- 1Institute of Statistical Science, Academia Sinica, Taipei, Taiwan
- 2Department of Pathology, Chung Shan Medical University Hospital, Taichung, Taiwan
- 3Department of Medical Research, China Medical University Hospital, Taichung, Taiwan
- 4Department of Laboratory Medicine, China Medical University Hospital, Taichung, Taiwan
- 5Bioinformatics Program, Taiwan International Graduate Program, Academia Sinica, Taipei, Taiwan
- 6Data Science Degree Program, Academia Sinica and National Taiwan University, Taipei, Taiwan
- 7Genome and Systems Biology Degree Program, Academia Sinica and National Taiwan University, Taipei, Taiwan
To identify rare variants (RVs) of gout, we sequenced the whole genomes of 321 male gout patients and combined these with those of 64 male gout patients and 682 normal controls at Taiwan Biobank. We performed ACAT-O to identify 682 significant RVs (p < 3.8 × 10−8) clustered on chromosomes 1, 7, 10, 16, and 18. To prioritize causal variants effectively, we sifted them by Combined Annotation-Dependent Depletion score >10 or |effect size| ≥ 1.5 for those without CADD scores. In particular, to the best of our knowledge, we identified the rare variants rs559954634, rs186763678, and 13-85340782-G-A for the first time to be associated with gout in Taiwanese males. Importantly, the RV rs559954634 positively affects gout, and its neighboring gene NPHS2 is involved in serum urate and expressed in kidney tissues. The kidneys play a major role in regulating uric acid levels. This suggests that rs559954634 may be involved in gout. Furthermore, rs186763678 is in the intron of NFIA that interacts with SLC2A9, which has the most significant effect on serum urate. Note that gene-gene interaction NFIA-SLC2A9 is significantly associated with serum urate in the Italian MICROS population and a Croatian population. Moreover, 13-85340782-G-A significantly affects gout susceptibility (odds ratio 6.0; P = 0.038). The >1% carrier frequencies of these potentially pathogenic (protective) RVs in cases (controls) suggest the revealed associations may be true; these RVs deserve further studies for the mechanism. Finally, multivariate logistic regression analysis shows that the rare variants rs559954634 and 13-85340782-G-A jointly are significantly associated with gout susceptibility.
Introduction
Gout is a joint and excruciating inflammatory arthritis caused by hyperuricemia and inflammation dysregulation. Furthermore, gout and serum urate levels are heritable. Several genes reported to be associated with serum uric acid (SUA) or gout are involved in the renal urate transporter system, such as SLC22A12 (Enomoto et al., 2002), SLC2A9 (Matsuo et al., 2008; Dinour et al., 2010) and ABCG2 (Matsuo et al., 2009; Higashino et al., 2017) that modulate uric acid levels (Reginato et al., 2012; Chen et al., 2018). However, the pathogenic mechanisms of hyperuricemia and gout differ. Furthermore, although many common variants of gout have been discovered, they can not fully explain its susceptibility. Moreover, most of the 400 million detected variants from the ∼53 K diverse human genomes are rare, and functional variants tend to be rare (Taliun et al., 2021). Thus, we aim to discover novel rare variants that may cause gout in this study.
Many common variants associated with gout have been reported, e.g., rs22331142 in ABCG2 (Chen et al., 2018) in a Taiwanese population. Matsuo and colleagues sequenced ABCG2 to reveal multiple common and rare variants in a Japanese population (Higashino et al., 2017). Nevertheless, most of the variants for gout identified thus far are common variants (MAF > 5%). In this study, we aim to identify rare variants (MAF < 1%) that are associated with gout in male Taiwanese, as rare variants can have much larger per-allele effect sizes than common variants (Cheng et al., 2022). We integrated whole genome sequencing (WGS) data of 321 male gout patients from China Medical University Hospital (CMUH), 64 male gout patients, and 682 normal controls from Taiwan Biobank (TWB), to reveal rare variants associated with gout disease.
