- 1Cognitive and Engineering Psychology, Institute of Psychology, Chemnitz University of Technology, Chemnitz, Germany
- 2OFFIS—Institute for Information Technology, Oldenburg, Germany
Automated vehicles promise transformational benefits for future mobility systems, but only if they will be used regularly. However, due to the associated loss of control and fundamental change of in-vehicle user experience (shifting from active driver to passive passenger experience), many humans have reservations toward driving automation, which question their sufficient usage and market penetration. These reservations vary based on individual characteristics such as initial attitudes. User-adaptive in-vehicle Human-Machine Interfaces (HMIs) meeting varying user requirements may represent an important component of higher-level automated vehicles providing a pleasant and trustworthy passenger experience despite these barriers. In a driving simulator study, we evaluated the effects of two HMI versions (with permanent vs. context-adaptive information availability) on the passenger experience (perceived safety, understanding of driving behavior, driving comfort, driving enjoyment) and trust in automated vehicles of 50 first-time users with varying initial trust (lower vs. higher trust group). Additionally, we compared the user experience of both HMIs. Presenting driving-related information via HMI during driving improved all assessed aspects of passenger experience and trust. The higher trust group experienced automated driving as safest, most understandable and most comfortable with the context-adaptive HMI, while the lower trust group tended to experience the highest safety, understanding and comfort with the permanent HMI. Both HMIs received positive user experience ratings. The context-adaptive HMI received generally more positive ratings, even though this preference was more pronounced for the higher trust group. The results demonstrate the potential of increasing the system transparency of higher-level automated vehicles through HMIs to enhance users’ passenger experience and trust. They also consolidate previous findings on varying user requirements based on individual characteristics. User group-specific HMI effects on passenger experience support the relevance of user-adaptive HMI concepts addressing varying needs of different users by customizing HMI features, such as information availability. Consequently, providing full information permanently cannot be recommended as a universal standard for HMIs in automated vehicles. These insights represent next steps toward a pleasant and trustworthy passenger experience in higher-level automated vehicles for everyone, and support their market acceptance and thus the realization of their expected benefits for future mobility and society.
Introduction
Self-driving cars have long been part of various visions of our society’s future, promising comfortable, accessible and sustainable mobility for everyone (ERTRAC, 2019). With recent advances in technology (especially computer systems and artificial intelligence) paving the way to intelligent mobility systems (Tasoudis and Perry, 2018; Araujo et al., 2019), this vision has moved into closer reach. The progress in driving automation can be classified according to the distribution of driving-related tasks between human driver and automated driving system. The well-established classification system provided by the Society of Automotive Engineers (SAE) defines six levels of driving automation ranging from no automation (Level 0: the human driver performs all driving-related tasks at any time) to full automation (Level 5: the automated driving system is able to perform all driving-related tasks at any time) (SAE International 2018). Lower levels of driving automation, such as partially automated vehicles (SAE-Level 2), are already obtainable and able to perform the driving task under specific circumstances. In these cases, the human driver is still required to monitor the environment and the system constantly and to take over the driving task if necessary. However, there is a strong developmental trend toward higher levels of driving automation, such as highly and fully automated vehicles (SAE-Levels 4–5), which are supposed to execute the driving task without the need for human monitoring or intervention (Araujo et al., 2019). Providing solutions for current mobility-related problems, this trend promises numerous benefits regarding the safety, efficiency and comfort, as well as the environmental and social sustainability of future transport systems (ERTRAC, 2019). The development and market penetration of such higher-level automated vehicles could thus be important enablers for a fundamental transformation of mobility and ultimately of society (Anderson et al., 2016; Kyriakidis et al., 2017).
However, while substantial technical progress toward this vision has been made in recent years, human factors research paints a rather nuanced picture. Even though potential future users state a general openness to driving automation (Fraedrich and Lenz 2015), they also report profound reservations toward the technology and its safety (Schoettle and Sivak, 2014; Kyriakidis et al., 2015; Wolf, 2016), which question a sufficient usage and dissemination level of automated vehicles. These reservations are attributed to the loss of control and the fundamental change of the in-vehicle user experience (shifting from an active driver experience to a passive passenger experience) that are provoked by handing over vehicle control to an automated system (Elbanhawi et al., 2015; Tasoudis and Perry, 2018). Consequently, the direction in which future mobility will develop will not only be decided by technical advances and accompanying societal and legal measures (Littman, 2020), but substantially by human factors (Kyriakidis et al., 2017; Shariff et al., 2017). Thus, the expected benefits of driving automation will only be achieved if potential users will trust and accept this technology and will be willing to use it on a regular basis (ERTRAC, 2019). Therefore, automated vehicles need to provide a pleasant and trustworthy passenger experience in order to make humans feel comfortable to “proverbially put their lives in the hands of computers” (Wintersberger et al., 2019, p. 2).
With the reported driving simulator study, we aim to contribute to overcoming this challenge through a user-centered design of automated vehicles. We thereby focus on higher levels of driving automation (SAE-Levels 4–5), since these levels are expected to provide the most benefits for our future mobility and society. Based on previously collected user requirements in this context (Hartwich et al., 2020), we believe that a pleasant and trustworthy passenger experience at higher levels of driving automation cannot be taken for granted even in the case of a safely working automated driving system. We therefore argue that 1) in-vehicle Human-Machine-Interfaces (HMIs) that enable passengers to monitor the system are useful to enhance passenger experience and trust in this context, even though human monitoring is actually no longer required at these automation levels. We further assume that 2) such HMI concepts should possibly not follow a one-size-fits-all-approach, but instead adapt to individually differing user requirements in order to optimize the passenger experience for all potential users. To examine these assumptions, we investigated the user group-specific effects of two HMI versions on the passenger experience and trust in automated driving reported by first-time system users. The goal of this user evaluation was to provide empirically based, user-centered implications for the design of automated vehicles that support their broad acceptance and consequently their market penetration once they become obtainable. In the broader context, these implications can contribute to achieving the expected benefits of driving automation for our future mobility and society, which can only become reality if potential users are able to trust and willing to use the technology. In the following, an overview of previous findings motivating this research is provided along with an integrated derivation of our specific research questions.
Related Work
Trust in Automated Driving
Trust in automation was chosen as a dependent variable for the presented evaluation, since it is a strong predictor of the acceptance and adoption of automated systems (Parasuraman and Riley, 1997; Lee and See, 2004), such as automated vehicles (Choi and Ji, 2015; Xu et al., 2018), but poses substantial challenges in the driving context (Mühl et al., 2019; Strauch et al., 2019; Schmidt et al., 2020). Defined as “the attitude that an agent will help achieve an individual’s goals in a situation characterized by uncertainty and vulnerability” (Lee and See, 2004, p. 51), trust is well-established to mediate the interaction between humans and automation (Sheridan and Hennessy, 1984). The concept is highly relevant in the context of automated vehicles, where system errors can lead to fatal consequences (high vulnerability) and most potential users lack system experience and knowledge due to the radical system novelty (high uncertainty). Generally, incorrectly calibrated trust can impair the usage of automated systems in two ways: While too much trust (over-trust) can lead to unintended and incorrect ways of using the system (misuse), too little trust (distrust) can result in rejection of the system (disuse) (Parasuraman and Riley, 1997). In this study, we focused on distrust, i.e. an unnecessary lack of trust in a functioning automated vehicle, which prevents potential users from benefiting from the technology. Previous research produced strong evidence that not everyone is immediately willing to hand over vehicle control to an automated system (Schoettle and Sivak, 2014; Wolf, 2016; König and Lambert, 2017). In recent examinations in simulated and real-world driving environments, passengers revealed lower trust in automated than in human vehicle control, even if driving maneuvers were executed identically, which manifested in lower trust ratings and more pronounced unnecessary control gaze behavior (Mühl et al., 2019; Strauch et al., 2019; Schmidt et al., 2020).
Passengers’ Driving Experience in Automated Driving
The pivotal role of a subjective feeling like uncertainty in the definition of trust suggests that the degree to which users rely on an automated system is strongly related (among others) to how they feel during its usage. In the literature, such a “momentary, primarily evaluative feeling […] while interacting with a product or a service” (Hassenzahl, 2008, p. 8) is defined as user experience. The reported study included several aspects of the in-vehicle user experience during automated driving (hereafter referred to as passenger experience) that appeared relevant in this context based on previous research. The identification of perceived safety as a major predictor of the acceptance of driving automation (Xu et al., 2018; Zoellick et al., 2019) clarifies that passengers need to experience automated vehicles as a safe form of mobility and for this purpose have to be able to understand their driving behavior. Both issues are considered necessary to feel comfortable during automated driving, which can be defined as feeling pleasantly relaxed based on the certainty that the vehicle is executing the driving task safely (Constantin et al., 2014). Conversely, if passengers are uncertain about the safety of the vehicle operations, this might result in an unpleasant state of tension defined as discomfort (Hartwich et al., 2018). In addition to these aspects, driving enjoyment has been identified as a crucial element of the interaction between automotive technologies and their users (Engelbrecht, 2013). However, previous research suggests that the pleasure of driving decreases for many users if they have to transfer the driving task to an automated system (Hartwich et al., 2018; Frison et al., 2019). Taking these findings into account, the operationalization of passenger experience in the presented study included passengers’ perceived safety, understanding of the vehicle’s driving behavior, driving comfort and driving enjoyment. All four aspects have been demonstrated to be significantly reduced during automated vehicle control in comparison to human vehicle control despite identical maneuver execution (Hartwich et al., 2020), emphasizing the impairing effects that driving automation can have on passenger experience and the resulting need for countermeasures.
