- 1Department of Psychology, Hunter College, The City University of New York, New York, NY, United States
- 2Department of Communication, University of California, Davis, Davis, CA, United States
- 3Research Group Computational Auditory Perception, Max Planck Institute for Empirical Aesthetics, Frankfurt, Germany
- 4Princeton and National Bureau of Economic Research, Department of Sociology and Office of Population Research, Princeton University, Princeton, NJ, United States
To solve the problems they face, online communities adopt comprehensive governance methods including committees, boards, juries, and even more complex institutional logics. Helping these kinds of communities succeed will require categorizing best practices and creating toolboxes that fit the needs of specific communities. Beyond such applied uses, there is also a potential for an institutional logic itself to evolve, taking advantage of feedback provided by the fast pace and large ecosystem of online communication. Here, we outline an experimental strategy aiming at guiding and facilitating such an evolution. We first review the advantages of studying collective action using recent technologies for efficiently orchestrating massive online experiments. Research in this vein includes attempts to understand how behavior spreads, how cooperation evolves, and how the wisdom of the crowd can be improved. We then present the potential usefulness of developing virtual-world experiments with governance for improving the utility of social feedback. Such experiments can be used for improving community rating systems and monitoring (dashboard) systems. Finally, we present a framework for constructing large-scale experiments entirely in virtual worlds, aimed at capturing the complexity of governance dynamics, to empirically test outcomes of manipulating institutional logic.
Introduction
Many online communities enjoy little voice regarding the governance of their fora, which are typically controlled by appointed administrators, along with rules and bylaws set by the platform owner—usually a corporation aiming at increasing traffic, or a “benevolent dictator” (Schneider, 2019). However, there is now a trend toward replacing such centralized approaches with more democratic governance methods. Toolkits such as Modular Politics (Schneider et al., 2020), PolicyKit (Zhang et al., 2020a), and others (Bojanowski et al., 2017; Matias and Mou, 2018; Jhaver et al., 2019) now provide online communities with self-governance tools that can be tailored to fit the needs and values of specific communities. These include online voting, juries, petition, elected boards, and even more complex institutional logics (e.g., see https://communityrule.info/templates/).
The relative ease through which online governance tools can be implemented and experimented with, across a variety of platforms, has important implications. Here, we focus on how institutional logic might evolve in online communities and how such an evolution can be guided by experimentation. We present an experimental approach for improving social feedback and for empirically optimizing institutional logic in controlled virtual worlds. We share methods for conducting such virtual worlds experiments, which could bridge between basic research and higher stakes community lead experiments in platform governance (Matias and Mou, 2018).
From an ecological perspective, online communities are ideal settings for the rapid evolution of governance: they are often young and growing, information spreads rapidly, and there is sometimes strong competition among platforms. Moreover, governance tools may rapidly drift from the roles intended by their authors, as unintended consequences of a small change cascade through a population. Such a dynamic was seen, for example, in the “Reddit Blackout,” in which community leaders creatively leveraged a simple mechanism for making channels private to affect a protest in which they disabled large portions of the site (one of the top 10 on the Internet), an action which led shortly thereafter to the resignation of the platform's CEO. Such shifts in governance may affect the survival (fitness) of communities, and lead to novel forms of governance via “natural” selection. But, of course, “mutations” in governance are rarely random as in the case of biological systems. Rather, they are directed, adaptive modifications of institutional logic aimed at coping with emerging needs and challenges. Indeed, in this way, they more resemble Lamarckian cultural evolution (Boyd and Richerson, 1988), proposing that an organism (here an organization) can control its evolution based on its experience. Lamarckian and Darwinian evolution often complement each other and there is a large body of work about cultural evolution in humans and other animals (Boyd and Richerson, 2005; Akcay et al., 2013).
Several experimental approaches could potentially guide and facilitate the evolution of online governance. To give an explicit example, we will focus on two fictive cases that may not capture the diverse needs of online communities, but include governance features that should be relevant to many communities. The first case is of an NGO that provides online services to a community (Figure 1A). Services are managed by a board that sets policies and resources for each service. Governance tools include a feedback system and a specialized committee to facilitate satisfaction with services. Note that here community feedback could be as simple as client rating scores for events of service provision. The second case represents a more open-ended challenge: a community that set a petition system to continuously guide governance (Figure 1B). To promote equality of influence, they set an annual petitions quota on each member. They set two committees and a board to process the stream of petitions: petitions are first sorted into clusters by one committee. A second committee then evaluates costs and feasibilities for each cluster. Finally, the board sets policies, which are guided by the petition system. Note that here community feedback is in the form of verbalizing views, grievances and needs, speaking and being heard, i.e., democratic discussion.
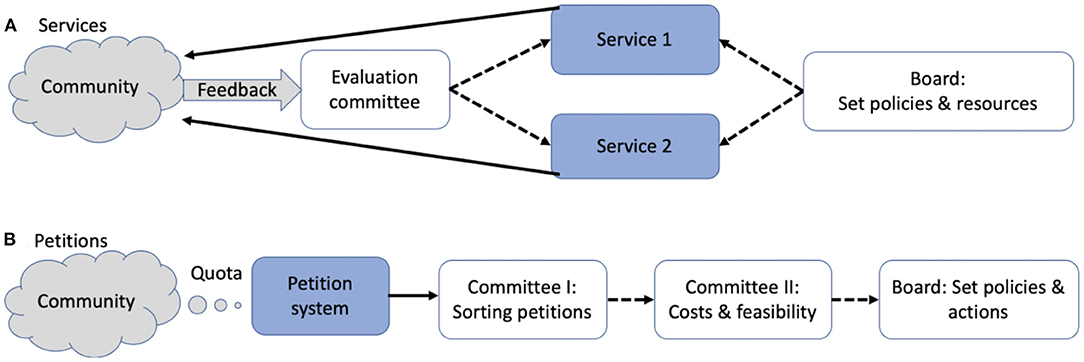
Figure 1. Governance structure for (A) NGO providing two types of online services to a community, (B) community governance via a petition system guiding policies and actions. Solid arrows represent primary processes and dotted arrows secondary (slower) processes.
In both cases (Figures 1A,B), the governance structure is relatively simple and yet, outcomes may depend on complex interactions between several variables. For example, in the petition system, the community needs to set a petitions quota, frequencies for committee meetings, thresholds for propagating and filtering petitions in each stage, etc. We outline three approaches for guiding the development of tools for helping such communities in improving their governance: the first is to improve the design of online governance by utilizing knowledge from basic collective action research (section Optimizing collective action). The second approach is to improve the utility of social feedback via experimental manipulations of simulated feedback systems in virtual worlds. Such experiments can be used for improving community rating systems and monitoring (dashboard) systems (section Virtual worlds experiments with social feedback). The third approach aims at capturing some of the complexity of governance dynamics, and empirically optimize institutional logic, by constructing large-scale experiments entirely in controlled virtual worlds (section Methods for exploring governance space with FSG).
We begin with a brief presentation of existing infrastructure that is available for conducting experiments with online governance (see section Materials and Equipment). Then, in the first Methods section, we review, from a translational perspective, recent research on improving collective action. We focus on technologies for efficiently running massive online experiments for studying collective action, which have revolutionized the social sciences (Salganik and Watts, 2009). In particular, new experimental systems (Hartshorne et al., 2019; Almaatouq et al., 2020; Dallinger dallinger.readthedocs.io) now allow the running of online experiments with thousands of participants, while providing for control of not only the content, but also of the network topology of social interactions (Centola, 2019). Via these methods, much progress has been made in understanding how behavior spreads in social networks (Mason et al., 2008), how cooperation evolves over time (Mao et al., 2017), how the wisdom of the crowd can be improved (Hertwig, 2012; Mannes et al., 2014; Prelec et al., 2017), and how cultural innovation propagates (Balietti et al., 2016). However, there is a large gap between our basic knowledge and our ability to translate it into the specifics of institutional logic. We conclude this section by suggesting practical approaches for implementing some of this knowledge for guiding social influences in ratings and petition systems, which could potentially benefit online communities.
In the second Methods section, we present a framework for experimenting with social feedback in modern online governance. We first discuss the challenge of obtaining high-quality information in online feedback systems. We next introduce the approach of designing virtual worlds experiments that simulate feedback systems in a setting that approximates ecologically valid environments. We then present methods for experimenting with improving information quality and engagement. This includes evaluating efficiencies of different strategies in providing greater opportunity for social learning. Finally, we discuss challenges in attempting continuous tuning of social feedback. In particular, we suggest that virtual worlds experiments can be used for testing risks and benefits of coupling between rating systems and marginal investments in service provision. This Methods section may be particularly relevant to the study of online communities that rely on continuous feedback from members.
