- 1Tytgat Institute for Liver and Intestinal Research, Academic Medical Center, Amsterdam, Netherlands
- 2Immunowell Foundation, Utrecht, Netherlands
- 3Bioinformatics Laboratory, Clinical Epidemiology, Biostatistics and Bioinformatics (KEBB), Academic Medical Center, Amsterdam, Netherlands
- 4Biosystems Data Analysis, Swammerdam Institute for Life Sciences (SILS), University of Amsterdam, Amsterdam, Netherlands
- 5Division of Pharmacology, Utrecht Institute for Pharmaceutical Sciences, Faculty of Science, Utrecht University, Utrecht, Netherlands
- 6Institute for Risk Assessment Sciences, Faculty of Veterinary Medicine, Utrecht University, Utrecht, Netherlands
- 7Department of Immunobiology, Biomedical Primate Research Centre, Rijswijk, Netherlands
- 8Department of Immunology, Erasmus University Medical Center, Rotterdam, Netherlands
- 9Department of Neuroscience, University Medical Center, University of Groningen, Groningen, Netherlands
- 10Institute of Psychology, Health, Medical, and Neuropsychology Unit, Faculty of Social and Behavioural Sciences, Leiden University, Leiden, Netherlands
- 11Laboratory of Translational Immunology, University Medical Center Utrecht, Utrecht, Netherlands
- 12Division of Pediatrics, Pediatric Immunology and Infectious Diseases, Wilhelmina Children’s Hospital, University Medical Center Utrecht, Utrecht, Netherlands
- 13Laboratory of Microbiology, Wageningen University, Wageningen, Netherlands
- 14Department of Psychology and Educational Sciences, Open University, Heerlen, Netherlands
- 15Institute for Life Sciences and Chemistry, HU University of Applied Sciences Utrecht, Utrecht, Netherlands
- 16Immunology Platform, Nutricia Research, Utrecht, Netherlands
- 17Institute for Positive Health, Amersfoort, Netherlands
- 18Department of Rheumatology and Clinical Immunology, University Medical Center Groningen, University of Groningen, Groningen, Netherlands
- 19Laboratory of Translational Immunology, Department of Rheumatology and Clinical Immunology, University Medical Center Utrecht, Utrecht, Netherlands
- 20Laboratory of Translational Immunology, Division of Pediatrics, Wilhelmina Children’s Hospital, University Medical Center Utrecht, Utrecht, Netherlands
- 21Laboratory of Medical Immunology, Department of Laboratory Medicine, Radboud University Medical Centre, Nijmegen, Netherlands
In order to combat chronic immune disorders (CIDs), it is an absolute necessity to understand the bigger picture, one that goes beyond insights at a one-disease, molecular, cellular, and static level. To unravel this bigger picture we advocate an integral, cross-disciplinary approach capable of embracing the complexity of the field. This paper discusses the current knowledge on common pathways in CIDs including general psychosocial and lifestyle factors associated with immune functioning. We demonstrate the lack of more in-depth psychosocial and lifestyle factors in current research cohorts and most importantly the need for an all-encompassing analysis of these factors. The second part of the paper discusses the challenges of understanding immune system dynamics and effectively integrating all key perspectives on immune functioning, including the patient’s perspective itself. This paper suggests the use of techniques from complex systems science in describing and simulating healthy or deviating behavior of the immune system in its biopsychosocial surroundings. The patient’s perspective data are suggested to be generated by using specific narrative techniques. We conclude that to gain more insight into the behavior of the whole system and to acquire new ways of combatting CIDs, we need to construct and apply new techniques in the field of computational and complexity science, to an even wider variety of dynamic data than used in today’s systems medicine.
Introduction
Chronic immune disorders (CIDs), comprising chronic immune-mediated inflammatory conditions, such as autoimmune diseases, allergies, immune deficiencies, and some psychiatric disorders (such as depression) are a large and growing health problem. Approximately 1 in 10 individuals living in Europe and North America are affected, and consequently, CIDs represent a significant cause of chronic morbidity and disability (1, 2), strongly impacting the quality of life.
This translates into a substantial (socio)economic challenge to rapidly improve prediction, prevention, diagnosis, and treatment of these diseases in order to significantly reduce health-care costs1 (3–5).
More than 50 years of immunological research has brought us detailed insights into immune pathways at both a molecular and cellular level but still leaves fundamental questions unanswered: which (common) factors contribute to the onset of CIDs and which mechanisms keep the immune system in homeostasis or can drive the system into disease states?
In order to combat CIDs, we need to understand the bigger picture that goes beyond insights at a one-disease, molecular, cellular, and static level. To unravel this bigger picture we should use an integral, cross-disciplinary approach (6, 7). Although the idea of such an approach is not entirely new, most challenges to transform it into truly integral scientific pan-disease projects are still to be met. It is crucial to embrace the complexity of the CID field and boldly start applying new techniques from the field of complex systems science to effectively combine different scientific perspectives, including the human (patient) perspective.
The Bigger Picture
In order to gain more insight into the driving mechanisms underlying CIDs we need to broaden our current reductionistic focus on molecular, cellular, and organ level of a single CID to a holistic strategy that considers multiple CIDs and incorporates the microbiome, psychological, social, and lifestyle determinants. Although several projects and consortia exist worldwide that incorporate some of the aforementioned immune parameters and general psychosocial aspects, a number of key factors are lacking. An all-encompassing analysis is needed. Here, we will provide a more detailed insight into factors that in our opinion could contribute to this bigger picture (Figure 1A).
