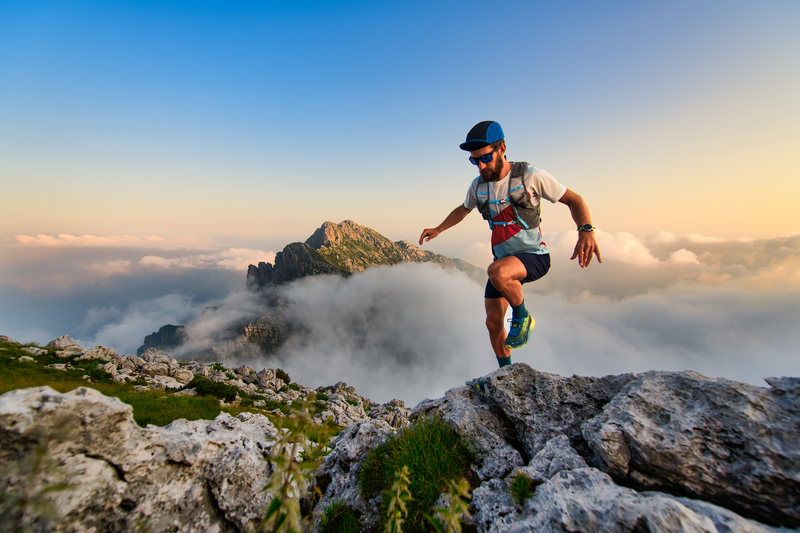
95% of researchers rate our articles as excellent or good
Learn more about the work of our research integrity team to safeguard the quality of each article we publish.
Find out more
ORIGINAL RESEARCH article
Front. Genet. , 25 July 2024
Sec. Livestock Genomics
Volume 15 - 2024 | https://doi.org/10.3389/fgene.2024.1430291
Background: In Sub-Saharan Africa (SSA), cattle are crucial for socioeconomic stability yet face numerous environmental stressors such as diseases, parasites, and extreme heat within pastoral and agropastoral systems. Despite their significance, gaps remain in understanding how genetic diversity and inbreeding influence traits essential for disease resistance and environmental adaptability. This study examines the genomic adaptations that enable SSA cattle to thrive under these conditions and assesses the impact of inbreeding on such adaptive traits.
Methods: We analyzed genomic data from 113 cattle across four breeds—Kuri, N’dama, Zebu-Fulani, and Zebu-Bororo—employing Runs of Homozygosity (ROH) and Integrated Haplotype Score (iHS) analyses to identify historical and recent genetic selections. Strict quality controls using PLINK software ensured accurate genomic pattern identification related to adaptation and inbreeding.
Results: ROH analysis revealed islands with genes such as RSAD2, CMPK2, and NOTCH1, which are involved in immune response and cellular stress management, highlighting regions of historical selection that have likely provided adaptive advantages in overcoming environmental and pathogenic stresses. In contrast, iHS analysis identified genes under recent selection like HIPK1, involved in stress response regulation, and EPHA5, which plays a crucial role in neural development and synaptic functions, potentially equipping these breeds with novel adaptations to ongoing and emergent environmental challenges.
Conclusion: This research confirms that selective pressures inherent in pastoral and agropastoral systems profoundly influence the genetic structure of SSA cattle. By delineating the genetic bases of key adaptive traits, our study offers crucial insights for targeted breeding programs to enhance cattle resilience and productivity. These findings provide a valuable framework for future genetic improvements and conservation strategies, crucial for sustainable livestock management and economic stability in SSA.
Sub-Saharan Africa (SSA) is home to diverse cattle production systems that are foundational to the livelihoods, culture, and food security of millions. Among these systems, pastoral and agropastoral systems are predominant and adaptive livestock management practices that have profoundly influenced the genetic architecture of indigenous cattle breeds over centuries through environmental and human-mediated selection pressures (Kim et al., 2020; Xia et al., 2023). These systems are characterized by seasonal and transhumant movements of livestock, responding to climatic variability and pasture availability while also significantly shaping the socioeconomic and cultural fabric of the region (Behnke et al., 2011).
The genetic diversity in SSA cattle, as represented by breeds such as the Kuri, N’dama, Zebu-Bororo, and Zebu-Fulani, exhibits a complex mosaic of traits evolved under a broad spectrum of environmental pressures. These breeds, shaped by both natural and anthropogenic selection pressures, demonstrate remarkable resilience to local stressors like diseases, parasites, and heat stress—traits that are less commonly observed in temperate and commercially improved breeds, which are often selected primarily for high productivity traits such as milk yield and growth rates (Orenge et al., 2012; Taye et al., 2017).
A central aspect of understanding the resilience and adaptability of these cattle breeds is the analysis of genetic phenomena such as runs of homozygosity (ROH) and selective sweeps. ROH, indicative of historical inbreeding and genetic drift, helps reveal genetic diversity patterns crucial for understanding the adaptive potential of cattle populations. Extensive studies in temperate and improved breeds have analyzed ROH, linking them to beneficial and deleterious traits. For example, a previous study investigated ROH patterns across cattle types and climatic zones, identifying genes associated with environmental adaptation, disease resistance, coat color, and production traits (Falchi et al., 2023). Similarly, another study identified temperament and body size genes crucial for stress responsiveness and climate tolerance in Brazilian cattle breeds (Peripolli et al., 2020).
Recent research has utilized various statistical methods and advanced genomic tools such as the integrated haplotype score (iHS) to identify candidate genes associated with traits like trypanotolerance in the Sheko breed, revealing genes such as MIGA1, SPAG11B, ERN1, and CAPG linked to anemia, immune response, and neurological functions (Mekonnen et al., 2019). Another study also highlighted the need for further functional investigations after identifying a prominent selection signature on BTA23 in the Muturu breed (Tijjani et al., 2019).
Despite the vital role of SSA cattle in their ecosystems and economies, there remains a notable deficiency in comprehensive genomic studies focused on these breeds. Research on genome-wide ROH and the analysis of selective sweeps is limited, and comparative studies on different genomic measures of inbreeding across various indigenous breeds within the region are lacking. This gap in research hinders a full understanding of how breeding and selection pressures have shaped these cattle’s genetic diversity and adaptive capacities, which are crucial for developing effective breeding strategies and conservation efforts.
This study aims to bridge these gaps by (i) characterizing the genomic diversity of SSA cattle through detailed analysis of ROH and assessing the impact of various genomic measures of inbreeding across different breeds and (ii) analyzing selective sweeps using ROH islands and iHS to identify genomic regions under selection that contribute to the adaptive traits of SSA cattle, thereby enhancing our understanding of their genetic resilience and adaptability.
The genetic data for this study was sourced from the Web-Interfaced Next-generation Database dedicated to Genetic Diversity Exploration (WIDDE), accessible at http://widde.toulouse.inra.fr/widde (Sempéré et al., 2015). Since the data were previously collected and publicly available, no specific ethics approval was required for this analysis. The dataset includes genetic information from 113 individuals of SSA cattle breeds, previously studied by Gautier et al. (2009), and was genotyped using the Illumina 50K SNP chip (Illumina Inc., San Diego, CA, United States). The chromosomal locations are based on the UMD 3.1 assembly of the bovine genome.
Details of the breeds studied are as follows:
• Kuri (KUR): 30 samples were collected from the Lake Chad islands. These cattle are traditionally managed under pastoral systems that capitalize on the extensive grazing available around Lake Chad (Moazami Goudarzi et al., 2001).
• N’dama (NDA): 17 samples were collected from the Samandeni Ranch in Burkina Faso. The NDA are known for their adaptability to agropastoral systems, which combine crop cultivation with cattle rearing. This breed is typically praised for its resilience to local diseases and environmental stressors. Extensive ancestral details are available in Souvenir Zafindrajaona et al. (1999).
• Zebu-Bororo (ZBO) and Zebu-Fulani (ZFU): 23 and 43 samples, respectively, sourced from Malanville in Benin. These breeds are extensively used for crossbreeding and adapted for draught, milk, and meat production. They are noted for their disease resistance, heat tolerance, and efficient resource utilization. Both breeds are managed under traditional pastoral systems, which involve extensive grazing and movement across different grazing areas, with production management practices described (Moazami Goudarzi et al., 2001) and further details about the breeds in other studies (Vanvanhossou et al., 2021a; Ouédraogo et al., 2021).
