- 1Department of Medicine, Division of Immunology and Rheumatology, Stanford University School of Medicine, Stanford, CA, USA
- 2Institute for Immunity, Transplantation, and Infection, Stanford University School of Medicine, Stanford, CA, USA
Autoimmunity is highly coincident with immunodeficiency. In a small but growing number of primary immunodeficiencies, autoantibodies are diagnostic of a given disease and implicated in disease pathogenesis. In order to improve our understanding of the role of autoantibodies in immunodeficiencies and to discover novel autoantibodies, new proteomic tools are needed. Protein microarrays have the ability to screen for reactivity to hundreds to many thousands of unique autoantigens simultaneously on a single chip using minimal serum input. Here, we review different types of protein microarrays and how they can be useful in framing the study of primary and secondary immunodeficiencies.
Introduction
Primary immunodeficiency is integrally tied to autoimmunity. A frequent misconception is that the immune system is a one-dimensional balance: that immunodeficiency is a state of immune system under-activation and autoimmunity is a state of over-activation. Although appealing theoretically, the large majority of diseases in either category contradict such a model. In fact, in many immunodeficiencies, autoimmunity is a persistent and dangerous clinical problem (1, 2). Conversely, in many autoimmune diseases, patients are more susceptible to both common and opportunistic infections (3). These clinical observations suggest a complex relationship between autoimmunity and immunodeficiency.
Measurement of circulating autoantibodies has been a critical tool for defining and understanding clinical autoimmune disease. From diagnostic and research perspectives, autoantibodies have the advantage of being highly stable secreted molecules present in blood at high concentrations. Additionally, they are plausible drivers of tissue damage (4). Unlike T cell receptors, the other mechanism by which adaptive immune cells generate specificity, antibodies directly recognize their cognate antigen without requiring antigen processing and presentation. These advantages make antibodies widely used reagents with applications ranging from biomarkers to therapeutics.
Historically, the first clinically relevant autoantibodies were discovered in the mid-20th century following observations of the morphological effects of serum on leukocytes (5, 6). Over the remainder of the 20th century, new techniques emerged which allowed for greater ease of use and specificity. Techniques such as the radiobinding assay, western blot, and enzyme-linked immunosorbent assay (ELISA) allowed for the detection of antibodies against a specific antigen or epitope, rather than against complex mixtures such as cell lysates (Table 1).
Each of these tools, however, required an a priori hypothesis or knowledge of the autoantigen. In a way, autoantibody profiling has been limited to single targets in a manner analogous to the limitations of the study of gene expression prior to DNA microarrays and high-throughput sequencing. To allow for the study of autoantibodies from a proteomic perspective, highly multiplexed tools are needed.
Here, we briefly review assays pertinent to the detection and study of autoantibodies. We then describe different types of autoantigen protein microarrays and discuss the advantages and disadvantages of each platform. We also review the literature of autoantibodies in immunodeficiency and discuss the role of protein microarrays in addressing unanswered questions. Lastly, we close with theoretical insights into the autoantibody response from a systems perspective made possible by the study of autoantibodies with microarrays.
Protein Microarray Technologies
DNA microarrays revolutionized the study of gene expression. The first generation of DNA microarrays was fabricated using a robotic printer to spot cDNA nucleotide features directly onto a planar surface, while some newer technologies use inkjet printing or maskless photolithography processes. In either case, fluorescently labeled cDNAs are incubated and allowed to hybridize to complementary features on the array. Arrays are washed, and feature binding is detected by a laser scanner (7, 8).
The paradigm shifting advantages of DNA microarrays were their highly multiplexed nature and minimal requirements for sample input, which allowed for an unbiased screen for relevant gene expression. The reproducibility and scalability of DNA microarrays also allowed for the creation of the Gene Expression Omnibus, a database repository of all published microarray data as a rich public resource (9).
Soon after the first DNA microarrays, it was demonstrated that protein microarrays could similarly be used for the detection of protein binding molecules, including autoantibodies in the serum of patients with autoimmune disease (10–14). Protein microarrays have been used as powerful tools to sub-classify patients with autoimmune diseases (15, 16), to monitor disease activity (17), and for the discovery of novel autoantibodies (18, 19). Although protein microarrays can be used to detect many types of molecules that bind to the printed features (20), in this review we will focus on protein microarrays for the detection of autoantibodies.
Protein Microarray Design and Implementation
Protein microarray protocols have been published previously (13, 18, 21, 22). Here, we provide an updated overview of protein microarray processing. We describe our experience and highlight different technologies and approaches relevant to protein microarrays in immunodeficiency. Detection and analysis of autoantibody reactivity by protein microarray have three key steps: (i) array design and fabrication; (ii) array probing, detection, and scanning; and (iii) image processing and data analysis (Figure 1).
