- 1Department of Psychology, Seconda Università di Napoli (SUN), Caserta, Italy
- 2International Institute for Advanced Scientific Studies (IIASS), Vietri sul Mare, Italy
- 3Signal and Image Processing Department, Telecom ParisTech/TSI, Paris, France
- 4School of Computing Science, University of Glasgow, Glasgow, UK
Most studies investigating the processing of emotions in depressed patients reported impairments in the decoding of negative emotions. However, these studies adopted static stimuli (mostly stereotypical facial expressions corresponding to basic emotions) which do not reflect the way people experience emotions in everyday life. For this reason, this work proposes to investigate the decoding of emotional expressions in patients affected by recurrent major depressive disorder (RMDD) using dynamic audio/video stimuli. RMDDs’ performance is compared with the performance of patients with adjustment disorder with depressed mood (ADs) and healthy (HCs) subjects. The experiments involve 27 RMDDs (16 with acute depression – RMDD-A and 11 in a compensation phase – RMDD-C), 16 Ads, and 16 HCs. The ability to decode emotional expressions is assessed through an emotion recognition task based on short audio (without video), video (without audio), and audio/video clips. The results show that AD patients are significantly less accurate than HCs in decoding fear, anger, happiness, surprise, and sadness. RMDD-As with acute depression are significantly less accurate than HCs in decoding happiness, sadness, and surprise. Finally, no significant differences were found between HCs and RMDD-Cs in a compensation phase. The different communication channels and the types of emotion play a significant role in limiting the decoding accuracy.
Introduction
Accurate processing of emotional information is an important social skill allowing one to correctly decode others’ verbal and non-verbal emotional expressions, to provide appropriate affective feedback, and to adopt consistent social behaviors (Esposito, 2013). Several studies showed that major depressive disorder (MDDs) produces deficits in emotional information processing and may trigger social problems like, e.g., avoidance of affective relations and poor social networking (Gotlib and Hammen, 1992; Klerman and Weissman, 1992; McNaughton et al., 1992; Zlotnick et al., 2000; Teo et al., 2013) as well as affective, physical, cognitive, and behavioral problems (DSM-IV; American Psychiatric Association, 2000). In general, investigations of how well depressed individuals decode emotional information exploit static facial expressions (photos) and follow the Ekman’s research paradigm (Ekman, 1992). This states that it is a universal and innate human ability to recognize the facial expressions corresponding to the six emotions called basic or primary (happiness, surprise, disgust, sadness, fear, and anger). The effectiveness of people with depression in decoding emotional expressions through photos was investigated with several methodologies, including the morphing task (Bediou et al., 2005; Joormann and Gotlib, 2006; Gilboa-Schechtman et al., 2008; LeMoult et al., 2009; Schaefer et al., 2010; Aldinger et al., 2013), the emotion recognition task (Kan et al., 2004; Leppänen et al., 2004; Gollan et al., 2008, 2010; Uekermann et al., 2008; Wright et al., 2009; Douglas and Porter, 2010; Milders et al., 2010; Naranjo et al., 2011; Punkanen et al., 2011; Péron et al., 2011; Watters and Williams, 2011; Schneider et al., 2012; Schlipf et al., 2013; Chen et al., 2014), the emotion attentional task (Gotlib et al., 2004; Joormann and Gotlib, 2007; Leyman et al., 2007; Kellough et al., 2008; Sanchez et al., 2013; Duque and Vázquez, 2014), the matching task (Milders et al., 2010; Liu et al., 2012; Chen et al., 2014), and the dot-probe detection task (Fritzsche et al., 2010).
Results from the abovementioned studies show that subjects with depression exhibit a selective attention toward faces expressing negative emotions when stimuli last more than 1 s. This suggests that abnormal emotional information processing during depression is due to cognitive rather than attentional processes (Gotlib et al., 2004; Leyman et al., 2007; Kellough et al., 2008; Fritzsche et al., 2010; Sanchez et al., 2013; Duque and Vázquez, 2014; Joormann and Gotlib, 2007). In particular, Milders et al. (2010) corroborate this hypothesis by showing that MDDs significantly differ from healthy controls (HCs) in the labeling task (involving explicit identification), but not in the matching one (involving implicit emotion processing). On this evidence, many studies prove that depressed patients show a labeling (recognition) bias toward negative emotions. More specifically, such investigations reported contradictory results, showing that MDDs can be either faster and/or more accurate (Mandal and Bhattacharya, 1985; Gilboa-Schechtman et al., 2002; Surguladze et al., 2004; Csukly et al., 2010; Milders et al., 2010; Liu et al., 2012) or slower and/or less accurate (Zuroff and Colussy, 1986; Cooley and Nowicki, 1989; Cerroni et al., 2007; Gollan et al., 2008; Csukly et al., 2009; Douglas and Porter, 2010; Anderson et al., 2011; Watters and Williams, 2011; Aldinger et al., 2013) than HC subjects in decoding fear, anger, and, in particular, sadness.
Although many studies confirm a bias toward negative emotions, others find a deficit in the decoding of positive emotions, especially happiness (Gur et al., 1992; Rubinow and Post, 1992; Mikhailova et al., 1996; Suslow et al., 2001; Surguladze et al., 2004; Karparova et al., 2005; Joormann and Gotlib, 2006; Gilboa-Schechtman et al., 2008; Harmer et al., 2009; LeMoult et al., 2009; Fritzsche et al., 2010; Chen et al., 2014), and/or a global recognition deficit on both positive and negative emotions (Feinberg et al., 1986; Persad and Polivy, 1993; Asthana et al., 1998). Finally, some studies do not detect any deficit (Archer et al., 1992; Gaebel and Wölwer, 1992; Mogg et al., 2000; Weniger et al., 2004; Bediou et al., 2005; Schaefer et al., 2010), whereas others report a bias toward ambiguous and neutral faces that were mostly judged as displaying negative emotions (Hale, 1998; Bouhuys et al., 1999; Leppänen et al., 2004; Gollan et al., 2008; Douglas and Porter, 2010).
The common characteristic of these studies is that they investigate the ability of depressed subjects to decode emotional expressions through the visual channel, using photos. This has two main limitations: (1) static stimuli do not reflect the way people experience emotions in their everyday life (facial emotional expressions are dynamic and are often accompanied by vocalizations and/or speech) and (2) photos of emotional faces are taken at high-intensity emotional levels and do not correspond to everyday life expressed emotions. On the contrary, multimodal dynamic stimuli have greater ecological validity and allow to investigate the amount of emotional information conveyed not only by the visual channel but also by the auditory one and by the combination of visual and auditory signals. Nevertheless, a few studies, only recently, exploit dynamic emotional stimuli to investigate on the depressed subjects’ ability to decode emotional expressions using either audio or audio/video stimuli (Kan et al., 2004; Uekermann et al., 2008; Naranjo et al., 2011; Punkanen et al., 2011; Péron et al., 2011; Schlipf et al., 2013).
In summary, the studies investigating the ability of depressed subjects to decode emotional stimuli are numerous and report different results. These differences may be attributed to the use of different methodologies in assessing the accuracy of depressed subjects, to the type of stimuli, the characteristics of the participants, and the different clinical states and depression degrees. Given various data, it remains an open issue whether depressed subjects exhibit a global or a specific emotional bias in decoding emotional expressions, as well as whether their clinical state (i.e., the acute or compensation phase) and depression degrees play a role in their performance. In addition, it is of interest to assess the role of the communication channels in conveying emotional information.
