- 1Department of Psychiatry, University of Cambridge, Cambridge, United Kingdom
- 2Wysa Inc., Boston, MA, United States
Background: Maternal mental health care is variable and with limited accessibility. Artificial intelligence (AI) conversational agents (CAs) could potentially play an important role in supporting maternal mental health and wellbeing. Our study examined data from real-world users who self-reported a maternal event while engaging with a digital mental health and wellbeing AI-enabled CA app (Wysa) for emotional support. The study evaluated app effectiveness by comparing changes in self-reported depressive symptoms between a higher engaged group of users and a lower engaged group of users and derived qualitative insights into the behaviors exhibited among higher engaged maternal event users based on their conversations with the AI CA.
Methods: Real-world anonymised data from users who reported going through a maternal event during their conversation with the app was analyzed. For the first objective, users who completed two PHQ-9 self-reported assessments (n = 51) were grouped as either higher engaged users (n = 28) or lower engaged users (n = 23) based on their number of active session-days with the CA between two screenings. A non-parametric Mann–Whitney test (M–W) and non-parametric Common Language effect size was used to evaluate group differences in self-reported depressive symptoms. For the second objective, a Braun and Clarke thematic analysis was used to identify engagement behavior with the CA for the top quartile of higher engaged users (n = 10 of 51). Feedback on the app and demographic information was also explored.
Results: Results revealed a significant reduction in self-reported depressive symptoms among the higher engaged user group compared to lower engaged user group (M–W p = .004) with a high effect size (CL = 0.736). Furthermore, the top themes that emerged from the qualitative analysis revealed users expressed concerns, hopes, need for support, reframing their thoughts and expressing their victories and gratitude.
Conclusion: These findings provide preliminary evidence of the effectiveness and engagement and comfort of using this AI-based emotionally intelligent mobile app to support mental health and wellbeing across a range of maternal events and experiences.
Introduction
Parenthood is a transition that can pose significant challenges to mental and physical health across the maternal spectrum, including pre-conception, antenatal period, and during or after giving birth (1–10).
Perinatal mental health disorders are common (11). In the United Kingdom (UK), perinatal mental health problems can affect 10%–20% of women either during pregnancy or within one year of giving birth (12). According to the American Pregnancy Association and Postpartum Support International, approximately 70%–80% of new mothers experience negative feelings after giving birth (13). For certain demographic groups this is higher, for example, up to 60% for adolescent mothers with a low income (14, 15). Depression during pregnancy and the postpartum period is associated with multiple poor outcomes for parental well-being and childhood development (7, 16–18).
Maternal mental health is a global public health and economic challenge (19–22). The estimated accumulated national economic cost of perinatal depression and anxiety in the UK is £6.6 billion (11). While treatments for maternal mental health care exist, research suggests that implementation can be challenging and variable (23).
Technology could play a significant role in addressing barriers to care for maternal mental health (24, 25). The acceptability, feasibility and effectiveness of perinatal depression digital interventions have been evaluated in various pilot studies, randomized controlled trials (RCTs) and systematic reviews (26–36). While maternal mental health digital technology could help improve accessibility, offer timely psychosocial support, as well as potentially improve the quality of information being collected, much more research is needed in this area to carefully examine its potential benefits vs. its limitations (37). A study that published recommendations based on user feedback to inform future development of digital maternal mental health support included the proposal of adding an Artificial Intelligence (AI) chatbot (38). Using dialogue-led personalized tools, AI-based Conversational agents (CAs; chatbots) could potentially facilitate effective and safe guided conversations and collect information beyond limited survey questions and self-guided sessions.
AI systems using CA have already been developed to provide medical information to support child physical health for new mothers (39–41). In more recent years, proposals have emerged on how AI could play a distinctive digital role in supporting maternal mental health and wellbeing (42). A clinical trial that randomized women during their birth hospitalization to either “chatbot plus treatment as usual” or “treatment as usual” (TAU) reported that many participants used the chatbot at least once every 2 weeks and that most users reported medium or high satisfaction with the CA AI system (43). The authors also reported that most participants reported medium or high degrees of therapeutic alliance and acceptability (43). Related to these findings, an additional RCT publication evaluated the effectiveness of the automated CA on changes in symptoms of anxiety and depression. The authors reported that at the 6-week postpartum follow-up there were no statistically significant group differences between the “chatbot use” or “TAU” (44). A later related publication reported that the CA intervention group (“WB001+ TAU”) had a significant reduction in depression scores as compared to a TAU-only control group 6-week postpartum after birth hospitalization (45).
A pre-pilot development and usability study examining an AI system involving a cohort of enrolled Kenyan pregnant women and new mothers reported that most women submitted at least three mood ratings, sent at least one message to the AI system and that approximately a third of women engaged beyond registration (46). Most AI users reported a positive attitude and having trust in using the AI system and that life changes were attributed to using it and reported an estimate that using the alpha version of the AI system may have improved mood (46).
A different approach using a supervised machine learning CA for perinatal mental healthcare was proposed by authors that could be an effective approach for monitoring the mental health status of perinatal women in real time while collecting user health data. The authors analyzed the 31 characteristics of 223 samples and trained a supervised machine learning model to determine the anxiety, depression, and hypomania index of perinatal women (47).
While this literature shows some degree of initial promise, much more research is required to determine how CA AI systems can safely support maternal mental health and hence the motivation to perform our study as a contribution toward furthering the understanding AI's potential role in maternal mental health.
In our study, we examine the use of an AI-based emotionally intelligent mobile app (“Wysa”) aimed at building mental resilience and promoting mental well-being using a text-based CA. Previous studies evaluating Wysa have shown significant reductions in depressive symptoms (48–50). The conversation-based tools and techniques encourage users to manage their anxiety, energy, focus, sleep, relaxation, loss, worries, conflicts, and other concerns. Wysa responds to emotions that a user expresses and recommends evidence-based self-help tools and techniques such as Cognitive Behavioural Therapy (CBT), Acceptance and Commitment Therapy (ACT), Dialectical Behaviour Therapy (DBT), motivational interviewing, positive behavior support, behavioral reinforcement, mindfulness, and guided micro actions that encourage users to build emotional resilience skills.
Our study has two objectives: (1) To examine the effectiveness of Wysa by comparing changes in self-reported depressive symptoms between higher vs. lower engaged groups (n = 51) involving users that self-reported a maternal event, and (2) To perform a qualitative analysis to understand the themes being raised for a subset of user messages provided by higher engaged users (n = 10). This study also discusses post hoc observations, clinical implications, and other research implications derived from our study.