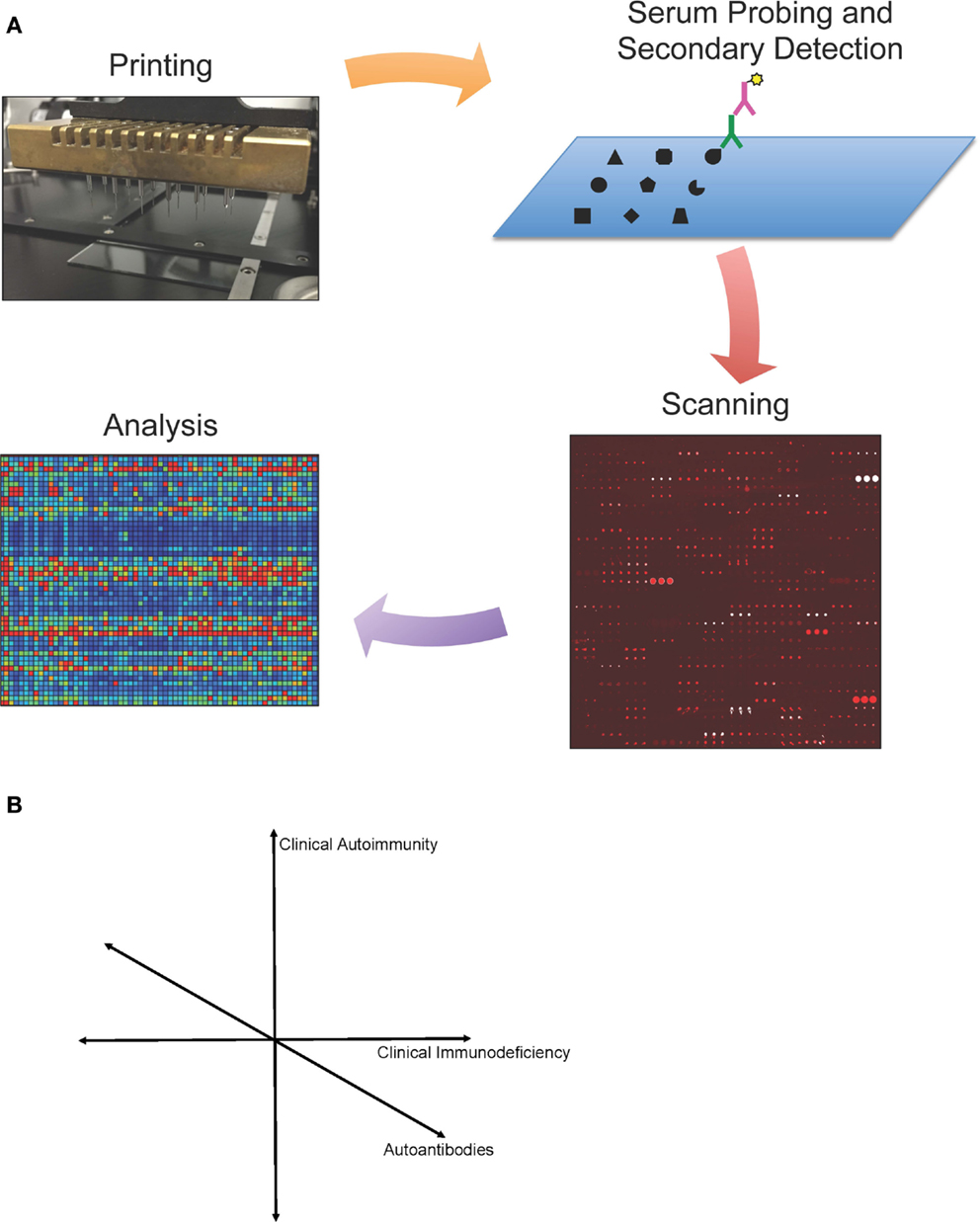
Figure 1. (A) Protein microarray technology. Schematic representation of protein microarrays used for autoantibody detection. Antigens are printed onto a specially coated microscope slide surface, and serum antibodies (green) are detected by a fluorescently conjugated secondary antibody (purple). Microarrays are then scanned, and images are analyzed using microarray software. Values are calculated for each antigen based on mean fluorescent intensity and a statistical analysis is performed. Data can be visualized in a heat map representation. (B) Simplified schematic representation of proposed map of primary immunodeficiencies.
Microarray Design and Fabrication
Protein microarrays can be designed and fabricated independently or purchased commercially. Array fabrication requires a microarray printer, purified antigens of interest (either expressed in the laboratory or purchased commercially), and a microarray surface on which to print, typically a specially coated microscope slide. Antigens are loaded into one or multiple 384 well plates at either a single concentration or a series of concentrations (our typical protein printing concentration is 200 μg/ml). A typical microarray printer can print on the scale of 100 microarrays over the course of 1 day.
The choice of surface on which to print should be guided by the technical requirements of each laboratory and also the chemistry of the antigens in question. Technically, some microarray scanner detectors are located on the opposite side of the laser source, which precludes the use of opaque microarray surfaces such as nitrocellulose. The two surfaces with which our lab has the most experience are nitrocellulose-coated (Maine Manufacturing) and epoxysilane-coated (SCHOTT) glass slides. The key trade-offs we have observed are that nitrocellulose has high protein-binding capacity, but also a high background fluorescence, which can vary depending on a patient’s serum. Epoxysilane-coated slides have almost no background fluorescence, which has the advantage of not requiring a background fluorescence subtraction step. However, this decreased background comes at the expense of a decreased quantity of protein bound per spot and a corresponding decrease in signal intensity.
Choice of array surface should also take into consideration the nature of antigen printed. Nucleic acids, proteins, carbohydrates, and lipids all have different mechanisms of binding to array substrates. Antigen chemistry should guide array substrate selection, both for adequate attachment of the molecule to the slide surface and to preserve the molecule’s structure. Other surfaces in various stages of development include amine-reactive surfaces, nitrocellulose film coated surfaces (23), plasmonic gold film surfaces for sensitivity enhancement (24), giant magnetoresistive (GMR) biosensor-based surfaces for kinetic measurements (25), and silicon surfaces which allow for high-density photolithographic peptide synthesis (26).
Antigen Content
Over the past decade and a half of protein microarray development, significant progress has been achieved in terms of increasing sensitivity of detection, the number of features printed on each microarray, and the diversity of molecules that can be printed. Initially printed with either nucleic acids or proteins, microarrays have been extended to profile complex mixtures of nucleic acids, proteins, peptides (27), lipids (28–30), and carbohydrates (31–33). Each of these molecules hold their own challenges discussed in the above references.
For laboratories without microarray printing expertise or equipment, protein, peptide, lipid, and carbohydrate microarrays are available from commercial vendors. Commercial microarray products span a spectrum from highly targeted microarrays with high coverage of a specific organism or antigen to broader coverage products with the capability to span the entire known proteome. Examples of targeted products include microarrays from Arrayit onto which key proteins from specific pathogens such as Plasmodium falciparum or Staphylococcus aureus are printed.
Examples of wide coverage microarray products include the ProtoArray® from Life Technologies, which includes over 9,000 full length human proteins expressed from cDNA constructs in an insect expression system (22). Another example of high-density commercial protein microarrays is peptide microarrays from PEPperPRINT. PEPperPrint sells both prefabricated or custom-printed peptide microarrays fabricated by combinatorial synthesis using laser printing (34).
Such large-scale screens for autoantibodies are appealing in theory, but often the vast number of features also comes at the cost of an increased background signal. Additionally, statistical correction for multiple observations further diminishes signal, in comparison to more focused experiments. Cost can also preclude larger experiments, as prices for each ProtoArray, for example, are approximately $1,000. Lastly, in these high coverage commercial products, each antigen is not necessarily validated beforehand, whereas for custom printed arrays, each antigen can be expressed or purchased and analyzed for quality and activity.
Other high coverage microarrays have been the product of large academic initiatives, most notably from the Swedish groups associated with the Human Protein Atlas initiative (35–37). The Protein Atlas group centered in Stockholm has tightly integrated multiple proteomic technologies including protein expression and monoclonal antibody production, and protein microarrays have been utilized throughout this process. Another center based at Arizona State University has developed nucleic acid programmable protein arrays, in which plasmids are printed on the chip and protein is expressed cell-free, in situ (38).
