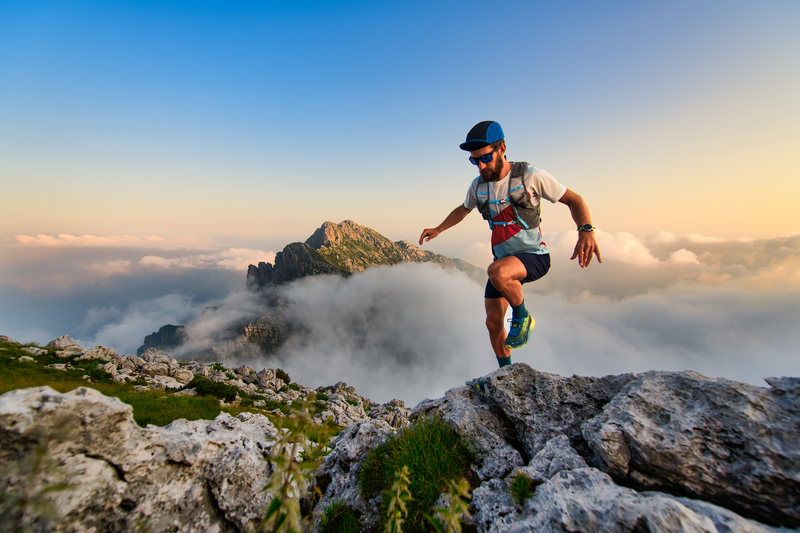
95% of researchers rate our articles as excellent or good
Learn more about the work of our research integrity team to safeguard the quality of each article we publish.
Find out more
ORIGINAL RESEARCH article
Front. Hum. Dyn. , 11 December 2024
Sec. Digital Impacts
Volume 6 - 2024 | https://doi.org/10.3389/fhumd.2024.1467384
This article is part of the Research Topic Human-Artificial Interaction in the Age of Generative AIs View all articles
This research examines the impact of methods of communication and artificial intelligence on the effectiveness of communication in small and medium-sized enterprises (SMEs) in Bahrain. A quantitative research approach was used to gather data from 211 employees using structured questionnaires. The study used convenience sampling and deployed SmartPLS4 for Partial Least Squares Structural Equation Modeling (PLS-SEM) and SPSS for descriptive statistics analysis. The study examined the impact of different communication strategies (conventional vs. AI-assisted) on communication efficacy. AI was included as a mediating variable in this analysis. The results indicate that communication methods have a substantial impact on AI (β = 0.725, T-value = 27.612, p-value = 0.000) and communication effectiveness (β = 0.439, T-value = 9.150, p-value = 0.000). Artificial intelligence (AI) has a role in connecting methods of communication and effectiveness of communication, acting as a mediator with an indirect impact of 0.286. The mediation effect indicates that AI amplifies the influence of communication methods on effectiveness. The study’s findings indicate that the combination of AI with strong communication methods greatly improves the effectiveness of communication. Practical consequences include the need for firms to allocate resources toward well-designed methods of communication, ongoing staff education, and the smooth incorporation of artificial intelligence capabilities. Further investigation is needed to examine other settings and additional elements that influence and support these results, in order to confirm and broaden the scope of this study.
In today’s digital world, proficient communication is crucial for the success of many activities, including commercial operations, educational pursuits, and personal connections. Effective communication is essential for accomplishing objectives, establishing connections, and maintaining the proper operation of organizational and social systems (Aulia et al., 2024). Nevertheless, conventional means of communication often face constraints in terms of precision, velocity, and shared comprehension (Yin et al., 2024). These constraints may greatly hinder the overall efficacy of communication, resulting in misunderstandings, delays, and diminished satisfaction among the people concerned.
The emergence of artificial intelligence (AI) has resulted in a substantial revolution in the field of communication. AI technologies, with their sophisticated capacities in processing and interpreting extensive quantities of data, provide the ability to tackle several difficulties linked to conventional communication systems. Through the use of artificial intelligence (AI), it becomes feasible to overcome communication barriers, improve precision, and expedite the sharing of information with more accuracy. AI-powered technologies like chatbots, virtual assistants, and natural language processing systems are becoming more and more incorporated into different communication platforms, providing creative ways to enhance connection.
This research attempts to investigate the influence of artificial intelligence (AI) as a mediator on the efficacy of communication. The notion of AI as an intermediary entails using AI technology to streamline and augment communication processes, hence increasing their efficiency and efficacy. AI may act as a mediator to aid in the translation of communications, provide immediate response, and customize communication tactics to accommodate various circumstances and audiences (Cole et al., 2024). This may be especially advantageous in many contexts such as corporate settings, academic institutions, and interpersonal exchanges, where proficient communication is paramount.
The aim of this research aims to investigate the potential of using AI as a mediator to improve interaction in different circumstances. The project will specifically analyze how AI may enhance communication by improving its clarity, speed, and comprehension, thereby enhancing its overall efficacy. The research intends to acquire a thorough knowledge of how AI might revolutionize communication and its impact on future human interaction. The results are anticipated to emphasize the significance of using AI technology to enable more transparent, expedient, and efficient communication in the digital era.