As rare variants appear very infrequently in the population, set-based methods that jointly analyze variants in a set are much more powerful than the single-variant analysis applied to common variants in genome-wide association studies (GWASs). Thus, we applied ACAT-O (Liu et al., 2019), which combines the strength of the sequence kernel association test (SKAT; Wu et al., 2011), the burden test (Li and Leal, 2008; Madsen and Browning, 2009; Price et al., 2010) and ACAT-V test (Liu et al., 2019). The power of ACAT-O to detect rare variants is robust against the sparsity of causal variants and the directionality of effects. After the rare variants associated with gout are detected, we further sift these variants by their Combined Annotation-Dependent Depletion (CADD (Kircher et al., 2014; Rentzsch et al., 2019; van der Velde et al., 2015), scores. CADD is a widely used measure of variant deleteriousness, and it can prioritize causal variants in genetic analysis. Next, we compute the odds ratio and proportion test of the rare variants with CADD ≥10 or |effect size| ≥ 1.5 for those without CADD scores.
Materials and methods
Study population
This study aimed to identify genes and rare variants associated with gout in Taiwanese male patients. Because there were no normal controls at China Medical University Hospital(CMUH henceforth, Taichung, Taiwan), we integrated the WGS data from CMUH with those from the Taiwan Biobank (TWB henceforth, Taipei, Taiwan) database. There were 321 male gout patients recruited at CMUH; 64 male gout patients and 682 normal controls (with serum urate level <6 mg/dL; according to the 2012 guideline of the American College of Rheumatology) from TWB, which provided WGS data, age, gout annotation, and serum urate levels for each sample. The 746 WGS data from TWB were the total male subjects sequenced when we started this investigation. The clinical information of gout patients and normal control analyzed are in Supplementary Table S1.
TWB is a database established in 2012 for research, a prospective study of 200,000 individuals aged 30–70 recruited from Taiwan. TWB consists of individuals, predominantly of Han Chinese ancestry, whose genomic profiles were integrated with lifestyle patterns to study the relationships between diseases and genetics. All participants underwent biochemical tests with blood and urine specimens and physical examination; they all signed informed consent for genotyping. The phenotype of gout disease was the self-report of physician-diagnosed gout, which was reported to retain good reliability and sensitivity (McAdams et al., 2011).
The IRB-BM committee of Academia Sinica (AS-IRB02-113170) and the CMUH Institutional Review Board in Taiwan (CMUH108-REC1-091) approved this study. All data from human participants were obtained from CMUH and the Taiwan Biobank database, for which data sharing and data linkage were parts of the consent, so a waiver of consent was granted by the CMUH IRB. Both the Declaration of Helsinki and the Good Clinical Practice Guidelines were followed, and informed consent was signed by all participants.
Genotyping
The whole genomes of male gout patients at CMUH and participants of TWB were sequenced by the Illumina NovaSeq 6000 platform at least 30x depth, mapped to reference genome (hg38) by Dragon and BWA, and variant calling (.gvcf) performed by Dragon and GATK, respectively; both .gvcf files were annotated by VEP (McLaren et al., 2016). As we only assessed .vcf files from TWB, we combined the .bed files (converted by PLINK version 1.9) from the two sources. Because the batch effect of the union of the called variants from both sites could not be adjusted well even using 99 principle components (PCs), we intersected both sets of variants to result in the overlappings, and we called these SNPs CMUH_TWB data henceforth.
Quality control
The standard per-individual and per-marker quality control (Anderson, et al., 2010) were performed on the CMUH_TWB data using PLINK 1.9 software. We first adopted the procedure of per-individual quality control. Individuals were excluded if identification of 1) individuals with incorrectly recorded or missing sex status, 2) individuals with genotyping call rates below 90% or outlying heterozygosity rate, namely homozygosity rate out of the sample mean ±3 s.e. confidence bounds, 3) individuals of divergent ancestry, or 4) uric acid levels missing. Moreover, we implemented per-marker quality control procedures to remove SNPs if 1) genotyping call rate below 100% (i.e., no missing allowed) or significant deviation from Hardy-Weinberg equilibrium in the controls (p < 0.001 and MAF >1%).