Methods
Study design and participants
The study duration occurred between February and September, 2019 (pre-pandemic). The participants were initially selected from a pool of real-world users (N = 380,500 users) who used the Wysa app during the study period and who submitted any of the maternal event keywords during their conversation with the CA (n = 5,373). Individuals reported at least once about an ongoing maternal event in response to one of these messages: (1) “Tell me about any recent major changes or events in your life. It could be something stressful or even a good change like moving home or getting a new job.”, (2) “Take a few deep breaths. Tell me the first thought on your mind?”, (3) “I’m here for you. What exactly happened?” (4) “Okay let's talk about that. Go on”, (5) “I understand. Is there more?”. Multiple example screenshots of the Wysa app that match the time period of this study can be found in Supplementary Material S1.
A subgroup of N = 2,037 users (2,037 out of 5,373 active users mentioned above) were then grouped into four maternal event categories: Pre-pregnancy, Pregnancy, Perinatal, and Postpartum, which are defined in Table 1. This categorization was used by the researcher for the thematic qualitative analysis (testing Objective 2) to assist in making observations that were categorised into the different maternal event stages (pre-pregnancy and pre-conception, pregnancy, perinatal, postpartum). Furthermore, eligibility (inclusion and exclusion) criteria was applied to determine user enrolment into the study, which is listed in Table 2 and Supplementary Multimedia Appendix A, the latter of which shows the sampling method used for this study.
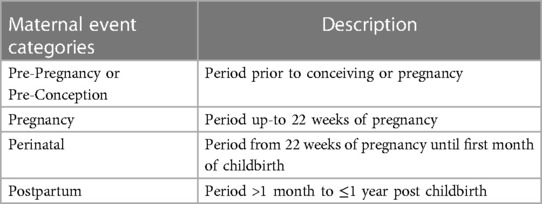
Table 1. Users were grouped into the following categories based on their self-reported maternal events.
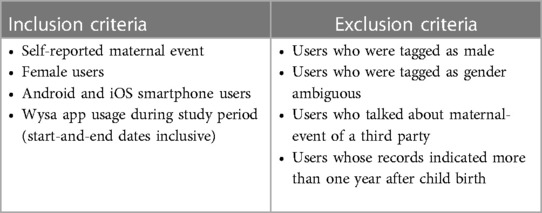
Table 2. The following inclusion and exclusion criteria were defined to determine participant eligibility.
A final sample of n = 10 users were included in the qualitative analysis. The selection method used a non-probability-based sampling to select 10 users who had an engagement density of greater than 75%. Furthermore, after additional eligibility screening was applied based on the criteria of completing the mental health self-reported depressive symptoms assessment questionnaire at two time points (defined below in the Data Analysis Section “Effectiveness Analysis” section) a final sample size of n = 51 users were included in the Effectiveness Analysis (testing Objective 1 using a statistical analysis approach).
Instruments (measures)
The Patient Health Questionnaire 9 (PHQ-9) was used to measure self-reported depressive symptoms at baseline and follow-up. This validated self-report questionnaire (51) consists of nine DSM-IV criteria (i.e., questions) and the participant answers (i.e., scores) each of those on a scale from 0 to 3 (“0” = not at all, “1” = several days, “2” = more than half the days, “3” = nearly every day), whereby the severity of depression is measured on the final score aggregated across the questions and range from 0 to 27 points. It is interpreted using these cut-off points: Scoring between 0 and 4 points indicates minimal depression, 5 and 9 points indicates mild depression, 10 and 14 points indicates moderate depression, 15 and 19 points indicates moderately severe depression, and 20 or more points indicates severe depression. The PHQ-9 assessments were voluntary, and users were notified once every two weeks.
Data collection
The app repository was queried for a predefined set of maternal event keywords (Table 3).
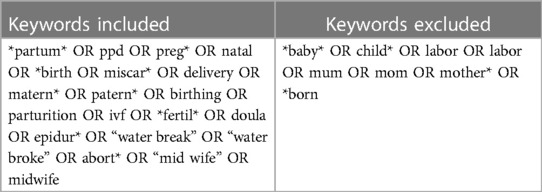
Table 3. The following inclusion and exclusion criteria for maternal event keywords were used to query the app repository for relevant user records event keywords.
Certain keywords were excluded that were considered to induce many false positives within the data (e.g., “born” outside of a maternal-related context, such as “I wish I was never born”). Messages (“user records”) with included keywords were extracted along with the user's app engagement information. The user records were cleared for any inadvertently submitted Personal Identifiable Information (PII) using Wysa's proprietary PII detection and redaction algorithm. The de-identified user records were tagged manually for the maternal event categories. The user records were also tagged for gender: “Female”, or “Male” or “No Tag Available” when gender-related information was not available.
Data analysis
A mixed-methods quantitative and qualitative approach was used to evaluate our two study objectives on efficacy and engagement, respectively.
Effectiveness analysis (objective 1)
The Patient Health Questionnaire (PHQ-9) was used to measure self-reported depressive symptoms at two time points. The sample size was comprised of 51 users who completed the PHQ-9 at least two weeks apart but not more than 5 weeks apart, and scored more than 3 in the PHQ-2 (the first 2 questions of the PHQ-9) (51). The self-reported PHQ-2 cut-off was set at >3 (minimum of 4, maximum of 6). The Self-Reported PHQ-9 was set at greater or equal to 5 (minimum of 5, maximum of 27, overall PHQ-9 score of 27; see Instruments measures section for more context).
Two comparison groups were identified: (1) a higher engaged user group (nh = 28) and (2) a lower engaged user group (nl = 23), based on the number of “session-days” (the days the users engaged with the CA between the two PHQ-9 screening days).
“Engagement density” is a normalized measure calculated for each user defined as the number of active session-days with the CA in-between the two PHQ-9 assessments divided by the available days between the two screenings. Users whose engagement density was ≥0.4 were grouped as “higher engaged group” and those with engagement density <0.4 were grouped as “lower engaged group”. A 40% engagement density translated to 14 active days of AI-enabled CA sessions over a 35-day period.
The average change in depressive symptoms (first PHQ-9 assessment score minus the second PHQ-9 assessment score) was compared between the higher vs. lower engagement groups. A two-tailed, 5% significance Mann–Whitney test was used to test the statistical significance of the difference in average change of symptoms between the two groups. The effect size was measured using the nonparametric common language effect size (CL) (52, 53).
We further examined the data related to objective 1 to assess the clinically meaningful impact from the quantitative results using the categories defined by the PHQ-9 to discuss the clinical implications.
