- 1Life Sciences Department, Barcelona Supercomputing Center, Barcelona, Spain
- 2Women’s Brain Project, Guntershausen, Switzerland
Historically, biomedical research has been led by and focused on men. The recent introduction of Artificial Intelligence (AI) in this area has further proven this practice to be discriminatory for other sexes and genders, more noticeably for women. To move towards a fair AI development, it is essential to include sex and gender diversity both in research practices and in the workplace. In this context, the Bioinfo4women (B4W) program of the Barcelona Supercomputing Center (i) promotes the participation of women scientists by improving their visibility, (ii) fosters international collaborations between institutions and programs and (iii) advances research on sex and gender bias in AI and health. In this article, we discuss methodology and results of a series of conferences, titled “Sex and Gender Bias in Artificial Intelligence and Health, organized by B4W and La Caixa Foundation from March to June 2021 in Barcelona, Spain. The series consisted of nine hybrid events, composed of keynote sessions and seminars open to the general audience, and two working groups with invited experts from different professional backgrounds (academic fields such as biology, engineering, and sociology, as well as NGOs, journalists, lawyers, policymakers, industry). Based on this awareness-raising action, we distilled key recommendations to facilitate the inclusion of sex and gender perspective into public policies, educational programs, industry, and biomedical research, among other sectors, and help overcome sex and gender biases in AI and health.
Introduction
Artificial intelligence (AI) is pervasive in our everyday lives, and increasingly so in the health domain, from biomedical research to clinical practice (1). Nevertheless, growing evidence of AI reflecting and perpetuating discrimination based on personal attributes, such as sex and gender (see Box 1), is leading to an increased attention to this issue (2) (see Box 1). Addressing bias in AI for biomedical applications requires consideration of ethical, societal and technical aspects that have an impact on the design, deployment and use of AI-based systems (3). Hence, achieving satisfactory outcomes in conventional metrics, such as classification accuracy, is no longer sufficient. To gain insights into health and advance Precision Medicine, it is crucial to factor in the unique biological, behavioral, and environmental characteristics of each individual, including sex and gender that can influence health outcomes and the development of diseases (3).
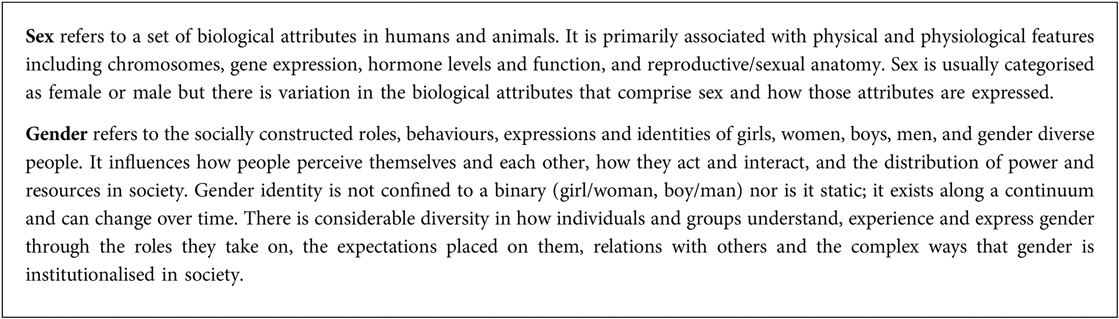
Box 1 Definitions of sex and gender (CIHR Institute of Gender & Health. Available online: https://cihr-irsc.gc.ca/e/48642.html)
The entire and equal participation of women in all areas of society, along with the development of AI-based systems and their fair representation in health data, is a fundamental human right as asserted by two pivotal documents that followed the Universal Declaration of Human Rights (4). The Convention on the Elimination of all Forms of Discrimination Against Women (CEDAW) (5), adopted by the United Nations (UN) in 1979, explicitly articulates that any discrimination against women, including that perpetuated by biased AI-based systems, “violates the principles of equality of rights and respect for human dignity”. The Beijing Declaration and Platform for Action (6), adopted by the UN in 1995, presents a global policy framework and guidance for action to effectively realize gender equality.
Enhancing women's representation in science, technology, engineering, and mathematics (STEM) disciplines, especially computational biology and bioinformatics, is crucial to fight existing and emerging sex and gender bias in AI and health. Underrepresentation of women in these fields has been largely reported (7, 8) and a number of initiatives, increasingly focused on intersectionality (9, 10), have been created. Forms of discrimination against women have been reported in several aspects related to computational biomedical research, including mainstream domain conferences (11), career transitions (12), citations in high-impact journals (13), among many others. Within this framework, the Barcelona Supercomputing Center (BSC) has created Bioinfo4Women (B4W) (14), a non-profit initiative of the Life Sciences Department that began its activities in 2018. In line with the BSC gender equality plan and the United Nations (UN) Sustainable Development Goals (SDGs) (15) (Supplementary Figure S1), B4W aims to increase the representation and visibility of women in bioinformatics and computational biology, specifically in high-level professional positions, through mentorship, training, conferences, and seminars. B4W also focuses on researching and addressing sex and gender biases in the application of AI to biomedicine and healthcare.
