- Department of Psychology, Nnamdi Azikiwe University, Awka, Nigeria
Internal displacement figures in conflicted affected regions of Nigeria is a significant problem that poses a risk of humanitarian crises accounted for by poor and a neglected emergency response system. The present study analyzed annual conflict/violence-induced internal displacement trends in Nigeria from 2009 to 2021 and predicted the annual figures for the next 10 years. Using the R software, the Autoregressive Integrated Moving Average (ARIMA) was adopted for analysis, while model (0, 0, 1) was chosen as the best fitting model with the minimum AIC coefficient. From the ARIMA forecast, 2022 showed a 4% decrease in displacement figures, further decreasing by 7% in 2023. The figures remained constant for the rest of the forecasted years, showing neither increases nor decreases from 2024 to 2031. Close observation revealed the overlapping trends between increased annual internal displacements and pre-election periods in Nigeria. This study will help inform humanitarian bodies on the significance of early detection and preparation for future displacement crises while considering the cycle of ethno-religious-related electoral violence. Furthermore, future studies should compare the accuracy of ARIMA with other models to ensure its validity using a similar sample.
Introduction
Humanitarian crises are global problems that greatly contribute to death and suffering, a phenomenon that is caused by disaster or conflict. The latter is a factor that is engineered by hostile parties through armed conflict and violence. According to Relief Web (2021), there will be about 274 million people globally who will need humanitarian assistance and protection by the end of 2022, a figure that increased from 235 million people the previous year. Nigeria is being challenged with adversities that adulterate the very essence of unity and peace among its citizenry. Violence perpetrated through several means and not limited to terrorism, ethnic conflict, and political conflict has claimed many lives and displaced millions of citizens from their host communities (Gwadabe et al., 2018; Letswa and Isyaku, 2018; United Nations High Commissioner for Refugees, 2020). Over 3 million persons live in internal displacements in Nigeria (Internal Displacement Monitoring Center, 2021a), fueling an uncontrollable surge in a humanitarian crisis (Bilak, 2019; Shehu and Abba, 2020; International Committee of the Red Cross, 2021). Over a million vulnerable populations, such as women and children, continue to take refuge in government and NGO set up IDP camps (Olusegun and Ogunfolu, 2019; UNICEF, 2020). IDP camps in Nigeria are currently at 143,110 by the end of 2021 (Kareem, 2022). Most of these IDP camps experience insufficient or shortage of supplies, causing displaced persons to live in deplorable conditions that expose them to risks of starvation, infectious diseases, and contaminations (United Nations, 2021; United Nations Office for the Coordination of Humanitarian Affairs, 2022).
Despite these welfare crises, violent armed groups continue to force families out of their communities, resulting in overcrowding in IDP camps. Meanwhile, it may be illogical to question how internal displacements will be reduced to the barest minimum when there are still progressions of overlapping triggering events of violence. Most significant are the attacks and killings by non-state armed groups that have ravaged the North-Eastern part of the country for over a decade in pursuit of basically Islamic and Pastoral agendas (Davidson, 2019; Okoli, 2019; United Nations Security Council, 2020). According to the United Nations High Commissioner for Refugees (2020), the Boko Haram insurgents, among other groups, are responsible for over 2.1 million internal displacements. The North-eastern states in Nigeria account for almost 99.1% of the origin of internally displaced individuals in IDP camps across the country, with Bornu state alone accounting for 83%, followed by Adamawa at 7%, Yobe at 6%, and Taraba at 3.1% (International Organization of Migration, 2021). In the political scenery, political sentimentalism, as depicted in the literature, has always been an endemic threat to social cohesion, national unity, and security in Nigeria. Although there is no reliable data on the proliferation and market of small arms in Nigeria, reports document that out of 10 million illicit small arms in West Africa, an estimated 1–3 million are in circulation in Nigeria (Abiodun et al., 2018). Security experts assert that are great quantity of these weapons wielded by civilians are used during election seasons to perpetrate violence (Tukur et al., 2020). Pre-election conflicts stem from ethnic, religious, and political divisions that fuel violent sentiments to influence the election season (Dokoupilová, 2019, 2022; Oyewole and Omotola, 2021). Nevertheless, it is essential to remind that corrupt leaders and groups engineer this violence to instrumentalize ethnic sentiments in the choice of leadership (Oyewole, 2022).
