry (10). These lymphocytes have the potential to drive the formation of organized tertiary tissue in an autocrine-dependent process. This process closely resembles the capacity of naive B cells to drive B cell follicle formation in secondary lymphoid tissues in a TNFα- and LTβ-dependent process and the capacity of activated B cells to generate the GC, a transient microenvironment that drives high-affinity immune responses in a self-regulating autocrine-dependent process. In both secondary immune tissues (LN, PPs, and spleen) and TLTs including ILFs and TLT, activated B cells prime the formation of the GC reaction. This specialized microenvironment contains both activated and proliferating B cells and different stromal compartments of CXCL12-secreting stroma (dark zone) and CXCL13-secreting FDCs (light zone). This facilitates the cyclic selection and expansion of antigen-specific B cells (11).
Non-lymphoid inflammatory immune structures, granulomas, can form in the liver, intestine, adipose tissue (crown-like structures), and lung induced by chronic infection/inflammation associated with tuberculosis, sarcoidosis leishmaniasis, schistosomiasis, cell death, and Crohn’s disease (12–14). The formation of these highly dynamic microenvironments superficially resembles TLT; however, their formation and organization is driven by activated macrophages rather than by the mesenchymal–lymphocyte cross talk observed in lymphoid tissues thus do not exhibit lymphocyte compartmentalization. Granuloma structures are very heterogeneous in presentation within individual patients in a continuum between early macrophage centric granulomas, self-resolving granulomas, and fibroblastic structures, these often being fibrotic rather than taking on a supportive stromal network phenotype. The triggers that drive granuloma formation instead of TLT formation appear not to be due to differences in the different chemotactic cues delivered by activated macrophages compared to those delivered by activated stromal fibroblasts, leading to a very different cellular make up to the inflammatory foci of leukocytes [primarily myelomonocytic (granuloma) vs. lymphocytic (TLT)].
Current Approaches to Studying Lymphoid Tissue Formation: Limits, Challenges, and New Approaches
Experimental studies, principally performed in gene knockout, lineage-specific fluorescent protein, and Cre reporter mouse lines have contributed significant insights into the roles of multiple different cell types and molecules in lymphoid tissue formation and function. This has been further validated using histology and flow cytometry analysis on human secondary lymphoid tissues. However, in contrast to secondary lymphoid tissues, there are some distinct differences in human tissue pathologies to those found in mice including the cellular composition of TLTs, granulomas, and other inflammatory tissues. This arises in part from genetic and physiological differences between human and mice including the timing and duration of the immune response (chronic vs. acute inflammation), the inflammatory triggers (infection, autoimmunity, and cancer), and transcriptional differences in immune cells in the different species. In general, mouse models of immune-mediated inflammatory disease are acute and fail to replicate the chronic human disease characterized by disease flairs followed by remission, limiting their translational capacity to human disease. Infection and tumor models in mice either rapidly resolve (too quickly for chronic pathology to establish) or lead to the mouse having to by euthanized for health and welfare prior to tertiary lymphoid pathology occurring. In comparison, humans may live the rest of their life with the disease pathology, particularly in the context of treatment with biologics and small molecules; thus, pathology has the opportunity to evolve from localized inflammation to fibrotic tissue failure, systemic inflammation, and autoimmunity working together to prevent disease resolution. Increasingly, human 3-dimensional tissue culture models containing both stroma and lymphocytes have become increasingly common and useful in understanding underlying molecule mechanisms of TLT formation. However, it is not currently possible to represent the full complexity of chronic human pathology in vitro.
Experimental systems (in vivo and in vitro) to date have proven limited in their ability to explain chronic clinical pathology and resolve established Sjogren’s pathology, although TNF has an important role in FDC differentiation and B cell organization, anti-TNF fails to induce resolution disease (15). To better understand the form and function of TLTs, current knowledge of stromal regulation through molecular signals and immune cell behavior within lymphoid tissue must be consolidated and considered in a quantitative, systems-based approach. The development of systems-level stochastic computational models can bring together a broad understanding across spatiotemporal scales of how genetic and molecular factors relate to cellular and tissue level form and function and give rise to the complex, functional architectures observed in secondary lymphoid organs and disease-specific TLTs. These models permit in silico experimentation providing a unique platform driving further experimentation and assessing novel mechanistic targets and intervention strategies where in vivo observed heterogeneity can be replicated.
