- 1Centre for Performance Science, Royal College of Music, London, United Kingdom
- 2Faculty of Medicine, Imperial College London, London, United Kingdom
While the expansion of technologies into the music education classroom has been studied in great depth, there is a lack of published literature regarding the use of digital technologies by students learning in individual settings. Do musicians take their technology use into the practice room and teaching studio, or does the traditional nature of the master-apprentice teaching model promote different attitudes among musicians toward their use of technology in learning to perform? To investigate these issues, we developed the Technology Use and Attitudes in Music Learning Survey, which included adaptations of Davis's 1989 scales for Perceived Usefulness and Perceived Ease of Use of Technology. Data were collected from an international cohort of 338 amateur, student, and professional musicians ranging widely in age, specialism, and musical experience. Results showed a generally positive attitude toward current and future technology use among musicians and supported the Technology Acceptance Model (TAM), wherein technology use in music learning was predicted by perceived ease of use via perceived usefulness. Musicians' self-rated skills with smartphones, laptops, and desktop computers were found to extend beyond traditional audio and video recording devices, and the majority of musicians reported using classic music technologies (e.g., metronomes and tuners) on smartphones and tablets rather than bespoke devices. Despite this comfort with and access to new technology, availability reported within one-to-one lessons was half of that within practice sessions, and while a large percentage of musicians actively recorded their playing, these recordings were not frequently reviewed. Our results highlight opportunities for technology to take a greater role in improving music learning through enhanced student-teacher interaction and by facilitating self-regulated learning.
Introduction
The expansion of technology within society is a defining feature of the twenty-first century, revolutionizing how people work, learn, communicate, and spend their leisure time. This is particularly true in the domain of music, where technology has become a presence, if not a requirement, in musical creation, production, expression, dissemination, promotion, and consumption (Hugill, 2012). Music education is no exception, seeing significant study and growth and building upon general trends of technology use in the modern classroom (Purves, 2012; Sweeney et al., 2017). However, the attention given to understanding how and where technology is being used in music classroom settings has not been applied to the same extent in one-to-one teaching environments. The master-apprentice model of instrumental teaching can give the impression of an environment resistant to technological innovation (Creech and Gaunt, 2012; Gaunt, 2017). The present study sought to address this gap by examining the use of and attitudes toward technology in the one-to-one learning and teaching of music performance.
The role of technology in the music classroom has benefited from two decades of close attention. Early work examined the emerging use of and access to technological resources in the music classroom (Bray, 1997; Naughton, 1997; Rogers, 1997; Salaman, 1997), implications for teacher training (Hunt and Kirk, 1997) and potential applications for students with profound learning disabilities (Ellis, 1997). By 2000, inspections of 106 music classrooms found a high degree of technology use, emphasizing that good practice stemmed from a knowledge of how the technology functioned, ability to model use of the technology, and minimized time loss from setup (Mills and Murray, 2000). In the United Kingdom, a 2003 government report found that 24% of secondary teachers were making substantial use of technology in their classrooms, and 30% reported a positive effect on their teaching (DfES, 2005) The demonstrated benefits of these tools in the classroom led to calls for technology-based professional development workshops (Bauer et al., 2003), and teachers continued to develop strategies to incorporate the available tools at the time—recording, editing, playback, early web-based resources and videos—into their practices (Ho, 2004; Anderson and Ellis, 2005). An independent review by the Department for Education on music education recommended that further work was needed to develop a national plan to embrace technological innovation and ensure that teachers are kept up-to-date with new developments (Henley, 2011). This supports data from the European Commission (2013) which showed a substantial increase in numbers of computers and quality of broadband access in European schools from 2006 to 2012 and marginal growth in use, although fewer than half of teachers were making use of ICT in more than 25% of their classes. While updated statistics on technology use in the music classroom have not been provided, more recent studies have found that technology use is on the rise and in a growing set of contexts (Purves, 2012; Webster, 2012). Himonides and Purves (2010) surveyed the field, finding ten distinct roles technology took in the classroom, ranging from improving performance skills to facilitating communication to increasing teachers' abilities to assess the success of their students and their own teaching strategies. While technology may remain underused in the classroom, with the barriers including a lack of availability, technical competence, and institutional support (Kenny and McDaniel, 2011; Fautley, 2013; Gall, 2013), its influence is growing.
The explosion of online music resources has also shaped the sphere of music learning, both in the classroom and beyond. Millions of instructional music videos can be found via online portals such as YouTube, used not only by individuals in informal learning practices but being actively incorporated into educational frameworks (Waldron, 2012; Smart and Green, 2017). This accessibility may belie their utility, however. Thorgersen and Zandén (2014) asked nine beginner students aged 20–30 to learn to play new instruments solely through the instruction of online resources. The students focussed primarily on instructional videos and charts, generally avoiding tools allowing for commutation and expert feedback such as forums or assessment tools. While students have ever-greater access to information, there is a risk of them being overwhelmed by choice and distraction and lacking the framework that teacher-led training and tailored support can provide.
Less has been published regarding the role of technology in one-to-one teaching settings and instrumental learning. Existing evidence tends to be anecdotal or out-of-date relative to the quickly changing world of technology, such as one question in a survey of 100 instrumental teachers by Barry and Mcarthur (1994) who found that the majority of instrumental music teachers did not use or encourage their students to use software-based music learning tools, although the technologies available would have been limited at the time. The use of distance learning via videoconferenced lessons is growing, with research finding that skills such as sight-reading can be taught effectively over the medium (Pike and Shoemaker, 2013) and that students and teachers are able to operate the equipment and make the most of the technical and physical limitations (Kruse et al., 2013). The use of audio and video recordings, both in creating and viewing them, is also common, although the degree to which each of these activities is done remains unclear. Experimental studies have demonstrated their potential as tools to improve self-assessment (Johnston, 1993; Robinson, 1993; Daniel, 2001; Hewitt, 2002; Silveira and Gavin, 2016). Volioti and Williamon (2017) examined the use of audio recordings among instrumental learners, finding that students reported greater use of them than professionals, particularly for elements including goal setting and developing an interpretive style. This supported earlier research that found only a small proportion of professional musicians listened to the recordings of others as part of their practice (Hallam, 1995). Lindström et al. (2003), in a survey of attitudes toward the learning and teaching of musical expression, asked music students whether they felt modern techniques (such as computer programs) could be used to learn to play expressively and whether they would use them, as well as reactions on a scale from 0 (very negative) to 10 (very positive) to a hypothetical technology that could record and analyze audio features related to their performance and suggest possible changes to enhance their expressivity. Responses were generally negative, with 83% responding that modern techniques could not be used, with a mean positivity rating of only 3.6 (out of 10). Free comments showed that many students questioned technology's utility for contributing clarity and understanding to a topic as complex as musical expressivity.
Considering the lack of published literature on musicians' use of and attitudes toward music technology in instrumental learning, and the explosion of new technologies now available to them, this study examined (1) musicians' skills with and attitudes toward technologies in their day-to-day lives, (2) how they engage with technology in the learning of musical instruments, (3) how attitudes as music learners differ from music teachers, and (4) musicians' attitudes toward potential new technologies and what factors predict adoption of new tools. To investigate this, an exploratory survey study was designed and disseminated to an international cohort of musicians varying in age, experience, and instrument specialism.
Materials and Methods
Respondents
The respondents were 338 musicians (57% female) with a mean age of 29.7 years (SD = 11.9, range = 16–82). They had a mean experience of 16.8 years (SD = 10.7, range = 1–68 years), with representation from professional (29%), student (44%), and amateur (27%) groups and 94% having taken formal lessons on their primary instrument for a mean 11.5 years (SD = 7.1, range = 1–50). The cohort was international, representing 43 countries across six continents, with a significant proportion being British (43%) and the next highest representation from the USA, Lithuania, Singapore, and Canada (5–6% each). The range of primary instruments included keyboard (36%), strings (including guitar and electric bass; 35%), and woodwind and brass (23%), with the remaining 6% comprising a mix of percussion, vocal, and other instruments. Three quarters (76%) of the cohort reported classical as their primary genre, with the remaining quarter comprising jazz, folk, pop, and other. The survey opened with an information sheet outlining the topic and purpose of the study and instructing respondents that, by beginning the survey, they were providing informed consent. Ethical approval for the study, including consenting procedures, was granted by the Conservatoires UK Research Ethics Committee following the guidelines of the British Psychological Society.
