- 1Shengli Clinical Medical College of Fujian Medical University, Fuzhou, China
- 2Fuzong Clinical Medical College of Fujian Medical University, Fuzhou, China
- 3Department of Emergency Trauma Surgery, Fujian Provincial Hospital, Fuzhou, China
- 4Fujian Trauma Medicine Center, Fuzhou, China
- 5Fujian Key Laboratory of Emergency Medicine, Fuzhou, China
- 6Department of Paediatric Orthopaedics, Fuzhou Second Hospital, The Third Clinical Medicine College of Fujian Medical University, Fuzhou, China
Background: Prior research has demonstrated that programmed cell death (PCD) and mitochondria assume pivotal roles in controlling cellular metabolism and maintaining bone cell equilibrium. Nonetheless, the comprehensive elucidation of their mode of operation in osteoporosis (OP) warrants further investigation. Therefore, this study aimed at analyzing the role of genes associated with PCD (PCD-RGs) and mitochondria (mortality factor-related genes; MRGs) in OP.
Methods: Differentially expressed genes (DEGs) were identified by subjecting the GSE56815 dataset obtained from the Gene Expression Omnibus database to differential expression analysis and comparing OP patients with healthy individuals. The genes of interest were ascertained through the intersection of DEGs, MRGs, and PCD-RGs; these genes were filtered using machine learning methodologies to discover potential biomarkers. The prospective biomarkers displaying uniform patterns and statistically meaningful variances were identified by evaluating their levels in the GSE56815 dataset and conducting quantitative real-time polymerase chain reaction-based assessments. Moreover, the functional mechanisms of these biomarkers were further delineated by constructing a nomogram, which conducted gene set enrichment analysis, explored immune infiltration, generated regulatory networks, predicted drug responses, and performed molecular docking analyses.
Results: Eighteen candidate genes were documented contingent upon the intersection between 2,354 DEGs, 1,136 MRGs, and 1,548 PCD-RGs. The biomarkers DAP3, BIK, and ACAA2 were upregulated in OP and were linked to oxidative phosphorylation. Furthermore, the predictive ability of the nomogram designed based on the OP biomarkers exhibited a certain degree of accuracy. Correlation analysis revealed a strong positive correlation between CD56dim natural killer cells and ACAA2 and a significant negative correlation between central memory CD4+ T cells and DAP3. DAP3, BIK, and ACAA2 were regulated by multiple factors; specifically, SETDB1 and ZNF281 modulated ACAA2 and DAP3, whereas TP63 and TFAP2C governed DAP3 and BIK. Additionally, a stable binding force was observed between the drugs (estradiol, valproic acid, and CGP52608) and the biomarkers.
Conclusion: This investigation evidenced that the biomarkers DAP3, BIK, and ACAA2 are associated with PCD and mitochondria in OP, potentially facilitate the diagnosis of OP in clinical settings.
1 Introduction
Osteoporosis (OP) is a persistent condition characterized by reduced bone density, degradation of bone composition, and fractures resulting from brittleness (Walker and Shane, 2023). This condition can lead to a diminished quality of life, premature mortality, disability, and an elevated economic burden (Reid and Billington, 2022; Ensrud and Crandall, 2024). The current clinical management of OP predominantly involves the administration of medications such as bisphosphonates, estrogen therapies, and calcitonin (van der Burgh et al., 2021). Nevertheless, adverse reactions associated with these medications impede their ability to effectively remediate OP. The etiology of OP is complex, with disturbances in bone metabolism increasing the likelihood of developing the disease. With advancements in research on osteoimmunology and the molecular mechanisms related to OP, there is growing acknowledgment of the significance of molecular and osteoimmune interventions in treating OP (Adejuyigbe et al., 2023). Consequently, this exerts an urgent requirement to develop innovative therapies for the treatment of OP.
Programmed cell death (PCD) is an active process through which cells die in response to specific signals or stimuli to maintain the balance of the internal environment. PCD is involved in various biochemical and morphological characteristics (Li Z. et al., 2023). The different types of PCD include apoptosis, necrosis, autophagy, lysosome-dependent cell death, ferroptosis, necroptosis, pyroptosis, and immunogenic cell death (Bedoui et al., 2020; Awuti et al., 2022). Under normal physiological conditions, PCD occurs in harmony to sustain cell stability. However, in conditions such as OP, imbalances in PCD types can arise and interact with each other, thereby affecting the onset and progression of OP. Efficiently controlled PCD plays a crucial role in maintaining osteoblast (OB) and bone metabolism stability, whereas excessive PCD can result in OB impairment, enhanced conversion of bone marrow mesenchymal stem cells (BMSCs) into adipocytes, and the accumulation of harmful substances, ultimately contributing to OP development (Daponte et al., 2024). Regulation of bone metabolism is significantly influenced by apoptosis, autophagy, ferroptosis, pyroptosis, and necrosis, all of which play crucial roles in bone cell activity (Zhang et al., 2023). Despite advancements in identifying essential molecules and signaling pathways involved in PCD, our understanding of how these pathways control the onset and progression of OP remains limited.
Mitochondria assume a crucial role in energy production, iron balance, and the synthesis of different compounds. Studies have shown that mitochondria are vital for regulating the equilibrium between osteoclast and OB functions, thereby affecting bone health (Wang et al., 2021). Furthermore, mitochondria govern cell growth, specialization, energy generation, signaling, and cell death. Any malfunction in mitochondrial function can lead to conditions such as OP and reduced OB activity, which are common in orthopedic ailments. Senile OP is linked to abnormal mitochondrial function, which hinders bone formation, increases osteoclast activity, and accelerates bone deterioration (Zheng et al., 2020). Mitochondria also participate in cell death, and irregular mitochondrial operations activate diverse forms of PCD (Flowers et al., 2023). The buildup of faulty mitochondria leads to the excessive production of reactive oxygen species (ROS), hastening cell death (Wang et al., 2012). Mitochondrial glycolysis and oxidative phosphorylation pathways, in conjunction with ROS generation, are essential for meeting the energy needs of bone-forming cell proliferation and differentiation (Qin et al., 2019). The intricate interplay between mitochondrial function and programmed cell death (PCD) plays a pivotal role in the pathogenesis of osteoporosis (OP). However, there is a dearth of bioinformatics research dedicated to elucidating and validating genes that are co-expressed with PCD and mitochondria during the progression of OP.
Therefore, this study aimed to elucidate and validate genes that exhibit co-expression with PCD and mitochondria during the progression of OP. Specifically, we employed a two-step approach: ① Identification and validation of markers using data from the Gene Expression Omnibus (GEO) database combined with quantitative real-time polymerase chain reaction (qRT-PCR) analysis on serum samples obtained from clinical subjects; ② Exploration of underlying mechanisms and prognostic potential through enrichment analyses, immune infiltration assessments, establishment of regulatory networks, and prediction of potential therapeutic drugs. In order to address the future clinical demands of osteoporosis treatment, it is imperative to enhance targeted regulation of mitochondrial function and programmed cell death, thereby fostering fundamental research and facilitating drug development in bone metabolism.
2 Materials and methods
2.1 Data extraction
Data pertaining to OP was acquired from the GEO database (http://www.ncbi.nlm.nih.gov/geo/). The GSE56815 dataset (platform: GPL96) served as the training set and comprised 40 blood samples (circulating monocytes), each derived from healthy individuals with high hip bone mineral density (BMD) and OP patients with low BMD. Each group of participants included 20 premenopausal and 20 postmenopausal women. A total of 1,548 PCD-related genes (PCD-RGs) and 1,136 genes associated with mitochondria (mortality factor-related genes; MRGs) were procured from extant literature (Supplementary Table S1) (Qin et al., 2023) and the MitoCarta 3.0 database (https://www.broadinstitute.org/mitocarta/mitocarta30-inventory-mammalian-mitochondrial-proteins-and-pathways), respectively.
2.2 Differential expression analysis
To identify differentially expressed genes (DEGs), the OP and control specimens within the GSE56815 dataset were subjected to differential expression analysis-based comparison using the limma package (v 3.57.11; significance threshold of p < 0.05) (Ritchie et al., 2015). Subsequently, the DEGs were visualized by generating volcano plots and heat maps using the ggplot2 (v. 3.4.2) (Gustavsson et al., 2022) and the ComplexHeatmap packages (v 2.17.0) (Gu et al., 2016), respectively.
2.3 Recognition and functional analysis of candidate genes
To distinguish the DEGs related to both PCD and mitochondria, the DEGs were overlapped with the MRGs and PCD-RGs using the ggVennDiagram package (v 1.2.3) (Gao et al., 2021). The resulting genes were denoted as potential candidate biomarker genes. Next, the aforementioned genes were annotated via Gene Ontology (GO) and pathway analyses using the clusterProfiler package (v 4.9.4) (Wu et al., 2021) with an adjusted p-value cutoff of <0.05. GO analysis encompassed biological processes (BPs), cellular components (CCs), and molecular functions (MFs). This was followed by an enrichment analysis using the Kyoto Encyclopedia of Genes and Genomes (KEGG) pathways. Additionally, the potential interactions between the selected genes were investigated by formulating a protein-protein interaction (PPI) network for the candidate genes using the STRING online database (https://string-db.org/) with an interaction score threshold of 0.4. The generated PPI network was displayed using Cytoscape version 3.7.1 (Shannon et al., 2003).