Furthermore, a post hoc analysis was performed to explore changes in the severity thresholds between the two comparison groups across increasing PHQ-9 severity (at ≥10, 15 and 20 intervals). Depressive symptom reductions were measured for statistical significance (M–W test) and effect size (CL) but not considered as actually significant due to the exploratory, post hoc nature and lack of correction for multiple testing.
Engagement analysis (objective 2)
A qualitative analysis examined behaviors exhibited by a subset of higher engaged users with maternal events based on their conversations with Wysa. A Braun and Clarke thematic analysis (54, 55) was performed on free-text responses from users (n = 10) who had an engagement density of greater than 75% based on their conversations (i.e., an anonymized sample of 216 free-text conversational snippets) with the AI CA. The main themes and subthemes, derived from the coding and analysis, helped understand users' expectations, experience and engagement. Prevalence of a theme was measured based on number of instances and number of responding users.
Ethics
Wysa is publicly available as a mobile application (android and iOS). The Conversational Agent (CA) is freely available and has been designed to prioritize safety, privacy and security-by-design. No user registration is required and no Personal Identifiable Information (PII) is asked for during app use. This provides users a private, anonymous space encouraging them to manage their mental well-being in a self-help context (56). All content and tools within the app are reviewed and validated by the Wysa clinical and safety teams. As the study involved analyzing real-world data from an anonymous nonclinical population, it was exempt from registration in a public trial registry (according to OHRP guidelines). Users voluntarily downloaded the app after having consented to the app Terms of Service and Privacy Policy (57, 58). For ethical and privacy reasons, the authors did not have access to all the user messages. Only minimal and limited conversation data at specific chat endpoints were used. The dataset was anonymised by redacting any inadvertent identifiers. User data was adequately secured according to the organization's privacy, security and safety policies. The organization's compliance and privacy officer is author VS who audited the study dataset for safety, privacy and security compliance prior to research use.
Results
Effectiveness analysis (objective 1)
The effectiveness statistical analysis was performed to test for group level differences in depressive symptom scoring between high vs. low engagement group of users. The statistical analysis revealed that the high engaged user group showed a significant depressive symptom reduction compared with the lower engaged user group (p = .004) [Figure 1, also refer to Table 4 for more information including a breakdown of PHQ-9 score averages and standard deviations (SD) for each group pre and post timepoints]. The effect size was high (large) (0.736) (also see Table 4), which is roughly equivalent to a high Cohen d (0.89) (52, 53). There was no statistical difference in average PHQ-9 baseline scores between the higher and lower engaged groups. The high engagement group at baseline had a PHQ-9 minimum of 6 and a maximum of 24 and at follow-up had a PHQ-9 minimum of 6 and a maximum of 27. The low engagement group at baseline had a PHQ-9 minimum of 9 and a maximum of 24 and at follow-up had a PHQ-9 minimum of 9 and a maximum of 27.
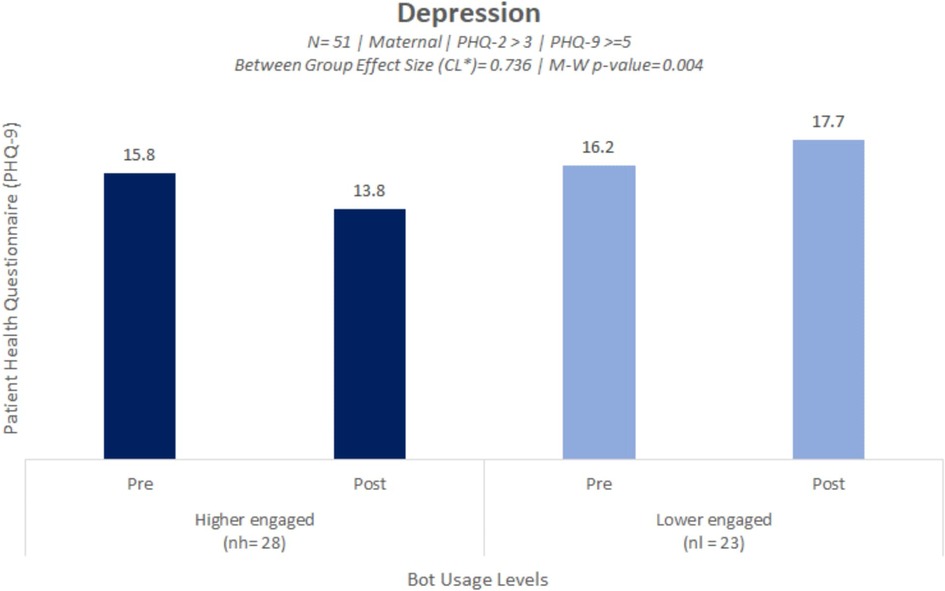
Figure 1. Bar plot illustrating a significant reduction in symptoms of self-reported depressive symptoms amongst the higher engaged user group compared to the lower engaged user group.

Table 4. The high engaged user group showed a significant reduction in depressive symptoms compared with the lower engaged users group (p = 0.004).
The decrease in PHQ-9 score for the higher engagement group was indicative of a shift in clinical improvement from “moderately severe” depression at baseline into the “moderate depression” category at follow-up (refer to Methods Section about PHQ-9 scoring assessment procedures). There was no clinical shift between scoring categories for the lower engagement group and at both time points the lower engagement group average PHQ-9 scores remained within the “moderately severe depression” range.
Post hoc quantitative analysis
As a post hoc analysis, we explored depressive symptom reductions between the groups across increasing PHQ-9 severity. Significant reductions were found among higher engaged users compared to lower engaged users as the severity increased in that the effect was stronger for higher PHQ-9 scoring category thresholds indicating the effect was stronger for more severe depression. Depressive symptom reductions were seen with large effect sizes (0.735–0.883) at PHQ-9 ≥ 10, 15 and 20 intervals as can be seen in Figure 2.