Increasing the Transparency of Automated Vehicles
A user-centered design of automated vehicles supporting the technology’s acceptance and adoption needs to provide solutions for these challenges related to trust and passenger experience. Design requirements stated by passengers after their first system experience often relate to the in-vehicle presentation of driving-related information and reveal their need for system transparency to decrease their uncertainty (Hartwich et al., 2020). Thus, even though there is no actual need to monitor a higher-level automated vehicle, many passengers still wish to not be completely excluded from the driving process (Ekman et al., 2018) and to receive information on the vehicle’s driving behavior (Feierle et al., 2020). In the research tradition on trust in automation, it is well-established that informing users about the status and behavior of an automated system can improve their level of trust (Lee and See, 2004; Hoff and Bashir, 2015). Thus, in-vehicle HMIs communicating such system information during driving are considered a key prerequisite for passengers’ trust in automated vehicles (König and Lambert, 2017; Kraus et al., 2020). Yet, passengers’ information requirements on higher automation levels are not completely clarified (Lau et al., 2020). Comparing users’ information needs at different automation levels in a driving simulator, Beggiato et al. (2015) identified that information that are useful to monitoring the vehicle were still evaluated as important during highly automated driving, even though as slightly less important than during partially automated driving. Diels and Thompson (2018) specified these requirements by determining two categories of information expected by passengers of higher-level automated vehicles: details on elements detected by the system and on upcoming driving maneuvers.
So far, the implementation of users’ information requirements and the user evaluation of resulting HMI concepts have mainly been focused on partially and conditional automated driving (SAE-Levels 2–3) due to the remaining human involvement in the driving task (Feierle et al., 2020; Oliveira et al., 2020). On these lower automation levels, an HMI serves specific safety-related purposes, such as supporting the human monitoring task or the transfer of vehicle control between human driver and automated driving system. Along with the human role, these HMI purposes change fundamentally at higher automation levels, where human involvement in the driving task is actually no longer necessary. Accordingly, it is not clear yet whether HMIs providing driving-related information still have a benefit or should be given less consideration in favor of other design elements (e.g. entertainment-related features) in this context. Currently, there are only a few studies evaluating the impact of displaying driving-related information during higher-level automated driving. These studies provided first indications of positive HMI effects on passengers’ trust in the automated vehicle (Wintersberger et al., 2019; Oliveira et al., 2020), but did not include passenger experience. In addition, such studies mainly focused on specific (i.e. complex or unpredictable) driving situations, leaving open questions about passengers information needs over the course of more realistic (i.e. longer and more diverse) rides. Both issues were addressed by the presented study in order to obtain more differentiated knowledge on HMI-effects at higher levels of driving automation.
Interindividual Differences in User Requirements
Another aspect mostly neglected by previous studies is the subjectivity included in the definitions of trust and passenger experience, which implies that users’ evaluations of automated systems do not only depend on system properties, but also on user characteristics, and therefore can differ between individuals (Frison et al., 2019). This implication is strongly supported by previous research identifying various user characteristics as relevant predictors of trust and passenger experience in the driving automation context, among them personality traits (Dettmann et al., 2021), system experience (Bauerfeind et al., 2017), manual driving habits (Mühl et al., 2019; Dettmann et al., 2021), or demographic factors, such as age (Hartwich et al., 2019). Also important seem to be initial attitudes, such as trust (Beggiato et al., 2015), already acquired prior to the first system experience, for example based on individuals’ knowledge of the system or their general propensity to trust. Such individual differences might also be reflected in passengers’ need for system transparency during automated driving and should therefore be considered in the design of in-vehicle HMIs. Consequently, there is an increasing demand for user-adaptive HMI concepts meeting varying user requirements (Feierle et al., 2020) in order to provide a trustworthy and pleasant passenger experience for everyone.
A solution might be different information profiles matching the requirements of different user groups or even individual users. Thus, someone with low initial trust in automated vehicles might require a permanently available high amount of driving-related information during driving, while someone with higher initial trust might not want to be constantly confronted with detailed information. Especially for the latter group, a permanent dynamic information display might even be distracting from the non-driving related activities enabled by higher-level automated driving, based on the human capacity for peripheral perception, which is especially sensitive to motion (McKee and Nakayama 1984). These assumptions were encouraged by a preceding driving simulator study, in which we compared the information requirements of system-unexperienced users with lower vs. higher initial trust in automated vehicles (Hartwich et al., 2020). Indeed, passengers with lower trust reported a significantly higher need for information during automated driving than passengers with higher trust. These group differences reflected in the importance ratings of almost all investigated information types. Nevertheless, both trust groups were largely in agreement about the hierarchy of information, assigning highest importance to information that are useful to monitor the driving automation (e.g. current speed, next driving maneuver, details on surrounding traffic), but also automation status, which is necessary to avoid mode confusion. In addition, the higher trust group exhibited a larger situation-dependence in their importance ratings. Based on these results, we concluded that the degree of information availability over time might be a relevant factor to define different information profiles addressing different user groups. In the follow-up study presented in this paper, we examined the user group-specific effects of two HMI versions differing in their information availability over the course of a whole ride in order to evaluate the approach of addressing different user requirements by user-adaptive HMI concepts.
Summary and Resulting Research Questions
Summing up previous research, there are theoretical and empirical indications for possible benefits of displaying driving-related information during higher-level automated driving on vehicle occupants’ passenger experience and trust in automated vehicles, which would in turn improve the expected acceptance and market penetration of the technology. However, these benefits have not been evaluated sufficiently yet. Following on from first findings on positive HMI effects on trust, we aimed at expanding the knowledge in this field by examining the following research questions:
1) Generally, we investigated whether increasing the system transparency through in-vehicle HMIs can improve the passenger experience and trust of vehicle occupants on higher levels of driving automation. Based on first findings regarding trust in automation, we hypothesized that passengers would report a more pleasant (i.e. safe, understandable, comfortable, enjoyable) driving experience and higher trust in the automated vehicle when driving with than without such an HMI.
2) For an evaluation of the potential of user-adaptive HMI-concepts, we examined whether the HMI’s information availability over time (permanent vs. context-adaptive) affects the degree of improvement of passenger experience and trust differently for users with lower vs. higher initial trust in automated driving. Information availability thereby served as an empirically derived example for HMI features that could be adapted to different users. Following the approach of user-adaptive HMI concepts, we assumed that the varying information needs of the two trust groups might reflect in their responses to the two HMI versions. Thus, passengers with lower initial trust in automated driving might benefit more from an HMI with permanent information availability, while the HMI with context-adaptive information availability might achieve higher levels of improvement for passengers with higher initial trust in automated driving. Nevertheless, we did not rule out the possibility that one of the two HMI versions might universally be more effective than the other one, resulting in comparable effects for both trust groups.
3) For a further comparison of the two HMI version, we examined whether the HMI’s information availability over time (permanent vs. context-adaptive) affects the evaluation of its user experience differently for users with lower vs. higher initial trust in automated driving. In accordance with our considerations on user group-specific HMI effects, we assumed that passengers with lower initial trust in automated driving might prefer the permanent HMI version, whereas passengers with higher initial trust might evaluate the context-adaptive HMI version more positively. However, again we did not rule out the possibility that one version might be evaluated as generally more user-friendly than the other one.
Materials and Methods
All research questions were examined in a driving simulator study, in which first-time system users were able to experience highly automated driving (SAE-Level 4) with different HMI versions in a standardized and safe environment along a diverse ride including a variety of driving situations.
Study Design
For the evaluation of HMI effects, we compared two HMI versions (differing in their degree of information availability over time) with a baseline condition without an automation-specific HMI. The HMI condition thereby served as a within-subjects (repeated-measures) factor with three experimental conditions (no HMI, permanent HMI, context-adaptive HMI). Accordingly, all participants experienced three identical automated rides along the same test track. The only difference between these three rides was the HMI information presented in the center stack display of the vehicle (see Human-Machine Interface). The participants experienced the three HMI conditions in fully balanced order (i.e. the six possible orders of HMI conditions were applied equally often over the course of the study) to compensate for order effects that might occur from repeatedly driving along the test track.
For the integration of interindividual differences, the participants’ initial trust in automated vehicles was included as a quasi-between-subjects factor. Therefore, the sample was divided into two groups (lower trust group, higher trust group) based on a screening questionnaire (see Data Acquisition). We made sure to avoid HMI order differences between the two trust groups by distributing all possible orders of HMI conditions equally often between them.
As dependent variables, we examined four aspects of passenger experience (perceived safety, understanding of driving behavior, driving comfort, driving enjoyment) and passengers’ trust in the automated vehicle, as well as the user experience provided by the two automation-specific HMI versions (permanent HMI, context-adaptive HMI).
Participants
The study was conducted with 50 participants (35 female, 15 male) aged from 20 to 38 years (M = 25.9, SD = 5.2). All of them held a valid driver's license, but had no prior experience with higher-level automated driving. The acquisition of this sample was carried out via a participant database of the university, in which persons who are generally interested in participating in scientific studies can register. For this study, registered persons meeting the criteria above were invited by email and could enroll through a brief online screening questionnaire.
Based on their initial trust in automated vehicles, participants were divided into a lower trust group and a higher trust group by a median split (Mdn = 65.0 on a scale from 0 to 100). The two trust groups did not differ significantly in terms of age, number of years obtaining a driver’s license and gender ration (see Table 1 for descriptive statistics).
Facilities and Driving Simulation
Automated driving was performed in a fixed-base driving simulator, which consisted of a projector-based vision system providing a 180° horizontal field of view as well as a vehicle with fully equipped interior including a rear-view mirror and two side mirrors. We used the SILAB 5.1 simulation environment to program two tracks: a short familiarization track and a test track. The familiarization track consisted of a 4 km long two-lane rural road. The 7 km long test track, which formed the basis for the HMI comparison, incorporated a 4 km long urban road section and a 3 km long rural road section. Driving along this route included starting, driving at different speed limits (50, 100 km/h), managing various complex traffic situations including other road users (e.g. intersections, lane changing to bypass obstacles on the road), and stopping. Automated driving along both tracks was prerecorded based on a dynamic, error-free driving style and was replayed identically for all participants and HMI conditions. While the participants sat on the passenger seat of the vehicle during all rides, pedals were inoperative and the steering wheel was turning automatically.