Lastly, there are core facilities, laboratories, and companies which offer printing and processing of microarrays as a send-out service, most notably from the University of Texas Southwestern Genomics and Microarray Core Facility, which offers a full printing, processing, and analysis service (16, 39).
Microarray Probing, Detection, and Scanning
Probing of microarrays is highly analogous to the probing and detection of a traditional ELISA. Printed microarrays are blocked in a blocking buffer to minimize non-specific binding. Serum is diluted into probing buffer (we typically dilute serum from immunodeficiency patients at 1:100, while for autoimmune diseases with higher signal intensity we dilute at 1:200). Using these dilutions, typically only 1–5 μl of serum or plasma per patient is required. Either serum or plasma can be used; however, it is important to be consistent in the use of either serum or plasma and to not to use them interchangeably, as there are key discrepancies, particularly among low reactivities, between the two blood products.
Given the highly multiplexed nature of the assay, care to avoid confounding factors in processing and analysis is key. Whenever possible, we run samples on the same day and in parallel. Additionally, randomizing the order in which controls and samples are probed aids in minimizing the potential for batch effects, which can be caused by discrepancies in incubation time lengths.
After probing, microarrays are washed and a fluorescent secondary antibody is applied. We typically use an IgG Fc-specific secondary antibody; however, many different isotypes and sub-classes can be measured through the use of isotype-specific secondary antibodies. For experiments where comparisons between isotype abundances are desired, samples can be processed in replicate, or a multi-color detection scheme can be employed (40); we typically use the Cy3 and/or Cy5 fluorescence channels. After incubation with secondary antibodies, the microarrays are then washed and dried. Best results are achieved when microarrays are scanned within 12 h of processing using a fluorescent microarray scanner per manufacturers’ instructions. Care should be taken to avoid repetitive scans of microarrays to avoid photobleaching.
Image Processing and Data Analysis
High quality microarray images are analyzed using microarray image processing software. The layout and orientation of antigens on the array are stored in one of multiple file formats, and loaded into the image software program, which overlays a corresponding grid of features over the scanned image. The mean fluorescent intensity (MFI) of each individual spot, or “feature,” on the microarray is then exported into a text file. We typically subtract local background fluorescence, and then average replicate features.
Normalization of microarray values should be approached with caution. In ideal settings, we run all samples on the same day to avoid the potential for normalization artifact. When normalization is necessary, median centering is one approach that has been useful (41). However, this approach operates under the assumption that the median value of each patient is equal, a situation that under certain circumstances (e.g., highly polyreactive samples) may not reflect true conditions. In particular, conditions of hypo- or hyper-gammaglobulinemia can alter these distributions. Another approach is to print standard features on each array, such as printing purified IgG or a fluorophore directly onto the array surface, and to normalize to this set of features. However, there can be subtle differences in how features are printed, e.g., the concentration of an antigen can vary due to evaporation during the progression of a print run.
A third approach to normalization when batches are run over multiple days is to use a standard sample with known reactivity as an internal control. Ratios of values from this one sample over different days can then be used as a normalization factor. Taken together, these approaches can be useful normalization tools when necessary; however, normalization should be approached with caution as each of these approaches has its own set of potential confounders.
Post-normalization, statistical differences in autoantibody reactivities can be assessed using significance analysis of microarrays (SAM) (42). SAM is a permutation-based algorithm for significance testing designed for microarray datasets that is less conservative than other corrections for multiple comparisons, such as the Bonferroni correction. In SAM, two or more groups can be compared, although for immunodeficiency studies it is typical to compare one immunodeficiency group to healthy controls. SAM is freely available and can be run either through a script in the R language or using software such as the Multiple Experiment Viewer (43). Through SAM, a false discovery rate can be set and statistically significant autoantibody reactivities identified.
We take the approach that any new result demonstrated by microarray should be replicated on a second independent platform such as ELISA, western blot, or a bead-based assay. This approach reduces the potential for platform-dependent artifacts. Further, platforms like ELISA can be more cost-effective to assess larger cohorts, after using microarray to identify select candidate antigens.
Reproducibility and standardization are critical for any autoantibody assay, and for decades, reference sera have been made available for standardization within and between institutions (44). Given new technologies in monoclonal antibody production, these reference sera can also be replaced with monoclonal antibodies of known affinity and specificity for the antigen of interest. Such monoclonal preparations have the advantage of being in infinite supply. Additionally, given the power of new technologies to discover novel autoantibodies, monoclonal antibodies may be more readily generated as reference standards than identifying reference sera. In our laboratory, we use both monoclonal and serum-based reference standards to ensure reproducibility across tests.
Current Knowledge of the Autoantibody Landscape in Primary Immunodeficiencies
Given the complex landscape of autoimmunity in immunodeficiency disorders, protein microarrays are a powerful platform to illuminate new aspects of disease pathophysiology and to identify novel biomarkers. Currently, relatively little is known about the full panoply of autoantibodies in primary immunodeficiency.
Most reports have studied individual disease cohorts and evaluated for classical autoantibodies. For example, a recent study found elevated levels of 12 autoantibodies in patients with DOCK8 deficiency (45). Additionally, cohort studies have found autoantibodies in such immunodeficiencies as immune dysregulation, polyendocrinopathy, enteropathy, X-linked (IPEX) (46) and selective IgA deficiency (47). However, larger scale studies of autoantibody reactivities in primary immunodeficiencies are lacking.
We recently profiled autoantibody responses in a blinded cohort of 58 serum samples from healthy individuals and a diverse set of patients with different immunodeficiencies. Using protein microarray data alone, we were able to accurately assign disease based on known anti-cytokine autoantibody reactivities, validating protein microarray technology for broader use. To extend these studies, we profiled a cohort of patients with RAG mutation-associated immunodeficiency and observed the presence of anti-cytokine autoantibodies (ACAAs) against type I interferons.
