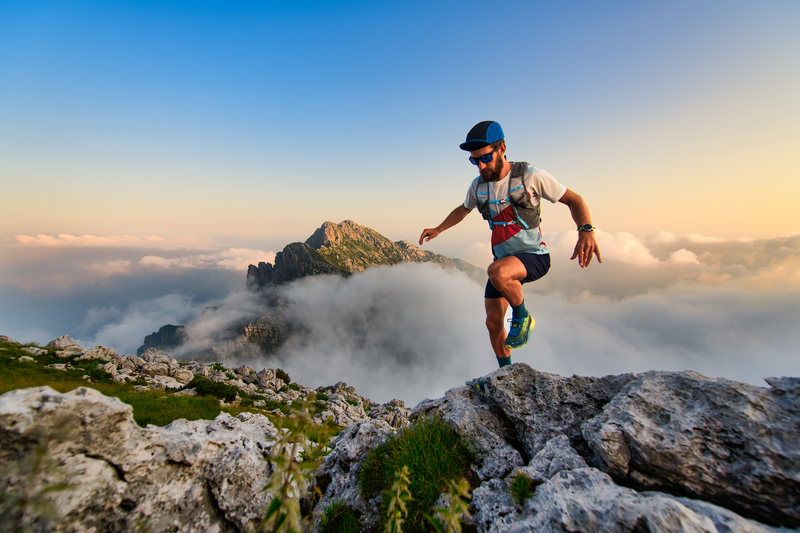
95% of researchers rate our articles as excellent or good
Learn more about the work of our research integrity team to safeguard the quality of each article we publish.
Find out more
ORIGINAL RESEARCH article
Front. Genet. , 17 March 2025
Sec. Computational Genomics
Volume 16 - 2025 | https://doi.org/10.3389/fgene.2025.1449466
This article is part of the Research Topic The role of genes and network pharmacology in new drug discovery View all 5 articles
Introduction: Lung cancer (LC) is a highly aggressive malignancy and remains a leading cause of cancer-related mortality worldwide. Non-small cell lung cancer (NSCLC), which includes adenocarcinoma (LUAD) and squamous cell carcinoma (LUSC), accounts for the majority of these deaths. Due to the lack of early clinical symptoms and late-stage diagnosis, there is an urgent need for precise and targeted therapeutic strategies. Cyclin-dependent kinase regulatory subunit 1B (CKS1B), a key regulator of the cell cycle, has been implicated in various human cancers. Emerging evidence suggests that its upregulation is associated with poor prognosis in NSCLC, highlighting its potential as a biomarker for early detection and targeted therapy.
Methods: In this study, we conducted a comprehensive bioinformatics analysis to evaluate the role of CKS1B in LUAD and LUSC. Differential gene expression analysis, survival analysis, immune infiltration correlation, and pathway enrichment analysis were performed using publicly available transcriptomic datasets. Additionally, gene interaction networks were analyzed to assess the functional significance of CKS1B in lung cancer progression.
Results: Our findings indicate a significant overexpression of CKS1B in LUAD and LUSC compared to normal lung tissues. Survival analysis demonstrated that higher CKS1B expression correlates with poor prognosis in NSCLC patients. Immune infiltration analysis revealed a potential role of CKS1B in modulating the tumor microenvironment, further supporting its relevance in lung cancer progression. Functional enrichment analysis highlighted its involvement in critical oncogenic pathways, including cell cycle regulation and immune modulation.
Discussion: The results suggest that CKS1B serves as a potential biomarker for early detection and prognosis in NSCLC. Its association with immune response pathways underscores its possible role in immunotherapy. However, despite these promising findings, further in vivo and in vitro studies are necessary to validate CKS1B's clinical applicability as a diagnostic and therapeutic target for lung cancer.
Cancer represents a complex group of diseases characterized by the uncontrolled proliferation of abnormal cells, often followed by their spread to surrounding tissues (What Is Cancer, 2021). In 2020, the global cancer burden was estimated at 19.3 million new cases and nearly 10 million deaths (Sung et al., 2021). Lung cancer (LC) remains a leading cause of cancer-related mortality, accounting for approximately 18.7% of cancer deaths and 12.4% of new cases annually. Non-small cell lung cancer (NSCLC) dominates LC cases, comprising about 80%–85%, with its two major histologic subtypes, lung adenocarcinoma (LUAD) and lung squamous cell carcinoma (LUSC), representing 40% and 30% of new LC cases, respectively (What Is Lung Cancer, 2021; Bender, 2014).
One of the significant challenges in treating NSCLC lies in its detection at advanced stages (National Lung Screening Trial Research Team, 2019). Early-stage patients often present no clear clinical symptoms, and the lack of effective early detection methods exacerbates the problem (Foss et al., 2011). Recent data highlights this issue, with a notable increase in Stage IV NSCLC diagnoses from 43.3% in 2013 to 49.3% in 2023 (Herms et al., 2024). Furthermore, the overall 5-year survival rate for LC remains at 20%, significantly lower than for most other cancers (Wong et al., 2017; Allemani et al., 2018; Bray et al., 2024). While advancements in therapies have improved outcomes for some, the 5-year survival rate for advanced NSCLC is still less than 15%, while early-stage NSCLC patients experience survival rates as high as 80% (Jemal et al., 2011). These figures emphasize the crucial need for early detection and accurate prognostic markers to improve survival outcomes.
In recent years, an increasing focus has been placed on identifying differentially expressed genes (DEGs) as potential biomarkers for early detection and prognosis of NSCLC subtypes such as LUAD and LUSC (Yu and Tian, 2020a). Tumor development involves a cascade of events including uncontrolled cell growth, genome instability, invasion of nearby tissues, and metastasis, which ultimately result in limited treatment options and poorer outcomes for patients (Shi et al., 2020a). The identification of reliable biomarkers can help in developing targeted therapies that selectively kill tumor cells while sparing healthy tissues, offering hope for improved treatment strategies.
Cyclin-dependent kinase regulatory subunit 1B (CKS1B) has emerged as a key player in cell cycle regulation and cancer progression. A member of the conserved cyclin kinase subunit 1 (CKS1) protein family, CKS1B regulates the eukaryotic mitotic cycle and plays a vital role in normal cell division and growth (Krishnan et al., 2009). Its protein, encoded by the CKS1B gene on chromosome 1q21, has a molecular weight of 9 kDa and consists of 79 amino acids. Dysregulation of CKS1B is associated with abnormal cell cycle progression, which can contribute to the onset and progression of various malignancies. The CKS1B protein interacts with cyclin-dependent kinases (CDKs) and their inhibitors, influencing key processes such as cell proliferation, invasion, metastasis, and chemoresistance (Krishnan et al., 2009; Stella et al., 2014; Zeng et al., 2019; Lee et al., 2011; Zhan et al., 2007).
CKS1B overexpression has been documented in several cancers, including hepatocellular carcinoma (Lee et al., 2011), colon cancer (Wang et al., 2010), lung cancer (Zolota et al., 2010), oral squamous cell carcinoma (Kitajima et al., 2004), breast cancer (Slotky et al., 2005), and retinoblastoma (Zeng et al., 2019). Additionally, its role as one of the 70 high-risk genes associated with poor prognosis in multiple myeloma (MM) has been established (Shaughnessy et al., 2006; Chen et al., 2012). These findings suggest that CKS1B could serve as a valuable therapeutic target across various malignancies (Huang et al., 2015; Hwang et al., 2019).