Finally, SNPs with MAF <1% were called to result in 8,703,559 rare variants, which satisfied the above QC procedures, from 287 CMUH and 63 TWB male gout patients and 671 TWB male controls. Supplementary Figure S1 shows that all subjects from CMUH and TWB share the same genetic background.
Batch effect and population stratification correction by PCA
To adjust the effect that the integrated SNPs were from two resources and population stratification (different ethnic groups among Taiwanese, e.g. Fujian and Hakka), we performed PCA on SNPs with MAF >1% and satisfying the Hardy-Weinberg equilibrium QC. When plotted against PC9 and PC10, the SNPs of the two sites were finally mixed, so the first nine PCs were used for correction of the batch effects; PCA plots are in Supplementary Figure S2).
Uncovering significant rare variants by ACAT-O
We applied the aggregated Cauchy association test (ACAT-O (Liu, et al., 2019); in R software to identify significant rare variants. ACATO is a set-based test that is particularly powerful when sparse causal variants exist in a variant set (window). ACAT-O first transforms the p-value of the burden test (Li and Leal, 2008; Madsen and Browning, 2009; Price, et al., 2010), SKAT test (Wu, et al., 2011), and ACAT-V (Liu, et al., 2019), to Cauchy variables. Then ACAT-O combines the above variables, each with two choices of weights, as the test statistic and evaluates the significance. We used the default Beta (1, 25) and Beta (1, 1) for the above two weights.
We split the 8,703,559 rare variants into ∼1.31 M windows of 4 kb with 2 kb overlaps in adjacent windows. In each window, we conducted ACAT-V, which implemented a logistic regression of gout status on all variants in the window conditional on covariates of age, uric acid levels, and the aforementioned nine PCs; similarly, the burden test and SKAT were also implemented. The significance threshold of ACAT-O was p < 3.8 × 10−8 after Bonferroni correction for multiple testing of ∼1.31 million windows.
Statistical analysis
There are 682 rare variants (MAF < 1%) among the 61 windows uncovered to be significantly associated with gout susceptibility by ACAT-O (P < 3.8 × 10−8), and these RVs are deemed significant according to ACAT-O. For each significant rare variant, we computed the odds ratio and the associated 95% confidence interval, as well as the two-sample t-test for the equality of the proportion in cases and controls. Finally, logistic regression adjusted on serum urate levels was performed to evaluate the effects of rare variants on gout susceptibility.
Results
Study population
This study includes 350 male gout patients (287 from China Medical University Hospital (CMUH henceforth) and 63 from the Taiwan Biobank database (TWB henceforth)), whose data passed our QC procedures. In addition, it also included 671 male normal controls from TWB, which provided gout history, uric acid levels, demographic, and whole genome sequencing data. There were 40, 644, 135 and 42, 571, 357 SNPs called from the WGS data of CMUH and TWB, respectively. We intersected the SNP called from the two sites (CMUH_TWB) and subjected the 18, 426, 362 overlapping SNPs to quality check, of which 8,703,559 rare variants (MAF < 1%) satisfied the QC procedures; please see SNP genotyping and quality control in Materials and Methods for details.
The mean age of male gout patients and normal controls were 49.56 years (±15.99) and 49.69 years (±11.58), respectively, which was not significantly different (P = 0.888). There was too many mssing values of the covariate BMI for patients from CMUH, so BMI was not analyzed. However, uric acid of the two groups was significantly different (P < 2.2 × 10−16); see Supplementary Table S1 for details. In the subsequent analysis, serum urate was treated as a potential confounder of gout.