In 2020, the B4W designed the project “Sex and Gender Biases in Artificial Intelligence and Health”, in collaboration with La Caixa Foundation, to provide an interdisciplinary framework for reflection about challenges and social problems that impact the population. Thus, during the year 2021, the project took off as a unique series of conferences, in which international experts and citizens collaborated with the common objective of raising awareness about sex and gender bias in AI and health. The conference series included nine hybrid events, from March to June 2021 (Figure 1), consisting of keynote sessions and seminars followed by round tables open to the general public, and two workshops with international invited experts from different professional backgrounds [biomedical researchers, engineers, sociologists, representatives of Non-Governmental Organizations (NGOs) and the industry, journalists, lawyers, policy-makers].
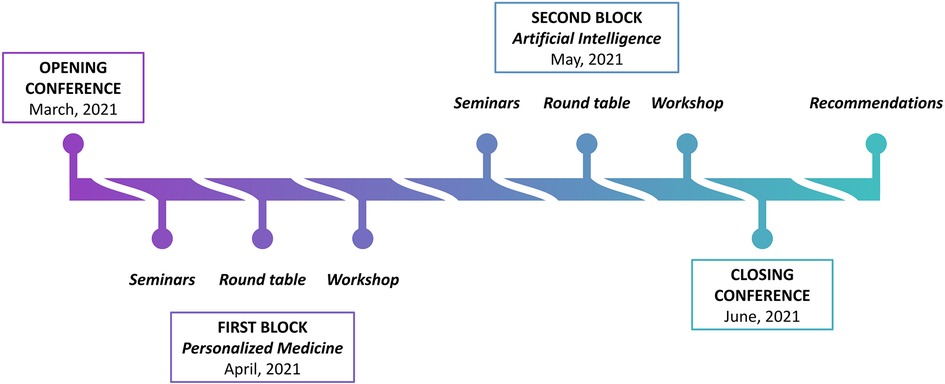
Figure 1. Infographic representing structure, timeline and outcomes of the B4W series of conferences “Sex and Gender Biases in Artificial Intelligence and Health” (Barcelona, March-June 2021). The series of conferences consisted of an opening conference, two blocks of activities focused on two themes (Personalized Medicine and Artificial Intelligence), and a closing conference. The main outcomes were distilled into recommendations generated after the end of the event. The detailed calendar of the series of conferences and participating domain experts are provided as Supplementary Table S1.
In this work, we summarize and discuss the main outcomes of this event, especially the two workshops, and the relevance of the identified solutions to raise awareness about sex and gender bias in AI and health. Based on the acquired knowledge, we identified four major strategic areas of action (lack of data; social impact and awareness; AI biases; regulatory aspects) and proposed specific recommendations, which focus on scientific, educational, and political strategies (e.g., enhancing women's representation in STEM fields) that would help overcome sex and gender bias in AI and ultimately benefit women's health.
Methods
Organization of the series of conferences
The series of conferences titled “Sex and Gender Biases in Artificial Intelligence and Health” was developed and organized by the B4W with the support of La Caixa Foundation (16). This initiative was carried out over four months, starting on 16th March 2021, and ending on 16th June 2021 (Supplementary Table S1). The series kicked off with an opening conference where members of B4W presented the programme, agenda, and objectives, with contributed talks from public institutions as well as from recognized researchers in the area. The two following conferences, held in April and May, tackled the problem of sex and gender bias from two perspectives: personalized medicine and AI. The topics were debated in the form of seminars and round tables open to the public, thus providing a collective space for reflection. Two closed participatory workshops with invited experts on the subjects subsequently followed the previous activities on the same days. Recommendations have been generated based on the outcomes of such workshops after the end of the series in June when a final conference summarized the main conclusions that emerged from the event. A depiction of the outlined process is reported (Figure 1). All the activities, except the closed participatory workshops, were recorded and are available, together with further information about the event, at the B4W website (17).
Design thinking workshops
The methodology applied in the two workshops of the series of conferences was based on Design Thinking (18, 19), a technique consisting of collaborative sessions that are used to innovate and focus on the needs of people. The work process has five steps that are recommended as key to the co-creation of research: empathize, define, ideate, prototype and test. The process considers both the socio-ethical and the technological aspects and ensures that the proposed innovative solutions are viable and sustainable. This methodology of co-creation and participatory research typically seeks to balance interests, benefits and responsibilities between the relevant stakeholders, focus attention on user opinions, needs, and make the whole process from planning to implementation, with a transparent and inclusive process with citizens (20). Practically, the discussion among the workshop participants was organized around key challenges (Supplementary Table S2) and facilitated by Co-emprèn (21) using the virtual whiteboard software Miró. The first workshop, titled “Building a future for equality: challenges and action strategies for personalized medicine”, gathered 30 experts. The second workshop, titled “Building a future for equality: a route map to an inclusive AI for a better health system”, gathered 28 experts. In both workshops, the invited participants, who contributed on a voluntary basis, were divided into 4 groups of 5 or 6 people. Each of these groups selected three challenges to discuss and exposed the proposed solutions to the others. All responses were collected using a system of codes (Lack of data; Social impact and awareness; AI biases; Regulatory aspects), reflecting the solutions provided by the specialists in the area. These proposed solutions were categorized by the authors into strategic areas of action (see Results, “Categorization of the proposed solutions”) and further examined to deduct general recommendations (see Results, “Recommendations”).