Human-Machine Interface
In the three HMI conditions, we presented different information in the center stack display of the vehicle. During the ride without any automation-specific HMI (no HMI), only a speedometer was displayed in order to provide the participants solely with information they were accustomed to from manual driving (see Figure 1, left). The other two HMI conditions both incorporated information on the automated driving system, but differed in their information availability over time. Therefore, a reduced and a full information mode were developed based on users’ information needs elaborated in the preceding study by Hartwich et al. (2020). The reduced information mode included a limited set of status information communicating that the automated driving system was currently working: system status (automated driving activated), current speed and speed limit, and the distance left to the destination of the ride. In the full information mode, this status information was supplemented by information enabling passengers to observe the functionality of the automated driving system more closely. Therefore, this mode provided a dynamic overview of the current traffic situation including detected road users and traffic control elements, such as traffic lights and traffic signs, as well as an announcement of the next driving maneuver including a countdown to its start. When the traffic situation involved a traffic light, the current traffic light phase and a countdown to the next phase were displayed, as well. In the permanent HMI condition, the full information mode was activated during the whole ride (100% of the ride duration) (see Figure 1, middle). In the context-adaptive HMI condition, the reduced information mode was activated during the whole ride. The full information mode was solely added in nine complex traffic situations along the test track, starting 10 s before arriving at the situation (e.g. an intersection or obstacle on the road) and ending immediately after passing the situation (see Figure 1, right). In sum, the full information mode was activated for about a quarter (26%) of the ride duration in the context-adaptive HMI condition.
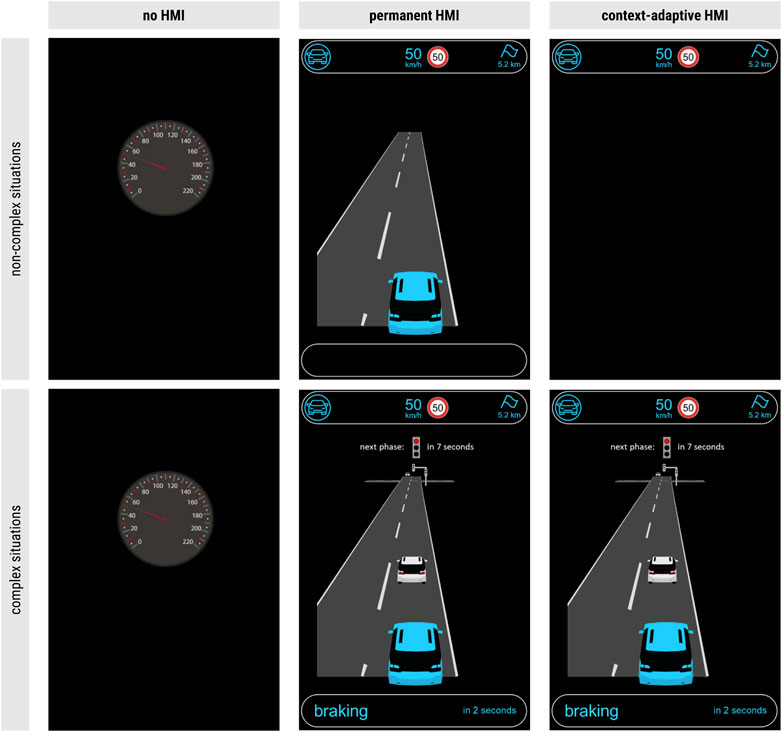
FIGURE 1. Information presented in the center stack display of the vehicle during the three HMI conditions. Note. Displayed traffic situations are exemplary for non-complex and complex situations along the test track. The cyan-colored vehicle represents the own automated vehicle.
Procedure
Prior to study conduct, all participants completed a brief online screening questionnaire. Next to questions on contact details and conditions of participation, this questionnaire included a written system description of highly automated driving and an economic assessment of the participants’ initial trust in the system (see Data Acquisition). Based on their ratings, participants were assigned to either the lower or the higher trust group.
The study was initiated by an explanation of the experimental procedure, including information about simulator sickness and data privacy. After signing an informed consent, participants completed a questionnaire assessing demographic variables. Subsequently, they took place in the passenger seat of the driving simulator and experienced a short automated ride along the familiarization track in order to get accustomed to the environment.
Sitting in the passenger seat, each participant then experienced three identical automated rides along the test track, which only differed in the information presented in the center stack display of the vehicle according to the respective HMI condition. The order of the three HMI conditions was fully balanced across participants to minimize order effects. Balancing was carried out separately within each trust group to ensure that all possible sequences of HMI conditions were equally distributed between the two groups. Prior to the full and the context-adaptive HMI rides, participants received an explanation of the respective HMI version.
During each ride along the test track, participants gave continuous real-time feedback on their passenger experience using a manual input device (see Data Acquisition). In addition, each ride was followed by a questionnaire on further aspects of their passenger experience as well as their trust in the automated vehicle. The questionnaires presented after the permanent and context-adaptive HMI condition additionally assessed the user experience of the respective HMI version.
Upon study completion, participants received 20 euros each as a monetary compensation. Overall, this procedure lasted approximately 2 h per participant.
Data Acquisition
The assessment of participants’ initial trust in automated vehicles was part of the brief online screening questionnaire applied during the process of participant acquisition. After a written system description, trust was assessed using a single item scale (“As a passenger of an automated vehicle, I would trust the capabilities of the vehicle to drive safely.”) with a continuous agreement scale ranging from 0 (totally disagree) to 100 (totally agree).
For continuous real-time feedback on driving discomfort as one aspect of the passenger experience during automated driving, the driving simulator was equipped with a handset control (for details on this method, see Hartwich et al., 2018). During all rides, participants held the handset control (see Figure 2) and pressed its lever gradually in accordance with the extent of their currently perceived discomfort. Higher discomfort corresponded to stronger pressing of the lever and higher values on the associated continuous response scale, which ranged from 0 (comfortable) to 100 (uncomfortable). Participants trained the correct use of the handset control during the familiarization ride at the beginning of the study, during which the center stack display of the vehicle provided visual feedback of the currently entered value on the response scale.
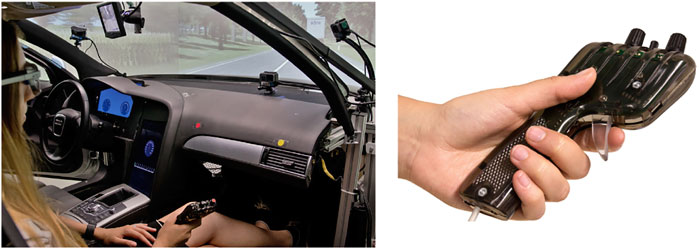
FIGURE 2. Real-time assessment of passenger experience (discomfort) during automated driving (left) via handset control (right).
In addition to this real-time assessment, further aspects of passenger experience as well as trust in the automated vehicle were assessed by questionnaire after each HMI condition. In the absence of a standardized questionnaire, we applied singe-item scales for the assessment of the four aspects of passenger experience (perceived safety, understanding of driving behavior, driving comfort, driving enjoyment). All single items were answered on a continuous agreement scale ranging from 0 (totally disagree) to 100 (totally agree) (see Table 2 for item wording).
Trust in the automated vehicle was assessed using the standardized Trust in Automation questionnaire (TiA) by Körber (2019). With a total of 19 five-point agreement scale items, the TiA provides measures for six dimensions of trust in automation based on subscale scores. These subscales represent the perceived trustworthiness of the system (reliability/competence, understandability/predictability) and its developers (intention of developers), the resulting user attitude toward the system (trust in automation), and user characteristics moderating the relation between both aspects (propensity to trust, familiarity). In this study, we followed the option given by Körber (2019) to analyze the subscale scores relevant for specific research questions. Our study was focused on user attitudes toward automated vehicles as a function of a system feature (system transparency modified via HMI). Therefore, our analysis comprised those dimensions representing pure system characteristics (reliability/competence, understandability/predictability) and resulting user attitudes (trust in automation).
For a more detailed analysis of user preferences regarding the two automation-specific HMI versions (permanent HMI, context-adaptive HMI), we assessed the participants’ HMI-related user experience using the standardized User Experience Questionnaire (UEQ; Laugwitz et al., 2008). With a total of 26 seven-point rating scale items in the form of semantic differentials, the UEQ contains six subscales: Attractiveness as a valence dimension representing users’ overall impression of a digital product, three subscales representing its pragmatic quality (efficiency, perspicuity, dependability) and two subscales representing its hedonic quality (stimulation, novelty). The questionnaire is explicitly designed to compare the user experience of different products based on the subscale means.
Data Preparation and Analysis
For standardized questionnaire (TiA, UEQ) data, we calculated the scale scores according to the respective questionnaire instructions by averaging associated item values. For a visual inspection of discomfort indicated during driving, the handset control values given in each HMI condition were averaged over all participants for each measurement time point along the respective ride. For inferential statistical testing of the discomfort data, we calculated one sum value per participant and HMI condition by summing up all handset control values given by each participant along the course of each ride. We then standardized these sum values per participant to control for individual differences in the sensibility to uncomfortable situations and corresponding usage of the handset control. Therefore, we summarized the handset control values given by each participant during all three rides and then calculated the percentage of each ride in this total sum. These standardized discomfort values indicate how uncomfortable each HMI condition was perceived by each participant in comparison to the other two MHI conditions. All dependent variables were analyzed using mixed design analyses of variance (ANOVAs). We chose an ANOVA procedure, as it is able to compare several means in one analysis, which had two benefits for our study: It minimized the number of tests necessary to compare all of our experimental conditions to one test per dependent variable, and it enabled the detection of interactions between different variables, which we were specifically aiming for. The mixed design ANOVA was chosen based on its fit to our study design, as it could account for the between-subjects factor (initial trust in automated vehicles) as well as the repeated-measures within-subjects factor (HMI condition).
Results
HMI Effects on the Passengers Experience in Automated Vehicles
We compared the participants’ passenger experience rated during/after the three rides along the test track (with no vs. permanent vs. context-adaptive HMI) to examine 1) if presenting driving-related information during automated driving improved passenger experience and whether this improvement was affected by the HMI’s information availability. For these comparisons, we took participants’ trust group membership (lower vs. higher trust group) into account to evaluate 2) whether different user groups did benefit differently from different levels of HMI information availability in automated vehicles. Table 3 provides an overview of all ANOVA test statistics.