Anti-Cytokine Autoantibodies
A review of proteomics in immunodeficiency would be incomplete without discussion of the burgeoning field of ACAAs, which have been recently expertly reviewed in Ref. (48, 49). Briefly, ACAAs have been found to be tightly correlated with a handful of immunodeficiencies. Perhaps the best described highly sensitive and specific ACAAs include those against interferon γ in atypical mycobacterial infection or other opportunistic infection in Southeast Asia (50). A second key example is ACAAs against type I interferons, which can be observed with or without ACAAs against IL-17A and IL-22, in Autoimmune Polyendocrine Syndrome Type I (APS-1) (51, 52). It is also worth mentioning that the former study was conducted using luciferase-antigen fusion proteins while the latter studies utilized a bead-based system for autoantibody detection. Both of these systems are useful tools with an intermediate level of depth (10–30 antigens) between protein microarrays and individual ELISAs.
Utilizing proteomic approaches to study ACAAs has the potential to be extremely applicable to the study of immunodeficiency on multiple fronts. First, discovery of novel ACAAs has the potential to improve our understanding of disease pathophysiology. Second, proteomic approaches open up new avenues for biomarker discovery. Third, they suggest possible therapeutic opportunities based on cytokine biology. Each of these are active areas of investigation.
Whenever discussing autoantibodies, and in particular with regard to ACAAs, a caveat should be placed that the presence of autoantibodies, even in exquisitely specific patterns, does not demonstrate that those autoantibodies exert a pathologic effect. In fact, there are multiple well-studied autoantibodies that are thought to be markers of disease but do not contribute to the disease process (53). Direct pathologic effect has perhaps been most convincingly shown for the role of ACAAs against GM-CSF in the disease pulmonary alveolar proteinosis, where the cytokine GM-CSF is integrally involved in the disease process, and passive transfer of autoantibodies purified from the serum of patients with the disease reproduces the disease phenotype in non-human primates (54). Yet for the large majority of described ACAAs, to assign a pathologic effect is purely speculative, and more research is needed.
Autoantibodies in Healthy Individuals
To understand autoantibodies in any perturbed state such as disease, we first need to understand the basal state in healthy individuals. Unfortunately, little is known about the prevalence and role of autoantibodies in healthy individuals’ immune homeostasis. Clinically it is widely appreciated that rheumatologic disease-“specific” serologies have widely varying levels of specificity (55). The nature and function of autoantibodies in healthy individuals however is poorly understood.
Nowhere is this complexity more apparent than in the study of ACAAs. Multiple studies have found high levels of ACAAs in healthy individuals, including in preparations of intravenous immunoglobulin (56–59). While in some diseases certain ACAAs are specific to a disease, other ACAAs may have variable disease penetrance, acting in combination with other ACAAs or other factors to influence disease. More experiments are needed to answer the questions regarding the prevalence of ACAAs in healthy individuals and whether ACAAs or programs of ACAAs associate with specific genotypes or phenotypes. In particular, large ACAA screens of healthy individuals at different stages of human development and under different natural or experimental perturbations such as vaccination may be highly informative.
Discussion
Protein microarrays, alongside other proteomic technologies, are valuable tools to define the autoantibody repertoire in primary immunodeficiency. Essential biological questions still remain to be answered. What are the complete autoantibody profiles in each of the primary immunodeficiencies? Are these antibodies pathogenic, and can they be used as biomarkers for screening, diagnosis, or disease monitoring? Additionally, the role of autoantibodies and, in particular, the role of ACAAs in healthy individuals remains to be elucidated.
As we learn more about the autoantibody repertoires in different diseases, we also will have an opportunity to ask systems-level questions. As we generate data on hundreds to thousands of autoantibodies, we can begin to pair these data with other proteomic, genomic, and clinical measurements, hopefully emerging with models of autoimmune regulation across different states of immune function. Given the overlap of autoimmunity and immunodeficiency, these connections may help us better understand both autoimmune and immunodeficiency phenomena.
For example, the immunodeficiency IPEX is caused by mutations in the gene FOXP3. However, there remains a set of “IPEX-like” patients with autoimmunity and enteropathy. We are currently using protein microarrays to profile autoantibody responses in these patients with the hypothesis that sub-groups may have similar prognoses or similar therapeutic response profiles. Another important example is common variable immunodeficiency (CVID), the most common primary immunodeficiency (60). For CVID patients, we have paired autoantibody profiling with genomic and clinical information in order to try to sub-classify this highly heterogeneous disease.
We envision that given sufficient data sets from individual diseases, one can then map primary immunodeficiency diseases in a complex multi-dimensional space. The most intuitive of these approaches would be to map each primary immunodeficiency in a 3-dimensional space of clinical autoimmunity, clinical immunodeficiency, and autoantibodies (Figure 1B). However, each of these axes can be broken down into hundreds or thousands of individual axes, allowing for bioinformatic analysis to compare across diseases. Such analysis could shed new light on the diagnosis, pathophysiology, or treatment of each primary immunodeficiency.
In addition to descriptive profiling studies discussed above, antibody heavy and light chain cloning technologies allow for the expression of monoclonal autoantibodies for use in mechanistic experiments (61–64). Purified monoclonal antibodies allow for in vitro and in vivo experiments to test the necessity and sufficiency of an antibody for a given phenotype. Once monoclonal antibodies are isolated, protein microarrays can again be used to identify a cognate antigen or antigens.
Lastly, given the growing role of autoantibodies and ACAAs in immunodeficiency, protein microarrays have the potential as diagnostics in the clinic (65). A single microarray chip can screen for several autoantibodies important in both rheumatologic and immunodeficiency disorders. In complex patients with unknown diagnoses, such a chip could fill a significant clinical diagnostic need. In summary, protein microarrays have utility and promise in the field of primary immunodeficiency from basic research through clinical application.
Methods
The search terms “Autoantibodies and Immunodeficiency” and “Protein Microarray” were queried in the following databases: Pubmed, Scopus, Google Scholar, Stanford University Lane Library, and Web of Science.
Conflict of Interest Statement
The authors declare that the research was conducted in the absence of any commercial or financial relationships that could be construed as a potential conflict of interest.
Acknowledgments
The authors would like to acknowledge David James Haddon for constructive feedback on this manuscript. JR is supported by the National Institutes of Health under award number T32GM007365. PU is the recipient of a Donald E. and Delia B. Baxter Foundation Career Development Award, a gift from The Floren Family Trust, a gift from The Ben May Charitable Trust of Mobile, Alabama, and is supported by NHLBI Proteomics Contract- HHSN288201000034C, NIH grants (1 U19-AI1110491, 1 U19-AI090019, 1 UH2 AR067676, 1 UM2 AR067678), Alliance for Lupus Research (Grant Number 21858), FP Grant #261, and the research leading to these results has received funding from the European Union Seventh Framework Programme (FP7/2007–2013) under grant agreement number [261382].