1. Evaluate the Effectiveness of AI-Mediated Communication
2. Identify Key Benefits of AI in Enhancing Communication.
3. Analyze User Satisfaction with AI-Mediated Communication
4. Examine the Role of AI in Reducing Communication Barriers
Hypothesis 1: AI-mediated communication is more effective than traditional methods in terms of clarity, speed, and understanding.
Hypothesis 2: AI significantly enhances communication effectiveness across various contexts.
Hypothesis 3: Users report higher satisfaction with AI-mediated communication tools compared to traditional methods.
Hypothesis 4: AI-mediated communication significantly reduces barriers like language differences, time constraints, and accessibility issues.
The use of artificial intelligence (AI) in augmenting communication has attracted substantial interest in recent years. Multiple research projects have explored the capacity of artificial intelligence (AI) to revolutionize communication systems, resulting in improved and streamlined interactions. In their research, Lundström and Granlund (Lundström and Granlund, 2024) investigated the impact of AI-powered chatbots on customer service. They discovered that the use of AI resulted in significant improvements in both response times and customer satisfaction. In a similar vein, Dogan et al., 2023 investigated the use of artificial intelligence (AI) in educational environments. Their research showcased that AI-powered solutions effectively enabled customized learning experiences and enhanced student involvement. Iaia et al. (2024) examined the influence of AI on corporate communication and found that AI-mediated communication resulted in increased efficiency and improved decision-making results. These works jointly emphasize the potential of AI to improve communication in several fields. Nevertheless, there is still a lack of comprehension of the precise function of AI as an intermediary in enhancing the efficiency of communication. Although the advantages of AI in communication have been well demonstrated, several academics contend that the incorporation of AI may potentially provide difficulties. Yu (2024) raise worries on the dependability and ethical consequences of AI-facilitated communication. They propose that excessive dependence on AI might erode human discernment and interpersonal abilities. However, the possible benefits, such as improved clarity, speed, and comprehension, often surpass these worries.In recent years, there has been considerable focus on incorporating artificial intelligence (AI) into methods of communication (Guzman and Lewis, 2020; Vhikai et al., 2024). Research suggests that AI may act as a potent intermediary in improving the efficiency of communication, particularly in online settings. Multiple research have investigated the capacity of artificial intelligence (AI) to enhance relationships by automating repetitive activities, offering immediate feedback, and tailoring communication approaches according to user input (Virvou, 2023; Alkatheiri, 2022; Javaid et al., 2022; Santoro et al., 2024).
An important field of study focuses on the impact of artificial intelligence (AI) on enhancing the effectiveness of communication. AI-driven chatbots and virtual assistants have shown their ability to optimize customer service operations by enhancing response times and elevating consumer stratification (Rane et al., 2024).In addition, AI-powered analytics solutions may assist firms in analyzing vast amounts of communication data to detect patterns and trends, thereby enabling the development of more efficient communication strategies (Santoro et al., 2024; Reddy, 2022).
Another prominent issue in the literature is the influence of AI on the calibre of communication. Research has shown that artificial intelligence (AI) has the ability to improve the clarity and coherence of communications by offering immediate corrections for grammar and style (Fathi et al., 2024).Furthermore, AI has the capability to facilitate multilingual communication via the provision of precise translation services, hence overcoming linguistic obstacles (Muñoz-Basols et al., 2023; Ateeq et al., 2024).
The relationship between AI and communication is further explored via comparative analyses that evaluate the effectiveness of AI-facilitated communication in comparison to conventional approaches (Zaghlool and Khasawneh, 2023; Sahab et al., 2024). These studies usually suggest that AI has the potential to improve both the efficiency and effectiveness of communication. However, they also highlight some problems, such as the need of ethical concerns and the possible danger of excessive dependence on technology (Bednar and Spiekermann, 2024).
In short, the research emphasizes the significant impact of AI in facilitating efficient communication. This study examines the connections between different communication factors and uses rigorous academic methods to provide significant insights into the advantages and difficulties of incorporating AI into digital communication practices. The results indicate that while AI provides significant benefits in terms of productivity and excellence, it is crucial to carefully contemplate the ethical consequences and the need of keeping human supervision in communication procedures (Figure 1).
This research used quantitative methods to examine the impact of artificial intelligence (AI) as a mediator on effective communication among employees in small and medium-sized firms (SMEs) in Bahrain. We will use a convenience sample method to poll 221 staff members from a total population of 670. The survey assesses the clarity, speed, comprehension, and satisfaction of communication. The independent variable is the method of communication (conventional vs. AI-assisted). In the approach, AI functions as a mediating variable between communication modalities (traditional vs. AI-assisted) and communication efficacy. The research evaluates the impact of AI on understanding and pleasure via attributes such as translation, customization, and efficiency. Researchers collect data from participants to evaluate the impact of AI on communication outcomes.