Survey
The Technology Use and Attitudes in Music Learning Survey was developed for this study. The complete survey is available as Supplementary Material. The first section focused on standard demographic descriptors including age, sex, primary instrument, nationality, and musical experience. The second section elicited information on technology use in day-to-day life, including self-perceived skill in using a range of standard technologies (smartphones, laptops, desktop computers, tablets, smartwatches, televisions, audio and video recording equipment, audio playback equipment, and motion capture technologies), as well as the degree to which they seek out, enjoy using, and enjoy learning to use new technologies on 7-point scales from 1 (not at all) to 7 (very much). Respondents were also asked the degree to which they find day-to-day technologies easy to use and useful in an adaptation of Davis's (1989) scales for Perceived Usefulness and Perceived Ease of Use of Technology, which together predict actual use of technology. These factors form the foundation of the Technology Acceptance Model (TAM; see Figure 1; Davis et al., 1989), which serves to predict attitudes toward, intention to use, and actual use of technology. The TAM has been replicated, adapted, and applied in numerous domains, including that of technology use in educational contexts (Adams et al., 1992; Venkatesh et al., 2003; Edmunds et al., 2012; Martí-Parreño et al., 2016; Wu and Chen, 2017).
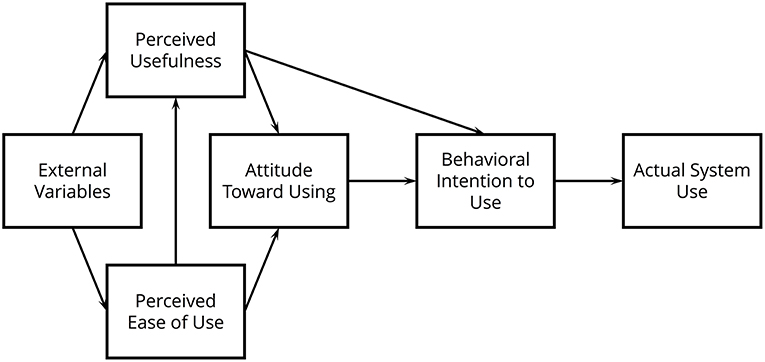
Figure 1. The Technology Acceptance Model (TAM). Figure adapted from Davis (1989, p. 985). In the model, perceived ease of use and usefulness of technologies predict attitudes toward and intention to use technology, which in turn predicts actual system use.
The third section asked about technology access, use, and attitudes in learning one's primary instrument, including whether the standard technologies listed above are available in their practice room and lesson space, whether the “classic” music technologies of metronomes, tuners, and audio/video recording devices are accessed via smartphone or on bespoke devices. They were then asked about drivers toward and barriers from incorporating technology into their music learning based on 7-point scales adapted from Gilbert (2015), how likely they are to use new technologies should they become available, and another adaption of the Davis (1989) scale of usefulness and ease of use in the context of music learning. The next questions investigated the degree to which musicians use technology to develop seven skill categories (technical, musical, ensemble, practice, presentation, career, and life) adapted from existing work to profile musicians' skills (Williamon et al., 2017) and including specific subskills (e.g., dynamics, rhythmic accuracy, tracking progress) for several of the categories. Closing this section, musicians were asked the degree to which they engaged in technology-driven musical activates including documenting how practice is spent, having videoconferenced lessons, and recording and viewing recordings of their own and others' playing.
The final section examined attitudes toward future technologies, including the perceived potential utility of new technology to help with the same skill categories and subskills listed above, and responses to three hypothetical technologies proposed by the authors involving the use of audio, video, and motion capture technologies to be used alone in the practice room or in conjunction with a teacher. A final section was presented for active music teachers only, briefly comparing their attitudes toward and use of technology in their roles as teachers to their roles as music learners and the degree to which they engaged in various technology-driven teaching activities including advertising, scheduling lessons, and tracking student progress. The survey also contained a section shown to violinists as part of a sister project, the results of which are not reported here and details of which are not included in the Supplementary Material.
Procedure and Analyses
The survey was distributed online via SurveyMonkey using social media channels and email lists, with assistance from a number of professional music organizations and educational institutions. The survey was designed to place general technology use early in the form, thus allowing for examination of the first area of focus (musicians' general skill with music technology in their day-to-day lives) with a data set prior to dropouts. Of the 338 respondents, complete data sets were recorded for 207. For all analyses, missing data were excluded casewise and N values and degrees of freedom are reported accordingly throughout.
To examine differences within participants' use of and attitudes toward technology, repeated-measures ANOVAs were employed with relevant items included as independent variables and the responses to those items (via commensurable 7-point scales) as the dependant variables. In cases where a rank-ordering of responses within survey item (e.g., skill at using devices) was of interest, items were ordered from highest to lowest mean descriptive value before entry into a repeated-measures ANOVA with a planned repeated contrast comparing each item with the one following. Thus, the contrast could determine where significant differences in skill existed within the ordering, and where groups of items emerged within which no significant difference could be found and serving as a “tie” in the rank ordering. For example, if a five-item scale were ordered A-E, items A and B may form a tied group in which A was not significantly higher than B. However, B may be significantly higher than C, after which no significant differences remain, leaving group A-B significantly higher than group C-E. Where Mauchly's W indicated a violation of sphericity (p < 0.05) when running analyses of variance (ANOVAs), Greenhouse-Geisser corrections are reported.
An adapted form of the Technology Acceptance Model (Davis, 1989; see Figure 1) was constructed and tested using partial least squares structural equation modeling (PLS-SEM; adapted model structure described below and in Figure 7) to examine predictors of perceived future technology use. The model was estimated using the software package SmartPLS (v. 3.2.7; Ringle et al., 2015), with a 500-sample bootstrapping procedure (bias-corrected and accelerated [BCa]) used to estimate significance levels.
Results
Musicians' General Technology Use
Musicians were asked the degree to which they were skilled at using a variety of technologies on 7-point scales. A repeated-measures ANOVA (with item as the independent variable and response as the dependent variable) with a repeated contrast was conducted to determine where significantly different groupings of skills occurred (as described above in “Procedure and Analyses”). The ANOVA revealed a significant main effect [F(6.02, 2028.68) = 429.10, p < 0.001, η2 = 0.56], with the contrast demonstrating five distinct groupings (see Table 1 and Figure 2). The highest skill confidence was found for laptops, smartphones, and desktop computers with no significant differences between them. This grouping was significantly higher than the television and tablet grouping, which was significantly higher than audio recording devices, itself significantly higher than the pairing of video recording devices and audio playback equipment. The lowest skills were reported for motion capture technologies and smartwatches with no significant differences between them but significantly lower than the video recording/audio playback grouping. Correlations between each of the skill categories and age were examined using Kendall's Tau. After applying a Bonferroni correction for multiple comparisons, the only significant relationships found were positive correlations between age and skill with audio recording devices and audio playback devices (τ = 0.11, p < 0.005; τ = 0.13, p < 0.001, respectively) and a negative correlation between age and skill with smartwatches (τ = −0.13, p < 0.005), although all correlations were weak (< 0.2). To examine sex differences, a series of between-groups t-tests was conducted. With the Bonferroni correction applied, significant small-effect differences were found for audio recording devices [women M = 4.00, SD = 1.97; men M = 4.76, SD = 1.95; t(336) = −3.51, p < 0.001, d = 0.38], video recording devices [women M = 3.70, SD = 1.96; men M = 4.41, SD = 1.94; t(336) = −3.32, p < 0.001, d = 0.36], and motion capture technologies [women M = 1.81, SD = 1.40; men M = 2.33, SD = 1.87; t(336) = −2.96, p < 0.005, d = 0.32], and a medium-effect difference found for audio playback devices [women M = 3.31, SD = 2.00; men M = 4.72, SD = 2.08; t(336) = −6.31, p < 0.001, d = 0.69], with men reporting higher figures in each case.