This study aims at clarifying these issues through the analysis of how people with depression decode emotional displays. The goal is to explore the MDDs’ ability to decode emotional multimodal dynamic stimuli and to match their performance with both AD and HC subjects. Our hypotheses are:
1 Recurrent major depressive disorder (RMDD) patients should show a negative bias (that is an emotional recognition deficit) toward specific basic emotions;
2 The bias is more evident when depressive symptoms are severe. Thus, the performance of acutely depressed patients should be worse than the performance of compensated ones;
3 The bias is independent of the communication mode. Thus, it should appear in the visual, auditory, and visual/auditory stimuli.
Dynamic stimuli either in visual or auditory or both visual and auditory form are used, extracted from Italian movies, and therefore embedded in the movie script context to increase the naturalness and ecological validity of the experiment.
Materials and Methods
Participants
Four groups of participants took part in this study:
(1) Outpatients with recurrent major depression in acute phase (RMDD-A). The initial group consisted of 20 subjects. A 51-year-old man was excluded for hearing impairments; a 39-year-old man was excluded because it was possible that the depressed mood was associated with brain surgery; a 38-year-old woman was excluded because she had not yet started the drug therapy; a 64-year-old woman was excluded because the psychiatrist did not provide her clinical history questionnaire. The final group consisted of 16 outpatients (10 males and 6 females; mean age = 53.3; SD = 9.8);
(2) 11 outpatients (3 males and 8 females, mean age = 48.8; SD = 11.9) with recurrent major depression in compensation phase (RMDD-C);
(3) Outpatients with adjustment disorder with depressed mood (AD). The initial group consisted of 18 subjects. A 55-year-old man and a 66-year-old woman were excluded because they just started to take medications. The final group consisted of 16 outpatients (5 males and 11 females; mean age = 54.5; SD = 9.5);
(4) Healthy control (HC): the initial group consisted of 18 subjects. A 38-year-old man and a 60-year-old woman were excluded because they were under anxiolytics. The final group consisted of 16 subjects (6 males and 10 females; mean age = 52; SD = 13.3).
A MDD is a “medical condition that includes abnormalities of affect and mood, neurovegetative functions (such as appetite and sleep disturbances), cognition (such as inappropriate guilt and feelings of worthlessness), and psychomotor activity (such as agitation or retardation)” (Fava and Kendler, 2000, p. 335). These symptoms are recurrent and occurring in patients in acute phase (RMDD-A), whereas RMDD-C patients are not showing them. Adjustment disorder with depressed mood “is a psychological reaction to overwhelming emotional or psychological stress, resulting in depression” [in Rosenthal (2010), p. 145].
The three groups of patients (RMDD-A, RMDD-C, and AD) were recruited at the Mental Health Service of Avellino, Italy. They received a diagnosis of Recurrent Major Depression Disorder and Adjustment Disorder with Depressed Mood according to DSM-IV criteria (American Psychiatric Association, 2000) and were under antidepressant medications (SSRI – Selective Serotonin Reuptake Inhibitors; SNRI – serotonin–norepinephrine reuptake inhibitor; tricyclic antidepressants; anxiolytics). HCs did not show any current or past history of psychiatric diseases (these were the selection criteria for them) and were recruited through invitation by phone. HCs were then met at their private homes and were administered both the BDI-II and the emotion recognition task. Table 1 summarizes the clinical and demographic characteristics of the four groups.
Stimuli, Measures, and Procedure
For each RMDD-A, RMDD-C, and AD patient, the psychiatrists of the Mental Health Service Center have provided the clinical history (diagnosis, type of drugs, and duration of treatment). Patients were excluded from the experiment if: (a) the depressed mood was associated with other disorders (e.g., personality disorders, psychosis, alcoholism, cognitive decline, or hearing impairment). The only exception is anxiety because it is often associated with depression; or (b) the period during which the patient was under drug therapy was shorter than 1 year.
The Italian version of the Beck Depression Inventory Second Edition (BDI II; Beck et al., 1996; Ghisi et al., 2006) was administered to the control group and to the three groups of patients. The BDI-II is a self-report questionnaire (21-statements each with 4 possible choices) widely used as a psychometric test for measuring the severity of depression in terms of four classes: normal, mild, moderate, and severe. The BDI-II is based on the DSM-IV and is in agreement with its diagnostic criteria for depression (American Psychiatric Association, 2000).
The emotion recognition task consisted of 60 emotional stimuli grouped in 20 videotaped facial expressions (without audio), 20 audiotaped vocal expressions (without video), and 20 audio/video recordings, all selected from the COST 2102 Italian Emotional database, which consists of 216 emotional video-clips extracted from Italian speaking movies (Esposito et al., 2009; Esposito and Riviello, 2010). In these video-clips, Italian actors/actresses act one of the following five basic emotions: happiness, fear, anger, surprise, and sadness. The emotional content of the video clips was assessed by 210 raters split into 3 groups: 70 raters have listened to the audio channel of each clip without seeing the video, 70 raters have watched the video channel of the clips without hearing the audio, and another 70 raters have watched the full clips (both audio and video channel available).
The recordings’ duration was kept short (between 2 and 3.5 s, the average stimulus’ length was 2.5 s, SD = ±1 s) to avoid overlaps of different emotions. The 60 stimuli selected from the abovementioned database were among those who received the greater raters’ agreement (more than the 70% of agreements).
Informed consent forms were signed by the participants after the study had been described to them. The tasks were administered to the subjects individually in a quiet room. Each participant first completed the BDI-II and then the emotion recognition task. No time limit was given to complete the task. The stimuli were presented one by one on a PC monitor. After the presentation of each stimulus, subjects’ were asked to label it as happiness, fear, anger, surprise, sadness, a different emotion, or no emotion, selecting the option that best described (for her/him) the emotional state acted in the audio, visual, or audio/video stimulus. After each labeling, they moved to the successive stimulus. The administration procedure lasted for approximately 30 min.
Data Analysis
The following statistical tests were performed to assess the collected data. Three one-way analyses of variance (ANOVA) analyses were performed to evaluate whether there were significant differences among groups in along age, BDI-II scores, and treatment duration (see Participants’ Clinical and Demographic Characteristics). The Fisher’s exact test 4 × 4 (4 groups and 4 education levels) was performed to assess differences among groups with respect to educational levels (see Participants’ Clinical and Demographic Characteristics).
The Pearson Correlation coefficient was used to evaluate correlations between, on the one hand, answers to the emotion recognition task and, on the other hand, BDI-II scores (see Beck Depression Inventory-II) and treatment duration (see Treatment Duration).
A repeated measures ANOVA (5 × 3) on the number of correct responses was separately performed to assess each involved group ability to decode the emotional stimuli (the five abovementioned basic emotions) portrayed through the three different communication modes (within-subject factor) – (see Statistical Analyses on Each Participating Group).
One-way ANOVA analyses were performed to assess differences between groups on communication modes and emotional categories (see Statistical Analyses on All Participating Groups).
A repeated measure ANOVA (5 × 3 × 4) was performed, with emotions (the five basic emotions) and communication modes (audio, mute video, and combined audio/video) as within-subject factors, and groups (RMDD-As, RMDD-Cs, ADs, and HCs) as between-subject factor. In addition, separate repeated measures ANOVA were performed. In particular, an ANOVA 5 × 3 × 3 to compare RMDD-A and RMDD-C performance with HC subjects, and an ANOVA 5 × 3 × 2 to match only RMDD-As and RMDD-Cs performances (see Statistical Analyses on All Participating Groups). Bonferroni post hoc comparisons were performed to assess statistical significance of differences. The confidence level was established at α = 0.05.
Confusion matrices were computed on the percentage of correct responses for each communication mode, to assess misperceptions among emotion categories (see Confusion Matrices).
Results
Participants’ Clinical and Demographic Characteristics
The four groups (RMDD-A, RMDD-C, AD, and HC) did not differ significantly in age [F(3,55) = 0.5, p-value = 0.64] and years of education [χ2(9) = 11.30, p-value = 0.18]. The three groups of patients (RMDD-A, RMDD-C, and AD) did not differ significantly in treatment duration [F(2,40) = 2.2, p-value = 0.12].