In the third Methods section we imagine a conceptual meta-framework for conducting large scale virtual worlds experiments aiming at capturing simple governance structure, and then attempt to improve cooperation and crowd wisdom. We propose an approach for designing such virtual world experiments, which simulate governance structures as in the cases presented in Figure 1. We then suggest that an approach some of us recently developed for exploring high dimensional perceptual space using machine learning (Harrison et al., 2020) could be potentially implemented for efficiently exploring the parameter space of governance in virtual world experiments. If successful, such experiments could be useful for tuning and guiding adaptive modifications to the institutional logic of online governance.
Materials and Equipment
The first part of the section Methods is purely conceptual. The second and third parts are based on a framework for conducting virtual world experiments, which we call Ferry Services Game (FSG). We developed this virtual world specifically for experimenting with governance. It is programmed in Unity (https://unity.com/). We made the code freely available for non-profit at https://github.com/oferon/FerryGame. An online demo of a basic FSG game designing options is available at http://u311.org/FerryServiceGame/. Note, however, that using our methods would also require infrastructure for connecting front-end virtual world engines and back-end engines to control the network of social interactions. It is beyond the scope of this article to describe the existing infrastructure in a manner that can guide experimentalists in choosing the proper tools for experimenting with online governance beyond the core methods presented here. Instead, we provide brief guidelines for dealing with three challenges that are unique to governance experiments: proper recruitment, proper compensation, and designing an appropriate experimental infrastructure.
Recruiting participants for massive online experiments can be done based on capturing their interest without paying them, for example, through traditional media (e.g., Müllensiefen et al., 2014), social media, or websites directed to attract public interest (Hartshorne et al., 2019; Zooniverse.org). Theoretically, it is possible to recruit hundred thousands participants in this way (Awad et al., 2018; Hartshorne et al., 2019), but such experiments are difficult to replicate and need to be adapted and gamified to attract and sustain public interest. An alternative approach is to pay participants through an online recruiting service that mediates between workers who would like to participate in experiments and experimenters who want to recruit participants. Currently, the main services that provide these capabilities are Amazon Mechanical Turk (or “MTurk”; Horton et al., 2011; Mason and Suri, 2012) and Prolific (Palan and Schitter, 2018). The inclusion of monetary compensation allows for performing long series of experiments as the experimenter can motivate repeated participation by providing monetary compensation proportional to the participation. Proper compensation design is important because compensation provides motivation that can potentially bias the simulation. We recommend structuring compensation as an object in the virtual world, to provide incentive that is an integral part of the experimental design. For example, while recruiting workers in MTurk, we set only a small fraction of the compensation in the HIT advertisement. The vast majority of the compensation is a bonus that is designed to provide an incentive that is part of the design of the virtual world and its governance (See an example in Figure 3).
In general, the demographic diversity of workers in recruitment services is fairly high with respect to age, gender, and income (Ross et al., 2010). Recently, tools were introduced in order to account for demographic biases in online experiments. For example, cloudresearch.com has been tracking and publishing fluctuations and biases MTurk workers demographics over time. In addition, researchers can use a wide range of questionnaires and pre-screening tasks to reduce biases in recruited populations (Harrison et al., 2020). However, social experiments with governance might be particularly sensitive to biases in participants' motivations to engage, which is likely to differ across recruitment approaches. For example, one may suspect MTurk participants of gaming the experimental system to save time, but we don't know if such factors are sensitive to recruitment methods. The design of the experiments can be made such that saving time would not be beneficial to the participants (for example by giving extra bonus for good performance). In addition, it is therefore advisable, whenever possible, to attempt replication over multiple recruitment methods. For example, one may compliment MTurk experiments with small scale validation experiments in the lab.
The third serious challenge is setting an appropriate experimental infrastructure for experimenting with governance: creating a complex online experiment requires infrastructure that can simplify the design process. The infrastructure allows the experimenter to focus on the unique aspects of each experiment (for example the interaction of participants with experimental logic) and provide a built-in solution for the other aspects of the experiment including (1) Managing the interaction with recruiting services (such as MTurk) to control the recruiting process, (2) compensating participants automatically, potentially allowing for differentiated compensation based on performance, (3) providing database service to record single participant data and synchronize information shared between participants, (4) orchestrating web servers to run the experiment, (5) managing real-time interaction between participants, (6) providing tools and dashboards to monitor the progress and health of the experiment, and (7) providing tools to simulate real participants with bots.
Several platforms focus on the single-participant user experience (jspsych: de Leeuw, 2015; Qualtrics; labaadvance: Finger et al., 2017). While it is possible to design experiments with multiple participants with these platforms, the platforms themselves provide only a few basic resources and abstractions for combining multiple participants. The state of the art in creating complex experiments are “virtual labs” (Psiturk: Gureckis et al., 2016; Dallinger; Empirica: Almaatouq et al., 2020; WEXTOR: Reips and Neuhaus, 2002; LIONESS; oTree: Chen et al., 2016; Breadboard: McKnight and Christakis, 2016; NodeGame: Balietti, 2017; TurkServer: Mao et al., 2017). These are “experiment engines” that provide infrastructure to design complex experiments (as mentioned above) with multiple participants. Because they provide useful abstractions, experimenters can use these platforms to implement complex designs. Finally, there are several platforms for game development (e.g., Unity, Blender, and Unreal), which allow in-browser (WebGL) deployment of multiplayer games.
The implementation presented here is of in-browser WebGL via Unity. A specific implementation of this system for testing a virtual rating system was recently published (Tchernichovski et al., 2019). Beyond this, we acknowledge that, despite the wealth of existing tools, there is not yet a consensus regarding the best ways to connect such virtual environments to the experimental infrastructures we reviewed above. It is also possible that different tools will be applicable for different projects, as different platforms deviate in their complexity and in the technical skills they demand of experimenters. Some platforms require little prior programming experience [e.g., LIONESS (Giamattei et al., 2020), Breadboard (McKnight and Christakis, 2016), WEXTOR (Reips and Neuhaus, 2002)]. Other platforms require significantly more experience: oTree (Chen et al., 2016), nodeGame (Balietti, 2017), Dallinger (https://github.com/Dallinger/Dallinger/), and TurkServer (Mao et al., 2017), empirica (Almaatouq et al., 2020).
Methods and Anticipated Results
Optimizing Collective Action
Modern governance is, to a large extent, a social system for facilitating and coordinating collective action (Bodin, 2017). In democratic societies, collective action stems from collective decisions and requires some voluntary cooperation. Good collective decisions should reinforce cooperation over time, and, vice versa, a high level of cooperation can potentially improve collective decisions. The challenge is, therefore, to both improve the quality of collective decisions and maximize cooperation. We begin with a synthesis across these two topics, which are typically studied separately.
Optimization of Crowd Wisdom
Due to a phenomenon called crowd wisdom, the quality of a collective's decisions can be higher than those of the individuals composing it (Galton, 1907). Galton observed that aggregating evaluations across individuals gave a more accurate estimate than that of the median evaluation across participants. Under what conditions is the crowd wiser than the individuals who compose it? There is a large body of literature about improving crowd wisdom (Hertwig, 2012; Becker et al., 2017; Prelec et al., 2017). In general, the understood model of crowd wisdom suggests that it depends upon a large, unbiased group of independent judges. In this manner, averaging over the responses reduces the noise of an individual's response, therefore making the crowd decision more accurate. However, recent experiments reveal that this is not always the case (Lorenz et al., 2011): Exposing subjects to evaluations given by their peers can often improve collective estimates, by allowing people who are less confident in their estimates to change their mind (Jayles et al., 2017).
In more practical situations that require deliberation and collective decisions, social communication can either improve or undermine crowd wisdom depending on the structure of the social network (Centola, 2010; Becker et al., 2017). Consider, for example, two online communities. In one of them the social communication network is highly centralized, with some popular people serving as “communication hubs” in a small world network (Watts, 1999). In the other community, communications are more distributed and decentralized. Counterintuitively, Becker et al. (2017) found that social influences improved the wisdom of the crowd in the decentralized social network. In other words, pooling biased estimates may be superior to unbiased estimates if the biases themselves are broadly distributed. In contrast, they found that in social networks where there was a high degree of centrality, social influence has the opposite effect: it decreases crowd wisdom.
Note that the structure of a social network may have two distinct influences on crowd wisdom (Figure 2B): First, the structure of the social network may influence signaling quality (the accuracy of individual evaluations) via direct social influences (Jayles et al., 2017). Second, the structure of the social network may also determine how information is “filtered” while propagating through it, potentially affecting crowd wisdom via an implicit (and often obscured) process of data aggregation (Becker et al., 2017). For example, depending on network topology, minority opinions might be filtered out or amplified from a debate in different rates (Li et al., 2013).