Recommendations derived from the event
The scope of the discussion generated during the workshops was very ample and interdisciplinary. It included possible solutions and goals to overcome the existing biases related to personalized medicine and AI, exposing the underrepresentation of women in the STEM fields and decision-making positions. The responses of the participants encompassed several areas, such as measures to promote institutional and legal changes in public policies, and at the level of knowledge, to fix the data and to improve the tools and realize a more effective personalized medicine, specifically tailored to women and men, as well as in the design of new technologies and correction of algorithms considering the sex and gender dimension in scientific research. Given such a high level of complexity, we first identified four major strategic areas of action, based on the system of codes that we used to collect the information (Lack of data; Social impact and awareness; AI biases; Regulatory aspects), and then derived specific recommendations at different levels (AI practitioners and academies; the industry; the civil society; policy-makers and governments) (see Results, “Recommendations”).
Results
A large-scale awareness-raising event to gather solutions to key challenges
The series of conferences organized by B4W and La Caixa Foundation (Figure 1) was a sizable event with a substantial impact and a complex organization that was specifically tailored to fulfill two major objectives. The first objective was to provide an open space for reflection that not only involved specialists but, most importantly, brought the citizens in contact with them. Despite the limitations due to the COVID-19 pandemic, the hybrid format enabled this objective to be satisfactorily fulfilled. The specialists represented academic and scientific professionals (university professors and researchers, scientists, independent researchers, social science specialists), NGOs and civil society organizations (executive directors, project coordinators, social workers, volunteers), and public sector professionals (public officials, politicians, professionals specialized in government areas), mostly from western European countries. The second objective was to design a large-scale event with a tangible outcome, which is represented by the recommendations provided in this work. This objective was fulfilled thanks to the rigorous organization and execution of two Design Thinking workshops with international experts (see Methods) faced with intended challenges and key questions (Supplementary Table S2).
Categorization of the proposed solutions
The activities of the series of conferences, and in particular the two workshops, offered the opportunity to bring people from different fields of expertise together. The Design Thinking methodology enhanced a dynamic debate in reduced groups followed by a general discussion that aimed to find the most relevant challenges and proposals to tackle them. These recommendations have a very broad scope from social, technical, ethical, regulatory and medical aspects, product of the interdisciplinary profile of the project that marks a complete approach and the results are applicable in the planning of AI in health with a sex and gender perspective. To ease the analysis, we categorized the main takeaways of the workshops into four major strategic areas of action: (1) Lack of data; (2) Social impact and awareness; (3) AI biases; (4) Regulatory aspects. The corresponding proposed solutions are provided in Table 1.
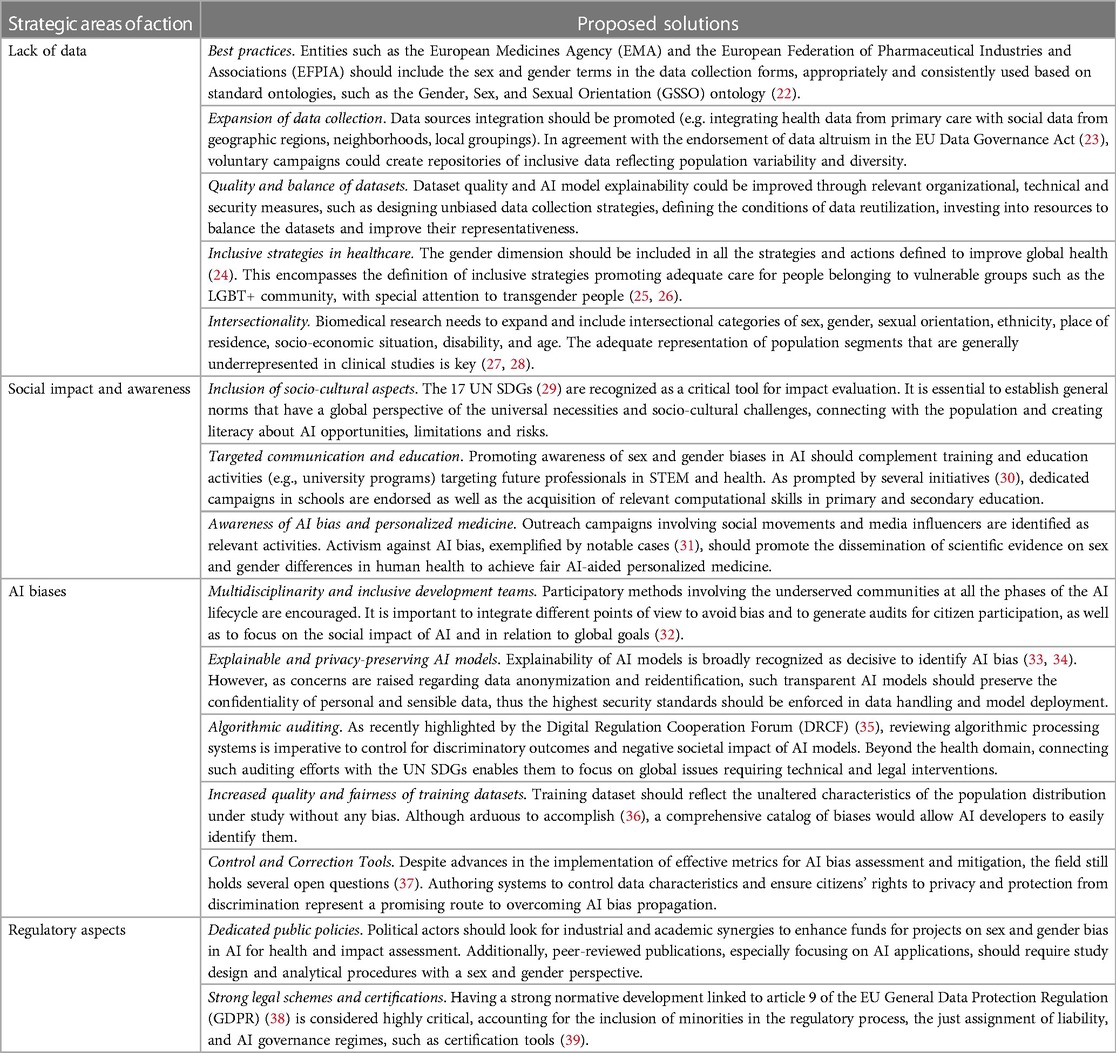
Table 1. Four major strategic areas of action identified in the workshops and the corresponding proposed solutions.