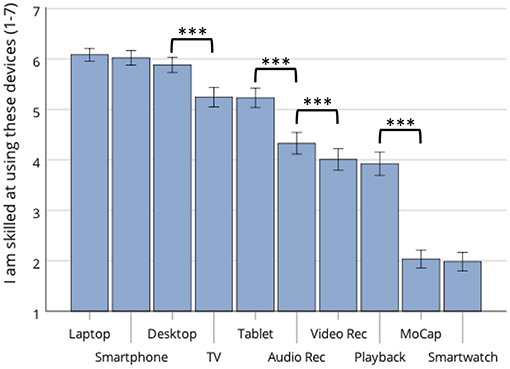
Figure 2. Mean self-reported skills for using technological devices. Musicians reported the highest skills in using laptop and desktop computers and smartphones, and the lowest for smart watches, and motion capture technologies. Skills with audio and video recording devices, as well as audio playback, were close to the midpoint. Age and sex had a relatively small effect on these ratings. Error bars show 95% CI. 1 = not at all, 7 = very; ***p < 0.001, as determined using a repeated measures ANOVA and a repeated contrast.
On the same 7-point scale, musicians were asked the degree to which they sought out new technologies (M = 4.41, SD = 1.72), enjoyed learning to use new technologies (M = 4.98, SD = 1.71), and enjoyed using new technologies (M = 5.08, SD = 1.61) in their day-to-day lives. Analyses using Kendall's Tau found no significant correlations with age, although Bonferroni-corrected t-tests showed men reporting a significantly higher tendency to seek out technology [women M = 4.01, SD = 1.63; men M = 4.93, SD = 1.71; t(336) = −5.04, p < 0.001, d = 0.55], to enjoy learning it [women M = 4.64, SD = 1.75; men M = 5.41, SD = 1.57; t(336) = −4.19, p < 0.001, d = 0.46], and to enjoy using it [women M = 4.75, SD = 1.63; men M = 5.50, SD = 1.48; t(336) = −4.32, p < 0.001, d = 0.47], with descriptive differences of approximately one point on the 7-point scale. Finally, musicians were asked the degree to which they found technology easy to use and useful in their day-to-day lives, using an adaption of Davis's (1989) scales. The six scales showed very high internal reliability (α > 0.90), with moderately high mean scores for the perceived usefulness (M = 5.72, SD = 0.97) and ease of use (M = 5.74, SD = 0.88) of their day-to-day technologies. No effects of age or sex were found.
Use of Technology in Music Learning
Musicians were asked whether they had access to a series of technologies in the spaces where they normally practice and receive lessons (see Table 2). Smartphones showed the highest prevalence (75%), followed by laptops (54%), tablets (38%), and audio recorders (36%). Across all technologies, approximately half of the musicians had regular access to technologies in the lesson space vs. practice space.
For four specific music technologies (metronomes, tuners, audio recorders, and video recorders) musicians were asked whether they primarily use these functionalities on a separate device, on their phone, or not at all (see Table 3). For all four devices, the majority of technology use was on a smartphone as opposed to a stand-alone device.
The next two questions examined drivers of and barriers preventing adoption of technology use in learning musical instruments, adapting scales by Gilbert (2015). For each set, a repeated measures ANOVA was conducted (with item as the independent variable and response as the dependent variable) with the questions rank-ordered and followed by a repeated contrast. A significant main effect was found among drivers to technology [F(6.23, 4136.25) = 89.83, p < 0.001, η2 = 0.24] where the strongest drivers of technology use were that it is useful, available, helps accomplish goals, and is easy to use, while the weakest included whether it was inexpensive and if its use is required. For barriers to technology use, a significant main effect was again found [F(5.60, 1560.48) = 38.88, p < 0.001, η2 = 0.12], with the strongest barriers being the lack of requirement and too high a cost (see Table 4). Results also demonstrated that, when compared as combined means, overall responses to positive uses of technology (M = 4.55, SD = 1.26) were significantly higher than negative uses [M = 3.16, SD = 1.27; t(285) = 12.55, p < 0.001, d = 0.82] and these two values were not significantly correlated.
As employed for general technology use, Davis's (1989) scales were adapted for use of technology in learning musical instruments. The six-item scales again showed very high internal reliability (α > 0.90), with moderately high mean scores for the perceived usefulness (M = 5.35, SD = 1.20) and ease of use (M = 5.62, SD = 1.12) of their day-to-day technologies, comparable to the scores for general use reported above (usefulness M = 5.72, SD = 0.97; ease of use M = 5.74, SD = 0.88). To examine differences between attitudes toward general and music-learning-specific technology use, a 2-way repeated measures ANOVA was conducted with construct (usefulness vs. ease of use) and application (general use vs. music-learning-specific) as independent factors. A small significant main effect of construct was found [F(1, 249) = 6.95, p < 0.01, η2 = 0.03], in which ease of use received slightly higher ratings than usefulness across applications. A small significant main effect of application was also found [F(1, 249) = 15.43, p < 0.001, η2 = 0.06], in which ease of use and usefulness received slightly higher ratings in general technology use than in music-specific cases. Finally, a significant interaction was found [F(1, 249) = 11.17, p < 0.001, η2 = 0.04] in which the construct difference was larger, although still relatively minimal, in the music-learning application vs. general use: a mean difference of 0.27 points vs. 0.02, respectively. Thus, attitudes toward technology were relatively stable across general and music-learning-specific applications, with the perceived usefulness of technology falling slightly when learning musical instruments.
Musicians were then asked where they use technology in music learning on a 7-point scale from always to never across seven skill development categories: technical, musical, ensemble, practice, presentation, career, and life. A repeated measures ANOVA (with item as the independent variable and response as the dependent variable) showed a significant effect of skill [F(4.85, 1120.67) = 10.71, p < 0.001, η2 = 0.04], and a repeated contrast (with the items in reverse rank order) showed four significantly different groupings (see Table 5 and Figure 3). Career skills (e.g., networking, budgeting, advertising) showed the highest score, and was significantly higher than the pairing of musical and technical skills, which had no significant difference between them. This was significantly higher than the grouping of practice, ensemble, and life (e.g., mental and physical health, nutrition) skills. Presentation skills (i.e., stage presentation) scored the lowest, significantly lower than life skills.
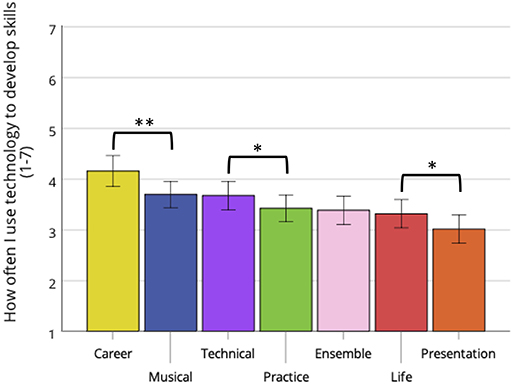
Figure 3. Self-reported use of technology to develop performance skills. Musicians reported the highest skills in career development, followed by developing musical and technical skills, then by practice, ensemble, and life skills, with presentation the lowest. Error bars show 95% CI. 1 = never, 7 = always; *p < 0.05, **p < 0.01, as determined by a repeated measures ANOVA using a repeated contrast.