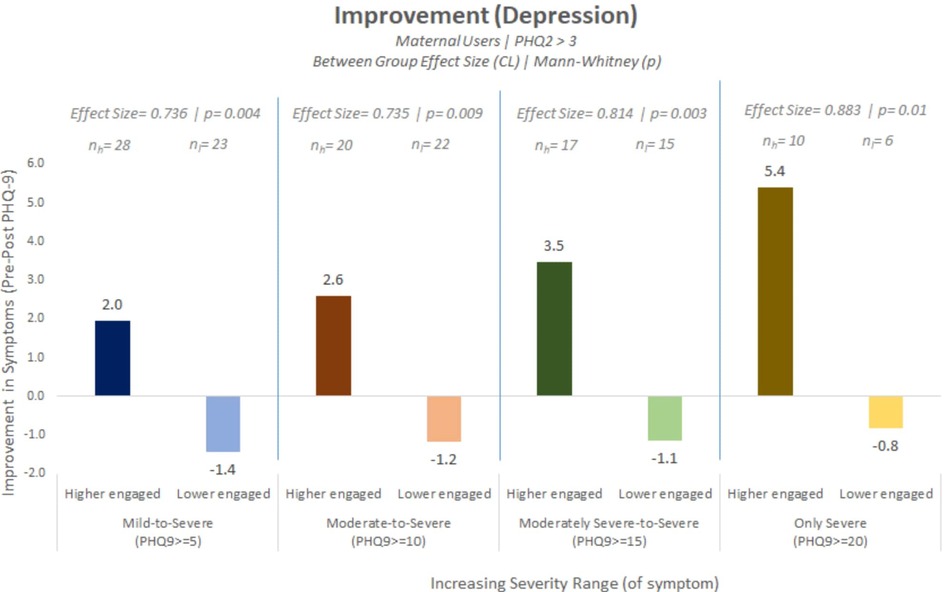
Figure 2. Post hoc analysis showing significant reductions in depressive symptoms among higher engaged users compared to lower engaged users across multiple PHQ-9 severity cut-off thresholds.
Engagement analysis (objective 2)
We report results derived from qualitative insights into the behaviors exhibited among higher engaged maternal event users (n = 10; engagement density <75%) based on their conversations (an anonymized sample of 216 free-text conversational snippets) with the AI CA. Five main behavioral themes emerged: (1) Concern, (2) Support, (3) Reframe, (4) Hope, and (5) Victory. More detailed information about these themes is reported in Table 5. For the thematic maps of themes and sub-themes, and user messages, please refer to Supplementary Multimedia Appendix B. In brief, some users used the app to explore and express their feelings or concerns, or were more critical of themselves or others, or actively and repeatedly used sleep, relaxation and anxiety related tools or techniques to manage their emotional states, or users completed CBT and reframed their negative thoughts as they shared their small victories and gratitudes during their self-created personalized self-help journey. Notably, none of the users sought help from the CA about their maternal health related matters. This was not an identified theme or sub-theme.
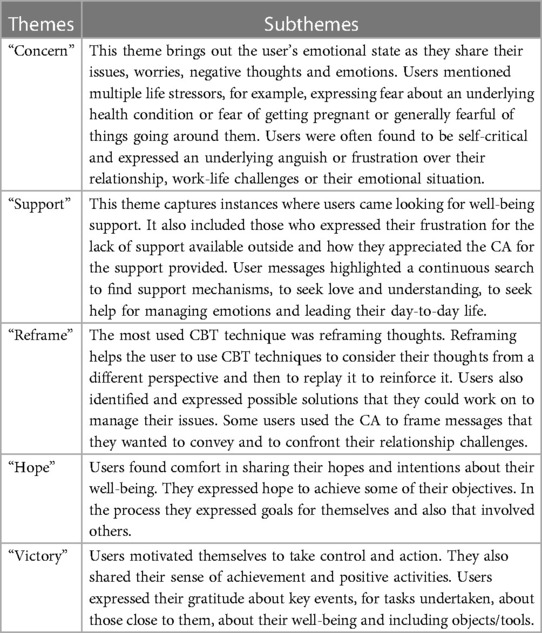
Table 5. Five main behavioral themes emerged from the qualitative analyses of free-text responses from higher engagement AI users.
Additional qualitative observations
These users also provided feedback on sessions within the app with overall sessions seeing 97% of users with high or mid-satisfaction. The user's geographical zone location was derived from the zone related to their smartphone. Participants were mainly located in North America, with a small minority located in Europe. Relationship status was inferred from conversation snippets, which showed that most of the participants (50%) were single and only 10% were married.
Stressors experienced by the users were also identified with key concerns being about relationships (100% of users, included marriage, parents, trauma, break-ups), financial distress (60% of users, included worries about savings and money), work (20% of users, work-life balance, coworker discomfort), life (30% of users, including feelings of loneliness, worthlessness, disinterest), and physical health (10% of users, included chronic illness).
Discussion
Technology's role in supporting mental health and wellbeing is increasingly evidenced. Our study adds to this literature showing how an AI-enabled CA can offer emotional support for maternal mental health and wellbeing. Given evidence that maternal depressive symptoms have increased during the COVID-19 pandemic (59, 60) our pre-covid study is important and requires follow-up to validate our preliminary findings.
Principal findings
This study evaluated the effectiveness of an AI-enabled CA-based mental health and wellbeing app on reducing depressive symptoms for users who reported maternal events. We found a significant reduction in self-reported depressive symptoms among the higher engaged user group compared to the lower engaged user group with a high effect size. This reduction in PHQ-9 score for the higher engaged group was indicative of a shift in clinical improvement from “moderately severe depression” to “moderate depression” at follow-up. A post hoc analysis showed that reductions in depressive symptoms were observed across all PHQ-9 severity score thresholds for the higher engaged group, with an observed increasing effect size as severity of symptoms increased.
This study also performed a qualitative analysis to examine user engagement, which revealed five thematic behaviors: Concern, Support, Reframe, Hope and Victory. Some users used the app to explore and express their feelings or concerns. Some were more critical of themselves or others. Some users actively and repeatedly used sleep, relaxation and anxiety related tools or techniques to manage their emotional states. Some users completed CBT and reframed their negative thoughts as they shared their small victories and gratitudes during their self-created personalized self-help journey.
None of the users asked for support for their maternal event or maternal health matters. Instead, users messaged and engaged with the CA about their emotions and the stressors they were experiencing. This could suggest that users were aware of the intended purpose of the well-being app. The overall in-app feedback indicated comfort of using a digital mental health and wellbeing CA for support.
Comparison with existing literature
We compared our findings with the existing literature focusing on publications that assessed the effectiveness of using CA AI systems for reducing maternal mental health depressive symptoms. Two publications of this type were identified (as this is a nascent research area).