To provide a comparative perspective on the genetic diversity of SSA cattle, we also included individuals from subtropical and temperate breeds. The subtropical breeds studied were Gir (GIR), Sahiwal (SAH), and Tharparkar (THA), with 17, 24, and 12 samples, respectively. The temperate breeds included were Holstein (HOL) and Jersey (JER), with 64 and 28 samples, respectively. The genetic data for these breeds were also sourced from WIDDE and previous research (Matukumalli et al., 2009; Decker et al., 2014).
Initially, pedigree (.ped) and map (.map) files were converted into binary format files (.bim, .bed, and.fam) using the --make-bed command in PLINK v1.9 software (Purcell et al., 2007). To ensure stringent quality control, we modified an R script written by Gorssen et al. (2021), which automated the application of specific quality control measures. Following their methodology, we retained only autosomal single nucleotide polymorphisms (SNPs) and set thresholds for individual call rates at a minimum of 90% (--mind 0.10) and SNP call rates at a minimum of 95% (--geno 0.05). We did not perform minor allele frequency pruning, Hardy-Weinberg equilibrium testing, or linkage disequilibrium pruning to maintain a comprehensive dataset conducive to ROH analysis (Meyermans et al., 2020). Before quality control, a common set of 51,998 SNPs covering the autosomes was extracted for each SSA cattle breed. These were then merged for cross-population analyses. After the application of quality control measures, the number of usable SNPs was reduced to 48,835. All individuals met the established quality criteria, resulting in no exclusions from the study.
After implementing initial quality control protocols, we conducted our ROH analysis using PLINK, guided by the methodologies suggested in Meyermans et al. (2020). To accurately identify ROH segments, we enforced strict criteria: no heterozygous SNPs were permitted within any ROH segment (--homozyg-window-het and--homozyg-het), and the allowance for a single missing SNP per window (--homozyg-window-missing) was made. The specific number of SNPs required per window (--homozyg-window-snp) and within each ROH segment (--homozyg-snp) was set based on the unique genetic traits of each breed, utilizing the L-parameter for guidance (Meyermans et al., 2020), with the settings adjusted to 61 for KUR, 66 for NDA, 65 for ZBO, and for 68 ZFU. Each window for ROH analysis was set at a minimum of 1,000 kb (--homozyg-kb), with a density requirement of one SNP every 150 kb (--homozyg-density). The maximum allowed gap between consecutive SNPs within an ROH was capped at 1,000 kb (--homozyg-gap), and window threshold of two outer SNPs ensuring continuity and integrity of the homozygous segments. Additionally, the proportion of each chromosome covered by ROH was calculated by dividing the mean ROH length per chromosome by its total length in Mb, providing normalized measurements of ROH coverage across the genome. Average SNP density was at least one SNP per 51 kb for all populations. This parameterization ensured that over 97% of the autosomal genome was covered, facilitating detection of ROH across the studied breeds. ROHs were categorized into four length classes to elucidate their potential genetic impacts: 1–4 Mb (short segments), 4–6 Mb (moderate segments), 6–8 Mb (long segments), and >8 Mb (very long segments).
We employed PLINK to calculate various genomic inbreeding coefficients to provide a multifaceted view of inbreeding. The genomic inbreeding coefficient (
To evaluate differences in ROH, genomic inbreeding metrics, and genome coverage among cattle breeds, we conducted analysis of variance (ANOVA) followed by a Tukey-Kramer post-hoc analysis using the aov function for ANOVA and HSD.test functions from the R package agricolae v1.3-7 (Felipe, 2023). This approach allowed us to identify significant disparities at
Detection of ROH islands was performed using PLINK to quantify ROH incidence, defined as the percentage of animals within a population having a SNP within an ROH segment. The visualization of these incidences was done using Manhattan plots via the R package qqman v0.1.9 (Turner, 2018). ROH islands, indicative of positive selection, were defined based on SNPs exceeding a population-specific threshold. This threshold, derived from standard normal z-scores of ROH incidences, set a cutoff where the top 0.1% of SNPs with a
To detect evidence of recent positive selection within SSA cattle populations, we employed the iHS using the R package rehh v3.2.2 (Gautier and Vitalis, 2012; Gautier et al., 2017). This statistical tool identifies alleles that have undergone selective sweeps by examining the extended haplotype homozygosity (EHH) around a core allele compared to its ancestral state. The iHS is particularly effective for pinpointing long haplotypes that appear more frequently than expected, suggesting recent positive selection.
We phased the genotype data for each chromosome of the Bos taurus populations using the SHAPEIT software (Delaneau et al., 2013), a crucial step for the subsequent iHS analysis (Voight et al., 2006). Following phasing, we determined the EHH for both the ancestral (
Following the methodology outlined by Voight et al. (2006), we standardized the
Here,
We associated
where
We then analyzed these iHS scores across the genome using sliding windows of 1 Mb, each overlapping the subsequent one by 100 kb. Each window required at least two extremal markers with scores exceeding a threshold. A threshold of 4, corresponding to a p-value of less than 0.0001, was set to identify significant selection signals, highlighting regions with significant genetic differentiation indicative of recent positive selection. Significant iHS scores are visualized using Manhattan plots generated by the same rehh package.
Following the detection of ROH island regions and other genomic areas under positive selection identified through iHS analysis, we used the Ensembl Genes 112 database specific to Bos taurus. We input the genomic coordinates of these regions into the Ensembl genome browser (https://www.ensembl.org) to facilitate the extraction of detailed gene annotations. To automate the retrieval of gene information from Ensembl, we employed the R package biomaRt v2.60.0 (Durinck S et al., 2009). This enabled us to effectively query the Ensembl REST API, obtaining comprehensive gene annotations including gene symbols and descriptions of their products. This annotation process allows the identification of known and potentially novel genes that may contribute to adaptive traits in SSA cattle.
We performed PCA to compare the genetic diversity of SSA cattle with subtropical and temperate breeds, facilitating a comprehensive evaluation of genetic adaptation across varied environmental conditions. PCA was conducted using PLINK software, employing a pruned dataset of SNPs to minimize the impact of linkage disequilibrium (Purcell et al., 2007). SNP pruning was performed with specific parameters: a window size of 50 SNPs, a step size of 10 SNPs per shift, and an
The output from PCA was visualized using the R package ggplot2 v3.5.0 (Wickham, 2016). This visualization process highlights the clustering and dispersion of genetic variability across the breeds studied, reflecting their evolutionary and geographical backgrounds. The generated plots facilitate the observation of distinct or overlapping genetic clusters among SSA, subtropical, and temperate cattle breeds.
Table 1 summarizes the distribution of ROH and inbreeding coefficients across four SSA cattle breeds: KUR, NDA, ZBO, and ZFU. In total, 720 ROH segments were detected: 150 in KUR, 128 in NDA, 146 in ZBO, and 296 in ZFU (results not shown). The analysis revealed notable differences in the extent and distribution of ROH among the breeds. The mean number of ROH segments did not significantly vary across the breeds, with values ranging from 5.0 ± 0.71 in KUR to 7.53 ± 2.52 in NDA (
Table 1. Distribution of runs of homozygosity (ROH) and inbreeding coefficients among Sub-Saharan African cattle breeds.
ROH segments between 4–6 Mb demonstrated significant differences across breeds, with a range of 1.24 ± 0.46 in NDA and 2.44 ± 0.26 in ZFU (
In terms of inbreeding coefficients calculated from ROH (
Additional inbreeding metrics such as the
Figure 1 illustrates the individual distribution of ROH in relation to the genomic length covered by these segments for four cattle breeds. For the KUR breed (panel A), a dense clustering of points within a narrow genomic length suggests a uniform extent of ROH across individuals. The NDA breed (panel B) shows a widespread coverage of ROH, indicating a heterogeneous pattern of genomic regions affected by inbreeding. The ZBO (panel C) and ZFU (panel D) breeds demonstrate a more dispersed set of points, indicating a broader range of inbreeding influences, with the ZFU exhibiting a larger number of ROH segments extending over greater genomic lengths.