2.4 Biomarker identification and validation
The LASSO regression analysis was employed in conjunction with support vector machine recursive feature elimination (SVM-RFE) algorithms to define specific candidate genes; the LASSO regression analysis and the SVM-RFE algorithm were performed using the glmnet package (v 4.1-8) (Sasikumar et al., 2022) with binomial family parameters and the e1071 package (v 1.7-13) (Yang et al., 2022), respectively. Candidate biomarkers were identified by merging the genes discerned using the aforementioned methods. Subsequently, the expression of the candidate biomarkers was analyzed using the GSE56815 dataset, and their validity was confirmed using quantitative real-time polymerase chain reaction (qRT-PCR).
Blood samples belonging to 10 patients diagnosed with OP and 10 individuals lacking the condition were obtained from the Biobank of the 900th Hospital of the Joint Logistics Support Force and subjected to qRT-PCR analysis using the 2xUniversal Blue SYBR Green qPCR Master Mix (Servicebio, Wuhan, China). This study was approved by the Biomedical Ethics Committee of the 900th Hospital of the Joint Logistics Support Force (Ethics Review No. 2023-72), and all study participants provided informed consent. RNA was extracted from all 20 samples using TRIzol (Ambion, Austin, TX, United States) per manufacturer guidelines And subsequently converted into cDNA using the SureScript First Strand cDNA Synthesis Kit (Servicebio). The PCR primer sequences have been listed in Supplementary Table S2, with GAPDH serving as the internal reference gene. The expression of the candidate biomarkers was determined using the 2−ΔΔCT method (Livak and Schmittgen, 2001). Biomarkers that displayed consistent trends in both the dataset and qRT-PCR results, as well as considerable differences between the OP and control groups, were identified and documented as potential biomarkers. The mRNALocator database (http://bio-bigdata.cn/mRNALocater/) was used to predict the subcellular positioning of these biomarkers, and the RCircos tool (v. 1.2.0) (Zhang et al., 2013) was used to examine their chromosomal dispersal.
2.5 Engineering and evaluation of nomogram
The rms software (version 6.7-1) was applied to biomarkers reported in a prior study (Xu et al., 2023) to construct a nomogram using the GSE56815 dataset and evaluate the combined potency of the candidate biomarkers to prognosticate OP. The predictive accuracy of the nomogram was evaluated using calibration, decision, and ROC curves.
2.6 Gene set enrichment analysis (GSEA)
Two background gene sets—c2.cp.kegg.v7.5.1. entrez.gmt for the KEGG signaling pathway and c5.go.bp.v2023.2—were initially considered to explore the roles and pathways linked to the biomarkers in OP progression. Hs.symbols.gmt was used for GO functional enrichment from the Molecular Signatures Database (https://www.gsea-msigdb.org/gsea/msigdb). The correlation coefficients between biomarkers and other genes within the GSE56815 dataset were determined, and subsequently, the genes were organized according to their correlation coefficients to establish a gene set for enrichment analysis. The identified biomarkers were then subjected to GSEA using the clusterProfiler package.
2.7 Immune infiltration analysis
To assess variations in immune status among OP patients, 28 immune cell infiltration scores between OP and control groups from the GSE56815 dataset were initially calculated using the ssGSEA algorithm of the GSVA package (v. 1.49.8) (Hänzelmann et al., 2013). The Wilcoxon test was used to compare discrepancies in the immune infiltration levels of these 28 immune cells between the OP and control groups. Subsequently, Spearman’s correlation analysis was performed to investigate the relationship between the biomarkers and diverse immune cells.
2.8 Construction of regulatory networks
To establish regulatory networks, the NetworkAnalyst database (https://www.networkanalyst.ca/) was used to predict the transcription factors (TFs) involved in the regulation of the recognized biomarkers; the predicted TFs were then intersected with the DEGs to identify the crucial TFs. Moreover, we utilized both the miRWalk (http://mirwalk.umm.uni-heidelberg.de/) and microT (https://dianalab.e-ce.uth.gr/microt_webserver/) databases to predict biomarker-targeting microRNAs (miRNAs). Subsequently, the results from these two databases were combined to determine the key miRNAs. Finally, the Cytoscape software was used to fashion networks representing mRNA-TF and mRNA-miRNA interactions.
2.9 Drug prediction and molecular docking analysis
The potential therapeutic capabilities of the candidate biomarkers were examined using the Comparative Toxicogenomics Database (http://ctdbase.org/) to identify prospective drugs targeting biomarkers with a screening criteria of reference count ≥1. Crystal structures of receptor proteins for the recognized biomarkers were obtained from UniProt (https://www.uniprot.org/) and the Protein Data Bank (PDB) database (https://www.rcsb.org/) in the PDB format. The 3D structures of the targeted drugs were downloaded as ligands from PubChem (https://pubchem.ncbi.nlm.nih.gov/), energy minimized using the OpenBabel software (v. 2.4.1) (Mehta et al., 2014) and saved in the mol2 format. PyMOL software (v 3.3.0) (Seeliger and de Groot, 2010) was used to dehydrate, de-ligandize, and hydrogenate the receptor proteins prior to saving them. The processed receptor proteins and target drug structures were then imported into the AutoDock Tools software (v 1.5.7) (Morris et al., 2009) and converted into the pdbqt format. Finally, molecular docking was performed using the AutoDock Vina software (v 1.2.2) (Trott and Olson, 2010), and the results were visualized using PyMOL software.
2.10 Statistical analysis
R software (4.2.2 version) was used for data processing and analysis. The Wilcoxon test was used to compare variances among diverse groups. A significance level of p < 0.05 was deemed statistically noteworthy.
3 Results
3.1 Eighteen candidate genes were associated with mitochondrial-related signaling pathways
Based on differential expression analysis, 2,354 DEGs were identified between the OP and control groups. Of these, 1,597 DEGs were upregulated in OP, whereas 757 were downregulated (Figures 1A, B). Based on the intersection of 2,354 DEGs, 1,136 MRGs, and 1,548 PCD-RGs, 18 candidate genes were identified (Figure 1C). Enrichment analyses were performed for the candidate genes to preliminarily explore the biological functions in which they were implicated. The results demonstrated significant enrichment of these candidate genes in 95 GO entries, comprising 74 BPs, 17 CCs, and 4 MFs. In addition, these genes were associated with 17 KEGG pathways. Specifically, the enriched GO entries encompassed crucial biological functions such as “regulation of apoptotic signaling pathway,” “regulation of mitochondrion organization,” and “negative regulation of the apoptotic signaling pathway” (Figure 1D). Furthermore, the enriched KEGG pathways included “pathways of neurodegeneration-multiple diseases,” “apoptosis,” “cGMP-PKG signaling pathway,” and others (Figure 1E). A PPI network was constructed based on the candidate genes containing 15 nodes and 22 edges. In particular, there were more interactions between VDAC2, BCL2L1, and MCL1 and other genes (Figure 1F).