Recommendations
The main outcome of the event is the creation of recommendations deducted from the information gathered in the two Design Thinking workshops. Specifically, the qualitative analysis of the solutions to the key challenges proposed by the 58 participating experts (see Results, “Categorization of the proposed solutions”) allowed us to derive a list of recommendations that specifically target distinct stakeholders: AI practitioners and academies; the industry; the civil society; policy-makers and governments (Table 2).
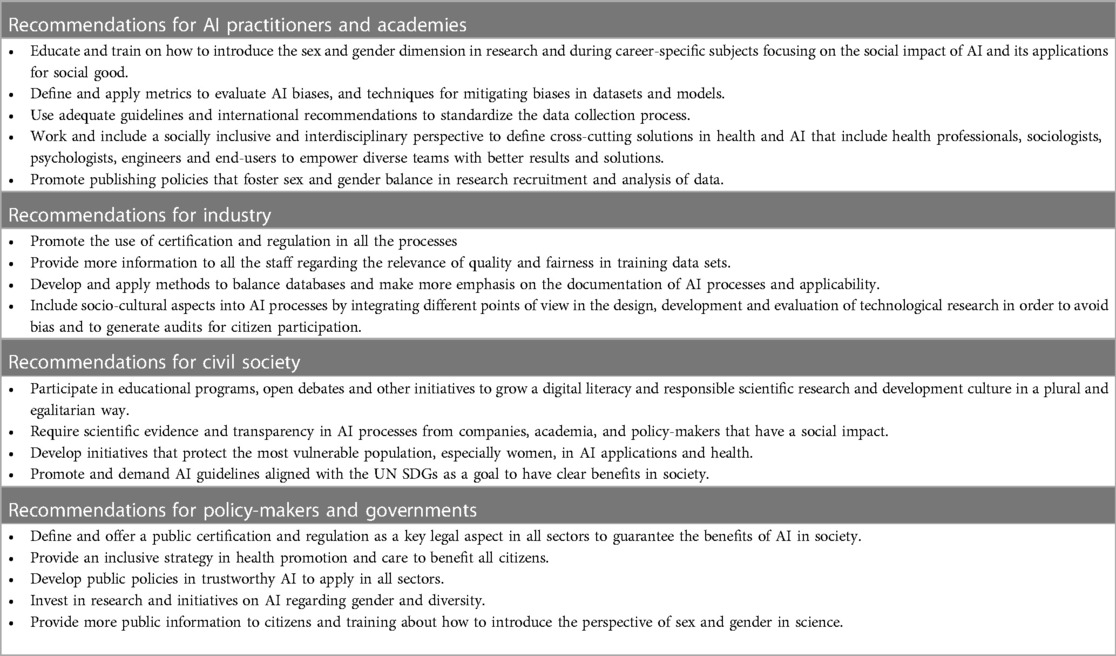
Table 2. Recommendations deducted from the information gathered in the two design thinking workshops.
Comparison with related work
A highly debated topic in the two workshops focused on the enhanced inclusion of women and diverse perspectives in STEM disciplines as a potential factor to break biases in AI (see Table 1, “Social impact and awareness”). A large amount of suggested actions to broaden participation in STEM can be found in the literature. Recommendations have been compiled by women in STEM (40), specialists in distinct fields [e.g., women in immunology (41)], or international agencies such as UNESCO (42). Moreover, recommendations have been systematically reviewed from national reports (43), and synthesized from conferences focusing on specific fields [e.g., women in neuroscience (44)]. Commonly, surveys are used as effective tools to distill recommendations, such as those on gender transformation in global science provided by the International Science Council (ISC) and collaborators (45). The ISC recommendations are based on the results of an extensive online survey conducted among over 250 organizations around the globe, including science academies, international disciplinary unions and associations covering engineering, medical and social sciences (45). Due to their wide scope, we used the ISC recommendations as a baseline to qualitatively assess the level of agreement and complementarity with B4W recommendations and mission (Table 3).