The categories of musical, technical, and practice skills comprised a series of sub-skills. A repeated measures ANOVA was employed (with item as the independent variable and response as the dependent variable) with sub-skills included among the overall rankings followed a deviation contrast in which every skill subset was compared with the grand mean of all skills combined. As a deviation contrast does not compare the first- (or last-) entered variable, the overall practice skill score was placed in first position as it was the category closest to the mean score of the seven skill categories (i.e., 3.53). A significant main effect was found [F(13.51, 3121.44) = 26.73, p < 0.001, η2 = 0.10] and contrast results were sorted by descending effect size to determine the skills with the largest significant deviations (see Figure 4). The largest difference was the practice skill of avoiding injury, which had the overall lowest score [M = 1.89, SD = 1.74; F(1, 231) = 216.55, p < 0.001, η2 = 0.48]. This was followed by the musical skill of rhythmic accuracy, which showed the highest overall score [M = 4.42, SD = 1.99; F(1, 231) = 106.63, p < 0.001, η2 = 0.32]. Next were low scores among the technical skills for handling the instrument [M = 2.22, SD = 2.04; F(1, 231) = 78.01, p < 0.001, η2 = 0.25] and posture [M = 2.37, SD = 1.91; F(1, 231) = 62.97, p < 0.001, η2 = 0.21]. The practice skill of reviewing feedback followed with a score below the midpoint [M = 2.56, SD = 2.17; F(1, 231) = 34.20, p < 0.001, η2 = 0.13], and the low score for the technical skill of timbre (M = 2.84, SD = 2.14) showed the smallest deviation effect to still reach statistical significance among the subskills [F(1, 231) = 8.14, p < 0.005, η2 = 0.03].
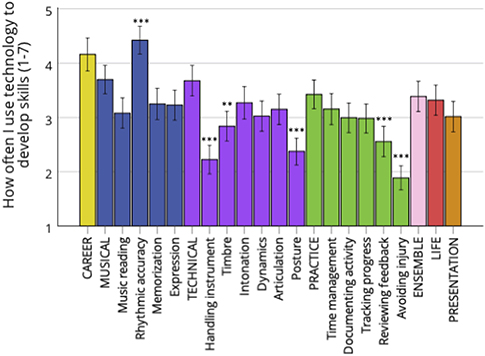
Figure 4. Self-reported use of technology to develop performance skills, including subskills. The highest technology use was for the musical skill (blue) of rhythmic accuracy, and the lowest use was for the technical skills of handling the instrument, posture, and developing timbre, and the practice skills of avoiding injury and reviewing feedback. Error bars show 95% CI. 1 = never, 7 = always; **p < 0.01, ***p < 0.001, representing deviation from the overall midpoint as determined by a repeated measures ANOVA using a deviation contrast.
The next section examined the frequency with which the musicians engage in technology-driven activities, namely keeping records of time spent practicing, having distance lessons over video, and various forms of performance recording and viewing (see Table 6). For documenting practice time, a Wilcoxon signed-rank test showed that significantly more documentation occurred without technology than with (i.e., handwritten notes; T = 3459.50, p < 0.05, r = 0.13; effect size r calculated following Rosenthal, 1991, showing a small effect), where 64% of musicians did not ever engage with technology-driven notes and 6% did so on at least a daily basis, while for traditional means 60% never used them and 14% engaged at least daily. While there was of course some overlap between the two paradigms (i.e., many that reported never using one method did engage with the other), further examination of the data showed that 46% of musicians reported never for documentation both with and without technology, and no more than 20% of musicians reported keeping a daily record with either means. The technological activity with the least engagement was lessons over video, with only one fifth of musicians engaging with the practice at least once per year and only 7% having such lessons at least weekly. Four types of audio/video recording activities were documented; recording and viewing recordings of both one's own and others' performances. Friedman's ANOVA, followed by pairwise comparisons (with effect sizes calculated via Wilcoxon signed-rank tests) was used to examine frequency differences. A significant main effect was found [χ = 165.07, p < 0.001], and pairwise tests showed that the musicians recorded their own playing with relatively similar frequency (i.e., not significantly different) than the degree to which they viewed the recordings of others. However, they recorded themselves significantly more often than they viewed those same recordings (T = 612.50, p < 0.001, r = 0.30), the latter of which was done with more frequency than the degree to which they recorded others' playing (T = 3014.50, p < 0.001, r = 0.22).
Use of Technology in Music Teaching
A short section was completed by musicians who reported themselves as active music teachers (n = 82), teaching a mean 20.88 students (SD = 27.53; Mdn = 11.50, IQR = 26) at the time of completing the survey. The first set of questions investigated how teachers' general attitudes toward and use of technology in their roles as teachers compared with their feelings as music learners (see Table 7). Teachers were generally more receptive to technology in their roles as teachers, being more likely to report increased use, willingness to try, usefulness of, and potential future usefulness of technology than to report decreased attitudes. The only reversal was that of time to try new technologies, where there was a tendency to report the same or less time as a teacher than as a musician.
Music teachers were then asked the degree to which they used technology in a series of teaching-specific activities. A repeated measures ANOVA showed a significant effect of skill [F(3.74, 303.21) = 34.35, p < 0.001, η2 = 0.30], and a repeated contrast (with the items in reverse rank order) showed four significantly different groupings (see Table 8 and Figure 5). Scheduling lessons showed the highest score, which was significantly higher than advertising for new students. Advertising was in turn significantly higher than giving students feedback, which was grouped with organizing students' practice time and tracking students' progress without significant differences between them. The lowest, reported significantly less often than tracking progress, was tracking students' practice time.
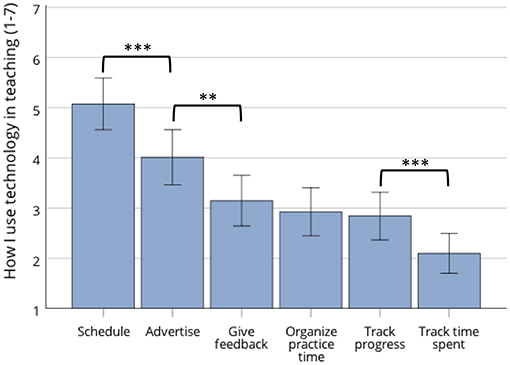
Figure 5. Self-reported use of technology for music teaching activities. The highest technology use was for scheduling lessons and advertising, while the lowest were giving feedback, tracking progress, and organizing and tracking students' time spent practicing. Error bars show 95% CI. 1 = never, 7 = always; **p < 0.01, ***p < 0.001, as determined by a repeated measures ANOVA using a repeated contrast.
Attitudes Toward and Predictors of Future Technology Use
The questions on skills developed using current technologies were repeated with reference to the potential usefulness of future technologies in addressing the same seven categories (see Figure 6). A repeated measures ANOVA (with item as the independent variable and response as the dependent variable) showed a significant effect of skill [F(4.64, 974.60) = 10.30, p < 0.001, η2 = 0.05], and a repeated contrast (with the items in reverse rank order) showed a relatively even ranking of categories with career skills receiving the highest score (M = 5.36, SD = 2.05) as it did in current technology use, and showing the only significant difference between the next highest rank [F(1, 210) = 25.55, p < 0.001, η2 = 0.11] with no significant differences between the remaining six skill categories. In comparing future technology usefulness with current use, a 2-way repeated measures ANOVA with skill category and paradigm (current use vs. future usefulness) as factors was conducted. The main effect of skill category was repeated [F(4.49, 943.15) = 12.78, p < 0.001, η2 = 0.06], and a significant main effect was found for paradigm in which musicians rated the potential usefulness of technology for each of the skill categories (combined M = 4.70, SD = 1.48) approximately one point higher than their current use [combined M = 3.53, SD = 1.48; F(1.00, 210.00) = 168.66, p < 0.001, η2 = 0.45]. The interaction was also significant [F(5.23, 1097.78) = 6.43, p < 0.001, η2 = 0.03], unsurprising due to the changing rank orders between the two paradigms.