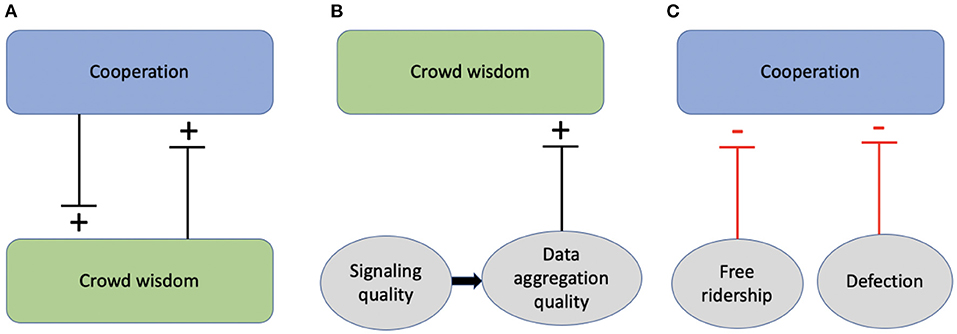
Figure 2. Interactions between cooperation and crowd wisdom in collective action. (A) High cooperation and crowd wisdom can potentially facilitate each other. (B) Crowd wisdom (Galton, 1907) depends on signal quality and also on how data are aggregated and weighted. (C) Cooperation is adversely affected by free-ridership (Marwell and Ames, 1979) and defection (Mao et al., 2017).
In sum, crowd wisdom studies indicate that accuracy of collative estimates should improve with sample size and (depending on network topology) with a balanced social influence. Although crowd wisdom and cooperation are typically studied separately, in governance systems that are based on voluntary feedback, the two may interact: both sample size and topologies of social influences are outcomes of cooperation (i.e., of the motivation to participate). If improving crowd wisdom can affect the quality of collective decisions, then crowd wisdom might also, in turn, affect cooperation (Figure 1A).
Tradeoff Between Communication Efficiency and Crowd Wisdom
Many social media platforms aim at maximizing communication efficiency, e.g., by nudging users to add connections and “friends.” However, it appears that efficiency and innovations of collective action depend on network structure in a complex manner (Mason et al., 2008). These researchers showed that expansive (wide-ranging) networks increase exploration, which is important in finding optimal solutions for complex problems, whereas highly connected (small world) networks may allow faster convergence but not necessarily on the optimal solution. Other studies further suggested that a focus on efficiency may engender a hidden cost with respect to crowd wisdom: One might imagine that informationally efficient collaboration networks should increase the ability of a group (say a task force) to find an innovative solution to a complex problem. However, networks that are efficient for gathering information are not necessarily efficient for performance (Kearns et al., 2006). For example, in an experiment, Brackbill and Centola (2020) gave groups of data scientists a task to find better solutions for complex statistical modeling problems. Participants were randomly assigned to either an efficient or an inefficient communication network. In both groups, subjects were exposed to the same “load” of information, the only difference was in the network efficiency of propagating information. Interestingly, groups in the efficient networks underperformed, while those assigned to inefficient communication networks reached highly efficient solutions. This result led Brackbill and Centola (2020) to suggest that there exists “a tradeoff between the network structures that promote a solution's rapid diffusion throughout a group and the network structures that promote the discovery of innovative solutions.” These results call for translational experiments with governance logic—e.g., regarding the efficiency of communication networks between committees and boards.
Optimization of Cooperation
Early studies outlined central government as a necessary tool for sustaining public goods in order to prevent a “tragedy of the commons” (Hardin, 1968). Three problematic behaviors, in particular, have been studied (Figure 2C): one is free ridership, where people take advantage of public goods but do not contribute enough resources to sustain them (Marwell and Ames, 1979). The second is the “prisoner's dilemma” defection strategy, where a greedy (and myopic) tendency of actors to maximize their immediate gains may erode cooperation over time. And the third is represented by “second-order social dilemmas,” in which monitoring, punishment, and other governance activities for preventing free-riders and defection themselves become vulnerable to free-riding or defection (Okada, 2008), because they are also costly and generate positive externalities. Later group and network studies, pioneered by Elinor Ostrom (see e.g., Janssen et al., 2010) and others (Ahn et al., 2009) suggested that subjects can and do creatively evade free-riding and defection in real-world situations that resemble second-order social dilemmas. Ostrom found that people can overcome these three types of behavior to organize locally and make sustainable arrangements for self-governing common pool resources, without any need for external government interventions. People are often willing to engage in altruistic (and costly) punishment of “free riders” (Ostrom, 1990). Consequently, over time, sanctioning institutions tend to be more competitive than institutions that do not punish free riders and defectors (Gürerk et al., 2006). In the same vein, several studies showed that, in a repeated game, reputation can play a major role in sustaining reciprocity (Axelrod and Hamilton, 1981).
Recently, however, massive online experiments showed that cooperation can persist via network level mechanisms even in the absence of punishment or reputational effects (Suri and Watts, 2011; Mao et al., 2017). Mao et al. (2017) performed an online experiment to study the long-term dynamics of cooperation. Participants played a prisoner's dilemma game repeatedly—that is, hundreds of times over several weeks. Although the Nash equilibrium corresponds to zero cooperation in the absence of mechanisms for punishing defectors, about 40% of participants were irrationally resilient cooperators: they would not be the first to defect, despite knowing that they were about to lose money. Interestingly, long-term social learning in the remaining (more rational) players slowly promoted an equilibrium of cooperation, with dynamics unfolding over long time-scales that could have never been revealed in a typical lab study. Further, willingness to directly reciprocate cooperative behavior has been shown to persist even in highly competitive settings, with NBA players continuing to reciprocate assists despite strong individual incentives not to Willer et al. (2012). Using a similar approach, Melamed et al. (2018) showed further that even in the complete absence of reputational memory, simply allowing network dynamics to evolve gives rise to clustering of participants that shield cooperators from defectors. Further, resilience of cooperation was also observed in a more complex online experiment: Melamed et al. (2020) showed that different modes of reciprocity are fairly independent from each other, such that the collapse of one form (such as direct reciprocity) does not necessarily affect the others (indirect and generalized reciprocity).
Translating From Basic Research to Governance “Wisdom”
Although discoveries such as those presented above seem relevant for designing efficient governance systems, it may be challenging to translate them into the specifics of institutional logic. Based on the studies discussed above we propose two practical approaches for improving crowd wisdom and cooperation.
First is the introduction of social influences into crowdsourced feedback and petition systems: The statistical gold standard for designing crowdsourced information systems has been to obtain independent and unbiased evaluations, in order to minimize sampling endogeneity (Heckman, 1979). But as noted above, in governance systems that rely on voluntary cooperation (Figure 1), outcomes may depend on dynamic interactions between cooperation and crowd wisdom. In other words, minimizing social influences may bear the cost of compromising crowd wisdom. More importantly, minimizing social influences may interfere with efforts to perpetuate cooperation (Figure 2A). Sacrificing the independence of evaluations in order to promote a virtuous feedback loop between crowd wisdom and cooperation can therefore make sense. For example, in a field study some of us reported a positive outcome of increasing social influences in a rating system (Tchernichovski et al., 2017). We found that exposing service clients to trends in rating scores—just prior to rating—was associated with a persistent improvement in satisfaction with services over time. It was also associated with the community sustaining a high feedback rate over years. In addition, introducing social influences may promote self-organization of social information. For example, in a petition system, presenting users with similar petitions while filling out the petition form, may prompt users to amend and endorse existing petitions instead of creating redundant new petitions. Finally, as suggested by the Becker et al. (2017) study, setting a decentralized process for deliberating petitions could be advantageous.
Note, however, that the injecting of social influences into early stages of crowdsourced information systems may open gateways for groups of activists with bad intentions to manipulate and distort information. There are well-established mechanisms for online communities to deal with individual bad actors, but much more challenging are disruptions by collective actions of online “mobs” (Trice and Potts, 2018).
Second is the methods of data aggregation and presentation: Typical governance challenges are very different than those presented in most crowd wisdom experiments. In such experiments people are typically asked to make a quantitative estimate where there is a known ground truth, which is rarely the case in governance. However, crowd wisdom might be more relevant to governance at the low level of aggregating feedback from the community. Online community members often share ratings and “likes” to posts and services, generating a constant stream of quantitative data. It is rarely possible to optimize crowd wisdom in aggregates of such subjective evaluations with respect to a ground truth (which is often intractable). However, it is often possible and practical to improve crowd wisdom in order to promote the early detection of trends (Tchernichovski et al., 2017). That is, crowd wisdom can be defined as the speed and accuracy of detecting a change (Tchernichovski et al., 2019). The early detection of trends can be particularly useful in online communities, where social feedback is continuous. Say, for example, that an online community changed a policy regarding publishing posts in their archive. Detecting negative user feedback early could allow a speedy correction before too many users have “voted” by exit (Schneider, 2019). In the next section we will introduce experimental methods of optimizing crowd wisdom in feedback systems, including challenges in sustaining high quality of signaling (Figure 2B), which often suffers from sampling endogeneity and from poor information quality (Stocker, 2006; Moe and Schweidel, 2011; Ho et al., 2014).