References
1. Todoric K, Koontz JB, Mattox D, Tarrant TK. Autoimmunity in immunodeficiency. Curr Allergy Asthma Rep (2013) 13:361–70. doi:10.1007/s11882-013-0350-3
2. Arkwright PD, Abinun M, Cant AJ. Autoimmunity in human primary immunodeficiency diseases. Blood (2002) 99:2694–702. doi:10.1182/blood.V99.8.2694
3. Grammatikos AP, Tsokos GC. Immunodeficiency and autoimmunity: lessons from systemic lupus erythematosus. Trends Mol Med (2012) 18:101–8. doi:10.1016/j.molmed.2011.10.005
Pubmed Abstract | Pubmed Full Text | CrossRef Full Text | Google Scholar
4. Elkon K, Casali P. Nature and functions of autoantibodies. Nat Clin Pract Rheumatol (2008) 4:491–8. doi:10.1038/ncprheum0895
Pubmed Abstract | Pubmed Full Text | CrossRef Full Text | Google Scholar
5. Holman H. The discovery of autoantibody to deoxyribonucleic acid. Lupus (2011) 20:441–2. doi:10.1177/0961203310392433
6. Holman HR, Kunkel HG. Affinity between the lupus erythematosus serum factor and cell nuclei and nucleoprotein. Science (1957) 126:162–3. doi:10.1126/science.126.3265.162
7. Schena M, Shalon D, Davis RW, Brown PO. Quantitative monitoring of gene expression patterns with a complementary DNA microarray. Science (1995) 270:467–70. doi:10.1126/science.270.5235.467
Pubmed Abstract | Pubmed Full Text | CrossRef Full Text | Google Scholar
8. Chee M, Yang R, Hubbell E, Berno A, Huang XC, Stern D, et al. Accessing genetic information with high-density DNA arrays. Science (1996) 274:610–4. doi:10.1126/science.274.5287.610
Pubmed Abstract | Pubmed Full Text | CrossRef Full Text | Google Scholar
9. Edgar R, Domrachev M, Lash AE. Gene expression omnibus: NCBI gene expression and hybridization array data repository. Nucleic Acids Res (2002) 30:207–10. doi:10.1093/nar/30.1.207
Pubmed Abstract | Pubmed Full Text | CrossRef Full Text | Google Scholar
10. Maecker HT, Lindstrom TM, Robinson WH, Utz PJ, Hale M, Boyd SD, et al. New tools for classification and monitoring of autoimmune diseases. Nat Rev Rheumatol (2012) 8:317–28. doi:10.1038/nrrheum.2012.66
Pubmed Abstract | Pubmed Full Text | CrossRef Full Text | Google Scholar
11. MacBeath G, Schreiber SL. Printing proteins as microarrays for high-throughput function determination. Science (2000) 289:1760–3.
12. Zhu H, Bilgin M, Bangham R, Hall D, Casamayor A, Bertone P, et al. Global analysis of protein activities using proteome chips. Science (2001) 293:2101–5. doi:10.1126/science.1062191
Pubmed Abstract | Pubmed Full Text | CrossRef Full Text | Google Scholar
13. Robinson WH, DiGennaro C, Hueber W, Haab BB, Kamachi M, Dean EJ, et al. Autoantigen microarrays for multiplex characterization of autoantibody responses. Nat Med (2002) 8:295–301. doi:10.1038/nm0302-295
Pubmed Abstract | Pubmed Full Text | CrossRef Full Text | Google Scholar
14. Robinson WH, Steinman L, Utz PJ. Protein arrays for autoantibody profiling and fine-specificity mapping. Proteomics (2003) 3:2077–84. doi:10.1002/pmic.200300583
Pubmed Abstract | Pubmed Full Text | CrossRef Full Text | Google Scholar
15. Hueber W, Kidd BA, Tomooka BH, Lee BJ, Bruce B, Fries JF, et al. Antigen microarray profiling of autoantibodies in rheumatoid arthritis. Arthritis Rheum (2005) 52:2645–55. doi:10.1002/art.21269
Pubmed Abstract | Pubmed Full Text | CrossRef Full Text | Google Scholar
16. Li Q-Z, Zhen QL, Xie C, Wu T, Mackay M, Aranow C, et al. Identification of autoantibody clusters that best predict lupus disease activity using glomerular proteome arrays. J Clin Invest (2005) 115:3428–39. doi:10.1172/JCI23587
Pubmed Abstract | Pubmed Full Text | CrossRef Full Text | Google Scholar
17. Robinson WH, Fontoura P, Lee BJ, de Vegvar HEN, Tom J, Pedotti R, et al. Protein microarrays guide tolerizing DNA vaccine treatment of autoimmune encephalomyelitis. Nat Biotechnol (2003) 21:1033–9. doi:10.1038/nbt859
Pubmed Abstract | Pubmed Full Text | CrossRef Full Text | Google Scholar
18. Price JV, Haddon DJ, Kemmer D, Delepine G, Mandelbaum G, Jarrell JA, et al. Protein microarray analysis reveals BAFF-binding autoantibodies in systemic lupus erythematosus. J Clin Invest (2013) 123(12):5135–45. doi:10.1172/JCI70231
Pubmed Abstract | Pubmed Full Text | CrossRef Full Text | Google Scholar
19. Ho PP, Kanter JL, Johnson AM, Srinagesh HK, Chang E-J, Purdy TM, et al. Identification of naturally occurring fatty acids of the myelin sheath that resolve neuroinflammation. Sci Transl Med (2012) 4:137ra73. doi:10.1126/scitranslmed.3003831
Pubmed Abstract | Pubmed Full Text | CrossRef Full Text | Google Scholar
20. Cahill DJ. Protein and antibody arrays and their medical applications. J Immunol Methods (2001) 250:81–91. doi:10.1016/S0022-1759(01)00325-8
Pubmed Abstract | Pubmed Full Text | CrossRef Full Text | Google Scholar
21. Im H, Snyder M. Preparation of recombinant protein spotted arrays for proteome-wide identification of kinase targets. Curr Protoc Protein Sci (2013) 27:27.4. doi:10.1002/0471140864.ps2704s72
Pubmed Abstract | Pubmed Full Text | CrossRef Full Text | Google Scholar
22. Schweitzer B, Meng L, Mattoon D, Rai AJ. Immune response biomarker profiling application on protoarray® protein microarrays. Methods Mol Biol (2010) 641:243–52. doi:10.1007/978-1-60761-711-2_14
Pubmed Abstract | Pubmed Full Text | CrossRef Full Text | Google Scholar
23. Angenendt P, Glökler J, Sobek J, Lehrach H, Cahill DJ. Next generation of protein microarray support materials: evaluation for protein and antibody microarray applications. J Chromatogr A (2003) 1009:97–104. doi:10.1016/S0021-9673(03)00769-6