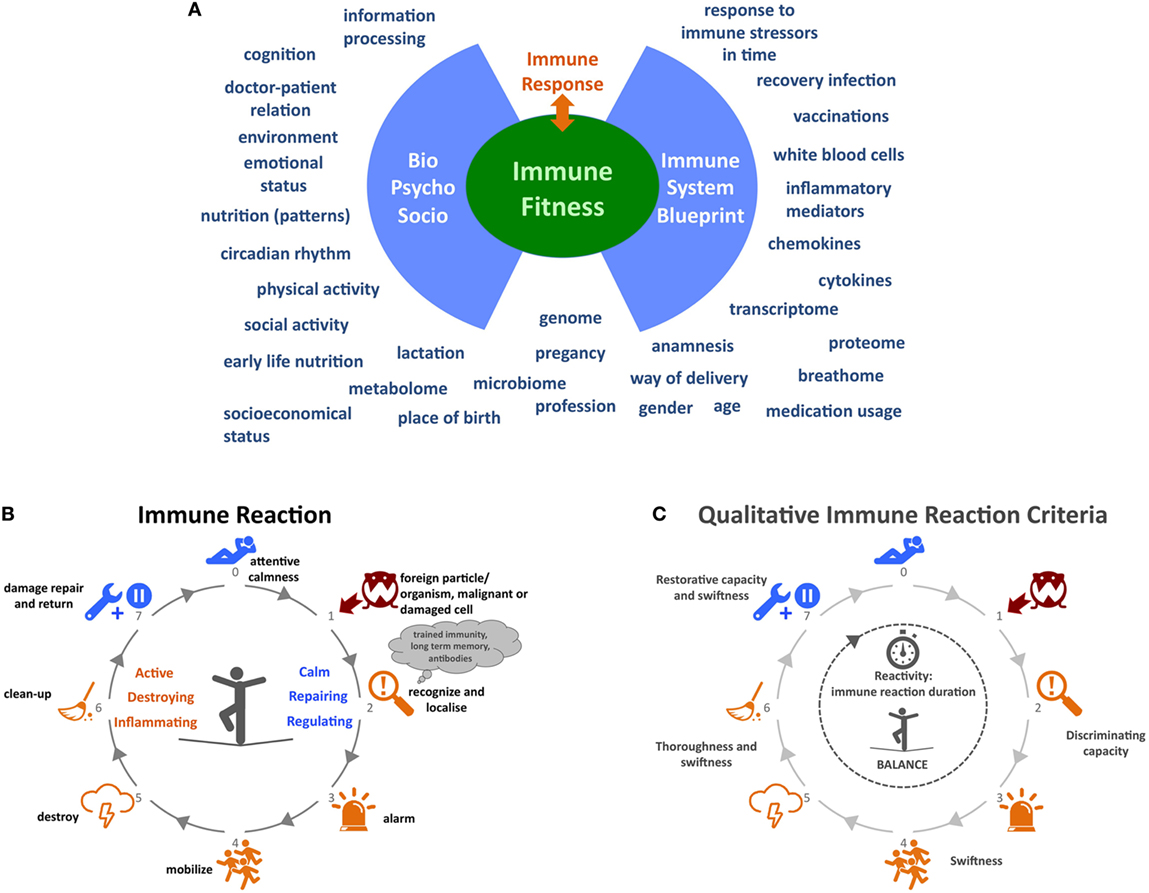
Figure 1. Immune functioning: embracing complexity. (A) A wide variety of determinants influencing immune function. (B) Process overview of the immune system behavior. (C) Qualitative criteria for the behavior of the immune system. At step 2 “recognize and localize” the system must be able to discriminate between true threats and harmless organisms or cells. The discriminating capacity of the system might be low in disorders like allergies, some autoimmune diseases, and immunodeficiencies. Between steps 3 and 5, the swiftness or speed of alarming, mobilizing, and destroying is key for the immune system’s effectiveness in combatting pathogens before they start growing or mutating. The same applies to the thoroughness of destroying and cleaning up pathogens in steps 5 and 6 so that no or very little strain on the body remains after the infection. The capacity to restore any damage after the infection at step 7 and the speed at which the system returns to an attemptive calmness is an often forgotten aspect of the immune system’s capabilities. In autoimmune diseases, this might be disrupted. In conclusion, the whole duration of the immune reaction to a certain pathogen can also be an indicator for (un)healthy behavior. Icons in panels (B,C) made by Katarina Stefanikova (lightning cloud) and Freepik (other icons) are from www.flaticon.com.
Common Pathways in CIDs
The existence of common pathogenic pathways in different types of CIDs indicates that in addition to focusing on single CID research we should make an effort to understand the commonalities of CIDs. In recent years, a large number of genome-wide association studies (GWAS) for CIDs have been performed to identify single nucleotide polymorphisms (SNPs) associated with disease phenotypes. Several hundreds of disease-associated SNPs have been identified including a large number of variants that are shared between CIDs (8–10). For the interpretation of the GWAS findings, the first steps are taken to understand the function of individual genes, their interactions, and to get insights into how these genetic variants are associated with the underlying immune disease (11–16). Follow-up studies should also incorporate epigenetics, transcriptomics, proteomics, and genome engineering to understand the functional relevance of SNPs (17, 18). Patient stratification based on cell-specific transcriptomic data was recently reported (19, 20). In addition, comorbidity of immune disorders, for example, in patients with inflammatory bowel diseases, psoriasis, or rheumatoid arthritis (21, 22) demonstrates that common mechanisms probably underlie immune disorders (23). Recent work in the field of systems medicine (24, 25) shows commonalities at the molecular and cellular level between subgroups of patients diagnosed with different CIDs. Also, indications that most of the CIDs share the same effector mechanisms and pathways of inflammation can be exemplified by the observation that specific therapeutic approaches in one specific CID can often be used in another CID as well. Examples are the use of anti-TNFα treatment in rheumatoid arthritis, psoriasis, and IBD (26–28) and targeting the IL-12/IL-23 pathway in various other CIDs (29). We can conclude that, despite the presentation of different clinical symptoms and involvement of different organs, distinct CIDs share common immune mechanisms.