A significant difference among the four groups was found (as expected) for the BDI-II scores [F(3,51) = 42.9, p-value ≪ 0.01]. Bonferroni post hoc showed no differences between HCs and RMDD-Cs (p-value = 0.29) and between RMDD-As and ADs (p-value = 0.07). Significant differences were found between HCs and RMDD-As (p-value ≪ 0.01), between HCs and ADs (p-value ≪ 0.01); RMDD-Cs and RMDD-As (p-value = 0.01); and RMDD-Cs and ADs (p-value ≪ 0.01). In summary, these results confirm that RMDD-As and ADs are severely depressed, while RMDD-Cs and HCs have typical scores. Participants’ clinical and demographic characteristics are reported in Table 1.
Beck Depression Inventory-II
No correlation (p-value > 0.05) was found between subjects’ (patients and control) BDI-II scores and their number of correct answers to the emotion recognition task suggesting that their performance was not correlated with the severity of the depressive symptoms. These results are illustrated in Table 2.
Treatment Duration
No correlation (p-value > 0.05) was found between the average treatment duration and patients’ performance, as illustrated in Table 3.
The Emotion Recognition Task
Figure 1 shows the accuracy for each participating group. Figure 2 reports the percentage of groups’ correct responses in each communication mode (audio, mute video, and audio/video).
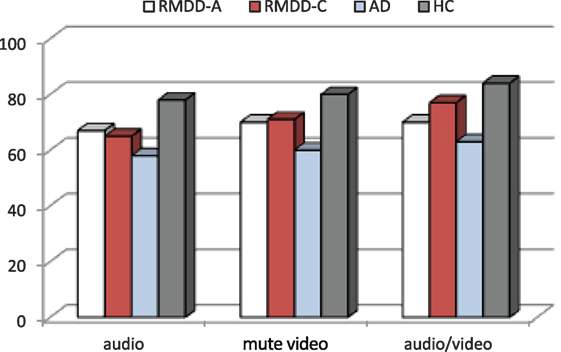
Figure 2. Percentage of groups’ correct responses in each communication mode (audio, mute video, and audio/video).
Table 4 reports accuracy mean scores and SDs for each participating group, emotion, and communication mode.
Statistical Analyses on Each Participating Group
The repeated measures ANOVA (5 × 3) performed to assess the ability of each group to decode emotional stimuli portrayed through the audio, mute video, and combined audio/video show that:
– RMDD-As did not show significant differences among emotional categories [F(4,60) = 1.56, p-value = 0.19] and communication modes [F(2,30) = 0.60, p-value = 0.55]. There is a significant interaction between emotion categories and communication modes [F(8,120) = 3.72, p-value = 0.001]. Post hoc shows that this interaction is due to:
(1) a significant difference in the audio between surprise and fear (p-value = 0.015), which are, respectively, the less and most accurately recognized emotions in this mode (see mean scores in Table 4);
(2) a significant difference in the mute video between surprise and fear (p-value = 0.009), and surprise and anger (p-value = 0.028) indicating that, in this mode, surprise is the least recognized emotional category, and fear and anger are the most recognized ones (see mean scores in Table 4).
– RMDD-Cs did not show significant differences among emotional categories [F(4,40) = 1.04, p-value = 0.39] and communication modes [F(2,20) = 3.84, p-value = 0.06]. A significant interaction between emotion and communication mode [F(8,80) = 2.57, p-value = 0.015] was found. Post hoc shows that this interaction is due to a significant difference in the mute video between surprise and fear (p-value = 0.016) indicating that they are the least and most accurately recognized emotional categories, respectively (see mean scores in Table 4).
– ADs did not show significant differences among emotional categories [F(4,60) = 1.87, p-value = 0.127] and communication modes [F(2,30) = 0.825, p-value = 0.448]. A significant interaction between emotions and communication modes [F(8,120) = 2.30, p-value = 0.025] was found. Post hoc shows that this interaction is due to a significant difference in the mute video between surprise and fear (p-value = 0.024), and sadness and fear (p-value = 0.002) indicating that fear is the most accurately recognized emotion, and surprise and sadness are the least accurately recognized ones (see mean scores in Table 4).
– HCs did not show significant differences among communication modes [F(2,30) = 1.51, p-value = 0.237]. Significant differences were found among emotion categories [F(4,60) = 2.88, p-value = 0.03]. A significant interaction was found between emotions and communication modes [F(8,120) = 7.32, p-value ≪ 0.01]. Post hoc in the mute video reveal for emotions, a significant difference between happiness and surprise (p-value = 0.032) indicating that they are, respectively, the most and least accurately recognized emotional categories. Post hoc on the emotions × communication modes interaction shows a significant difference in the mute video between surprise and all the other emotions (p-value ≪ 0.01), indicating that in this communication mode surprise is the emotion least accurately recognized (see mean scores in Table 4).
Statistical Analyses on All Participating Groups
Emotional Categories
A one-way ANOVA on each emotional category, independently from the communication mode, shows that there are significant differences among the groups for fear [F(3,55) = 3.00, p-value = 0.038], anger [F(3,55) = 4.78, p-value = 0.005], and sadness [F(3,55) = 3.93, p-value = 0.013]; no significant differences were found for happiness [F(3,55) = 2.51, p-value = 0.068] and surprise [F(3,55) = 1.88, p-value = 0.143]. Post hoc shows a significant difference between ADs and HCs with respect to fear (p-value = 0.025), anger (p-value = 0.004), and sadness (p-value = 0.007). See the percentage of emotion recognition accuracy in Figure 1.
Communication Channels
A one-way ANOVA on each communication mode, independently from the emotional categories, shows significant differences among the groups for the audio [F(3,55) = 4.47, p-value = 0.007], mute video [F(3,55) = 5.87, p-value = 0.002], and audio/video [F(3,55) = 4.82, p-value = 0.005]. Post hoc shows significant differences between ADs and HCs for audio (p-value = 0.004), mute video (p-value = 0.001), and audio/video (p-value = 0.004). See the percentage of correct responses in each communication mode in Figure 2.
Emotion Categories and Communication Channels
The repeated measures ANOVA (5 × 3 × 4) shows as main effects:
(a) A significant difference among the groups [F(3,55) = 6.58, p-value = 0.001]. Post hoc reports a significant difference between ADs and HCs (p ≪ 0.01). On the average, ADs are less accurate than all the other groups, as clearly appears also in Figures 1 and 2.
(b) A significant effect was found among emotional categories [F(4,220) = 4.46, p-value = 0.004]. Some emotions are more accurately recognized than the others. In particular, post hoc reports a significant difference between surprise and anger (p-value = 0.031). As shown in Figure 1, surprise is, the emotion less accurately recognized and anger is the most accurately recognized one by all but not the AD group.
(c) A significant effect was found among the communication modes [F(2,110) = 6.16, p-value = 0.003]. Post hoc shows that this effect was due to a significant difference between the audio and audio/video (p-value = 0.005). The accuracy mean scores indicate that all groups are less accurate in the audio and more accurate in the audio/video (see Figure 2).
The ANOVA analysis also reports significant interactions between (a) emotions and communication modes [F(8,440) = 9.10, p-value ≪ 0.01] and (b) emotions, communication modes, and groups [F(24,440) = 1.78, p-value = 0.013].
(a) The significant interaction between emotions and communication modes indicates that the emotion decoding accuracy depends on the communication mode. More specifically, post hoc shows that:
(1) There are no significant differences among communication modes for the recognition accuracy of happiness and sadness (p-value > 0.05).