Despite the widespread occurrence of LUAD and LUSC, limited biomarkers exist for early detection, and current treatment options often result in suboptimal survival rates. Prognostic markers can help guide treatment strategies by identifying key tumor characteristics, but many biomarkers still lack the necessary sensitivity and specificity for routine clinical use in lung cancer detection (Lemjabbar-Alaoui et al., 2015). Given the crucial role of CKS1B in cell cycle regulation and its association with poor prognosis in several cancers, we hypothesized that CKS1B expression may serve as a prognostic biomarker for the early diagnosis and treatment of LUAD and LUSC. In this study, we explored the oncogenic role of CKS1B in NSCLC subtypes through a bioinformatics approach. Our findings revealed a significant correlation between elevated CKS1B expression and poor survival outcomes in LUAD and LUSC, suggesting its potential as a valuable biomarker for early detection and prognosis. The study also highlights the possibility of targeting CKS1B for novel therapeutic strategies aimed at improving patient outcomes. Further in vivo and in vitro investigations are warranted to validate its clinical utility and explore its role in the regulation of key signaling pathways involved in lung cancer progression.
Three different databases are entitled Oncomine (https://www.oncomine.org) (Rhodes et al., 2004), GEPIA2 (http://gepia2.cancer-pku.cn) (Tang et al., 2019), and GENT2 (http://gent2.appex.kr) (Park et al., 2019) were used to analyze the expression of CKS1B expression in multiple cancerous tissues and their normal, non-cancerous counterparts. The Oncomine database contains 715 independent datasets that store experimental information of 86,733 cancer samples and 12,764 standardized tissue samples. This server is well-known for its high reliability, accuracy, consistency, and scalability (Rhodes et al., 2004). This platform was employed to assess gene expression data from curated datasets. In the Oncomine database, the default parameters i.e., p-value threshold of 1E-4, fold change threshold of 2.0, and Top 10% Gene rank, were set. GEPIA2 (Gene Expression Profiling Interactive Analysis) database is widely utilized to analyze the mRNA expression profiling of a variety of genes. The server houses the information of 9,736 cancerous and 8,587 normal tissue samples, extracted from the TCGA (The Cancer Genome Atlas) and GTEx (Genotype-Tissue Expression) projects (Tang et al., 2019). This server integrates TGCA and GTEx data for differential expression and survival analysis. During analysis by the GEPIA2 server, all the parameters were kept at their default values. Along with the above-mentioned servers, GENT2 (Gene Expression database of Normal and Tumor tissues-2) was also used to analyze the expression of the CKS1B gene in more than 68,000 tumor and normal tissue samples stored in its database. This server generates reliable and quite accurate outcomes by exploiting the Apache Lucene indexing and Google Web Toolkit (GWT) framework (Park et al., 2019). It is used to analyze gene expression profiles. Finally, the expression of the CKS1B gene was observed for both LUAD and LUSC, according to the TIMER analysis (http://timer.cistrome.org/).
Four different web servers, namely, Oncomine (Rhodes et al., 2004), GEPIA2 (Tang et al., 2019), UALCAN (http://ualcan.path.uab.edu/) (Chandrashekar et al., 2017), and HPA (https://www.proteinatlas.org/) (Uhlén et al., 2015) were used to determine the expression profile of CKS1B mRNA in normal and cancerous LUAD and LUSC tissues. UALCAN web-server allows the users to detect novel cancer biomarkers and carry out numerous in silico analyses to map the expression of target genes by providing free access to the cancer OMICS datasets like the TCGA database (Chandrashekar et al., 2017). The relative expression pattern of CKS1B mRNA in both LUAD and LUSC samples was also analyzed using the TCGA database. The HPA (Human Protein Atlas) database was used to make a visual comparison between LUAD and LUSC tissues and normal lung tissues through immunohistochemistry. This project aims to map all human proteins in cells, tissues, and organs by combining various omics technologies such as mass spectrometry-based proteomics, transcriptomics, antibody-based imaging, and so on (Uhlén et al., 2015). The default parameters for all these servers were used and the p-value less than 0.05 was considered significant. This analysis enabled a direct comparison of staining intensities between cancerous and non-cancerous tissues.
The UALCAN server was used to determine the correlation of CKS1B overexpression with clinical features (Chandrashekar et al., 2017). Individual cancer stages, patient’s race, patient’s gender, patient’s age, patient’s smoking habit, tumor histology, nodal metastasis status, and TP53 mutation status are the clinical features that were analyzed in the server keeping all the parameters default. After that, the UCSC Xena Functional Genomic Explorer (https://xenabrowser.net/) was used to find out the DNA methylation pattern of CKS1B gene promoter associated with LUAD and LUSC (Goldman et al., 2020). The TCGA server analyzed experimental data of 706 LUAD and 626 LUSC samples, and the DNA Methylation of 27K and 450K patterns of CKS1B for LUAD and LUSC respectively. Statistical significance (p-value <0.05) was determined for each correlation.
The pattern of mutations and copy number alterations of the CKS1B gene in association with LUAD and LUSC was determined using the cBioPortal server (https://www.cbioportal.org/; designed to explore, visualize, and interpret multidimensional cancer genomics data) (Cerami et al., 2012). This platform was utilized to investigate DNA methylation levels in the promoter region of CKS1B using data from Illumina Human Methylation 450K and 27K arrays. Differential methylation patterns between LUAD and LUSC were assessed to identify potential epigenetic mechanisms influencing gene expression. In this study, this platform provided an overview of mutations and copy number variations (CNVs) in CKS1B across 2,983 LUAD and 1,176 LUSC samples. We categorized these alterations by frequency and type, including missense mutations, amplifications, and deep deletions, to assess their potential contributions to lung cancer pathogenesis. The mutation spectrum of the CKS1B gene responsible for cancer development was determined by the cBioPortal server, keeping all the parameters as default.
PrognoScan server (http://dna00.bio.kyutech.ac.jp/PrognoScan/) was exploited to determine the relationship of the survival of patients with LUAD and LUSC and CKS1B gene expression. PrognoScan is a database for meta-analysis of genes that scans the freely available microarray datasets to predict the interaction between the expression of a query gene and the prognosis of cancer (Mizuno et al., 2009). The server uses the Kaplan-Meier statistical method to plot the gene expression in the X-axis and the possibility of patient survival in the Y-axis. During the analysis, default parameters were used and any Cox p-value less than 0.05 was considered to be statistically significant. Datasets with significant associations (Cox p-value <0.05) were included to determine the relationship between CKS1B expression and overall survival (OS) and relapse-free survival (RFS).
Three different servers i.e., Oncomine (Rhodes et al., 2004), GEPIA2 (Tang et al., 2019), and UCSC Xena web browser (Goldman et al., 2020) were used to determine the genes which tend to co-express with CKS1B. Firstly, the Oncomine server was used to search the list of co-expressed genes. The server ranks the co-expressed genes based on correlation scores. Thereafter, the analysis of the relationship between the CKS1B gene and the gene that generated the highest correlation score in the Oncomine server was carried out using the GEPIA2 server. Finally, the UCSC Xena web browser was utilized to design the gene expression pattern of the selected genes in LUAD and LUSC patients.