Accurate forecasting of internal displacements in these conflict-affected regions and states can contribute a significant benefit in the early planning and budgeting in managing the future displaced persons and better prevent any possible humanitarian crises associated with internal displacements by conflicts. Government agencies operating IDP camps in Nigeria have been paying less focus to improve their crisis response system for future displacements caused by conflict through funding, policies, and training (Eweka and Olusegun, 2016). Ramírez and Rubiano (2009) proposed that emergency preparedness is an important element in the Disaster Risk Management Policy Framework applicable to internal displacements. This framework underscores the relevance of early warning apparatus, logistics, supplies, and training in managing IDPs emergencies that occur unawares. Given that conflicts have persisted in being absorbed into the framework of ethnic, religious, and political structures as a tool for dominance, terror, and encroachment in Nigeria (Ejiofor, 2021), the majority of affected and soon-to-be affected populations may most probably continue to become displaced in future years. However, the focus comes down to what figures we expect in the coming years and why relevant humanitarian agencies must be readily responsive. Given this objective, the current study will require a time series analysis, which looks at previous annual trends to answer this research question.
Time series analysis is a form of trend assessment that uses observed or actual values from historical data to create a model that estimates possible future values or figures (Ihaka, 2005; Adhikari and Agrawal, 2013). Various time series models have been used for forecast in different contexts, such as the Auto-Regressive Integrated Moving Average (ARIMA), Exponential Smoothing (ETS), and Neural Network Models. They have undergone evaluations for validity and reliability to ensure they can apply accuracy to the outcome of predicted events (Wang and Chaovalitwongse, 2011; Mehdiyev et al., 2016; Nanthakumaran and Tilakaratne, 2017). These approaches engendered the significant relevance of the need for prediction interval levels where the values of estimated events fall within (Gardner, 1988; Chatfield, 2001). The ARIMA model has been reported to show reliability in fitting the best models and accounting for trends in seasonal time series data (Ho and Xie, 1998; Ilie et al., 2020; Schaffer et al., 2021). Thus, the ARIMA method will be adopted in the current study to forecast the number of persons expected to be displaced due to conflict and violence. The study will highlight the implications of improving planning and budgeting by humanitarian government agencies to effectively manage and prevent a future humanitarian crisis in these camps.
Materials and methods
Data resource
The data used in the current time series forecasting was sourced from the Global Internal Displacement Database (GIDD) (Internal Displacement Monitoring Center, 2021b) (https://www.internal-displacement.org/database/displacement-data). The data frame on annual internal displacement due to violence and conflict in Nigeria from 2009 to 2021 was extracted and exported as an independent time series dataset. The data were calculated as annual figures of persons internally displaced.
Statistical analysis
Due to the absence of seasonality of the time series data (absence of daily, monthly, or quarterly frequencies or components), the ARIMA model was the best time series forecasting method to choose the best fitting model for the prediction. Thus, the Auto-regressive Integrated Moving Average ARIMA was used as the statistical method for the time series forecast in the study and was carried out using R Studio and Microsoft Excel.
Method
The ARIMA model is defined by combining three important terms, which are the p, d, and q in the time series model, where:
p = autoregressive term.
d = non-seasonal difference term.
q = moving average term.
Several analytical steps were followed to ensure the conditions for establishing the p, d, and q terms were appropriate to use the ARIMA method. The data frame was imported to R Studio was transformed to a time series data type using the code [idpdata=ts(vector,start =,end =,frequency =)]. This step ensures that R understands that the data class is time series data. The next step was identifying whether the time series data was stationary by running the Augmented Dickey-Fuller Test. However, to make the data stationary, the [auto.arima] R function for Akaike Information Criterion was used to obtain the best ARIMA model (p, d, q). The forecast was executed at a 95% confidence interval when the best fitting model was identified. The Box L. Jung analysis was used to evaluate the time series data has no significant serial autocorrelation.
Ethics approval
The secondary data obtained was made publicly available by Global Internal Displacement Database (GIDD) and required no application for data licensing for usage. Given the conditions to use the data, the data source was referenced in the study. Therefore, the data does not require any ethical approval.