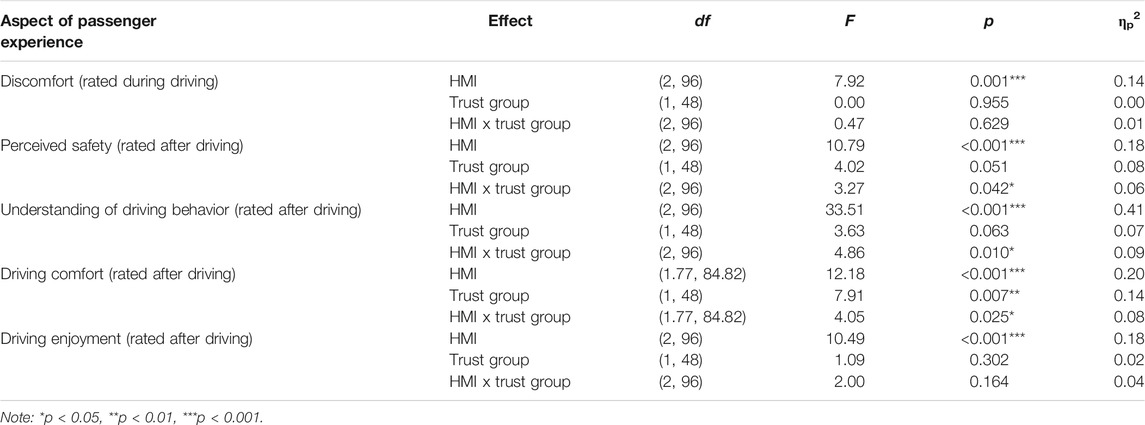
TABLE 3. Mixed design ANOVA results on the effects of HMI condition and trust group membership on the passenger experience in automated driving.
Passenger Experience Indicated During Driving
An ANOVA of the standardized handset control values could not identify a significant effect of trust group membership or a significant interaction between trust group membership and HMI condition. According to these results, participants’ initial trust in automated vehicles did not affect the level of discomfort they indicated during driving via handset control. In contrast, the ANOVA revealed a large, significant effect of HMI condition, which can be assumed for members of both trust groups (see Table 3). According to post hoc tests, participants indicated to perceive significantly higher discomfort during automated driving without HMI than during driving with the permanent HMI (p = 0.004) or the context-adaptive HMI (p = 0.011). Both HMI versions reduced discomfort in a comparable manner, without significant differences between them (p = 1.000).
Figure 3 gives a detailed impression of the discomfort values indicated along the test track. Based on the comparable results for both trust groups, handset control values were averaged for the entire sample in this illustration. The middle section of Figure 3 contains a schematic representation of the test track and information on the information timing of the context-adaptive HMI. Averaged discomfort values indicated by all participants along the test track in each HMI condition are presented in the upper section. The development of these values supports the assumption behind the context-adaptive HMI that more complex traffic situations represent a greater challenge for the passenger experience in automated driving than less complex traffic situations. Thus, discomfort values peaked at all intersections and situations including interactions with other road users. Accordingly, discomfort values in such situations were lower with both HMI versions than without HMI, since both versions provided information in these situations. In contrast, participants indicated almost no discomfort in non-complex traffic situations regardless of the HMI condition. The comparably low discomfort values in such situations were mainly indicated during speed adaptions to changing speed limits.
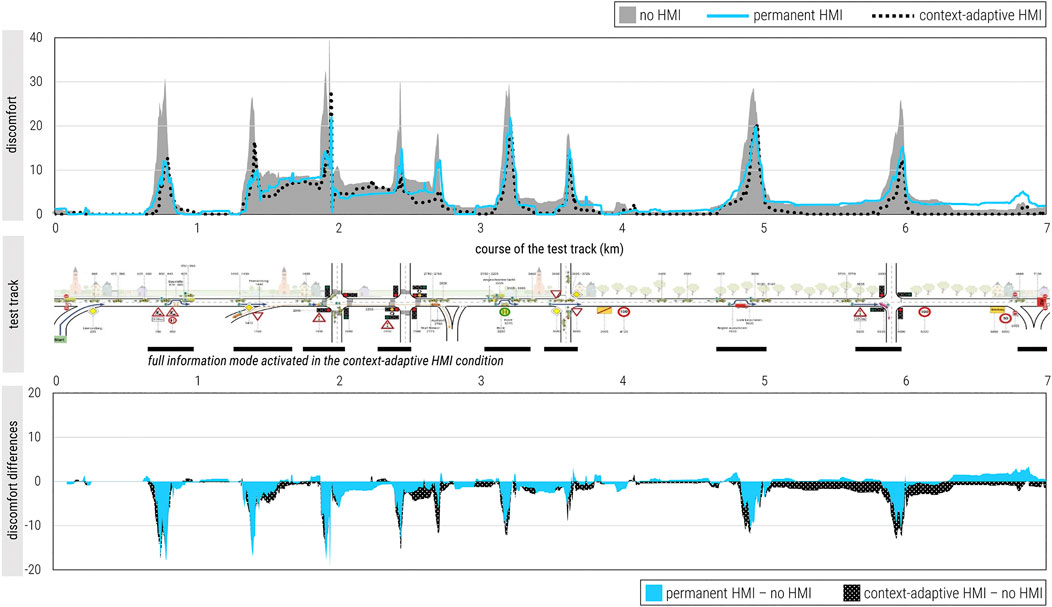
FIGURE 3. Discomfort indicated via handset control during driving along the test track, separated by HMI condition. Note. Higher handset control values represent a more uncomfortable passenger experience indicated during driving (on a scale from 0 to 100). Negative discomfort differences represent an improvement of passenger experience due to the respective HMI; positive values indicate a deterioration.
For a closer comparison of the two HMI versions, the lower section of Figure 3 depicts the differences between the discomfort levels indicated during each of these conditions and the condition without HMI. The displayed differences in handset control values emphasize the situation-specific reduction of perceived discomfort due to both HMI versions. In line with the fact that both HMI versions displayed the identical full information mode in most of the more uncomfortable situations, the figure also illustrates the absence of systematic differences between the effects of the context-adaptive and the permanent HMI on the level of discomfort indicated during driving.
Passenger Experience Rated After Driving
For a broader examination of the passenger experience provided during the three HMI conditions, Figure 4 displays the averaged ratings of perceived safety, understanding of the vehicle’s driving behavior, driving comfort and driving enjoyment given by both trust groups after driving. Overall, the figure indicates that the lower trust group reported a less pleasant driving experience than the higher trust group in almost all cases, but especially for the driving condition without HMI. In addition, both trust groups perceived the driving condition without HMI as least pleasant in terms of all four aspects of passenger experience. Corresponding ANOVAs confirm this impression with highly significant, large HMI effects on all four aspects (see Table 3 for test statistics).
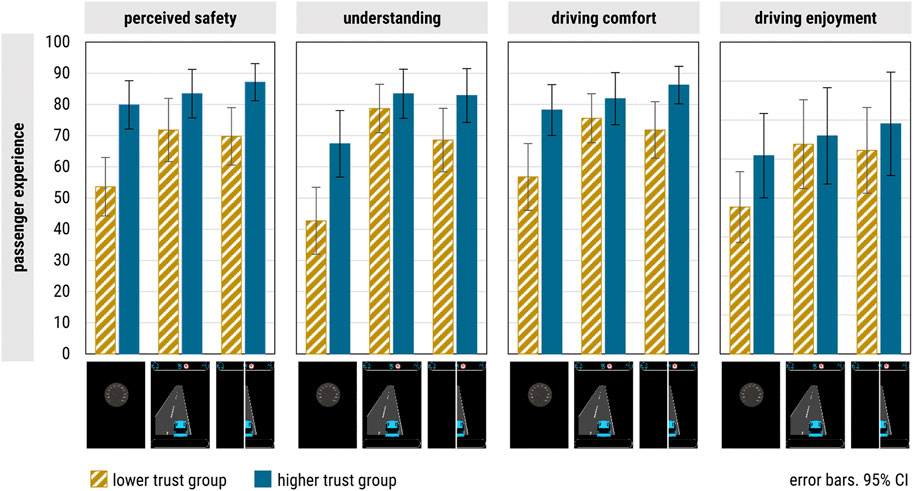
FIGURE 4. Trust group-specific passenger experience reported after automated driving with no (left), permanent (middle) and context-adaptive (right) HMI.
In the cases of perceived safety and driving comfort, these HMI effects varied between the two trust groups, as indicated by significant, medium-sized interactions between HMI condition and trust group membership. While both trust groups reported automated driving to feel least safe/comfortable without HMI, they slightly differed in their safety/comfort evaluation of driving with the two HMI versions. Thus, the higher trust group reported the highest safety/comfort values for the context-adaptive HMI condition, while the lower trust group tended to report the highest safety/comfort values for the permanent HMI condition. Nevertheless, the higher trust group indicated higher perceived safety/comfort than the lower trust group in all HMI conditions, resulting in a significant, large trust group effect on comfort (the trust group effect on perceived safety nearly missed statistical significance). However, since both HMI versions improved the safety/comfort ratings of the lower trust group considerably more than those of the higher trust group, these group differences were strongest in the condition without HMI and decreased under the influence of both HMI versions.
In line with these results, participants’ understanding of the vehicle’s driving behavior was also affected by a significant, medium-sized interaction between HMI condition and trust group membership. Again, ratings given by the two trust groups were lowest in the condition without HMI, but improved differently in the two other HMI conditions: While the higher trust group reported high, but comparable values for both HMI versions, the lower trust group indicated the best understanding of the vehicle’s driving behavior for the permanent HMI.
According to the generally lower driving enjoyment values (in comparison to the other three aspects), automated driving did not provide a highly enjoyable passenger experience. However, compared to driving without HMI, both the permanent HMI (p = 0.002) and the context-adaptive HMI (p < 0.001) increased the participants’ driving enjoyment significantly and to a comparable extend, without significant differences between them (p = 1.000). Given the absence of a significant trust group effect or a significant interaction between HMI condition and trust group membership, these HMI effects can be assumed for both trust groups.