Pubmed Abstract | Pubmed Full Text | CrossRef Full Text | Google Scholar
24. Chen Z, Tabakman SM, Goodwin AP, Kattah MG, Daranciang D, Wang X, et al. Protein microarrays with carbon nanotubes as multicolor Raman labels. Nat Biotechnol (2008) 26:1285–92. doi:10.1038/nbt.1501
Pubmed Abstract | Pubmed Full Text | CrossRef Full Text | Google Scholar
25. Gaster RS, Xu L, Han S-J, Wilson RJ, Hall DA, Osterfeld SJ, et al. Quantification of protein interactions and solution transport using high-density GMR sensor arrays. Nat Nanotechnol (2011) 6:314–20. doi:10.1038/nnano.2011.45
Pubmed Abstract | Pubmed Full Text | CrossRef Full Text | Google Scholar
26. Price JV, Tangsombatvisit S, Xu G, Yu J, Levy D, Baechler EC, et al. On silico peptide microarrays for high-resolution mapping of antibody epitopes and diverse protein-protein interactions. Nat Med (2012) 18:1434–40. doi:10.1038/nm.2913
Pubmed Abstract | Pubmed Full Text | CrossRef Full Text | Google Scholar
27. Andresen H, Bier FF. Peptide microarrays for serum antibody diagnostics. Methods Mol Biol (2009) 509:123–34. doi:10.1007/978-1-59745-372-1_8
Pubmed Abstract | Pubmed Full Text | CrossRef Full Text | Google Scholar
28. Feng L. Probing lipid-protein interactions using lipid microarrays. Prostaglandins Other Lipid Mediat (2005) 77:158–67. doi:10.1016/j.prostaglandins.2004.09.003
Pubmed Abstract | Pubmed Full Text | CrossRef Full Text | Google Scholar
29. Kanter JL, Narayana S, Ho PP, Catz I, Warren KG, Sobel RA, et al. Lipid microarrays identify key mediators of autoimmune brain inflammation. Nat Med (2006) 12:138–43. doi:10.1038/nm1344
Pubmed Abstract | Pubmed Full Text | CrossRef Full Text | Google Scholar
30. Saliba A-E, Vonkova I, Ceschia S, Findlay GM, Maeda K, Tischer C, et al. A quantitative liposome microarray to systematically characterize protein-lipid interactions. Nat Methods (2014) 11:47–50. doi:10.1038/nmeth.2734
Pubmed Abstract | Pubmed Full Text | CrossRef Full Text | Google Scholar
31. Fukui S, Feizi T, Galustian C, Lawson AM, Chai W. Oligosaccharide microarrays for high-throughput detection and specificity assignments of carbohydrate-protein interactions. Nat Biotechnol (2002) 20:1011–7. doi:10.1038/nbt735
Pubmed Abstract | Pubmed Full Text | CrossRef Full Text | Google Scholar
32. Blixt O, Head S, Mondala T, Scanlan C, Huflejt ME, Alvarez R, et al. Printed covalent glycan array for ligand profiling of diverse glycan binding proteins. Proc Natl Acad Sci U S A (2004) 101:17033–8. doi:10.1073/pnas.0407902101
Pubmed Abstract | Pubmed Full Text | CrossRef Full Text | Google Scholar
33. Schneider C, Smith DF, Cummings RD, Boligan KF, Hamilton RG, Bochner BS, et al. The human IgG anti-carbohydrate repertoire exhibits a universal architecture and contains specificity for microbial attachment sites. Sci Transl Med (2015) 7:269ra1. doi:10.1126/scitranslmed.3010524
Pubmed Abstract | Pubmed Full Text | CrossRef Full Text | Google Scholar
34. Beyer M, Nesterov A, Block I, König K, Felgenhauer T, Fernandez S, et al. Combinatorial synthesis of peptide arrays onto a microchip. Science (2007) 318:1888–1888. doi:10.1126/science.1149751
Pubmed Abstract | Pubmed Full Text | CrossRef Full Text | Google Scholar
35. Uhlen M, Oksvold P, Fagerberg L, Lundberg E, Jonasson K, Forsberg M, et al. Towards a knowledge-based human protein atlas. Nat Biotechnol (2010) 28:1248–50. doi:10.1038/nbt1210-1248
36. Uhlén M, Björling E, Agaton C, Szigyarto CA-K, Amini B, Andersen E, et al. A human protein atlas for normal and cancer tissues based on antibody proteomics. Mol Cell Proteomics (2005) 4:1920–32. doi:10.1074/mcp.M500279-MCP200
Pubmed Abstract | Pubmed Full Text | CrossRef Full Text | Google Scholar
37. Ayoglu B, Häggmark A, Khademi M, Olsson T, Uhlén M, Schwenk JM, et al. Autoantibody profiling in multiple sclerosis using arrays of human protein fragments. Mol Cell Proteomics (2013) 12:2657–72. doi:10.1074/mcp.M112.026757
Pubmed Abstract | Pubmed Full Text | CrossRef Full Text | Google Scholar
38. Ramachandran N, Raphael JV, Hainsworth E, Demirkan G, Fuentes MG, Rolfs A, et al. Next-generation high-density self-assembling functional protein arrays. Nat Methods (2008) 5:535–8. doi:10.1038/nmeth.1210
Pubmed Abstract | Pubmed Full Text | CrossRef Full Text | Google Scholar
39. Li Q-Z, Zhou J, Wandstrat AE, Carr-Johnson F, Branch V, Karp DR, et al. Protein array autoantibody profiles for insights into systemic lupus erythematosus and incomplete lupus syndromes. Clin Exp Immunol (2007) 147:60–70. doi:10.1111/j.1365-2249.2006.03251.x
Pubmed Abstract | Pubmed Full Text | CrossRef Full Text | Google Scholar
40. Kattah MG, Alemi GR, Thibault DL, Balboni I, Utz PJ. A new two-color Fab labeling method for autoantigen protein microarrays. Nat Methods (2006) 3:745–51. doi:10.1038/nmeth910
Pubmed Abstract | Pubmed Full Text | CrossRef Full Text | Google Scholar
41. Kinloch AJ, Chang A, Ko K, Henry Dunand CJ, Henderson S, Maienschein-Cleine M, et al. Vimentin is a dominant target of in situ humoral immunity in human lupus tubulointerstitial nephritis. Arthritis Rheumatol (2014) 66(12):3359–70. doi:10.1002/art.38888
Pubmed Abstract | Pubmed Full Text | CrossRef Full Text | Google Scholar
42. Tusher VG, Tibshirani R, Chu G. Significance analysis of microarrays applied to the ionizing radiation response. Proc Natl Acad Sci U S A (2001) 98:5116–21. doi:10.1073/pnas.091062498
43. Saeed AI, Sharov V, White J, Li J, Liang W, Bhagabati N, et al. TM4: a free, open-source system for microarray data management and analysis. Biotechniques (2003) 34:374–8.