Result
General characteristics
Figure 1 shows the time series plot of the annual total number of people who have been internally displaced due to conflict and violence from 2009 to 2021 in Nigeria. The plot indicates a 1,200% increase in the number of internally displaced persons in 2011 (see Figure 2 for % changes). Later in 2013, there was a steep 648% increase in the figures. The plot also indicates an all-time high ATH of (↑107%) 975,000 people internally displaced due to conflict and violence in Nigeria in 2014. Following the trend of a percentage decrease in IDPs figures from 2015 to 2017, a 94% increase in IDPs figures followed in 2018. A similar percentage decreasing pattern occurred from 2019 to 2020 before a steep 122% increase in figures (376,000) took place in 2021.
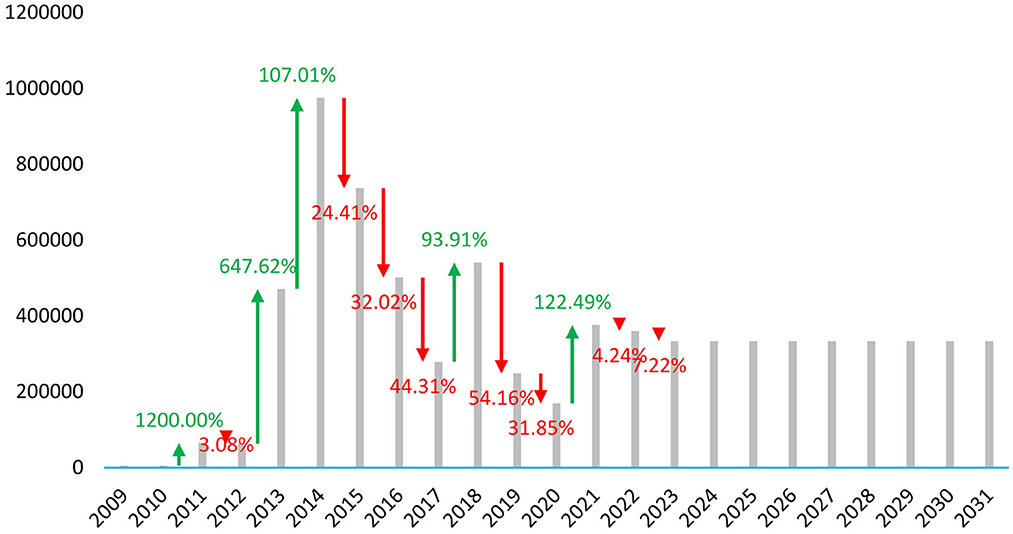
Figure 2. Percentage change in annual figures of internally displaced persons in Nigeria due to conflict and violence from 2009 to 2031.
Forecast of the ARIMA best-fitting model
Given the criterion for the Autoregressive Integrated Moving Average ARIMA Test for time series forecasting, it was pertinent to know if the time series data is stationary or non-stationary. The Augmented Dickey-Fuller analysis shows that the data was not stationary at 95% CI (ADF= −1.480, p > 0.05). The output indicates that the best ARIMA p, d, q model is (0, 0, 1), which has the lowest AIC value possible.
The forecast code was run upon fulfilling the forecast criteria, and the result is presented in Table 1. The forecast plot shows that in 2022, there will be a predicted estimated figure of 360,056 internally displaced persons due to conflict and violence in Nigeria, indicating a 4% decrease (95% CI [−115,409.7, 835,522.4]) based on the previous year's figure (see Figure 1 and Table 1). The forecast plot also shows that there will be a predicted 7% further decrease in the figures of internally displaced persons in 2023. Furthermore, the forecast predicted no difference in annual figures from the last figure in 2023 to the end of the forecast year 2031 [95% CI (−227,427.8, 895,555.6)] (see Figures 1, 2).