Figure 1. Scatter plots depicting the relationship between the number of runs of homozygosity (ROH) per individual and the cumulative genomic length covered by ROH across four Sub-Saharan African cattle breeds: Kuri (A), N’dama (B), Zebu-Bororo (C), and Zebu-Fulani (D).
Figure 2 presents the correlation matrices that detail the relationships between various inbreeding metrics across four SSA cattle breeds. In the KUR breed (A),
Figure 2. Pearson correlation matrices for inbreeding metrics and ROH categories across four Sub-Saharan African cattle breeds: Kuri (A), N’dama (B), Zebu-Bororo (C), and Zebu-Fulani (D). The matrices display correlations between different inbreeding metrics, including ROH-based inbreeding coefficients (FROH) for segment lengths (all, 1–4 Mb, 4–6 Mb, 6–8 Mb, >8 Mb), genetic relationship matrix-based inbreeding coefficient (FGRM), inbreeding coefficient based on excess homozygosity (FHOM), and inbreeding coefficient from correlation between uniting gametes (FUNI). Positive correlations are shown in blue and negative in red, with statistical significance as
In the ZBO breed (C), there were substantial correlations between
Overall,
Figure 3 depicts the chromosomal distribution and coverage of ROH for four SSA cattle breeds, indicated by bar graphs and overlaid line graphs, respectively. For the KUR breed (Panel A), ROHs were most concentrated on chromosomes 7, 5, and 3, with counts of 12, 11, and 10, respectively. Chromosome 15 showed the highest genomic coverage at approximately 10.52%, whereas the lowest coverage was observed on chromosome 23 at about 1.14%. In the NDA breed (Panel B), chromosome 10 displayed the highest ROH count with 10 segments, closely followed by chromosomes 11 and 3, each also registering 8 segments. The greatest coverage was on chromosome 1 at 8.94%, with the smallest coverage on chromosome 14, recording only 0.78%. The ZBO breed (Panel C) exhibited a broad spread of ROHs, notably on chromosomes 5 and 7, with 19 and 13 segments respectively. The highest coverage was on chromosome 5 at approximately 6.99%, and the lowest was on chromosome 27 with 1.23%. For the ZFU breed (Panel D), there was a significant accumulation of ROHs on chromosomes 1, 6, and 8, each showing at least 27 segments. Chromosome 3 had notable coverage at about 6.25%, while chromosome 29 showed the least coverage at roughly 1.57%.
Figure 3. Chromosomal distribution and coverage of runs of homozygosity (ROH) across four Sub-Saharan African cattle breeds: Kuri (A), N’dama (B), Zebu-Bororo (C), and Zebu-Fulani (D).
Figure 4 presents Manhattan plots for SNP incidence within ROH across four SSA cattle breeds. The red horizontal line at the 20% mark on each plot is a threshold for distinguishing significant ROH islands. KUR (Panels A) and ZFU (Panel D) show few incidences below the threshold, indicating a scattered presence of significant ROH islands within these breeds. Conversely, NDA (Panel B) and ZBO (Panel C) display multiple chromosomal locations where the incidence of SNPs surpasses the 20% threshold, which suggests a greater presence of significant ROH islands.
Figure 4. Manhattan plots of SNP incidence within runs of homozygosity (ROH) across four Sub-Saharan African cattle breeds: Kuri (A), N’dama (B), Zebu-Bororo (C), and Zebu-Fulani (D). A red line marks the 20% threshold for identifying significant ROH islands.
Our genomic analysis detected 16 and 5 candidate regions on chromosomes 11 and 28, respectively, in the NDA breed, and 5 regions on chromosome 5 of the ZBO breed. From these regions, a total of 308 genes symbols were identified, as detailed in Supplementary Table S1. Among these, 14 are represented solely by marker names and lack functional annotations. Notable among the functionally annotated genes are RSAD2, CMPK2, SOX11, GGTA1, OR1J1, DENND1A, and NOTCH1 on chromosome 11; and ARHGAP22 and CXCL12 on chromosome 28 for NDA. In the ZBO breed, significant genes on chromosome 5 include SLC25A17, and EFCAB6.
Figure 5 depicts Manhattan plots of iHS for cattle breeds, where each dot represents a SNP. The dashed horizontal lines denote suggestive thresholds for positive selection signals, with data points above these lines indicating regions potentially under selection.
Figure 5. Manhattan plots of Integrated Haplotype Scores (iHS) across autosomal chromosomes for four Sub-Saharan African cattle breeds: Kuri (A), N’dama (B), Zebu-Bororo (C), and Zebu-Fulani (D). The plots illustrate -log10
For the KUR breed (Panel A), a notable peak is present on chromosome 3, where the gene HIPK1 is identified as a candidate for positive selection. The NDA breed (Panel B) exhibits multiple regions with significant iHS signals on several chromosomes, including strong peaks associated with genes like EPHA5, CFAP54, SENP6 and RIMS1. The ZBO breed (Panel C) shows a pronounced selection signal on chromosome 12, with the gene FRY highlighted. The ZFU breed (Panel D) has a distinct peak on chromosome 6, where PPARGC1A is identified among the candidate genes. Supplementary Table S2 complements these iHS findings by providing a detailed summary of the candidate genomic regions, including the bovine chromosome number (BTA), the specific locations of these regions, gene symbols, and products. For instance, it elucidates that the NDA breed has putative sweeps on chromosomes 5, 6, and 9, including ELK3, HTR1B, and MEI4, suggesting these areas have undergone selection sweeps. Similarly, for the ZBO, the table highlights a large genomic region on chromosome 12 with 2939 exons and several candidate genes, such as BRCA2 and ALOX5AP, indicating a substantial genomic section subject to selection.
Figure 6 illustrates the genetic diversity among cattle breeds through PCA. In Figure 6A, focusing on SSA cattle, PC1 and PC2 captured 36.70% and 12.71% of the genetic variance, respectively, as detailed in Supplementary Figure S1. KUR forms a distinct and tight cluster, indicative of high genetic homogeneity. NDA also forms a clear cluster but shows more dispersion than KUR, suggesting higher genetic variability within this breed. Notably, ZBO and ZFU exhibit significant overlap, indicating their close genetic relationship and shared genetic background.
Figure 6. Principal component (PC) analysis (PCA) of genetic diversity in cattle breeds: Sub-Saharan African breeds (A) and breeds from diverse climatic regions (B). KUR: Kuri, NDA: N’dama, ZBO: Zebu-Bororo, ZFU: Zebu-Fulani, GIR: Gir, SAH: Sahiwal, THA: Tharparkar, HOL: Holstein, JER: Jersey.
Contrasting with Figures 6A, B includes breeds from subtropical and temperate regions, showing that PC1 and PC2 explain a larger proportion of the variance, at 48.67% and 15.57%, respectively. Among the subtropical breeds, GIR, SAH, and THA form a distinct cluster with significant overlap, highlighting a close genetic relationship. Meanwhile, the temperate breeds, HOL and JER, are closely positioned yet form separate clusters with no overlap, underscoring their unique genetic characteristics. HOL is particularly noted for its tight clustering, suggesting high genetic homogeneity.
This study used comprehensive genomic tools to explore genetic diversity and adaptive mechanisms among SSA cattle, commonly found within pastoral and agropastoral systems. These environments are integral to the region’s livelihoods and cultural heritage, imposing unique environmental and selective pressures that shape the cattle’s genetic structure. We have revealed the complex interplay between inbreeding effects and selective adaptations through genomic analyses, including ROH and heterozygosity assessments. Our methodology underscores the resilience and adaptability of these indigenous breeds, filling significant knowledge gaps regarding how genetic diversity supports their survival under varied and challenging conditions. The insights gained are pivotal for informing sustainable livestock management and genetic conservation strategies, enhancing SSA food security and economic stability.
Our analysis of ROH and inbreeding coefficients across four SSA cattle breeds offers valuable insights into their genetic structure influenced by both historical and contemporary breeding practices (Table 1; Figure 1). The KUR breed displayed a significant prevalence of shorter ROH segments, generally under 4 Mb, suggesting ancient inbreeding or shared ancestry (Purfield et al., 2012). These genetic signatures indicate a long-standing genetic stability likely fostered by extensive pastoral systems. Such systems have historically promoted genetic diversity through natural migration and minimal human-directed breeding, suggesting a breed well-adapted through natural selection to its environment (Orenge et al., 2012; Taye et al., 2017; Kim et al., 2020; Xia et al., 2023). This adaptation process reflects the breed’s well-suited nature to its environment, aligning with the findings from Ouédraogo et al. (2021), which describe moderate levels of genomic inbreeding within local cattle populations, suggesting a balanced management of inbreeding akin to some well-managed European breeds.