Alan Turing (of code breaking fame) in seminal early work in mathematical biology (16) noted that gastrulation arose from symmetry breaking, and this leads to fundamental insights and principles that drive modern mathematical and computational biology: the notion that chaotic, non-linear behavior of individual biological processes, including the self-organization of complex biological structures (e.g., TLT), can result in emergent properties that cannot be understood from consideration of each individual component in isolation. The development of models that capture the essential, emergent behavior of specific biological processes, with extraneous components excluded, enables understanding of how complex molecular and cellular interactions govern complex, emergent biological processes and can therefore lead to new insights and quantitative predictions (17). Emergent properties in a TLT model would include stromal networks, lymphocyte organization, migration and interactions with antigen-presenting cells, and localized cytokine/chemokine production.
Application of Model-Driven Experimentation (MDE) to Understand Mechanisms of Lymphoid Tissue Development and Function
Advances in computing resources and computational modeling technology have provided the capacity to generate complex in silico models of lymphoid tissues that incorporate space, time, and cellular heterogeneity found in immune tissues including TLT. Applying in silico approaches to understand secondary lymphoid tissue formation and function requires the integration of experimental data across cellular, molecular, and tissue levels of organization. Ensuring that the biological processes are appropriately described requires a fine balance between model abstraction and interpretation (quantitative and qualitative) of experimental data. A number of different modeling approaches may be utilized (summarized in Table 1), increasingly, integration of different mathematical/computational techniques into a hybrid model is a common strategy to address the limitations of using each technique in isolation. This approach also facilitates the consolidation of data across different levels of organization (molecular, cellular, tissue, and patient) into a single multi-scale model. For example, an agent-based model can capture an individual cell, which in turn incorporates a differential equation-based model capturing a “lower-level” aspect of that individual’s behavior, such as surface expression of a receptor (42). Adopting an in silico approach provides a platform that can provide insights and generate predictions that can be verified in vivo: verification that can lead to increased biological understanding and incrementally improved in silico models for further experimentation. This iterative approach of combining in vivo, in vitro, and in silico approaches has been termed “model-driven experimentation” (18).
Case Study 1: Insights from MDE to Secondary Lymphoid Tissue Formation
Peyer’s patches are specialized secondary lymphoid tissues of the intestine that develop during a fixed window in fetal development and have an essential role in maintaining intestinal immunity. PPs form stochastically along the midgut, with mice developing 8–12 patches; however, as the absence of or reduction in the number of PPs is observed in several different gene knockouts, the molecular process that triggers patch formation was unclear (19). Using an MDE-based approach had the potential to provide new insight into how different signaling pathways (RET, chemokine receptors, cytokine receptors, TNF superfamily, and adhesion molecules) might integrate to induce PP development in silico and to subsequently design key experiments to test hypotheses in vivo. PPSim is an agent-based PP simulator that captures key processes during the 72-h period of tissue development in prenatal mice and replicates (statistically similar) emergent cell behaviors found in vivo, specifically populations of hematopoietic cells, known as lymphoid tissue initiator (LTin) and lymphoid tissue inducer (LTi) cells, migrates into the developing gut, with data from laboratory observations suggesting these cells follow a random motion. Both cell populations express receptors for the adhesion molecule VCAM-1, expressed by stromal LTo cells residing in the gut wall (20, 21). In this computational model, LTi and LTin are captured as individual entities that migrate into the developing midgut serosa and undergo a random walk, interacting with their localized simulated environment through signaling pathways including GDRFs/Ret signaling pathways, adhesion molecules, and chemokine receptors, as is observed in vivo. On ensuring PPsim adequately represented individual cell responses, statistical analysis techniques, specifically sensitivity analyses, were used to explore mechanisms driving prenatal lymphoid organ formation (22, 23). This exploration of the simulated biological pathways revealed which pathways had significant impacts on simulated cell behavior at different time points during PP development. By examining correlations in the level of activity of simulated pathways and cell behavior, the hypothesis was derived that contact between LTin and LTo cells that leads to the localized upregulation of VCAM-1 on stromal cells was the key triggering event that determined the site of PP formation on the midgut (21). Utilizing this prediction, an in vitro assay imaging fetal midgut explants incubated in the presence or absence of anti-VCAM-1 antibodies was developed. Using this assay, it was verified that early upregulation of VCAM-1 was the triggering event that was essential for the initiation of LTi and LTin cell clustering. The model simulation results, supported by replicated experimentation and safety-critical systems-based fitness-for-purpose argumentation that details the knowledge integration in model composition, provide evidence that the simulation was fit for the purpose of aiding exploration of this specific research question: understanding the triggering of lymphoid tissue development, which was not possible by conventional genetic approaches (24, 25).