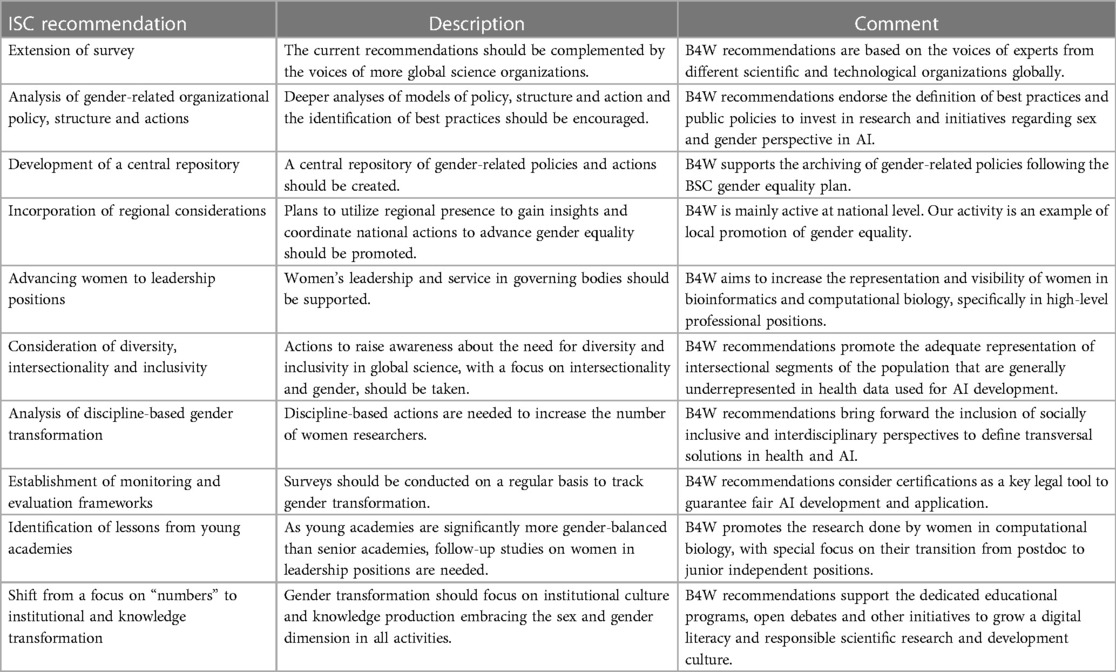
Table 3. Recommendations form a study on gender equality by the international science council (ISC) and collaborators (45) compared with B4W recommendations and mission.
Discussion
AI systems are able to deliver cost-effective, dynamic access to health information and efficient handling of complex problems related to human diseases. However, if the application of such technologies results in unfair and unjust outcomes, they can propagate inequality and discrimination in our society, especially against women and other underserved communities, having a severe impact on the health system and the lives of women and men (46–48). The emergence of sex and gender bias in AI and health is linked to several unresolved issues, such as the diversity gap in clinical trials (49, 50), poor data management (51, 52), the lack of data collection accountability (53, 54), among many others. Thus, different strategies need to be defined to help the society and the experts understand and address these issues, especially in high-stakes AI applications (55, 56). Raising awareness and improving social impact by involving all stakeholders impacted by AI is of the highest relevance to avoid inequality and human's rights violations. In particular, the role of policy-makers and governments is fundamental to implement expert recommendations. Policies should boost research and development in sex and gender issues in health and AI following ethical values, normative environments, educational strategies, certification frameworks, and a transparent the financial flow requiring AI trustworthiness in requests and tenders of funds, as recently indicated by the Ethical Funding for Trustworthy AI framework (EFTAI) (57).
Dedicated initiatives at institutional and research level, such as B4W, represent effective ways to discuss, investigate and find solutions to sex and gender bias in AI and health. One of the main objectives of B4W is to promote biomedical research that includes the sex and gender perspective to highlight the relevance of these categories in medical research and technological development to achieve a fair realization of personalized medicine.
The conference series “Sex and Gender Bias in Artificial Intelligence and Health”, organized by B4W in collaboration with La Caixa Foundation, represented a unique opportunity to introduce social, ethical, legal and technical challenges of AI, providing a interdisciplinary perspective to a broad and diverse audience. Topics of gender diversity in STEM disciplines and the impact of sex and gender biases on women's health were discussed. The event ecompassed a variety of activities including keynotes, open debates and Design Thinking workshops, providing a space for open debate among different domain experts and the society on sex and gender bias in AI and health. The main outcomes of the event can be summarized in a call of action to include the sex and gender dimension in research and technology to guarantee scientific quality and excellence.
The Design Thinking methodology applied to the workshops allowed us to obtain insights from different perspectives, discussing risks and opportunities in an egalitarian environment. This project showed the relevance of defining participatory strategies regarding AI aiming to raise awareness and define a roadmap to apply discipline-specific measures and social impact assessment. Following the schemes established during the sessions, specific actions to change in the present situation were identified at different levels of science, citizenship, and the public political sector, which resulted in a series of recommendations that are reported in this work. Also, it is essential to acknowledge the inherent limitations of such an initiative and its outcomes, mainly related to challenges associated with inclusivity, limited reach, and skewed perspectives.
As the organization of the event itself demonstrated, promoting collaboration and debate among different experts and networks that could contribute to knowledge generation and to inspire and promote research projects with a social impact is crucial to raise awareness about the issue of sex and gender bias in AI and health. As a result, B4W members have been subsequently invited to participate in initiatives such as Women in Data Science (WiDS Barcelona) (58), and the Association for Computing Machinery's Council on Women in Computing (ACM-W) chapter (59). Moreover, bringing society closer to the health implications of sex and gender biases in AI helps identify and make innovative proposals to address them, inspiring and guiding future work with a more inclusive approach. Finally, implementing public policies, affirmative actions, and legislation will allow underrepresented communities, such as women and girls in STEM, to effectively participate in the workforce that will produce the new generations of AI systems (60–62). In this regard, B4W was invited to the Catalan Parliament to expose the conclusions of the event and co-authored a study report for the Panel of the Future of Science and Technology (STOA) of the European Parliament (54).