General Psychosocial and Lifestyle Factors Associated with Immune Functioning
Next to genetic or physiological processes related to immune functioning, other general factors might impact immune functioning in both healthy individuals and patients with CIDs. It is important to look into the evidence regarding the role of psychosocial and lifestyle factors on different elements of immune system functioning, as an insight into these factors may provide leads to improved immune functioning in immunocompromised populations by means of non-invasive, non-medical strategies.
Psychosocial Factors
There is convincing evidence supporting a role of psychosocial factors in influencing immune processes and inflammatory function in both healthy and immunocompromised conditions. For example, since the seminal paper by Cohen and colleagues (30), consistent evidence has been reported for the link between both acute and chronic psychological stress and the suppression of immune system functioning (e.g., percentage of T suppressor/cytotoxic cells, levels of pro-inflammatory cytokines, and antibody response to immunization) (31–33). Next to the role of stress exposure, cognitive behavioral ways of dealing with stress, such as more passive-avoidant coping, and social resources, for instance, lack of social support, influence longer-term immune-related outcomes, such as disease progression, as shown for example in natural course studies of acute infections or patients with chronic inflammatory diseases (31). Although more research is needed into the mechanisms that underlie the association between psychological factors and immune system functioning, the available evidence suggests that corresponding psychosocial factors impact generic inflammatory parameters of immune function and as such are potentially useful factors for intervention in order to improve immune functioning.
In line with the evidence for psychosocial factors as potentially impacting on immune function, psychosocial interventions, particularly stress-management interventions and cognitive behavioral therapies, have been shown to affect indicators of immune function, such as lymphocyte counts, pro-inflammatory cytokine levels, or inflammatory activity (32–34). Evidence for the beneficial effects of interventions at this level is somewhat less consistent than for stress research, particularly due to the lower number of studies systematically measuring the same immune-related outcome parameters. Corresponding findings across diseases for both psychosocial factors, such as stress, and psychosocial interventions, such as stress-management interventions, suggest the involvement of common psychosocial mechanisms both in a fit and disturbed immune function, independent of the disease-specific pathogenic mechanisms.
At a molecular and cellular level several known neuro–immune interactions also support these findings. For instance, neurons produce cytokines and express “immunological receptors” like toll-like, immunoglobulin, cytokine, and chemokine receptors. Vice versa, immune cells produce neuropeptides and neurotransmitters and express their receptors (35, 36). The bidirectional loop between immune and nervous system was also highlighted by the fact that CID patients have an elevated risk for depression (37–40).
In view of the common psychological mechanisms that seem to play a role for both a fit and disturbed immune function, independent of the disease-specific pathways, integrated research strategies across conditions are needed. However, knowledge about possible common processes is not yet systematically used and integrated in the disease-oriented research traditions. Consequently, different lines of research usually independently exist, and exchange of knowledge across diseases or for both diseases and well-functioning immune function is very limited. Integration of knowledge from different disciplines enables an integrated theoretical and clinical approach that takes into account both the role of disease-specific and generic psychosocial and biological factors as well as their possible interactions in stimulating and disturbing immune function for both healthy subjects and patients, with the ultimate goal to better understand immune function across health and disease.
Lifestyle Factors
We are what we eat and where or how we live (41). The environment shapes our immune system. It is now well-known that lifestyle (e.g., diet, drinking) has an enormous influence on our immune system directly or indirectly through the microbiome (42–46). Also, the duration of exposure to lifestyle factors (our age) is an important factor, as are many other mostly under-investigated factors such as sleep rhythm. To demonstrate just the tip of the iceberg in lifestyle factors potentially impacting on immune function, two factors are discussed here, intestinal microbiome and physical activity.
The intestinal microbiota contributes to human health through its ability to release energy and nutrients from food as well as regulating host immune and metabolic functions (47). The microbiota lives in symbiosis with the host. In the adult human, the two main phyla are Firmicutes, Gram-positive bacteria, and Bacteroidetes, Gram-negative bacteria (48). The development of the intestinal microbiota blueprint occurs in the first 1,000 days of life and is a dynamic process influenced by mode of delivery, antibiotic use, and early-life nutrition (49). Many experimental models show that the gut microbiota is vital for normal immune development and regulation (50). Pioneer bacteria colonizing the infant intestinal tract and gradual diversification toward a stable gut ecosystem play a crucial role in establishing stable host–microbe interactions and an optimal symbiosis between them. A crucial step in healthy postnatal microbiota development is the developing mucosal immune system that distinguishes between beneficial and pathogenic (microbial) entities. As such, an altered microbiota composition in early or later life can be linked to a number of CIDs (51). In addition, there is a parallel and interacting microbiota and immune system development throughout the life span with critical periods such as early life, but also adolescence (52–54) and old age (55–58), the latter possibly more involved in late-onset CIDs. Although multifactorial aspects are involved in CIDs, changes in microbiota and activity are implicated in a broad range of these chronic conditions (43). The altered intestinal colonization patterns in CIDs are associated with reduced microbial diversity as a common denominator. All of this is regarded as dysbiosis: “an imbalanced microbiota composition and/or activity, which disrupts the host–microbiota homeostasis” (59, 60), resulting in an overactive immune response associated with CIDs.
An additional environmental factor with possible pronounced effects on the function of the immune system is physical activity (61). It is, for instance, often reported that walking a marathon is not healthy, which has been confirmed in studies in recreational marathon runners showing a number of adverse changes in diverse immune system components that may leave the individual more susceptible to infectious diseases for a short time period (62). In line with this, several immune parameters have been shown to be suppressed when people train intensively for a prolonged period of time, including decrease of neutrophil function, immunoglobulin concentration, and natural killer cell counts, being associated with increased infection susceptibility (61, 63–65). On the other hand, epidemiological data show that regular moderate physical activity contributes to the prevention of disease and promotion of health (63, 64). Recently, in a systematic review based on studies examining PBMC gene expression, it has been demonstrated that prolonged and regular physical activity promotes inflammation dampening effects, possibly resulting in a reduced risk for the development or exacerbation of CIDs (66).