(2) There are significant differences in the recognition accuracy of fear between the mute video and audio (p-value = 0.01), and the mute video and audio/video (p-value ≪ 0.01). Subjects made less errors in decoding fear from the mute video rather than the audio and audio/video;
(3) There are significant differences in the recognition accuracy of anger between the audio and mute video (p-value = 0.002), and the audio and audio/video (p-value ≪ 0.01). Subjects made more errors in decoding anger from the audio rather than the mute video and audio/video;
(4) There are significant differences in the recognition accuracy of surprise between the audio and mute video (p-value = 0.005), the audio and audio/video (p-value = 0.02), and the mute video and audio/video (p-value ≪ 0.01) indicating that subjects made more errors in decoding surprise from the mute video, as well as, from the audio;
(b) The interaction between emotions × communication modes × groups indicates that the emotion decoding accuracy depends, on both the communication mode and the involved groups. More specifically, post hoc comparisons show:
– a significant difference for happiness in the mute video [F(3,55) = 3.88, p-value = 0.014] between RMDD-As and HCs (p-value = 0.02) and ADs and HCs (p-value = 0.02) indicating that happiness is less accurately recognized by both RMDD-As and ADs than by HCs (see mean scores in Table 4);
– a significant difference for fear in the audio between ADs and HCs [F(3,55) = 3.35, p-value = 0.025]indicating that ADs are less accurate than HCs (see mean scores in Table 4);
– a significant difference for anger in:
• the mute video between ADs and HCs [F(3,55) = 2.93, p-value = 0.042];
• the audio/video [F(3,55) = 4.89, p-value = 0.004] between ADs and RMDD-Cs (p-value = 0.01) and ADs and HCs (p-value = 0.01)
indicating that ADs are less accurate than HCs and RMDD-Cs in the decoding of anger (see mean scores in Table 4);
– a significant difference for surprise in the audio [F(3,55) = 4.96, p-value = 0.004] between ADs and HCs (p-value = 0.01) and RMDD-As and HCs (p-value = 0.01)indicating that ADs and RMDD-As are less accurate than HCs. In addition, for surprise, all the participating groups make significant errors in the mute video (see mean scores in Table 4);
– a significant difference for sadness in the mute video [F(3,55) = 4.88, p-value = 0.004] and in the audio/video [F(3,55) = 3.62, p-value = 0.019] between ADs and HCs indicating that ADs are less accurate than HCs (see mean scores in Table 4).
From the above analyses, it clearly emerges that ADs are less accurate with respect to the other participating groups, no matter the emotion category and communication mode.
A 5 × 4 × 3 repeated measures ANOVA was made in order to finely assess possible other differences between RMDD-As and RMDD-Cs, as well as HCs. Results showed a significant difference between RMDD-As and HCs for happiness in the mute video [F(2,40) = 4.96, p-value = 0.012], surprise in the audio [F(2,40) = 5.98, p-value = 0.005], and a slight difference for sadness in the audio/video [F(2,40) = 3.06, p-value = 0.05].
No significant differences were found between RMDD-As and RMDD-Cs [F(1,25) = 0.139, p-value = 0.712], even though RMDD-Cs’ performance is slightly better than RMDD-As, as illustrated by Figure 1 and Table 4.
Confusion Matrices
Table 5 reports confusion matrices for each emotion, communication mode, and participating group (correct recognition accuracy is expressed in percentages).
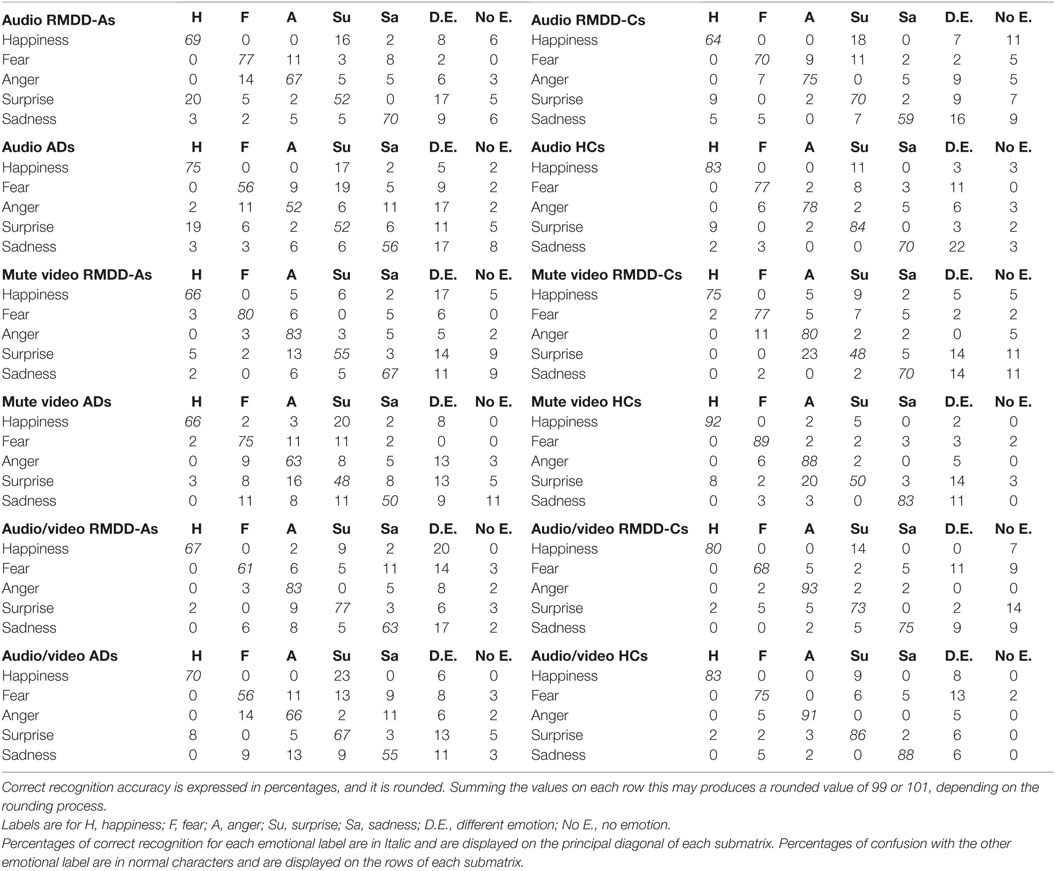
Table 5. Confusion matrices for each emotion and communication mode obtained from each participating group.
It is worth to discuss, at this stage, the confusion matrices reported in Table 5, in order to highlight where confusions are made and for which emotional category. Table 5 shows that:
(1) Happiness, in the mute video, is less accurately decoded by RMDD-As and ADs than by HCs (accuracy is 66% for both groups vs. 92% for HCs) which mostly confused it with a different emotion (17%) and surprise (20%), respectively.
(2) Fear is less accurately recognized in the audio by ADs than by HCs (56 vs. 77%). ADs mostly confuse fear with surprise (19%).
(3) Anger is less accurately recognized by ADs than by HCs in the audio, and audio/video (52 vs. 78% in the audio; 66 vs. 91% in the audio/video). ADs mostly confuse anger with a different emotion (17% in the audio and 13% in the audio/video).
(4) Surprise is less accurately recognized by ADs and RMDD-As than by HCs (52 vs. 84%) in the audio. ADs and RMDD-As mostly confuse surprise with happiness (19% for ADs and 20% for RMDD-As) and a different emotion (11% for ADs and 17% for RMDD-As). In addition, surprise, in the mute video, is the least accurately decoded emotions by all participating groups. There, surprise is mostly confused with anger and a different emotion (see percentages in Table 5).
(5) Sadness is less accurately recognized by ADs than by HCs (50 vs. 83%) in the mute video. ADs mostly confuse sadness with fear, surprise, and no emotion (11%).