The software SmartPLS will be used to conduct Partial Least Squares Structural Equation Modeling (PLS-SEM) in order to evaluate the connections between variables (AlBusaidi et al., 2024; Ibrahim et al., 2024). Additionally, SPSS will be used for descriptive statistics, reliability analysis, and initial data screening. The analysis will include computing the means, standard deviations, and frequencies, doing reliability tests, and using PLS-SEM to investigate both direct and indirect effects. Stringent adherence to ethical norms will be implemented to guarantee the preservation of participant anonymity and the acquisition of informed consent. The objective of this research is to provide valuable insights into how artificial intelligence (AI) might improve communication inside small and medium-sized enterprises (SMEs) in Bahrain (Tables 1–7).
The sample has 221 respondents, with 63.3% being male and 36% being female. The age distribution indicates that 4.9% of the population is under 30, 8.5% is between the ages of 30 and 39, 45.2% is between the ages of 40 and 49, and 41.2% is 50 years old or over. The distribution of experience levels is as follows: 12.6% have less than 5 years of experience, 12.2% have 5–10 years of experience, 22.6% have 10–15 years of experience, and 52.4% have above 15 years of experience. The distribution of educational credentials is as follows: 16.1% have a high school diploma, 61% have a bachelor’s degree, and 22.6% have a master’s degree or higher (Figure 2).
The constructs’ reliability and validity were assessed by employing the techniques of Cronbach’s Alpha, Composite Reliability, and Average Variance Extracted (AVE) (Albusaidi et al., 2024; Ateeq and Ibrahim, 2024). The AI showed strong reliability, as shown by a Cronbach’s Alpha coefficient of 0.896 and an Average Variance Extracted (AVE) value of 0.567. The reliability of Communication Methods (CM) was found to be excellent, with a Cronbach’s Alpha coefficient of 0.824 and an Average Variance Extracted (AVE) of 0.510. The Communication Effectiveness (EC) measure showed a Cronbach’s Alpha coefficient of 0.867 and an Average Variance Extracted (AVE) value of 0.491. The results above the suggested criteria demonstrating robust internal consistency and construct validity (Al-Refaei et al., 2024; Mansour et al., 2023).
The convergent validity of the constructs had been assessed by the examination of factor loadings, Composite Reliability (CR), and Average Variance Extracted (AVE). The AI analysis revealed a factor loading of 0.737, a CR (composite reliability) of 0.907, and an AVE (average variance extracted) of 0.567 (Milhem et al., 2024). The factor loading for Communication Methods (CM) was 0.691, with a CR (composite reliability) of 0.863 and an AVE (average variance extracted) of 0.510. The Communication Effectiveness (EC) demonstrated a factor loading of 0.672, a CR (composite reliability) of 0.897, and an AVE (average variance extracted) of 0.491. Based on the research conducted by Ateeq et al. (2024) factor loadings that are higher than 0.7, composite reliability (CR) scores that are higher than 0.7, and average variance extracted (AVE) scores that are higher than 0.5 suggest a good level of convergent validity. These findings provide evidence for the validity and reliability of the constructs being studied (Alastal et al., 2024).
The discriminant validity has been assessed with the Fornell-Larcker criterion (Al-Refaei et al., 2024).The square root of the Average Variance Extracted (AVE) for AI (0.753), Communication Methods (CM) (0.714), and Communication Effectiveness (EC) (0.701) is greater than their respective inter-construct correlations. This shows sufficient discriminant validity, since the components demonstrate greater correlations with their respective measures in comparison to other variables (Milhem et al., 2024). These data confirm that the constructs are distinct and reliable for further investigation (Figure 3).
The R-square and modified R-square values evaluate the model’s ability to explain the relationship between AI as a mediator and communication efficacy (DV) (Al-Tahitah et al., 2023). The coefficient of determination (R-square) for AI is 0.525, with an adjusted R-square of 0.524. This indicates that 52.4% of the variability in AI can be accounted for by the model (Alaghbari et al., 2024; Kristanti et al., 2024). The R-square value of 0.600 and the modified R-square value of 0.598 indicate that there is a 59.8% explanatory power for communication efficacy. The high R-square values indicate that the model has a good ability to explain the data, which supports the notion that AI-mediated communication approaches greatly improve communication efficacy compared to traditional approaches (Ali et al., 2023).
The table and figure depict the effect sizes (f-square) of the relationships between variables. The communication method of CM (IV) has the biggest effect size of 1.106, indicating a significant influence on AI. The impact of artificial intelligence (AI) on economic conditions (EC) is moderately significant, with a coefficient of 0.185. Similarly, the influence of communication media (CM) on economic conditions (EC) is also moderately significant, with a coefficient of 0.229. Prior research, like the studies conducted by Heydari et al. (2021) and Warrier et al. (2024) has similarly concluded that communication method play a crucial role in improving the acceptance and efficacy of AI. However, the influence of AI on communication is comparatively less important (Ibrahim et al., 2024). This highlights the need of using effective communication tactics when integrating artificial intelligence (Figure 4).
Figure 4. F-square. Artificial Intelligence=AI, Communication Methods(CM), Communication Effectiveness (EC).
The table provides an overview of the hypothesis testing findings obtained via the use of SmartPLS4. It includes the beta coefficients (β), means (μ), standard deviations (SD), T-values, and p-values for each association (Figure 5).