HMI Effects on Passengers’ Trust in Automated Vehicles
We compared the participants’ ratings on the three TiA subscales reliability/competence, understandability/predictability and trust in automation given after experiencing each HMI condition (no vs. permanent vs. context-adaptive HMI) to examine 1) if presenting driving-related information during automated driving improved passengers’ trust in the system and whether this improvement was affected by the HMI’s information availability. These comparisons took the two trust groups (lower vs. higher trust group) into account to evaluate 2) whether different HMI versions provoked different levels of trust among different user groups. Figure 5 gives an overview of the averaged group ratings in all conditions.
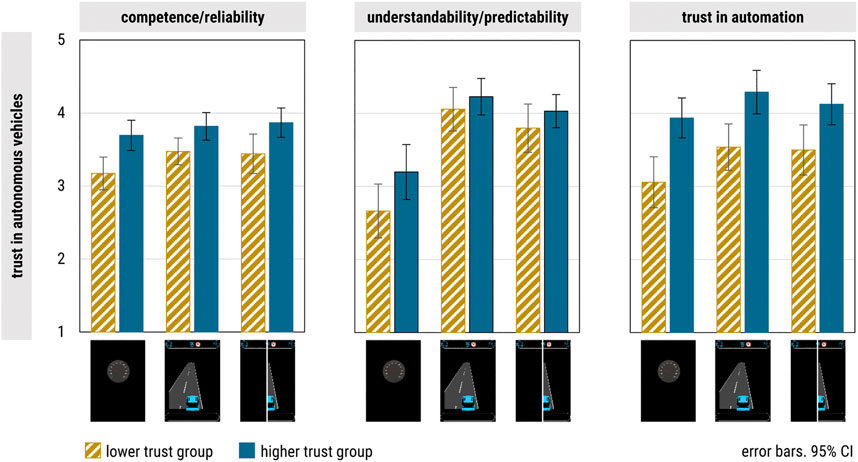
FIGURE 5. Trust group-specific trust in automated vehicles reported after automated driving with no (left), permanent (middle) and context-adaptive (right) HMI.
According to the performed ANOVAs (see Table 4 for test statistics), all examined dimensions of trust were rated significantly lower by the lower trust group than by the higher trust group, which is observable for all HMI conditions. These medium-sized to large group differences become especially apparent for the trust in automation subscale. In additions, ratings regarding all three dimensions differed significantly and with medium to large effect sizes depending on the HMI condition. Thus, ratings were always lowest after driving without an HMI, with values in the slightly negative to medium scale range for the lower trust group and values in the slightly positive scale range for the higher trust group.
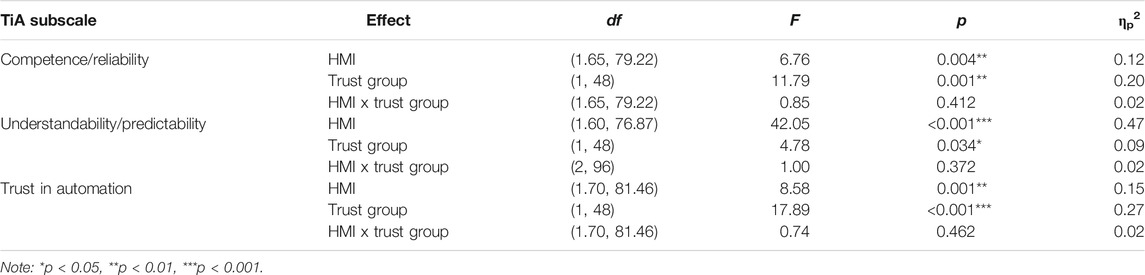
TABLE 4. Mixed design ANOVA results on the effects of HMI condition and trust group membership on passengers’ trust in automated vehicles.
The participants’ evaluation of the vehicles’ competence/reliability was significantly higher after driving with the permanent HMI (p = 0.008) or the context-adaptive HMI (p = 0.026), but did not differ significantly between both HMI versions (p = 1.000). The largest improvements after experiencing the permanent HMI (p < 0.001) or the context-adaptive HMI (p < 0.001) concerned the vehicle’s understandability/predictability. These improvements were comparable for both HMI versions, given the absence of a significant difference between them (p = 0.082). This evaluation of the vehicle’s trustworthiness also reflected in passengers’ attitudes. Thus, the participants reported a significantly higher trust in automation after driving with the permanent HMI (p = 0.001) and the context-adaptive HMI (p = 0.039) than after driving with no HMI. The two HMI versions did not differ significantly in terms of the resulting trust in automation (p = 0.659).
User Experience of the HMI Versions
For a further comparison of the permanent and the context-adaptive HMI 3), we examined the participants’ user experience of both versions based on the six UEQ subscales. This comparison also took into account the participants’ trust group membership (lower vs. higher trust group) in order to identify potential user group-specific HMI preferences.
As shown in Figure 6, members of both trust groups evaluated both HMI versions positively on all user experience dimensions except novelty. The figure also indicates that the context-adaptive HMI was evaluated more positively than the permanent HMI on all dimensions, which is confirmed by significant, medium to large HMI effects revealed in the corresponding ANOVAS (see Table 5 for test statistics). These HMI effects can be assumed for the lower and the higher trust group, given the absence of significant trust group effects or significant interactions between HMI condition and trust group membership. As only exception, we found a medium-sized, significant interaction effect for the attractiveness subscale, which represents the participants’ overall impressions of the HMIs. According to this effect, the permanent HMI was perceived as more attractive by the lower trust group than by the higher trust group, while the context-adaptive HMI received higher attractiveness ratings from the higher trust group than from the lower trust group. Nevertheless, both trust groups evaluated the context-adaptive HMI as more attractive than the permanent HMI, but the difference between both HMI versions was considerably more pronounced for the higher trust group than for the lower trust group.
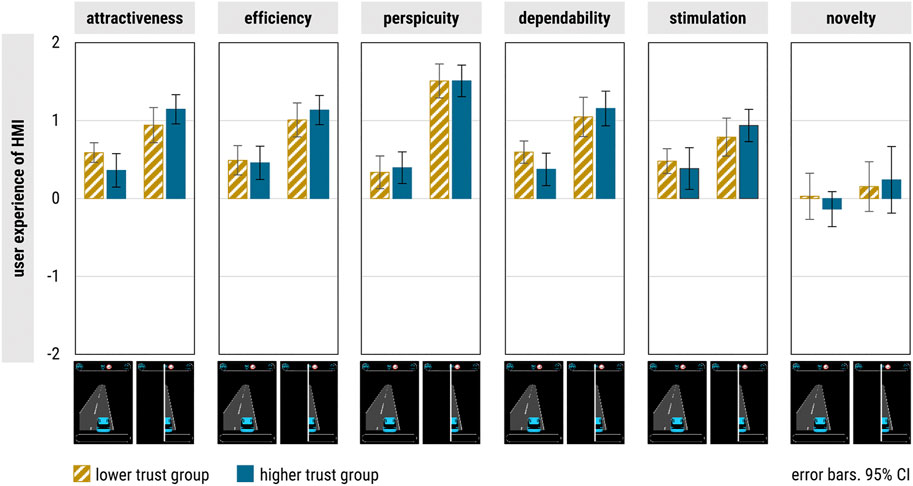
FIGURE 6. Trust group-specific user experience provided by the permanent (left) and context-adaptive (right) HMI.
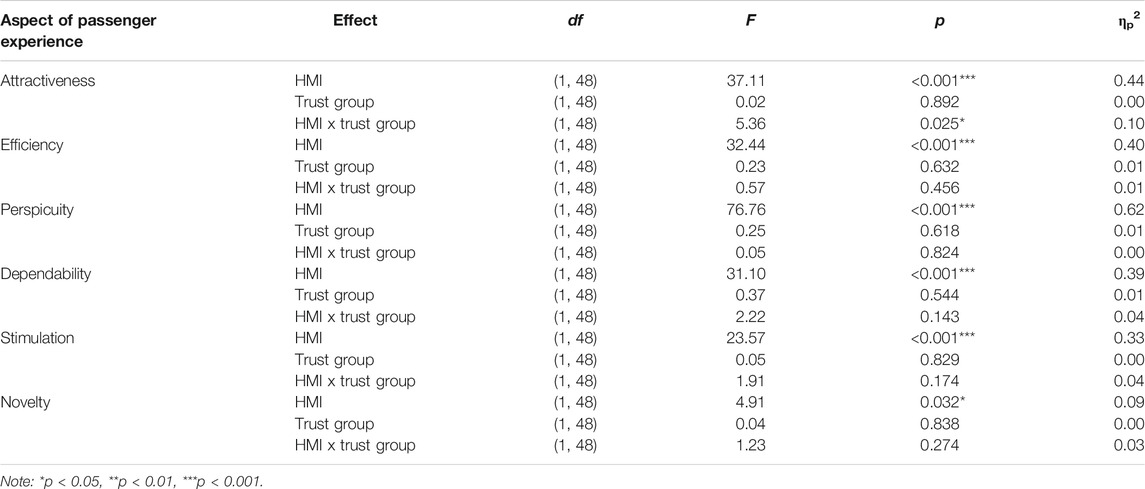
TABLE 5. Mixed design ANOVA results on the effects of HMI version and trust group membership on HMI-Related user experience.
Discussion
In the reported driving simulator study, we 1) evaluated the potential of in-vehicle HMIs to enhance vehicle occupants’ passenger experience and trust at higher levels of driving automation, such as highly and fully automated vehicles (SAE-Levels 4–5). We further evaluated the demand for user-adaptive HMI concepts in this context by examining 2) whether different HMI versions can optimize the passenger experience and trust of different user groups and 3) would be preferred by different user groups in terms of user experience. Therefore, 50 participants with lower vs. higher initial trust in automated vehicles experienced three highly automated rides with different HMI conditions (no vs. permanent vs. context-adaptive HMI) along a diverse route in a driving simulator.