In contrast, the NDA breed shows a higher occurrence of longer ROH segments, particularly those exceeding 8 Mb. These variations in ROH segments indicate recent inbreeding (Purfield et al., 2012; Sumreddee et al., 2021). Despite these indicators of recent inbreeding or intense selection for specific traits, the overall genetic diversity of the breed remains high, as indicated by non-significant overall inbreeding coefficients (
The ZBO and ZFU breeds showcase a mixture of genetic influences, with a prominent distribution of medium-length ROH segments (4–6 Mb). These segments, statistically significant but not distinct enough to separate the breeds post hoc, reflect past inbreeding or shared ancestry. This genetic pattern is likely influenced by traditional pastoral systems where extensive grazing and movement facilitate genetic exchange, supporting the development of traits like drought resistance and enhanced milk production commonly selected in these breeds.
It is imperative to blend traditional knowledge with modern genomic tools to manage genetic diversity effectively, which includes establishing breed registries, employing rotational mating systems, and introducing new genetic material to preserve breed characteristics and enhance diversity (Eusebi et al., 2020; Oldenbroek, 2021). Conservation programs, such as those implemented for Ankole cattle in Uganda, demonstrate the effectiveness of integrating scientific plans with community participation to boost cattle productivity and genetic health (Nabasirye, 2012).
Our analysis across SSA cattle breeds detailed the interactions between various genomic inbreeding metrics, providing essential insights into the genetic structure of these populations (Figure 2). This study highlights the critical role of different inbreeding coefficients in assessing the genetic makeup and historical breeding practices of cattle.
Strong correlations observed between
In contrast, the consistently low correlation between
The negative correlation between
These correlation trends offer valuable insights into how different inbreeding metrics can be interpreted in the context of breed management. They highlight the importance of using a combination of these metrics to gain a comprehensive understanding of both inbreeding levels and the broader genetic health of the breeds, which is crucial for making informed decisions in breeding programs aimed at maintaining genetic diversity and vigor.
The distribution of ROH across SSA cattle breeds underscores historical selection pressures and provides crucial insights into contemporary genetic management and evolutionary strategies shaping these populations. The variance in ROH coverage among breeds such as KUR and NDA highlights regions under intense selection, likely reflecting adaptations to environmental pressures like disease resistance and drought tolerance (Dixit et al., 2020). Conversely, breeds such as ZBO and ZFU, exhibiting extensive ROH across multiple chromosomes, might represent strategies to enhance multi-trait resilience, which is beneficial for breeds in varied agricultural roles (Vanvanhossou et al., 2021b). This widespread selection could have evolved through breeding practices focused on developing robust cattle capable of thriving under diverse environmental conditions.
Areas with lower ROH indicate preserved genetic diversity, essential for a breed’s adaptability to future challenges like climate change or emerging diseases (Zhang et al., 2015; Illa et al., 2024). On the other hand, high-density areas of ROH might signal genetic bottlenecks or extensive inbreeding, potentially predisposing breeds to genetic disorders or limiting their adaptability to new stressors.
The detailed understanding of these chromosomal locations with high ROH can guide genomic selection efforts, allowing breeders to enhance traits linked to these areas while introducing genetic variability to mitigate inbreeding risks (Sonesson et al., 2012; Dixit et al., 2020; Meuwissen et al., 2020; Illa et al., 2024). For example, targeted crossbreeding programs could introduce fresh genetic material into high ROH areas, enhancing genetic health and trait diversity.
The patterns observed in ROH distribution provide a foundation for genetic studies in similar contexts and emphasize the importance of conservation strategies that balance trait enhancement with genetic diversity preservation. Initiatives such as gene banks and other genetic conservation measures are vital for maintaining the genetic heritage of these breeds, ensuring their long-term sustainability (Mapiye et al., 2019; Tenzin et al., 2023).
The integration of ROH and iHS analyses elucidates the complex genetic landscape of SSA cattle, emphasizing the influence of historical and contemporary selection pressures in defining breed-specific traits. This genetic overview, illustrated in Figures 4, 5 and elaborated in Supplementary Tables S1, S2, traces the evolutionary paths shaped by varied environmental and human-related factors.
For the NDA and ZBO breeds, significant ROH islands highlight regions of intense historical selection likely spurred by environmental challenges, as documented by Purfield et al. (2012). In contrast, the KUR and ZFU breeds exhibit fewer ROH islands, suggesting a history of milder selection pressures. This could indicate a broader genetic diversity within these breeds, possibly due to extensive mating pools common in pastoral and agropastoral systems (Moazami Goudarzi et al., 2001; Gautier et al., 2009; Gorssen et al., 2021). Such diversity might provide these breeds with a greater capacity to adapt to fluctuating environmental conditions, essential for managing future climatic changes.
In the NDA breed, genes located within ROH islands like RSAD2 and CMPK2 could indicate a genetic predisposition towards enhanced disease resistance, crucial for survival in areas burdened by endemic diseases. RSAD2 plays a role in antiviral defense and immune responses, especially during pregnancy (Rocha et al., 2023). CMPK2 has been associated with immune function and disease resistance, such as its involvement in bovine digital dermatitis and antiviral responses (Lai et al., 2021; Oelschlaegel et al., 2022). Previous studies in West African cattle have identified adaptive selection footprints linked to immune responses in West African taurine breeds, paralleling our discovery of RSAD2 and CMPK2 genes in NDA cattle, which suggest enhanced disease resistance (Gautier et al., 2009; Tijjani et al., 2019).
The GGTA1 and OR1J1 genes have potential roles in NDA cattle. GGTA1, encoding alpha-1,3-galactosyltransferase, is significant in immune modulation and various biological processes, particularly in xenotransplantation (Day and Rocha, 2008). Its role in NDA cattle may involve enhancing immune responses, though this remains speculative. OR1J1, a key gene in the olfactory system, potentially enhances foraging efficiency. This gene is regulated by the MOR4 motif and shows expression differences among bulls, steers, and heifers (Lee et al., 2013; Kubo et al., 2016; Samuel and Dinka, 2020). It is crucial for feed appetence (Roura et al., 2008), suggesting an adaptive advantage in extensive pastoral landscapes.
Furthermore, the NDA breed shows potential genetic adaptations for physical resilience and metabolic efficiency, vital for breeds used in labor-intensive pastoral tasks. The ARHGAP22 gene plays a crucial role in regulating actin dynamics, affecting synaptic plasticity and cognitive function in mice (Longatti et al., 2021). While its specific role in cattle is not well-documented, it could enhance cellular adaptability to physical stresses in NDA cattle. The inclusion of genes such as NOTCH1, with its role in developmental processes, might suggest its contribution to cellular differentiation and organ development. NOTCH1 regulates cell proliferation and maintains the expression of key genes in early embryonic development (Li et al., 2021). In mice, disruption of NOTCH1 leads to widespread cell death in embryos, indicating its essential role in post implantation development (Swiatek et al., 1994). These findings suggest that NOTCH1 likely plays a similar role in cattle, supporting cellular differentiation and organ development, which are crucial for the breed’s overall health and productivity.
For ZBO cattle, genes like SLC25A17 has been associated with higher milk yields in Chinese Holstein cattle (Lv et al., 2011), suggesting a role in metabolic efficiency. EFCAB6 plays a crucial role in lipid metabolism and adipocyte proliferation (Chen et al., 2017; Junjvlieke et al., 2019). These genes likely contribute to breed’s survival in environments with limited resources.
The Manhattan plots of iHS provide insights into the ongoing selection pressures and identify genes critical for adaptive traits across SSA cattle breeds, enhancing our understanding of their genetic basis for adaptability and resilience.