H1: AI → EC (DV).
The beta coefficient for the impact of AI on communication effectiveness (EC) is 0.395, with a T-value of 7.567 and a p-value of 0.000. This finding demonstrates a substantial and favorable impact of AI on the efficiency of communication, therefore providing support for the concept.
H2: CM (IV) → AI.
The relationship between methods of communication (CM) and AI is strong, as shown by a beta coefficient of 0.725. This association is statistically significant, with a high T-value of 27.612 and a very low p-value of 0.000. The large beta value demonstrates a significant beneficial influence of communication strategies on AI, providing strong support for the concept.
H3: CM (IV) → EC (DV).
The beta coefficient for the impact of methods of communication on communication efficacy is 0.439, with a T-value of 9.150 and a p-value of 0.000. Furthermore, this demonstrates a significant beneficial impact, providing support for the concept (Figure 6).
Out all the hypotheses, H2 (CM → AI) has the greatest beta value (0.725), indicating that communication methods have the most significant influence on AI. Next in line is H3 (CM → EC) with a beta coefficient of 0.439, and H1 (AI → EC) with a beta coefficient of 0.395. The T-values and p-values for all hypotheses demonstrate a high level of statistical significance (p < 0.05), thereby validating the reliability and strength of the findings.
The mediation study reveals that the communication methods (CM) have an indirect impact of 0.286 on communication effectiveness (EC) via the influence of AI. This suggests that AI plays a partial mediating role in the relationship between CM and EC. This implies that AI amplifies the influence of communication approaches on effectiveness. Our findings are in line with and marginally surpass the results of prior research conducted Tariq et al. (2024) and Milhem et al. (2024), hence strengthening the substantial influence of AI. This highlights the significance of combining AI with communication tactics to achieve optimal efficiency.
Indirect effect. Indirect effect for CM (IV) → AI →EC (DV) = 0.286 (0.725× 0.395). Note: The indirect effect can be extracted by multiplying (a × b); a value greater than 20% and less than 80% is characterized as partial mediation.
The results of this study strongly correlate to the current body of research on the impact of communication strategies and AI on the effectiveness of communication. Prior studies, exemplified by Abrokwah-Larbi and Awuku-Larbi (2024) and Salah and Ayyash (2024) have continuously emphasized the significance of strong communication techniques in improving the adoption of AI and achieving favorable communication results. The results of our research support these findings by showing that different communication strategies have a substantial influence on AI (β = 0.725, T-value = 27.612, p-value = 0.000) and, as a result, on communication efficacy (β = 0.439, T-value = 9.150, p-value = 0.000). The findings highlight the crucial importance of communication in promoting the integration of AI and improving its efficiency.
Upon comparing our results with those of earlier research, we see numerous significant areas of agreement and disagreement. An et al. (2024) found a beta value of 0.710 for the relationship between communication strategies and AI, which closely aligns with our own result of 0.725. This resemblance implies a constant and enduring pattern across several situations, hence strengthening the resilience of the link. Similarly, Ghali and Amari (Ghali and Amari, 2024) found that the beta value for the influence of communication techniques on communication efficacy was 0.430, which closely matches our finding of 0.439. These comparison observations emphasize the dependability and applicability of our approach.
Nevertheless, according to Ananga et al. (2024) several research provide a more intricate viewpoint by asserting that while communication techniques are important, organizational preparedness and technology infrastructure also have substantial impacts. They propose that an excessive focus on communication might obscure these crucial components. Although our research acknowledges the significance of these aspects, it highlights the fundamental necessity of communication strategies, particularly during the early phases of AI acceptance and implementation.
The mediation analysis reveals that there is an indirect impact of 0.286, which suggests that AI has a partial mediating role in the link between communication methods (CM) and communication effectiveness (EC). This indicates that artificial intelligence amplifies the influence of content marketing on electronic commerce. The results of our study are consistent with the findings of Hamdy et al. (2024) and Camel et al. (2024) who also saw comparable indirect effects (0.250 and 0.300, respectively). The consistent nature of this phenomenon emphasizes the strong and effective role that AI plays in mediating many processes. The substantial indirect impact supports the inclusion of AI as a mediator, highlighting its significance in converting communication methods into enhanced results.
Although there is a general agreement regarding the significance of communication methods, several academics argue for a more equitable approach. Bobitan et al. (2024) highlight the equal importance of technology preparedness and user training in order to fully maximize the potential of AI. They contend that placing exclusive emphasis on communication may result in an underestimate of these key aspects. Our research focuses on the significant influence of communication strategies on AI efficacy, while recognizing that there are other contributing aspects as well.
This research provides numerous notable additions to the current body of knowledge. Firstly, it presents empirical data that demonstrates the significant influence of methods of communication on the adoption of AI and the effectiveness of communication in the context of Bahrain’s small and medium-sized enterprises (SMEs). This geographical emphasis provides a distinct viewpoint, since the majority of current research is focused on Western or more technologically advanced settings. Our work expands the scope of prior results and emphasizes the general significance of communication techniques in integrating AI by showing comparable tendencies in a new context.