GeneMANIA (https://genemania.org) is a web-based platform that uses a large database of functional interaction data to assess the relationship between a gene of interest and other genes. Centered on protein and genetic interactions, pathways, co-expression, co-localization, and protein domain similarity, this platform was used to construct an interaction network of CKS1B and related gene. In addition, using the International Molecular Exchange Consortium (IMEx) protein interactions database, the previously reported 20 associated genes and CKS1B was used in NetworkAnalyst (https://www.networkanalyst.ca/) to establish the protein-protein interaction at a generic level. The RTK/Ras pathway, P13K/AKT pathway, MAPK, and Rap1 signaling pathway were found to involve CKS1B in the KEGG 2019 database. Following that, PathwayMapper in the cBioPortal server was used to analyze these pathways in detail as well as to estimate the frequency of CKS1B alterations.
The Enrichr server (http://amp.pharm.mssm.edu/Enrichr/) was used for gene ontology (GO) and cell signaling pathways of CKS1B. The server generates the annotation enrichment results of a target gene set by comparing multiple genomics datasets containing previous biological knowledge (Chen et al., 2013; Kuleshov et al., 2016). To do the analysis, the CKS1B along with the other co-expressed genes found in the Oncomine server was used. The GO terms (i.e., GO biological process, GO molecular function, and GO cellular component) and the signaling pathways (from BioPlanet 2019; KEGG 2019 Human, and Reactome 2016 databases) were analyzed using the Enrichr web server. Validation was performed across multiple databases to enhance reproducibility and reliability.
CKS1B is substantially upregulated in various cancer cells, including LC, according to 56 studies out of 452 retrieved datasets (Figure 1A). GEPIA2 results also indicate a similar pattern of CKS1B upregulation in 33 different types of cancer cells including LUAD and LUSC (Figure 1B). CKS1B is overexpressed in various cancers, including LUAD and LUSC, according to a review of GENT2 databases using HG-U133pLUS 2 and HG-U133A platforms (Figure 1Ci, Cii). In addition, the TIMER analysis revealed that CKS1B was overexpressed in both LUAD and LUSC as compared to other cancer forms (Figure 1D). Overall, the findings showed that the CKS1B gene is significantly upregulated in LC tissues as compared to normal tissues and other cancer cells (Figure 1).
Figure 1. CKS1B tissue-wide expression profile across multiple cancer types in humans. (A) Disease summary heatmap displaying differential expression of CKS1B across various cancers. Red denotes higher expression in cancer tissues compared to normal tissues, while blue indicates lower expression. (B) Dot plot illustrating the gene expression profile of CKS1B in 33 human cancers, including both tumor and healthy tissue samples. (C) Box plots representing CKS1B mRNA expression in cancerous and corresponding healthy tissues, using the Affymetrix HG-U133Plus 2 (Ci) and HG-U133A (Cii) platforms from the GENT2 database. Boxes show the median, dots represent outliers, and red boxes indicate cancerous tissues, while blue boxes represent healthy tissues. (D) Differential expression of CKS1B mRNA in LUAD and LUSC tissues relative to normal tissues (*P < 0.05; **P < 0.01; ***P < 0.001, based on differential analysis).
Comparative analysis using Oncomine server between LC (LUAD and LUSC) and normal tissue revealed an increased expression of the CKS1B gene in both LC subtypes (Figure 2Ai-vii; Supplementary Table S1). Additional evaluation of TCGA datasets using the UALCAN and GEPIA2 server also represented a significant overexpression of CKS1B in the LC subtypes (Figures 2B, C). Moreover, relative immunohistochemistry analysis of healthy and cancerous tissues using the HPA database showed moderate to weak staining signals in normal alveolar cells (Figure 2F), and strong signals in both LUAD (Figure 2D) and LUSC (Figure 2E) tissue samples (Figure 2; Supplementary Table S1).
Figure 2. Evaluation of CKS1B expression in LUAD and LUSC. (A) Box plots comparing CKS1B expression between normal (left) and cancerous (right) tissues in LUAD and LUSC (Ai–Avii). (B) Box plots showing CKS1B mRNA expression in tumor and normal tissues for LUAD and LUSC using the UALCAN platform. (C) CKS1B mRNA expression in LUAD and LUSC analyzed using GEPIA2. (D–F) Immunohistochemistry images showing CKS1B expression in LUAD (D), LUSC (E), and normal tissues (F) from the HPA database.
Analysis of the UALCAN database revealed that CKS1B upregulation for LUAD and LUSC varied in individual cancer stages (Figures 3Ai, ii) due to patient ethnicity, sex, age, smoke addiction, tumor histology, nodal metastasis, and TP53 mutation status (Figure 3; Supplementary Tables S2, S3). Correlated overexpression of CKS1B was also found in the patient of Asian (Figures 3Bi, ii), male (Figures 3Ci, ii), age group range of 21–40 years old (Figure 3Di, ii), smoking habit (Figures 3Ei, ii), tumor histology (Figures 3Fi, ii), nodal metastasis (Figures 3Gi, ii), and TP53 mutation status (Figures 3Hi, ii), for both LUAD and LUSC (Supplementary Tables S2, S3). These findings suggest a potential correlation of CKS1B upregulation and clinical characteristics with LC than normal patients.
Figure 3. Correlation of CKS1B expression with diagnostic criteria in LUAD and LUSC patients. CKS1B mRNA expression is analyzed across individual cancer stages, race, gender, age group, smoking habits, histological subtypes, nodal metastasis, and TP53 status for (Ai–Hi) LUAD and (Aii–Hii) LUSC.
Association between CKS1B expression and DNA methylation was evaluated using two separate methylation patterns present in the server, namely, Human Methylation 27K and Human Methylation 450K for both the LC subtypes (Figures 4A, B). Retrieved heat maps showed a close negative correlation between CKS1B expression and some CpG islands in LUAD (Figure 4A), whereas LUSC (Figure 4B) suggested that the overexpression of CKS1B associated with decreased DNA methylation in LUAD and LUSC, and vice versa (Figure 4).
Figure 4. CKS1B gene promoter methylation in lung cancer tissues. Heatmaps illustrate the CKS1B expression and DNA methylation status in (A) LUAD and (B) LUSC tissues.
An analysis of the CKS1B gene alterations in both the subtypes of LC was done using the cBioPortal database; totaling 2,983 samples from 8 LUAD studies and 1,176 samples from 3 LUSC studies (Table 1). Studies revealed 191 (6%) alteration in the CKS1B gene in LUAD samples (Figure 5Ai), with a somatic mutation frequency of 0.1 percent and 42 (4%) altered CKS1B gene in LUSC samples with a somatic mutation frequency of 0.3 percent (Figure 5Aii). CKS1B is found between 154,974,681 and 154,979,251 bp on chromosome 1q21.3. (http://atlasgeneticsoncology.org/Genes/CKS1BID40092ch1q21.html). Two mutations including one missense and one nonsense were found between 40–50 residues of the CKS domain of the 79 AA long CKS1B protein in LUAD samples (Figure 5Ai) whereas three missense mutations in the same mutation site (7th residue), as well as one fusion, were found in LUSC samples (Figure 5Aii). Furthermore, as seen in Figure 5Bi, amplification was found to be the most prevalent alteration type in both LC subtypes, with the highest frequency of 14.78% of 230 cases in the LUAD TCGA, Nature 2014 dataset. The LUSC TCGA, Firehose Legacy dataset had the highest alteration frequency of the three, at 4.18% of 502 cases. In the alteration study of LUAD TCGA, Nature 2014 dataset, both mutation and amplification of CKS1B have been identified with a frequency of 0.2% (1 case) and 3.98% (20 cases), respectively. In the LUSC TCGA, PanCancer Atlas, a fusion was also observed with a frequency of 0.21% (Figure 5Bii). Additionally, the CKS1B mRNA expression profile exhibited higher amplification, fewer gains, and twelve cases of shallow deletions in LUAD samples (Figure 5Ci). In LUSC samples, two missense mutations and one fusion along with some gains, diploids, and shallow deletions were found, as appeared in Figure 5Cii.