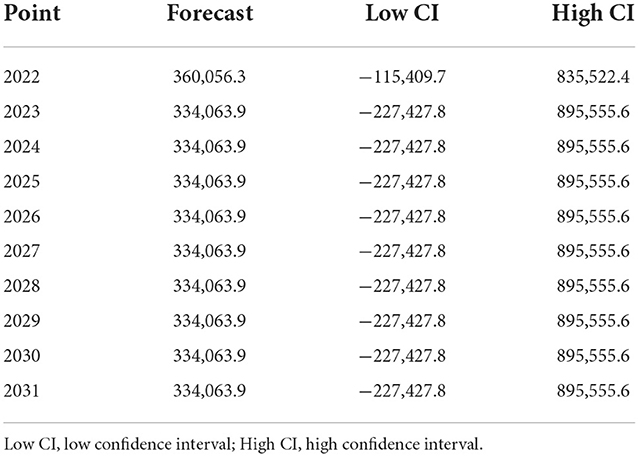
Table 1. Out-of-sample forecast at 95% confidence intervals of IDPs annual figures due to conflict and violence (2022–2031).
The Box L. Jung test analysis carried out shows that there is the absence of any serial autocorrelation at lag 1, 5, and 10 in the data model (p > 0.05), indicating that the time series values are independent (see Table 2).
Discussion
The study executed an ARIMA forecast model to predict the trends of internal displacement due to conflict and violence in Nigeria from 2022 to 2031, using observed trends from 2009 to 2021. Nigeria ranks third among the nations with the most numbers of internally displaced from conflicts in Africa (Kamer, 2022). It is still regarded as one of the world's most neglected affected countries (Norwegian Refugee Council, 2022). Furthermore, Islamic State insurgents and electoral bigots continue to perpetrate the killings of innocent populations in Nigeria. Perhaps, any attempt to abate the accumulating displacement figures will be nugatory without organized counter-measures in neutralizing the impacts of armed banditry and terrorism in these affected regions.
The Danish Refugee Council (2021) executed a forecast trend model as part of a Global Forecast Report to predict future displacement figures and percentage change in Nigeria. The report indicated that the figures were expected to increase by 280,000 in 2021, accounting for a 9% increase based on the previous year's figures. The percentage change in trend (see Figure 2) in the current study shows an increase in internal displacement but a 122% increase in degree compared to the 7% increase the Danish Refugee Council (2021) predicted. They further predicted that the figures will continue to increase and could record a 334% increase representing a figure of more than 1 million displaced persons by the end of 2022. The current study's forecast model reported a 4% decrease representing 360,056 displaced persons by the end of 2022. Moreover, Danish Refugee Council (2021) estimated figure of more than 1,000,000 does not fall within the confidence intervals of the forecast for 2022 in the current study. Thus, this forecast result does not support the Danish Refugee Council (2021) forecast for the year 2022 figure.
Although the seasonality effect was not analyzed in the current time series forecast, the Danish Refugee Council (2021) noted that lesser displacements figures were expected to occur during rainy seasons based on the limitations in operations and mobility of armed groups perpetrating violence during this season. In addition, the current study noticed a pattern of how an increase in displacement figures precipitated or overlapped with general elections that have been held in Nigeria. In 2011, the displacement figures increased by 1,200% during the period when the 2011 general elections were held. There was a 107% increase in displacement figures in 2014, just a year before the 2015 general election. There was also a 93% increase in displacement figures in 2018, a year before the 2019 general elections. Although not aligned with the current study forecast, the Danish Refugee Council (2021) forecast predicts a 334% increase in displacement figures in 2022, a year just before the 2023 general elections. This result aligns with the assertions of scholars who have described the mechanism fostering the link between electoral-induced religious, ethnical, and political conflicts and forced displacements (Dokoupilová, 2019, 2022; Oyewole and Omotola, 2021; Oyewole, 2022). Arms trafficking flourishing through the porous Nigerian borders accounts for the proliferation of civilian-styled arms that are mostly used to promote killings and violence before and during election seasons. Similar to assertions by Bashir (2014) and Abiodun et al. (2018) highlighted that the frequent interception of illegal arms trade and trafficking into Nigeria is becoming a worrisome phenomenon and that over 70% of these arms recorded in West Africa are in Nigeria. However, the worrisome surge in arms trafficking in Nigeria and West Africa has been significantly linked to Libya's political crisis and Gadaffi's 40 years regime (United Nations Office on Drugs Crime, 2013). In Nigeria, politicians have been reported to attend functions of their political parties armed with assault rifles and hydrochloric or sulfuric acid to be used against political opponents (Adeyemi and Adeyemi, 2003). The problem of arms trafficking is not a novel in Nigeria, but the increasing availability to civilians, even at cheap costs, is propelling and prolonging Ethno-religious-related electoral conflicts in Nigeria (Ero and Ndinga, 2004; John et al., 2007; Onuoha, 2011). A situation that intensifies insecurity thereby escalating violence to community-wide conflicts, forcing causalities to leave their communities to other neighboring states for refuge and safety.