Summary and Interpretation of Results
The first aim of this study was to verify that increasing the system transparency of higher-level automated vehicles via HMI improves passenger experience and trust. Indeed, both HMI versions significantly improved all examined aspects of passenger experience and trust in automated driving. Thus, participants experienced automated driving with an HMI as safer, more understandable, more comfortable and more enjoyable than without an HMI. Accordingly, they evaluated the automated vehicle as more reliable, predictable and trustworthy with than without an HMI. These results confirm our hypothesis that presenting driving-related information during driving supports a pleasant and trustworthy passenger experience even in higher-level automated vehicles, where human monitoring is actually no longer required. Thereby, they consolidate first indications of positive HMI effects on passengers’ trust in the automated vehicle, which were previously available for a few selected driving situations (Wintersberger et al., 2019; Oliveira et al., 2020), and clarify their validity for more realistic (i.e. longer and more diverse) routes. In addition, they point out the broad benefits of such HMI concepts for different aspects of passenger experience. In sum, the study demonstrates the potential of in-vehicle HMIs to improve two important predictors (passenger experience and trust) of the user acceptance and therefore market penetration of higher-level automated vehicles.
Our second aim was to examine whether different user groups would particularly benefit from different HMI versions customized to their group-specific information needs. The two HMI versions included in this study differed in their information availability over time (permanent vs. context-adaptive), which served as an empirically derived example for HMI features that could be adapted to varying user requirements. Based on the findings of a preceding driving simulator study (Hartwich et al., 2020), we supposed that the passenger experience and trust of passengers with lower initial trust might improve the most based on the permanent HMI, while passengers with higher initial trust might benefit most from the context-adaptive HMI. In line with these expectations, we identified significant interaction effects supporting the assumption of group-specific HMI effects for most aspects of passenger experience. Thus, the lower trust group experienced automated driving as safest, most understandable and most comfortable with the permanent HMI, while the higher trust group tended to experience the highest safety, understanding and comfort with the context-adaptive HMI. Deviating results were obtained for driving enjoyment and driving discomfort. Regarding driving enjoyment, both HMIs improved both user group ratings to a comparable extend. Thus, both groups were able to enjoy automated driving more with than without an HMI, regardless of the HMI version. Nevertheless, enjoyment ratings were generally lower than most other ratings related to passenger experience, which is in line with previous research pointing out the impairing effects of driving automation on driving enjoyment (Hartwich et al., 2018). It is not completely surprising that these impairments are not fully compensated by HMI concepts without any entertainment functions. Driving discomfort indicated during driving via handset control represents the greater deviation, since it did not seem to be affected by trust group membership in all three HMI conditions, which stands in contrast to the driving comfort ratings indicated via questionnaire. These differences between handset control and questionnaire ratings could have methodological as well as content-related causes. Methodologically, it is possible that the participants had difficulties in indicating exact values using the handset control without visual feedback despite the training during the familiarization drive. Therefore, the handset control might be able to identify larger discomfort differences, which appeared between different traffic situations or between driving with vs. without HMI, but it might be unsuitable to identify smaller differences, as between the two trust groups or the two HMI versions. Content-related, the differences between both assessment methods could implicate that retrospective evaluations made after driving are more than the sum or mean of all real-time evaluations made during driving. Thus, the retrospective questionnaire ratings, which are more reliant on memory, might be affected more strongly by already existing attitudes than the real-time ratings during driving. As a result, initial trust differences would reflect stronger in questionnaire ratings than in handset control values. Further research is required to follow up on these considerations. Regarding trust in automated driving rated after system experience, both HMI versions did not result in group-specific improvements. Instead, all aspects of trust increased significantly for both trust groups after driving with both HMIs. Thus, passengers evaluated automated driving with HMI as more reliable, predictable and trustworthy than without HMI, regardless of the HMI version.
For a closer comparison of the two HMIs, a third aim of the study was to examine whether users with different levels of initial trust in automated driving would prefer the user experience of different HMI versions customized to their information needs. In general, members of both groups evaluated the user experience of both HMI versions positively. Thereby, the context-adaptive HMI generally received even more positive ratings than the permanent HMI. Interestingly, this result applied to both trust groups, even though the preference for the context-adaptive HMI tended to be more pronounced for the higher trust group. In case of attractiveness, which represents users’ overall impressions of the HMIs and therefore the most global aspect of user experience, this tendency resulted in a significant interaction. Thus, the permanent HMI was perceived as more attractive by the lower trust group than by the higher trust group, while the context-adaptive HMI received higher attractiveness ratings from thy higher trust group than from the lower trust group. Among the two HMI versions evaluated in this study, these results imply a general preference for the context-adaptive HMI in terms of user experience, but do not rule out the possibility of user group-specific HMI preferences.
Strengths, Limitations, and Future Research
The reported driving simulator study represents one of few attempts so far to evaluate the potential benefits of increasing system transparency through in-vehicle HMIs in higher-level automated vehicles (SAE-Levels 4–5). In addition to previous research focusing on benefits for users’ trust in automation (Wintersberger et al., 2019; Oliveira et al., 2020), special emphasis was thereby placed on various aspects of their passenger experience. While there is an increasing demand for user-adaptive HMI concepts meeting varying user requirements (Feierle et al., 2020), this approach has not been evaluated systematically yet. In an attempt to close this research gap, the study provided first data on user group-specific HMI effects at higher levels of driving automation. While the results clearly point out the overall positive HMI effects on passenger experience and trust in automated driving, they are not completely consistent regarding user group-specific effects. While both trust groups’ passenger experience could be optimized by different HMI versions in line with a user-adaptive HMI approach, their improvement of trust and their evaluation of the HMIs did not differ systematically between both HMI versions (even though corresponding trends were observable for several variables). Reasons for the inconsistent results might stem from some methodological limitations of the study, which should be addressed in future research in order to evaluate the approach of user-adaptive HMI concepts in more detail.
First, the simulated driving environment might have decreased the potential to discover rather nuanced interindividual differences based on its restricted external validity. While driving simulations currently represent the only method to provide the experience of higher levels of driving automation along realistic (i.e. diverse and rather long) routes, the participants probably felt safer and more comfortable in this simulated than in a real traffic environment based on their awareness that system failures would not have had actual safety effects. As this issue directly affects the dependent variables of the study, differences between the trust groups or HMI conditions could be underestimated in our results and should therefore be evaluated in real automated vehicles in the future.
Second, the study’s sample was not representative for the population and might have been too homogeneous in terms of initial trust in automation. Since participation in the study was voluntary, it was subject to some degree of self-selection and therefore may have led to a sample with an above-average interest in technology or general openness for automated driving. Indeed, the two trust groups did not represent extreme groups with totally low vs. totally high initial trust in automated driving, but somewhat artificial categories based on a median split. Therefore, we expect group-specific effects of the two HMI versions to be much more pronounced in samples that are more representative and thus heterogeneous. In addition, individual information needs during automated driving might not only depend on initial attitudes, but also on other user characteristics, such as personality traits (Dettmann et al., 2021), manual driving habits (Mühl et al., 2019), or demographic factors (Hartwich et al., 2019). Therefore, user groups based on additional user characteristics might have been even more responsive to customized HMI versions. Further, we cannot exclude the possibility that the sample was not representative in terms of user characteristics relevant for the dependent variables of our study, which would limit the generalizability of the results. Apparent examples might be the age and gender ratio of the sample. Even though both characteristics were comparable between both trust groups, younger women were generally overrepresented in the sample, which might restrict the validity of the results for other age groups and genders. For these reasons, further examinations of user group-specific HMI effects based on more heterogeneous sampling are desirable.
Starting from the first insights provided by this study, there is still much to learn. For example, it would be interesting for further research to include non-driving related activities in the study design. Passengers’ engagement in such activities could serve as a behavioral measure for trust in automation and therefore provide further insight in user group-specific HMI effects. In addition, it should be examined to what extend the effects and user experience of both HMI versions could change due to the opportunity to pursue such activities during driving. Further, our study captured passengers’ first experiences of higher-level automated driving. However, some participants mentioned that their information requirements might decrease after a longer period of usage. Therefore, the evolution of passenger experience, trust and HMI effects in the long-term usage of automated vehicles is an important topic for future research.
Practical Implications
On a conceptual level, the results strengthen the relationship between passenger experience and trust in automated vehicles, which appear to be closely related to each other as well as commonly related to system properties, such as system transparency. In addition, the persistent differences between the two trust groups demonstrate that passenger experience and trust in automated vehicles are not only affected by system properties, but also by rather stable user characteristics. These results strengthen the increasing demand for user-adaptive HMI concepts addressing individually differing user characteristics (Feierle et al., 2020).
At application level, the study highlights the potential of in-vehicle HMIs to support a pleasant and trustworthy passenger experience in higher-level automated vehicles. Displaying driving-related information that enable passengers to monitor the automated driving system if desired improved all examined aspects of the participants’ passenger experience and trust. These improvements were especially pronounced for passengers with a lower initial trust in driving automation, indicating the particular HMI benefits for persons that are otherwise expected to be less willing to use automated vehicles. Given the importance of passenger experience and trust as predictors of potential users’ acceptance of driving automation (Choi and Ji, 2015; Xu et al., 2018; Zoellick et al., 2019), we therefore expect in-vehicle HMIs to make a significant contribution to the future market acceptance and penetration of automated vehicles. For those vehicles still offering the opportunity for humans to intervene in the driving task if desired, such HMIs might even provide additional benefits related to driving safety. Thus, increasing passengers’ perceived safety and comfort as well as trust via HMI might prevent them from unnecessarily taking over the driving task based on distrust in the automated driving system’s abilities in a specific situation. Thereby, the HMI might not only benefit automated vehicle users, but other road users, as well. Summarizing these considerations, we recommend leaving passengers of higher-level automated vehicles the option to inform themselves on the system status, detected elements of the traffic situations and upcoming driving maneuvers during driving via in-vehicle HMIs.
Regarding the demand to design user-adaptive HMI concepts meeting varying user requirements (Feierle et al., 2020) instead of a one-size-fits-all-HMI, the study provides first empirical indications that strengthen this approach. Even though user group-specific effects of the two evaluated HMI versions could not be identified for all examined variables, the passenger experience of users with lower vs. higher initial trust was optimized by different levels of information availability of the HMI (indicated by significant interaction effects). Thus, passengers with lower initial trust felt most comfortable and safe when driving-related information were presented permanently, while passengers with higher initial trust felt most comfortable and safe when driving-related information were only presented in selected driving situations. Considering the methodological limitations of the study (see Strengths, Limitations, and Future Research), we expect such user group-specific HMI effects to be more pronounced and consistent in real-world environments and for more heterogeneous samples. Based on these results, we cannot recommend providing full information permanently during driving as a universal standard for in-vehicle HMIs in higher-level automated vehicles. While HMIs with such a permanent information availability might be the most fitting HMI solution for some passengers (e.g. those with low initial trust), it might not optimize the passenger experience of others (e.g. those with high initial trust). Therefore, we support the approach of user-adaptive HMIs that are able to customize their features (e.g. information availability over time) to different users with varying requirements. However, further research is required in order to identify additional relevant user characteristics determining varying HMI demands as well as additional relevant HMI features to address these demands.