Figure 5. CKS1B gene alterations and mutations in LUAD and LUSC tissues. (Ai) Lollipop plot showing mutation spots in the CKS domain of CKS1B in LUAD tissues (residues 40–50). (Aii) Lollipop plot showing mutation spots in CKS1B in LUSC tissues. (Bi, Bii) Bar diagrams depicting mutation frequencies and genome alterations in CKS1B for LUAD and LUSC, respectively. (Ci, Cii) Correlation between CKS1B expression and copy number alterations for LUAD and LUSC in the TCGA dataset.
Consequently, the observations suggest that CKS1B overexpression in LUSC and LUAD may not have an established correlation with mutations or copy number alterations in the CKS1B gene (Figure 5). Nevertheless, these genetic modifications can serve as prognostic measures for LUAD and LUSC, using CKS1B as a biomarker. Also, the correlation of CKS1B to other functional proteins which are important for cell cycle, proliferation, and DNA repair, makes it a key component for increasing human susceptibility to diseases or detrimental effects, in case of mutations or alterations. This raises the importance of thorough future evaluations in regards to mutations and copy number alterations.
A negative correlation between CKS1B expression and LC patient survival (significant level was held at Cox p-value 0.05 and HR > 1) was found using the PrognoScan database. CKS1B expression was observed to be negatively associated with patient survival. This finding indicated that patients with elevated CKS1B expression might have a lower survival risk, whereas those with poor or average CKS1B expression may have a higher survival (overall or relapse-free) rate (Figure 6; Supplementary Table S4). The datasets showed an increased overall survival risk in patients with low CKS1B expression, while those with higher CKS1B expression had a considerably low overall survival rate (Figure 6). The investigation suggests a correlation between increased CKS1B expression and a poor prognosis in LC patients.
Figure 6. Correlation of CKS1B expression with lung cancer patient survival. Kaplan-Meier survival plots comparing high (red) and low (blue) CKS1B expression for overall survival (A–F) and relapse-free survival (G, H).
The Oncomine database was utilized to investigate the co-expression profile of CKS1B with 20 other genes, using 34 samples from LUAD and LUSC patients (Figure 7A). Among the 20 genes analyzed, the most co-expressed (R = 1.00) was the Src homology 2 domain-containing transforming protein C1 (SHC1). Following this, the GEPIA2 server also indicated a positive correlation with a Spearman coefficient in both LUAD (R = 0.25) and LUSC (R = 0.21) between CKS1B and SHC1 (Figure 7Bii). This was further supported by Pearson and Spearman correlation analyses results obtained from the UCSC Xena server, using the TCGA database (Figures 7C, D). The Pearson correlation values for LUAD (Figures 7Ci–Di) and LUSC (Figures 7Cii–Dii) patients were 0.3563 and 0.2327, and the Spearman correlation values for LUAD and LUSC were 0.4073 and 0.2176, respectively (Figures 7C, D). These values suggest that CKS1B and SHC1 can share a common biosynthetic pathway, or combine to form a large protein complex with LC progression.
Figure 7. CKS1B and co-expressed gene profiles in lung cancer. (Ai) Co-expression profile of CKS1B retrieved from the Oncomine database. (Bi-ii) Correlation analysis between CKS1B and SHC1 from the GEPIA2 server. (Ci-ii) Heatmap showing mRNA expression of CKS1B and SHC1 from the TCGA database. (Di-ii) Co-expression analysis of CKS1B and SHC1 in lung cancer using the UCSC Xena server.
The GeneMANIA server retrieves a complete network of CKS1B gene and its crosstalk genes of interaction in LC, displaying the physical interactions (67.40%), co-expression (13.82%), predicted (6.33%), co-localization (6.14%), pathways (4.33%), genetic interactions (1.39%) and shared protein domains (0.59%) (Figure 8A). Moreover, based on the International Molecular Exchange Consortium (IMEx) protein interactions database, protein-protein interaction (PPI) network was constructed for the co-expressed genes, and using NetworkAnalyst, these interactions were further analyzed for LUAD and LUSC progressions. Figure 8B shows the PPI network in which, the degree of a node is the number of connections among the node, and betweenness is the smallest path amongst nodes showing SHC1 (Degree: 207, Betweenness: 65,895.06), MUC1 (Degree: 31, Betweenness: 7,217.707), ADAM15 (Degree: 31, Betweenness: 9,000.452), TRIM46 (Degree: 30, Betweenness: 10,467.46), DAP3 (Degree: 28, Betweenness: 8,994.579), CKS1B (Degree: 27, Betweenness: 9,277.062), and ZBTB7B (Degree: 19, Betweenness: 6,702.692) as the major proteins of the network.
Figure 8. Gene interaction networks for CKS1B. (A) Interaction network of CKS1B with neighboring genes, categorized into physical interactions (67.40%), co-expression (13.82%), predicted (6.33%), co-localization (6.14%), pathways (4.33%), genetic interactions (1.39%), and shared protein domains (0.59%). (B) Protein-protein interaction network of CKS1B from the IMEx database.
The PathwayMapper tab in the cBioPortal server exhibits the CKS1B alteration frequency (in percentage) over multiple pathways on the LUAD and LUSC datasets (Figures 9A, B). RTK-Ras-RAF, PI3K/AKT, and TP3 pathways are mainly altered due to CKS1B gene products. These alterations coordinate the progression of LUAD and LUSC. Alterations related to CKS1B mainly induce the changes of EGFR (18.8%), KRAS (20.5%), FGFR1 (8.5%), and BRAF (6.0%) genes for regulation of RTK-Ras signaling pathway (Figure 9A) as well as PTEN (6.4%), PIK3CA (18.0%), STK11 (10.8%), and RICTOR (9.8%) in the regulation of P13K/AKT signaling pathway (Figure 9B); TP53 (48.9%), and CDKN2A (22.4%) in TP53 signaling pathway (Figure 9C) for cancer proliferation and development.
Figure 9. Pathway analysis for CKS1B and related genes. Alteration frequency in (A) RTK-Ras-RAF signaling pathway, (B) P13K/AKT signaling pathway, and (C) TP53 pathway in relation to CKS1B expression.
Results from three databases shown in Figures 10A–C were used for pathway determination. The KEGG human 2019 database analysis revealed different significant associations of different pathways in LC progression including RTK/Ras pathway, P13K/AKT pathway, MAPK, and Rap1 signaling pathway (Figure 10A). Additionally, the Reactome 2016 database showed LC progression depends on the pathways related to EPHA or EPH-Ephrin mediated signaling and repulsion of the cells, etc. (Figure 10B). The analysis of the Bioplanet 2019 database also revealed the Ephrin receptor A forward pathway, and p27 phosphorylation regulation during cell cycle progression alongside other important pathways associated with LC (Figure 10C). Following this, GO terms were also checked for the corresponding genes which mainly include, positive regulation of aspartic-type peptidase activity, axon guidance and axogenesis (Figure 10D), ephrin receptor binding and transmembrane-ephrine receptor activity (Figure 10F), and possible interactions with beta-catenin-TCF complex, mitochondrial small ribosomal subunit, and an anchored component of the plasma membrane (Figure 10E).