In KUR cattle, the HIPK1 gene emerges as a key factor in stress response adaptability, potentially aiding in coping with environmental extremes such as drought and high temperatures around Lake Chad. HIPK1 plays a crucial role in cell growth and stress responses, including activating p53 to manage cellular stress (Isono et al., 2006; Rey et al., 2013). While the link between HIPK1 and environmental stressors has not been directly studied, its role in cellular stress responses suggests it may help KUR cattle cope with harsh enviroments, which is crucial for breeds exposed to variable and harsh climatic conditions.
For NDA cattle, adapted to the mixed agropastoral systems of Burkina Faso, genes like EPHA5 and RIMS1, linked to neural development and synaptic functions. EPHA5 is involved in neural connectivity and immune response modulation (Fu et al., 2010; Staquicini et al., 2015; Huang et al., 2021). RIMS1, is crucial for neurotransmitter release and synaptic plasticity, impacting cognitive abilities (Sisodiya et al., 2007; Kaeser et al., 2008). Given their roles in neural and synaptic functions, it is hypothesized that EPHA5 and RIMS1 could influence complex behaviors in cattle, such as navigational abilities and social hierarchy management. These behaviors require complex neural processing, which these genes are known to support. Additionally, genes such as SENP6 and MYO6, associated with apoptosis and cellular transport, may contribute to the breed’s resilience against diseases like trypanosomiasis, common in their geographic area. MYO6 plays a crucial role in muscle development in other species (Tan et al., 2024), suggesting a similar function in cattle, potentially impacting overall health and disease resilience. SENP6 is involved in genome stability, inflammation regulation, and apoptosis, essential for managing cellular stress during infections (Wagner et al., 2019; Mao et al., 2022). These roles in cellular processes suggest that SENP6 and MYO6 might enhance the breed’s resilience to diseases.
For ZBO cattle, genes like FRY and ZAR1L, identified through iHS analysis, may support traits linked to reproductive efficiency and cellular organization, essential under the extensive pastoral systems prevalent in their native Benin. FRY plays a crucial role in kidney development and function in mice (Byun et al., 2018), with potential involvement in fertility and milk production traits in cattle (Cai et al., 2019). ZAR1L, a maternal factor, is expressed during various stages of embryonic development, indicating its role in these processes (Brevini et al., 2004). The related ZAR1-like gene supports its role in oocyte development (Sangiorgio et al., 2008). These genes likely contribute to reproductive efficiency and cellular organization in ZBO cattle.
For ZFU cattle, the PPARGC1A gene is highlighted for its association with milk fat synthesis and overall milk composition traits, including fat and protein yield, critical for breeds extensively used for dairy production under challenging environmental conditions (Weikard et al., 2005; Pasandideh et al., 2015).
The PCA analysis conducted in this study provides a comprehensive overview of the genetic diversity and structural relationships among various cattle breeds, with a focus on SSA breeds alongside subtropical and temperate counterparts, as depicted in Figure 6. This analytical approach reveals distinct genetic clusters, effectively illustrating the variations across different geographical and breeding contexts.
In the SSA group, the KUR breed displays a high degree of genetic homogeneity, which can be attributed to the pastoral systems prevalent around Lake Chad. These systems support natural migration and exert minimal selective pressures on breeding, resulting in a tightly clustered genetic profile. Such uniformity could confer adaptive advantages, allowing for stable existence in relatively undisturbed natural environments (Vanvanhossou et al., 2021b). On the other hand, the NDA breed demonstrates a more dispersed genetic pattern on the PCA, suggesting greater genetic variability. This is likely due to the breed’s integration into agropastoral systems that promote genetic diversity through random mating, thereby bolstering resilience against environmental and climatic challenges.
The genetic overlays of the ZBO and ZFU breeds indicate a closely knit genetic relationship, likely stemming from historical interbreeding and similar pastoral management practices. Adapted to various agricultural roles, these breeds exhibit traits that are advantageous in diverse and demanding environments, indicative of a strategic breeding approach to sustain a resilient genetic foundation (Vanvanhossou et al., 2021b).
Contrastingly, subtropical breeds such as the GIR, SAH, and THA exhibit tight clustering, reflecting their shared adaptations for heat tolerance and disease resistance. These traits are essential for thriving in subtropical climates and are a result of selective breeding processes focused on enhancing survival in hot environments (Dixit et al., 2020; Strucken et al., 2021). In a stark difference, temperate breeds like HOL and JER are distinctly separated on the PCA, showcasing the influence of intensive selective breeding aimed at optimizing traits like milk production. These breeds, developed under controlled breeding programs, highlight the divergent genetic paths taken to maximize agricultural productivity in temperate zones (Dixit et al., 2020; Xia et al., 2023).
The genomic analysis delineated in this study highlight the crucial role of genetic diversity and inbreeding in shaping the adaptability and resilience of SSA cattle. We acknowledge certain methodological limitations, including reliance on SNP chips, which might not capture the entire spectrum of genetic diversity, particularly rare variants crucial for adaptive traits. The primary data source, sourced from public databases (Sempéré et al., 2015), might not comprehensively represent the genetic variability within each breed, potentially influencing perceived genetic structures and levels of inbreeding. To address these limitations, future studies should incorporate whole-genome sequencing to provide a more detailed genetic landscape and expand sampling to enhance the robustness of findings. Comparative analysis with cattle breeds under similar pressures in different geographical contexts will further elucidate how selective and environmental pressures shape genetic diversity globally (Freitas et al., 2021).
Our findings underscore the necessity for detailed breeding strategies incorporating genomic tools to enhance traits such as disease resistance and environmental adaptability (Kim et al., 2017b). Linking genetic traits with economic and cultural practices in SSA highlights the importance of preserving genetic diversity for conservation purposes and sustaining local communities’ livelihoods (Vanvanhossou et al., 2021b). This approach supports rural SSA’s cultural heritage and economic stability (Vanvanhossou et al., 2021b).
There is substantial potential for interdisciplinary collaborations among geneticists, breeders, and socio-economists to address the holistic challenges SSA cattle breeds face. Future research could apply genome-wide association studies to pinpoint specific genes linked to desirable characteristics in SSA cattle (Taye et al., 2017). Longitudinal studies tracking genetic changes in these populations will assess the impact of different breeding strategies on genetic diversity and health outcomes.
This study has yielded pivotal genetic insights into SSA cattle breeds like KUR, NDA, ZBO, and ZFU, which are integral to pastoral and agropastoral systems in the region. Our analysis revealed substantial ROH across these breeds, signaling diverse inbreeding patterns. The NDA breed, in particular, demonstrated the highest inbreeding coefficients, reflecting its history of intense selective breeding. Our findings identified critical ROH islands containing genes such as RSAD2, CMPK2, and NOTCH1, which are linked to immune response and cellular stress mechanisms. These genes likely represent regions of historical selection, conferring adaptive advantages essential for overcoming environmental stresses and diseases prevalent in harsh African environments. Conversely, iHS analysis has revealed recent selective sweeps involving genes like HIPK1 in KUR cattle, which is known to regulate stress responses and may potentially enhance heat stress resilience. Similarly, SENP6 and RIMS1 in NDA cattle are implicated in immune responses and are suggested to play roles in disease resistance. These findings differentiate the roles of genetic markers detected through ROH and iHS, underscoring the breeds’ innate capacities for environmental adaptability and disease resistance. This genetic blueprint provides crucial insights for targeted breeding programs aimed at amplifying these valuable traits. Our work lays the groundwork for informed strategies in livestock management and conservation, ensuring the sustainable development of cattle breeds that are vital to the socioeconomic stability of Sub-Saharan Africa.
Publicly available datasets were analyzed in this study. This data can be found here: http://widde.toulouse.inra.fr/widde/ under Cattle.
Ethical approval was not required for the studies involving animals in accordance with the local legislation and institutional requirements because no experimental study was carried out. Written informed consent was not obtained from the owners for the participation of their animals in this study because datasets are publicly available.
OA: Conceptualization, Data curation, Formal Analysis, Funding acquisition, Investigation, Software, Validation, Visualization, Writing–original draft, Writing–review and editing. AbM: Data curation, Formal Analysis, Software, Visualization, Writing–original draft, Writing–review and editing. Lalmuansangi: Formal Analysis, Software, Writing–review and editing. SS: Writing–review and editing. SM: Writing–review and editing. AnM: Resources, Supervision, Writing–review and editing.