Case Study 2: Applying MDE to Understand GC Dynamics and Function
The GC reaction is a transient microenvironment in which affinity maturation occurs in response to immunization and infection, bearing key similarities to TLT in its evolution in the role of lymphocytes in inducing highly organized stromal networks, the essential role of TNF superfamily members in regulating its induction and the induction of chemokine gradients (10, 26). However, in comparison to TLT, the GC is a self-resolving tertiary lymphoid microenvironment. Recent technological advances, particularly the advent of intravital multiphoton imaging including photo-activated fluorescent proteins has led to the unprecedented availability of data on the dynamics B-cell migration and selection (27–30). However, imaging datasets provide a narrow window of insight into a process that occurs over a timescale of days and weeks. Furthermore, as imaging techniques are optimized for a given time and length scale, they are limited in their ability to link molecular, cellular, and tissue level processes. This has made the interpretation of imaging datasets in the context of the wider literature challenging. To address this issue, modeling approaches have been used to test the validity of different hypotheses of mechanisms controlling B-cell migration and selection within the GC (31–34).
In the GC reaction, model-derived insights have proved useful not only in the analysis of existing datasets but also as a driver for further experimentation. Specifically, an MDE approach has been used to examine the effects of antibody feedback on the process of affinity maturation (35). Analysis of an in silico GC reaction yielded the prediction that GC B-cells, which require antigen on FDCs for positive selection, were competing for antigen by early low-affinity antibodies. Only higher affinity B-cells were able to outcompete for antigen to receive the necessary survival signals. To experimentally validate this prediction, the authors manipulated the GC response with monoclonal antibodies of defined affinities and were able to confirm that antibody feedback provides a dynamic selection threshold to maximize Ig affinities (35). A similar approach was employed to investigate the role of toll-like receptor 4 (TLR4) on the GC where an iterative cycle of in silico and in vivo experimentation dissected the importance of TLR4 signaling on the maturation of FDCs, key regulators of B-cell selection in the light zone of the GC (36). Both of these MDE examples highlight the use of in silico experimentation as a means of refining experimental design through the identification of key time points and conditions to test in vivo. These case studies together provide example of how theoretical models can consolidate data from different sources as a platform for the development novel hypotheses and a driver for further experimentation.