The forecast model in the current study also predicted that there will be a 7% decrease in displacement figures in 2023 and will subsequently neither decrease nor increase till the end of 2031. Just as there will be no increase in displacement figures during the 2024–2031 period, there will be no decrease. Opinions hold that pre-election periods are described with greater violence and terror than the election period, which may explain the predicted 7% decrease in displacement figures in 2023 (general election period). However, the general observations and predictions in the current study can help inform humanitarian projects (e.g., IOM Nigeria Crisis Response Plan, 2022) and agencies (e.g., National Emergency Management Agency) in the effort to mitigate the risk factors of humanitarian crises caused by poor emergency response system through tackling issues of under-budgeting, poor planning, and lack of fund/supplies toward IDPs.
A potential implication of this study is that it will help sensitize government and private humanitarian agencies to consider the cycle of violence that occurs in pre- and mid-electoral seasons while planning, budgeting, and implementing their crisis response strategies. Also, it will provide future analysts with an empirical framework upon which they can implement a similar displacement forecast while considering the limitations reported and observed in this study and prior literature. Critical stakeholders in humanitarian planning and logistics are encouraged to refrain from waiting until there is displacement in a period of conflict before they start making emergency supplies and project planning. These setbacks in readiness and response time can underestimate the magnitude of the potential displacement crisis. This approach can cause undersupply of relevant life aids and dwelling spaces for displaced persons in IDP camps.
This study is faced with analytical limitations that may have impacted the forecast outcome, such as the small sample of observed values used in the ARIMA forecast model. Other forecast models, such as the Exponential smoothing and the Prophet, should be adopted in the future in comparison with the ARIMA outputs to ensure the predictions are not confounded by the type of method used. However, ARIMA is a reliable method of time series predictions. It can be used to replicate the methodological framework of this study for reliability assessment in a similar internal displacement sample, but this time taking into consideration monthly and quarterly seasonality.
Data availability statement
The original contributions presented in the study are included in the article/supplementary material, further inquiries can be directed to the corresponding author.
Author contributions
CI conceived this study, designed the methodology, collected the data, analyzed the data, and interpreted the findings.
Conflict of interest
The author declares that the research was conducted in the absence of any commercial or financial relationships that could be construed as a potential conflict of interest.
Publisher's note
All claims expressed in this article are solely those of the authors and do not necessarily represent those of their affiliated organizations, or those of the publisher, the editors and the reviewers. Any product that may be evaluated in this article, or claim that may be made by its manufacturer, is not guaranteed or endorsed by the publisher.
References
Abiodun, T. F., Ayo-Adeyekun, O. O., and Nwannenaya, C. (2018). Small arms and light weapons proliferation and its threats to Nigeria's internal security. Int. J. Soc. Sci. Hum. Res. 6, 34–45. Available online at: https://www.researchpublish.com//upload/book/SMALL%20ARMS%20AND%20LIGHT%20WEAPONS-6062.pdf
Adeyemi, T. T., and Adeyemi, M. M. (2003). The proliferation of arms and its effect on the development of democracy in Nigeria. Am. Int. J. Res. Hum. Arts Soc. Sci. 5, 233–238. Available online at: http://iasir.net/AIJRHASSpapers/AIJRHASS14-196.pdf
Adhikari, R., and Agrawal, R. K. (2013). An Introductory Study on Time Series Modeling and Forecasting. Mauritius: Lambert Academic Publishing.