The author(s) declare that financial support was received for the research, authorship, and/or publication of this article. The publication of this article was funded by the Open Access Fund of the FBN.
We thank the Director of ICAR-National Dairy Research Institute, Karnal, and the CV Raman Fellowship for their essential support and assistance throughout this study. We also thank Henry Reyer from the Research Institute for Farm animal Biology (FBN), Dummerstorf, for his constructive comments, which significantly enhanced the quality of this work.
The authors declare that the research was conducted in the absence of any commercial or financial relationships that could be construed as a potential conflict of interest.
All claims expressed in this article are solely those of the authors and do not necessarily represent those of their affiliated organizations, or those of the publisher, the editors and the reviewers. Any product that may be evaluated in this article, or claim that may be made by its manufacturer, is not guaranteed or endorsed by the publisher.
The Supplementary Material for this article can be found online at: https://www.frontiersin.org/articles/10.3389/fgene.2024.1430291/full#supplementary-material
Behnke, R. H., Fernandez-Gimenez, M. E., Turner, M. D., and Stammler, F. (2011). “Pastoral migration: mobile systems of livestock husbandry,” in Animal migration: a synthesis. Editors E. J. Milner-Gulland, J. M. Fryxell, and A. R. E. Sinclair (Oxford University Press). doi:10.1093/acprof:oso/9780199568994.003.0010
Brevini, T. A. L., Cillo, F., Colleoni, S., Lazzari, G., Galli, C., and Gandolfi, F. (2004). Expression pattern of the maternal factor zygote arrest 1 (Zar1) in bovine tissues, oocytes, and embryos. Mol. Reprod. Dev. 69, 375–380. doi:10.1002/mrd.20140
Byun, Y. S., Kim, E. K., Araki, K., Yamamura, K. I., Lee, K., Yoon, W. K., et al. (2018). Fryl deficiency is associated with defective kidney development and function in mice. Exp. Biol. Med. 243, 408–417. doi:10.1177/1535370218758249
Cai, Z., Guldbrandtsen, B., Lund, M. S., and Sahana, G. (2019). Prioritizing candidate genes for fertility in dairy cows using gene-based analysis, functional annotation and differential gene expression. BMC Genomics 20, 255. doi:10.1186/s12864-019-5638-9
Chen, S., He, H., and Liu, X. (2017). Tissue expression profiles and transcriptional regulation of elongase of very long chain fatty acid 6 in bovine mammary epithelial cells. PLoS One 12, e0175777. doi:10.1371/journal.pone.0175777
Day, A., and Rocha, D. (2008). Allelic variation of the porcine alpha-1,3-galactosyltransferase 1 (GGTA1) gene. J. Appl. Genet. 49, 75–79. doi:10.1007/BF03195251
Decker, J. E., McKay, S. D., Rolf, M. M., Kim, J. W., Molina Alcalá, A., Sonstegard, T. S., et al. (2014). Worldwide patterns of ancestry, divergence, and admixture in domesticated cattle. PLoS Genet. 10, e1004254. doi:10.1371/journal.pgen.1004254
Delaneau, O., Howie, B., Cox, A. J., Zagury, J. F., and Marchini, J. (2013). Haplotype estimation using sequencing reads. Am. J. Hum. Genet. 93, 687–696. doi:10.1016/j.ajhg.2013.09.002
Dixit, S. P., Singh, S., Ganguly, I., Bhatia, A. K., Sharma, A., Kumar, N. A., et al. (2020). Genome-wide runs of homozygosity revealed selection signatures in Bos indicus. Front. Genet. 11, 92. doi:10.3389/fgene.2020.00092
Durinck, S., Spellman, P., Birney, E., and Huber, W. (2009). Mapping identifiers for the integration of genomic datasets with the R/Bioconductor package biomaRt. Nat. Protoc. 4, 1184–1191. doi:10.1038/nprot.2009.97
Eusebi, P. G., Martinez, A., and Cortes, O. (2020). Genomic tools for effective conservation of livestock breed diversity. Diversity 12, 8. doi:10.3390/d12010008
Falchi, L., Cesarani, A., Mastrangelo, S., Senczuk, G., Portolano, B., Pilla, F., et al. (2023). Analysis of runs of homozygosity of cattle living in different climate zones. J. Anim. Sci. 101, skad061. doi:10.1093/jas/skad061
Felipe, M. (2023). Agricolae: statistical procedures for agricultural research. R packag version 1.3-7. Available at: https://cran.r-project.org/package=agricolae.
Freitas, P. H. F., Wang, Y., Yan, P., Oliveira, H. R., Schenkel, F. S., Zhang, Y., et al. (2021). Genetic diversity and signatures of selection for thermal stress in cattle and other two Bos species adapted to divergent climatic conditions. Front. Genet. 12, 604823. doi:10.3389/fgene.2021.604823
Fu, D. Y., Wang, Z. M., Wang, B. L., Chen, L., Yang, W. T., Shen, Z. Z., et al. (2010). Frequent epigenetic inactivation of the receptor tyrosine kinase EphA5 by promoter methylation in human breast cancer. Hum. Pathol. 41, 48–58. doi:10.1016/j.humpath.2009.06.007
Gautier, M., Flori, L., Riebler, A., Jaffrézic, F., Laloé, D., Gut, I., et al. (2009). A whole genome Bayesian scan for adaptive genetic divergence in West African cattle. BMC Genomics 10, 550. doi:10.1186/1471-2164-10-550
Gautier, M., Klassmann, A., and Vitalis, R. (2017). Rehh 2.0: a reimplementation of the R package rehh to detect positive selection from haplotype structure. Mol. Ecol. Resour. 17, 78–90. doi:10.1111/1755-0998.12634
Gautier, M., and Vitalis, R. (2012). Rehh an R package to detect footprints of selection in genome-wide SNP data from haplotype structure. Bioinformatics 28, 1176–1177. doi:10.1093/bioinformatics/bts115
Gorssen, W., Meyermans, R., Janssens, S., and Buys, N. (2021). A publicly available repository of ROH islands reveals signatures of selection in different livestock and pet species. Genet. Sel. Evol. 53, 2. doi:10.1186/s12711-020-00599-7
Huang, W., Lin, A., Luo, P., Liu, Y., Xu, W., Zhu, W., et al. (2021). EPHA5 mutation predicts the durable clinical benefit of immune checkpoint inhibitors in patients with lung adenocarcinoma. Cancer Gene Ther. 28, 864–874. doi:10.1038/s41417-020-0207-6
Illa, S. K., Mumtaz, S., Nath, S., Mukherjee, S., and Mukherjee, A. (2024). Characterization of runs of Homozygosity revealed genomic inbreeding and patterns of selection in indigenous sahiwal cattle. J. Appl. Genet. 65, 167–180. doi:10.1007/s13353-023-00816-1
Isono, K., Nemoto, K., Li, Y., Takada, Y., Suzuki, R., Katsuki, M., et al. (2006). Overlapping roles for homeodomain-interacting protein kinases hipk1 and hipk2 in the mediation of cell growth in response to morphogenetic and genotoxic signals. Mol. Cell. Biol. 26, 2758–2771. doi:10.1128/MCB.26.7.2758-2771.2006
Junjvlieke, Z., Mei, C. G., Khan, R., Zhang, W. Z., Hong, J. Y., Wang, L., et al. (2019). Transcriptional regulation of bovine elongation of very long chain fatty acids protein 6 in lipid metabolism and adipocyte proliferation. J. Cell. Biochem. 120, 13932–13943. doi:10.1002/jcb.28667
Kaeser, P. S., Kwon, H. B., Chiu, C. Q., Deng, L., Castillo, P. E., and Südhof, T. C. (2008). RIM1alpha and RIM1beta are synthesized from distinct promoters of the RIM1 gene to mediate differential but overlapping synaptic functions. J. Neurosci. 28, 13435–13447. doi:10.1523/JNEUROSCI.3235-08.2008