Challenges: Embracing Complexity
Integrating and Extending Data and Including Immune System Dynamics
Considering the immune system, there is still a giant leap between the current limited sets of parameters determined in the various consortia (67–70) and the actual usage of the data for integration, as depicted in Table 1, there are many parameters of the immune system that can be measured, but most initiatives limit measurements to a subset of parameters based on genetics or immune phenotyping.
The main paradox in most current approaches is that measuring static immune function parameters does not comply with the fact that the immune system is a reactive as well as a dynamic system. Checking a static situation by a single measurement of serum proteins at a certain point in time does not reflect the response capacities of an individual’s immune system. An already well-explored option is to perform longitudinal ex vivo experiments with (isolated) blood or tissue-derived cells of the person in question and expose these cells to a number of different relevant immune stimuli, such as bacterial and viral compounds or immune proteins (cytokines or immunoglobulins) (71). An obvious, albeit inevitable, limitation of this approach is that the cells are removed from their physiological context and placed in an artificial culture system. Nevertheless, these experiments can show us the dimensions and variability of the response to distinct immunological stimuli. From these data, network representations of the immune system that reflect the dynamic feedback-regulated interactions involving many different components can be constructed (72). In addition, we will need to include as much information as possible about (epi)genetic, transcriptomic, proteomic, environmental, and psychosociological factors. When including the last mentioned factors into the equation, human research is inevitable and calls for new techniques from the field of big data science to be able to identify essential interactions between the immune system and its internal and external surrounding systems. Furthermore, new techniques are needed to describe and simulate the behavior of the immune system as a complex adaptive system. For this we suggest to apply complex adaptive systems science, for example, those that are successfully applied in studying and modeling of ecosystems (73).
Measures for Healthy Systems Behavior
In its interaction with other physiological systems in the body and its versatile reaction to external factors, the immune system of a person might show typical behaviors that could indicate susceptibility to certain CIDs. The systematic investigation of this system behavior requires measurement methods that include the system’s dynamics.
In 2008, Mark Davis, immunologist at Stanford University, asked the question “How is my immune system?” and elaborated on the fact that we are unable to give an answer, because we lack metrics of immunological health in humans (74). To develop such metrics, we need to start developing meaningful metrics for the behavior, adaptiveness, and responsiveness of the system as a whole. These metrics could be a measure for the “fitness” of the immune system. Currently, our knowledge does not allow us to determine the immune fitness of an individual and, consequently, it is impossible to determine how (much) an individual patient’s immune function deviates from a fit immune system. Such a view would also help us to define and understand different degrees of immune fitness. The big immunological picture will enable us to treat patients much more efficiently and effectively and successfully revert the “sick” immune system to a fit state. It will also help healthy individuals to stay fit. The depicted immune reaction process in Figure 1B is a simplified attempt to describe the behavior, adaptiveness, and responsiveness of the system as a whole, in order to demonstrate that a different approach can lead to helpful new ideas. In Figure 1C, a selection of qualitative criteria are shown that are derived from this approach and that could be developed into quantitative and normative criteria for the behavior of the immune system.
The Need to Integrate Experience-Based Knowledge
We are in great need for innovative multidisciplinary scientific research that enriches immunological knowledge with experience-based knowledge of a large group of CID patients, which incorporates a broad view on health, including psychosocial and lifestyle aspects (75).
A way to integrate experience-based knowledge into scientific research directly from the source (the patients themselves) is to translate patient narratives into hypotheses and include data from the narratives into the all-encompassing analyses. By designing a specific mix of qualitative, story-provoking questions and quantitative questions that have been designed to add meaning to certain aspects of their narratives, patients are invited to tell about their experiences with their CID(s) from their own perspective (76). For instance, patients could tell about life events that might be associated with their CID(s) or lifestyle factors that, to their experience, influence their disease activity. When a sufficient number of narratives will be collected, an evaluation and analysis of the narratives can point out certain patterns that emerge. If both the design of the questions and the evaluation of the narratives are done by a group that includes not only scientists and specialists but also patients, this bottom-up “participatory narrative inquiry” method is a powerful method for gaining unexpected insights and leads in the complex domain of CIDs.2
For the analysis of (extended) immune system (sub)phenotypes, we need to collect multiple data from well-defined patients and healthy volunteer cohorts, using a multidisciplinary and longitudinal approach. Collaboration between immunologists, computational biologists, patients, and their family suffering from CID(s), computer scientists, health-care professionals, physicians, psychologists, sociologists, physiologists, physicists, pharmacologists, pharmacists, microbiologists, dieticians, and others is essential to find creative ideas and solutions to complex problems [see also Ref. (77) on collaborations]. Current genotype–phenotype databases have their limitations because they are generally disease-focused, not publicly available, and contain incomplete data and annotation (78). We should collect as many individual data as we can, without overlooking things because of prior exclusion of supposedly irrelevant information or only include those hypothesized to be directly involved, to be able to find new subtypes as well as common pathways of CIDs, and additionally possible prevention strategies and new ways of treatment (79). In this way, we will refrain from the reductionist view that is based on incomplete knowledge of the biological mechanisms of gene functions in isolated cells and tissues or in inbred mice. From the analysis of these data, new hypotheses can be generated (80). To ensure transparency, reproducibility, and reusability, the data management should be guided by the recently published FAIR (Findability, Accessibility, Interoperability, and Reusability) data principles (81).
Both top-down and bottom-up approaches (82–84) can be used to investigate the commonalities between different CIDs. Using top-down approaches and data from public resources, one may attempt to reconstruct biological networks that are common in multiple CIDs. Alternatively, bottom-up approaches can be used to develop and integrate smaller but more detailed mathematical models to understand properties of the immune system. The integration of psychosocial factors is only possible when data are available from studies in which these factors were actually assessed, which generally will not be the case. Therefore, we believe that additional large-scale studies are required to investigate the effects of such factors on biological networks. Bottom-up approaches may also be used to study perturbation of disease pathways involved in comorbidity. This requires knowledge of such pathways. (Hypothesized) Psychosocial effects might be included in such mathematical models. To the best of our knowledge, top-down and bottom-up approaches have not yet been used to study commonalities in CIDs. Using these approaches, the comparison of patients with CIDs and healthy individuals may lead to a better definition of immune fitness. With this approach, we might also be able to learn to understand the physiology of unexplained complaints such as discomfort and fatigue.