Results of rare variant association tests
The overall rare-variant analysis identified 61 windows significantly associated with gout disease (p < 3.8 × 10−8; ACAT-O), out of 1,314,794 windows analyzed. These windows consist of 682 distinct rare variants (Supplementary Table S2). The Manhattan plot depicted all p-values after the negative logarithm transformation of all ∼8.7 million RVs that passed QC across 22 pairs of autosomal chromosomes; note all RVs in a window share the same p-value from ACAT-O (Figure 1A). We further used balls with sizes proportional to the number of significant rare variants in each window (Figure 1B), and found significant RVs are clustered on chromosomes 1, 7, 10, 16, and 18.
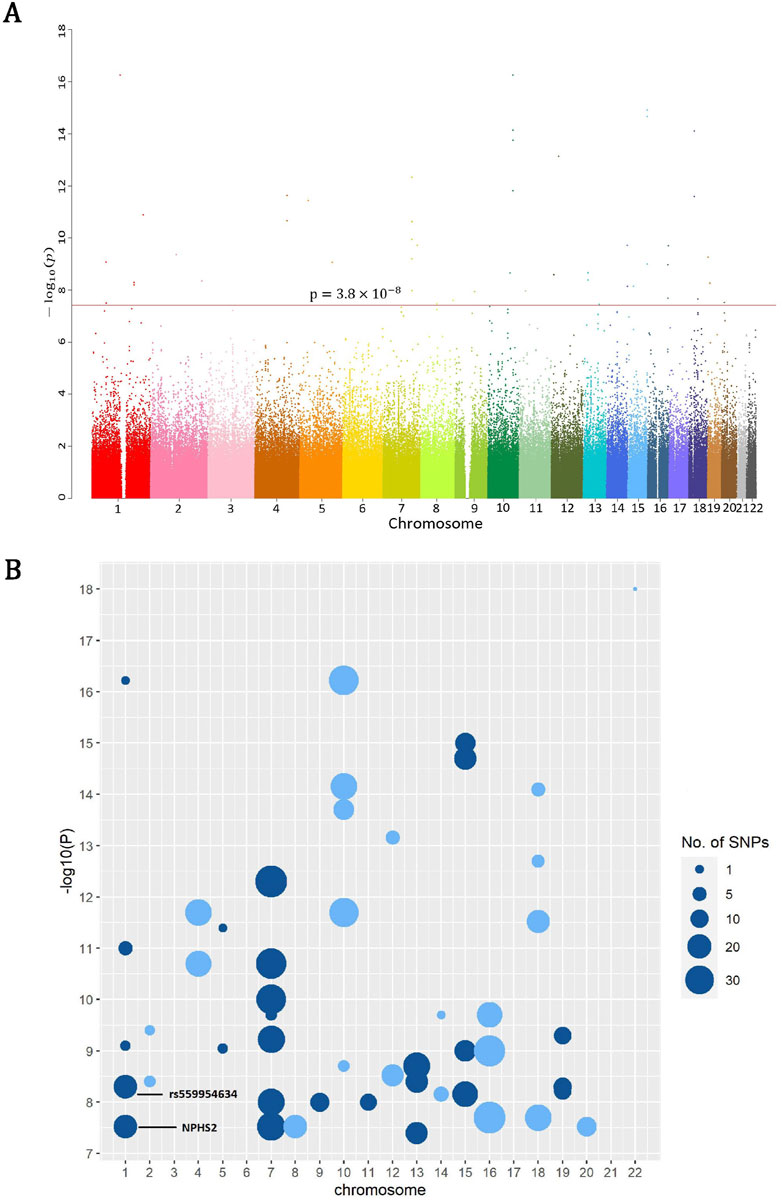
Figure 1. (A) The Manhattan plot depicted all p-values after the negative logarithm transformation of all ∼8.7 million RVs which passed QC across 22 pairs of autosomal chromosomes, where the red line corresponds to the threshold 3.8 × 10−8 (ACAT-O) for significant rare variants. (B) The log10 (1/P) of rare variants significantly associated with gout susceptibility show clusters in chromosomes 1, 7, 10, 16 and 18, where larger balls denote more variants in the corresponding windows.