An RCT study (44) was identified that evaluated the effect of an automated CA on postpartum mental health using three questionnaires, including the PHQ-9. The authors reported no significant difference between the “CA intervention group” and the “treatment as usual (TAU) control group” between baseline (after giving birth) and 6 weeks postpartum. That study differed from our study in multiple ways. Our study used a real-world anonymous remote setting approach to enrol users and we included a wide range of maternal events (pre-pregnancy and pre-conception, pregnancy, perinatal, postpartum) whereas the RCT study (44) took place in hospital settings with stringent recruitment eligibility criteria. The studies also differ by control group criteria, study durations, sample size, and differing PHQ-9 cut-off thresholds. The RCT reported low baseline PHQ-9 mean scores (intervention and control group average PHQ-9 scores of 4.6 and 3.3, respectively) and similarly low at 6-week follow-up (intervention and control groups, 3.1 and 3.1, respectively) whereas our study showed much higher group mean PHQ-9 scores (Table 4). It could be possible that differences in study designs, PHQ-9 score severity, and durations of questionnaire assessments are, in part, explaining differences in statistical findings.
Another RCT (45) [which might be closely related to the other RCT (44)] evaluated an automated CA on postpartum mental health using two mental health questionnaires, including the PHQ-9, and reported that the CA intervention group (“WB001+ TAU participants”) had a statistically significant reduction in depressive symptom scores compared to the TAU-only control group between baseline after giving birth and 6 weeks postpartum (45). The RCT reported similarly low PHQ-9 mean scores at baseline and 6-week follow-up (scores below 5). It is not possible to compare our study findings with this publication (45), however, as the methodology used is not described.
To our knowledge these two maternal mental health publications are the only literature currently available for direct comparison of statistical findings on CA AI system effectiveness using the PHQ-9 to assess changes in depressive symptoms. We were unable to compare efficacy with a third study (46) as that pre-pilot study did not include two timepoints for the PHQ-9 for various reasons. For example, the depression screening was too long to administer on a repeating basis, the researchers wanted to avoid frustrating users and distracting them from potential engagement with the intervention (46).
Clinical implications
For the higher engagement group, the statistically significant decrease in PHQ-9 score was indicative of a clinical shift from “moderately severe depression” at baseline into the “moderate depression” category at follow-up. In contrast, for the lower engagement group there was no clinical shift between depressive symptom categories (it remained as “moderately severe depression”). If the higher engagement group used Wysa for a longer duration than two weeks it could be hypothesized that this would further reduce symptomology bringing users “below caseness”, which is defined by NHS IAPT services as being in either “minimal” (PHQ9 score of 0–4) or “mild” (PHQ9 score of 5–9) categories (61).
Our post hoc observations showing a reduction in depressive symptoms across all PHQ-9 severity score thresholds for the higher engaged group supports a prior meta-analysis, which showed that patients with more severe depression at baseline had at least as much clinical benefit from low intensity interventions as less severely depressed patients, inferring that low intensity interventions could be offered to more severe symptom groups (62).
In clinical settings, AI-enabled CA agents could potentially enhance health information systems to capture the right measurements at the right time and offer the right support in a timely manner, which could help to alleviate the burden of data collection by health-care workers in healthcare settings (63). This technology could also potentially support early detection given that many postnatal depression cases are undiagnosed (30). This is important in low-and middle-income countries (given the lack of trained professionals); however, even in countries where trained professionals are more readily available communication gaps in identifying concerns remain an issue (64), which is where an AI-enabled CA agent facilitate better communication.
There is much potential for using CA-based support for maternal mental health and wellbeing, but this will require more in-depth research and must ensure that safety and privacy protects users, especially given ongoing global events related to reproductive justice (65) and the role of technology companies (66). The mental health and wellbeing CA examined in our study prioritizes privacy and security by design and default. Two recent Mozilla Foundation reports found that reproductive health apps (67) and most digital mental health and wellbeing apps (68) investigated were problematic in terms of data protection and privacy with their report showing that Wysa was only 1 of 2 digital mental health and wellbeing apps to pass their privacy investigation (68).
In terms of generalizability, users came from 150 global time zones and both android and iOS versions of the app were used. Furthermore, this study included individuals who self-reported experiencing a wide range of maternal events that had an impact on their mental health and wellbeing. This helps to highlight the importance of offering AI-enabled CA support to wider demographics who need emotional support for different reasons with different needs and preferences (48–50).
This study has several limitations. As RCTs are typically regarded as the “gold standard” for evaluating the efficacy of interventions, a lack of controlled settings could lead to non-handling of biases. Our study has no way of knowing whether demographics were balanced across groups (e.g., diagnoses, other psychological support they might have sought, etc. could impact the analysis). While the PHQ-9 scores are indicative of depressive symptomatology, scores do not confirm or refute the presence of depression. Our study only collected PHQ-9 scores from two time points close together and was not able to follow-up on later outcomes. It did not use other assessments such as EPDS, which could be more appropriate to check concordance and discordance (69). This study used small, unbalanced comparison groups with no use of a treatment-as-usual control group. Users had voluntarily used the app and were likely to have high readiness to explore self-care tools. There may be human bias in labeling data for analysis, such as gender ambiguity, given the app doesn't capture gender for privacy reasons.
Overall, this study demonstrates that an AI-enabled CA-based support can play an important role in reducing depressive symptoms and offering support across diverse maternal events. It adds to a nascent yet growing literature demonstrating the acceptability and comfort of using AI-enabled CA-based digital mental health and wellbeing apps for emotional support.
Data availability statement
The raw data supporting the conclusions of this article will be made available by the authors, without undue reservation.
Ethics statement
Wysa is publicly available as a mobile application (android and iOS). The Conversational Agent (CA) is freely available and has been designed to prioritize safety, privacy and security-by-design. No user registration is required and no Personal Identifiable Information (PII) is asked for during app use. This provides users a private, anonymous space encouraging them to manage their mental well-being in a self-help context (56). All content and tools within the app are reviewed and validated by the Wysa clinical and safety teams. As the study involved analyzing real-world data from an anonymous nonclinical population, it was exempt from registration in a public trial registry (according to OHRP guidelines). Users voluntarily downloaded the app after having consented to the app Terms of Service and Privacy Policy (57, 58). For ethical and privacy reasons, the authors did not have access to all the user messages. Only minimal and limited conversation data at specific chat endpoints were used. The dataset was anonymised by redacting any inadvertent identifiers. User data was adequately secured according to the organization's privacy, security and safety policies. The organization's compliance and privacy officer is author VS who audited the study dataset for safety, privacy and security compliance prior to research use.
Author contributions
BI: conceptualization, methodology, writing—reviewing and editing. MK: investigation, formal analysis, data curation, writing—review & editing. VS: investigation, formal analysis, data curation, writing—review & editing. All authors contributed to the article and approved the submitted version.