Discussion
The goal of this study is to investigate the ability to decode multimodal emotional expressions in outpatients with RMDD. For this purpose, multimodal dynamic stimuli selected from the COST 2102 Italian databases (Esposito et al., 2009; Esposito and Riviello, 2010), through an emotion recognition task, have been exploited. The COST 2102 databases include a set of audio, mute video, and audio/video recordings of short durations, in which actors/actresses express one of the following five primary emotions: happiness, fear, anger, surprise, and sadness (either through sentences with no emotional semantic content and/or facial expressions).
To the best of our knowledge, this is the first study investigating the capability to decode emotional expressions in RMDD patients also in the compensation phase of the disorder. Indeed, usually, the comparison is made between patients in the acute and remission phase (Joormann and Gotlib, 2007; LeMoult et al., 2009; Fritzsche et al., 2010; Anderson et al., 2011; Aldinger et al., 2013). Therefore, this is the first data on such cases. In addition, this is the first study matching MDDs’ with AD patients’ performances. Such patients, according to DSM-IV, do not suffer from a mood disorder, even though, their clinical state is characterized by predominant depressive symptoms.
BDI-II, Drugs, and Emotion Recognition Task
In our study, the severity of depressive symptoms (scored through the BDI-II questionnaire) does not correlate with the patients’ (RMDD-As, RMDD-Cs, and ADs) emotion recognition task accuracy (see Table 2). This suggests that the poorer decoding accuracy toward primary emotions may be independent of the patients’ clinical state. This observation is consistent with previous results (Milders et al., 2010; Naranjo et al., 2011) and supports Beck’s theory, suggesting that biases toward environmental stimuli are a pre-depressive personality trait that becomes active when it meets negative events. In addition, there is no correlation between the patients’ (RMDD-As, RMDD-Cs, and ADs) emotion recognition task accuracy and their treatment duration (see Table 3). It can be assumed that drugs’ diversities and individual reactions to specific drugs may be a factor influencing the different patients’ performance (Bhagwagar et al., 2004; Harmer et al., 2009). However, this aspect is not considered in this study. Further research is needed to investigate the effect of different drugs.
Emotion Categories
The results reported in this paper indicate that the capability to decode emotional expressions is more impaired in AD than in RMDD patients (either RMDD-As or RMDD-Cs). ADs are, with respect to the other groups, especially impaired in recognizing negative emotions of fear, anger, and sadness, while they perform similar to RMDD-As (see Figure 1) for happiness and surprise.
For the ADs’ performances, there is no support by data reported in literature, since former studies report only comparisons between HCs and MDDs. Since our results indicate that ADs, more than MDDs, are unable to decode emotional expressions, it is worth to hypothesize that this inability is associated with depressive symptoms independently of the causes originating them.
Our results also indicate that RMDD-As are significantly less accurate than HCs in decoding sadness and exhibit a general worst performance for anger and fear, supporting the first hypothesis formulated in the Section “Introduction” (even though, not significant).
To explain the ADs worst performance with respect to RMDDs, in decoding negative emotional stimuli, it is worth to consider Beck’s theoretical framework (Beck et al., 1979, 1996; Beck, 2002). Such theory assumes that erroneous interpretations of environmental stimuli are due to maladaptive cognitive schemas becoming active and dominant when stressful events occur. Since ADs’ depressive symptoms are directly triggered by stressful events (with a consciousness of symptoms’ causes), their maladaptive cognitive schemas are active and dominant, thus worsening the bias in decoding negative emotional stimuli, accomplished through a psychological avoidance to recognize them in the others. RMDD disorder is not directly connected to specific stressful events, even though a subsequent stressful event can worsen depressive symptoms. Consequently, RMDDs’ emotional decoding bias is latent (being triggered only when specific stressful events occur) granting to RMDDs a superior performance with respect to ADs in decoding negative emotional stimuli.
Adjustment disorder with depressed mood and RMDD-As also exhibit a poor decoding of happiness with respect to HCs (see Figure 1). This result is consistent with other studies reporting a deficit in the recognition of static happy stimuli (Rubinow and Post, 1992; Surguladze et al., 2004; LeMoult et al., 2009; Csukly et al., 2010; Chen et al., 2014). Bias toward happiness may be due to anhedonia (Ribot, 1896) that is a loss of capacity to experience pleasure from activities and situations usually considered rewarding (e.g., social relations, sports, hobbies, and sexual activities). Indeed, RMDD-As and ADs report a mean score of 2.0 (SD = 1.15) and 1.63 (SD = 1.43), respectively, to the BDI-II’s item 4 measuring “loss of pleasure,” suggesting that they suffer from a moderate degree of this symptom. Alternatively, the bias toward happiness may be explained through the maladaptive cognitive schemas suggested by Beck (2002) (Beck et al., 1979, 1996) not allowing ADs and RMDD-As to correctly decode positive stimuli since they are incongruent with their depressed thinking and mood.
The RMDD-Cs show slightly superior performances than RMDD-As (as asserted by the second hypothesis formulated in the Section “Introduction”) and slightly worse performances than HCs (no significant differences are found, see Figure 1). RMDD-Cs make substantial errors in decoding sadness and surprise. However, more data are needed to explain these slight differences because of the small number of subjects in this group.
Finally, confusion matrices show that, when depressed subjects make errors in the emotion labeling task, they often chose “a different emotion” as an option. Probably, this label is selected either when none of the listed labels fits the patients’ perceived emotional stimulus or when they were not able to identify the portrayed emotion.
Confusion among emotions can also arise because of emotional expression common features depending from the communication mode. For instance, in the mute video, happiness and surprise may have in common the movements of certain facial muscles (Ekman and Friesen, 1972). Nevertheless, the clinical state was one of the main confusing factors.
These results show that depressed subjects do not exhibit a global deficit toward emotional stimuli, rather their performance depends on the specific emotion and (see Results in Section “Statistical Analyses on All Participating Groups”) communication channels (see Discussion below).
Communication Modes
Analyzing the subjects’ recognition accuracy through the three communication modes (audio, mute video, and audio/video), independently from the emotional category, it appears that the audio and audio/video are the ones in which all groups make most and least errors (see Figure 2), respectively. The audio seems to be the poorer emotional communication mode. This result may appear to contradict previously reported experiments, where it was shown that Italian native speakers perform equally well in decoding emotional states from the audio alone and the audio/video combined (Esposito, 2007; Esposito et al., 2009). However, the differences in these results can easily be attributed to the different clinical states of the participating groups and the different experimental set-up (the age of the involved subjects, for example, was different).
When emotion categories are accounted for, it appears that communication modes may affect the subjects’ ability to decode specific emotional states “independently” from the clinical state. This is particularly true for, surprise and fear in the mute video, where all groups perform similarly. Surprise is a quite ambiguous emotion that can assume positive or negative valence, and a mute visual stimulus may not be capable to convey enough information for its correct interpretation (Esposito et al., 2009; Esposito and Riviello, 2010). Conversely, the accurate recognition in the mute video of facial expressions of fear may be attributed to our survival abilities to sense imminent attacks or dangers.
The dependency from the clinical state in the ability of recognizing emotional states explain all the other differences in the emotion recognition task accuracy.
These results are in support to the third hypothesis formulated in the Section “Introduction,” in the sense that depression causes a deficit in the emotion recognition capability in all communication modes and for all the emotional categories (in the sense that patients make more errors than healthy subjects), although this deficit is more marked for specific emotions portrayed through a specific communication channel.
Conclusion
In this study, we found that depressed subjects have an impairment in the decoding of dynamic emotional expressions (vocal and facial expressions). It can be assumed that this misinterpretation of others’ emotional expressions not only contributes to their interpersonal difficulties but also does not allow them to correctly decode the emotions they experience, further aggravating the depressive symptoms. In addition, it may become more debilitating when subjects have to face stressful and negative events.