Perspective on MDE as Applied to TLT Formation, Function, and Therapeutic Resolution
When computational modeling is combined with knowledge derived from imaging, multi-dimensional cytometry, and gene expression analysis of human TLT pathology, MDE has the potential to provide novel insights to key questions on molecular and cellular mechanisms involved in TLT formation, maintenance, and function similar to its capacity to impact on our understanding of lymphoid stromal network and granuloma dynamics (Table 2) (37–40). One of the key advantages of applying multi-scale modeling is it permits capture of a wide range of different phenomena that occur on different orders of magnitude in terms of time and length scales that are critical in the stochastic processes involved in TLT induction. These include different cell types, states and interactions, inflammatory molecules, extracellular matrix, adhesion molecules, and chemotactic signals all in the context of an evolving tissue microenvironment. Developing in silico models permits temporal inhibition of different signaling pathways and cellular depletions during different stages of TLT pathology using statistical tools (Figure 1). This permits identification of key pathways that could be targeted to induce resolution of pre-existing TLT rather than inhibiting its formation as has been used to make in silico predictions for the treatment of tuberculosis (41). A large number of novel antibody therapies, biologics, and small molecular inhibitors have been developed to target immune function for the treatment of immune-mediated inflammatory diseases. These therapies are unlikely to show maximal efficacy against existing tissue pathology when used as monotherapies, rather it is more likely that use of therapeutic combinations that is most likely to show clinical efficacy. The clinical challenge is that there are already over 20,000 possible different combinations using existing therapeutics that would need to be trialed to find optimal targeting strategy to resolve TLT pathology. Thus, MDE-based approaches provide a rational approach to identify novel combination therapeutic regimes that have a best potential in clinical trials (42).
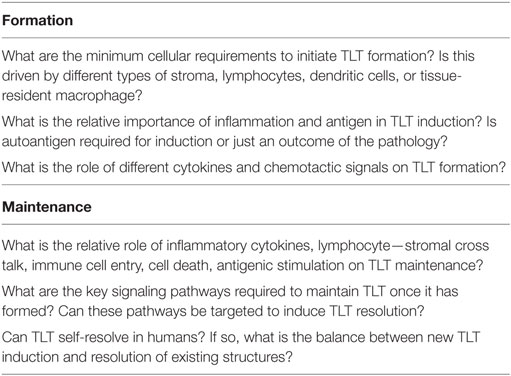
Table 2. Key questions on tertiary lymphoid tissue (TLT) formation and maintenance that can be address in hybridized TLT models.
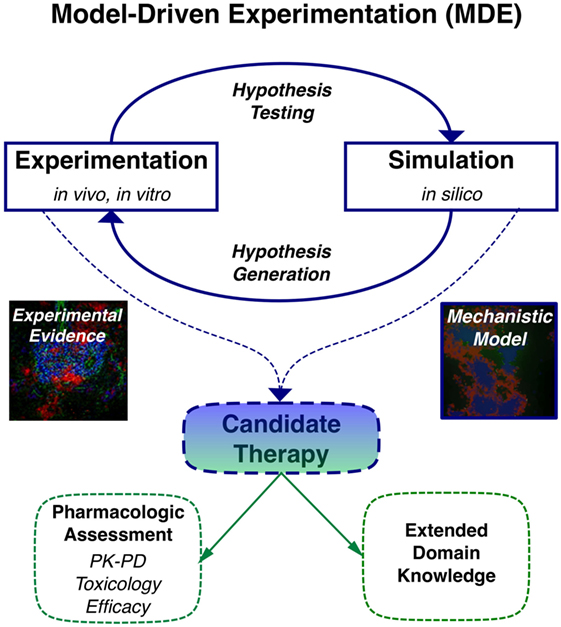
Figure 1. Application of model-driven experimentation to develop new mechanistic understanding of tertiary lymphoid tissue (TLT) formation and maintenance permitting identification of novel therapeutic approaches to resolve localized TLT pathology.
Although the adoption of MDE has only recently started to impact on immunology research, it is starting to have a very significant impact on other areas of biology. We propose that the increased accessibility of computational models, the high-performance computing resources, the increased familiarity and understanding of simulations as tools to understand immune function, and the capacity to apply in silico approaches to identify potential therapeutic approaches and disease biomarkers will accelerate the application of MDE as a methodology understand and target disease resolution.
Author Contributions
MC wrote this paper and is the corresponding author. JB, JC, KA and JT contributed to the writing of this perspective.
Conflict of Interest Statement
The authors declare that the research was conducted in the absence of any commercial or financial relationships that could be construed as a potential conflict of interest.