Once we were able to determine the boundaries and dynamics of an adequate immune reaction, it is possible to identify aberrances in patients with CIDs. In this way, we might find an answer to the question whether derailing immunity is a consequence of a high (or low) responsive state in groups of patients determined by a certain genetic and environmental make-up. By doing so, we can develop comprehensive risk models that integrate a certain immune status with extensive omics and a broad environmental risk profiles that includes cognitive, emotional, dietary, and social risk factors. This will result in tools such as biomarkers and predictive algorithms that can support prevention and clinical management.
Conclusion
In this position document, we propose the concept that elucidating treatment and prevention of CIDs can be much accelerated when we start connecting diseases to underlying processes and place disease mechanisms at a molecular and cellular level in a much broader perspective. In order to understand which (common) factors contribute to the onset or exacerbation of CIDs and which mechanisms keep the immune system in homeostasis or drive the system into disease states, components such as life style, emotions, cognitions, and behaviors must also be incorporated. Current computational and complexity science will allow us to generate insight into the complex behavior of the whole system if we dare to start developing new techniques from this field and apply it to unravel the big picture.
Author Contributions
All the authors contributed to the conception and design of the work and interpretation of the data; AV, TB, AvK, AK, AE, HM, CB, and IJ did the acquisition and analysis of the data and drafted the work; and BH, EH, JM, LJ-B, RP, KK, MH, AB, JG, TR, and BP revised it critically for important intellectual content. All the authors approved the final version of the manuscript and agreed to be accountable for all aspects of the work.
Conflict of Interest Statement
The authors declare that the research was conducted in the absence of any commercial or financial relationships that could be construed as a potential conflict of interest.
Acknowledgments
The authors thank Marlou Mackus, Thom de Milliano, Bastiaan de Graaf, and Hugo McGurran for their analysis of consortia topics and the patients and other members of the Immunowell initiative for inspiring and stimulating discussions.
Footnotes
References
1. Cooper GS, Bynum ML, Somers EC. Recent insights in the epidemiology of autoimmune diseases: improved prevalence estimates and understanding of clustering of diseases. J Autoimmun (2009) 33(3–4):197–207. doi: 10.1016/j.jaut.2009.09.008
2. El-Gabalawy H, Guenther LC, Bernstein CN. Epidemiology of immune-mediated inflammatory diseases: incidence, prevalence, natural history, and comorbidities. J Rheumatol Suppl (2010) 85:2–10. doi:10.3899/jrheum.091461
3. Global Burden of Disease Study Collaborators. Global, regional, and national incidence, prevalence, and years lived with disability for 301 acute and chronic diseases and injuries in 188 countries, 1990–2013: a systematic analysis for the Global Burden of Disease Study 2013. Lancet (2015) 386(9995):743–800. doi:10.1016/S0140-6736(15)60692-4
4. Hood L, Auffray C. Participatory medicine: a driving force for revolutionizing healthcare. Genome Med (2013) 5(12):110. doi:10.1186/gm514
5. Kvedar JC, Fogel AL, Elenko E, Zohar D. Digital medicine’s march on chronic disease. Nat Biotechnol (2016) 34(3):239–46. doi:10.1038/nbt.3495
6. Uzzi B, Mukherjee S, Stringer M, Jones B. Atypical combinations and scientific impact. Science (2013) 342(6157):468–72. doi:10.1126/science.1240474
7. Elliott JH, Grimshaw J, Altman R, Bero L, Goodman SN, Henry D, et al. Informatics: make sense of health data. Nature (2015) 527(7576):31–2. doi:10.1038/527031a
8. Cotsapas C, Hafler DA. Immune-mediated disease genetics: the shared basis of pathogenesis. Trends Immunol (2013) 34(1):22–6. doi:10.1016/j.it.2012.09.001
9. Cho JH, Feldman M. Heterogeneity of autoimmune diseases: pathophysiologic insights from genetics and implications for new therapies. Nat Med (2015) 21(7):730–8. doi:10.1038/nm.3897
10. Li YR, Li J, Zhao SD, Bradfield JP, Mentch FD, Maggadottir SM, et al. Meta-analysis of shared genetic architecture across ten pediatric autoimmune diseases. Nat Med (2015) 21(9):1018–27. doi:10.1038/nm.3933
11. Lee MN, Ye C, Villani AC, Raj T, Li W, Eisenhaure TM, et al. Common genetic variants modulate pathogen-sensing responses in human dendritic cells. Science (2014) 343(6175):1246980. doi:10.1126/science.1246980
12. Fairfax BP, Humburg P, Makino S, Naranbhai V, Wong D, Lau E, et al. Innate immune activity conditions the effect of regulatory variants upon monocyte gene expression. Science (2014) 343(6175):1246949. doi:10.1126/science.1246949
13. Roederer M, Quaye L, Mangino M, Beddall MH, Mahnke Y, Chattopadhyay P, et al. The genetic architecture of the human immune system: a bioresource for autoimmunity and disease pathogenesis. Cell (2015) 161(2):387–403. doi:10.1016/j.cell.2015.02.046