Of the 682 significant rare variants, we further sifted these by CADD scores (Kircher, et al., 2014; Rentzsch, et al., 2019; van der Velde, et al., 2015) greater than 10; CADD is a widely used measure for variant deleteriousness that can prioritize causal variants effectively in genetic analysis, in particular highly penetrate contributors to severe Mendelian disorders. CADD is an annotation integrated from >60 genomic features, such as surrounding sequence context and gene model annotations. For any given variant, all the features are integrated into a CADD score which is a phred-scale rank score for all ∼9 billion potential single nucleotide variants (Rentzsch et al., 2019).
In particular, we identified rare variant rs559954634 (p = 6.4 × 10−9; ACAT-O; CADD = 13.5), and rs186763678 (p = 3.2 × 10−8; ACAT-O; CADD = 10.5) which is in intron of NFIA. NFIA is known to interact with SLC2A9 that has the largest effect on serum urate levels. Gene-gene interaction NFIA-SLC2A9 was reported to be significantly associated with serum urate in the Italian MICROS population (n = 1,201) and replicated in a Croatian population (n = 1772) (Wei, et al., 2011). The rare variant rs186763678 has an effect size of −1.79, odds ratio 0.24 (P = 0.176), and the proportion test of gout patients versus controls (P = 0.075) in this study.
The rare variant rs559954634 positively affects gout susceptibility (odds ratio = 4.85; P = 0.060); this is the first report. This variant has been annotated in dbSNP (Sherry, et al., 2001) but not in ClinVar (Landrum, et al., 2018) and GWAS (Uffelmann, et al., 2021). Table 1 consists of significant rare variants (p < 3.8 × 10−8; ACAT-O) with CADD score >10 or without CADD scores but |effect size| > 1.5, as WGS may reveal unannotated rare variants, so we also report the latter. Supplementary Table S3-1 summarizes their effect sizes, odds ratios, P values (the proportion test of gout patients versus normal controls, and logistic regression adjusted on serum urate), and CADD phred scores.