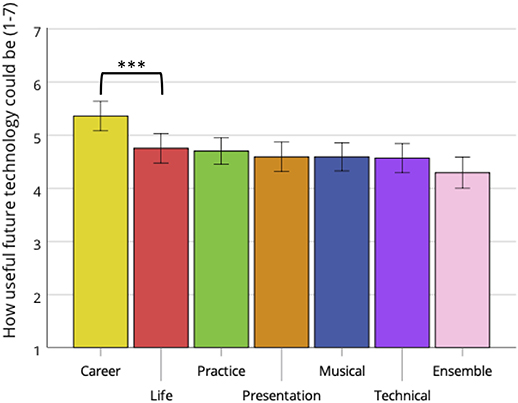
Figure 6. Perceived usefulness of future technologies for developing performers' skills. As with current technology use, musicians gave the highest scoring for career development skills. The remaining categories did not show significant differences between them. Error bars show 95% CI. 1 = not at all, 7 = very; ***p < 0.001 as measured by a repeated measures ANOVA using a repeated contrast.
An adapted form of the Technology Acceptance Model (Davis, 1989; see Figure 1) was constructed and tested using PLS-SEM to examine predictors of perceived future technology use (see Figure 7). Five latent variables were included. The first two—(1) perceived ease of use and (2) usefulness of technologies for music learning—were used directly from the TAM using the six-component scales described above. To this was added two latent variables—(3) musicians' current use of music technology and (4) perceived usefulness of potential future technologies to develop music-performance related skills—each aggregated from the seven skill categories as reported above. These were hypothesized to be predictive of the final item, (5) the degree to which musicians intend to use future technologies, comprising a 7-point scale in which musicians were asked whether they would use more technology for music learning were it available (see question 19 in the Supplementary Table) and three questions where musicians were asked how likely they would be to use three hypothetical technologies devised by the authors to capture respondent's reactions to specific potential technologies in addition to the general hypothetical (see questions 25–27 in the Supplementary Table). Responses to these questions of hypothetical future use were moderately high (M = 4.70, SD = 1.93; M = 4.81, SD = 1.86; M = 4.36, SD = 2.01; M = 4.48, SD = 2.08, respectively), and a mixed repeated measures ANOVA with the four questions as a repeated factor and sex as a between groups factor (added to the model due to the finding, described above, of men reporting a higher tendency to seek out new technologies) showed no significant main effects of or interaction between the two factors. Figure 7 shows the construction of the model, to which musicians' age and musical experience were added as direct predictors of the future use of technologies for music learning. Missing data were excluded casewise, leaving n = 207 for this analysis. While partial least squares structural equation modeling, unlike covariance-based SEM approaches, do not have available a global goodness of fit measure (Garson, 2016), the standardized root mean square residual (SRMR) was below the 0.08 cutoff considered to be a conservative indicator that the average magnitude of differences between the observed and model-implied correlation matrices was lower and indicative of good fit (Hu and Bentler, 1998). R2 values for each dependent variable were significant (p < 0.001), and significant path relationships (β) were demonstrated (see Figure 7). Perceived ease of use had a significant effect on perceived usefulness (β = 0.62, p < 0.001), accounting for 38% of variance (p < 0.001). As predictors of current use of technology, only usefulness showed a significant effect (β = 0.46, p < 0.001) with 21% of variance accounted for. The perceived usefulness of future technologies was significantly predicted with a small effect by usefulness of current technologies (β = 0.15, p < 0.05), although current technology use was a much stronger predictor (β = 0.56, p < 0.001) with a combined 41% of variance explained. Finally, intention to use future hypothetical technologies was tested with five predictors for a combined 35% of variance accounted for. The strongest significant predictor was the perceived potential usefulness of future music technologies (β = 0.32, p < 0.001), followed closely by perceived usefulness of current technologies (β = 0.31, p < 0.001). Musicians' current technology use, age, and musical experience did not significantly predict their intention to use future technologies.
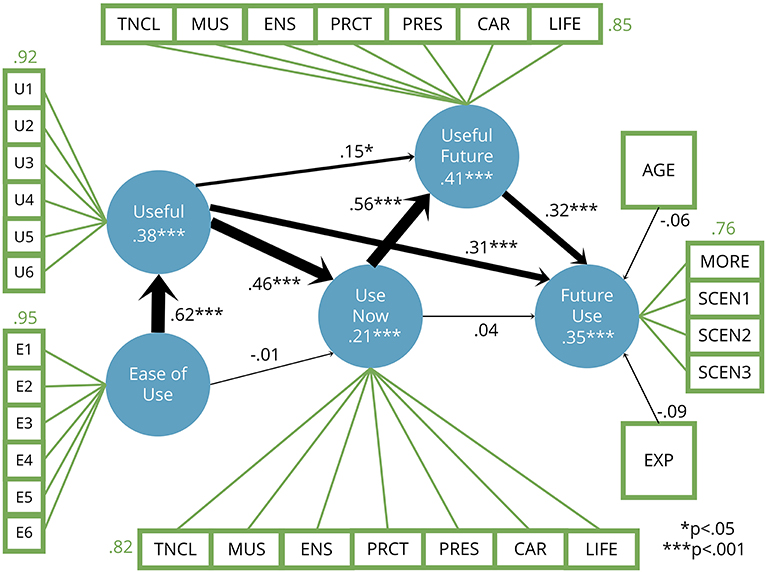
Figure 7. Adapted Technology Acceptance Model for current and potential future use of technologies for music learning. The model was tested using partial least squares structural equation modeling (missing data excluded casewise, n = 207). Blue circles show latent variables and contain R2 values; green squares show directly measured variables and, when forming composite latent variables, are accompanied by alpha values (Cronbach's) in green. Path arrow thickness indicates relative weight and is accompanied by β values that represent standardized regression coefficients resulting from the Partial Least Squares analysis, demonstrating the relative weighting of each path on a scale from −1 to 1. Significance values of R2 and β were calculated using 500-sample bootstrapping (bias-corrected and accelerated; [BCa]). Current use of technology was predicted by perceived technology usefulness but not ease of use, which itself predicted usefulness. Future use of technology was predicted by perceived current and future music technology usefulness, but not by current technology use, age, or musical experience. TNCL, Technical; MUS, Musical; ENS, Ensemble; PRCT, Practice; PRES, Presentation; CAR, Career. *p < 0.05, ***p < 0.001.
Discussion
This research examined the use of and attitudes toward technology in musicians' individual learning and teaching, investigating their current use of technology in day-to-day life and in their learning, as well as opinions toward future hypothetical technologies. Across the survey, musicians were shown to be generally positive in their attitudes toward technology, active in their use, and optimistic regarding future possibilities, although notable deficits remain.
In their day-to-day lives, musicians were most skilled at using smartphones, laptops, and desktop computers to a significantly greater degree than audio and video recording devices, and playback equipment. While this is perhaps not surprising due to the relatively recent surge of such technologies in the personal sphere, it signifies that musicians are less confident with the audio and video recording devices that might be considered central to the practice of musical learning and training. Such a shift toward mobile devices and computers was also seen in the increased accessibility of these devices in the practice and lesson space compared with audio/video recording and playback equipment, as well as the fact that the majority of musicians were found to be engaging with the “classic” music technologies of metronomes, tuners, and audio/video recording functions on their smartphones as opposed to bespoke equipment. It is notable that significant differences in sex and age were not found for mobile devices and computers, suggesting the new universality of these devices that transcend stereotypical barriers. While the means reported suggested slightly higher increases in confidence with some of the lower-rated technologies (i.e., audio/video recording playback and motion capture), as well as a greater tendency to seek out and enjoy the search for new technologies, it may indicate that the established trend of men (and boys) showing greater confidence with music technologies (e.g., Colley et al., 1997) is waning with the explosion of personal computing and smartphones and the emergence of a new generation of digital learners (Sánchez et al., 2011). The sex differences may also have resulted from an effect of stereotype threat, in which women may have been more likely to self-report lower engagement with technology resulting from cultural assumptions of women's relationship with STEM-related subjects (Stout et al., 2011). The musician's toolkit is evolving; pedagogues, teachers, institutions, and technology creators must work to keep up. That the accessibility of every technology here investigated halved when moving from the practice to the teaching space suggests that these tools have not yet found their place in one-to-one learning settings, mirroring the continued (but shrinking) underuse of technology in classrooms, particularly for formative assessment (Fautley, 2013) and distance learning (Kruse et al., 2013). It was promising that music teachers in this study tended to report higher use of technology as teachers than as learners, although the majority of this activity was used outside of the lesson space, such as lesson scheduling and advertising.