14. Zhang Y, Su HC, Lenardo MJ. Genomics is rapidly advancing precision medicine for immunological disorders. Nat Immunol (2015) 16(10):1001–4. doi:10.1038/ni.3275
15. Gorenshteyn D, Zaslavsky E, Fribourg M, Park CY, Wong AK, Tadych A, et al. Interactive big data resource to elucidate human immune pathways and diseases. Immunity (2015) 43(3):605–14. doi:10.1016/j.immuni.2015.08.014
16. Li Y, Oosting M, Deelen P, Ricaño-Ponce I, Smeekens S, Jaeger M, et al. Inter-individual variability and genetic influences on cytokine responses to bacteria and fungi. Nat Med (2016) 22(8):952–60. doi:10.1038/nm1016-1192b
17. Tak YG, Farnham PJ. Making sense of GWAS: using epigenomics and genome engineering to understand the functional relevance of SNPs in non-coding regions of the human genome. Epigenetics Chromatin (2015) 8:57. doi:10.1186/s13072-015-0050-4
18. Farh KK, Marson A, Zhu J, Kleinewietfeld M, Housley WJ, Beik S, et al. Genetic and epigenetic fine mapping of causal autoimmune disease variants. Nature (2015) 518(7539):337–43. doi:10.1038/nature13835
19. Bradley SJ, Suarez-Fueyo A, Moss DR, Kyttaris VC, Tsokos GC. T cell transcriptomes describe patient subtypes in systemic lupus erythematosus. PLoS One (2015) 10(11):e0141171. doi:10.1371/journal.pone.0141171
20. Lee JC, Lyons PA, McKinney EF, Sowerby JM, Carr EJ, Bredin F, et al. Gene expression profiling of CD8+ T cells predicts prognosis in patients with Crohn disease and ulcerative colitis. J Clin Invest (2011) 121(10):4170–9. doi:10.1172/JCI59255
21. Li WQ, Han JL, Chan AT, Qureshi AA. Psoriasis, psoriatic arthritis and increased risk of incident Crohn’s disease in US women. Ann Rheum Dis (2013) 72(7):1200–5. doi:10.1136/annrheumdis-2012-202143
22. Schmitt J, Schwarz K, Baurecht H, Hotze M, Fölster-Holst R, Rodríguez E, et al. Atopic dermatitis is associated with an increased risk for rheumatoid arthritis and inflammatory bowel disease, and a decreased risk for type 1 diabetes. J Allergy Clin Immunol (2016) 137(1):130–6. doi:10.1016/j.jaci.2015.06.029
23. Loftus EV Jr. Inflammatory bowel disease extending its reach. Gastroenterology (2005) 129(3):1117–20. doi:10.1053/j.gastro.2005.07.042
24. Barrenäs F, Chavali S, Alves AC, Coin L, Jarvelin MR, Jörnsten R, et al. Highly interconnected genes in disease-specific networks are enriched for disease-associated polymorphisms. Genome Biol (2012) 13(6):R46. doi:10.1186/gb-2012-13-6-r46
25. Gustafsson M, Nestor CE, Zhang H, Barabási AL, Baranzini S, Brunak S, et al. Modules, networks and systems medicine for understanding disease and aiding diagnosis. Genome Med (2014) 6(10):82. doi:10.1186/s13073-014-0082-6
26. Cantini F, Niccoli L, Nannini C, Cassara E, Kaloudi O, Giulio Favalli E, et al. Tailored first-line biologic therapy in patients with rheumatoid arthritis, spondyloarthritis, and psoriatic arthritis. Semin Arthritis Rheum (2016) 45(5):519–32. doi:10.1016/j.semarthrit.2015.10.001
27. Elyoussfi S, Thomas BJ, Ciurtin C. Tailored treatment options for patients with psoriatic arthritis and psoriasis: review of established and new biologic and small molecule therapies. Rheumatol Int (2016) 36(5):603–12. doi:10.1007/s00296-016-3436-0
28. Danese S, Vuitton L, Peyrin-Biroulet L. Biologic agents for IBD: practical insights. Nat Rev Gastroenterol Hepatol (2015) 12(9):537–45. doi:10.1038/nrgastro.2015.135
29. Teng MW, Bowman EP, McElwee JJ, Smyth MJ, Casanova JL, Cooper AM, et al. IL-12 and IL-23 cytokines: from discovery to targeted therapies for immune-mediated inflammatory diseases. Nat Med (2015) 21(7):719–29. doi:10.1038/nm.3895
30. Cohen S, Tyrrell DA, Smith AP. Psychological stress and susceptibility to the common cold. N Engl J Med (1991) 325(9):606–12. doi:10.1056/NEJM199108293250903
31. Segerstrom SC, Miller GE. Psychological stress and the human immune system: a meta-analytic study of 30 years of inquiry. Psychol Bull (2004) 130(4):601–30. doi:10.1037/0033-2909.130.4.601
32. Kiecolt-Glaser JK, Glaser R. Psychoneuroimmunology: can psychological interventions modulate immunity? J Consult Clin Psychol (1992) 60(4):569–75. doi:10.1037/0022-006X.60.4.569
33. Miller GE, Cohen S. Psychological interventions and the immune system: a meta-analytic review and critique. Health Psychol (2001) 20(1):47–63. doi:10.1037/0278-6133.20.1.47
34. Morgan N, Irwin MR, Chung M, Wang C. The effects of mind-body therapies on the immune system: meta-analysis. PLoS One (2014) 9(7):e100903. doi:10.1371/journal.pone.0100903
35. Cohen S, Janicki-Deverts D, Doyle WJ, Miller GE, Frank E, Rabin BS, et al. Chronic stress, glucocorticoid receptor resistance, inflammation, and disease risk. Proc Natl Acad Sci U S A (2012) 109(16):5995–9. doi:10.1073/pnas.1118355109
36. Cohen S, Miller GE, Rabin BS. Psychological stress and antibody response to immunization: a critical review of the human literature. Psychosom Med (2001) 63(1):7–18. doi:10.1097/00006842-200101000-00002
37. Mikocka-Walus A, Knowles SR, Keefer L, Graff L. Controversies revisited: a systematic review of the comorbidity of depression and anxiety with inflammatory bowel diseases. Inflamm Bowel Dis (2016) 22(3):752–62. doi:10.1097/MIB.0000000000000620