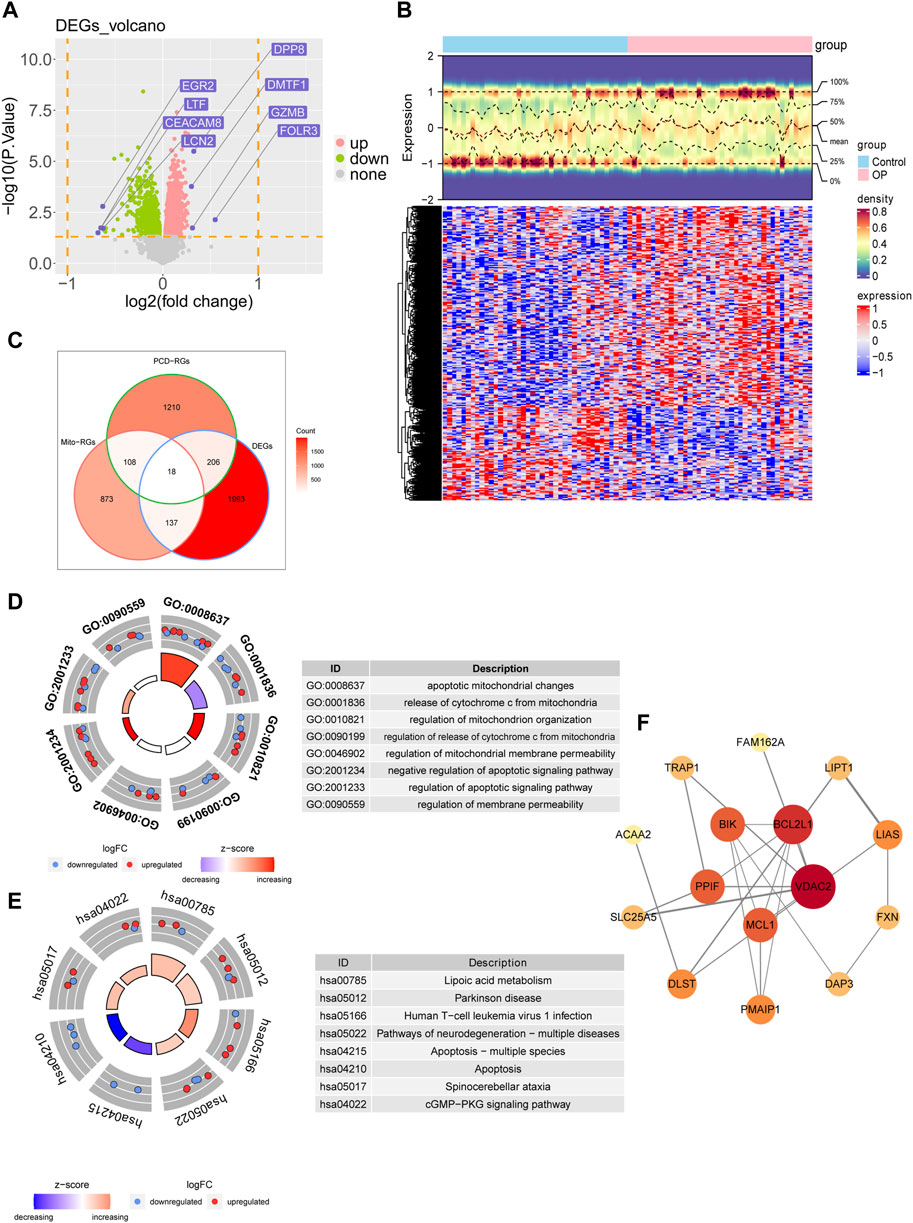
Figure 1. Preliminary identification and analysis of candidate genes. (A) Differential volcano plot analysis of the GSE56815 dataset. (B) Differential heat map analysis of the GSE56815 dataset. (C) Analysis of differential genes with Mito-RGs and PCD-RGs (D) GO enrichment analysis. (E) KEGG enrichment analysis. (F) Reconstruction of the PPI network.
3.2 Genes encoding death-associated protein 3 (DAP3), Bcl-2-interacting killer (BIK), and acetyl-CoA-acyltransferase 2 (ACAA2) were identified as biomarkers
Screening of candidate genes by LASSO regression analysis (Figure 2A) and the SVM-RFE algorithm (Figure 2B) yielded 12 and 10 characterized genes, respectively. By crossing these two parts of the characterized genes, eight candidate biomarkers were identified: DAP3, POLB, BLOC1S1, MCL1, BIK, PMAIP1, TRAP1, and ACAA2 (Figure 2C). The Wilcoxon test showed that in the OP group, ACAA2, BIK, DAP3, POLB, and TRAP1 were markedly upregulated, whereas BLOC1S1, MCL1, and PMAIP1 were visibly downregulated compared to the control group (Figure 2D). The qRT-PCR analysis indicated that DAP3, BIK, and ACAA2 exhibited expression patterns consistent with the dataset and were significantly elevated in the OP group (Figure 2E). Consequently, DAP3, BIK, and ACAA2 were identified as potential biomarkers. Subcellular and chromosomal localization analyses were performed to investigate the distribution of biomarkers. The results demonstrated that BIK and DAP3 were mainly expressed in the cytoplasm, whereas ACAA2 was mainly localized in the nucleus (Figure 2F). BIK, DAP3, and ACAA2 are located on chromosomes 22, 1, and 18, respectively (Figure 2G).
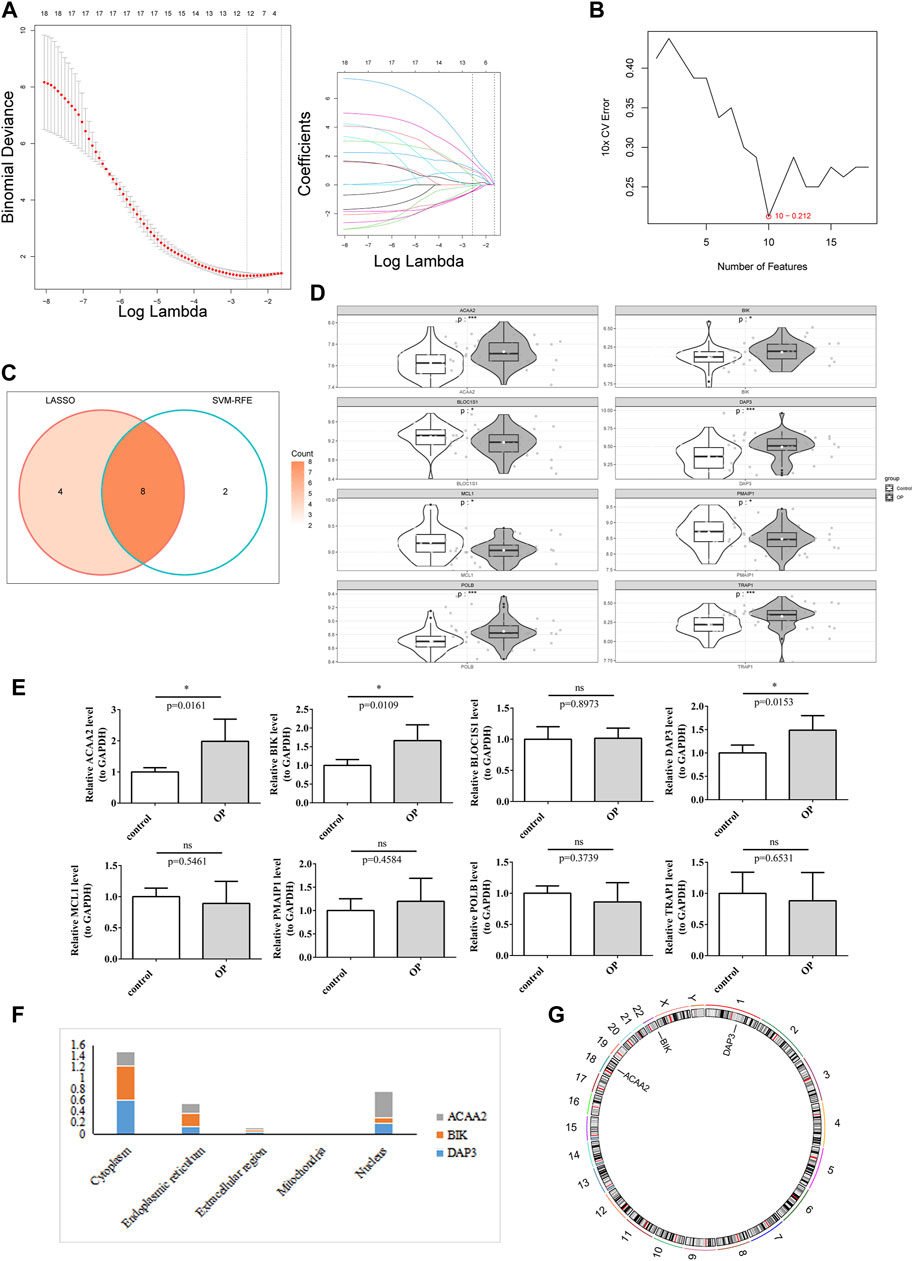
Figure 2. DAP3, BIK, and ACAA2 were identified as biomarkers. (A) LASSO regression analysis. (B) SVM-RFE algorithm regression analysis. (C) Convergence of the LASSO and SVM-RFE algorithms. (D) Expression of key genes in the training data set. White represents disease samples, gray portrays normal samples. The statistical method used is the Wilcox test. “*” indicates p < 0.05, “**” symbolizes p < 0.01, “***” signifies p < 0.001, “****” depicts p < 0.0001, and “ns” denotes no significant difference. (E) Expression of key genes in qPCR. The expression of DAP3, BIK, and ACAA2 exhibited significant disparities (p < 0.05). (F) Subcellular localization prediction of biomarkers. (G) Chromosomal localization of biomarkers.
3.3 The nomogram displayed remarkable potency in prognosticating OP
Based on the identified biomarkers DAP3, BIK, and ACAA2, a nomogram was constructed to assess the ability of the biomarkers to predict OP (Figure 3A). We also evaluated its predictive ability. Specifically, the calibration curve demonstrated a close-to-unity slope for the nomogram (Figure 3B), whereas decision curve analysis indicated that patients with OP could benefit from the constructed diagnostic model (Figure 3C). Additionally, the ROC curve exhibited an area under the curve value of 0.807 (Figure 3D). Collectively, these findings substantiate that the predictive ability of the nomogram constructed based on biomarkers for OP exhibited a certain degree of accuracy.