Acknowledgments
The authors would like to thank Tanya Malik, Namrata Roa Mangina, Chaitali Sinha, and Madhavi Roy for their comments that greatly supported the development and refinement of this manuscript. We would also like to thank Alina Paik, for her input with regards to the depression severity scoring scale.
Conflict of interest
BI is a scientific advisor to Wysa. VS is the head of compliance at Wysa. MK is the analytic lead at Wysa. Wysa funded the publication fees for the paper.
Publisher's note
All claims expressed in this article are solely those of the authors and do not necessarily represent those of their affiliated organizations, or those of the publisher, the editors and the reviewers. Any product that may be evaluated in this article, or claim that may be made by its manufacturer, is not guaranteed or endorsed by the publisher.
Supplementary material
The Supplementary Material for this article can be found online at: https://www.frontiersin.org/articles/10.3389/fgwh.2023.1084302/full#supplementary-material.
References
1. Underwood L, Waldie K, D’Souza S, Peterson ER, Morton S. A review of longitudinal studies on antenatal and postnatal depression. Arch Womens Ment Health. (2016) 19(5):711–20. doi: 10.1007/s00737-016-0629-1
2. Burns LH. An exploratory study of perceptions of parenting after infertility. Fam Syst Med. (1990) 8(2):177–89. doi: 10.1037/h0089398
3. Siegel RS, Brandon AR. Adolescents, pregnancy, and mental health. J Pediatr Adolesc Gynecol. (2014) 27(3):138–50. doi: 10.1016/j.jpag.2013.09.008
4. Zia Y, Mugo N, Ngure K, Odoyo J, Casmir E, Ayiera E, et al. Psychosocial experiences of adolescent girls and young women subsequent to an abortion in sub-saharan Africa and globally: a systematic review. Front Reprod. (2021) 3:638013. doi: 10.3389/frph.2021.638013
5. Farren J, Mitchell-Jones N, Verbakel JY, Timmerman D, Jalmbrant M, Bourne T. The psychological impact of early pregnancy loss. Hum Reprod. (2018) 24(6):731–49. doi: 10.1093/humupd/dmy025
6. American Psychological Association. Leis-Newman E. Miscarriage and loss (2012). Available at: https://www.apa.org/monitor/2012/06/miscarriage (Accessed April 18, 2023).
7. Dadi AF, Miller ER, Bisetegn TA, Mwanri L. Global burden of antenatal depression and its association with adverse birth outcomes: an umbrella review. BMC Public Health. (2020) 20(1):173. doi: 10.1186/s12889-020-8293-9
8. Ogbo FA, Eastwood J, Hendry A, Jalaludin B, Agho KE, Barnett B, et al. Determinants of antenatal depression and postnatal depression in Australia. BMC Psychiatry. (2018) 18(1):49. doi: 10.1186/s12888-018-1598-x
9. Lambrenos K, Weindling AM, Calam R, Cox AD. The effect of a child’s disability on mother’s mental health. Arch Dis Child. (1996) 74(2):115–20. doi: 10.1136/adc.74.2.115
10. Slomian J, Honvo G, Emonts P, Reginster JY, Bruyère O. Consequences of maternal postpartum depression: a systematic review of maternal and infant outcomes. Womens Health. (2019) 15:1745506519844044. doi: 10.1177/1745506519844044
11. Howard LM, Khalifeh H. Perinatal mental health: a review of progress and challenges. World Psychiatry. (2020) 19(3):313–27. doi: 10.1002/wps.20769
12. Bauer A, Parsonage M, Knapp M, Iemmi V, Adelaja B. The costs of perinatal mental health problems. London: Centre for Mental (2014). doi: 10.13140/2.1.4731.6169
13. American Pregnancy Association. Baby blues (2019). Available at: https://americanpregnancy.org/healthy-pregnancy/first-year-of-life/baby-blues/ (Accessed April 18, 2023).
14. Earls MF. Committee on psychosocial aspects of child and family health American academy of pediatrics. Incorporating recognition and management of perinatal and postpartum depression into pediatric practice. Pediatrics. (2010) 126(5):1032–9. doi: 10.1542/peds.2010-2348
15. Earls MF, Yogman MW, Mattson G, Rafferty J. Committee on psychosocial aspects of child and family health. Incorporating recognition and management of perinatal depression into pediatric practice. Pediatrics. (2019) 143(1):e20183260. doi: 10.1542/peds.2018-3259
16. Jarde A, Morais M, Kingston D, Giallo R, MacQueen GM, Giglia L, et al. Neonatal outcomes in women with untreated antenatal depression compared with women without depression: a systematic review and meta-analysis. JAMA Psychiatry. (2016) 73(8):826–37. doi: 10.1001/jamapsychiatry.2016.0934
17. Goodman JH. Perinatal depression and infant mental health. Arch Psychiatr Nurs. (2019) 33(3):217–24. doi: 10.1016/j.apnu.2019.01.010
18. Lewis AJ, Austin E, Galbally M. Prenatal maternal mental health and fetal growth restriction: a systematic review. J Dev Orig Health Dis. (2016) 7(4):416–28. doi: 10.1017/S2040174416000076
19. Atif N, Lovell K, Rahman A. Maternal mental health: the missing “m” in the global maternal and child health agenda. Semin Perinatol. (2015) 39(5):345–52. doi: 10.1053/j.semperi.2015.06.007
20. World Health Organization & United Nations Population Fund. Mental health aspects of women’s reproductive health: A global review of the literature. Geneva: World Health Organization (2009). 168 p.