Figure 10. Gene ontology and pathway enrichment analysis for CKS1B in lung cancer. Ontologies and pathways retrieved from (A) KEGG human 2019, (B) Reactome 2016, (C) BioPlanet 2019, (D) GO Biological Process 2018, (E) GO Molecular Function 2018, and (F) GO Cellular Component 2018. Bar length represents significance level, with brighter colors indicating higher significance.
Lung cancer (LC) remains the leading cause of cancer-related mortality worldwide (Cancer Genome Atlas Research Network, 2014). NSCLC consisting of both LUAD and LUSC solely constitutes approximately 85% of all LC cases. LC is notoriously difficult to diagnose at an early stage, with most patients (about 75%) being diagnosed in advanced stages (stage III/IV), highlighting the need for early detection (Walters et al., 2013). Early diagnosis is crucial for better prognosis and survival rates, as shown by the significantly higher 5-year survival rates (70%–90%) in patients with stage I NSCLC following surgical resection (Shah et al., 1996; Nesbitt et al., 1995; Goldstraw et al., 2016). Despite advances in treatment, early detection remains the key to improving survival outcomes (Bradley et al., 2019).
In this study, we evaluated the role of CKS1B as a potential prognostic biomarker for the early diagnosis of LUAD and LUSC using bioinformatics approaches. Our analysis revealed a positive correlation between CKS1B expression levels and LC progression. High CKS1B expression was also negatively correlated with overall and relapse-free survival in LUAD and LUSC, as evidenced by an overall hazard ratio (HR) greater than 1. This suggests that elevated CKS1B expression is associated with poor survival outcomes, corroborating previous studies linking high CKS1B levels to adverse prognosis in LC (Shi et al., 2020b).
Further analysis revealed that CKS1B expression patterns in LUAD and LUSC were significantly associated with key clinical features such as tumor histology, patient demographics (e.g., race, gender, age, smoking status), and nodal metastasis (Figure 3). This highlights the importance of comprehensive studies that consider these variables, as CKS1B overexpression may serve as a marker of cancer progression (Figure 6). The immunohistochemical analysis, enhanced by computer-aided systems and digital imaging, revealed robust nuclear immunoreactivity for CKS1B in LUAD and LUSC tissues, with significantly more intense staining observed in cancer cells compared to normal alveolar cells (Figure 2). This suggests elevated CKS1B expression in lung cancer cells. Such findings indicate that CKS1B plays a pivotal role in the pathogenesis of NSCLC, reflecting the complex histopathological processes driven by somatically acquired genetic, epigenetic, transcriptomic, and proteomic alterations responsible for cancer progression (Laurinaviciene et al., 2012; Laurinavicius et al., 2016; Yu and Tian, 2020b).
On a molecular level, somatic genetic alterations, such as copy number changes and mutations, are known to drive cancer progression. Our analysis using the cBioPortal webserver indicated that the CKS1B gene was altered in 6% of LUAD samples and 4% of LUSC samples, with missense mutations being the most common genetic change (Figures 5A–C). This suggests that genetic alterations in CKS1B may contribute to LC pathogenesis, although their low frequency highlights the need for further investigation.
Interestingly, we observed a negative correlation between CKS1B expression and DNA methylation of CpG islands in gene promoters, a finding consistent with previous studies linking promoter methylation to transcriptional silencing in various cancer types, including LC (Slotky et al., 2005). Additionally, co-expression and correlation analysis identified 20 genes positively correlated with CKS1B, with SHC1 emerging as a key player in NSCLC progression through the Ras/ERK and PI3K/AKT signaling pathways (Figure 7) (Liu et al., 2019). This supports the idea that CKS1B and its co-expressed genes may interact in a network that influences tumor cell behavior and malignancy.
Moreover, we explored the functional network of CKS1B and its neighboring genes in lung cancer, highlighting significant interactions that include co-localization, pathways, and genetic interactions (Figure 8). CKS1B interacts with cyclin-dependent kinases (CDKs) and S-phase kinase-associated proteins (SKPs), suggesting its critical role in regulating the cell cycle. CDK1, in particular, is essential for G2/M and G1/S transitions and is often overexpressed in malignancies, which correlates with poor patient prognosis (Santamaria et al., 2007; Malumbres and Barbacid, 2009; Enserink and Kolodner, 2010; Ravindran Menon et al., 2018; Li et al., 2020). Furthermore, CKS1B promotes the degradation of the cell cycle inhibitor p27Kip1 via its interaction with SKP2, leading to increased cell proliferation (You et al., 2015). This dysregulation of CKS1B can impact a broader network of co-expressed genes, which are regulated by shared transcriptional programs, thereby influencing multiple biological pathways (Weirauch, 2011; Melak and Gakkhar, 2015). Additionally, proteins such as SHC1, MUC1, and ADAM15 display high betweenness centrality scores, indicating their importance as mediators in lung cancer progression (Gursoy et al., 2008). These insights underscore the potential of targeting CKS1B and its associated pathways for therapeutic strategies in lung cancer.
Our study also highlighted the importance of CKS1B in regulating multiple signaling pathways associated with cancer. CKS1B overexpression was found to alter the RTK-Ras-RAF and PI3K/AKT/mTOR signaling pathways, both of which are heavily implicated in LUAD and LUSC pathogenesis (Figures 9A, B). Genetic alterations in key components of these pathways, such as EGFR, BRAF, KRAS, PIK3CA, and PTEN, have been well-documented in NSCLC, and our study suggests that CKS1B may play a role in modulating these alterations to drive cancer progression (McCubrey et al., 2007; Desai et al., 2014; Herbst et al., 2018; Campbell et al., 2016; Desai et al., 2016; Beigel et al., 2020; Saini et al., 2016; Bethune et al., 2010; Tan, 2020; Yuan and Cantley, 2008; Scheffler et al., 2015). Additionally, the TP53 pathway, a critical regulator of tumor suppression, was found to be downregulated in connection with CKS1B expression, further emphasizing its role in LC progression (Figure 9C).
Finally, pathway and gene ontology (GO) enrichment analyses reinforced the significance of CKS1B and its co-expressed genes in multiple oncogenic processes. Notably, ephrin-mediated signaling, aspartic-type peptidase activity (involving Napsin A), and the TCF/β-catenin complex were implicated in LC development, suggesting that CKS1B and its associated genes influence diverse molecular pathways in LC (Pasquale, 2010; Ueno et al., 2008; Gao et al., 2018) (Figure 10).
Overall, our study provides substantial evidence supporting the role of CKS1B as a prognostic biomarker for early diagnosis in LUAD and LUSC. Although these findings are promising, further in vitro and in vivo studies are necessary to validate CKS1B’s clinical application in NSCLC diagnosis and treatment.
LC is the predominant cause of cancer-related deaths worldwide. This study aimed to identify the molecular signatures involved in the development and progression of LUAD and LUSC. Cancer prognostic factors are essential for early diagnosis and effective treatment, reducing the risk of overtreatment for patients. CKS1B appears to be involved in both LUAD and LUSC, according to our findings. The study further points to the possibility of using CKS1B as a biomarker for early LC detection and medication screening in treatment strategies. Furthermore, since CKS1B is involved in several important signaling pathways, inhibiting it may be a new way to prevent or reduce LC. CKS1B and its expression in LC progression were also linked to potential signaling mechanisms and gene ontological characteristics, according to the study. Researchers could be able to stop cancer from spreading by working on these pathways. As a result, CKS1B has been suggested as a useful biomarker and potential therapeutic target for human LC regulation or prevention.
The original contributions presented in the study are included in the article/Supplementary Material, further inquiries can be directed to the corresponding author.