Our data are partly consistent with those of other studies that also exploited dynamic stimuli. Indeed, Schneider et al. (2012) used short audio/video clips in which actors expose emotional narratives and found that MDDs exhibit a general emotion processing deficit (happiness, sadness, fear, and disgust). Naranjo et al. (2011) used musical, vocal, and static facial stimuli and found that depressed subjects are less accurate than the control group in all the three emotion recognition tasks. In addition, they found that depressed subjects are more likely to attribute negative emotions to neutral voices and faces. Péron et al. (2011) used a set of vocal stimuli (consisting of pseudo-words with emotional intonation but no semantic content) and found that the depressed group displayed significant impairment in the identification of emotional prosody cues of fear, sadness, and happiness. Punkanen et al. (2011) reported that depressed subjects mostly confuse musical expressions of fear and sadness with anger, showing also that they were less accurate than the control group in decoding happiness and tenderness. Uekermann et al. (2008) exploiting prosodic emotional stimuli found that depressed subjects show impairments for anger, happiness, fear, and neutral expressions (but not for sadness). Kan et al. (2004) exploiting mute video and audio recordings found a poorer decoding accuracy of surprise for the audio stimuli. Schlipf et al. (2013) reported that depressed subjects are impaired in processing positive emotional words exploiting a set of emotional adjectives (of positive, negative, and neutral valence) administered through speech vocal presentation.
The diverse results discussed earlier are not necessarily contradictory. Differences can be attributed to patients’ characteristics (acute, remission, recurrent, chronic, and so on), their medical status (inpatient, outpatient), pharmacological treatments, the severity of disorder, the stimuli, and methodological paradigms. With respect to the last factor, the distinct stimuli and tasks may involve distinct cognitive processes (i.e., memory, selective attention, and recognition) causing different performances. Given these multiple and different results, it is clear that standardized methodologies and ecological stimuli are necessary to assess the depressed subjects capability to decode others’ emotional expressions.
Our future plans are:
– to further investigate depressive disorder’s effects on the emotional information processing by increasing the number of participants;
– to investigate whether and which dysfunctional cognitive patterns are associated with depression;
– to check for anxiety effects, since depression is often associated with anxiety (Belzer and Schneier, 2004; Hranov, 2007) and anxiety may affect the decoding of emotional expressions (Bouhuys et al., 1997); and
– to test for antidepressant effects, since antidepressants may influence the emotional information processing (Bhagwagar et al., 2004; Harmer et al., 2009).
Author Contributions
All authors listed have made substantial, direct, and intellectual contribution to the work and approved it for publication.
Conflict of Interest Statement
The authors declare that the research was conducted in the absence of any commercial or financial relationships that could be construed as a potential conflict of interest.
Acknowledgments
The authors acknowledge for their participation and support nurses, patients, and the following medical doctors from the Mental Health Center of Avellino (ASL Avellino), Italy: Acerra Giuseppe, M.D., Bianco Pietro, M.D., Fina Emilio, M.D., Florio Sergio, M.D., Nappi Giuseppe, M.D., Panella Maria Teresa, M.D., Prizio Domenica, M.D., Tarantino Costantino, M.D., and Tomasetti Antonio, M.D.
References
Aldinger, M., Stopsack, M., Barnow, S., Rambau, S., Spitzer, C., Schnell, K., et al. (2013). The association between depressive symptoms and emotion recognition is moderated by emotion regulation. Psychiatry Res. 205, 59–66. doi: 10.1016/j.psychres.2012.08.032
American Psychiatric Association. (2000). Manuale Diagnostico e Statistico dei Disturbi Mentali (IV ed.). Washington, DC: Masson MI.
Anderson, I. M., Shippen, C., Juhasz, G., Chase, D., Thomas, E., Downey, D., et al. (2011). State-dependent alteration in face emotion recognition in depression. Br. J. Psychiatry 198, 302–308. doi:10.1192/bjp.bp.110.078139
Archer, J., Hay, D. C., and Young, A. W. (1992). Face processing in psychiatric conditions. Br. J. Clin. Psychol. 31, 45–61. doi:10.1111/j.2044-8260.1992.tb00967.x
Asthana, H. S., Mandal, M. K., Khurana, H., and Haque-Nizamie, S. (1998). Visuospatial and affect recognition deficit in depression. J. Affect. Disord. 48, 57–62. doi:10.1016/S0165-0327(97)00140-7
Beck, A. T. (2002). “Cognitive models of depression,” in Clinical Advances in Cognitive Psychotherapy: Theory on Application, Vol. 2012, eds R. L. Leahy and E. T. Dowd (New York: Springer Publishing Company), 29–61.
Beck, A. T., Rush, A. J., Shaw, B. F., and Emery, G. (1979). Cognitive Therapy of Depression. New York: The Guildford Press.
Beck, A. T., Steer, R. A., and Brown, G. K. (1996). Comparison of the Beck depression inventories-IA and II in psychiatric outpatients. J. Pers. Assess. 67, 588–597. doi:10.1207/s15327752jpa6703_13
Bediou, B., Krolak-Salmon, P., Saoud, M., Henaff, M. A., Burt, M., Dalery, J., et al. (2005). Facial expression and sex recognition in schizophrenia and depression. Can. J. Psychiatry 50, 525–533. doi:10.1177/070674370505000905
Belzer, K., and Schneier, F. R. (2004). Comorbidity of anxiety and depressive disorders: issues in conceptualization, assessment, and treatment. J. Psychiatr. Pract. 10, 296–306. doi:10.1097/00131746-200409000-00003
Bhagwagar, Z., Cowen, P. J., Goodwin, G. M., and Harmer, C. J. (2004). Normalization of enhanced fear recognition by acute SSRI treatment in subjects with a previous history of depression. Am. J. Psychiatry 161, 166–168. doi:10.1176/appi.ajp.161.1.166
Bouhuys, A., Geerts, E., and Gordijn, M. C. M. (1999). Gender-specific mechanisms associated with outcome of depression: perception of emotions, coping and interpersonal functioning. Psychiatry Res. 85, 247–261. doi:10.1016/S0165-1781(99)00003-7
Bouhuys, A., Geerts, E., and Mersch, P. P. A. (1997). Relationship between perception of facial emotions and anxiety in clinical depression: does anxiety-related perception predict persistence of depression? J. Affect. Disord. 43, 213–223. doi:10.1016/S0165-0327(97)01432-8
Cerroni, G., Tempesta, D., Riccardi, I., Stratta, P., Struglia, F., and Rossi, A. (2007). Il riconoscimento emotivo delle espressioni facciali nella schizofrenia e nella depressione. Epidemiol. Psichiatr. Soc. 16, 179–182. doi:10.1017/S1121189X00004814
Chen, J., Ma, W., Zhang, Y., Wu, X., Wei, D., Liu, G., et al. (2014). Distinct facial processing related negative cognitive bias in first-episode and recurrent major depression: evidence from the N170 ERP component. PLoS ONE 9:e109176. doi:10.1371/journal.pone.0109176
Cooley, E. L., and Nowicki, S. (1989). Discrimination of facial expressions of emotion by depressed subjects. Genet. Soc. Gen. Psychol. Monogr. 115, 449–465.