Funding
JC is supported by Wellcome Trust (Computational Approaches in Translational Science) WT095024MA. Research in MC providing a basis for this perspective is supported by MRC MR/K021125/1.
References
1. Junt T, Scandella E, Ludewig B. Form follows function: lymphoid tissue microarchitecture in antimicrobial immune defence. Nat Rev Immunol (2008) 8:764–75. doi: 10.1038/nri2414
2. van de Pavert SA, Mebius R. New insights into the development of lymphoid tissues. Nat Rev Immunol (2010) 10:664–74. doi:10.1038/nri2832
3. Jarjour M, Jorquera A, Mondor I, Wienert S, Narang P, Coles M, et al. Fate mapping reveals origin and dynamics of lymph node follicular dendritic cells. J Exp Med (2014) 211(6):1109–22. doi:10.1084/jem.20132409
4. Mowat A, Agace W. Regional specialization within the intestinal immune system. Nat Rev Immunol (2014) 14:667–85. doi:10.1038/nri3738
5. Mittal S, Revell M, Barone F, Hardie DL, Matharu GS, Davenport AJ, et al. Lymphoid aggregates that resemble tertiary lymphoid organs define a specific pathological subset in metal-on-metal hip replacements. PLoS One (2013) 8(5):e63470. doi:10.1371/journal.pone.0063470
6. Bombardieri M, Barone F, Lucchesi D, Nayar S, van den Berg WB, Proctor G, et al. Inducible tertiary lymphoid structures, autoimmunity, and exocrine dysfunction in a novel model of salivary gland inflammation in C57BL/6 mice. J Immunol (2012) 189(7):3767–76. doi:10.4049/jimmunol.1201216
7. Bagabir R, Byers RJ, Chaudhry IH, Müller W, Paus R, Bayat A. Site-specific immunophenotyping of keloid disease demonstrates immune upregulation and the presence of lymphoid aggregates. Br J Dermatol (2012) 167(5):1053–66. doi:10.1111/j.1365-2133.2012.11190.x
8. Dieu-Nosjean MC, Giraldo NA, Kaplon H, Germain C, Fridman WH, Sautès-Fridman C. Tertiary lymphoid structures, drivers of the anti-tumor responses in human cancers. Immunol Rev (2016) 271(1):260–75. doi:10.1111/imr.12405
9. Guilloton F, Caron G, Ménard C, Pangault C, Amé-Thomas P, Dulong J, et al. Mesenchymal stromal cells orchestrate follicular lymphoma cell niche through the CCL2-dependent recruitment and polarization of monocytes. Blood (2012) 119(11):2556–67. doi:10.1182/blood-2011-08-370908
10. De Silva NS, Klein U. Dynamics of B cells in germinal centres. Nat Rev Immunol (2015) 15:137–48. doi:10.1038/nri3804
11. Zhang Y, Garcia-Ibanez L, Toellner KM. Regulation of germinal center B-cell differentiation. Immunol Rev (2016) 270(1):8–19. doi:10.1111/imr.12396
12. Sandor M, Weinstock JV, Wynn TA. Granulomas in schistosome and mycobacterial infections: a model of local immune responses. Trends Immunol (2003) 24(1):44–52. doi:10.1016/S1471-4906(02)00006-6
13. Kaye PM, Beattie L. Lessons from other diseases: granulomatous inflammation in leishmaniasis. Semin Immunopathol (2016) 38(2):249–60. doi:10.1007/s00281-015-0548-7
14. Bolus WR, Gutierrez DA, Kennedy AJ, Anderson-Baucum EK, Hasty AH. CCR2 deficiency leads to increased eosinophils, alternative macrophage activation, and type 2 cytokine expression in adipose tissue. J Leukoc Biol (2015) 98(4):467–77. doi:10.1189/jlb.3HI0115-018R
15. Sankar V, Brennan MT, Kok MR, Leakan RA, Smith JA, Manny J, et al. Etanercept in Sjogren’s syndrome: a twelve-week randomized, doubleblind, placebo-controlled pilot clinical trial. Arthritis Rheum (2004) 50:2240–5. doi:10.1002/art.20299