44. Smolen JS, Butcher B, Fritzler MJ, Gordon T, Hardin J, Kalden JR, et al. Reference sera for antinuclear antibodies. II. Further definition of antibody specificities in international antinuclear antibody reference sera by immunofluorescence and western blotting. Arthritis Rheum (1997) 40:413–8. doi:10.1002/art.1780400304
Pubmed Abstract | Pubmed Full Text | CrossRef Full Text | Google Scholar
45. Janssen E, Morbach H, Ullas S, Bannock JM, Massad C, Menard L, et al. Dedicator of cytokinesis 8-deficient patients have a breakdown in peripheral B-cell tolerance and defective regulatory T cells. J Allergy Clin Immunol (2014) 134:1365–74. doi:10.1016/j.jaci.2014.07.042
Pubmed Abstract | Pubmed Full Text | CrossRef Full Text | Google Scholar
46. Tsuda M, Torgerson TR, Selmi C, Gambineri E, Carneiro-Sampaio M, Mannurita SC, et al. The spectrum of autoantibodies in IPEX syndrome is broad and includes anti-mitochondrial autoantibodies. J Autoimmun (2010) 35:265–8. doi:10.1016/j.jaut.2010.06.017
Pubmed Abstract | Pubmed Full Text | CrossRef Full Text | Google Scholar
47. Aytekin C, Tuygun N, Gokce S, Dogu F, Ikinciogullari A. Selective IgA deficiency: clinical and laboratory features of 118 children in Turkey. J Clin Immunol (2012) 32:961–6. doi:10.1007/s10875-012-9702-3
Pubmed Abstract | Pubmed Full Text | CrossRef Full Text | Google Scholar
48. Browne SK, Holland SM. Anticytokine autoantibodies in infectious diseases: pathogenesis and mechanisms. Lancet Infect Dis (2010) 10:875–85. doi:10.1016/S1473-3099(10)70196-1
Pubmed Abstract | Pubmed Full Text | CrossRef Full Text | Google Scholar
49. Browne SK. Anticytokine autoantibody-associated immunodeficiency. Annu Rev Immunol (2014) 32:635–57. doi:10.1146/annurev-immunol-032713-120222
Pubmed Abstract | Pubmed Full Text | CrossRef Full Text | Google Scholar
50. Browne SK, Burbelo PD, Chetchotisakd P, Suputtamongkol Y, Kiertiburanakul S, Shaw PA, et al. Adult-onset immunodeficiency in Thailand and Taiwan. N Engl J Med (2012) 367:725–34. doi:10.1056/NEJMoa1111160
Pubmed Abstract | Pubmed Full Text | CrossRef Full Text | Google Scholar
51. Puel A, Döffinger R, Natividad A, Chrabieh M, Barcenas-Morales G, Picard C, et al. Autoantibodies against IL-17A, IL-17F, and IL-22 in patients with chronic mucocutaneous candidiasis and autoimmune polyendocrine syndrome type I. J Exp Med (2010) 207(2):291–7. doi:10.1084/jem.20091983
Pubmed Abstract | Pubmed Full Text | CrossRef Full Text | Google Scholar
52. Kisand K, Bøe Wolff AS, Podkrajšek KT, Tserel L, Link M, Kisand KV, et al. Chronic mucocutaneous candidiasis in APECED or thymoma patients correlates with autoimmunity to Th17-associated cytokines. J Exp Med (2010) 207:299–308. doi:10.1084/jem.20091669
Pubmed Abstract | Pubmed Full Text | CrossRef Full Text | Google Scholar
53. Smolen JS, Steiner G. Are autoantibodies active players or epiphenomena? Curr Opin Rheumatol (1998) 10:201–6. doi:10.1097/00002281-199805000-00008
54. Sakagami T, Uchida K, Suzuki T, Carey BC, Wood RE, Wert SE, et al. Human GM-CSF autoantibodies and reproduction of pulmonary alveolar proteinosis. N Engl J Med (2009) 361:2679–81. doi:10.1056/NEJMc0904077
55. van Boekel MA, Vossenaar ER, van den Hoogen FH, van Venrooij WJ. Autoantibody systems in rheumatoid arthritis: specificity, sensitivity and diagnostic value. Arthritis Res (2001) 4:1–7. doi:10.1186/ar395
56. Kim K, Waterer G, Thomson R, Yang IA, Nashi N, Tan DBA, et al. Levels of anti-cytokine antibodies may be elevated in patients with pulmonary disease associated with non-tuberculous mycobacteria. Cytokine (2014) 66:160–3. doi:10.1016/j.cyto.2014.01.005
Pubmed Abstract | Pubmed Full Text | CrossRef Full Text | Google Scholar
57. Meager A, Cludts I, Thorpe R, Wadhwa M. Are neutralizing anti-GM-CSF autoantibodies present in all healthy persons? Blood (2010) 115:433–4. doi:10.1182/blood-2009-08-241018
58. Watanabe M, Uchida K, Nakagaki K, Kanazawa H, Trapnell BC, Hoshino Y, et al. Anti-cytokine autoantibodies are ubiquitous in healthy individuals. FEBS Lett (2007) 581:2017–21. doi:10.1016/j.febslet.2007.04.029
Pubmed Abstract | Pubmed Full Text | CrossRef Full Text | Google Scholar
59. Galle P, Svenson M, Bendtzen K, Hansen MB. High levels of neutralizing IL-6 autoantibodies in 0.1% of apparently healthy blood donors. Eur J Immunol (2004) 34:3267–75. doi:10.1002/eji.200425268
Pubmed Abstract | Pubmed Full Text | CrossRef Full Text | Google Scholar
60. Park MA, Li JT, Hagan JB, Maddox DE, Abraham RS. Common variable immunodeficiency: a new look at an old disease. Lancet (2008) 372:489–502. doi:10.1016/S0140-6736(08)61199-X
Pubmed Abstract | Pubmed Full Text | CrossRef Full Text | Google Scholar
61. Wang Y, Thomson CA, Allan LL, Jackson LM, Olson M, Hercus TR, et al. Characterization of pathogenic human monoclonal autoantibodies against GM-CSF. Proc Natl Acad Sci U S A (2013) 110:7832–7. doi:10.1073/pnas.1216011110