21. McNab S, Fisher J, Honikman S, Muvhu L, Levine R, Chorwe-Sungani G, et al. Comment: silent burden no more: a global call to action to prioritize perinatal mental health. BMC Pregnancy Childbirth. (2022) 22(1):308. doi: 10.1186/s12884-022-04645-8
22. Bauer A, Knapp M, Parsonage M. Lifetime costs of perinatal anxiety and depression. J Affect Disord. (2016) 192:83–90. doi: 10.1016/j.jad.2015.12.005
23. Webb R, Uddin N, Ford E, Easter A, Shakespeare J, Roberts N, et al. Barriers and facilitators to implementing perinatal mental health care in health and social care settings: a systematic review. Lancet Psychiatry. (2021) 8(6):521–34. doi: 10.1016/S2215-0366(20)30467-3
24. Novick AM, Kwitowski M, Dempsey J, Cooke DL, Dempsey AG. Technology-based approaches for supporting perinatal mental health. Curr Psychiatry Rep. (2022) 24:419–29. doi: 10.1007/s11920-022-01349-w
25. van den Heuvel JF, Groenhof TK, Veerbeek JH, van Solinge WW, Lely AT, Franx A, et al. Ehealth as the next-generation perinatal care: an overview of the literature. J Med Internet Res. (2018) 20(6):e202. doi: 10.2196/jmir.9262
26. Heller HM, Hoogendoorn AW, Honig A, Broekman BFP, van Straten A. The effectiveness of a guided internet-based tool for the treatment of depression and anxiety in pregnancy (MamaKits online): randomized controlled trial. J Med Internet Res. (2020) 22(3):e15172. doi: 10.2196/15172
27. Haga SM, Drozd F, Lisøy C, Wentzel-Larsen T, Slinning K. Mamma mia—a randomized controlled trial of an internet-based intervention for perinatal depression. Psychol Med. (2019) 49(11):1850–8. doi: 10.1017/S0033291718002544
28. Shin DC. Development and application of an in-house health care program to improve the physical and mental health of working mothers: a pilot study. Health Care Women Int. (2020) 41(3):284–92. doi: 10.1080/07399332.2019.1621868
29. Dennis CL, Chung-Lee L. Postpartum depression help-seeking barriers and maternal treatment preferences: a qualitative systematic review. Birth. (2006) 33(4):323–31. doi: 10.1111/j.1523-536X.2006.00130.x
30. Doherty K, Barry M, Marcano-Belisario J, Arnaud B, Morrison C, Car J, et al. A mobile app for the self-report of psychological well-being during pregnancy (BrightSelf): qualitative design study. JMIR Ment Health. (2018) 5(4):e10007. doi: 10.2196/10007
31. Maloni JA, Przeworski A, Damato EG. Web recruitment and internet use and preferences reported by women with postpartum depression after pregnancy complications. Arch Psychiatr Nurs. (2013) 27(2):90–5. doi: 10.1016/j.apnu.2012.12.001
32. Loughnan SA, Butler C, Sie AA, Grierson AB, Chen AZ, Hobbs MJ, et al. A randomized controlled trial of “MUMentum postnatal”: internet-delivered cognitive behavioural therapy for anxiety and depression in postpartum women. Behav Res Ther. (2019) 116:94–103. doi: 10.1016/j.brat.2019.03.001
33. Forsell E, Bendix M, Holländare F, Szymanska von Schultz B, Nasiell J, Blomdahl-Wetterholm M, et al. Internet delivered cognitive behavior therapy for antenatal depression: a randomized controlled trial. J Affect Disord. (2017) 221:56–64. doi: 10.1016/j.jad.2017.06.013
34. Clifton J, Parent J, Seehuus M, Worrall G, Forehand R, Domar A. An internet-based mind/body intervention to mitigate distress in women experiencing infertility: a randomized pilot trial. PLoS One. (2020) 15(3):e0229379. doi: 10.1371/journal.pone.0229379
35. Kim DR, Hantsoo L, Thase ME, Sammel M, Epperson CN. Computer-assisted cognitive behavioral therapy for pregnant women with major depressive disorder. J Womens Health. (2014) 23(10):842–8. doi: 10.1089/jwh.2014.4867
36. Lee EW, Denison FC, Hor K, Reynolds RM. Web-based interventions for prevention and treatment of perinatal mood disorders: a systematic review. BMC Pregnancy Childbirth. (2016) 16:1–8. doi: 10.1186/s12884-016-0831-1
37. Feldman N, Perret S. Digital mental health for postpartum women: perils, pitfalls, and promise. NPJ DigitMed. (2023) 6(1):11. doi: 10.1038/s41746-023-00756-4
38. Moorhead A, Bond R, Mulvenna M, O’Neill S, Murphy N. A self-management app for maternal mental health. Proceedings of the 32nd International BCS Human Computer Interaction Conference (HCI) (2018) Human Computer Interaction Conference; 2018 Jul 4–6.
39. Verduci E, Vizzuso S, Frassinetti A, Mariotti L, Del Torto A, Fiore G, et al. Nutripedia: the fight against the fake news in nutrition during pregnancy and early life. Nutrients. (2021) 13(9):2998. doi: 10.3390/nu13092998
40. Chung K, Cho HY, Park JY. A chatbot for perinatal women’s and partners’ obstetric and mental health care: development and usability evaluation study. JMIR Med Inform. (2021) 9(3):e18607. doi: 10.2196/18607
41. de Barreto ICHC, Barros NBS, Theophilo RL, Viana VF, de Silveira FRV, de Souza O, et al. Development and evaluation of the GISSA mother-baby ChatBot application in promoting child health. Cien Saude Colet. (2021) 26(5):1679–90. doi: 10.1590/1413-81232021265.04072021
42. Delanerolle G, Yang X, Shetty S, Raymont V, Shetty A, Phiri P, et al. Artificial intelligence: a rapid case for advancement in the personalization of gynaecology/ obstetric and mental health care. Women’s Health. (2021) 17:1–20. doi: 10.1177/17455065211018111
43. Ramachandran M, Suharwardy S, Leonard SA, Gunaseelan A, Robinson A, Darcy A, et al. Acceptability of postnatal mood management through a smartphone-based automated conversational agent. Am J Obstet Gynecol. (2020) 74:S62. doi: 10.1016/j.ajog.2019.11.090
44. Suharwardy S, Ramachandran M, Leonard SA, Gunaseelan A, Robinson A, Darcy A, et al. 116: effect of an automated conversational agent on postpartum mental health: a randomized, controlled trial. Am J Obstet Gynecol. (2020) 222(1):S91. doi: 10.1016/j.ajog.2019.11.132
45. Darcy A, Beaudette A, Chiauzzi E, Daniels J, Goodwin K, Mariano TY, et al. Anatomy of a woebot® (WB001): agent guided CBT for women with postpartum depression. Expert Rev Med Devices. (2022) 19(4):287–301. doi: 10.1080/17434440.2022.2075726
46. Green EP, Pearson N, Rajasekharan S, Rauws M, Joerin A, Kwobah E, et al. Expanding access to depression treatment in Kenya through automated psychological support: protocol for a single-case experimental design pilot study. JMIR Res Protoc. (2019) 8(4):e11800. doi: 10.2196/11800