Data availability statement
The original contributions presented in the study are publicly available. This data can be found here: https://bioinfo4women.bsc.es/sex-and-gender-bias-in-artificial-intelligence-and-health/.
Ethics statement
Ethical review and approval was not required for this study in accordance with the local legislation and institutional requirements. Written informed consent from the program evaluation focus group participants was not required to participate in this study in accordance with the national legislation and the institutional requirements.
Author contributions
NB, AC, SCS, DC and MJ contributed to the design and implementation of this study and the analysis of the outcomes of the B4W conference series. All authors contributed to the article and approved the submitted version.
Funding
This work was funded by the “5a Convocatòria de projectes de Reflexió del Palau Macaya”. Fundación La Caixa, project: PM19-00039 “Gender Bias in Science”.
Acknowledgments
The authors would like to acknowledge all the members of B4W involved in the preparation and organization of the event, as well as the speakers, the attendees, and the staff of Palau Macaya.
Conflict of interest
The authors declare that the research was conducted in the absence of any commercial or financial relationships that could be construed as a potential conflict of interest.
Publisher's note
All claims expressed in this article are solely those of the authors and do not necessarily represent those of their affiliated organizations, or those of the publisher, the editors and the reviewers. Any product that may be evaluated in this article, or claim that may be made by its manufacturer, is not guaranteed or endorsed by the publisher.
Supplementary material
The Supplementary Material for this article can be found online at: https://www.frontiersin.org/articles/10.3389/fgwh.2023.970312/full#supplementary-material
References
1. Rajpurkar P, Chen E, Banerjee O, Topol EJ. AI in health and medicine. Nat Med. (2022) 28:31–8. doi: 10.1038/s41591-021-01614-0
2. Cirillo D, Catuara-Solarz S, Guney E, editors. Sex and gender bias in technology and artificial intelligence: Biomedicine and healthcare applications. Amsterdam, Netherlands: Elsevier (2022).
3. Mauvais-Jarvis F, Bairey Merz N, Barnes PJ, Brinton RD, Carrero JJ, DeMeo DL, et al. Sex and gender: modifiers of health, disease, and medicine. Lancet. (2020) 396(10250):565–82. doi: 10.1016/S0140-6736(20)31561-0
5. Convention on the Elimination of All Forms of Discrimination against Women New York, 18 December 1979. OHCHR n.d. Available at: https://www.ohchr.org/en/instruments-mechanisms/instruments/convention-elimination-all-forms-discrimination-against-women (Accessed January 3, 2023).
6. Fourth World Conference on Women. Beijing Declaration n.d. Available at: http://www.un-documents.net/beijingd.htm (Accessed January 3, 2023).
7. Bonham KS, Stefan MI. Women are underrepresented in computational biology: an analysis of the scholarly literature in biology, computer science and computational biology. PLoS Comput Biol. (2017) 13:e1005134. doi: 10.1371/journal.pcbi.1005134
8. Holman L, Stuart-Fox D, Hauser CE. The gender gap in science: how long until women are equally represented? PLoS Biol. (2018) 16:e2004956. doi: 10.1371/journal.pbio.2004956
9. Chirigati F, Rastogi A. Connecting black women in computational biology. Nat Comput Sci. (2021) 1:11–3. doi: 10.1038/s43588-020-00013-8
10. BlackWomenCompBio n.d. Available at: https://www.blackwomencompbio.org/ (Accessed January 4, 2023).
11. Tran D, Valtchanov A, Ganapathy K, Feng R, Slud E, Goldblum M, et al. Analyzing the machine learning conference review process 2020. doi: 10.48550/arXiv.2011.12919
12. Lerchenmueller MJ, Sorenson O. The gender gap in early career transitions in the life sciences. Res Policy. (2018) 47:1007–17. doi: 10.1016/j.respol.2018.02.009
13. Chatterjee P, Werner RM. Gender disparity in citations in high-impact journal articles. JAMA Netw Open. (2021) 4:e2114509. doi: 10.1001/jamanetworkopen.2021.14509
14. Bioinfo 4 women – Outstanding Young Female Bioinformaticians n.d. Available at: https://bioinfo4women.bsc.es/ (Accessed January 4, 2023).
15. Take Action for the Sustainable Development Goals. United Nations Sustainable Development 2018. Available at: https://www.un.org/sustainabledevelopment/sustainable-development-goals/ (Accessed June 13, 2022).
16. “la Caixa” Foundation n.d. Available at: http://fundacionlacaixa.org/en/ (Accessed January 4, 2023).
17. SEX AND GENDER BIAS IN ARTIFICIAL INTELLIGENCE AND HEALTH n.d. Available at: https://bioinfo4women.bsc.es/sex-and-gender-bias-in-artificial-intelligence-and-health/ (Accessed June 13, 2022).
18. McLaughlin JE, Wolcott MD, Hubbard D, Umstead K, Rider TR. A qualitative review of the design thinking framework in health professions education. BMC Med Educ. (2019) 19:98. doi: 10.1186/s12909-019-1528-8
19. Pauceanu AM. Design thinking for innovation. Innovation, innovators and business. Singapore: Springer Nature Singapore (2023). 71–98.