In sum, improving crowd wisdom and cooperation should be considered while designing online governance. We highlighted two aspects where this might be particularly relevant: one is in the design of social influences during submission of petitions or evaluations, and the second is in the design of data aggregation in evaluations and ratings. We will return to these issues below while presenting frameworks for virtual worlds experiments with governance.
Virtual Worlds Experiments With Social Feedback
We first briefly review the utilities and limitations of correlational and experimental studies in online community governances. We then introduce virtual world experiments and present methods for experimenting with social feedback.
Correlational Studies in Online Communities
Observational studies on the governance systems of online communities revealed important dynamic relations between evolving governance structure and outcomes. For instance, Tan and Zargham (2021) recently published the GovBase database: a crowdsourced summary of tools used for online governance. The database allows for exploration of how different governance structures that have been adopted by online communities may correlate with (and perhaps predict) the survival of those communities over time. Several studies explored the evolution of governance in online communities. For example, Frey and Sumner (2019) studied governance rules in 5,000 online communities of video game players and described how they evolve over time. They found that the structure (number and scope) of governance rules given population size can, to a certain extent, predict growth of the group of core members. Because users can be enculturated to prefer a new form of governance, and conversely, can exert selective pressure on expressed governance forms by opting out of communities they don't like, it is possible for social feedback loops to drive the evolution of both governance forms and preferences. To this end, a follow-up study by Zhong and Frey (2020) finds evidence that, although influence is evident in both potential directions, the selective pressure mechanism is much stronger than the cultural evolution of preferences. Observational studies have illuminated several questions about the formation of self-directed governance systems. For example, how strong a force is emergent centralization? Shaw and Hill (2014) documented the unexpected emergence of oligarchy in the radically egalitarian domain of “wiki” knowledge bases. They observe that a small administrator class becomes increasingly distinguishable as wikis grow, and that the goals and standards of that class diverge from those of regular volunteers. How do different modalities of positive and negative interactions between agents aggregate to produce social outcomes? Szell et al. (2010) analyze the multidimensional relationships between hundreds of thousands of players in an online game to illuminate the game's emergent system of power dynamics. With a multiplex network approach, they demonstrate alliance and conflict dynamics playing out in a coordinated manner over several types of game action, including trade, conflict, and friendship relations. There are, however, strong limitations to such observational studies: causality is difficult to assert, and the space of scenarios that can be explored is constrained by the current state of the world. With an experimental approach to the governance of online communities, one can test cause and effect directly and explore governance outcomes in social scenarios that cannot be easily observed otherwise.
Experimenting in Social Media Governance
The challenge we would like to focus on hereafter is how to better connect the basic research of optimizing collective actions with observations of online governance (section Correlational studies in online communities) in order to test practical solutions. This would require translational experiments. Experimenting directly with social media governance may raise ethical concerns, but when done properly it can be highly valuable (Lazer et al., 2020). Concerns about early experiments and ethically questionable data-mining have strongly constrained subsequent experiments (Kramer et al., 2014; Lazer et al., 2020). That said, among the most promising recent approaches is the Matias CivilServant System (Matias and Mou, 2018) for community-led experiments in platform governance. An example implementation of CivilServant is a recent experiment that was done in a Reddit community with 13 million subscribers. An announcement about a new community rule was randomly assigned to some of the new members in an online science discussion forum (Matias, 2019). Presenting the announcement informed new participants about the community's rules: no jokes, no abusive content, and so on. The question was if this message would influence newcomers' choices to contribute content, and if it would affect harassment levels. Results showed that the intervention reduced cases of harassment without decreasing participation in the forum. We hope that such an experimental approach would become increasingly useful in optimizing cooperation, hence promoting a virtuous loop between cooperation and high-quality collective decisions, as discussed earlier (Figure 2). One way of achieving this is by complementing community-led experiments with virtual world experiments, where risks and attendant ethical concerns can be minimized. In addition, virtual world experiments provide the possibility to perform much more substantial control experiments and test more radical modification to the services including the possibility of “collapse” of the community, something that should be avoided in a community-led experiments.
A Framework for Virtual Worlds Experiments With Governance
As discussed above, online experiments in crowd wisdom and cooperation have contributed to a deeper understanding of collective behavior in humans. The key to this progress is in technological innovations that now allow for the testing of thousands of participants over hundreds of iterations while controlling the topologies of social communication networks. But given the highly stylized nature of experimental exchange systems, even such large-scale experiments have limited bearing on complex, real-life governance. We now turn to the challenge of conducting online experiments in virtual worlds, with the aim of studying governance in simulated, complex environments. There are two motivations to conduct such experiments: First, the governance systems of the virtual world can permit adventurous experiments in the varieties of social life with low enough stakes that they do not risk any meaningful form of social collapse. Second, placing people in situations that allow them to tweak or elaborate their setting makes it possible to study the evolution of complex institutions from simple ones. This means that we need not only to conduct long-term experiments, but also to place people in situations that mirror the real-world complexities of pooling and sharing multiple resources. Former studies utilize virtual worlds for conducting simple experimental interventions. For example, introducing bots into popular areas in the multiplayer game Second Life allowed researchers to examine how the “age” and “gender” of bots' avatars affected the avatar's chances of obtaining help from other players (Zhang et al., 2020b). They can also provide and validate governance tools for virtual worlds, such as research providing governance tools on the integrated game chat platform Discord (Zhang et al., 2020a), or community monitoring tools on the video game Minecraft (Müller et al., 2015). However, to our knowledge, this article is the first to present a framework for virtual world experiments that are designed specifically for studying governance.
We developed a method for experimenting with governance in a virtual world, which we call Ferry Services Game (FSG). FSG is an in-browser WebGL game (see Material and equipment). Participants (e.g., MTurk workers) manipulate their avatar in a 3D world to collect coins, which are redeemed for money at the end of the game (as a compensation bonus). The 3D world is composed of a long chain of islands (Figure 3A). Participants must use simulated ferry services to visit each island, but some ferries are fast and others are slow, and players are motivated to have faster ferries since time spent on ferries competes with time spent earning revenue (Figures 3B,D). Through several mechanisms, players may gain and contribute information about ferry characteristics. After a ferry ride the player may be prompted to rate the service. Ratings may be pooled and shared with other players via a dashboard. The experimenter sets the distributions of service speeds and delays, and then evaluates the ferry rating scores against the ground truth of that distribution. Therefore, as in many real-world situations, rating information can help players select (or collectively own) better services.
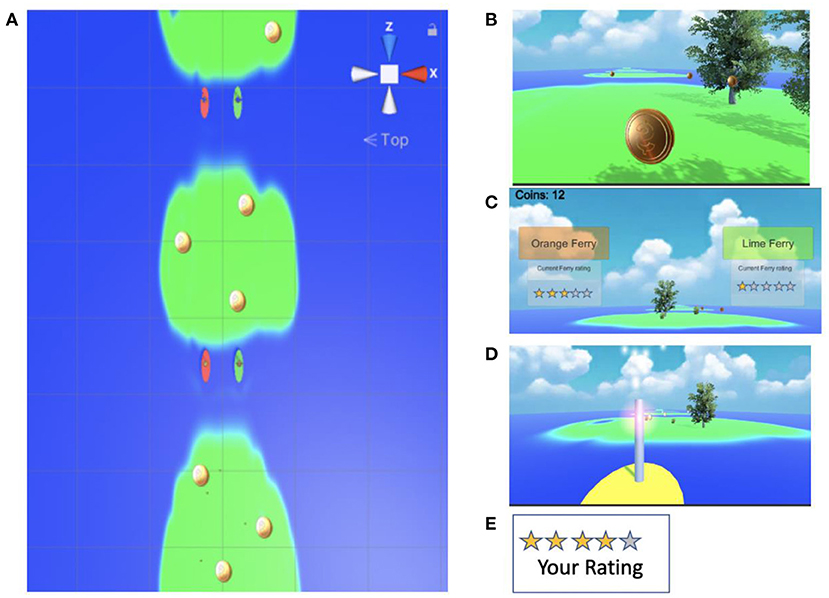
Figure 3. Ferry Services Game. (A) A 3D virtual environment composed of islands with coins scattered in different locations. (B) Collecting coins in each island. (C) Ferry services are used to cross between islands. Dashboard represents ferry rating scores. (D) Ferry services differ in speed and delays. (E) At the end of each ride player might be prompted to rate ferry performance.