16. Turing AM. The chemical basis of morphogenesis. Philos Trans R Soc Lond B Biol Sci (1952) 237(641):37–72. doi:10.1098/rstb.1952.0012
17. Callard RE, Yates AJ. Immunology and mathematics: crossing the divide. Immunology (2005) 115(1):21–33. doi:10.1111/j.1365-2567.2005.02142.x
18. Ganesan A, Levchenko A. Principles of model building: an experimentation-aided approach to development of models for signaling networks. Methods Cell Biol (2012) 110:1–17. doi:10.1016/B978-0-12-388403-9.00001-1
19. Veiga-Fernandes H, Coles MC, Foster KE, Patel A, Williams A, Natarajan D, et al. Tyrosine kinase receptor RET is a key regulator in Peyer’s patch organogenesis. Nature (2007) 446(7135):547–51. doi:10.1038/nature05597
20. Alden K, Timmis J, Andrews PS, Veiga-Fernandes H, Coles M. Pairing experimentation and computational modelling to understand the role of tissue inducer cells in the development of lymphoid organs. Front Immunol (2012) 3:172. doi:10.3389/fimmu.2012.00172
21. Patel N, Harker L, Moreira-Santos M, Ferreira K, Alden J, Timmis K, et al. Differential RET signaling pathways drive development of the enteric lymphoid and nervous systems. Sci Signal (2012) 5:235. doi:10.1126/scisignal.2002734
22. Alden K, Read M, Timmis J, Andrews P, Veiga-Fernades H, Coles M. Spartan: a comprehensive tool for understanding uncertainty in simulations of biological systems. PLoS Comput Biol (2013) 9(2):e1002916. doi:10.1371/journal.pcbi.1002916
23. Butler JA, Alden K, Veiga Fernandex H, Timmis J, Coles M. Novel approaches to the visualization and quantification of biological simulations by emulating experimental techniques. ALIFE 14: Proceedings of the Fourteenth International Conference on the Synthesis and Simulation of Living Systems (Vol. 14), New York: MIT Press (2014). p. 614–21.
24. Alden K, Andrews PS, Veiga-Fernandes H, Timmis J, Coles M. Utilising a simulation platform to understand the effect of domain model assumptions. Nat Comput (2015) 14(1):99–107. doi:10.1007/s11047-014-9428-7
25. Alden K, Andrews PS, Polack FA, Veiga-Fernandes H, Coles MC, Timmis J. Using argument notation to engineer biological simulations with increased confidence. J R Soc Interface (2015) 12(104):20141059. doi:10.1098/rsif.2014.1059
26. Victora GD, Mesin L. Clonal and cellular dynamics in germinal centers. Curr Opin Immunol (2014) 28:90–6. doi:10.1016/j.coi.2014.02.010
27. Allen CDC, Okada T, Tang HL, Cyster JG. Imaging of germinal center selection events during affinity maturation. Science (2007) 315:528–31. doi:10.1126/science.1136736
28. Schwickert TA, Lindquist RL, Shakhar G, Livshits G, Skokos D, Kosco-Vilbois MH, et al. In vivo imaging of germinal centres reveals a dynamic open structure. Nature (2007) 446:83–7. doi:10.1038/nature05573
29. Shulman Z, Gitlin AD, Targ S, Jankovic M, Pasqual G, Nussenzweig MC, et al. T follicular helper cell dynamics in germinal centers. Science (2013) 341:673–7. doi:10.1126/science.1241680
30. Shulman Z, Gitlin AD, Weinstein JS, Lainez B, Esplugues E, Flavell RA, et al. Dynamic signaling by T follicular helper cells during germinal center B cell selection. Science (2014) 345:1058–62. doi:10.1126/science.1257861
31. Chan C, Billard M, Ramirez SA, Schmidl H, Monson E, Kepler TB. A model for migratory B cell oscillations from receptor down-regulation induced by external chemokine fields. Bull Math Biol (2013) 75:185–205. doi:10.1007/s11538-012-9799-9