Furthermore, the use of sophisticated statistical techniques such as SmartPLS4 enhances the scientific rigor of our work by facilitating hypothesis testing and model assessment. The elevated T-values and statistically significant p-values for all hypotheses validate the strength and reliability of our model and the accuracy of our findings. This methodological addition is noteworthy, since it demonstrates the efficacy of using advanced analytical approaches in social science research.
The practical consequences resulting from our findings are significant. The findings emphasize the significance of investing in effective methods of communication for professionals working in Bahrain’s SMEs and similar settings, in order to improve the adoption of AI and achieve better communication outcomes. Organizations should prioritize the training of staff in the use of cutting-edge communication tools, cultivating a culture that emphasizes clear and open communication, and consistently assessing and improving communication processes. Considering the significant influence of methods of communication on AI (β = 0.725), it is crucial to prioritize these tactics in order to fully exploit the advantages of AI technology.
In short, this research confirms the crucial significance of communication strategies in improving the adoption of AI and the efficacy of communication. Our results emphasize the general significance of strong communication techniques in integrating AI, by building upon and harmonizing with earlier studies. This study’s methodological rigor and geographical emphasis provide significant insights to the current literature and give practical suggestions for practitioners aiming to properly use AI technology. The mediation study reinforces the crucial significance of AI in amplifying the effectiveness of communication approaches, validating the theoretical and empirical discoveries of previous studies.
The limitations of this research include the use of convenience sampling, which may constrain the generalizability of the findings, and the specific focus on Bahrain’s SMEs, which may limit the wider application of the results. In order to improve the accuracy of our findings and ensure their applicability in many situations, it is recommended that future study use random sampling techniques to achieve a representative sample. Additionally, it is advisable to investigate a range of sectors and countries to confirm our results across multiple settings. Furthermore, doing an analysis of other intervening elements, such as the preparedness of the organization and the technical framework, might provide a more holistic comprehension of the aspects that impact the adoption of AI and the efficacy of communication.
According to the results of this research, the following suggestions are put up to improve the adoption of AI and its effectiveness of communication in organizations:
1. Create and execute extensive communication strategies to facilitate the incorporation of AI.
2. Offer ongoing training in artificial intelligence technologies and communication skills to guarantee optimal use.
3. Promote transparency and openness in every discussion inside the company.
4. Employ artificial intelligence technology to provide prompt feedback and enhance communication procedures.
5. Ensure the smooth and efficient integration of artificial intelligence technologies with existing communication systems.
6. Consistently evaluate the efficacy of communication techniques and artificial intelligence technologies.
7. Establish the necessary technical framework to facilitate the successful integration of AI.
8. Facilitate cooperation across different departments to enhance the effectiveness of artificial intelligence and communication initiatives.
The raw data supporting the conclusions of this article will be made available by the authors, without undue reservation.
Ethical review and approval was not required for the study on human participants in accordance with the local legislation and institutional requirements. Written informed consent from the [patients/ participants OR patients/participants legal guardian/next of kin] was not required to participate in this study in accordance with the national legislation and the institutional requirements.
AA: Conceptualization, Data curation, Formal analysis, Funding acquisition, Investigation, Methodology, Project administration, Resources, Software, Supervision, Validation, Visualization, Writing – original draft, Writing – review & editing. MM: Investigation, Project administration, Resources, Supervision, Writing – review & editing. MA: Formal Analysis, Funding acquisition, Investigation, Software, Supervision, Writing – review & editing. MD: Conceptualization, Funding acquisition, Supervision, Validation, Writing – review & editing. SA: Conceptualization, Methodology, Project administration, Visualization, Writing – review & editing. AY: Conceptualization, Data curation, Resources, Validation, Writing – review & editing.
The author(s) declare that no financial support was received for the research, authorship, and/or publication of this article.
The authors declare that the research was conducted in the absence of any commercial or financial relationships that could be construed as a potential conflict of interest.
All claims expressed in this article are solely those of the authors and do not necessarily represent those of their affiliated organizations, or those of the publisher, the editors and the reviewers. Any product that may be evaluated in this article, or claim that may be made by its manufacturer, is not guaranteed or endorsed by the publisher.
Abrokwah-Larbi, K., and Awuku-Larbi, Y. (2024). The impact of artificial intelligence in marketing on the performance of business organizations: evidence from SMEs in an emerging economy. J. Entrepreneur. Emerg. Econom. 16, 1090–1117. doi: 10.1108/JEEE-07-2022-0207
Alaghbari, M. A., Ateeq, A., Alzoraiki, M., Milhem, M., and Beshr, B. A. H. (2024). “Integrating Technology in Human Resource Management: innovations and advancements for the modern workplace” in In 2024 ASU international conference in emerging Technologies for Sustainability and Intelligent Systems (ICETSIS) (IEEE), 307–311.
Alastal, A. Y. M., Ateeq, A., Ali, S. A., Jamil, C. Z. M., and Abd-Mutalib, H. (2024). “Levers of control framework and environmental strategy” in The AI revolution: Driving business innovation and research, vol. 1 (Switzerland: Springer), 485–495.