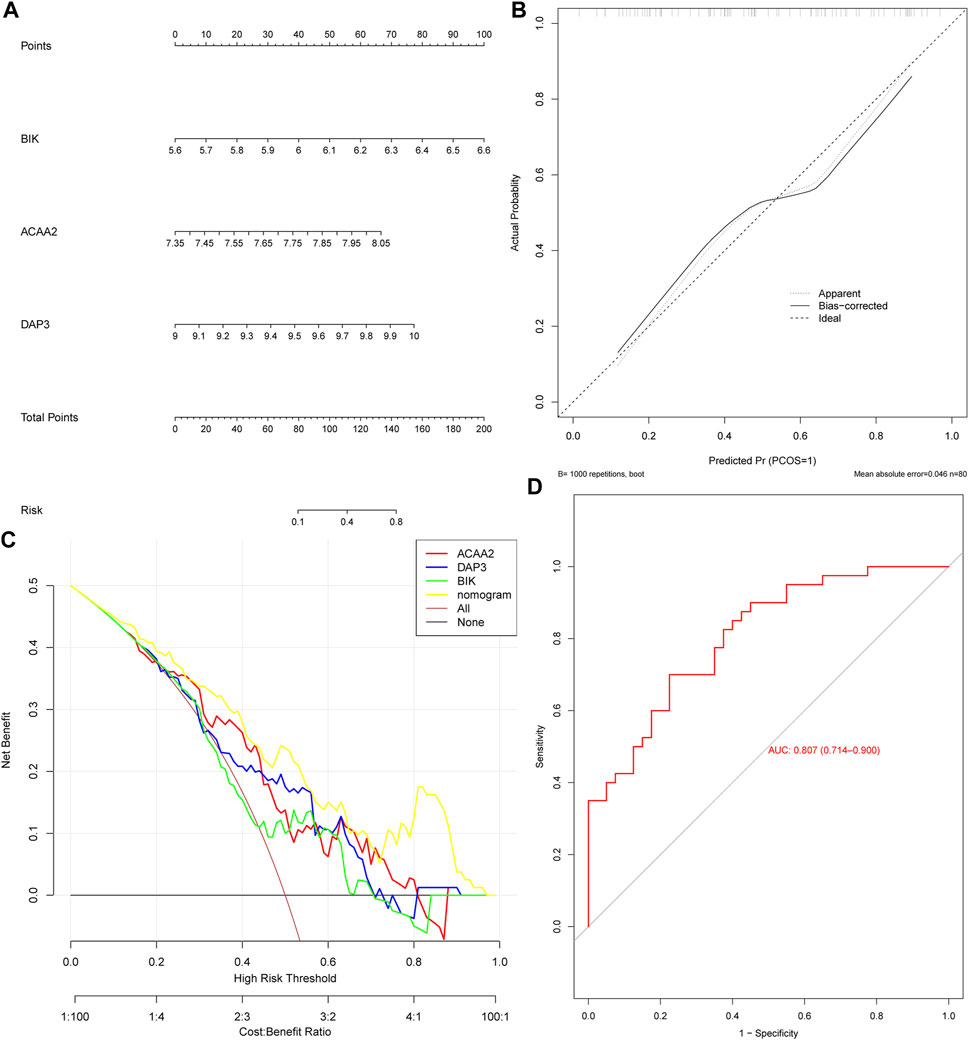
Figure 3. The nomogram exhibited excellent predictive potential for OP. (A) Nomogram construction. Evaluation of the diagnostic model of nomogram: (B) Calibration curve and (C) Decision curve. (D) ROC curve.
3.4 Biomarkers were linked to oxidative phosphorylation
GSEA was used to probe the signaling pathways involving DAP3, BIK, and ACAA2. Based on the GO functional enrichment gene set, DAP3 and BIK were co-enriched for “Cytoplasmic Translation” (Figure 4A). Based on the KEGG functional enrichment gene set, DAP3, BIK, and ACAA2 were co-enriched in “oxidative phosphorylation,” “proteasome,” and “spliceosome” (Figure 4B).
3.5 Central memory CD4+ T cells exhibited substantial negative correlation with DAP3
The distribution of the 28 immune cell infiltration scores between the OP and control groups in the GSE56815 dataset has been illustrated in Figure 5A. Significant differences were observed between the control and OP groups in terms of CD56dim natural killer cells and central memory CD4+ T cells at a significance level of p < 0.05. Specifically, compared to the control group, the infiltration scores of CD56dim natural killer cells were significantly higher in the OP group, whereas central memory CD4+ T cells exhibited an inverse relationship (Figure 5B). Furthermore, correlation analysis revealed a highly significant positive correlation between CD56dim natural killer cells and ACAA2 (cor = 0.291, p = 0.009), whereas a highly significant negative correlation was observed between central memory CD4+ T cells and DAP3 (cor = −0.381, p = 0.0004; Figure 5C; Supplementary Table S3).
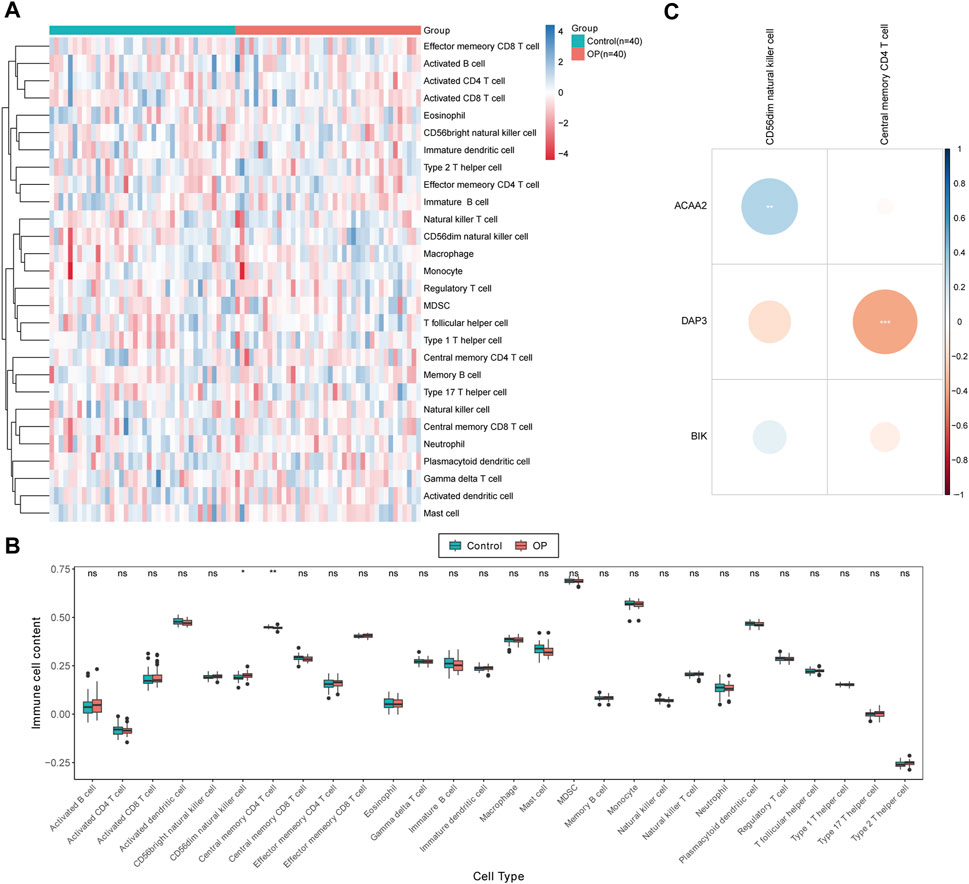
Figure 5. Immunoinfiltration analysis. (A) Immune score heat map of immune cell types in different samples obtained by using ssGSEA algorithm. (B) Box plot of immune scores acquired by using ssGSEA algorithm for immune cell types in different samples between OP patients and normal controls. The statistical method was Wilcox.test; ns indicates no significant difference, “*” symbolizes p < 0.05, “**” depicts p < 0.01. (C) Differential immune cell and biomarker correlation heat map. Correlation analysis revealed a highly significant positive correlation between CD56dim natural killer cells and ACAA2 (cor = 0.291, p = 0.009), while a highly significant negative correlation was observed between central memory CD4+ T cells and DAP3 (cor = −0.381, p = 0.0004).
3.6 DAP3, BIK, and ACAA2 were regulated by multiple factors
Using the NetworkAnalyst database, 24, 15, and 27 TFs were predicted to regulate the expression of DAP3, BIK, and ACAA2. After deduplication and merging, 49 TFs were identified. Eight key TFs were identified by overlapping 49 TFs and 2,354 DEGs (Figure 6A). Based on the key TFs and biomarkers identified, an mRNA-TF network containing 11 nodes and 12 edges was constructed. In this regulatory network, SETDB1 and ZNF281 regulate both ACAA2 and DAP3, whereas TP63 and TFAP2C regulate both DAP3 and BIK (Figure 6B). Additionally, 11 key miRNAs were identified by analyzing the intersection of the predicted miRNAs from the two databases. Specifically, there were one, four, and six miRNAs targeting BIK, DAP3, and ACAA2, respectively (Figure 6C). ACAA2 is regulated by several miRNAs, including hsa-miR-371b-5P, hsa-miR-548w, and hsa-miR-4733-5p.