Several game parameters are adjustable: Rating the ferry may be either voluntary or obligatory. Players may be given no incentive to rate the ferry (which is often the case in real world rating systems). Alternatively, rating the ferry can be made a social process. For example, in Figure 3C we present a design where players can choose between two ferry services in each island. Here, sharing rating scores with other players would benefit all players by allowing them to quickly pool their knowledge about which ferry service is better. However, this public goods game incentivizes free ridership (Marwell and Ames, 1979). In a different design, only members of a “club” may share rating information, as in a common pool resource game. Here, club governance rules may be either randomly assigned, or set by the players. In this manner, layers of governance can be superimposed on the game, as we will show in the next section. In this section, we focus on low-level experimentation with optimizing social feedback.
Optimization of Feedback Systems With FSG
Many online communities use feedback systems that provide quantitative evaluations such as “likes,” and rating scores. Feedback systems have evolved rapidly over the last two decades. They turned from an industry standard mechanism designed for obtaining information from—and retaining—clients, into a rich ecosystem of independent crowdsourced rating platforms, which now guide many everyday decisions.
FSG experiments can be designed for optimizing feedback systems at three levels. First is the optimization of the rating device: Most systems use rating devices that implement Likert scales, e.g., a 1–5 “star” ratings, where the rating device is a trivial “click and submit” radio group. Pooled star ratings can then be made visible to both creators and consumers of content, or in e-commerce, to service providers and potential clients (Figure 4A). There are many concerns about the quality (and honesty) of such rating scores (Luca, 2016). A recent FSG experiment found that even without any conflicts of interest or incentives to cheat, pooled rating scores could explain only about 14% of the variance in the speed of ferries (Tchernichovski et al., 2019). However, pooled rating accuracy was about twice as high when ratings were submitted via a device that imposed time costs of a few seconds on reporting extreme scores. Such an improvement in feedback information quality could be useful in the case of the NGO providing online services presented earlier (Figure 1A): With better rating accuracy the evaluation committee should be able to detect a change in satisfaction with its service outcome much faster. This could have two practical advantages: First, services can respond to the change faster. Second, the sooner a change can be detected the easier it is to identify its cause. In other words, reducing latency in detecting a change is likely to improve reinforcement learning in a multi-agent scenario (Sutton and Barto, 2018).
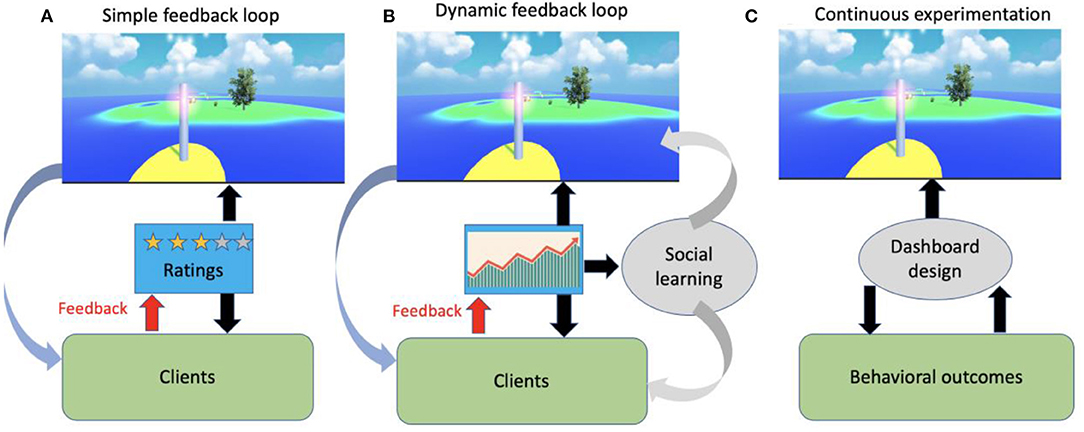
Figure 4. From rating systems to governance evolution. (A) A FSG experiment with a typical online rating system, where both service provider (e.g., restaurant) and client's behaviors are guided by feedback ratings. (B) Replacing rating scores with a dashboard presenting trends to promote social learning in FSG experiment. (C) A framework for ongoing experimentation with dashboard design.
These results demonstrate the utility of using FSG for testing different designs of rating devices. The FSG game template we shared (https://github.com/oferon/FerryGame) can be used for testing such designs. FSG is coded in the gaming platform Unity, where building arbitrarily complex 3D rating devices (game objects) with physics can be easily implemented. The game can be then compiled as is into either a desktop application for lab experiments, or into a WebGL for online experiments via a browser, or into a phone app, for long-term experiments.
A second manner in which FSG experiments can be used for improving feedback systems is via experimentation with distributed feedback monitoring methods. Performance measurement systems with dashboards are widely used in many industries (Bititci et al., 2000) in order to monitor and coordinate performance benchmarks and optimize institutional learning. In principle, similar dashboard systems can be developed to optimize social learning in a non-commercial setting of online communities. Such dashboards may be particularly useful for online communities that offer services that are used repeatedly by a pool of members. In such cases, it might make sense to present dashboards with trends (Tchernichovski et al., 2017) rather than mean rating scores. FSG experiments can be designed for testing the utility of presenting such trends (Figure 4B). FSG experiments can test, for example, if the presentation of trends in rating scores of ferry services improves social learning, prompting players to adjust their strategies more efficiently. Note that even in a small community that runs a simple operation, social learning may show complex temporal dynamics: the detection of a trend gives both clients and service providers an opportunity for social learning in real time: service providers can “experimentally” adjust their behavior/product in real time in response to trends, while consumers can make choices with more up-to-date information. The challenge is how to tune the parameters of the trend presentation such that each side can learn most effectively from the other? Returning to the case of an NGO providing online services (Figure 1A): should trends in satisfaction with each service type be presented over days, weeks, or months? Presenting short term trends should minimize delay and improve social learning. However, presenting long term trends may promote stability and improve the clarity of social signals. For the NGO, manipulating such parameters can be risky, but FSG experiments can be designed for testing such dashboard calibrations while keeping the temporal dynamics of service provision fixed. For example, one may assign participants into ferry rider and ferry driver groups, and allow them to learn from each other only via a (service provider) dashboard presenting trends. Simulating the dynamics of social learning via feedback in different conditions could then guide the NGO on how to design and tune performance measurement systems in their non-market setting (a monosponistic environment).
Third, FSG experiments can be designed for testing the utility and risks of implementing machine learning for continuous optimization of rating system features. Such features may include, for example, the physics of a rating device (Tchernichovski et al., 2019), or the temporal resolution of a dashboard presenting trends (Tchernichovski et al., 2017), or both. About a decade ago, much of the software industry adopted continuous deployment, such that software is continually released and experimented with (Kevic et al., 2017). Such commercial systems for automated experimentation with platform design are often based on a closed-loop feedback system (Figure 4C). For example, the “style” of a banner in a web page includes many features, such as screen coordinates, size, colors, and fonts, which can be manipulated while monitoring changes in client behavior. Here, the typical feedback is not rating scores, but changes in traffic, clicking on ads, and so on. Using standard machine learning approaches (Mattos et al., 2017; Gauci et al., 2018), such systems can continuously “nudge” the design, with the aim of maximizing outcomes desired by the platform owner. Matias and Mou (2018) suggested that a similar testing approach might be useful in the implementation of online governance policies (Matias, 2019).
One may treat a subset of governance logic in a community as a set of features that can be continuously optimized as in the typical case of an ad banner. This could include gain and delay parameters (how often a certain committee should meet), or setting the threshold of consensus required for votes to enable a petition to pass, etc. In this spirit, we imagine FSG experiments combining continuous rating feedback (which can be seen as continuous voting) with continuous exploration of governance logic (Figure 4C). We emphasize that trying such an approach in real communities could be dangerous and possibly unethical, and that virtual worlds experiments should be regarded as sandboxes where thresholds for tipping points can be safely established. An example of such an FSG experiment would be implementing a machine learning algorithm to find the temporal resolution of a dashboard that maximizes both service usage and satisfaction with service outcomes over time. There are several risks in running such experiments in an online community. For example, people may (wrongly) perceive that information is being manipulated by bad actors, or the algorithm may become unstable, reducing public confidence.
Finally, an experimenter could allow feedback from dashboards to directly guide marginal investments in simulated services via continuous experimentation. The role of such FSG experiments could be to serve as a playground for exploring utility, but even more so, for discovering and then reducing risks prior to deployment in real-world online communities.
Methods for Exploring Governance Space With FSG
The core of the FSG methods we have presented so far is the calibration of feedback systems that are attached to specific activities. For example, in a virtual world where participants collect coins (real money) in islands and ride ferries to get to these islands, the ferry services can be either public goods, common pool resources, or private companies. Either way, participants should care about those services, and may share information about their satisfaction via a dashboard. At this point there is already a need for governance: should information be available to everyone (public good) or only to members (common pool resource)? How to advertise it? How to display the information in an optimal manner? For example, a dashboard could show trends of satisfaction with ferry services before riding a ferry (Tchernichovski et al., 2017, 2019). The experiential challenge is to allow such a dashboard to directly nudge policies and governance logic. For this purpose, we suggest a generic framework for attempting long term FSG experiments that simulate a virtual city, where participants are engaged in a variety of activities, providing other services, instituting taxes and tolls, and forming simple governance institutions (Figure 5).