38. Kiecolt-Glaser JK, Derry HM, Fagundes CP. Inflammation: depression fans the flames and feasts on the heat. Am J Psychiatry (2015) 172(11):1075–91. doi:10.1176/appi.ajp.2015.15020152
39. Miller AH, Raison CL. The role of inflammation in depression: from evolutionary imperative to modern treatment target. Nat Rev Immunol (2016) 16(1):22–34. doi:10.1038/nri.2015.5
40. Gibney SM, Drexhage HA. Evidence for a dysregulated immune system in the etiology of psychiatric disorders. J Neuroimmune Pharmacol (2013) 8(4):900–20. doi:10.1007/s11481-013-9462-8
41. Brussow H, Parkinson SJ. You are what you eat. Nat Biotechnol (2014) 32(3):243–5. doi:10.1038/nbt.2845
42. Thorburn AN, Macia L, Mackay CR. Diet, metabolites, and “western-lifestyle” inflammatory diseases. Immunity (2014) 40(6):833–42. doi:10.1016/j.immuni.2014.05.014
43. Forbes JD, Van Domselaar G, Bernstein CN. The gut microbiota in immune-mediated inflammatory diseases. Front Microbiol (2016) 7:1081. doi:10.3389/fmicb.2016.01081
44. Putignani L, Dallapiccola B. Foodomics as part of the host-microbiota-exposome interplay. J Proteomics (2016) 147:3–20. doi:10.1016/j.jprot.2016.04.033
45. Thaiss CA, Zmora N, Levy M, Elinav E. The microbiome and innate immunity. Nature (2016) 535(7610):65–74. doi:10.1038/nature18847
46. Basson A, Trotter A, Rodriguez-Palacios A, Cominelli F. Mucosal interactions between genetics, diet, and microbiome in inflammatory bowel disease. Front Immunol (2016) 7:290. doi:10.3389/fimmu.2016.00290
47. Cénit MC, Matzaraki V, Tigchelaar EF, Zhernakova A. Rapidly expanding knowledge on the role of the gut microbiome in health and disease. Biochim Biophys Acta (2014) 1842(10):1981–92. doi:10.1016/j.bbadis.2014.05.023
48. Lozupone CA, Stombaugh JI, Gordon JI, Jansson JK, Knight R. Diversity, stability and resilience of the human gut microbiota. Nature (2012) 489(7415):220–30. doi:10.1038/nature11550
49. Wopereis H, Oozeer R, Knipping K, Belzer C, Knol J. The first thousand days – intestinal microbiology of early life: establishing a symbiosis. Pediatr Allergy Immunol (2014) 25(5):428–38. doi:10.1111/pai.12232
50. West CE, Renz H, Jenmalm MC, Kozyrskyj AL, Allen KJ, Vuillermin P, et al. The gut microbiota and inflammatory noncommunicable diseases: associations and potentials for gut microbiota therapies. J Allergy Clin Immunol (2015) 135(1):3–13; quiz 14. doi:10.1016/j.jaci.2014.11.012
51. O’Mahony SM, Stilling RM, Dinan TG, Cryan JF. The microbiome and childhood diseases: focus on brain-gut axis. Birth Defects Res C Embryo Today (2015) 105(4):296–313. doi:10.1002/bdrc.21118
52. Agans R, Rigsbee L, Kenche H, Michail S, Khamis HJ, Paliy O. Distal gut microbiota of adolescent children is different from that of adults. FEMS Microbiol Ecol (2011) 77(2):404–12. doi:10.1111/j.1574-6941.2011.01120.x
53. Guarino A, Wudy A, Basile F, Ruberto E, Buccigrossi V. Composition and roles of intestinal microbiota in children. J Matern Fetal Neonatal Med (2012) 25(Suppl 1):63–6. doi:10.3109/14767058.2012.663231
54. Ringel-Kulka T, Cheng J, Ringel Y, Salojärvi J, Carroll I, Palva A, et al. Intestinal microbiota in healthy U.S. young children and adults – a high throughput microarray analysis. PLoS One (2013) 8(5):e64315. doi:10.1371/journal.pone.0064315
55. Biagi E, Nylund L, Candela M, Ostan R, Bucci L, Pini E, et al. Through ageing, and beyond: gut microbiota and inflammatory status in seniors and centenarians. PLoS One (2010) 5(5):e10667. doi:10.1371/journal.pone.0010667
56. Biagi E, Candela M, Turroni S, Garagnani P, Franceschi C, Brigidi P. Ageing and gut microbes: perspectives for health maintenance and longevity. Pharmacol Res (2013) 69(1):11–20. doi:10.1016/j.phrs.2012.10.005
57. Claesson MJ, Jeffery IB, Conde S, Power SE, O’Connor EM, Cusack S, et al. Gut microbiota composition correlates with diet and health in the elderly. Nature (2012) 488(7410):178–84. doi:10.1038/nature11319
58. Claesson MJ, Cusack S, O’Sullivan O, Greene-Diniz R, de Weerd H, Flannery E, et al. Composition, variability, and temporal stability of the intestinal microbiota of the elderly. Proc Natl Acad Sci U S A (2011) 108(Suppl 1):4586–91. doi:10.1073/pnas.1000097107
59. Sommer F, Backhed F. The gut microbiota – masters of host development and physiology. Nat Rev Microbiol (2013) 11(4):227–38. doi:10.1038/nrmicro2974
60. West CE, Jenmalm MC, Prescott SL. The gut microbiota and its role in the development of allergic disease: a wider perspective. Clin Exp Allergy (2015) 45(1):43–53. doi:10.1111/cea.12332
61. Lancaster GI, Febbraio MA. Exercise and the immune system: implications for elite athletes and the general population. Immunol Cell Biol (2016) 94(2):115–6. doi:10.1038/icb.2015.103
62. Nieman DC. Marathon training and immune function. Sports Med (2007) 37(4–5):412–5. doi:10.2165/00007256-200737040-00036
63. Walsh NP, Gleeson M, Pyne DB, Nieman DC, Dhabhar FS, Shephard RJ, et al. Position statement. Part two: maintaining immune health. Exerc Immunol Rev (2011) 17:64–103.