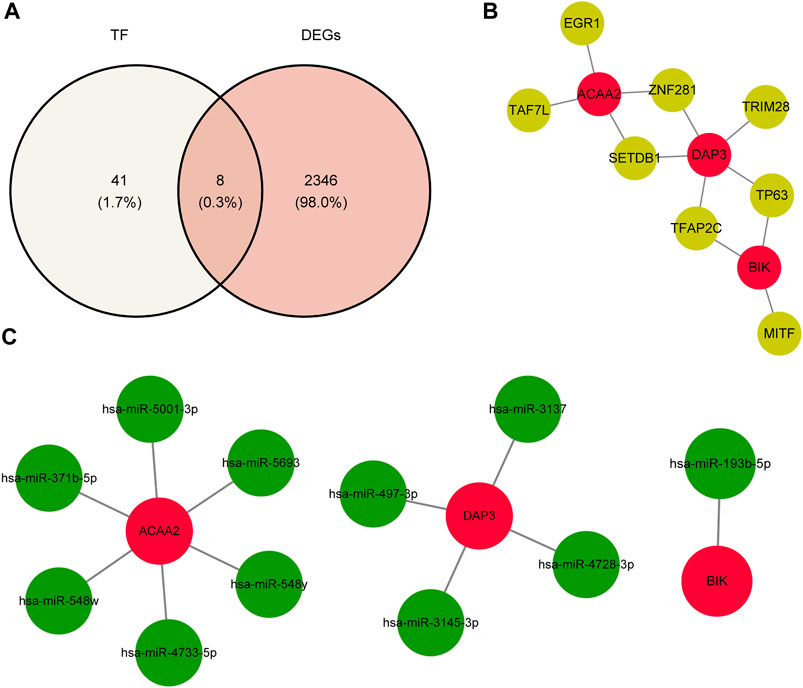
Figure 6. TF-biomarker regulatory network construction. (A) Relevant acquisition of TFs. (B) Construction of TF-biomarker network map. (C) Construction of miRNA-biomarker network map.
3.7 A stable binding force was observed between the drugs and biomarkers
Based on the screening criteria of reference count ≥1, we predicted 3, 10, and 7 drugs targeting DAP3, BIK, and ACAA2, respectively. Subsequently, a drug-biomarker network containing 18 nodes and 20 edges was constructed. Aflatoxin B1 simultaneously targeted DAP3, BIK, and ACAA2 (Figure 7A; Supplementary Table S4). Based on the reference count, estradiol, valproic acid, and CGP52608 were selected for molecular docking analysis using BIK, ACAA2, and DAP3. The results revealed the formation of covalent bonds between BIK and amino acid residues ARG-102, ILE-04, and TRP-84 of estradiol (binding energy = −5.9 kcal/mol). ACAA2 was found to form covalent bonds with amino acid residues SER-251 and ALA-322 of valproic acid (binding energy = −5.0 kcal/mol), while DAP3 formed covalent bonds with amino acid residues ASN-292 and ASP-238 of CGP52608 (binding energy = −5.2 kcal/mol; Figure 7B; Table 1).
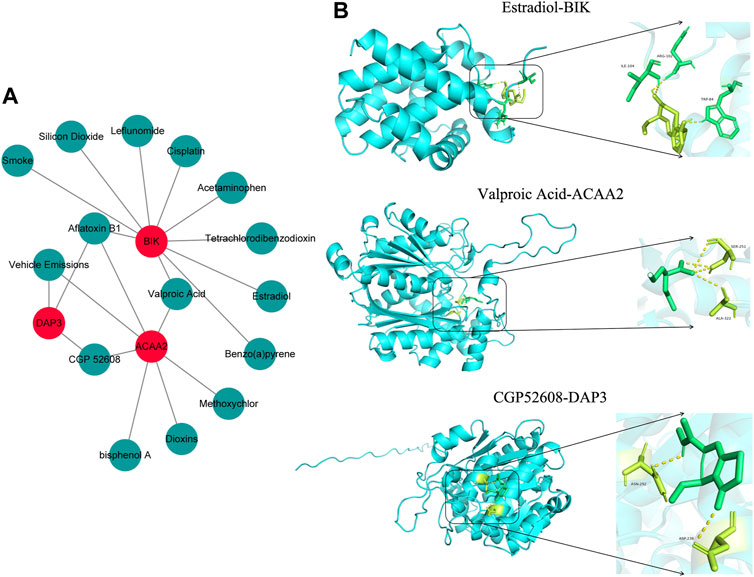
Figure 7. Drug prediction and molecular docking. (A) Drug-biomarker network map. (B) Visualization of drug molecular docking results.
4 Discussion
OP is the primary cause of morbidity and mortality among the elderly worldwide. Several studies have shown that apoptosis is a key factor in OP (Yu and Wang, 2000; Zhang et al., 2019). Mitochondria play a vital role in preserving cellular functions, such as redox balance, calcium equilibrium, energy generation, metabolic processes, and cell demise (Spinelli and Haigis, 2018; Ventura-Clapier et al., 2019). Studies have suggested that irregularities in mitochondrial mass, marked by abnormal oxidative stress, dynamics, and autophagy, have a significant impact on the management of apoptosis linked to bone metabolism (Indo et al., 2017; Cai et al., 2019; Jin et al., 2020; Xu et al., 2021; Li W. et al., 2023). This implies that targeting mitochondrial function and apoptosis may be crucial therapeutic strategies for the treatment of OP.
4.1 Enrichment pathways of candidate genes
This study identified 18 candidate genes that are differentially expressed and involved in mitochondria and PCD. The functions of these genes were analyzed through KEGG and GO enrichment pathways, including apoptosis-related pathways, mitochondrial function-related pathways, and the cGMP-PKG signaling pathway. Apoptosis, a type of PCD, is a controlled cellular demise process triggered by the organism to maintain balance and includes death receptors such as TNFR and Fas in the extrinsic pathway of apoptosis and the intrinsically regulated apoptotic pathway mediated by mitochondria (Maiuri et al., 2007; Fischer and Haffner-Luntzer, 2022). Signaling pathways like PI3K/Akt, ERK5, JNK, Wnt/β-catenin, NF-κB, and P38 are involved in mitochondrial pathway apoptosis during OP formation, regulating caspase, Bcl-2 family proteins, and other key targets to affect OB apoptosis (Liu et al., 2024). Studies have shown that the PI3K pathway promotes OB proliferation and differentiation while inhibiting apoptosis in the mitochondrial pathway, ultimately promoting bone formation and increasing bone mass at the tissue level (Meng et al., 2023). JNK is not only involved in inducing apoptosis through the endoplasmic reticulum pathway but also plays a crucial role in inducing apoptosis through the mitochondrial pathway (Guo et al., 2016). The inhibition of the JNK pathway can effectively suppress OB apoptosis, which is beneficial for the treatment of OP. Recent studies have indicated that targeting the NO-cGMP-PKG pathway is pivotal in regulating bone homeostasis and has the potential as an approach for OP treatment (Pal et al., 2020; Friebe et al., 2023). Although nitrates initially garnered attention due to their side effects, soluble guanosine receptor agonists are now FDA-approved for the treatment of pulmonary hypertension and erectile dysfunction (Kim et al., 2021).
4.2 Functional analysis of biomarkers
Our OP-related datasets were acquired from the GEO database, whereas the literature was referenced to obtain PCD-RGs and mito-RGs. Bioinformatic analysis and machine learning techniques were used to identify the OP biomarkers ACAA2, DAP3, and BIK. ACAA2 assumes a pivotal role in fatty acid oxidation. The enzyme encoded by ACAA2 catalyzes the final step of the fatty acid β-oxidation pathway, contributing to the elongation and degradation of mitochondrial fatty acids. Acetyl-CoA, the final result of lipid β-oxidation, is also important in the creation of ketone bodies. ACAA2 contains an unremovable amino-terminal signal for targeting and is associated with a range of biological functions. For instance, it influences preadipocyte differentiation in sheep, affecting body fat deposition and the lean meat rate (Han et al., 2021). Excessive accumulation of body fat is associated with obesity, diabetes, fatty liver disease, gallbladder disease, hypertension, and endocrine disorders. Although the direct association between ACAA2 and bone metabolism remains unclear, its role in fatty acid oxidation suggests potential connections with metabolic diseases, such as obesity and diabetes. These conditions may indirectly influence bone metabolism (Villuendas et al., 2006). Research indicates that ACAA2 expression levels are correlated with the rate of glycolysis and are significant in type 2 diabetes progression (Zhao et al., 2023). DAP3 is a serine/threonine protein kinase known for its pro-apoptotic activity and is primarily associated with cell death. In vitro experiments have demonstrated its capability to induce apoptosis, steer recipient cells toward programmed death, and impede cell growth and proliferation (Wazir et al., 2015). Moreover, the cell cycle can be controlled by DAP3 through the inhibition of cell proliferation-associated genes such as cyclin D1 and CDK2, which in turn inhibits the progression of the normal cell cycle and result in cell cycle arrest at either the G0/G1 or G2/M phase, ultimately suppressing abnormal cell growth (Song et al., 2023). However, direct evidence linking DAP3 to bone metabolism is lacking. Bone metabolism is a multifaceted biological process that involves various cells, hormones, and molecules. Although some proteins related to apoptosis and cell cycle control may indirectly affect bone metabolism, extensive investigation is required to clarify the precise function of DAP3 in this mechanism. BIK constitutes part of the BH3-only protein group of the BCL2 homology domain 3 and assumes a role in the mitochondrial apoptotic pathway (Kale et al., 2018). The protein encoded by BIK affects cell viability or apoptosis by interacting with other members of the BCL2 family (Hatok and Racay, 2016). A burgeoning number of studies have confirmed the significance of apoptosis within the mitochondrial pathway in the regulation of bone metabolism (Fortner et al., 2017; Wang et al., 2018; Gao et al., 2022). Therefore, we postulate that targeting consistent apoptosis through enhancement of mitochondrial status represents a pivotal therapeutic strategy for addressing osteoporosis.