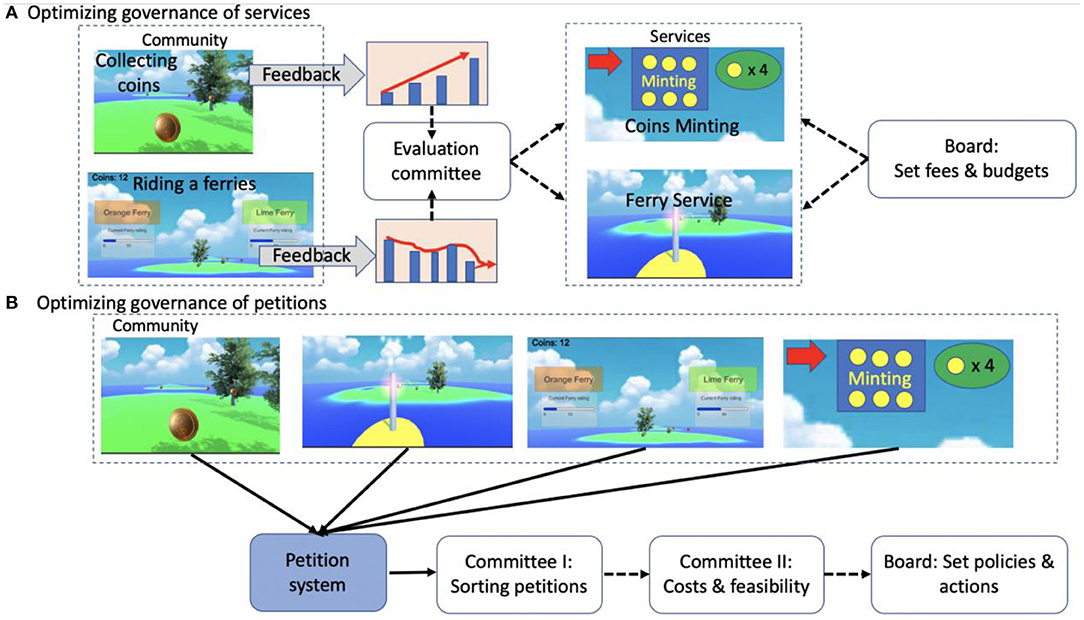
Figure 5. Ferry Services Game experiment with governance. (A) FSG design simulating governance of pooled resources (services) via feedback in a community (no- market, as in Figure 1A). Groups of participants work in providing simulated services (e.g., driving ferries or minting coins) and another group represents community members who use these services (riding ferries and collecting coins). Feedback from participants' satisfaction and service usage is aggregated into dashboard presenting trends. These trends then feed into governance logic. (B) FSG design simulating a petition governance (as in Figure 1B).
Here, the experimenter needs to design an ecosystem with several groups of players. For example, in Figure 5A, one group of players represents community members who use two common pool resources: coins and ferries. They pay tolls for those services, and rate them. The measure of performance in this group is net coin earning, and the level of cooperation is the feedback rate. The second and third groups of players are service providers: ferry drivers and coin minters. Each of these groups plays a public goods game: in each round these players decide how much of their income (from services and tolls) to invest in these services. The more they invest, the faster the ferries move and the more coins can be collected in each island. If properly designed, such a system may stabilize on different levels of cooperation: the community may decide to pay more or fewer tolls based on their satisfaction with the services. The service providers may decide to invest more or less of their toll income in the quality of these services.
Note that the FSG design presented in Figure 5A is a simulation of the community presented in Figure 1A, including two rating systems, two dashboards displaying trends, a committee and a board. Once participants are recruited to play on a regular basis, communication channels can be used for implementing governance structure including committees, boards and voting. The experimenter could impose community rules or, alternatively, allow participants to negotiate them. We may see some communities where cooperation collapses and others where cooperation persists, in much the manner that online communities can be observed to develop rich and varied governance systems for overcoming their online governance challenges (Frey and Sumner, 2019). In sum, there is a potential value in developing such virtual worlds experiments to facilitate the Lamarckian evolution of governance.
Finally, there are two inherent weaknesses of the approaches we presented for the FSG experiments for exploring governance space, which are worth consideration. The first is a potential experimental failure due to the complexity of the design. How can FSG experiments succeed in exploring a complex governance space? This challenge is somewhat similar to that of experimenting in exploring human perceptual space in the field of cognitive neuroscience. One may ask, for example, what set of acoustic features add up to an abstract percept such as the sound of a violin. Until recently, exploring the space of such high-dimensional acoustic features was not experimentally feasible. Recently, however, combining machine learning with human judgement was shown to be successful in efficiently identifying such perceptual categories (Harrison et al., 2020). In such experiments, participants are presented with a slider, which they manipulate to approximate a category (e.g., determine which sound resembles a violin). Although the participant repeatedly manipulates the same slider, in each round the slider represents a different acoustic feature. The algorithm pools these evaluations across participants in order to explore the perception of an arbitrarily complex space of acoustic features in an efficient manner. At least conceptually, a similar approach could be implemented in FSG experiments. Here, instead of presenting participants with a slider for manipulating values of acoustic features, they can be presented with sliders representing their preferences in governance space. For example, a slider can be presented to cast a preference for the ferry toll, or to vote for a governance rule about feedback quota, etc. The point is, even if this governance space includes several parameters, it may still be possible to efficiently explore it by implementing modern machine learning methods as in Harrison et al. (2020). The utility of such an approach is in detecting and characterizing stable states in the space of governance features.
A second limitation is that a significant aspect of online governance consists of a democratic debate, where participants are verbalizing and communicating views, grievances and needs. Experimenting with feedback systems and exploring governance space using FSG, where governance is based primarily on simple quantitative measures, may fail to capture or acknowledge the minority view and its legitimacy or even nuances in the views of the majority. Although we cannot yet offer specific solutions, we hope that future development of FSG game simulating a petition governance (as in Figure 5B) can allow experimenting with the tradeoff between openness and control. The challenge in such experiments is how to channel activism away from the wild (and mostly futile) social dynamics of echo chambers and internet-storms into petition systems where participant influence is balanced, petitions can evolve, and where one can quantify the extent to which feedback and deliberation can become more constructive in a controlled environment.
Discussion
We began by reviewing studies of crowd wisdom and studies of cooperation. We claim that with respect to democratic governance, crowd wisdom and cooperation may interact and influence collective action. We then showed that whereas early studies focused on the role of punishment and reputation in sustaining cooperation, more recent studies revealed the importance of the structure (topology) of communication networks in sustaining cooperation. The communication network influences the success of collective action via the manner through which diffusion of knowledge and influence affect crowd wisdom and cooperation. We argued that, on one hand, such studies of network topology are highly relevant to the problem of optimizing governance, but on the other hand translational studies have remained rare. We then presented a framework for virtual worlds experiments, Ferry Service Game (FSG), and presented experimental approaches aiming at overcoming the challenges of conducting translational research in online governance.
From an experimentalist perspective, online communities are wonderful playgrounds for studying the evolution of governance logic. We reviewed several studies that reveal the complex relations between adoption of governance rules and their statistical outcomes. We then discussed how virtual worlds experiments with FSG can complement community-led experiments, making the argument that some of the most exciting directions to explore are too risky and perhaps unethical to experiment with in online communities.
We concluded by suggesting how user feedback systems that provide continuous streams of rating information could potentially be leveraged for continuous optimization of governance logic. We reviewed the idea of using such feedback systems in a manner that generalizes standard commercial design of continuous experimentation with design—which we propose can be extended to governance operation and logic. We propose that large-scale virtual worlds experiments can address this problem. Finally, we presented a method for running FSG experiments with governance. We hope that sharing out methods will encourage adventurous studies in complex virtual social environments aiming at exploring governance beyond the current boundaries of existing governance models. Such experimentation with new types of social contracts could potentially guide our social evolution in new and exciting directions.
Author Contributions
OT wrote the initial version. SF and DC edited and expanded the main frame of the MS. NJ wrote section Discussion. All authors worked together editing the manuscript.
Funding
This work was supported in part by NSF GCR 2020751 Jumpstarting Successful Open-Source Software Projects With Evidence-Based Rules and Structures.
Conflict of Interest
The authors declare that the research was conducted in the absence of any commercial or financial relationships that could be construed as a potential conflict of interest.
The handling editor declared a shared research group with the author SF at time of review.
Acknowledgments
The authors wish to thank Nathan Schneider and the Metagovernance Seminar.