Kim, J., Hanotte, O., Mwai, O. A., Dessie, T., Salim, B., Diallo, B., et al. (2017a). The genome landscape of indigenous African cattle. Genome Biol. 18, 34. doi:10.1186/s13059-017-1153-y
Kim, K., Kwon, T., Dessie, T., Yoo, D. A., Mwai, O. A., Jang, J., et al. (2020). The mosaic genome of indigenous African cattle as a unique genetic resource for African pastoralism. Nat. Genet. 52, 1099–1110. doi:10.1038/s41588-020-0694-2
Kim, S. J., Ka, S., Ha, J. W., Kim, J., Yoo, D. A., Kim, K., et al. (2017b). Cattle genome-wide analysis reveals genetic signatures in trypanotolerant N’Dama. BMC Genomics 18, 371. doi:10.1186/s12864-017-3742-2
Kubo, H., Otsuka, M., and Kadokawa, H. (2016). Sexual polymorphisms of vomeronasal 1 receptor family gene expression in bulls, steers, and estrous and early luteal-phase heifers. J. Vet. Med. Sci. 78, 271–279. doi:10.1292/jvms.15-0300
Lai, J. H., Wu, D. W., Wu, C. H., Hung, L. F., Huang, C. Y., Ka, S. M., et al. (2021). Mitochondrial CMPK2 mediates immunomodulatory and antiviral activities through IFN-dependent and IFN-independent pathways. iScience 24, 102498. doi:10.1016/j.isci.2021.102498
Lee, K., Nguyen, D. T., Choi, M., Cha, S. Y., Kim, J. H., Dadi, H., et al. (2013). Analysis of cattle olfactory subgenome: the first detail study on the characteristics of the complete olfactory receptor repertoire of a ruminant. BMC Genomics 14, 596. doi:10.1186/1471-2164-14-596
Li, S., Shi, Y., Dang, Y., Luo, L., Hu, B., Wang, S., et al. (2021). NOTCH signaling pathway is required for bovine early embryonic development. Biol. Reprod. 105, 332–344. doi:10.1093/biolre/ioab056
Longatti, A., Ponzoni, L., Moretto, E., Giansante, G., Lattuada, N., Colombo, M. N., et al. (2021). Arhgap22 disruption leads to RAC1 hyperactivity affecting hippocampal glutamatergic synapses and cognition in mice. Mol. Neurobiol. 58, 6092–6110. doi:10.1007/s12035-021-02502-x
Lv, Y., Wei, C., Zhang, L., Lu, G., Liu, K., and Du, L. (2011). Association between polymorphisms in the SLC27A1 gene and milk production traits in Chinese holstein cattle. Anim. Biotechnol. 22, 1–6. doi:10.1080/10495398.2011.527567
Mao, M., Xia, Q., Zhan, G. F., Chu, Q. J., Li, X., and Lian, H. K. (2022). SENP6 induces microglial polarization and neuroinflammation through de-SUMOylation of Annexin-A1 after cerebral ischaemia–reperfusion injury. Cell Biosci. 12, 113. doi:10.1186/s13578-022-00850-2
Mapiye, C., Chikwanha, O. C., Chimonyo, M., and Dzama, K. (2019). Strategies for sustainable use of indigenous cattle genetic resources in Southern Africa. Diversity 11, 214. doi:10.3390/d11110214
Matukumalli, L. K., Lawley, C. T., Schnabel, R. D., Taylor, J. F., Allan, M. F., Heaton, M. P., et al. (2009). Development and characterization of a high density SNP genotyping assay for cattle. PLoS One 4, e5350. doi:10.1371/journal.pone.0005350
McQuillan, R., Leutenegger, A.-L., Abdel-Rahman, R., Franklin, C. S., Pericic, M., Barac-Lauc, L., et al. (2008). Runs of homozygosity in European populations. Am. J. Hum. Genet. 83, 359–372. doi:10.1016/j.ajhg.2008.08.007
Mekonnen, Y. A., Gültas, M., Effa, K., Hanotte, O., and Schmitt, A. O. (2019). Identification of candidate signature genes and key regulators associated with trypanotolerance in the Sheko breed. Front. Genet. 10, 1095. doi:10.3389/fgene.2019.01095
Meuwissen, T. H. E., Sonesson, A. K., Gebregiwergis, G., and Woolliams, J. A. (2020). Management of genetic diversity in the era of genomics. Front. Genet. 11, 880. doi:10.3389/fgene.2020.00880
Meyermans, R., Gorssen, W., Buys, N., and Janssens, S. (2020). How to study runs of homozygosity using plink? a guide for analyzing medium density snp data in livestock and pet species. BMC Genomics 21, 94. doi:10.1186/s12864-020-6463-x
Moazami Goudarzi, K., Belemsaga, D. M. A., Ceriotti, G., Laloë, D., Fagbohoum, F., Kouagou, N. T., et al. (2001). Caractérisation de la race bovine Somba à l’aide de marqueurs moléculaires. Rev. d’élevage médecine vétérinaire Des. pays Trop. 54, 129. doi:10.19182/remvt.9791
Nabasirye, M. (2012). Population structure and dynamics of Ankole cattle in Uganda: implications for sustainable utilisation of the breed. Afr. J. Agric. Reseearch 7. doi:10.5897/ajar12.583
Oelschlaegel, D., Wensch-Dorendorf, M., Kopke, G., Jungnickel, R., Waurich, B., Rosner, F., et al. (2022). Functional variants associated with CMPK2 and in ASB16 influence bovine digital dermatitis. Front. Genet. 13, 859595. doi:10.3389/fgene.2022.859595
Oldenbroek, K. J. (2021). The use of genomic information for the conservation of animal genetic diversity. Animals 11, 3208. doi:10.3390/ani11113208
Orenge, C. O., Munga, L., Kimwele, C. N., Kemp, S., Korol, A., Gibson, J. P., et al. (2012). Trypanotolerance in N’Dama x Boran crosses under natural trypanosome challenge: effect of test-year environment, gender, and breed composition. BMC Genet. 13, 87. doi:10.1186/1471-2156-13-87
Ouédraogo, D., Soudré, A., Yougbaré, B., Ouédraogo-Koné, S., Zoma-Traoré, B., Khayatzadeh, N., et al. (2021). Genetic improvement of local cattle breeds in West Africa: a review of breeding programs. Sustain 13, 2125. doi:10.3390/su13042125
Pasandideh, M., Mohammadabadi, M. R., Esmailizadeh, A. K., and Tarang, A. (2015). Association of bovine PPARGC1A and OPN genes with milk production and composition in Holstein cattle. Czech J. Anim. Sci. 60, 97–104. doi:10.17221/8074-CJAS
Peripolli, E., Reimer, C., Ha, N. T., Geibel, J., MacHado, M. A., Panetto, J. C. D. C., et al. (2020). Genome-wide detection of signatures of selection in indicine and Brazilian locally adapted taurine cattle breeds using whole-genome re-sequencing data. BMC Genomics 21, 624. doi:10.1186/s12864-020-07035-6
Purcell, S., Neale, B., Todd-Brown, K., Thomas, L., Ferreira, M. A. R., Bender, D., et al. (2007). PLINK: a tool set for whole-genome association and population-based linkage analyses. Am. J. Hum. Genet. 81, 559–575. doi:10.1086/519795
Purfield, D. C., Berry, D. P., McParland, S., and Bradley, D. G. (2012). Runs of homozygosity and population history in cattle. BMC Genet. 13, 70. doi:10.1186/1471-2156-13-70
Rey, C., Soubeyran, I., Mahouche, I., Pedeboscq, S., Bessede, A., Ichas, F., et al. (2013). HIPK1 drives p53 activation to limit colorectal cancer cell growth. Cell Cycle 12, 1879–1891. doi:10.4161/cc.24927
Rocha, C. C., Martins, T., Silva, F. A. C. C., Sponchiado, M., Pohler, K. G., and Binelli, M. (2023). Viperin (RSAD2) gene expression in peripheral blood mononuclear cells of pregnant crossbred beef cows is altered by Bos indicus genetics. Theriogenology 209, 226–233. doi:10.1016/j.theriogenology.2023.07.003
Roura, E., Humphrey, B., Tedó, G., and Ipharraguerre, I. (2008). Unfolding the codes of short-term feed appetence in farm and companion animals. A comparative oronasal nutrient sensing biology review. Can. J. Anim. Sci. 88, 535–558. doi:10.4141/CJAS08014
Samuel, B., and Dinka, H. (2020). In silico analysis of the promoter region of olfactory receptors in cattle (Bos indicus) to understand its gene regulation. Nucleosides, Nucleotides Nucleic Acids 39, 853–865. doi:10.1080/15257770.2020.1711524