4.3 GSEA enrichment analysis and mechanism of action
GSEA enrichment analysis revealed that the three common genes were closely associated with oxidative phosphorylation pathway signaling. The cellular energy metabolism pathways consists of glycolysis, which is dominated by anaerobic respiration, and oxidative phosphorylation, which is dominated by aerobic respiration. The oxidative phosphorylation pathway is particularly relevant to osteogenic and osteoclastic differentiation. Shares et al. (2018) observed a significant increase in endogenous ATP and oxygen consumption during osteogenic differentiation of MSCs, indicating a reliance on mitochondrial oxidative phosphorylation for energy. Snoeck (2017) also found that the energy for osteogenic and OB differentiation of MSCs is mainly provided through the oxidative phosphorylation pathway. Furthermore, Kim et al., 2021; Ledesma-Colunga et al., 2023 demonstrated that inhibition of the mitochondrial oxidative phosphorylation energy supply pathway hindered osteoclast differentiation, underscoring the crucial role of this pathway in osteoclast function. In conclusion, targeting the oxidative fatty acid metabolic pathway is imperative for enhancing energy supply during the osteoblastic process, thereby offering a promising therapeutic approach for managing osteoporosis.
4.4 Immune infiltration and drug prediction analysis
The bone marrow contains hematopoietic stem cells and various mature immune cells that influence OBs and osteoclasts, which are crucial for bone structural integrity and repair. This interaction between cells leads to bone remodeling, a dynamic process of formation and resorption (Brylka and Schinke, 2019; Saxena et al., 2021). Our research uncovered noteworthy differences in CD56dim natural killer cells and central memory CD4+ T cells between the control and OP cohorts. Natural killer cells are part of the innate immune system and are associated with cellular senescence, whereas CD4+ T cells contribute to bone loss through the osteoimmune system (Antonangeli et al., 2019). The discovery that activated CD4+ T cells regulate osteoclast bone resorption is one of the driving forces for the development of bone immunology (Okamoto and Takayanagi, 2023). Through further elucidation of the interplay between bone and the immune system, insights into the intricate relationship between osteogenesis and inflammation in the realm of bone immunology are unveiled. Consequently, as investigations into helper T cell subsets and rare lymphoid cells progress within the field of immunology, it is increasingly evident that diverse types of T cells exert multifaceted effects on bone metabolism contingent upon the immune milieu. Comprehending the distinct impacts of T cells on bone is pivotal for unraveling bone immunomodulatory networks across various biological contexts.
The drug prediction results suggested stable binding between the drugs and biomarkers, with estrogen demonstrating a protective effect on human bone OBs by exhibiting an anti-apoptotic effect. Estrogen inhibits OB apoptosis and promotes bone formation (Lu and Tian, 2023). Additionally, studies have shown that valproate prevents glucocorticoid-induced femoral head necrosis in rats (Zhou et al., 2018). Additionally, prior research has verified the anti-osteoporotic potential of most drug predictions, indicating the clinical significance of predicted biomarkers.
4.5 Limitations and prospects
There are certain limitations associated with this study. The relatively small number of clinical samples may introduce bias into the outcomes. Additionally, there is a lack of support for further validation through animal experiments. To address these deficiencies, we intend to conduct animal studies to enhance the reliability of the biomarkers and will continue monitoring the progress of ACAA2, DAP3, and BIK in relation to osteoporosis.
5 Conclusion
This study used bioinformatics and qRT-PCR analyses to identify three biomarkers associated with PCD and mitochondria in OP, namely, ACAA2, DAP3, and BIK. Enrichment and immune infiltration analyses, regulatory network construction, drug prediction, and additional assessments were performed to investigate the potential mechanisms of action of these biomarkers.
Data availability statement
The codes for analysis and data are available online at https://github.com/yangxiu1992/. The names of the repository/repositories and accession number(s) can be found in the article/Supplementary Material.
Ethics statement
The studies involving humans were approved by the Biomedical Ethics Committee of the 900th Hospital of the Joint Logistics Support Force (Ethics Review No. 2023-72). The studies were conducted in accordance with the local legislation and institutional requirements. The participants provided their written informed consent to participate in this study.
Author contributions
XY: Investigation, Methodology, Writing–original draft. Z-CZ: Data curation, Investigation, Writing–review and editing. Y-NL: Data curation, Investigation, Writing–review and editing. H-LC: Investigation, Methodology, Writing–review and editing. H-SW: Investigation, Methodology, Writing–review and editing. TL: Investigation, Methodology, Writing–review and editing. Q-QC: Supervision, Writing–review and editing. J-SC: Supervision, Writing–review and editing. W-BH: Conceptualization, Funding acquisition, Supervision, Writing–original draft, Writing–review and editing.
Funding
The author(s) declare that financial support was received for the research, authorship, and/or publication of this article. This research was funded by Joint Funds for the innovation of science and Technology, Fujian province (Grant number: 2023Y9323), the Startup Fund for scientific research, Fujian Medical University (Grant number: 2023QH1355) and the Hospital-Level Project of the 900th Hospital of the Joint Logistics Support Force of the PLA (Grant number: 2021JQ06).
Acknowledgments
We would like to express their gratitude to W-BH, J-SC, and Q-QC for their proficient guidance during this study. We would like to thank Editage (www.editage.cn) for English language editing.
Conflict of interest
The authors declare that the research was conducted in the absence of any commercial or financial relationships that could be construed as a potential conflict of interest.
Publisher’s note
All claims expressed in this article are solely those of the authors and do not necessarily represent those of their affiliated organizations, or those of the publisher, the editors and the reviewers. Any product that may be evaluated in this article, or claim that may be made by its manufacturer, is not guaranteed or endorsed by the publisher.
Supplementary material
The Supplementary Material for this article can be found online at: https://www.frontiersin.org/articles/10.3389/fgene.2024.1439171/full#supplementary-material
Abbreviations
BMD, bone mineral density; BMSCs, bone marrow mesenchymal stem cells; BP, biological processes; CC, cellular components; DAP3, death-associated protein 3; DEGs, differentially expressed genes; GEO, Gene Expression Omnibus; GO, Gene Ontology; GSEA, gene set enrichment analysis; KEGG, Kyoto Encyclopedia of Genes and Genomes; MF, molecular functions; miRNAs, microRNAs; MRGs, mortality factor-related genes; OB, osteoblast; OP, osteoporosis; PCD, programmed cell death; PCD-RGs, genes linked to programmed cell death; PDB, Protein Data Bank; PPI, protein-protein interaction; qRT-PCR, real-time polymerase chain reaction; ROS, reactive oxygen species; SVM-RFE, support vector machine recursive feature elimination; TFs, transcription factors.