Regarding use of technology for music learning, musicians were found to rate the drivers to new technology significantly higher than barriers preventing them from using it. The highest rated drivers included usefulness, availability, ability to accomplish goals, and ease of use. The lowest included whether the technologies were time saving, inexpensive, and required for use. The strongest barriers were whether it was not required, its expense, and its availability, with the lowest being knowledge of use, difficulty of use, and whether support was available. This somewhat contradicts findings within the general music education classroom, where Gall (2013) found lack of availability, technical competence, and staff support to be the largest barriers to technology adoption, and Gilbert (2015) found it to be a lack of time, especially in difficulty to set-up. Further research should examine explicitly differences in attitudes between individual and classroom-based music learning paradigms. In examining which music-related skills are developing using these technologies, the highest skill category was for career development, including networking, budgeting, and advertising. The pronounced role of social media may in part account for this, as well as a wealth of online resources aimed at managing and maintaining a freelance career. The skill groups that followed—musical, technical, and practice-based—were marked by notable deviations of certain subskills. That the musical skill of rhythmic accuracy scored the highest in technology use is perhaps unsurprising due to the prevalence of metronomes. The low scores for the skills of instrument handling, maintaining good posture, and avoiding injury all speak to the physical aspects of technology, an area that could see further growth soon due to the increasing development of optical and wearable sensors for music performance and corresponding experimental pedagogical applications (e.g., Ng et al., 2007; Van der Linden et al., 2009; Johnson et al., 2010; Volpe et al., 2017). The low score for the skill of good tone or timbre may also speak to the complexity of the construct and a lack of market-ready technologies to analyze and develop this skill, although new strides are being made in this area (Himonides, 2009; Giraldo et al., 2017, 2019).
The lower score for the subskill of reviewing feedback highlights a gap in the use of technology to aid in self-directed learning and practice. As it provides tools that can be used to help plan, monitor, and review one's performance, technology has great potential to improve the efficacy of learning by harnessing the principles of deliberate practice through self-regulated learning, which calls for a cycle of explicit planning, deliberate execution, and thoughtful evaluation of one's practice that cycles back into preparing for the next practice session (Zimmerman, 1990; Jørgensen, 2004; Ritchie and Williamon, 2013; Hatfield et al., 2016; Williamon et al., 2017). Unfortunately, these results suggest that significant gaps remain in this learning cycle among musicians. Nearly half of musicians reported not keeping any kind of record of their activities in the practice room, with or without technology-enhanced means, with fewer than a fifth of musicians undertaking this daily. While approximately half of the sampled musicians reported recording their own playing at least once weekly, these recordings were reviewed with significantly lower frequency. They also engaged with the recordings of others more than they did with their own, supporting previous research highlighting the important and varied role such activity can play in developing an interpretative style, particularly in the early stages of practice (Volioti and Williamon, 2017). While the act of making the recording alone may to some degree simulate the pressure of a performance situation, and thus trigger the physiological and psychological arousal that can accompany mock performances (Williamon et al., 2014), without reviewing the recordings musicians do not experience the positive effects this can have on the act of self-assessment (Silveira and Gavin, 2016).
The results from the structural equation modeling support Davis (1989); Davis et al. (1989) Technology Acceptance Model, where we found that current use of technology for music learning was predicted by perceived usefulness and ease of use. In this case, however, ease of use was not found to be a direct predictor of active technology use; instead, it was shown to predict the perceived usefulness of technology which then predicted use. This result replicates previous findings in educational video games (Martí-Parreño et al., 2016) and suggests that ease of use on its own is not enough to drive use in music learning situations. The technology must be perceived as being useful, although by being easier to use these tools may be giving users a greater chance to recognize and appreciate their utility. The TAM was not initially designed for speculation on hypothetical future use of technology, thus no hypotheses were drawn on the predictors of future intentions. In this case, current technology use, age, and musical experience had no predictive power on attitudes toward using future technologies, with the significant predictors being perceived current usefulness and hypothetical future usefulness. On the one hand, this is promising; there appear to be few barriers to technology uptake beyond making sure they fulfill a need for musicians. On the other, behavioral intention often falls short of actual behavior, functioning more as a moderator than a direct predictor (Armitage and Christian, 2003; Sniehotta et al., 2014). The true test of attitudes toward future technologies will be how they are perceived and used when ultimately presented.
While the study was able to reach an international cohort varying in age, experience, and nationality, generalizability of this study is limited by the nature of the convenience sampling used. In particular, participants had to engage with technology (i.e., emails, social media, internet browsers, etc.) in order to complete a survey on the use of technology. However, the near ubiquitous access to the internet and use of email in the target population minimizes the risk that major subgroups were excluded. Future research should expand on these findings by exploring deeper the reasons for and processes by which musicians choose the technology they use and the innovative ways by which they are incorporating them into their pedagogy. This work could also examine the degree to which musicians continue to use technology once it has been adapted, as continued satisfaction with technology and the potential negative effects of overhyped and under-delivered features have been shown to be powerful drivers of and barriers to continued technology engagement (Bhattacherjee and Premkumar, 2004).
Conclusions
The traditional models of learning music can give the impression of a rarefied culture resistant to change. The present study suggests that this is not the case. We found that technology use is being actively pursued and demanded by a population of musicians with a high degree of technological aptitude, one that particularly favors mobile devices over bespoke equipment to record audio and video or to set metronomic time. Technology, in addition to its role as a tool to network and communicate, is being used to enhance the development of technical and musical skills. However, gaps remain in technology use, particularly for aspects relating to kinematics such as posture and the avoidance of injury. Music teachers are making use of technologies to communicate with and organize time with their students, though more research is required to reveal how technology is being employed within the teaching studio and what innovations may be possible therein. New technologies, through advanced and interactive systems of behavioral analysis and feedback, have the potential to enhance communication, efficiency, efficacy, and healthy practice in music learning. By understanding the challenges faced and attitudes held by musicians that may be impeding the take-up of such systems, researchers and designers will be able to develop genuinely useful technologies for the next generation of performers, teachers will be able to enhance the feedback they can give in their classrooms and studios, and musicians will be able to expand their toolkit to build the full range of skills required for their art and their careers.
Ethics Statement
Ethical approval for the study, including approval of the study protocol, was granted by the Conservatoires UK Research Ethics Committee following the guidelines of the British Psychological Society. The survey opened with an information sheet outlining the topic and purpose of the study and informing respondents that, by beginning the survey, they were providing informed consent. Written consent was not explicity requested following the guidelines of the British Psychological Society for questionnaire-based research examining non-vulnerable populations (aged 16+).
Author Contributions
Both authors contributed significantly to the study design, data collection and analysis, and preparation of this manuscript.
Funding
The research reported in this article was supported by TELMI (Technology Enhanced Learning of Musical Instrument Performance), a project funded by the European Commission's Horizon 2020 research and innovation program (grant no. 688269).
Conflict of Interest Statement
The authors declare that the research was conducted in the absence of any commercial or financial relationships that could be construed as a potential conflict of interest.
Acknowledgments
We would like to thank Rafael Ramirez, Alfonso Pérez, Gualtiero Volpe, Manuel Oliveira, Maria Margoudi, Madeleine Mitchell, and the TELMI team for their contributions to the survey design and dissemination, as well as the Associated Board of the Royal Schools of Music, British Association for Performing Arts Medicine, Brent Music Service, European String Teacher's Association, Musician's Union, and the Royal College of Music for their aid in distributing the survey.