Since our study focused on system-inexperienced users, it might be possible that the design recommendations derived from the results will be especially relevant during the early stages of market penetration, when unexperienced passengers need to build adequate trust in automated vehicles. However, we believe that system transparency and individual adaptability could also remain important beyond these early stages within the scope of modern car sharing concepts with constantly changing vehicle users, which will be necessary to achieve the potential environmental benefits of automated vehicles. In both application context, HMIs increasing the system transparency of automated vehicles could be a decisive factor to make driving automation more accessible for those groups of people who would otherwise refrain from using them due to their mistrust in the technology. The user-specific adaptability of HMI features (such as information availability) for an optimized passenger experience could be realized through active user input, automated user state recognition (Beggiato et al., 2019) or user profiles (Drewitz et al., 2019).
Conclusion
In summary, this research demonstrates the huge potential of in-vehicle HMIs increasing the system transparency of automated vehicles and thereby enabling passengers to monitor the system at higher levels of driving automation (SAE-Levels 4–5), even though human monitoring or intervention is actually no longer required at these automation levels. By significantly enhancing vehicle occupants’ passenger experience and trust in the automated vehicle, such HMIs could make a considerable contribution to the future acceptance and consequently market penetration of highly and fully automated vehicles. Further, the study provides first empirical indications that strengthen the approach to design user-adaptive HMI concepts meeting varying user requirements instead of one-size-fits-all-HMIs. Based on the indicated potential to optimize different user groups’ passenger experience with different HMI versions, user-adaptive HMI concepts could enable very different user groups to feel comfortable and safe in automated vehicles. Once such concepts are fully developed based on further research, user-specific adaptability could be an important factor to gain a broad acceptance and market penetration of driving automation.
In the broader context, is has already been established that the expected benefits of driving automation will only be achieved if potential users will feel comfortable to hand over vehicle control to automated driving systems and consequently use them on a regular basis. By supporting these requirements through enhanced passenger experience and trust, HMI-based system transparency and user-specific adaptability could represent two steps on the way toward the realization of the versatile benefits which driving automation is promising for our future mobility and society.
Data Availability Statement
The raw data supporting the conclusions of this article are available on request to the corresponding author.
Ethics Statement
The study was carried out in accordance with the Declaration of Helsinki. Ethical review and approval was not required for the study in accordance with the local legislation and institutional requirements. The participants were thoroughly informed and provided their written informed consent to participate in this study.
Author Contributions
All authors, FH, CH, DJ, and JK, contributed to the conception and design of the presented driving simulator study. FH and CH were responsible for data acquisition and analysis. DJ programmed the evaluated HMIs, which were conceptualized by FH, CH, and DJ. JK performed supervision of the whole research process. FH wrote the initial draft of this paper, which was critically reviewed by CH, DJ, and JK including comments and revisions.
Funding
This study is part of the research project AutoAkzept, which was funded by the German Federal Ministry of Transport and Digital Infrastructure (BMVI) under grant no. 16AVF2126E. The publication of this article was funded by Chemnitz University of Technology.
Conflict of Interest
The authors declare that the research was conducted in the absence of any commercial or financial relationships that could be construed as a potential conflict of interest.
Acknowledgments
The authors would like to thank the professorship of Ergonomics and Innovation Management of Chemnitz University of Technology for renting out their driving simulator for this study. We also thank Lars Weber for his HMI programming support. Special thanks goes to Sebastian Scholz for his outstanding commitment to driving simulator programming and HMI implementation.
References
Anderson, J., Kalra, N., Stanley, K., Sorensen, P., Samaras, C., and Oluwatola, O. (2016). Autonomous Vehicle Technology: A Guide for Policymakers. Santa Monica, CA: RAND CorporationAvailable at: http://www.rand.org/pubs/research_reports/RR443-2.html.
Araujo, H. L. S., Verdezoto, N. X., Wali, S., Damasceno, C. D. N., Dimitrova, R., Kefalidou, G., et al. (2019). “Trusted Autonomous Vehicles: An Interactive Exhibit,” In 2019 IEEE International Conferences On Ubiquitous Computing Communications (IUCC) and Data Science And Computational Intelligence (DSCI) and Smart Computing Networking Service (Smartcns), Shenyang, China, October 21–23, 2019, 386–393. doi:10.1109/IUCC/DSCI/SmartCNS.2019.00091
Bauerfeind, K., Stephan, A., Hartwich, F., Othersen, I., Hinzmann, S., and Bendewald, L. (2017). “Analysis of Potentials of an HMI-Concept Concerning Conditional Automated Driving for System-Inexperienced vs. System-Experienced Users”, in Proceedings of the Human Factors and Ergonomics Society Europe Chapter 2017 Annual Conference (online). Editors D. de Waard, F. Di Nocera, D. Coelho, J. Edworthy, K. Brookhuis, F. Ferlazzoet al. (Rome, Italy). Available at: https://www.hfes-europe.org/wp-content/uploads/2017/10/Bauerfeind2017.pdf.
Beggiato, M., Hartwich, F., Schleinitz, K., Krems, J., Othersen, I., and Petermann-Stock, I. (2015). What Would Drivers like to Know during Automated Driving? Information Needs at Different Levels of Automation. Munich, Germany. doi:10.13140/RG.2.1.2462.6007
Beggiato, M., Hartwich, F., and Krems, J. (2019). Physiological Correlates of Discomfort in Automated Driving. Transportation Res. F: Traffic Psychol. Behav. 66, 445–458. doi:10.1016/j.trf.2019.09.018
Choi, J. K., and Ji, Y. G. (2015). Investigating the Importance of Trust on Adopting an Autonomous Vehicle. Int. J. Human-Computer Interaction 31 (10), 692–702. doi:10.1080/10447318.2015.1070549
Constantin, D., Nagi, M., and Mazilescu, C.-A. (2014). Elements of Discomfort in Vehicles. Proced. - Soc. Behav. Sci. 143, 1120–1125. doi:10.1016/j.sbspro.2014.07.564
Dettmann, A., Hartwich, F., Roßner, P., Beggiato, M., Felbel, K., Krems, J., et al. (2021). Comfort or Not? Automated Driving Style and User Characteristics Causing Human Discomfort in Automated Driving. Int. J. Human-Computer Interaction 37 (4), 331–339. doi:10.1080/10447318.2020.1860518
Diels, C., and Thompson, S. (2018). “Information Expectations in Highly and Fully Automated Vehicles,” in Advances In Human Aspects Of Transportation. Editor N. A. Stanton (Cham: Springer International Publishing), 742–748. doi:10.1007/978-3-319-60441-1_71
Drewitz, U., Ihme, K., Oehl, M., Frank, S., Voßwinkel, R., Hartwich, F., et al. (2019). “Automation Ohne Unsicherheit: Vorstellung Des Förderprojekts AutoAkzept Zur Erhöhung Der Akzeptanz Automatisierten Fahrens [Automation without Uncertainty: Introducing the Research Projekt AutoAkzept for Enhanc-Ing the Acceptance of Automated Driving].” in Mensch-Maschine-Mobilität 2019. Der (Mit-)Fahrer Im 21. Jahrhundert!?, Braunschweig, Germany, November 05–06, 2019. Editors V. D. I. Berichte. Düsseldorf: VDI-Verlag 2360, 1–9.
Ekman, F., Johansson, M., and Sochor, J. (2018). Creating Appropriate Trust in Automated Vehicle Systems: A Framework for HMI Design. IEEE Trans. Human-mach. Syst. 48 (1), 95–101. doi:10.1109/THMS.2017.2776209
Elbanhawi, M., Simic, M., and Jazar, R. (2015). In the Passenger Seat: Investigating Ride Comfort Measures in Autonomous Cars. IEEE Intell. Transport. Syst. Mag. 7 (3), 4–17. doi:10.1109/MITS.2015.2405571
Engelbrecht, A. (2013). Fahrkomfort Und Fahrspaß Bei Einsatz von Fahrerassistenzsystemen [Driving Comfort and Driving Enjoyment When Using ADAS]. Hamburg: Disserta-Verlag.
ERTRAC (2019). Connected Automated Driving Roadmap. Brussels, Belgium: The European Road Transport Research Advisory CouncilAvilable at: https://www.ertrac.org/uploads/documentsearch/id57/ERTRAC-CAD-Roadmap-2019.pdf.
Feierle, A., Danner, S., Steininger, S., and Bengler, K. (2020). Information Needs and Visual Attention during Urban, Highly Automated Driving-An Investigation of Potential Influencing Factors. Information 11 (2), 62. doi:10.3390/info11020062
Fraedrich, Eva., and Lenz, Barbara. (2015). “Gesellschaftliche Und Individuelle Akzeptanz Des Autonomen Fahrens [Social and Individual Acceptance of Autonomous Driving],” in Autonomes Fahren: Technische, Rechtliche Und Gesellschaftliche Aspekte. Editors M. Maurer, J. Christian Gerdes, and B. Lenz. and H. Winner (Berlin, Heidelberg: Springer), 639–660.