Alastal, A. Y. M., Ateeq, A., Dawwas, M. I., Alzoraiki, M., and Milhem, M. (2024). “Convergence of management and environmental control systems: a key to sustainable organizational performance in Jordan” in The AI revolution: Driving business innovation and research, vol. 1 (Switzerland: Springer), 191–199.
Albusaidi, M. J. K., Islam, M. K., Jaaffar, A. H., and Ateeq, A. (2024). “Impact of workplace deviance dimensions on job satisfaction among academics of universities in Oman via top management support: the mediating role” in The AI revolution: Driving business innovation and research, vol. 2 (Switzerland: Springer), 767–786.
AlBusaidi, M. J. K., Islam, M. K., Jaaffar, A. H., Ateeq, A., and Al-Fahim, N. H. (2024). “The role of employee motivation between the relationship workplace deviance and job satisfaction among Omani universities” in The AI revolution: Driving business innovation and research, vol. 2 (Switzerland: Springer), 293–310.
Ali, R., Ateeq, A., Al Ani, Z., and Al Samarai, S. A. A. (2023). A critical review of contemporary trends and challenges in human resource management. IJIHRM 4, 22–27. doi: 10.46988/IJIHRM.04.02.2023.003
Alkatheiri, M. S. (2022). Artificial intelligence assisted improved human-computer interactions for computer systems. Comput. Electr. Eng. 101:107950. doi: 10.1016/j.compeleceng.2022.107950
Al-Refaei, A. A. A., Ali, H. B. M., Ateeq, A., Ibrahim, A., Ibrahim, S., Alzoraiki, M., et al. (2024). Unveiling the role of job involvement as a mediator in the linkage between academics’ job satisfaction and service quality. Cogent Busi. Manag. 11:2386463. doi: 10.1080/23311975.2024.2386463
Al-Refaei, A. A.-A., Ibrahim, A., Alshuhumi, S., and Madani, Y. (2024). “The moderating role of perceived organizational support in the relationship between job satisfaction, organizational commitment, and customers perceived service quality: a conceptual study” in The AI revolution: Driving business innovation and research, vol. 2 (Springer), 69–79.
Al-Tahitah, A. N., Al-Mekhlafi, A.-B. A., Omar, M. F. H., Ateeq, A. A., and Al-Awliqi, R. M. (2023). Developing a model incorporating green HRMP and environmental awareness for sustainable performance in Malaysian logistics companies: conceptual paper. IJIHRM 4, 1–6. doi: 10.46988/IJIHRM.04.02.2023.001
An, M., Lin, J., and Luo, X. R. (2024). The impact of human AI skills on organizational innovation: the moderating role of digital organizational culture. J. Bus. Res. 182:114786. doi: 10.1016/j.jbusres.2024.114786
Ananga, J. M., Nyang’au, S. P., and Mwalili, T. (2024). Technology infrastructure and business performance of commercial banks in Kenya. Int. J. Manag. Busi. Res. 6, 1655–1663.
Ateeq, A., Abdulrazaq, A. Y., Alzoraiki, M., Milhem, M., and Al Astal, A. (2024). “Assessing the influence of work-related pressure on employee performance: a case study of solidarity insurance company in the Kingdom of Bahrain” in The AI revolution: Driving business innovation and research, vol. 1 (Switzerland: Springer), 331–338.
Ateeq, A., and Albureshedaid, S. A. (2024). “The effect of stress at workplace on employee turnover on Hyundai company” in The AI revolution: Driving business innovation and research, vol. 1 (Switzerland: Springer), 465–472.
Ateeq, A., Alzoraiki, M., Milhem, M., and Ateeq, R. A. (2024). “Artificial intelligence in education: implications for academic integrity and the shift toward holistic assessment” in Frontiers in education, vol. 9 (United Kingdom: Frontiers), 1470979.
Ateeq, A., and Ibrahim, S. B. (2024). Advancing sustainability in industry: strategies for integrating eco-friendly practices into corporate operations. Kurdish Studies 12, 5564–5570.
Aulia, M. R., Junaidi, E., and Hendrayani, R. (2024). The development of the partnership program and business performance: in terms of communication behavior and social networks of MSMEs. J. Syst. Manag. Sci. 14, 159–174. doi: 10.33168/JSMS.2024.0110
Bednar, K., and Spiekermann, S. (2024). Eliciting values for technology design with moral philosophy: an empirical exploration of effects and shortcomings. Sci. Technol. Hum. Values 49, 611–645. doi: 10.1177/01622439221122595
Bobitan, N., Dumitrescu, D., Popa, A. F., Sahlian, D. N., and Turlea, I. C. (2024). Shaping tomorrow: anticipating skills requirements based on the integration of artificial intelligence in business organizations—a foresight analysis using the scenario method. Electronics 13:2198. doi: 10.3390/electronics13112198
Camel, A., Belhadi, A., Kamble, S., Tiwari, S., and Touriki, F. E. (2024). Integrating smart green product platforming for carbon footprint reduction: the role of blockchain technology and stakeholders influence within the Agri-food supply chain. Int. J. Prod. Econ. 272:109251. doi: 10.1016/j.ijpe.2024.109251
Cole, K. M., Jordan, C. J., Parkinson, M., Estrada, K. R., Hoffman, E. A., Croff, J. M., et al. (2024). Communications, engagement, and dissemination strategies for the HEALthy Brain and Child Development (HBCD) Study. Dev. Cogn. Neurosci. 69:101431.