47. Wang R, Wang J, Liao Y, Wang J. Supervised machine learning chatbots for perinatal mental healthcare. International Conference on Intelligent Computing and Human-Computer Interaction (ICHCI); Sanya, China (2020). p. 378–83. doi: 10.1109/ICHCI51889.2020.00086
48. Inkster B, Sarda S, Subramanian V. An empathy-driven, conversational artificial intelligence agent (wysa) for digital mental well-being: real-world data evaluation mixed-methods study. JMIR Mhealth Uhealth. (2018) 6(11):e12106. doi: 10.2196/12106
49. Leo AJ, Schuelke MJ, Hunt DM, Metzler JP, Miller JP, Areán PA, et al. Digital mental health intervention for orthopedic patients with symptoms of depression and/or anxiety: pilot feasibility study. JMIR Form Res. (2022) 6(2):e34889. doi: 10.2196/34889
50. Leo AJ, Schuelke MJ, Hunt DM, Miller JP, Areán PA, Cheng AL. Digital mental health intervention plus usual care compared with usual care only and usual care plus in-person psychological counseling for orthopedic patients with symptoms of depression or anxiety: cohort study. JMIR Form Res. (2022) 6(5):e36203. doi: 10.2196/36203
51. Mitchell AJ, Yadegarfar M, Gill J, Stubbs B. Case finding and screening clinical utility of the patient health questionnaire (PHQ-9 and PHQ-2) for depression in primary care: a diagnostic meta-analysis of 40 studies. BJPsych Open. (2016) 2(2):127–38. doi: 10.1192/bjpo.bp.115.001685
52. Ruscio J. A probability-based measure of effect size: robustness to base rates and other factors. Psychol Methods. (2008) 13(1):19–30. doi: 10.1037/1082-989X.13.1.19
53. Rice ME, Harris GT. Comparing effect sizes in follow-up studies: ROC area, Cohen’s d, and r. Law Hum Behav. (2005) 29(5):615–20. doi: 10.1007/s10979-005-6832-7
54. Braun V, Clarke V. Using thematic analysis in psychology. Qual Res Psychol. (2006) 3(2):77–101. doi: 10.1191/1478088706qp063oa
55. Malik T, Ambrose AJ, Sinha C. Evaluating user feedback for an artificial intelligence-enabled, cognitive behavioral therapy-based mental health app (wysa): qualitative thematic analysis. JMIR Hum Factors. (2022) 9(2):e35668. doi: 10.2196/35668
56. Wysa. Available at: https://www.wysa.com (Accessed April 18, 2023).
57. Wysa. Terms of service. Available at: https://legal.wysa.io/terms (Accessed April 18, 2023).
58. Wysa. Privacy policy. Available at: https://legal.wysa.io/privacy-policy (Accessed April 18, 2023).
59. Myers S, Emmott EH. Communication across maternal social networks during England’s first national lockdown and its association with postnatal depressive symptoms. Front Psychol. (2021) 12:648002. doi: 10.3389/fpsyg.2021.648002
60. Davenport MH, Meyer S, Meah VL, Strynadka MC, Khurana R. Moms are not OK: COVID-19 and maternal mental health. Front Glob Womens Health. (2020) 1:1. doi: 10.3389/fgwh.2020.00001
61. The improving access to psychological therapies manual appendices and helpful resources. Prepared by the National Collaborating Centre for Mental Health. Gateway reference: 07534 Version number: 2 Updated: December 2019; First published: June 2018. Available at: https://www.rcpsych.ac.uk/docs/default-source/improving-care/nccmh/iapt/nccmh-iapt-manual-appendices-helpful-resources-v2.pdf?sfvrsn=a607ef5_4 (Accessed April 18, 2023).
62. Bower P, Kontopantelis E, Sutton A, Kendrick T, Richards DA, Gilbody S, et al. Influence of initial severity of depression on effectiveness of low intensity interventions: meta-analysis of individual patient data. Br Med J. (2013) 346:f540. doi: 10.1136/bmj.f540
63. Brizuela V, Tunçalp Ö. A road to optimizing maternal and newborn quality care measurement for all. Lancet Glob Health. (2021) 9(3):e221–2. doi: 10.1016/S2214-109X(20)30519-2
64. Boots Family Trust Alliance London. Perinatal mental health: experiences of women and Health Professionals (2013). Available at: https://maternalmentalhealthalliance.org/wp-content/uploads/Boots-Family-Trust-Alliance-report.pdf (Accessed April 18, 2023).
65. Shachar C. HIPAA, privacy, and reproductive rights in a post-roe era. JAMA. (2022) 328(5):417–8. doi: 10.1001/jama.2022.12510
66. Bloomberg. Google maps regularly misleads people searching for abortion clinics (2022) Available at: https://www.bloomberg.com/graphics/2022-google-search-abortion-clinic-crisis-pregnancy-center/ (Accessed April 18, 2023).
67. Mozilla Foundation. Privacy not included: a buyer’s guide for connected products. Available at: https://foundation.mozilla.org/en/privacynotincluded/categories/reproductive-health/ (Accessed April 18, 2023).
68. Mozilla Foundation. Top mental health and prayer apps fail spectacularly at privacy, security (2022). Available at: https://foundation.mozilla.org/en/blog/top-mental-health-and-prayer-apps-fail-spectacularly-at-privacy-security/ (Accessed April 18, 2023).
69. Cox J, Holden J. Perinatal mental health: A guide to the Edinburgh postnatal depression scale (EPDS). Washington: Royal College of Psychiatrists (2003). https://psycnet.apa.org/record/2004-14522-000 (Accessed April 18, 2023).
Keywords: maternal mental health and wellbeing, artificial intelligence, psychotherapy, depression, conversational agent (CA), chatbot
Citation: Inkster B, Kadaba M and Subramanian V (2023) Understanding the impact of an AI-enabled conversational agent mobile app on users’ mental health and wellbeing with a self-reported maternal event: a mixed method real-world data mHealth study. Front. Glob. Womens Health 4:1084302. doi: 10.3389/fgwh.2023.1084302
Received: 30 October 2022; Accepted: 12 May 2023;
Published: 2 June 2023.
Edited by:
Farhad Fatehi, The University of Queensland, AustraliaReviewed by:
Saeideh Valizadeh-Haghi, Shahid Beheshti University of Medical Sciences, IranHamed Mehdizadeh, Mazandaran University of Medical Sciences, Iran
© 2023 Inkster, Kadaba and Subramanian. This is an open-access article distributed under the terms of the Creative Commons Attribution License (CC BY). The use, distribution or reproduction in other forums is permitted, provided the original author(s) and the copyright owner(s) are credited and that the original publication in this journal is cited, in accordance with accepted academic practice. No use, distribution or reproduction is permitted which does not comply with these terms.
*Correspondence: Becky Inkster becky@beckyinkster.com