Sangiorgio, L., Strumbo, B., Brevini, T. A. L., Ronchi, S., and Simonic, T. (2008). A putative protein structurally related to zygote arrest 1 (Zar1), Zar1-like, is encoded by a novel gene conserved in the vertebrate lineage. Comp. Biochem. Physiol. - B Biochem. Mol. Biol. 150, 233–239. doi:10.1016/j.cbpb.2008.03.007
Sempéré, G., Moazami-Goudarzi, K., Eggen, A., Laloë, D., Gautier, M., and Flori, L. (2015). WIDDE: a Web-Interfaced next generation database for genetic diversity exploration, with a first application in cattle. BMC Genomics 16, 940. doi:10.1186/s12864-015-2181-1
Sisodiya, S. M., Thompson, P. J., Need, A., Harris, S. E., Weale, M. E., Wilkie, S. E., et al. (2007). Genetic enhancement of cognition in a kindred with cone-rod dystrophy due to RIMS1 mutation. J. Med. Genet. 44, 373–380. doi:10.1136/jmg.2006.047407
Sonesson, A. K., Woolliams, J. A., and Meuwissen, T. H. E. (2012). Genomic selection requires genomic control of inbreeding. Genet. Sel. Evol. 44, 27. doi:10.1186/1297-9686-44-27
Souvenir Zafindrajaona, P., Zeuh, V., Moazami Goudarzi, K., Laloë, D., Bourzat, D., Idriss, A., et al. (1999). Etude du statut phylogénétique du bovin Kouri du lac Tchad à l’aide de marqueurs moléculaires. Rev. d’élevage médecine vétérinaire Des. pays Trop. 52, 155–162. doi:10.19182/remvt.9690
Staquicini, F. I., Qian, M. D., Salameh, A., Dobroff, A. S., Edwards, J. K., Cimino, D. F., et al. (2015). Receptor tyrosine kinase EphA5 is a functional molecular target in human lung cancer. J. Biol. Chem. 290, 7345–7359. doi:10.1074/jbc.M114.630525
Strucken, E. M., Gebrehiwot, N. Z., Swaminathan, M., Joshi, S., Al Kalaldeh, M., and Gibson, J. P. (2021). Genetic diversity and effective population sizes of thirteen Indian cattle breeds. Genet. Sel. Evol. 53, 47. doi:10.1186/s12711-021-00640-3
Sumreddee, P., Hay, E. H., Toghiani, S., Roberts, A., Aggrey, S. E., and Rekaya, R. (2021). Grid search approach to discriminate between old and recent inbreeding using phenotypic, pedigree and genomic information. BMC Genomics 22, 538. doi:10.1186/s12864-021-07872-z
Swiatek, P. J., Lindsell, C. E., Del Amo, F. F., Weinmaster, G., and Gridley, T. (1994). Notch1 is essential for postimplantation development in mice. Genes Dev. 8, 707–719. doi:10.1101/gad.8.6.707
Tan, H., Ma, L., Qin, T., Liu, K., Liu, Y., Wen, C., et al. (2024). Myo6 mediates osteoclast function and is essential for joint damage in collagen-induced arthritis. Biochim. Biophys. Acta - Mol. Basis Dis. 1870, 166902. doi:10.1016/j.bbadis.2023.166902
Taye, M., Kim, J., Yoon, S. H., Lee, W., Hanotte, O., Dessie, T., et al. (2017). Whole genome scan reveals the genetic signature of African Ankole cattle breed and potential for higher quality beef. BMC Genet. 18, 11. doi:10.1186/s12863-016-0467-1
Tenzin, J., Chankitisakul, V., and Boonkum, W. (2023). Current status and conservation management of farm animal genetic resources in Bhutan. Vet. Sci. 10, 281. doi:10.3390/vetsci10040281
Tijjani, A., Utsunomiya, Y. T., Ezekwe, A. G., Nashiru, O., and Hanotte, O. (2019). Genome sequence analysis reveals selection signatures in endangered trypanotolerant West African Muturu cattle. Front. Genet. 10, 442. doi:10.3389/fgene.2019.00442
Turner, S. D. (2018). qqman: an R package for visualizing GWAS results using Q-Q and manhattan plots. J. Open Source Softw. 3, 731. doi:10.21105/joss.00731
Vanvanhossou, S. F. U., Dossa, L. H., and König, S. (2021a). Sustainable management of animal genetic resources to improve low-input livestock production: insights into local beninese cattle populations. Sustain 13, 9874. doi:10.3390/su13179874
Vanvanhossou, S. F. U., Yin, T., Scheper, C., Fries, R., Dossa, L. H., and König, S. (2021b). Unraveling admixture, inbreeding, and recent selection signatures in West African indigenous cattle populations in Benin. Front. Genet. 12, 657282. doi:10.3389/fgene.2021.657282
Voight, B. F., Kudaravalli, S., Wen, X., and Pritchard, J. K. (2006). A map of recent positive selection in the human genome. PLoS Biol. 4, e72–e0458. doi:10.1371/journal.pbio.0040072
Wagner, K., Kunz, K., Piller, T., Tascher, G., Hölper, S., Stehmeier, P., et al. (2019). The SUMO isopeptidase SENP6 functions as a rheostat of chromatin residency in genome maintenance and chromosome dynamics. Cell Rep. 29, 480–494. doi:10.1016/j.celrep.2019.08.106
Wei, T., and Viliam, S. (2021). R package “corrplot”: visualization of a Correlation Matrix. R package version 0.92. Available at: https://github.com/taiyun/corrplot.
Weikard, R., Kühn, C., Goldammer, T., Freyer, G., and Schwerin, M. (2005). The bovine PPARGC1A gene: molecular characterization and association of an SNP with variation of milk fat synthesis. Physiol. Genomics 21, 1–13. doi:10.1152/physiolgenomics.00103.2004
Wickham, H. (2016). ggplot2: elegant graphics for data analysis. New York: Springer-Verlag. Available at: https://ggplot2.tidyverse.org.
Xia, X., Qu, K., Wang, Y., Sinding, M. H. S., Wang, F., Hanif, Q., et al. (2023). Global dispersal and adaptive evolution of domestic cattle: a genomic perspective. Stress Biol. 3, 8. doi:10.1007/s44154-023-00085-2
Yang, J., Lee, S. H., Goddard, M. E., and Visscher, P. M. (2011). GCTA: a tool for genome-wide complex trait analysis. Am. J. Hum. Genet. 88, 76–82. doi:10.1016/j.ajhg.2010.11.011
Keywords: runs of homozygosity, integrated haplotype score, cattle genetic adaptation, inbreeding effects, tropical livestock genetics, disease resistance in cattle, environmental stress adaptability
Citation: Akinsola OM, Musa AA, Muansangi L, Singh SP, Mukherjee S and Mukherjee A (2024) Genomic insights into adaptation and inbreeding among Sub-Saharan African cattle from pastoral and agropastoral systems. Front. Genet. 15:1430291. doi: 10.3389/fgene.2024.1430291
Received: 09 May 2024; Accepted: 11 July 2024;
Published: 25 July 2024.
Edited by:
Mario Barbato, University of Messina, ItalyReviewed by:
Gabrielle Becker, University of Idaho, United StatesCopyright © 2024 Akinsola, Musa, Muansangi, Singh, Mukherjee and Mukherjee. This is an open-access article distributed under the terms of the Creative Commons Attribution License (CC BY). The use, distribution or reproduction in other forums is permitted, provided the original author(s) and the copyright owner(s) are credited and that the original publication in this journal is cited, in accordance with accepted academic practice. No use, distribution or reproduction is permitted which does not comply with these terms.
*Correspondence: Abdulraheem A. Musa, bXVzYUBmYm4tZHVtbWVyc3RvcmYuZGU=
Disclaimer: All claims expressed in this article are solely those of the authors and do not necessarily represent those of their affiliated organizations, or those of the publisher, the editors and the reviewers. Any product that may be evaluated in this article or claim that may be made by its manufacturer is not guaranteed or endorsed by the publisher.
Research integrity at Frontiers
Learn more about the work of our research integrity team to safeguard the quality of each article we publish.