MdH: Funding acquisition, Resources, Validation, Writing–original draft, Writing–review and editing. TI: Funding acquisition, Resources, Validation, Writing–original draft, Writing–review and editing. NF: Formal Analysis, Methodology, Writing–original draft, Writing–review and editing. TR: Methodology, Visualization, Writing–original draft, Writing–review and editing. YS: Resources, Writing–original draft, Writing–review and editing. SiS: Resources, Writing–original draft, Writing–review and editing. AM: Conceptualization, Formal Analysis, Methodology, Project administration, Visualization, Writing–original draft, Writing–review and editing. YA: Writing–original draft, Writing–review and editing. IA: Writing–original draft, Writing–review and editing. SoS: Writing–original draft, Writing–review and editing. MoH: Supervision, Writing–original draft, Writing–review and editing.
The author(s) declare that no financial support was received for the research, authorship, and/or publication of this article.
The authors declare that the research was conducted in the absence of any commercial or financial relationships that could be construed as a potential conflict of interest.
All claims expressed in this article are solely those of the authors and do not necessarily represent those of their affiliated organizations, or those of the publisher, the editors and the reviewers. Any product that may be evaluated in this article, or claim that may be made by its manufacturer, is not guaranteed or endorsed by the publisher.
The Supplementary Material for this article can be found online at: https://www.frontiersin.org/articles/10.3389/fgene.2025.1449466/full#supplementary-material
Allemani, C., Matsuda, T., Di Carlo, V., Harewood, R., Matz, M., Nikšić, M., et al. (2018). Global surveillance of trends in cancer survival 2000–14 (CONCORD-3): analysis of individual records for 37,513,025 patients diagnosed with one of 18 cancers from 322 population-based registries in 71 countries. Lancet 391 (10125), 1023–1075. doi:10.1016/S0140-6736(17)33326-3
Beigel, J. H., Tomashek, K. M., Dodd, L. E., Mehta, A. K., Zingman, B. S., Kalil, A. C., et al. (2020). Remdesivir for the treatment of covid-19 - final report. N. Engl. J. Med. 383, 1813–1826. doi:10.1056/NEJMoa2007764
Bender, E. (2014). Epidemiology: the dominant malignancy. Nature 513 (7517), S2–S3. doi:10.1038/513S2a
Bethune, G., Bethune, D., Ridgway, N., and Xu, Z. (2010). Epidermal growth factor receptor (EGFR) in lung cancer: an overview and update. J. Thorac. Dis. 2 (1), 48–51.
Bradley, S. H., Kennedy, M. P., and Neal, R. D. (2019). Recognising lung cancer in primary care. Adv. Ther. 36 (1), 19–30. doi:10.1007/s12325-018-0843-5
Bray, F., Laversanne, M., Sung, H., Ferlay, J., Siegel, R. L., Soerjomataram, I., et al. (2024). Global cancer statistics 2022: GLOBOCAN estimates of incidence and mortality worldwide for 36 cancers in 185 countries. CA Cancer J. Clin. 74 (3), 229–263. doi:10.3322/caac.21834
Campbell, J. D., Alexandrov, A., Kim, J., Wala, J., Berger, A. H., Pedamallu, C. S., et al. (2016). Distinct patterns of somatic genome alterations in lung adenocarcinomas and squamous cell carcinomas. Nat. Genet. 48, 607–616. doi:10.1038/ng.3564
Cancer Genome Atlas Research Network (2014). Comprehensive molecular profiling of lung adenocarcinoma. Nature 511, 543–550. doi:10.1038/nature13385
Cerami, E., Gao, J., Dogrusoz, U., Gross, B. E., Sumer, S. O., Aksoy, B. A., et al. (2012). The cBio cancer genomics portal: an open platform for exploring multidimensional cancer genomics data. Cancer Discov. 2, 401–404. doi:10.1158/2159-8290.CD-12-0095
Chandrashekar, D. S., Bashel, B., Balasubramanya, S. A., Creighton, C. J., Ponce-Rodriguez, I., Chakravarthi, B. V., et al. (2017). UALCAN: a portal for facilitating tumor subgroup gene expression and survival analyses. Neoplasia 19 (8), 649–658. doi:10.1016/j.neo.2017.05.002
Chen, E. Y., Tan, C. M., Kou, Y., Duan, Q., Wang, Z., Meirelles, G. V., et al. (2013). Enrichr: interactive and collaborative HTML5 gene list enrichment analysis tool. BMC Bioinforma. 14 (1), 128. doi:10.1186/1471-2105-14-128
Chen, M., Qi, C., Reece, D., and Chang, H. (2012). Cyclin kinase subunit 1B nuclear expression predicts an adverse outcome for patients with relapsed/refractory multiple myeloma treated with bortezomib. Hum. Pathol. 43 (6), 858–864. doi:10.1016/j.humpath.2011.07.013
Desai, A., Menon, S. P., and Dy, G. K. (2016). Alterations in genes other than EGFR/ALK/ROS1 in non-small cell lung cancer: trials and treatment options. Cancer Biol. and Med. 13 (1), 77–86. doi:10.28092/j.issn.2095-3941.2016.0008
Desai, T. J., Brownfield, D. G., and Krasnow, M. A. (2014). Alveolar progenitor and stem cells in lung development, renewal and cancer. Nature 507 (7491), 190–194. doi:10.1038/nature12930
Enserink, J. M., and Kolodner, R. D. (2010). An overview of Cdk1-controlled targets and processes. Cell Div. 5, 11. doi:10.1186/1747-1028-5-11
Foss, K., Sima, C., Ugolini, D., Neri, M., Allen, K., and Weiss, G. (2011). miR-1254 and miR-574-5p: serum-based microRNA biomarkers for early-stage non-small cell lung cancer. J. Thorac. Oncol. 6 (3), 482–488. doi:10.1097/JTO.0b013e318208c785
Gao, C., Wang, Y., Broaddus, R., Sun, L., Xue, F., and Zhang, W. (2018). Exon 3 mutations of CTNNB1 drive tumorigenesis: a review. Oncotarget 9 (4), 5492–5508. doi:10.18632/oncotarget.23695
Goldman, M. J., Craft, B., Hastie, M., Repečka, K., McDade, F., Kamath, A., et al. (2020). Visualizing and interpreting cancer genomics data via the Xena platform. Nat. Biotechnol. 22, 675–678. doi:10.1038/s41587-020-0546-8
Goldstraw, P., Chansky, K., Crowley, J., Rami-Porta, R., Asamura, H., Eberhardt, W. E. E., et al. (2016). The IASLC lung cancer staging project: proposals for revision of the TNM stage groupings in the forthcoming (eighth) edition of the TNM classification for lung cancer. J. Thorac. Oncol. 11, 39–51. doi:10.1016/j.jtho.2015.09.009
Gursoy, A., Keskin, O., and Nussinov, R. (2008). Topological properties of protein interaction networks from a structural perspective. Biochem. Soc. Trans. 36 (6), 1398–1403. doi:10.1042/BST0361398