Bashir, M. (2014). Small arms and light weapons proliferation and its implication for west African regional security. Int J. Hum. Soc. Sci. 4, 260–269. Available online at: https://www.ijhssnet.com/journals/Vol_4_No_8_June_2014/27.pdf
Bilak, A. (2019). North-East Nigeria, A Massive Internal Displacement Crisis: A Photo Essay on the Conflict- and Disaster-Induced Displacement Crisis Unfolding in Nigeria. Geneva: Internal Displacement Monitoring Centre (IDMC). Available online at: https://www.internal-displacement.org/features/nigeria-internal-displacement-crisis-conflict-floods (accessed July 29, 2022).
Chatfield, C. (2001). “Prediction intervals for time-series forecasting,” in Principles of Forecasting, ed J. S. Armstrong (Berlin: Springer). doi: 10.1007/978-0-306-47630-3_21
Danish Refugee Council (2021). Global Displacement Forecast. Copenhagen: Danish Refugee Council. Available online at: https://drc.ngo/media/gzhpxxlt/drc-global-displacement-forecast-report-2021.pdf (accessed July 20, 2022).
Davidson, E. (2019). The menace of Fulani herdsmen attack and its effects on the socio-economic development of Nigeria. SSRN. 6, 7. doi: 10.2139/ssrn.3476374
Dokoupilová, E. (2019). Pre-electoral Violence in Nigeria (Dissertation/bachelor's thesis). [MUNI Portal]: Masaryk University. Available online at: https://is.muni.cz/th/tn5q5/Bachelors-Thesis-Eva-Dokoupilova_FINAL.pdf (accessed July 30, 2022).
Dokoupilová, E. (2022). Pre-electoral Violence in Nigeria and Pakistan: Comparative Case Study (Dissertation/master's thesis). [MUNI Portal]: Masaryk University. Available online at: https://is.muni.cz/th/swfp6/ (accessed July 30, 2022).
Ejiofor, P. F. (2021). The fear of ethnic domination: explaining the persistence of natural resource conflicts in Nigeria. Ethnopolitics. 22, 1–21 doi: 10.1080/17449057.2021.2006521
Ero, C., and Ndinga, M. A. (2004). “Small arms and light weapons,” in West African Security Challenges: Building Pace in a Troubled the Region, eds A. Adebajo, and I. Rashid (London: Lynne Rienner Publishers).
Eweka, O., and Olusegun, T. O. (2016). Management of internally displaced persons in Africa: comparing Nigeria and cameroon. Afr. Res. Rev. 10, 193–210. doi: 10.4314/afrrev.v10i1.15
Gardner, E. S. (1988). A simple method of computing prediction intervals for time series forecasts. Manag. Sci. 34, 541–546.
Gwadabe, N. M., Salleh, M. A., Ahmad, A. A., and Jamil, S. (2018). Forced displacement and the plight of internally displaced persons in Northeast Nigeria. Hum. Soc. Sci. Res. 1, 46–52. doi: 10.30560/hssr.v1n1p46
Ho, S. L., and Xie, M. (1998). The use of ARIMA models for reliability forecasting and analysis. Comput. Ind. Eng. 35, 213–216. doi: 10.1016/S0360-8352(98)00066-7
Ihaka, R. (2005). Time Series Analysis: Lecture Notes for 475.726. Auckland: Statistics Department, University of Auckland. Available Online at: https://www.stat.auckland.ac.nz/~ihaka/726/notes.pdf (accessed July 20, 2022).
Ilie, O. D., Ciobica, A., and Doroftei, B. (2020). Testing the accuracy of the ARIMA models in forecasting the spreading of COVID-19 and the associated mortality rate. Medicina 56, 566. doi: 10.3390/medicina56110566
Internal Displacement Monitoring Center (2021a). Country Profile: Nigeria. Geneva: Internal Displacement Monitoring Center. Available online at: https://www.internal-displacement.org/countries/nigeria (accessed July 22, 2022).
Internal Displacement Monitoring Center (2021b). Global Internal Displacement Database. Geneva: Internal Displacement Monitoring Center. Available online at: https://www.internal-displacement.org/database/displacement-data (accessed July 13, 2022).
International Committee of the Red Cross (2021). Refugee Crisis in Nigeria. Geneva: International Committee of the Red Cross. Available online at: https://www.icrc.org/en/where-we-work/africa/nigeria/nigeria-refugee-crisis-idps (accessed July 28, 2022).