Supplementary Material
The Supplementary Material for this article can be found online at: https://www.frontiersin.org/articles/10.3389/fict.2019.00011/full#supplementary-material
References
Adams, D. A., Nelson, R. R., and Todd, P. A. (1992). Perceived usefulness, ease of use, and usage of information technology: a replication. MIS Quart. 16, 227–247. doi: 10.2307/249577
Anderson, A. J., and Ellis, A. (2005). Desktop video assisted music teaching and learning: new opportunities for design and delivery. Br. J. Educ. Technol. 36, 915–917. doi: 10.1111/j.1467-8535.2005.00496.x
Armitage, C. J., and Christian, J. (2003). From attitudes to behaviour: basic and applied research on the theory of planned behaviour. J. Curr. Psychol. 22, 187–195. doi: 10.1007/s12144-003-1015-5
Barry, N. H., and Mcarthur, V. (1994). Teaching practice strategies in the music studio: a survey of applied music teachers. Psychol. Music 22, 44–55. doi: 10.1177/0305735694221004
Bauer, W. I., Reese, S., and McAllister, P. A. (2003). Transforming music teaching via technology: the role of professional development. J. Res. Music Educ. 51, 289–301. doi: 10.2307/3345656
Bhattacherjee, A., and Premkumar, G. (2004). Understanding changes in belief and attitude toward information technology usage: a theoretical model and longitudinal test. MIS Quart. 34, 229–254. doi: 10.2307/25148634
Bray, D. (1997). CD ROM in music education. Brit. J. Mus. Ed. 14:137. doi: 10.1017/S0265051700003582
Colley, A., Comber, C., and Hargreaves, D. (1997). IT and music education: what happens to boys and girls in coeducational and single sex schools. Brit. J. Mus. Ed. 14:119. doi: 10.1017/S0265051700003569
Creech, A., and Gaunt, H. (2012). “The changing face of individual instrumental tuition: value, purpose and potential,” in The Oxford Handbook of Music Education, Vol. 1, eds G. E. McPherson and G. F. Welch (Oxford: Oxford University Press), 694–711. doi: 10.1093/oxfordhb/9780199730810.013.0042
Daniel, R. (2001). Self assessment in performance. Br. J. Music Educ. 18, 215–226. doi: 10.1017/S0265051701000316
Davis, F. D. (1989). Perceived usefulness, perceived ease of use, and user acceptance of information technology. MIS Quart. 1989, 319–340. doi: 10.2307/249008
Davis, F. D., Bagozzi, R. P., and Warshaw, P. R. (1989). User acceptance of computer technology: a comparison of two theoretical models. Manag. Sci. 35, 982–1003. doi: 10.1287/mnsc.35.8.982
DfES Department for Education and Skills. (2005). Support for Parents: The Best Start for Children. London: Stationery Office.
Edmunds, R., Thorpe, M., and Conole, G. (2012). Student attitudes towards and use of ICT in course study, work and social activity: a technology acceptance model approach: exploring student perceptions of ICT in three contexts. Br. J. Educ. Technol. 43, 71–84. doi: 10.1111/j.1467-8535.2010.01142.x
Ellis, P. (1997). The Music of Sound: a new approach for children with severe and profound and multiple learning difficulties. Brit. J. Mus. Ed. 14:173. doi: 10.1017/S0265051700003624
European Commission (2013). Survey of Schools: ICT in Education. Luxembourg: Publications Office of the European Union.
Fautley, M. (2013). The potential of audio and video for formative assessment purposes in music education in the lower secondary school in England: issues arising from a small-scale study of trainee music teachers. J. Music Technol. Educ. 6, 29–42. doi: 10.1386/jmte.6.1.29_1
Gall, M. (2013). Trainee teachers' perceptions: factors that constrain the use of music technology in teaching placements. J. Music Technol. Educ. 6, 5–27. doi: 10.1386/jmte.6.1.5_1
Garson, G. D. (2016). Partial Least Squares: Regression and Structural Equation Models. Statistical Associates Blue Book Series. Asheboro, NC: Statistical Associates Publishing.
Gaunt, H. (2017). “Apprenticeship and empowerment: the role of one-to-one lessons,” in Musicians in the Making: Pathways to Creative Performance, eds J. Rink, H. Gaunt, and A. Williamon (Oxford: Oxford University Press), 28–56.
Gilbert, A. D. (2015). An Exploration of the Use of and the Attitudes Toward Technology in First-Year Instrumental Music. (Unpublished PhD thesis), University of Nebraska.
Giraldo, S., Ramirez, R., Waddell, G., and Williamon, A. (2017). “A real-time feedback learning tool to visualize sound quality in violin performances,” in Proceedings of the 10th International Workshop on Machine Learning and Music, eds. R. Ramirez, D. Conklin, and J. M. Iñesta (Barcelona), 19–24.
Giraldo, S., Waddell, G., Nou, I., Ortega, A., Mayor, O., Perez, A., et al. (2019). Automatic assessment of tone quality in violin music performance. Front. Psychol. 10:334. doi: 10.3389/fpsyg.2019.00334
Hallam, S. (1995). Professional musicians' orientations to practice: implications for teaching. Br. J. Music Educ. 12, 3–19. doi: 10.1017/S0265051700002357
Hatfield, J. L., Halvari, H., and Lemyre, P.-N. (2016). Instrumental practice in the contemporary music academy: a three-phase cycle of Self-Regulated Learning in music students. Music. Sci. 2016, 316–337. doi: 10.1177/1029864916658342
Henley, D. (2011). Music Education in England: A Review by Darren Henley for the Department for Education and the Department for Culture, Media and Sport. Available online at: https://www.gov.uk/government/publications/music-education-in-england-a-review-by-darren-henley-for-the-department-for-education-and-the-department-for-culture-media-and-sport
Hewitt, M. P. (2002). Self-evaluation tendencies of junior high instrumentalists. J. Res. Music Educ. 50, 215–226. doi: 10.2307/3345799
Himonides, E. (2009). Mapping a beautiful voice: theoretical considerations. J. Music Technol. Educ. 2, 25–54. doi: 10.1386/jmte.2.1.25/1
Himonides, E., and Purves, R. (2010). “The role of technology,” in Music Education in the 21st Century in the United Kingdom: Achievements, Analysis and Aspirations, eds S. Hallam and A. Creech (London, Institute of Education), 123–140.
Ho, W. C. (2004). Use of information technology and music learning in the search for quality education. Br. J. Educ. Technol. 35, 57–67. doi: 10.1111/j.1467-8535.2004.00368.x
Hu, L.- T., and Bentler, P. M. (1998). Fit indices in covariance structure modeling: sensitivity to underparameterized model misspecification. Psychol. Methods 3:424. doi: 10.1037/1082-989X.3.4.424
Hugill, A. (2012). The Digital Musician, 2nd Ed. New York, NY: Routledge. doi: 10.4324/9780203111796
Hunt, A., and Kirk, R. (1997). Technology and music: incompatible subjects. Brit. J. Mus. Ed. 14:151. doi: 10.1017/S0265051700003600
Johnson, R. M., van der Linden, J., and Rogers, Y. (2010). “MusicJacket: the efficacy of real-time vibrotactile feedback for learning to play the violin,” in CHI'10 Extended Abstracts on Human Factors in Computing Systems (Atlanta: ACM), 3475–3480. doi: 10.1145/1753846.1754004
Johnston, H. (1993). The use of video self-assessment, peer-assessment, and instructor feedback in evaluating conducting skills in music student teachers. Br. J. Music Educ. 10:57. doi: 10.1017/S0265051700001431
Jørgensen, H. (2004). “Strategies for individual,” in Musical Excellence: Strategies and Techniques to Enhance Performance, ed A. Williamon (Oxford: Oxford University Press), 85–104. doi: 10.1093/acprof:oso/9780198525356.003.0005
Kenny, R. F., and McDaniel, R. (2011). The role teachers' expectations and value assessments of video games play in their adopting and integrating them into their classrooms: expectancy-value. Br. J. Educ. Technol. 42, 197–213. doi: 10.1111/j.1467-8535.2009.01007.x
Kruse, N. B., Harlos, S. C., Callahan, R. M., and Herring, M. L. (2013). Skype music lessons in the academy: intersections of music education, applied music and technology. J. Music Technol. Educ. 6, 43–60. doi: 10.1386/jmte.6.1.43_1
Lindström, E., Juslin, P. N., Bresin, R., and Williamon, A. (2003). “Expressivity comes from within your soul”: a questionnaire study of music students' perspectives on expressivity. Res. Stud. Music Educ. 20, 23–47. doi: 10.1177/1321103X030200010201
Martí-Parreño, J., Sánchez-Mena, A., and Aldás-Manzano, J. (2016). “Teachers' intention to use educational video games: a technology acceptance model approach,” in Proceedings of the 10th European Conference on Games Based Learning (Reading, UK), 434–441.