Herbst, R. S., Morgensztern, D., and Boshoff, C. (2018). The biology and management of non-small cell lung cancer. Nature 553 (7689), 446–454. doi:10.1038/nature25183
Herms, L., Su, Z., Robert, N. J., O'Sullivan, A. K., and Paulus, J. K. (2024). Assessing secular trends in lung cancer stage in the United States community oncology setting from 2013 to 2023. J. Clin. Oncol. 42 (16_Suppl. l), 11178. doi:10.1200/jco.2024.42.16_suppl.11178
Huang, J., Zhou, Y., Thomas, G., Gu, Z., Yang, Y., Xu, H., et al. (2015). NEDD8 inhibition overcomes CKS1B-induced drug resistance by upregulation of p21 in multiple myeloma. Clin. Cancer Res. 21 (24), 5532–5542. doi:10.1158/1078-0432.CCR-15-0254
Hwang, J. S., Jeong, E. J., Choi, J., Lee, Y. J., Jung, E., Kim, S. K., et al. (2019). MicroRNA-1258 inhibits the proliferation and migration of human colorectal cancer cells through suppressing CKS1B expression. Genes 10 (11), 912. doi:10.3390/genes10110912
Jemal, A., Bray, F., Center, M., Ferlay, J., Ward, E., and Forman, D. (2011). Global cancer statistics. CA A Cancer J. Clin. 61 (2), 69–90. doi:10.3322/caac.20107
Kitajima, S., Kudo, Y., Ogawa, I., Bashir, T., Kitagawa, M., Miyauchi, M., et al. (2004). Role of Cks1 overexpression in oral squamous cell carcinomas: cooperation with Skp2 in promoting p27 degradation. Am. J. Of Pathology 165 (6), 2147–2155. doi:10.1016/S0002-9440(10)63264-6
Krishnan, A., Nair, S., and Pillai, M. (2009). Loss of cks1 homeostasis deregulates cell division cycle. J. Of Cell. And Mol. Med. 14 (1-2), 154–164. doi:10.1111/j.1582-4934.2009.00698.x
Kuleshov, M. V., Jones, M. R., Rouillard, A. D., Fernandez, N. F., Duan, Q., Wang, Z., et al. (2016). Enrichr: a comprehensive gene set enrichment analysis web server 2016 update. Nucleic acids Res. 44 (W1), W90–W97. doi:10.1093/nar/gkw377
Laurinaviciene, V. O., Dasevicius, D., Jarmalaite, S., and Lazutka, J. (2012). Immunohistochemistry profiles of breast ductal carcinoma: factor analysis of digital image analysis data. Diagn. Pathol. 7 (27), 27. doi:10.1186/1746-1596-7-27
Laurinavicius, A., Plancoulaine, B., Herlin, P., and Laurinaviciene, A. (2016). Comprehensive immunohistochemistry: digital, analytical and integrated. Pathobiology 83 (2-3), 156–163. doi:10.1159/000442389
Lee, E., Kim, D., Kim, J., and Yoon, Y. (2011). Cell-cycle regulator Cks1 promotes hepatocellular carcinoma by supporting NF-κB-Dependent expression of interleukin-8. Cancer Res. 71 (21), 6827–6835. doi:10.1158/0008-5472.CAN-10-4356
Lemjabbar-Alaoui, H., Hassan, O., Yang, Y., and Buchanan, P. (2015). Lung cancer: biology and treatment options. Biochimica Biophysica Acta (BBA) - Rev. Cancer 1856 (2), 189–210. doi:10.1016/j.bbcan.2015.08.002
Li, M., He, F., Zhang, Z., Xiang, Z., and Hu, D. (2020). CDK1 serves as a potential prognostic biomarker and target for lung cancer. J. Int. Med. Res. 48 (2), 0300060519897508. doi:10.1177/0300060519897508
Liu, L., Yang, Y., Liu, S., Tao, T., Cai, J., Wu, J., et al. (2019). EGF-induced nuclear localization of SHCBP1 activates β-catenin signaling and promotes cancer progression. Oncogene 38 (5), 747–764. doi:10.1038/s41388-018-0473-z
Malumbres, M., and Barbacid, M. (2009). Cell cycle, CDKs and cancer: a changing paradigm. Nat. Rev. Cancer 9, 153–166. doi:10.1038/nrc2602
McCubrey, J. A., Steelman, L. S., Chappell, W. H., Abrams, S. L., Wong, E. W., Chang, F., et al. (2007). Roles of the Raf/MEK/ERK pathway in cell growth, malignant transformation and drug resistance. Biochimica Biophysica Acta (BBA)-Molecular Cell Res. 1773 (8), 1263–1284. doi:10.1016/j.bbamcr.2006.10.001
Melak, T., and Gakkhar, S. (2015). Comparative genome and network centrality analysis to identify drug targets of mycobacterium tuberculosis H37Rv. BioMed Res. Int. 2015, 212061. doi:10.1155/2015/212061
Mizuno, H., Kitada, K., Nakai, K., and Sarai, A. (2009). PrognoScan: a new database for meta-analysis of the prognostic value of genes. BMC Med. genomics 2 (1), 18. doi:10.1186/1755-8794-2-18
National Lung Screening Trial Research Team (2019). Lung cancer incidence and mortality with extended follow-up in the national lung screening trial. J. Thorac. Oncol. 14 (10), 1732–1742. doi:10.1016/j.jtho.2019.05.044
Nesbitt, J. C., Putnam, J. B., Walsh, G. L., Roth, J. A., and Mountain, C. F. (1995). Survival in early-stage non-small cell lung cancer. Ann. Thorac. Surg. 60, 466–472. doi:10.1016/0003-4975(95)00169-L
Park, S. J., Yoon, B. H., Kim, S. K., and Kim, S. Y. (2019). GENT2: an updated gene expression database for normal and tumor tissues. BMC Med. genomics 12 (5), 101. doi:10.1186/s12920-019-0514-7
Pasquale, E. B. (2010). Eph receptors and ephrins in cancer: bidirectional signalling and beyond. Nat. Rev. Cancer 10 (3), 165–180. doi:10.1038/nrc2806
Ravindran Menon, D., Luo, Y., Arcaroli, J. J., Liu, S., KrishnanKutty, L. N., Osborne, D. G., et al. (2018). CDK1 interacts with Sox2 and promotes tumor initiation in human melanoma. Cancer Res. 78, 6561–6574. doi:10.1158/0008-5472.CAN-18-0330
Rhodes, D. R., Yu, J., Shanker, K., Deshpande, N., Varambally, R., Ghosh, D., et al. (2004). ONCOMINE: a cancer microarray database and integrated data-mining platform. Neoplasia (New York, NY) 6 (1), 1–6. doi:10.1016/S1476-5586(04)80047-2
Saini, R., Batra, U., Jain, A., and Agrawal, C. (2016). EGFR mutation--a commonly neglected mutation in squamous cell lung carcinoma. J. Cancer Metastasis Treat. 2, 253–254. doi:10.20517/2394-4722.2016.07
Santamaria, D., Barriere, C., Cerqueira, A., Hunt, S., Tardy, C., Newton, K., et al. (2007). Cdk1 is sufficient to drive the mammalian cell cycle. Nature 448, 811–815. doi:10.1038/nature06046
Scheffler, M., Bos, M., Gardizi, M., König, K., Michels, S., Fassunke, J., et al. (2015). PIK3CA mutations in non-small cell lung cancer (NSCLC): genetic heterogeneity, prognostic impact and incidence of prior malignancies. Oncotarget 6, 1315–1326. doi:10.18632/oncotarget.2834
Shah, R., Sabanathan, S., Richardson, J., Mearns, A. J., and Goulden, C. (1996). Results of surgical treatment of stage I and II lung cancer. J. Cardiovasc. Surg. 37, 169–172.