20. WHO Centre for Indigenous Peoples’ Nutrition and Environment, “INDIGENOUS PEOPLES & PARTICIPATORY HEALTH RESEARCH” n.d. Available at: https://www.mcgill.ca/cine/files/cine/partreresearch_english.pdf (Accessed January 3, 2023).
21. Emprenedoria, innovació, col.laboració. Co-emprèn n.d. Available at: https://coempren.com/ (Accessed January 4, 2023).
22. Kronk C, Tran GQ, Wu DTY. Creating a queer ontology: the gender, sex, and sexual orientation (GSSO) ontology. Stud Health Technol Inform. (2019) 264:208–12. doi: 10.3233/SHTI190213
23. EUR-Lex - 32022R0868 - EN - EUR-Lex n.d. Available at: https://eur-lex.europa.eu/legal-content/EN/TXT/?uri=CELEX%3A32022R0868 (Accessed January 5, 2023).
24. Clark J, Horton R. A coming of age for gender in global health. Lancet. (2019) 393:2367–9. doi: 10.1016/S0140-6736(19)30986-9
25. Safer JD. Research gaps in medical treatment of transgender/nonbinary people. J Clin Invest. (2021) 131(4):e142029. doi: 10.1172/JCI142029
26. Santora T. How four transgender researchers are improving the health of their communities. Nat Med. (2021) 27:2074–7. doi: 10.1038/s41591-021-01597-y
27. Boden-Albala B. Confronting legacies of underrepresentation in clinical trials: the case for greater diversity in research. Neuron. (2022) 110:746–8. doi: 10.1016/j.neuron.2021.12.008
28. Bierer BE, Meloney LG, Ahmed HR, White SA. Advancing the inclusion of underrepresented women in clinical research. Cell Rep Med. (2022) 3:100553. doi: 10.1016/j.xcrm.2022.100553
29. UN, “THE 17 GOALS” n.d. Available at: https://sdgs.un.org/es/goals (Accessed January 5, 2023).
30. Herold B. Why schools need to talk about racial bias in AI-powered technologies. Education Week. (2022) 12:13. Available at: https://www.edweek.org/leadership/why-schools-need-to-talk-about-racial-bias-in-ai-powered-technologies/2022/04 (Accessed January 5, 2023).
31. Buolamwini J. Gender Shades: Intersectional Accuracy Disparities in Commercial Gender Classification –. MIT Media Lab n.d. Available at: https://www.media.mit.edu/publications/gender-shades-intersectional-accuracy-disparities-in-commercial-gender-classification/ (Accessed January 5, 2023).
32. Hsu Y-C, Verma H, Mauri A, Nourbakhsh I, Bozzon A. Empowering local communities using artificial intelligence. Patterns. (2022) 3:100449. doi: 10.1016/j.patter.2022.100449
33. Linardatos P, Papastefanopoulos V, Kotsiantis S. Explainable AI: a review of machine learning interpretability methods. Entropy. (2020) 23(1):18. doi: 10.3390/e23010018
34. Krenn M, Pollice R, Guo SY, Aldeghi M, Cervera-Lierta A, Friederich P, et al. On scientific understanding with artificial intelligence. Nat Rev Phys. (2022) 4:761–9. doi: 10.1038/s42254-022-00518-3
35. Auditing algorithms: the existing landscape, role of regulators and future outlook. GOVUK n.d. Available at: https://www.gov.uk/government/publications/findings-from-the-drcf-algorithmic-processing-workstream-spring-2022/auditing-algorithms-the-existing-landscape-role-of-regulators-and-future-outlook (Accessed January 6, 2023).
36. Baeza-Yates R. Data and algorithmic bias in the web. Proceedings of the 8th ACM conference on web science - WebSci ‘16; New York, New York, USA: ACM Press (2016). doi: 10.1145/2908131.2908135
37. Jones GP, Hickey JM, Di Stefano PG, Dhanjal C, Stoddart LC, Vasileiou V. Metrics and methods for a systematic comparison of fairness-aware machine learning algorithms 2020. doi: 10.48550/arXiv.2010.03986
38. Art. 9 GDPR – Processing of special categories of personal data - General Data Protection Regulation (GDPR). General Data Protection Regulation (GDPR) n.d. Available at: https://gdpr-info.eu/art-9-gdpr/ (Accessed January 6, 2023).
39. AI Certification: Advancing Ethical Practice by Reducing Information Asymmetries n.d. Available at: https://ieeexplore.ieee.org/document/9427056 (Accessed January 6, 2023).
40. Guevara-Ramírez P, Ruiz-Pozo VA, Cadena-Ullauri S, Salazar-Navas G, Bedón AA, V-Vázquez JF, et al. Ten simple rules for empowering women in STEM. PLoS Comput Biol. (2022) 18:e1010731. doi: 10.1371/journal.pcbi.1010731
41. Pierce SK, Schwartzberg PL, Shah NN, Taylor N. Women in immunology: 2020 and beyond. Nat Immunol. (2020) 21:254–8. doi: 10.1038/s41590-020-0618-4
42. [No title] n.d. Available at: https://unesdoc.unesco.org/ark:/48223/pf0000245717 (Accessed January 3, 2023).