Frison, A.-K., Wintersberger, P., Liu, T., and Riener, A. (2019). “Why Do You like to Drive Automated?: A Context-Dependent Analysis of Highly Automated Driving to Elaborate Requirements for Intelligent User Interfaces,” in IUI ’19: Proceedings of the 24th International Conference On Intelligent User Interfaces, Marina del Ray, CA, March 17-20, 2019. Editors W. T. Fu, S. Pan, O. Brdiczk, P. Chau, and G. Calvary (New York: ACM), 528–537. doi:10.1145/3301275.3302331
Hartwich, F., Beggiato, M., and Krems, J. F. (2018). Driving comfort, Enjoyment and Acceptance of Automated Driving - Effects of Drivers' Age and Driving Style Familiarity. Ergonomics 61 (8), 1017–1032. doi:10.1080/00140139.2018.1441448
Hartwich, F., Schmidt, C., Gräfing, D., and Krems, J. F. (2020). “In the Passenger Seat: Differences in the Perception of Human vs. Automated Vehicle Control and Resulting HMI Demands of Users,” in Automated Driving and In-Vehicle Experience Design. Editor H Krömker (Cham: Springer International Publishing), 31–45. doi:10.1007/978-3-030-50523-3_3
Hartwich, F., Witzlack, C., Beggiato, M., and Krems, J. F. (2019). The First Impression Counts - A Combined Driving Simulator and Test Track Study on the Development of Trust and Acceptance of Highly Automated Driving. Transportation Res. Part F: Traffic Psychol. Behav. 65, 522–535. doi:10.1016/j.trf.2018.05.012
Hassenzahl, M. (2008). “User Experience (UX): Towards an Experiential Perspective on Product Quality,” in Proceedings Of the 20th Conference On l’Interaction Homme-Machine, Metz, France, September 02–05, 2008. Editor. E. Brangier, G. Michel, J. M. Christian Bastien, and N. Carbonell (New York: Association for Computing Machinery), 11–15. doi:10.1145/1512714.1512717
Hoff, K. A., and Bashir, M. (2015). Trust in Automation. Hum. Factors 57 (3), 407–434. doi:10.1177/0018720814547570
König, M., and Neumayr, L. (2017). Users' Resistance towards Radical Innovations: The Case of the Self-Driving Car. Transportation Res. Part F: Traffic Psychol. Behav. 44, 42–52. doi:10.1016/j.trf.2016.10.013
Körber, M. (2019). “Theoretical Considerations and Development of a Questionnaire to Measure Trust in Automation,” in Advances in Intelligent Systems and Computing. Editors S. Bagnara, R. Tartaglia, S. Albolino, T. Alexander, and Y. Fujita (Cham: Springer), 13–30. doi:10.1007/978-3-319-96074-6_2
Kraus, J., Scholz, D., Stiegemeier, D., and Baumann, M. (2020). The More You Know: Trust Dynamics and Calibration in Highly Automated Driving and the Effects of Take-Overs, System Malfunction, and System Transparency. Hum. Factors 62 (5), 718–736. doi:10.1177/0018720819853686
Kyriakidis, M., de Winter, J. C. F., Stanton, N., Bellet, T., van Arem, B., Brookhuis, K., et al. (2017). A Human Factors Perspective on Automated Driving. Theor. Issues Ergon. Sci. 20 (3), 223–249. doi:10.1080/1463922X.2017.1293187
Kyriakidis, M., Happee, R., and de Winter, J. C. F. (2015). Public Opinion on Automated Driving: Results of an International Questionnaire Among 5000 Respondents. Transportation Res. Part F: Traffic Psychol. Behav. 32, 127–140. doi:10.1016/j.trf.2015.04.014
Lau, M., Wilbrink, M., Dodiya, J., and Oehl, M. (2020). “Users' Internal HMI Information Requirements for Highly Automated Driving,” in In HCI International 2020 – Late Breaking Posters, Copenhagen, Denmark, July 19–24, 2020. Editors C. Stephanidis, M. Antona, and S. Ntoa, (Cham: Springer International Publishing), 585–592. doi:10.1007/978-3-030-60703-6_75
Laugwitz, B., Held, T., and Schrepp, M. (2008). “Construction and Evaluation of a User Experience Questionnaire,” In HCI and Usability For Education And Work. USAB 2008, Graz, Austria, November 20–21, 2008. Editors A. Holzinger Lecture Notes in Computer Science (Berlin, Heidelberg: Springer), 5298, 63–76. doi:10.1007/978-3-540-89350-9_6
Lee, J. D., and See, K. A. (2004). Trust in Automation: Designing for Appropriate Reliance. hfes 46 (1), 50–80. doi:10.1518/hfes.46.1.50_3039210.1518/hfes.46.1.50.30392
Littman, Todd. (2020). “Autonomous Vehicle Implementation Predictions Implications for Transport Planning”, Victoria, Canada: Victoria Transport Policy InstituteAvailable at: https://www.vtpi.org/avip.pdf.
McKee, S. P., and Nakayama., K. (1984). The Detection of Motion in the Peripheral Visual Field. Vis. Res. 24 (1), 25–32. doi:10.1016/0042-6989(84)90140-8
Mühl, K., Strauch, C., Grabmaier, C., Reithinger, S., Huckauf, A., and Baumann, M. (2019). Get Ready for Being Chauffeured. Hum. Factors 62 (8), 1322–1338. doi:10.1177/0018720819872893
Oliveira, L., Burns, C., Luton, J., Iyer, S., and Birrell, S. (2020). The Influence of System Transparency on Trust: Evaluating Interfaces in a Highly Automated Vehicle. Transportation Res. Part F: Traffic Psychol. Behav. 72, 280–296. doi:10.1016/j.trf.2020.06.001
Parasuraman, R., and Riley, V. (1997). Humans and Automation: Use, Misuse, Disuse, Abuse. Hum. Factors 39 (2), 230–253. doi:10.1518/001872097778543886
SAE International (2018). “Taxonomy and Definitions for Terms Related to Driving Automation Systems for On-Road Motor Vehicles”, J3016_201806. Available at: https://www.sae.org/standards/content/j3016_201806 (Accessed January 05, 2020).
Schmidt, C., Hartwich, F., and Krems, J. F. (2020). “Looking at Driving Automation from a Passenger's Perspective: Driving Experience and Gaze Behavior during Fully Automated vs. Human Vehicle Control,” in Human Interaction, Emerging Technologies And Future Applications IIIT, Paris, France, August 27–29, 2020. Editors A. Ahram, R. Taiar, K. Langlois, and A. Choplin (Cham: Springer International Publishing), 3–9. doi:10.1007/978-3-030-55307-4_1
Schoettle, B., and Sivak, M. (2014). “Public Opinion about Self-Driving Vehicles in China. India, Japan, the U S, the U.K., and Australia”, UMTRI-2014-30 Ann Arbor, MI: the U.S. The University of Michigan. doi:10.1109/iccve.2014.7297637 Available at: https://deepblue.lib.umich.edu/bitstream/handle/2027.42/109433/103139.pdf (Accessed January 05, 2021).
Shariff, A., Bonnefon, J.-F., and Rahwan, I. (2017). Psychological Roadblocks to the Adoption of Self-Driving Vehicles. Nat. Hum. Behav. 1 (10), 694–696. doi:10.1038/s41562-017-0202-6
Sheridan, T. B., and Hennessy, R. T. (1984). Research and Modeling of Supervisory Control Behavior: Report of a Workshop. Washington, DC: The National Academies Press. doi:10.17226/1937610.21236/ada149621
Strauch, C., Mühl, K., Patro, K., Grabmaier, C., Reithinger, S., Baumann, M., et al. (2019). Real Autonomous Driving from a Passenger's Perspective: Two Experimental Investigations Using Gaze Behaviour and Trust Ratings in Field and Simulator. Transportation Res. Part F: Traffic Psychol. Behav. 66, 15–28. doi:10.1016/j.trf.2019.08.013
Tasoudis, S., and Perry, M. (2018). Participatory Prototyping to Inform the Development of a Remote UX Design System in the Automotive Domain. Mti 2 (4), 74. doi:10.3390/mti2040074
Wintersberger, P., Frison, A.-K., Riener, A., and Sawitzky, T. V. (2019). Fostering User Acceptance and Trust in Fully Automated Vehicles: Evaluating the Potential of Augmented Reality. PRESENCE: Virtual and Augmented Reality 27 (1), 46–62. doi:10.1162/pres_a_00320
Wolf, I. (2016). “The Interaction between Humans and Autonomous Agents,” In Autonomous Driving: Technical, Legal And Social Aspects. Editors M Maurer, J. Christian Gerdes, B Lenz, and W Hermann (Berlin, Heidelberg: Springer), 103–124. doi:10.1007/978-3-662-48847-8_6
Xu, Z., Zhang, K., Min, H., Wang, Z., Zhao, X., and Liu, P. (2018). What Drives People to Accept Automated Vehicles? Findings from a Field Experiment. Transportation Res. C: Emerging Tech. 95, 320–334. doi:10.1016/j.trc.2018.07.024
Zoellick, J. C., Kuhlmey, A., Schenk, L., Schindel, D., and Blüher, S. (2019). Amused, Accepted, and Used? Attitudes and Emotions towards Automated Vehicles, Their Relationships, and Predictive Value for Usage Intention. Transportation Res. Part F: Traffic Psychol. Behav. 65, 68–78. doi:10.1016/j.trf.2019.07.009
Keywords: in-vehicle human-machine interface (HMI), system transparency, driving comfort, discomfort, trust in automation, individual user characteristics, driving simulator study, autonomous driving (AD)
Citation: Hartwich F, Hollander C, Johannmeyer D and Krems JF (2021) Improving Passenger Experience and Trust in Automated Vehicles Through User-Adaptive HMIs: “The More the Better” Does Not Apply to Everyone. Front. Hum. Dyn 3:669030. doi: 10.3389/fhumd.2021.669030
Received: 17 February 2021; Accepted: 08 June 2021;
Published: 28 June 2021.
Edited by:
Genovefa Kefalidou, University of Leicester, United KingdomReviewed by:
Andrea Bunt, University of Manitoba, CanadaMaria Mikela Chatzimichailidou, WSP Civils, United Kingdom
Copyright © 2021 Hartwich, Hollander, Johannmeyer and Krems. This is an open-access article distributed under the terms of the Creative Commons Attribution License (CC BY). The use, distribution or reproduction in other forums is permitted, provided the original author(s) and the copyright owner(s) are credited and that the original publication in this journal is cited, in accordance with accepted academic practice. No use, distribution or reproduction is permitted which does not comply with these terms.
*Correspondence: Franziska Hartwich, franziska.hartwich@psychologie.tu-chemnitz.de