Csukly, G., Czobor, P., Szily, E., Takács, B., and Simon, L. (2009). Facial expression recognition in depressed subjects. The impact of intensity level and arousal dimension. J. Nerv. Ment. Dis. 197, 98–103. doi:10.1097/NMD.0b013e3181923f82
Csukly, G., Telek, R., Filipovits, D., Takács, B., Unoka, Z., and Simon, L. (2010). What is the relationship between the recognition of emotions and core beliefs: associations between the recognition of emotions in facial expressions and the maladaptive schemas in depressed patients. J. Behav. Ther. Exp. Psychiatry 42, 129–137. doi:10.1016/j.jbtep.2010.08.003
Douglas, K. M., and Porter, R. J. (2010). Recognition of disgusted facial expressions in severe depression. Br. J. Psychiatry 197, 156–157. doi:10.1192/bjp.bp.110.078113
Duque, A., and Vázquez, C. (2014). Double attention bias for positive and negative emotional faces in clinical depression: evidence from an eye-tracking study. J. Behav. Ther. Exp. Psychiatry 46, 107–114. doi:10.1016/j.jbtep.2014.09.005
Ekman, P. (1992). An argument for basic emotions. Cogn. Emot. 6, 169–200. doi:10.1080/02699939208411068
Ekman, P., and Friesen, W. V. (1972). Hand movements. J. Commun. 22, 353–374. doi:10.1111/j.1460-2466.1972.tb00163.x
Esposito, A. (2007). “The amount of information on emotional states conveyed by the verbal and nonverbal channels: some perceptual data,” in Progress in Nonlinear Speech Processing. LNCS 4391, eds Y. Stylianou, M. Faundez-Zanuy and A. Esposito (Heidelberg: Springer-Verlag), 245–268.
Esposito, A. (2013). “The situated multimodal facets of human communication,” in Coverbal Synchrony in Human-Machine Interaction, Chap. 7, eds M. Rojc and N. Campbell (Boca Raton, FL: CRC Press, Taylor & Francis Group), 173–202.
Esposito, A., and Riviello, M. T. (2010). “The new Italian audio and video emotional database,” in Development of Multimodal Interfaces: Active Listening and Synchrony, LNCS 5967, eds A. Esposito, N. Campbell, C. Vogel, A. Hussain and A. Nijholt (Berlin, Heidelberg: Springer-Verlag), 406–422.
Esposito, A., Riviello, M. T., and Di Maio, G. (2009). “The COST 2102 Italian audio and video emotional database,” in Frontiers in Artificial Intelligence and Applications, Vol. 204, eds B. Apolloni, S. Bassis, A. Esposito and F. C. Morabito (Netherlands: IOS Press), 51–61.
Fava, M., and Kendler, K. S. (2000). Major depressive disorder. Neuron 28, 335–341. doi:10.1016/S0896-6273(00)00112-4
Feinberg, T., Rifkin, A., Schaffer, C., and Walker, E. (1986). Facial discrimination and emotional recognition in schizophrenia and affective disorders. Arch. Gen. Psychiatry 43, 276–279. doi:10.1001/archpsyc.1986.01800030094010
Fritzsche, A., Dahme, B., Gotlib, I. H., Joormann, J., Magnussen, H., Watz, H., et al. (2010). Specificity of cognitive biases in patients with current depression and remitted depression and in patients with asthma. Psychol. Med. 40, 815–826. doi:10.1017/S0033291709990948
Gaebel, W., and Wölwer, W. (1992). Facial expression and emotional face recognition in schizophrenia and depression. Eur. Arch. Psychiatry Clin. Neurosci. 242, 46–52. doi:10.1007/BF02190342
Ghisi, M., Flebus, G. B., Montano, A., Sanavio, E., and Sica, C. (2006). Beck Depression Inventory-II. Manuale italiano. Firenze: Organizzazioni Speciali.
Gilboa-Schechtman, E., Erhard-Weiss, D., and Jeczemien, P. (2002). Interpersonal deficits meet cognitive biases: memory for facial expressions in depressed and anxious men and women. Psychiatry Res. 113, 279–293. doi:10.1016/S0165-1781(02)00266-4
Gilboa-Schechtman, E., Foa, E., Vaknin, Y., Marom, S., and Hermesh, H. (2008). Interpersonal sensitivity and response bias in social phobia and depression: labeling emotional expressions. Cogn. Ther. Res. 32, 605–618. doi:10.1007/s10608-008-9208-8
Gollan, J. K., Pane, H., McCloskey, M., and Coccaro, E. F. (2008). Identifying differences in biased affective information processing in major depression. Psychiatry Res. 159, 18–24. doi:10.1016/j.psychres.2007.06.011
Gollan, J. K., McCloskey, M., Hoxha, D., and Coccaro, E. F. (2010). How do depressed and healthy adults interpret nuanced facial expressions? J. Abnorm. Psychol. 119, 804–810. doi:10.1037/a0020234
Gotlib, I. H., and Hammen, C. L. (1992). Psychological Aspects of Depression: Toward a Cognitive – Interpersonal Integration. Chichester: Wiley.
Gotlib, I. H., Joormann, J., Krasnoperova, E., and Yue, D. N. (2004). Attentional biases for negative interpersonal stimuli in clinical depression. J. Abnorm. Psychol. 113, 127–135. doi:10.1037/0021-843X.113.1.121
Gur, R. C., Erwin, R. J., Gur, R. E., Zwil, A. S., Heimberg, C., and Kraemer, H. C. (1992). Facial emotion discrimination: II. Behavioral findings in depression. Psychiatry Res. 2, 241–251. doi:10.1016/0165-1781(92)90116-K
Hale, W. W. (1998). Judgment of facial expressions and depression persistence. Psychiatry Res. 80, 265–274. doi:10.1016/S0165-1781(98)00070-5
Harmer, C. J., O’Sullivan, U., Favaron, E., Massey-Chase, R., Ayres, R., Reinecke, A., et al. (2009). Effect of acute antidepressant administration on negative affective bias in depressed patients. Am. J. Psychiatry 166, 1178–1184. doi:10.1176/appi.ajp.2009.09020149
Hranov, L. G. (2007). Comorbid anxiety and depression: illumination of a controversy. Int. J. Psychiatry Clin. Pract. 11, 171–189. doi:10.1080/13651500601127180
Joormann, J., and Gotlib, I. H. (2006). Is this happiness I see? Biases in the identification of emotional facial expressions in depression and social phobia. J. Abnorm. Psychol. 115, 705–714. doi:10.1037/0021-843X.115.4.705
Joormann, J., and Gotlib, I. H. (2007). Selective attention to emotional faces following recovery from depression. J. Abnorm. Psychol. 116, 80–85. doi:10.1037/0021-843X.116.1.80
Kan, Y., Mimura, M., Kamijima, K., and Kawamura, M. (2004). Recognition of emotion from moving facial and prosodic stimuli in depressed patients. J. Neurol. Neurosurg. Psychiatry 75, 1667–1671. doi:10.1136/jnnp.2004.036079
Karparova, S. P., Kersting, A., and Suslow, T. (2005). Disengagement of attention from facial emotion in unipolar depression. Psychiatry Clin. Neurosci. 59, 723–729. doi:10.1111/j.1440-1819.2005.01443.x
Kellough, J. L., Beevers, C. G., Ellis, A. J., and Wells, T. T. (2008). Time course of selective attention in clinically depressed young adults: an eye tracking study. Behav. Res. Ther. 46, 1238–1243. doi:10.1016/j.brat.2008.07.004
Klerman, G. L., and Weissman, M. M. (1992). The course, morbidity, and costs of depression. Arch. Gen. Psychiatry 49, 831–834. doi:10.1001/archpsyc.1992.01820100075013
LeMoult, J., Joormann, J., Sherdell, L., Wright, Y., and Gotlib, I. H. (2009). Identification of emotional facial expressions following recovery from depression. J. Abnorm. Psychol. 118, 828–833. doi:10.1037/a0016944
Leppänen, J. M., Milders, M., Bell, J. S., Terriere, E., and Hietanen, J. K. (2004). Depression biases the recognition of emotionally neutral faces. Psychiatry Res. 128, 123–133. doi:10.1016/j.psychres.2004.05.020
Leyman, L., De Raedt, R., Schacht, R., and Koster, E. H. W. (2007). Attentional biases for angry faces in unipolar depression. Psychol. Med. 37, 393–402. doi:10.1017/S003329170600910X
Liu, W., Huang, J., Wang, L., Gong, Q., and Chan, R. (2012). Facial perception bias in patients with major depression. Psychiatry Res. 97, 217–220. doi:10.1016/j.psychres.2011.09.021
Mandal, M. K., and Bhattacharya, B. B. (1985). Recognition of facial affect in depression. Percept. Mot. Skills 61, 13–14. doi:10.2466/pms.1985.61.1.13
McNaughton, M. L., Patterson, T., Irwin, M. R., and Grant, I. (1992). The relationship of life adversity, social support, and coping to hospitalization with major depression. J. Nerv. Ment. Dis. 180, 475–541.