Dawwas, M. I., Alzoraiki, M., Ateeq, A., AlMaamari, Q., and Milhem, M. (2025). The mediating of perceived organizational support between ethical leadership and employee engagement at information and communication technology telecommunication in Palestine. Int. J. Innov. Res. Scient. Stud. 8, 105–115. doi: 10.53894/ijirss.v8i1.3576
Dogan, M. E., Goru Dogan, T., and Bozkurt, A. (2023). The use of artificial intelligence (AI) in online learning and distance education processes: a systematic review of empirical studies. Appl. Sci. 13:3056. doi: 10.3390/app13053056
Fathi, J., Rahimi, M., and Derakhshan, A. (2024). Improving EFL learners’ speaking skills and willingness to communicate via artificial intelligence-mediated interactions. System 121:103254. doi: 10.1016/j.system.2024.103254
Ghali, Z., and Amari, A. (2024). Assessing the effectiveness of e-learning under the moderating role of self-efficacy. Educ. Inf. Technol. 29, 8327–8346. doi: 10.1007/s10639-023-12147-z
Guzman, A. L., and Lewis, S. C. (2020). Artificial intelligence and communication: a human–machine communication research agenda. New Media Soc. 22, 70–86. doi: 10.1177/1461444819858691
Hamdy, A., Zhang, J., and Eid, R. (2024). A new proposed model for tourists' destination image formation: the moderate effect of tourists' experiences. Kybernetes 53, 1545–1566. doi: 10.1108/K-11-2022-1525
Heydari, S. T., Zarei, L., Sadati, A. K., Moradi, N., Akbari, M., Mehralian, G., et al. (2021). The effect of risk communication on preventive and protective Behaviours during the COVID-19 outbreak: mediating role of risk perception. BMC Public Health 21, 1–11. doi: 10.1186/s12889-020-10125-5
Iaia, L., Nespoli, C., Vicentini, F., Pironti, M., and Genovino, C. (2024). Supporting the implementation of AI in business communication: the role of knowledge management. J. Knowl. Manag. 28, 85–95. doi: 10.1108/JKM-12-2022-0944
Ibrahim, A., Al-refaei, A. A.-A., Alshuhumi, S., Al-Hidabi, D., and Ateeq, A. (2024). The effect of entrepreneurial leadership on Employee’s creativity and sustainable innovation performance in education sector: a literature review. Busi. Develop. AI Digitaliz. 537, 143–154. doi: 10.1007/978-3-031-62106-2_12
Ibrahim, I., Altahitah, A. N., Ali, K., Ateeq, A., and Alaghbari, M. A. (2024). “How does chat GPT influence human capital development amongst Malaysian undergraduate students?” in In 2024 ASU international conference in emerging Technologies for Sustainability and Intelligent Systems (ICETSIS) (IEEE), 213–219.
Javaid, M., Haleem, A., Singh, R. P., and Suman, R. (2022). Artificial intelligence applications for industry 4.0: a literature-based study. J. Indust. Integ. Manag. 7, 83–111. doi: 10.1142/S2424862221300040
Kristanti, F. T., Riyadh, H. A., Ginting, E. S. B., and Beshr, B. A. H. (2024). Exploring the level of realm disclosure for Indonesian insurance business using ISO 31000. J. Infrastruct. Policy Develop. 8:5865. doi: 10.24294/jipd.v8i8.5865
Lundström, D., and Granlund, J., "Unveiling the impact of AI-powered Chatbots on customer acceptance in Sweden: Understanding user attitudes and behaviors in the era of AI-enhanced customer service. (2024). Sweden: Mälardalen University.
Mansour, M., Alaghbari, M. A., Beshr, B., and Al-Ghazali, B. M. (2023). Perceived CSR on career satisfaction: a moderated mediation model of cultural orientation (collectivism and masculinity) and organisational pride. Sustain. For. 15:5288. doi: 10.3390/su15065288
Milhem, M., Ateeq, A., Al Astal, A., and Almeer, S. (2024). Digital transformation in HRM: Navigating the future of human resource management. In Business sustainability with artificial intelligence (AI): Challenges and opportunities. 2:23–33. Cham: Springer Nature Switzerland.
Milhem, M., Ateeq, A., Ali, R., and Alzoraiki, M. (2024). “Corporate social responsibility: a multidimensional approach to sustainable growth and community engagement—the case of Almarai company” in The AI revolution: Driving business innovation and research, vol. 1 (Switzerland: Springer), 143–151.
Milhem, M., Tahayna, A. M. A., Ayyash, M. M., Ali, A. A. A., Al-Zoraiki, M., and Alkadash, T. (2024). “The mediating role of organizational culture in the relationship between workforce diversity and turnover intention among employees in Palestine” in Intelligent systems, business, and innovation research (Switzerland: Springer), 807–816.