43. Holloman T, Lee WC, London J, Halkiyo A, Jew G, Watford B. A historical and policy perspective on broadening participation in STEM: insights from national reports (1974–2016). 2018 CoNECD - the collaborative network for engineering and computing diversity conference 2018.
44. Berryhill ME, Desrochers TM. Addressing the gender gap in research: insights from a women in neuroscience conference. Trends Neurosci. (2021) 44:419–21. doi: 10.1016/j.tins.2021.03.004
45. Gender Equality in Science - Home. Gender Equality in Science - Home n.d. Available at: http://stories.council.science/3122062/ (Accessed January 3, 2023)).
46. Cirillo D, Catuara-Solarz S, Morey C, Guney E, Subirats L, Mellino S, et al. Sex and gender differences and biases in artificial intelligence for biomedicine and healthcare. NPJ Digit Med. (2020) 3:81. doi: 10.1038/s41746-020-0288-5
47. de Hond AAH, Leeuwenberg AM, Hooft L, Kant IMJ, Nijman SWJ, van Os HJA, et al. Guidelines and quality criteria for artificial intelligence-based prediction models in healthcare: a scoping review. NPJ Digit Med. (2022) 5:2. doi: 10.1038/s41746-021-00549-7
48. Vokinger KN, Feuerriegel S, Kesselheim AS. Mitigating bias in machine learning for medicine. Commun Med. (2021) 1:25. doi: 10.1038/s43856-021-00028-w
49. Sharma A, Palaniappan L. Improving diversity in medical research. Nat Rev Dis Primers. (2021) 7:74. doi: 10.1038/s41572-021-00316-8
50. Center for Drug Evaluation, Research. Enhancing the Diversity of Clinical Trial Populations — Eligibility Criteria, Enrollment Practices, and Trial Designs Guidance for Industry. US Food and Drug Administration n.d. Available at: https://www.fda.gov/regulatory-information/search-fda-guidance-documents/enhancing-diversity-clinical-trial-populations-eligibility-criteria-enrollment-practices-and-trial (Accessed January 5, 2023).
51. Lakens D. Is my study useless? Why researchers need methodological review boards. Nature. (2023) 613:9. doi: 10.1038/d41586-022-04504-8
52. Qureshi R, Gough A, Loudon K. The SPIRIT checklist-lessons from the experience of SPIRIT protocol editors. Trials. (2022) 23:359. doi: 10.1186/s13063-022-06316-7
53. Hutchinson B, Smart A, Hanna A, Denton E, Greer C, Kjartansson O, et al. Towards accountability for machine learning datasets. Proceedings of the 2021 ACM conference on fairness, accountability, and transparency; New York, NY, USA: ACM (2021). doi: 10.1145/3442188.3445918
54. Auditing the quality of datasets used in algorithmic decision-making systems n.d. Available at: https://www.europarl.europa.eu/thinktank/en/document/EPRS_STU(2022)729541 (Accessed January 5, 2023).
55. Topol EJ. High-performance medicine: the convergence of human and artificial intelligence. Nat Med. (2019) 25:44–56. doi: 10.1038/s41591-018-0300-7
56. Zhang A, Xing L, Zou J, Wu JC. Shifting machine learning for healthcare from development to deployment and from models to data. Nat Biomed Eng. (2022) 6:1330–45. doi: 10.1038/s41551-022-00898-y
57. Gardner A, Smith AL, Steventon A, Coughlan E, Oldfield M. Ethical funding for trustworthy AI: proposals to address the responsibilities of funders to ensure that projects adhere to trustworthy AI practice. AI Ethics. (2022) 2:277–91. doi: 10.1007/s43681-021-00069-w
58. Women in Data Science Barcelona & Madrid. Aula Magna Business School 2021. Available at: https://ambs.education/wids/ (Accessed March 31, 2023).
59. ACM-W supporting, celebrating, and advocating for Women in Computing n.d. Available at: https://women.acm.org/ (Accessed March 31, 2023).
60. Cassels R, Duncan A. Gender equity insights 2020: Delivering the business outcomes. Bankwest Curtin Economics Centre (2020).
61. Stone M. Investing in Girls’ STEM Education in Developing Countries. Council on Foreign Relations 2019. Available at: https://www.cfr.org/report/investing-girls-stem-education-developing-countries (Accessed June 13, 2022).
Keywords: gender bias, AI, health, bias in science, gender policies
Citation: Buslón N, Cortés A, Catuara-Solarz S, Cirillo D and Rementeria MJ (2023) Raising awareness of sex and gender bias in artificial intelligence and health. Front. Glob. Womens Health 4:970312. doi: 10.3389/fgwh.2023.970312
Received: 15 June 2022; Accepted: 18 August 2023;
Published: 6 September 2023.
Edited by:
Sanne Peters, Imperial College London, United KingdomReviewed by:
Carinna Hockham, Imperial College London, United Kingdom© 2023 Buslón, Cortés, Catuara-Solarz, Cirillo and Rementeria. This is an open-access article distributed under the terms of the Creative Commons Attribution License (CC BY). The use, distribution or reproduction in other forums is permitted, provided the original author(s) and the copyright owner(s) are credited and that the original publication in this journal is cited, in accordance with accepted academic practice. No use, distribution or reproduction is permitted which does not comply with these terms.
*Correspondence: Nataly Buslón bmF0YWx5LmJ1c2xvbkBic2MuZXM= Maria José Rementeria bWFyaWEucmVtZW50ZXJpYUBic2MuZXM=