References
Ahn, T. K., Esarey, J., and Scholz, J. T. (2009). Reputation and cooperation in voluntary exchanges: comparing local and central institutions. J. Polit. 71, 398–413. doi: 10.1017/S0022381609090355
Akcay, E., Roughgarden, J., Fearon, J. D., Ferejohn, J. A., and Weingast, B. R. (2013). Biological Institutions: The Political Science of Animal Cooperation. doi: 10.2139/ssrn.2370952
Almaatouq, A., Becker, J., Houghton, J. P., Paton, N., Watts, D. J., and Whiting, M. E. (2020). Empirica: a virtual lab for high-throughput macro-level experiments. arXiv [Preprint] arXiv:2006.11398. doi: 10.3758/s13428-020-01535-9
Awad, E., Dsouza, S., Kim, R., Schulz, J., Henrich, J., Shariff, A., et al. (2018). The moral machine experiment. Nature 563, 59–64. doi: 10.1038/s41586-018-0637-6
Axelrod, R., and Hamilton, W. D. (1981). The evolution of cooperation. Science 211, 1390–1396. doi: 10.1126/science.7466396
Balietti, S. (2017). nodegame: Real-time, synchronous, online experiments in the browser. Behav. Res. Methods 49:16961715. doi: 10.3758/s13428-016-0824-z
Balietti, S., Goldstone, R. L., and Helbing, D. (2016). Peer review and competition in the Art Exhibition Game. Proc. Natl. Acad. Sci. U. S. A. 113, 8414–8419. doi: 10.1073/pnas.1603723113
Becker, J., Brackbill, D., and Centola, D. (2017). Network dynamics of social influence in the wisdom of crowds. Proc. Natl. Acad. Sci. U.S.A. 114, E5070–E5076. doi: 10.1073/pnas.1615978114
Bititci, U. S., Turner, U., and Begemann, C. (2000). Dynamics of performance measurement systems. Int. J. Oper. Prod. Manag. 20, 692–704. doi: 10.1108/01443570010321676
Bodin, Ö. (2017). Collaborative environmental governance: achieving collective action in social-ecological systems. Science. 357:eaan1114. doi: 10.1126/science.aan1114
Bojanowski, P., Grave, E., Joulin, A., and Mikolov, T. (2017). Enriching word vectors with subword information. Trans. Assoc. Comput. Linguist. 5, 135–146. doi: 10.1162/tacl_a_00051
Boyd, R., and Richerson, P. J. (1988). Culture and the Evolutionary Process. University of Chicago Press.
Boyd, R., and Richerson, P. J. (2005). The Origin and Evolution of Cultures. Oxford: Oxford University Press.
Brackbill, D., and Centola, D. (2020). Impact of network structure on collective learning: An experimental study in a data science competition. PLoS ONE 15:e0237978. doi: 10.1371/journal.pone.0237978
Centola, D. (2010). The spread of behavior in an online social network experiment. Science 329, 1194–1197. doi: 10.1126/science.1185231
Centola, D. (2019). How Behavior Spreads: The Science of Complex Contagions. Princeton, NJ: Princeton University Press.
Chen, D. L., Schonger, M., and Wickens, C. (2016). oTree-An open-source platform for laboratory, online, and field experiments. J. Behav. Exp. Finance 9, 88–97. doi: 10.1016/j.jbef.2015.12.001
de Leeuw, J. R. (2015). jsPsych: a JavaScript library for creating behavioral experiments in a Web browser. Behav. Res. Methods 47, 1–12. doi: 10.3758/s13428-014-0458-y
Finger, H., Goeke, C., Diekamp, D., Standvoß, K., and König, P. (2017). “Labvanced: a unified JavaScript framework for online studies,” in International Conference on Computational Social Science (Cologne).
Frey, S., and Sumner, R. W. (2019). Emergence of integrated institutions in a large population of self-governing communities. PLoS One 14:e0216335. doi: 10.1371/journal.pone.0216335
Gauci, J., Conti, E., Liang, Y., Virochsiri, K., He, Y., Kaden, Z., et al. (2018). Horizon: Facebook's open source applied reinforcement learning platform. arXiv [Preprint]. arXiv:1811.00260.
Giamattei, M., Yahosseini, K. S., Gächter, S., and Molleman, L. (2020). LIONESS Lab: a free web-based platform for conducting interactive experiments online. J. Econ. Sci. Assoc. 6, 95–111. doi: 10.1007/s40881-020-00087-0
Gureckis, T. M., Martin, J., McDonnell, J., Rich, A. S., Markant, D., Coenen, A., et al. (2016). psiTurk: an open-source framework for conducting replicable behavioral experiments online. Behav. Res. Methods 48, 829–842. doi: 10.3758/s13428-015-0642-8
Gürerk, Ö., Irlenbusch, B., and Rockenbach, B. (2006). The competitive advantage of sanctioning institutions. Science 312, 108–111. doi: 10.1126/science.1123633
Hardin, G. (1968). The tragedy of the commons. Science 162, 1243–1248. doi: 10.1126/science.162.3859.1243
Harrison, P., Marjieh, R., Adolfi, F., van Rijn, P., Anglada-Tort, M., Tchernichovski, O., et al. (2020). Gibbs sampling with people. Adv. Neural Inf. Process. Syst. 33. Available online at: https://proceedings.neurips.cc/paper/2020/file/7880d7226e872b776d8b9f23975e2a3d-Paper.pdf
Hartshorne, J. K., de Leeuw, J. R., Goodman, N. D., Jennings, M., and O'Donnell, T. J. (2019). A thousand studies for the price of one: accelerating psychological science with Pushkin. Behav. Res. Methods 51, 1782–1803. doi: 10.3758/s13428-018-1155-z
Heckman, J. J. (1979). Sample selection bias as a specification error. Econ. J. Econ. Soc. 47, 153–161. doi: 10.2307/1912352
Hertwig, R. (2012). Tapping into the wisdom of the crowd-with confidence. Science 336, 303–304. doi: 10.1126/science.1221403
Ho, Y.-C., Tan, Y., and Wu, J. (2014). Effect of Disconfirmation on Online Rating Behavior: A Dynamic Analysis. MIS Work. Available online at: http://www.krannert.purdue.edu/academics/MIS/workshop/Online_Rating_Behavior.pdf (accessed November 24, 2014).
Horton, J. J., Rand, D. G., and Zeckhauser, R. J. (2011). The online laboratory: conducting experiments in a real labor market. Exp. Econ. 14:399425. doi: 10.1007/s10683-011-9273-9
Janssen, M. A., Holahan, R., Lee, A., and Ostrom, E. (2010). Lab experiments for the study of social-ecological systems. Science 328, 613–617. doi: 10.1126/science.1183532
Jayles, B., Kim, H. R., Escobedo, R., Cezera, S., Blanchet, A., Kameda, T., et al. (2017). How social information can improve estimation accuracy in human groups. Proc. Natl. Acad. Sci. 114, 12620–12625. doi: 10.1073/pnas.1703695114
Jhaver, S., Birman, I., Gilbert, E., and Bruckman, A. (2019). Human-machine collaboration for content regulation: the case of reddit automoderator. ACM Trans. Comput. Interact. 26, 1–35. doi: 10.1145/3338243
Kearns, M., Suri, S., and Montfort, N. (2006). An experimental study of the coloring problem on human subject networks. Science 313, 824–827. doi: 10.1126/science.1127207
Kevic, K., Murphy, B., Williams, L., and Beckmann, J. (2017). “Characterizing experimentation in continuous deployment: a case study on Bing,” in Proceedings of the 2017 IEEE/ACM 39th International Conference on Software Engineering: Software Engineering in Practice Track, ICSE-SEIP 2017 (Institute of Electrical and Electronics Engineers Inc.) (Buenos Aires), 123–132.
Kramer, A. D. I., Guillory, J. E., and Hancock, J. T. (2014). Experimental evidence of massive-scale emotional contagion through social networks. Proc. Natl. Acad. Sci. U. S. A. 111, 8788–8790. doi: 10.1073/pnas.1320040111
Lazer, D. M. J., Pentland, A., Watts, D. J., Aral, S., Athey, S., Contractor, N., et al. (2020). Computational social science: obstacles and opportunities. Science 369, 1060–1062. doi: 10.1126/science.aaz8170
Li, Q., Braunstein, L. A., Wang, H., Shao, J., Stanley, H. E., and Havlin, S. (2013). Non-consensus opinion models on complex networks. J. Stat. Phys. 151, 92–112. doi: 10.1007/s10955-012-0625-4
Lorenz, J., Rauhut, H., Schweitzer, F., and Helbing, D. (2011). How social influence can undermine the wisdom of crowd effect. Proc. Natl. Acad. Sci. U. S. A. 108, 9020–9025. doi: 10.1073/pnas.1008636108
Luca, M. (2016). Reviews, Reputation, and Revenue: The Case of Yelp. Harvard Business School NOM Unit Working Paper 12-016.