References
Adejuyigbe, B., Kallini, J., Chiou, D., and Kallini, J. R. (2023). Osteoporosis: molecular pathology, diagnostics, and therapeutics. Int. J. Mol. Sci. 24, 14583. doi:10.3390/ijms241914583
Antonangeli, F., Zingoni, A., Soriani, A., and Santoni, A. (2019). Senescent cells: living or dying is a matter of NK cells. J. Leukoc. Biol. 105, 1275–1283. doi:10.1002/JLB.MR0718-299R
Awuti, K., Wang, X., Sha, L., and Leng, X. (2022). Exploring the regulatory mechanism of osteoporosis based on intestinal flora: a review. Med. (Baltim) 101, e32499. doi:10.1097/MD.0000000000032499
Bedoui, S., Herold, M. J., and Strasser, A. (2020). Emerging connectivity of programmed cell death pathways and its physiological implications. Nat. Rev. Mol. Cell Biol. 21, 678–695. doi:10.1038/s41580-020-0270-8
Brylka, L. J., and Schinke, T. (2019). Chemokines in physiological and pathological bone remodeling. Front. Immunol. 10, 2182. doi:10.3389/fimmu.2019.02182
Cai, W. J., Chen, Y., Shi, L. X., Cheng, H. R., Banda, I., Ji, Y. H., et al. (2019). AKT-GSK3β signaling pathway regulates mitochondrial dysfunction-associated OPA1 cleavage contributing to osteoblast apoptosis: preventative effects of hydroxytyrosol. Oxid. Med. Cell Longev. 2019, 4101738. doi:10.1155/2019/4101738
Daponte, V., Henke, K., and Drissi, H. (2024). Current perspectives on the multiple roles of osteoclasts: mechanisms of osteoclast-osteoblast communication and potential clinical implications. eLife 13, e95083. doi:10.7554/eLife.95083
Ensrud, K. E., and Crandall, C. J. (2024). Osteoporosis. Ann. Intern Med. 177, ITC1–ITC16. doi:10.7326/AITC202401160
Fischer, V., and Haffner-Luntzer, M. (2022). Interaction between bone and immune cells: implications for postmenopausal osteoporosis. Semin. Cell Dev. Biol. 123, 14–21. doi:10.1016/j.semcdb.2021.05.014
Flowers, S., Kothari, R., Torres Cleuren, Y. N., Alcorn, M. R., Ewe, C. K., Alok, G., et al. (2023). Regulation of defective mitochondrial DNA accumulation and transmission in C. elegans by the programmed cell death and aging pathways. Elife 12, e79725. doi:10.7554/elife.79725
Fortner, R. T., Sarink, D., Schock, H., Johnson, T., Tjønneland, A., Olsen, A., et al. (2017). Osteoprotegerin and breast cancer risk by hormone receptor subtype: a nested case-control study in the EPIC cohort. BMC Med. 15, 26. doi:10.1186/s12916-017-0786-8
Friebe, A., Kraehling, J. R., Russwurm, M., Sandner, P., and Schmidtko, A. (2023). The 10th International Conference on cGMP 2022: recent trends in cGMP research and development-meeting report. Naunyn Schmiedebergs Arch Pharmacol. The 10th Int. Conf. cGMP 396, 1669–1686. doi:10.1007/s00210-023-02484-8
Gao, C. H., Yu, G., and Cai, P. (2021). ggVennDiagram: an intuitive, easy-to-use, and highly customizable R package to generate venn diagram. Front. Genet. 12, 706907. doi:10.3389/fgene.2021.706907
Gao, X., Yu, X., Zhang, C., Wang, Y., Sun, Y., Sun, H., et al. (2022). Telomeres and mitochondrial metabolism: implications for cellular senescence and age-related diseases. Stem Cell Rev. Rep. 18, 2315–2327. doi:10.1007/s12015-022-10370-8
Gu, Z., Eils, R., and Schlesner, M. (2016). Complex heatmaps reveal patterns and correlations in multidimensional genomic data. Bioinformatics 32, 2847–2849. doi:10.1093/bioinformatics/btw313
Guo, C., Yang, X. G., Wang, F., and Ma, X. Y. (2016). IL-1α induces apoptosis and inhibits the osteoblast differentiation of MC3T3-E1 cells through the JNK and p38 MAPK pathways. Int. J. Mol. Med. 38, 319–327. doi:10.3892/ijmm.2016.2606
Gustavsson, E. K., Zhang, D., Reynolds, R. H., Garcia-Ruiz, S., and Ryten, M. (2022). Ggtranscript: an R package for the visualization and interpretation of transcript isoforms using ggplot2. Bioinformatics 38, 3844–3846. doi:10.1093/bioinformatics/btac409
Han, F., Zhou, L., Zhao, L., Wang, L., Liu, L., Li, H., et al. (2021). Identification of miRNA in sheep intramuscular fat and the role of miR-193a-5p in proliferation and differentiation of 3T3-L1. Front. Genet. 12, 633295. doi:10.3389/fgene.2021.633295
Hänzelmann, S., Castelo, R., and Guinney, J. (2013). GSVA: gene set variation analysis for microarray and RNA-seq data. BMC Bioinforma. 14, 7. doi:10.1186/1471-2105-14-7
Hatok, J., and Racay, P. (2016). Bcl-2 family proteins: Master regulators of cell survival. Biomol. Concepts 7, 259–270. doi:10.1515/bmc-2016-0015
Indo, H. P., Hawkins, C. L., Nakanishi, I., Matsumoto, K. I., Matsui, H., Suenaga, S., et al. (2017). Role of mitochondrial reactive oxygen species in the activation of cellular signals, molecules, and function. Handb. Exp. Pharmacol. 240, 439–456. doi:10.1007/164_2016_117
Jin, W., Zhu, X., Yao, F., Xu, X., Chen, X., Luo, Z., et al. (2020). Cytoprotective effect of Fufang Lurong Jiangu capsule against hydrogen peroxide-induced oxidative stress in bone marrow stromal cell-derived osteoblasts through the Nrf2/HO-1 signaling pathway. Biomed. Pharmacother. 121, 109676. doi:10.1016/j.biopha.2019.109676
Kale, J., Osterlund, E. J., and Andrews, D. W. (2018). BCL-2 family proteins: changing partners in the dance towards death. Cell Death Differ. 25, 65–80. doi:10.1038/cdd.2017.186
Kim, S. M., Yuen, T., Iqbal, J., Rubin, M. R., and Zaidi, M. (2021). The NO-cGMP-PKG pathway in skeletal remodeling. Ann. N. Y. Acad. Sci. 1487, 21–30. doi:10.1111/nyas.14486
Ledesma-Colunga, M. G., Passin, V., Lademann, F., Hofbauer, L. C., and Rauner, M. (2023). Novel Insights into osteoclast energy metabolism. Curr. Osteoporos. Rep. 21, 660–669. doi:10.1007/s11914-023-00825-3
Li, W., Jiang, W. S., Su, Y. R., Tu, K. W., Zou, L., Liao, C. R., et al. (2023b). PINK1/Parkin-mediated mitophagy inhibits osteoblast apoptosis induced by advanced oxidation protein products. Cell Death Dis. 14, 88. doi:10.1038/s41419-023-05595-5
Li, Z., Li, D., Chen, R., Gao, S., Xu, Z., and Li, N. (2023a). Cell death regulation: a new way for natural products to treat osteoporosis. Pharmacol. Res. 187, 106635. doi:10.1016/j.phrs.2022.106635
Liu, J., Gao, Z., and Liu, X. (2024). Mitochondrial dysfunction and therapeutic perspectives in osteoporosis. Front. Endocrinol. (Lausanne) 15, 1325317. doi:10.3389/fendo.2024.1325317
Livak, K. J., and Schmittgen, T. D. (2001). Analysis of relative gene expression data using real-time quantitative PCR and the 2(-Delta Delta C(T)) Method. Methods 25, 402–408. doi:10.1006/meth.2001.1262
Lu, L., and Tian, L. (2023). Postmenopausal osteoporosis coexisting with sarcopenia: the role and mechanisms of estrogen. J. Endocrinol. 259, e230116. doi:10.1530/JOE-23-0116
Maiuri, M. C., Zalckvar, E., Kimchi, A., and Kroemer, G. (2007). Self-eating and self-killing: crosstalk between autophagy and apoptosis. Nat. Rev. Mol. Cell Biol. 8, 741–752. doi:10.1038/nrm2239
Mehta, A., Sonam, S., Gouri, I., Loharch, S., Sharma, D. K., and Parkesh, R. (2014). SMMRNA: a database of small molecule modulators of RNA. Nucleic Acids Res. 42, D132–D141. doi:10.1093/nar/gkt976
Meng, K., Liu, Y., Ruan, L., Chen, L., Chen, Y., and Liang, Y. (2023). Suppression of apoptosis in osteocytes, the potential way of natural medicine in the treatment of osteonecrosis of the femoral head. Biomed. Pharmacother. 162, 114403. doi:10.1016/j.biopha.2023.114403
Morris, G. M., Huey, R., Lindstrom, W., Sanner, M. F., Belew, R. K., Goodsell, D. S., et al. (2009). AutoDock4 and AutoDockTools4: automated docking with selective receptor flexibility. J. Comput. Chem. 30, 2785–2791. doi:10.1002/jcc.21256
Okamoto, K., and Takayanagi, H. (2023). Effect of T cells on bone. Bone 168, 116675. doi:10.1016/j.bone.2023.116675
Pal, S., Rashid, M., Singh, S. K., Porwal, K., Singh, P., Mohamed, R., et al. (2020). Skeletal restoration by phosphodiesterase 5 inhibitors in osteopenic mice: evidence of osteoanabolic and osteoangiogenic effects of the drugs. Bone 135, 115305. doi:10.1016/j.bone.2020.115305