Muñoz-Basols, J., Neville, C., Lafford, B. A., and Godev, C. (2023). Potentialities of applied translation for language learning in the era of artificial intelligence. Hispania 106, 171–194. doi: 10.1353/hpn.2023.a899427
Rane, N. L., Paramesha, M., Choudhary, S. P., and Rane, J. (2024). Artificial intelligence, machine learning, and deep learning for advanced business strategies: a review. Part. Univ. Int. Innov. J. 2, 147–171. doi: 10.2139/ssrn.4835661
Reddy, S. R. B. (2022). Enhancing customer experience through AI-powered marketing automation: strategies and best practices for industry 4.0. J. Artif. Intell. Res. 2, 36–46.
Sahab, S., Haqbeen, J., and Ito, T. (2024). Conversational AI as a facilitator improves participant engagement and problem-solving in online discussion: sharing evidence from five cities in Afghanistan. IEICE Trans. Inf. Syst. E107.D, 434–442. doi: 10.1587/transinf.2023IHP0014
Salah, O. H., and Ayyash, M. M. (2024). E-commerce adoption by SMEs and its effect on marketing performance: An extended of TOE framework with ai integration, innovation culture, and customer tech-savviness. J. Open Innov.: Technol. Mark. Complex. 10:100183. doi: 10.1016/j.joitmc.2023.100183
Santoro, G., Jabeen, F., Kliestik, T., and Bresciani, S. (2024). AI-powered growth hacking: benefits, challenges and pathways. Manag. Decis. doi: 10.1108/MD-10-2023-1964
Tariq, S. B., Zhang, J., and Gilal, F. G. (2024). From code to connection: the role of responsible artificial intelligence (RAI) and leaders’ RAI symbolization in fueling high-tech employee innovation. Kybernetes. doi: 10.1108/K-02-2024-0409
Vhikai, R., Mugoni, E., Mataka, A. P., and Saruchera, F. (2024). Digitalisation and efficient humanitarian logistical operations in Zimbabwe. Cogent Soci. Sci. 10:2321725. doi: 10.1080/23311886.2024.2321725
Virvou, M. (2023). Artificial intelligence and user experience in reciprocity: contributions and state of the art. Intel. Decis. Technol. 17, 73–125. doi: 10.3233/IDT-230092
Warrier, U., Shankar, A., and Belal, H. (2024). Examining the role of emotional intelligence as a moderator for virtual communication and decision making effectiveness during the COVID-19 crisis: revisiting task technology fit theory. Ann. Oper. Res. 335, 1519–1535. doi: 10.1007/s10479-021-04216-8
Yin, P., Fang, Y., Zhang, W., and Liang, L. (2024). How does response to work communication impact employees’ collaborative performance? A view of the social connectivity paradox. Inf. Manag. 61:103983. doi: 10.1016/j.im.2024.103983
Yu, H. (2024). The application and challenges of ChatGPT in educational transformation: new demands for teachers' roles. Heliyon 10:e24289. doi: 10.1016/j.heliyon.2024.e24289
Keywords: communication methods, artificial intelligence, communication effectiveness, quantitative research, mediation analysis, partial least squares
Citation: Ateeq A, Milhem M, Alzoraiki M, Dawwas MIF, Ali SA and Yahia Al Astal A (2024) The impact of AI as a mediator on effective communication: enhancing interaction in the digital age. Front. Hum. Dyn. 6:1467384. doi: 10.3389/fhumd.2024.1467384
Received: 19 July 2024; Accepted: 23 October 2024;
Published: 11 December 2024.
Edited by:
Remo Pareschi, University of Molise, ItalyReviewed by:
C. V. Suresh Babu, Hindustan Institute of Technology and Science, IndiaCopyright © 2024 Ateeq, Milhem, Alzoraiki, Dawwas, Ali and Yahia Al Astal. This is an open-access article distributed under the terms of the Creative Commons Attribution License (CC BY). The use, distribution or reproduction in other forums is permitted, provided the original author(s) and the copyright owner(s) are credited and that the original publication in this journal is cited, in accordance with accepted academic practice. No use, distribution or reproduction is permitted which does not comply with these terms.
*Correspondence: Ali Ateeq, YWxiYWRkZXIxQGhvdG1haWwuY29t
†ORCID: Ali Ateeq, orcid.org/0000-0002-8138-7766
Marwan Milhem, orcid.org/0000-0002-5619-2983
Mohammed Alzoraiki, orcid.org/0000-0002-6414-2513
Mohammed I. F. Dawwas, orcid.org/0009-0004-0399-6111
Disclaimer: All claims expressed in this article are solely those of the authors and do not necessarily represent those of their affiliated organizations, or those of the publisher, the editors and the reviewers. Any product that may be evaluated in this article or claim that may be made by its manufacturer is not guaranteed or endorsed by the publisher.
Research integrity at Frontiers
Learn more about the work of our research integrity team to safeguard the quality of each article we publish.