International Organization of Migration. (2021). Nigeria—North East—Displacement Report 38 (June–July 2021). Grand-Saconnes: International Organization of Migration.Available online at: https://displacement.iom.int/reports/nigeria-north-east-displacement-report-38-june-july-2021 (accessed July 22, 2022).
IOM Nigeria Crisis Response Plan (2022). Nigeria Crisis Response Plan 2022. Available online at: https://nigeria.iom.int/nigeria-crisis-response-plan-2022 (accessed July 20, 2022).
John, I. A., Mohammed, A. Z., Pinto, A. D., and Nkanta, C. A. (2007). Gun violence in Nigeria: a focus on ethno-religious conflict in Kano. J. Public Health Policy 28, 420–431. doi: 10.1057/palgrave.jphp.3200155
Kamer, L. (2022). African Countries with the Largest Number of Internally Displaced Persons (IDPs) as of 2021. New York, NY: Statista. Available online at: https://www.statista.com/statistics/1237268/number-of-internally-displaced-persons-in-africa/ (accessed July 19, 2022).
Kareem, K. (2022). Chart of the Day: In 2021, Number of Displaced Persons in Nigeria Reach 2.18 Million. Abuja: Dataphyte. Available online at: https://www.dataphyte.com/latest-reports/security/chartoftheday-in-2021-number-of-displaced-persons-in-nigeria-reach-2-18-million/ (accessed July 21, 2022).
Letswa, A. M., and Isyaku, S. S. (2018). Insurgency and internally displaced persons IDPs in Nigeria: a reflection on the causes, implication and way forward. Int. J. Innov. Stud. Sociol. Hum. 3, 36–48. Available online at: https://ijissh.org/storage/Volume3/Issue5/IJISSH-030506.pdf
Mehdiyev, N., Enke, D., Fettke, P., and Loos, P. (2016). Evaluating forecasting methods by considering different accuracy measures. Proced. Comput. Sci. 95, 264–271. doi: 10.1016/j.procs.2016.09.332
Nanthakumaran, P., and Tilakaratne, C. D. (2017). “A comparison of accuracy of forecasting models: a study on selected foreign exchange rates,” in Seventeenth International Conference on Advances in ICT for Emerging Regions (ICTer), New Jersey, NJ: IEEE. 1–8. doi: 10.1109/ICTER.2017.8257789
Norwegian Refugee Council (2022). The World's Most Neglected Displacement Crises in 2021. Washington, DC: Norwegian Refugee Council. Available online at: https://www.nrc.no/shorthand/fr/the-worlds-most-neglected-displacement-crises-in-2021/index.html (accessed July 20, 2022).
Okoli, C. A. I. (2019). What Can be Done to Fight Rural Banditry in Northern Nigeria. Lagos: The Conversation. Available online at: https://theconversation.com/what-can-be-done-to-fight-rural-banditry-in-northern-nigeria-122776 (accessed July 19, 2022).
Olusegun, O. O., and Ogunfolu, A. (2019). Protecting internally displaced children in armed conflicts: Nigeria in focus. Notre Dame J. Int. Compar. Law 9, 34–55. Available online at: https://scholarship.law.nd.edu/ndjicl/vol9/iss2/4
Onuoha, F. C. (2011). Small arms and light weapons proliferation and human security in Nigeria. Conflict Trends 1, 50–56. Available online at: https://hdl.handle.net/10520/EJC16110
Oyewole, S. (2022). There's Violence Every Election Season in Nigeria: What Can be Done to Stop It. Melbourne: The Conversation. Available online at: https://theconversation.com/theres-violence-every-election-season-in-nigeria-what-can-be-done-to-stop-it-183690 (accessed July 31, 2022).
Oyewole, S., and Omotola, J. S. (2021). Violence in Nigeria's 2019 general elections: trend and geospatial dimensions. Geogr. J. 87, 2393–2403. doi: 10.1007/s10708-021-10375-9
Ramírez, F., and Rubiano, D. (2009). Incorporating Disaster Risk Management in Territorial Planning. Lima: Disaster Prevention in the Andean Community (PREDECAN).