Qin, H., Abulaiti, A., Maimaiti, A., Abulaiti, Z., Fan, G., Aili, Y., et al. (2023). Integrated machine learning survival framework develops a prognostic model based on inter-crosstalk definition of mitochondrial function and cell death patterns in a large multicenter cohort for lower-grade glioma. J. Transl. Med. 21, 588. doi:10.1186/s12967-023-04468-x
Qin, X., Zhao, Y., Gong, J., Huang, W., Su, H., Yuan, F., et al. (2019). Berberine protects glomerular podocytes via inhibiting drp1-mediated mitochondrial fission and dysfunction. Theranostics 9, 1698–1713. doi:10.7150/thno.30640
Reid, I. R., and Billington, E. O. (2022). Drug therapy for osteoporosis in older adults. Lancet 399, 1080–1092. doi:10.1016/S0140-6736(21)02646-5
Ritchie, M. E., Phipson, B., Wu, D., Hu, Y., Law, C. W., Shi, W., et al. (2015). Limma powers differential expression analyses for RNA-sequencing and microarray studies. Nucleic Acids Res. 43, e47. doi:10.1093/nar/gkv007
Sasikumar, D., Takano, Y., Zhao, H., Kohara, R., Hamada, M., Kobori, Y., et al. (2022). Caging and photo-triggered uncaging of singlet oxygen by excited state engineering of electron donor-acceptor-linked molecular sensors. Sci. Rep. 12, 11371. doi:10.1038/s41598-022-15054-4
Saxena, Y., Routh, S., and Mukhopadhaya, A. (2021). Immunoporosis: role of innate immune cells in osteoporosis. Front. Immunol. 12, 687037. doi:10.3389/fimmu.2021.687037
Seeliger, D., and de Groot, B. L. (2010). Ligand docking and binding site analysis with PyMOL and Autodock/Vina. J. Comput. Aided Mol. Des. 24, 417–422. doi:10.1007/s10822-010-9352-6
Shannon, P., Markiel, A., Ozier, O., Baliga, N. S., Wang, J. T., Ramage, D., et al. (2003). Cytoscape: a software environment for integrated models of biomolecular interaction networks. Genome Res. 13, 2498–2504. doi:10.1101/gr.1239303
Shares, B. H., Busch, M., White, N., Shum, L., and Eliseev, R. A. (2018). Active mitochondria support osteogenic differentiation by stimulating β-catenin acetylation. J. Biol. Chem. 293, 16019–16027. doi:10.1074/jbc.RA118.004102
Snoeck, H. W. (2017). Mitochondrial regulation of hematopoietic stem cells. Curr. Opin. Cell Biol. 49, 91–98. doi:10.1016/j.ceb.2017.12.010
Song, H., Liu, H., Wang, X., Yang, Y., Zhao, X., Jiang, W. G., et al. (2023). Death-associated protein 3 in cancer-discrepant roles of DAP3 in tumours and molecular mechanisms. Front. Oncol. 13, 1323751. doi:10.3389/fonc.2023.1323751
Spinelli, J. B., and Haigis, M. C. (2018). The multifaceted contributions of mitochondria to cellular metabolism. Nat. Cell Biol. 20, 745–754. doi:10.1038/s41556-018-0124-1
Trott, O., and Olson, A. J. (2010). AutoDock Vina: improving the speed and accuracy of docking with a new scoring function, efficient optimization, and multithreading. J. Comput. Chem. 31, 455–461. doi:10.1002/jcc.21334
van der Burgh, A. C., de Keyser, C. E., Zillikens, M. C., and Stricker, B. H. (2021). The effects of osteoporotic and non-osteoporotic medications on fracture risk and bone mineral density. Drugs 81, 1831–1858. doi:10.1007/s40265-021-01625-8
Ventura-Clapier, R., Piquereau, J., Veksler, V., and Garnier, A. (2019). Estrogens, estrogen receptors effects on cardiac and skeletal muscle mitochondria. Front. Endocrinol. (Lausanne) 10, 557. doi:10.3389/fendo.2019.00557
Villuendas, G., Botella-Carretero, J. I., López-Bermejo, A., Gubern, C., Ricart, W., Fernández-Real, J. M., et al. (2006). The ACAA-insertion/deletion polymorphism at the 3′ UTR of the IGF-II receptor gene is associated with type 2 diabetes and surrogate markers of insulin resistance. Eur. J. Endocrinol. 155, 331–336. doi:10.1530/eje.1.02217
Walker, M. D., and Shane, E. (2023). Postmenopausal osteoporosis. N. Engl. J. Med. 389, 1979–1991. doi:10.1056/NEJMcp2307353
Wang, F. S., Wu, R. W., Chen, Y. S., Ko, J. Y., Jahr, H., and Lian, W. S. (2021). Biophysical modulation of the mitochondrial metabolism and redox in bone homeostasis and osteoporosis: how biophysics converts into bioenergetics. Antioxidants (Basel) 10, 1394. doi:10.3390/antiox10091394
Wang, W. D., Kang, W. B., Zhou, X. Q., Yao, G. F., and Wang, X. J. (2018). Mitochondrial protein OPA mediates osteoporosis induced by radiation through the P38 signaling pathway. Eur. Rev. Med. Pharmacol. Sci. 22, 8091–8097. doi:10.26355/eurrev_201812_16499
Wang, Z., Jiang, H., Chen, S., Du, F., and Wang, X. (2012). The mitochondrial phosphatase PGAM5 functions at the convergence point of multiple necrotic death pathways. Cell 148, 228–243. doi:10.1016/j.cell.2011.11.030
Wazir, U., Orakzai, M. M., Khanzada, Z. S., Jiang, W. G., Sharma, A. K., Kasem, A., et al. (2015). The role of death-associated protein 3 in apoptosis, anoikis and human cancer. Cancer Cell Int. 15, 39. doi:10.1186/s12935-015-0187-z
Wu, T., Hu, E., Xu, S., Chen, M., Guo, P., Dai, Z., et al. (2021). clusterProfiler 4.0: a universal enrichment tool for interpreting omics data. Innovation (Camb) 2, 100141. doi:10.1016/j.xinn.2021.100141
Xu, J., Yang, T., Wu, F., Chen, T., Wang, A., and Hou, S. (2023). A nomogram for predicting prognosis of patients with cervical cerclage. Heliyon 9, e21147. doi:10.1016/j.heliyon.2023.e21147
Xu, K., Lu, C., Ren, X., Wang, J., Xu, P., and Zhang, Y. (2021). Overexpression of HIF-1α enhances the protective effect of mitophagy on steroid-induced osteocytes apoptosis. Environ. Toxicol. 36, 2123–2137. doi:10.1002/tox.23327
Yang, L., Pan, X., Zhang, Y., Zhao, D., Wang, L., Yuan, G., et al. (2022). Bioinformatics analysis to screen for genes related to myocardial infarction. Front. Genet. 13, 990888. doi:10.3389/fgene.2022.990888
Yu, B., and Wang, C. Y. (2000). Osteoporosis and periodontal diseases – an update on their association and mechanistic links. Periodontol 89, 99–113. doi:10.1111/prd.12422
Zhang, G., Liu, Z., Li, Z., Zhang, B., Yao, P., and Qiao, Y. (2023). Therapeutic approach of natural products that treat osteoporosis by targeting epigenetic modulation. Front. Genet. 14, 1182363. doi:10.3389/fgene.2023.1182363
Zhang, H., Meltzer, P., and Davis, S. (2013). RCircos: an R package for Circos 2D track plots. BMC Bioinforma. 14, 244. doi:10.1186/1471-2105-14-244
Zhang, Q., Zhao, L., Shen, Y., He, Y., Cheng, G., Yin, M., et al. (2019). Curculigoside protects against excess-iron-induced bone loss by attenuating Akt-FoxO1-dependent oxidative damage to mice and osteoblastic MC3T3-E1 cells. Oxid. Med. Cell Longev. 2019, 9281481. doi:10.1155/2019/9281481
Zhao, R., Xiong, C., Zhao, Z., Zhang, J., Huang, Y., Xie, Z., et al. (2023). Exploration of the shared hub genes and biological mechanism in osteoporosis and type 2 diabetes mellitus based on machine learning. Biochem. Genet. 61, 2531–2547. doi:10.1007/s10528-023-10390-0
Zheng, C. X., Sui, B. D., Qiu, X. Y., Hu, C. H., and Jin, Y. (2020). Mitochondrial regulation of stem cells in bone homeostasis. Trends Mol. Med. 26, 89–104. doi:10.1016/j.molmed.2019.04.008
Keywords: osteoporosis, programmed cell death, mitochondria, nomogram, machine learning
Citation: Yang X, Zhang Z-C, Lu Y-N, Chen H-L, Wang H-S, Lin T, Chen Q-Q, Chen J-S and He W-B (2024) Identification and experimental validation of programmed cell death- and mitochondria-associated biomarkers in osteoporosis and immune microenvironment. Front. Genet. 15:1439171. doi: 10.3389/fgene.2024.1439171
Received: 06 June 2024; Accepted: 08 July 2024;
Published: 26 July 2024.
Edited by:
Bufu Tang, Fudan University, ChinaReviewed by:
Guoqing Li, Beijing Jishuitan Hospital, ChinaXu Liang, Nanjing Drum Tower Hospital, China
Copyright © 2024 Yang, Zhang, Lu, Chen, Wang, Lin, Chen, Chen and He. This is an open-access article distributed under the terms of the Creative Commons Attribution License (CC BY). The use, distribution or reproduction in other forums is permitted, provided the original author(s) and the copyright owner(s) are credited and that the original publication in this journal is cited, in accordance with accepted academic practice. No use, distribution or reproduction is permitted which does not comply with these terms.
*Correspondence: Jin-Shui Chen, cjswater1@163.com; Wu-Bing He, hewb120@sina.com
†These authors have contributed equally to this work and share first authorship