64. Walsh NP, Gleeson M, Shephard RJ, Gleeson M, Woods JA, Bishop NC, et al. Position statement. Part one: immune function and exercise. Exerc Immunol Rev (2011) 17:6–63.
65. Horn P, Kalz A, Lim CL, Pyne D, Saunders P, Mackinnon L, et al. Exercise-recruited NK cells display exercise-associated eHSP-70. Exerc Immunol Rev (2007) 13:100–11.
66. Gjevestad GO, Holven KB, Ulven SM. Effects of exercise on gene expression of inflammatory markers in human peripheral blood cells: a systematic review. Curr Cardiovasc Risk Rep (2015) 9(7):34. doi:10.1007/s12170-015-0463-4
67. Benoist C, Lanier L, Merad M, Mathis D; Immunological Genome Project. Consortium biology in immunology: the perspective from the Immunological Genome Project. Nat Rev Immunol (2012) 12(10):734–40. doi:10.1038/nri3300
68. Sheridan C. Omics-driven startups challenge healthcare model. Nat Biotechnol (2015) 33(9):887–9. doi:10.1038/nbt0915-887
69. Arazi A, Pendergraft WF III, Ribeiro RM, Perelson AS, Hacohen N. Human systems immunology: hypothesis-based modeling and unbiased data-driven approaches. Semin Immunol (2013) 25(3):193–200. doi:10.1016/j.smim.2012.11.003
70. Germain RN, Meier-Schellersheim M, Nita-Lazar A, Fraser ID. Systems biology in immunology: a computational modeling perspective. Annu Rev Immunol (2011) 29:527–85. doi:10.1146/annurev-immunol-030409-101317
71. Duffy D, Rouilly V, Libri V, Hasan M, Beitz B, David M, et al. Functional analysis via standardized whole-blood stimulation systems defines the boundaries of a healthy immune response to complex stimuli. Immunity (2014) 40(3):436–50. doi:10.1016/j.immuni.2014.03.002
72. Subramanian N, Torabi-Parizi P, Gottschalk RA, Germain RN, Dutta B. Network representations of immune system complexity. Wiley Interdiscip Rev Syst Biol Med (2015) 7(1):13–38. doi:10.1002/wsbm.1288
73. Scheffer M, van Nes EH. Self-organized similarity, the evolutionary emergence of groups of similar species. Proc Natl Acad Sci U S A (2006) 103(16):6230–5. doi:10.1073/pnas.0508024103
74. Davis MM. A prescription for human immunology. Immunity (2008) 29(6):835–8. doi:10.1016/j.immuni.2008.12.003
75. Huber M, Knottnerus AJ, Green L, van der Horst H, Jadad AR, Kromhout D, et al. How should we define health? BMJ (2011) 343:d4163. doi:10.1136/bmj.d4163
76. Kuper A, Reeves S, Levinson W. An introduction to reading and appraising qualitative research. BMJ (2008) 337:a288. doi:10.1136/bmj.a288
77. Bourzac K. Collaborations: mining the motherlodes. Nature (2015) 527(7576):S8–9. doi:10.1038/527S8a
78. Brookes AJ, Robinson PN. Human genotype-phenotype databases: aims, challenges and opportunities. Nat Rev Genet (2015) 16(12):702–15. doi:10.1038/nrg3932
79. Chan AC, Behrens TW. Personalizing medicine for autoimmune and inflammatory diseases. Nat Immunol (2013) 14(2):106–9. doi:10.1038/ni.2473
80. Mazzocchi F. Could Big Data be the end of theory in science? A few remarks on the epistemology of data-driven science. EMBO Rep (2015) 16(10):1250–5. doi:10.15252/embr.201541001
81. Wilkinson MD, Dumontier M, Aalbersberg IJ, Appleton G, Axton M, Baak A, et al. The FAIR Guiding Principles for scientific data management and stewardship. Sci Data (2016) 3:160018. doi:10.1038/sdata.2016.18
82. Bruggeman FJ, Westerhoff HV. The nature of systems biology. Trends Microbiol (2007) 15(1):45–50. doi:10.1016/j.tim.2006.11.003
83. Petranovic D, Vemuri GN. Impact of yeast systems biology on industrial biotechnology. J Biotechnol (2009) 144(3):204–11. doi:10.1016/j.jbiotec.2009.07.005
Keywords: chronic immune disorders, common pathways, data integration, life style, psychosocial factors
Citation: te Velde AA, Bezema T, van Kampen AHC, Kraneveld AD, 't Hart BA, van Middendorp H, Hack EC, van Montfrans JM, Belzer C, Jans-Beken L, Pieters RH, Knipping K, Huber M, Boots AMH, Garssen J, Radstake TR, Evers AWM, Prakken BJ and Joosten I (2016) Embracing Complexity beyond Systems Medicine: A New Approach to Chronic Immune Disorders. Front. Immunol. 7:587. doi: 10.3389/fimmu.2016.00587
Received: 09 June 2016; Accepted: 28 November 2016;
Published: 12 December 2016
Edited by:
Masaaki Murakami, Hokkaido University, JapanCopyright: © 2016 te Velde, Bezema, van Kampen, Kraneveld, 't Hart, van Middendorp, Hack, van Montfrans, Belzer, Jans-Beken, Pieters, Knipping, Huber, Boots, Garssen, Radstake, Evers, Prakken and Joosten. This is an open-access article distributed under the terms of the Creative Commons Attribution License (CC BY). The use, distribution or reproduction in other forums is permitted, provided the original author(s) or licensor are credited and that the original publication in this journal is cited, in accordance with accepted academic practice. No use, distribution or reproduction is permitted which does not comply with these terms.
*Correspondence: Anje A. te Velde, a.a.tevelde@amc.nl