32. Figge MT, Garin A, Gunzer M, Kosco-Vilbois M, Toellner K-M, Meyer-Hermann M. Deriving a germinal center lymphocyte migration model from two-photon data. J Exp Med (2008) 205:3019–29. doi:10.1084/jem.20081160
33. Meyer-Hermann ME, Maini PK, Iber D. An analysis of B cell selection mechanisms in germinal centers. Math Med Biol (2006) 23:255–77. doi:10.1093/imammb/dql012
34. Meyer-Hermann M, Mohr E, Pelletier N, Zhang Y, Victora GD, Toellner K-M. A theory of germinal center B cell selection, division, and exit. Cell Rep (2012) 2:162–74. doi:10.1016/j.celrep.2012.05.010
35. Zhang Y, Meyer-Hermann M, George LA, Figge MT, Khan M, Goodall M, et al. Germinal center B cells govern their own fate via antibody feedback. J Exp Med (2013) 210:457–64. doi:10.1084/jem.20120150
36. Garin A, Meyer-Hermann M, Contie M, Figge MT, Buatois V, Gunzer M, et al. Toll-like receptor 4 signaling by follicular dendritic cells is pivotal for germinal center onset and affinity maturation. Immunity (2010) 33:84–95. doi:10.1016/j.immuni.2010.07.005
37. Kislitsyn A, Savinkov R, Novkovic M, Onder L, Bocharov G. Computational approach to 3D modeling of the lymph node geometry. Computation (2015) 3(2):222–34. doi:10.3390/computation3020222
38. Novkovic M, Onder L, Cupovic J, Abe J, Bomze D, Cremasco V, et al. Topological small-world organization of the fibroblastic reticular cell network determines lymph node functionality. PLoS Biol (2016) 14(7):e1002515. doi:10.1371/journal.pbio.1002515
39. Warsinske HC, Wheaton AK, Kim KK, Linderman JJ, Moore BB, Kirschner DE. Computational modeling predicts simultaneous targeting of fibroblasts and epithelial cells is necessary for treatment of pulmonary fibrosis. Front Pharmacol (2016) 23(7):183. doi:10.3389/fphar.2016.00183
40. Marino S, Gideon HP, Gong C, Mankad S, McCrone JT, Lin PL, et al. Computational and empirical studies predict Mycobacterium tuberculosis-specific T cells as a biomarker for infection outcome. PLoS Comput Biol (2016) 12(4):e1004804. doi:10.1371/journal.pcbi.1004804
41. Pienaar E, Dartois V, Linderman JJ, Kirschner DE. In silico evaluation and exploration of antibiotic tuberculosis treatment regimens. BMC Syst Biol (2015) 9:79. doi:10.1186/s12918-015-0221-8
Keywords: multi-scale modeling, tertiary lymphoid tissue, systems immunology, mechanistic modelling, model-driven experimentation
Citation: Butler JA, Cosgrove J, Alden K, Timmis J and Coles MC (2017) Model-Driven Experimentation: A New Approach to Understand Mechanisms of Tertiary Lymphoid Tissue Formation, Function, and Therapeutic Resolution. Front. Immunol. 7:658. doi: 10.3389/fimmu.2016.00658
Received: 26 August 2016; Accepted: 16 December 2016;
Published: 04 April 2017
Edited by:
Andreas Habenicht, Ludwig Maximilian University of Munich, GermanyCopyright: © 2017 Butler, Cosgrove, Alden, Timmis and Coles. This is an open-access article distributed under the terms of the Creative Commons Attribution License (CC BY). The use, distribution or reproduction in other forums is permitted, provided the original author(s) or licensor are credited and that the original publication in this journal is cited, in accordance with accepted academic practice. No use, distribution or reproduction is permitted which does not comply with these terms.
*Correspondence: Mark Christopher Coles, mark.coles@york.ac.uk
†Co-first authors.