Mikhailova, E. S., Vladimirova, T. V., Iznak, A. F., Tsusulkovskaya, E. J., and Sushko, N. V. (1996). Abnormal recognition of facial expression of emotions in depressed patients with major depression disorder and schizotypal personality disorder. Biol. Psychiatry 40, 697–705. doi:10.1016/0006-3223(96)00032-7
Milders, M., Bell, S., Platt, J., Serrano, R., and Runcie, O. (2010). Stable expression recognition abnormalities in unipolar depression. Psychiatry Res. 179, 38–42. doi:10.1016/j.psychres.2009.05.015
Mogg, K., Millar, N., and Bradley, B. P. (2000). Biases in eye movements to threatening facial expressions in generalized anxiety disorder and depressive disorder. J. Abnorm. Psychol. 109, 695–704. doi:10.1037/0021-843X.109.4.695
Naranjo, C., Kornreich, C., Campanella, S., Noël, X., Vandriette, Y., Gillain, B., et al. (2011). Major depression is associated with impaired processing of emotion in music as well as in facial and vocal stimuli. J. Affect. Disord. 128, 243–251. doi:10.1016/j.jad.2010.06.039
Péron, J., El Tamer, S., Grandjean, D., Leray, E., Travers, D., Drapier, D., et al. (2011). Major depressive disorder skews the recognition of emotional prosody. Prog. Neuropsychopharmacol. Biol. Psychiatry 35, 987–996. doi:10.1016/j.pnpbp.2011.01.019
Persad, S. M., and Polivy, J. (1993). Differences between depressed and nondepressed individuals in the recognition of and response to facial emotional cues. J. Abnorm. Psychol. 102, 358–368. doi:10.1037/0021-843X.102.3.358
Punkanen, M., Eerola, T., and Erkkilä, J. (2011). Biased emotional recognition in depression: perception of emotions in music by depressed patients. J. Affect. Disord. 130, 118–126. doi:10.1016/j.jad.2010.10.034
Rosenthal, R. (ed.) (2010). Managing Depressive Symptoms in Substance Abuse Clients during Early Recovery. Available at: http://store.samhsa.gov/shin/content//SMA13-4353/SMA13-4353.pdf
Rubinow, D. R., and Post, R. M. (1992). Impaired recognition of affect in facial expression in depressed patients. Biol. Psychiatry 31, 947–953. doi:10.1016/0006-3223(92)90120-O
Sanchez, A., Vazquez, C., Marker, C., LeMoult, J., and Joormann, J. (2013). Attentional disengagement predicts stress recovery in depression: an eye-tracking study. J. Abnorm. Psychol. 122, 303–313. doi:10.1037/a0031529
Schaefer, K. L., Baumann, J., Rich, B. A., Luckenbaugh, D. A., and Zarate, C. A. Jr. (2010). Perception of facial emotion in adults with bipolar or unipolar depression and controls. J. Psychiatr. Res. 44, 1229–1235. doi:10.1016/j.jpsychires.2010.04.024
Schlipf, S., Batra, A., Walter, G., Zeep, C., Wildgruber, D., Fallgatter, A., et al. (2013). Judgment of emotional information expressed by prosody and semantics in patients with unipolar depression. Front. Psychol. 4:461. doi:10.3389/fpsyg.2013.00461
Schneider, D., Regenbogen, C., Kellermann, T., Finkelmeyer, A., Kohn, N., Derntl, B., et al. (2012). Empathic behavioral and physiological responses to dynamic stimuli in depression. Psychiatry Res. 200, 294–305. doi:10.1016/j.psychres.2012.03.054
Surguladze, S. A., Young, A. W., Senior, C., Brébion, G., Travis, M. J., and Phillips, M. L. (2004). Recognition accuracy and response bias to happy and sad facial expressions in patients with major depression. Neuropsychology 18, 212–218. doi:10.1037/0894-4105.18.2.212
Suslow, T., Junghanns, K., and Arolt, V. (2001). Deficit of facial expressions of emotions in depression. Percept. Mot. Skills 92, 857–868. doi:10.2466/pms.2001.92.3.857
Teo, A., Choi, H., and Valenstein, M. (2013). Social relationships and depression: ten-year follow-up from a nationally representative study. PLoS ONE 8:e62396. doi:10.1371/journal.pone.0062396
Uekermann, J., Abdel-Hamid, M., Lehmkämper, C., Vollmoeller, W., and Daum, I. (2008). Perception of affective prosody in major depression: a link to executive functions? J. Int. Neuropsychol. Soc. 14, 552–561. doi:10.1017/S1355617708080740
Watters, A. J., and Williams, M. L. (2011). Negative biases and risk for depression; integrating self-report and emotion task markers. Depress. Anxiety 28, 703–718. doi:10.1002/da.20854
Weniger, G., Claudia Lange, C., Eckart Rüther, E., and Irle, E. (2004). Differential impairments of facial affect recognition in schizophrenia subtypes and major depression. Psychiatry Res. 128, 135–146. doi:10.1016/j.psychres.2003.12.027
Wright, S. L., Langenecker, S. A., Deldin, P. J., Rapport, L. J., Nielson, K. A., Kade, A. M., et al. (2009). Gender-specific disruptions in emotion processing in younger adults with depression. Depress. Anxiety 26, 182–189. doi:10.1002/da.20502
Zlotnick, C., Khon, R., Keitner, G., and Della Grotta, S. A. (2000). The relationship between quality of interpersonal relationships and major depressive disorder: findings from the National Comorbidity Survey. J. Affect. Disord. 59, 205–215. doi:10.1016/S0165-0327(99)00153-6
Keywords: recurrent major depressive disorder, adjustment disorder with depressed mood, basic emotions, emotion recognition task, dynamic stimuli, emotional decoding bias, communication channels
Citation: Scibelli F, Troncone A, Likforman-Sulem L, Vinciarelli A and Esposito A (2016) How Major Depressive Disorder Affects the Ability to Decode Multimodal Dynamic Emotional Stimuli. Front. ICT 3:16. doi: 10.3389/fict.2016.00016
Received: 26 May 2016; Accepted: 08 August 2016;
Published: 12 September 2016
Edited by:
Mohamed Chetouani, Pierre-and-Marie-Curie University, FranceReviewed by:
Maria Koutsombogera, Institute for Language and Speech Processing, GreeceAndrej Košir, University of Ljubljana, Slovenia
Copyright: © 2016 Scibelli, Troncone, Likforman-Sulem, Vinciarelli and Esposito. This is an open-access article distributed under the terms of the Creative Commons Attribution License (CC BY). The use, distribution or reproduction in other forums is permitted, provided the original author(s) or licensor are credited and that the original publication in this journal is cited, in accordance with accepted academic practice. No use, distribution or reproduction is permitted which does not comply with these terms.
*Correspondence: Anna Esposito, aWlhc3MuYW5uYWVzcEB0aW4uaXQ=