Pubmed Abstract | Pubmed Full Text | CrossRef Full Text | Google Scholar
62. Robinson WH. Sequencing the functional antibody repertoire-diagnostic and therapeutic discovery. Nat Rev Rheumatol (2015) 11:171–82. doi:10.1038/nrrheum.2014.220
Pubmed Abstract | Pubmed Full Text | CrossRef Full Text | Google Scholar
63. Tan Y-C, Kongpachith S, Blum LK, Ju C-H, Lahey LJ, Lu DR, et al. Barcode-enabled sequencing of plasmablast antibody repertoires in rheumatoid arthritis. Arthritis Rheumatol (2014) 66:2706–15. doi:10.1002/art.38754
Pubmed Abstract | Pubmed Full Text | CrossRef Full Text | Google Scholar
64. Castiello MC, Bosticardo M, Pala F, Catucci M, Chamberlain N, van Zelm MC, et al. Wiskott-Aldrich syndrome protein deficiency perturbs the homeostasis of B-cell compartment in humans. J Autoimmun (2014) 50:42–50. doi:10.1016/j.jaut.2013.10.006
Pubmed Abstract | Pubmed Full Text | CrossRef Full Text | Google Scholar
65. Cretich M, Damin F, Chiari M. Protein microarray technology: how far off is routine diagnostics? Analyst (2014) 139:528–42. doi:10.1039/c3an01619f
Pubmed Abstract | Pubmed Full Text | CrossRef Full Text | Google Scholar
66. Pincus T, Schur PH, Rose JA, Decker JL, Talal N. Measurement of serum DNA-binding activity in systemic lupus erythematosus. N Engl J Med (1969) 281:701–5. doi:10.1056/NEJM196909252811304
67. Palmer JP, Asplin CM, Clemons P, Lyen K, Tatpati O, Raghu PK, et al. Insulin antibodies in insulin-dependent diabetics before insulin treatment. Science (1983) 222:1337–9. doi:10.1126/science.6362005
Pubmed Abstract | Pubmed Full Text | CrossRef Full Text | Google Scholar
68. Buchner C, Bryant C, Eslami A, Lakos G. Anti-nuclear antibody screening using HEp-2 cells. J Vis Exp (2014) 23(88):e51211. doi:10.3791/51211
Pubmed Abstract | Pubmed Full Text | CrossRef Full Text | Google Scholar
69. Johnson GD, Holborow EJ, Glynn LE. Antibody to smooth muscle in patients with liver disease. Lancet (1965) 2:878–9. doi:10.1016/S0140-6736(65)92505-5
70. Young BJ, Mallya RK, Leslie RD, Clark CJ, Hamblin TJ. Anti-keratin antibodies in rheumatoid arthritis. Br Med J (1979) 2:97–9. doi:10.1136/bmj.2.6182.97
71. Sebbag M, Simon M, Vincent C, Masson-Bessière C, Girbal E, Durieux JJ, et al. The antiperinuclear factor and the so-called antikeratin antibodies are the same rheumatoid arthritis-specific autoantibodies. J Clin Invest (1995) 95:2672–9. doi:10.1172/JCI117969
Pubmed Abstract | Pubmed Full Text | CrossRef Full Text | Google Scholar
72. Maguire GA, Ginawi A, Lee J, Lim AYN, Wood G, Houghton S, et al. Clinical utility of ANA measured by ELISA compared with ANA measured by immunofluorescence. Rheumatology (2009) 48:1013–4. doi:10.1093/rheumatology/kep137
73. Savige J, Davies D, Falk RJ, Jennette JC, Wiik A. Antineutrophil cytoplasmic antibodies and associated diseases: a review of the clinical and laboratory features. Kidney Int (2000) 57:846–62. doi:10.1046/j.1523-1755.2000.057003846.x
Pubmed Abstract | Pubmed Full Text | CrossRef Full Text | Google Scholar
74. Döffinger R, Helbert MR, Barcenas-Morales G, Yang K, Dupuis S, Ceron-Gutierrez L, et al. Autoantibodies to interferon-γ in a patient with selective susceptibility to mycobacterial infection and organ-specific autoimmunity. Clin Infect Dis (2004) 38:e10–4. doi:10.1086/380453
Pubmed Abstract | Pubmed Full Text | CrossRef Full Text | Google Scholar
75. O’Connell E, Rosen LB, LaRue RW, Fabre V, Melia MT, Auwaerter PG, et al. The first US domestic report of disseminated Mycobacterium avium complex and anti-interferon-γ autoantibodies. J Clin Immunol (2014) 34:928–32. doi:10.1007/s10875-014-0073-9
Pubmed Abstract | Pubmed Full Text | CrossRef Full Text | Google Scholar
Keywords: autoantibodies, primary immunodeficiencies, autoimmunity, protein microarrays, immunodeficiency
Citation: Rosenberg JM and Utz PJ (2015) Protein microarrays: a new tool for the study of autoantibodies in immunodeficiency. Front. Immunol. 6:138. doi: 10.3389/fimmu.2015.00138
Received: 10 February 2015; Accepted: 12 March 2015;
Published online: 07 April 2015.
Edited by:
Luigi Daniele Notarangelo, Harvard Medical School, USAReviewed by:
Silvia Gregori, San Raffaele Telethon Institute for Gene Therapy (HSR-TIGET), ItalyAndrew Gennery, Newcastle University, UK
Copyright: © 2015 Rosenberg and Utz. This is an open-access article distributed under the terms of the Creative Commons Attribution License (CC BY). The use, distribution or reproduction in other forums is permitted, provided the original author(s) or licensor are credited and that the original publication in this journal is cited, in accordance with accepted academic practice. No use, distribution or reproduction is permitted which does not comply with these terms.
*Correspondence: Jacob M. Rosenberg, 269 Campus Drive, Stanford, CA 94305, USA e-mail: jakeros@stanford.edu