Relief Web (2021). Global Humanitarian Overview 2022 (Abridged Arabic Report). New York, NY: Relief Web. Available online at: https://reliefweb.int/report/world/global-humanitarian-overview-2022-abridged-arabic-report (accessed November 20, 2022).
Schaffer, A. L., Dobbins, T. A., and Pearson, S. A. (2021). Interrupted time series analysis using autoregressive integrated moving average (ARIMA) models: a guide for evaluating large-scale health interventions. BMC Med. Res. Methodol. 21, 1–12. doi: 10.1186/s12874-021-01235-8
Shehu, M., and Abba, A. (2020). Humanitarian crisis and sustainable development: perspectives and preferences of internally displaced persons in the north-eastern Nigeria. Int. J. Hum. Act 5, 1–10. doi: 10.1186/s41018-020-00084-2
Tukur, A., Hamza, K., and Rabiu, I. (2020). The impact of proliferation of small arms and light weapons on Nigeria's national security: a case study of Kogi State (2015–2018). KIU J. Soc. Sci. 6, 51–66. Available online at: https://www.ijhumas.com/ojs/index.php/kiujoss/article/view/1079
UNICEF (2020). 19 Million Children Internally Displaced by Conflict and Violence in 2019 is Highest Number Ever. New York, NY: UNICEF. Available online at: https://www.unicef.org/nigeria/press-releases/19-million-children-internally-displaced-conflict-and-violence-2019-highest-number (accessed July 21, 2022).
United Nations (2021). Displaced in Northeast Nigeria ‘Knocking on the Door of Starvation': WFP. San Francisco, CA: United Nations. Available online at: https://news.un.org/en/story/2021/10/1103252 (accessed July 20, 2022).
United Nations High Commissioner for Refugees (2020). Nigeria Emergency. Geneva: United Nations High Commissioner for Refugees. Available online at: https://www.unhcr.org/nigeria-emergency.html (accessed July 19, 2022).
United Nations Office for the Coordination of Humanitarian Affairs (2022). Nigeria: 2022 Humanitarian Needs Overview. New York, NY: United Nations Office for the Coordination of Humanitarian Affairs. Available online at: https://www.humanitarianresponse.info/en/operations/nigeria/document/nigeria-2022-humanitarian-needs-overview (accessed July 29, 2022).
United Nations Office on Drugs Crime (2013). Transnational Organized Crime in West Africa. Vienna: United Nations Office on Drugs and Crime. Available online at: https://www.unodc.org/documents/toc/Reports/TOCTAWestAfrica/West_Africa_TOC_FIREARMS.pdf (accessed November 19, 2022).
United Nations Security Council (2020). Islamic State West Africa Province (ISWAP). New York, NY: United Nations Security Council. Available online at: https://www.un.org/securitycouncil/content/islamic-state-west-africa-province-iswap-0 (accessed July 22, 2022).
Keywords: displacement, emergency response, Nigeria, humanitarian crisis, planning, conflict
Citation: Izuakor CF (2022) Trends in forced displacement due to conflict from 2009 to 2021: A decade forecast for effective humanitarian emergency response system in Nigeria. Front. Hum. Dyn. 4:1009651. doi: 10.3389/fhumd.2022.1009651
Received: 02 August 2022; Accepted: 24 November 2022;
Published: 09 December 2022.
Edited by:
Khandaker Jafor Ahmed, University of Adelaide, AustraliaReviewed by:
Ayobami Abayomi Popoola, University of KwaZulu-Natal, South AfricaMonica Adhiambo Onyango, Boston University, United States
Copyright © 2022 Izuakor. This is an open-access article distributed under the terms of the Creative Commons Attribution License (CC BY). The use, distribution or reproduction in other forums is permitted, provided the original author(s) and the copyright owner(s) are credited and that the original publication in this journal is cited, in accordance with accepted academic practice. No use, distribution or reproduction is permitted which does not comply with these terms.
*Correspondence: Chidubem Fidelis Izuakor, aXp1YWtvcmZpZGVsaXNAZ21haWwuY29t
†ORCID: Chidubem Fidelis Izuakor orcid.org/0000-0002-8420-4654