Mills, J., and Murray, A. (2000). Music technology inspected: good teaching in key stage 3. Br. J. Music Educ. 17, 129–156. doi: 10.1017/S026505170000022X
Naughton, C. (1997). Music technology tools and the implications of socio-cognitive research. Brit. J. Mus. Ed. 14:111. doi: 10.1017/S0265051700003557
Ng, K. C., Weyde, T., Larkin, O., Neubarth, K., Koerselman, T., and Ong, B. (2007). “The 3D augmented mirror: a multimodal interface for learning and teaching string instruments,” in ICMI '07 Proceedings of the 9th International Conference on Multimodal Interfaces (New York, NY), 339–345. doi: 10.1145/1322192.1322252
Pike, P. D., and Shoemaker, K. (2013). The effect of distance learning on acquisition of piano sight-reading skills. J. Music Technol. Educ. 6, 147–162. doi: 10.1386/jmte.6.2.147_1
Purves, R. (2012). “Technology and the educator,” in The Oxford Handbook of Music Education, Vol. 2, eds G.E. McPherson and G. F. Welch (Oxford: Oxford University Press), 457–475. doi: 10.1093/oxfordhb/9780199928019.013.0030
Ringle, C. M., Wende, S., and Becker, J.-M. (2015). “SmartPLS 3.” Boenningstedt: SmartPLS GmbH. Available online at: http://www.smartpls.com.
Ritchie, L., and Williamon, A. (2013). Measuring musical self-regulation: linking processes, skills, and beliefs. J. Educ. Training Stud. 1, 106–117. doi: 10.11114/jets.v1i1.81
Robinson, C. R. (1993). Singers' self-assessment of choral performance: next-day recollections versus concert tape evaluation. Southeastern J. Music Educ. 4, 224–233.
Rogers, K. (1997). Resourcing music technology in secondary schools. Br. J. Music Educ. 14:129. doi: 10.1017/S0265051700003570
Rosenthal, R. (1991). Meta-Analytic Procedures for Social Research 2nd Ed. Newbury Park, CA: Sage. doi: 10.4135/9781412984997
Salaman, W. (1997). Keyboards in schools. Br. J. Music Educ. 14, 143–149. doi: 10.1017/S0265051700003594
Sánchez, J., Salinas, A., Contreras, D., and Meyer, E. (2011). Does the new digital generation of learners exist? A qualitative study. Br. J. Educ. Technol. 42, 543–556. doi: 10.1111/j.1467-8535.2010.01069.x
Silveira, J. M., and Gavin, R. (2016). The effect of audio recording and playback on self-assessment among middle school instrumental music students. Psychol. Music 44, 880–892. doi: 10.1177/0305735615596375
Smart, T., and Green, L. (2017). “Informal learning and musical performance,” in Musicians in the Making: Pathways to Creative Performance, eds J. Rink, H. Gaunt, and A. Williamon (Oxford: Oxford University Press), 108–125.
Sniehotta, F. F., Presseau, J., and Araújo-Soares, V. (2014). Time to retire the theory of planned behaviour. Health Psychol. Rev. 8, 1–7. doi: 10.1080/17437199.2013.869710
Stout, J. G., Dasgupta, N., Hunsinger, M., and McManus, M. A. (2011). STEMing the tide: using ingroup experts to inoculate women's self-concept in science, technology, engineering, and mathematics (STEM). J. Pers. Soc. Psychol. 100, 255–270. doi: 10.1037/a0021385
Sweeney, T., West, D., Groessler, A., Haynie, A., Higgs, B. M., Macaulay, J., et al. (2017). Where's the transformation? Unlocking the potential of technology-enhanced assessment. TLI 5, 1–13. doi: 10.20343/5.1.5
Thorgersen, K., and Zandén, O. (2014). The internet as teacher. J. Music Technol. Educ. 7, 233–244. doi: 10.1386/jmte.7.2.233_1
Van der Linden, J., Schoonderwaldt, E., and Bird, J. (2009). “Towards a real-time system for teaching novices correct violin bowing technique,” in Proceedings of HAVE, the IEEE International Workshop on Haptic Audio Visual Environments and Games (Lecco). doi: 10.1109/HAVE.2009.5356123
Venkatesh, V., Morris, M. G., Davis, G. B., and Davis, F. D. (2003). User acceptance of information technology: toward a unified view. MIS Quart. 27, 425–478. doi: 10.2307/30036540
Volioti, G., and Williamon, A. (2017). Recordings as learning and practising resources for performance: exploring attitudes and behaviours of music students and professionals. Music. Sci. 21, 499–523. doi: 10.1177/1029864916674048
Volpe, G., Kolykhalova, K., Volta, E., Ghisio, S., Waddell, G., Alborno, P., et al. (2017). “A multimodal corpus for technology enhanced learning of violin playing,” in Proceedings of the 12th Biannual Conference of the Italian SIGCHI Chapter, eds F. Paternò and L. D. Spano (New York, NY: ACM Publications). doi: 10.1145/3125571.3125588
Waldron, J. (2012). Conceptual frameworks, theoretical models and the role of YouTube: Investigating informal music learning and teaching in online music community. J. Music Technol. Educ. 4, 189–200. doi: 10.1386/jmte.4.2-3.189_1
Webster, P. R. (2012). Key research in music technology and music teaching and learning. J. Music Technol. Educ. 4, 115–130. doi: 10.1386/jmte.4.2-3.115_1
Williamon, A., Aufegger, L., and Eiholzer, H. (2014). Simulating and stimulating performance: introducing distributed simulation to enhance musical learning and performance. Front. Psychol. 5:25. doi: 10.3389/fpsyg.2014.00025
Williamon, A., Clark, T., and Küssner, M. (2017). “Learning in the spotlight: approaches to self-regulating and profiling performance,” in Musicians in the Making: Pathways to Creative Performance, eds J. Rink, H. Gaunt, and A. Williamon (Oxford University Press), 206–221.
Wu, B., and Chen, X. (2017). Continuance intention to use MOOCs: integrating the technology acceptance model (TAM) and task technology fit (TTF) model. Comput. Hum. Behav. 67, 221–232. doi: 10.1016/j.chb.2016.10.028
Keywords: technology, learning, musicians, performance, technology acceptance model
Citation: Waddell G and Williamon A (2019) Technology Use and Attitudes in Music Learning. Front. ICT 6:11. doi: 10.3389/fict.2019.00011
Received: 14 March 2018; Accepted: 29 April 2019;
Published: 31 May 2019.
Edited by:
Robin Lee Bargar, Columbia College Chicago, United StatesReviewed by:
Carlos Vaz De Carvalho, Polytechnic Institute of Porto, PortugalAnton Nijholt, University of Twente, Netherlands
Copyright © 2019 Waddell and Williamon. This is an open-access article distributed under the terms of the Creative Commons Attribution License (CC BY). The use, distribution or reproduction in other forums is permitted, provided the original author(s) and the copyright owner(s) are credited and that the original publication in this journal is cited, in accordance with accepted academic practice. No use, distribution or reproduction is permitted which does not comply with these terms.
*Correspondence: Aaron Williamon, YWFyb24ud2lsbGlhbW9uQHJjbS5hYy51aw==