Shaughnessy, J., Zhan, F., Burington, B., Huang, Y., Colla, S., Hanamura, I., et al. (2006). A validated gene expression model of high-risk multiple myeloma is defined by deregulated expression of genes mapping to chromosome 1. Blood 109 (6), 2276–2284. doi:10.1182/blood-2006-07-038430
Shi, W., Huang, Q., Xie, J., Wang, H., Yu, X., and Zhou, Y. (2020a). CKS1B as drug resistance-inducing gene-A potential target to improve cancer therapy. Front. Oncol. 10, 582451. doi:10.3389/fonc.2020.582451
Shi, W., Huang, Q., Xie, J., Wang, H., Yu, X., and Zhou, Y. (2020b). CKS1B as drug resistance-inducing gene-A potential target to improve cancer therapy. Front. Oncol. 10, 582451. doi:10.3389/fonc.2020.582451
Slotky, M., Shapira, M., Ben-Izhak, O., Linn, S., Futerman, B., Tsalic, M., et al. (2005). The expression of the ubiquitin ligase subunit Cks1 in human breast cancer. Breast Cancer Res. 7 (5), R737–R744. doi:10.1186/bcr1278
Stella, F., Pedrazzini, E., Baialardo, E., Fantl, D., Schutz, N., and Slavutsky, I. (2014). Quantitative analysis of CKS1B mRNA expression and copy number gain in patients with plasma cell disorders. Blood Cells. Mol. And Dis. 53 (3), 110–117. doi:10.1016/j.bcmd.2014.05.006
Sung, H., Ferlay, J., Siegel, R., Laversanne, M., Soerjomataram, I., Jemal, A., et al. (2021). Global cancer statistics 2018: GLOBOCAN estimates of incidence and mortality worldwide for 36 cancers in 185 countries. CA A Cancer J. Clin. 68, 394–424. doi:10.3322/caac.21492
Tan, A. C. (2020). Targeting the PI3K/AKT/mTOR pathway in non-small cell lung cancer (NSCLC). Thorac. cancer 11 (3), 511–518. doi:10.1111/1759-7714.13328
Tang, Z., Kang, B., Li, C., Chen, T., and Zhang, Z. (2019). GEPIA2: an enhanced web server for large-scale expression profiling and interactive analysis. Nucleic acids Res. 47 (W1), W556-W560–60. doi:10.1093/nar/gkz430
Ueno, T., Elmberger, G., Weaver, T. E., Toi, M., and Linder, S. (2008). The aspartic protease napsin A suppresses tumor growth independent of its catalytic activity. Lab. Investig. 88 (3), 256–263. doi:10.1038/labinvest.3700718
Uhlén, M., Fagerberg, L., Hallström, B. M., Lindskog, C., Oksvold, P., Mardinoglu, A., et al. (2015). Proteomics. Tissue-based map of the human proteome. Science 347 (6220), 1260419. doi:10.1126/science.1260419
Walters, S., Maringe, C., Coleman, M. P., Peake, M. D., Butler, J., Young, N., et al. (2013). Lung cancer survival and stage at diagnosis in Australia, Canada, Denmark, Norway, Sweden and the UK: a population-based study, 2004-2007. Thorax 68, 551–564. doi:10.1136/thoraxjnl-2012-202297
Wang, X., Xu, J., Ju, S., Ni, H., Zhu, J., and Wang, H. (2010). Livin gene plays a role in drug resistance of colon cancer cells. Clin. Biochem. 43 (7-8), 655–660. doi:10.1016/j.clinbiochem.2010.02.004
Weirauch, M. T. (2011). Gene coexpression networks for the analysis of DNA microarray data. Appl. statistics Netw. Biol. methods Syst. Biol. 1, 215–250. doi:10.1002/9783527638079.ch11
What Is Cancer (2021). Improving the lives of all people through cancer research. Available at: https://www.cancer.gov/about-cancer/understanding/what-is-cancer (Accessed March 19, 2021).
What Is Lung Cancer (2021). Types of lung cancer. Available at: https://www.google.com/amp/s/amp.cancer.org/cancer/lung-cancer/about/what-is.html (Accessed March 19, 2021).
Wong, M., Lao, X., Ho, K., Goggins, W., and Tse, S. (2017). Incidence and mortality of lung cancer: global trends and association with socioeconomic status. Sci. Rep. 7 (1), 14300. doi:10.1038/s41598-017-14513-7
You, H., Lin, H., and Zhang, Z. (2015). CKS2 in human cancers: clinical roles and current perspectives (Review). Mol. Clin. Oncol. 3 (3), 459–463. doi:10.3892/mco.2015.501
Yu, Y., and Tian, X. (2020a). Analysis of genes associated with prognosis of lung adenocarcinoma based on GEO and TCGA databases. Medicine 99 (19), e20183. doi:10.1097/MD.0000000000020183
Yu, Y., and Tian, X. (2020b). Analysis of genes associated with prognosis of lung adenocarcinoma based on GEO and TCGA databases. Med. Baltim. 99 (19), e20183. doi:10.1097/MD.0000000000020183
Yuan, T. L., and Cantley, L. C. (2008). PI3K pathway alterations in cancer: variations on a theme. Oncogene 27, 5497–5510. doi:10.1038/onc.2008.245
Zeng, Z., Gao, Z., Zhang, Z., Jiang, H., Yang, C., Yang, J., et al. (2019). Downregulation of CKS1B restrains the proliferation, migration, invasion and angiogenesis of retinoblastoma cells through the MEK/ERK signaling pathway. Int. J. Of Mol. Med. 44, 103–114. doi:10.3892/ijmm.2019.4183
Zhan, F., Colla, S., Wu, X., Chen, B., Stewart, J., Kuehl, W., et al. (2007). CKS1B, overexpressed in aggressive disease, regulates multiple myeloma growth and survival through SKP2- and p27Kip1-dependent and -independent mechanisms. Blood 109 (11), 4995–5001. doi:10.1182/blood-2006-07-038703
Keywords: CKS1B gene, lung adenocarcinoma, lung squamous cell carcinoma, gene expression, biomarker, mRNA expression and regulation, gene mutation, signaling pathways
Citation: Hossain MS, Islam Tusar T, Faruqui NA, Rahaman TI, Sharker YA, Santo SS, Moin AT, Araf Y, Afif IK, Saikat S and Hosen MJ (2025) Exploring the oncogenic role and prognostic value of CKS1B in human lung adenocarcinoma and squamous cell carcinoma. Front. Genet. 16:1449466. doi: 10.3389/fgene.2025.1449466
Received: 15 June 2024; Accepted: 11 February 2025;
Published: 17 March 2025.
Edited by:
Quan Zou, University of Electronic Science and Technology of China, ChinaReviewed by:
Magdalena Julita Orzechowska, Medical University of Lodz, PolandCopyright © 2025 Hossain, Islam Tusar, Faruqui, Rahaman, Sharker, Santo, Moin, Araf, Afif, Saikat and Hosen. This is an open-access article distributed under the terms of the Creative Commons Attribution License (CC BY). The use, distribution or reproduction in other forums is permitted, provided the original author(s) and the copyright owner(s) are credited and that the original publication in this journal is cited, in accordance with accepted academic practice. No use, distribution or reproduction is permitted which does not comply with these terms.
*Correspondence: Mohammad Jakir Hosen, amFraXItZ2VuQHN1c3QuZWR1
†These authors have contributed equally to this work
Disclaimer: All claims expressed in this article are solely those of the authors and do not necessarily represent those of their affiliated organizations, or those of the publisher, the editors and the reviewers. Any product that may be evaluated in this article or claim that may be made by its manufacturer is not guaranteed or endorsed by the publisher.
Research integrity at Frontiers
Learn more about the work of our research integrity team to safeguard the quality of each article we publish.