Mannes, A. E., Soll, J. B., and Larrick, R. P. (2014). The wisdom of select crowds. J. Pers. Soc. Psychol. 107, 276–299. doi: 10.1037/a0036677
Mao, A., Dworkin, L., Suri, S., and Watts, D. J. (2017). Resilient cooperators stabilize long-run cooperation in the finitely repeated Prisoner's Dilemma. Nat. Commun. 8:13800. doi: 10.1038/ncomms13800
Marwell, G., and Ames, R. E. (1979). Experiments on the provision of public goods. I. Resources, interest, group size, and the free-rider problem. Am. J. Sociol. 84, 1335–1360. doi: 10.1086/226937
Mason, W., and Suri, S. (2012). Conducting behavioral research on Amazon's Mechanical Turk. Behav. Res. Methods 44:123. doi: 10.3758/s13428-011-0124-6
Mason, W. A., Jones, A., and Goldstone, R. L. (2008). Propagation of innovations in networked groups. J. Exp. Psychol. Gen. 137, 422–433. doi: 10.1037/a0012798
Matias, J. N., and Mou, M. (2018). “CivilServant: Community-led experiments in platform governance,” in Proceedings of the Conference on Human Factors in Computing Systems (New York, NY: Association for Computing Machinery), 1–13.
Matias, N. J. (2019). Preventing harassment and increasing group participation through social norms in 2,190 online science discussions. Proc. Natl. Acad. Sci. U. S. A. 116, 9785–9789. doi: 10.1073/pnas.1813486116
Mattos, D. I., Bosch, J., and Olsson, H. H. (2017). “Your system gets better every day you use it: towards automated continuous experimentation,” in 2017 43rd Euromicro Conference on Software Engineering and Advanced Applications (SEAA) (Vienna: IEEE), 256–265.
McKnight, M. E., and Christakis, N. A. (2016). Breadboard. Breadboard: Software for Online Social Experiments, Version 2 [Computer Software]. Yale University.
Melamed, D., Harrell, A., and Simpson, B. (2018). Cooperation, clustering, and assortative mixing in dynamic networks. Proc. Natl. Acad. Sci. U. S. A. 115, 951–956. doi: 10.1073/pnas.1715357115
Melamed, D., Simpson, B., and Abernathy, J. (2020). The robustness of reciprocity: Experimental evidence that each form of reciprocity is robust to the presence of other forms of reciprocity. Sci. Adv. 6:eaba0504. doi: 10.1126/sciadv.aba0504
Moe, W. W., and Schweidel, D. A. (2011). Online product opinions: incidence, evaluation, and evolution. Mark. Sci. 31, 372–386. doi: 10.1287/mksc.1110.0662
Müllensiefen, D., Gingras, B., Musil, J., and Stewart, L. (2014). The musicality of non-musicians: an index for assessing musical sophistication in the general population. PLoS One 9:e89642. doi: 10.1371/journal.pone.0089642
Müller, S., Kapadia, M., Frey, S., Klingler, S., Mann, R., Solenthaler, B., et al. (2015). “Statistical analysis of player behavior in Minecraft,” in Proceedings of the 10th International Conference on the Foundations of Digital Games. Society for the Advancement of the Science of Digital Games.
Okada, A. (2008). The second-order dilemma of public goods and capital accumulation. Public Choice 135, 165–182. doi: 10.1007/s11127-007-9252-z
Ostrom, E. (1990). Governing the Commons: The Evolution of Institutions for Collective Action. Cambridge University Press.
Palan, S., and Schitter, C. (2018). Prolific.aca subject pool for online experiments. J. Behav. Exp. Finance 17, 22–27. doi: 10.1016/j.jbef.2017.12.004
Prelec, D., Seung, H. S., and McCoy, J. (2017). A solution to the single-question crowd wisdom problem. Nature 541, 532–535. doi: 10.1038/nature21054
Reips, U.-D., and Neuhaus, C. (2002). WEXTOR: A Web-based tool for generating and visualizing experimental designs and procedures. Behav. Res. Methods Instrum. Comput. 34, 234–240. doi: 10.3758/BF03195449
Ross, J., Irani, I., Silberman, M. S., Zaldivar, A., and Tomlinson, B. (2010). “Who are the crowdworkers?: shifting demographics in Amazon mechanical turk,” in CHI EA 2010, 2863–2872.
Salganik, M. J., and Watts, D. J. (2009). Web-based experiments for the study of collective social dynamics in cultural markets. Topics Cogn. Sci. 1, 439–468. doi: 10.1111/j.1756-8765.2009.01030.x
Schneider, N. (2019). OSF | Admins, Mods, and Benevolent Dictators for Life: The Implicit Feudalism of Online Communities. Available online at: https://osf.io/epgwr/?view_only=11c9e93011df4865951f2056a64f5938 (accessed October 26, 2020).
Schneider, N., De Filippi, P., Frey, S., Tan, J. Z., and Zhang, A. X. (2020). Modular politics: toward a governance layer for online communities. arXiv [preprint] arXiv:2005.1370.
Shaw, A., and Hill, B. M. (2014). Laboratories of oligarchy? How the iron law extends to peer production. J. Commun. 64, 215–238. doi: 10.1111/jcom.12082
Stocker, G. (2006). Avoiding the Corporate Death Spiral: Recognizing and Eliminating the Signs of Decline. Quality Press.
Suri, S., and Watts, D. J. (2011). Cooperation and contagion in web-based, networked public goods experiments. PLoS One 6:e16836. doi: 10.1371/journal.pone.0016836
Szell, M., Lambiotte, R., and Thurner, S. (2010). Multirelational organization of large-scale social networks in an online world. Proc. Natl. Acad. Sci. U.S.A. 107, 13636–13641. doi: 10.1073/pnas.1004008107
Tan, J., and Zargham, M. (2021). Introducing Govbase: An Open Database of Projects and Tools in Online Governance. Available online at: https://thelastjosh.medium.com/introducing-govbase-97884b0ddaef
Tchernichovski, O., King, M., Brinkmann, P., Halkias, X., Fimiarz, D., Mars, L., et al. (2017). Tradeoff between distributed social learning and herding effect in online rating systems. SAGE Open 7:215824401769107. doi: 10.1177/2158244017691078
Tchernichovski, O., Parra, L. C., Fimiarz, D., Lotem, A., and Conley, D. (2019). Crowd wisdom enhanced by costly signaling in a virtual rating system. Proc. Natl. Acad. Sci. U. S. A. 116, 7256–7265. doi: 10.1073/pnas.1817392116
Trice, M., and Potts, L. (2018). Building dark patterns into platforms: how GamerGate perturbed Twitter's user experience. Present Tense J. Rhetoric Soc. 6. Available online at: https://www.presenttensejournal.org/volume-6/building-dark-patterns-into-platforms-how-gamergate-perturbed-twitters-user-experience/
Watts, D. J. (1999). Networks, dynamics, and the small-world phenomenon. Am. J. Sociol. 105, 493–527.
Willer, R., Sharkey, A., and Frey, S. (2012). Reciprocity on the hardwood: passing patterns among professional basketball players. PLoS One 7:e49807. doi: 10.1371/journal.pone.0049807
Zhang, A. X., Hugh, G., and Bernstein, M. S. (2020a). PolicyKit: building governance in online communities. arXiv [preprint]. arXiv:2008.04236. doi: 10.1145/3379337.3415858
Zhang, Y. G., Dang, M. Y., and Chen, H. (2020b). An explorative study on the virtual world: investigating the avatar gender and avatar age differences in their social interactions for help-seeking. Inf. Syst. Front. 22, 911–925. doi: 10.1007/s10796-019-09904-2
Keywords: online governance, crowd wisdom, cooperation, costly signaling, collective action, virtual worlds
Citation: Tchernichovski O, Frey S, Jacoby N and Conley D (2021) Experimenting With Online Governance. Front. Hum. Dyn. 3:629285. doi: 10.3389/fhumd.2021.629285
Received: 14 November 2020; Accepted: 23 March 2021;
Published: 26 April 2021.
Edited by:
Nathan Schneider, University of Colorado Boulder, United StatesReviewed by:
Arild Bergh, Norwegian Defence Research Establishment, NorwayUta Kohl, University of Southampton, United Kingdom
Copyright © 2021 Tchernichovski, Frey, Jacoby and Conley. This is an open-access article distributed under the terms of the Creative Commons Attribution License (CC BY). The use, distribution or reproduction in other forums is permitted, provided the original author(s) and the copyright owner(s) are credited and that the original publication in this journal is cited, in accordance with accepted academic practice. No use, distribution or reproduction is permitted which does not comply with these terms.
*Correspondence: Ofer Tchernichovski, b3RjaGVybmkmI3gwMDA0MDtodW50ZXIuY3VueS5lZHU=