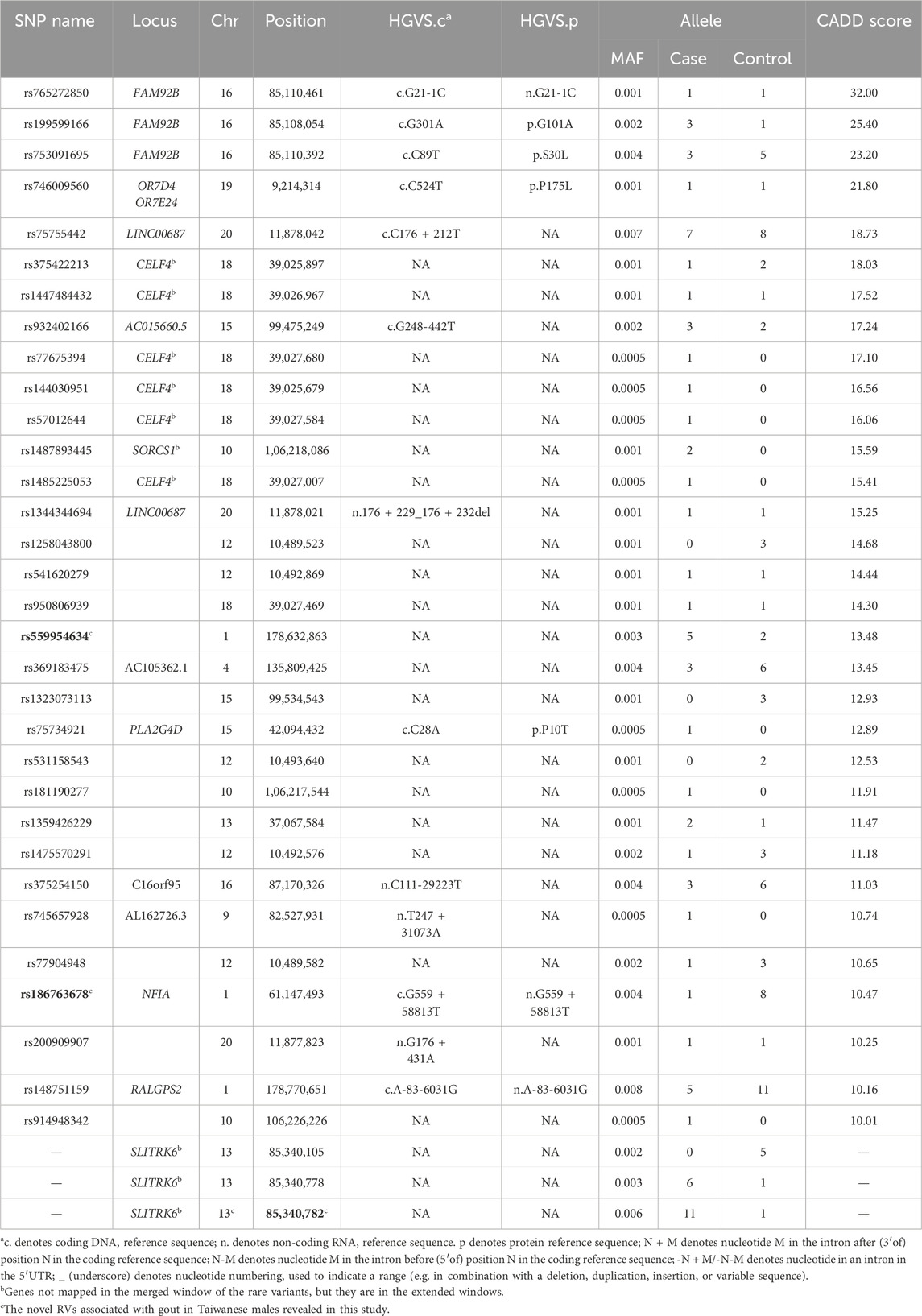
Table 1. The significant rare variants with CADD ≥10 or without CADD score but |effect size| ≥ 1.5 found in this study.
Next, we merged adjacent significant windows of ACAT-O into larger ones and extended both edges of these windows upstream and downstream 1 Mb. Of these extended windows, the window of rs559954634 intersected with NPHS2, which is involved in serum urate and indirectly linked to gout through hyperuricemia. Moreover, NPHS2 expresses in kidney tissues (queried from GTEx portal), and the kidneys play a major role in regulating uric acid levels. This suggests rs559954634 may be involved in gout, though further research is warranted. All overlapping genes in the 1 Mb-extended windows of rs559954634 are summarized in Supplementary Table S3-2. Moreover, there are 39 genes in the 1-Mb extended window of rs186763678; however, none is related to gout or serum urate, so we summarize these 39 genes in Supplementary Table S3-3.
Prioritization of the rare variants in NPHS2
Gene NPHS2 encodes podocin, which is directly involved with serum urate levels (hyperuricemia; (Romi et al., 2017). Of the rare variants that passed the QC checks, 39 distinct rare variants are in NPHS2; however, none is significant in ACAT-O. Among these rare variants, rs202036853 (effect size = 5.79) shows a nominal association with gout adjusted on serum urate (P = 0.038; logistic regression) and has a CADD score of 11.0. Figure 2A shows the positions of these variants and the corresponding effect sizes. Figure 2B depicts that the rare variants of NPHS2 with higher CADD scores are associated with more significant positive effects on gout susceptibility. The effect size, proportion test, logistic regression, and CADD score of these variants in NPHS2 are in Supplementary Table S3-4.
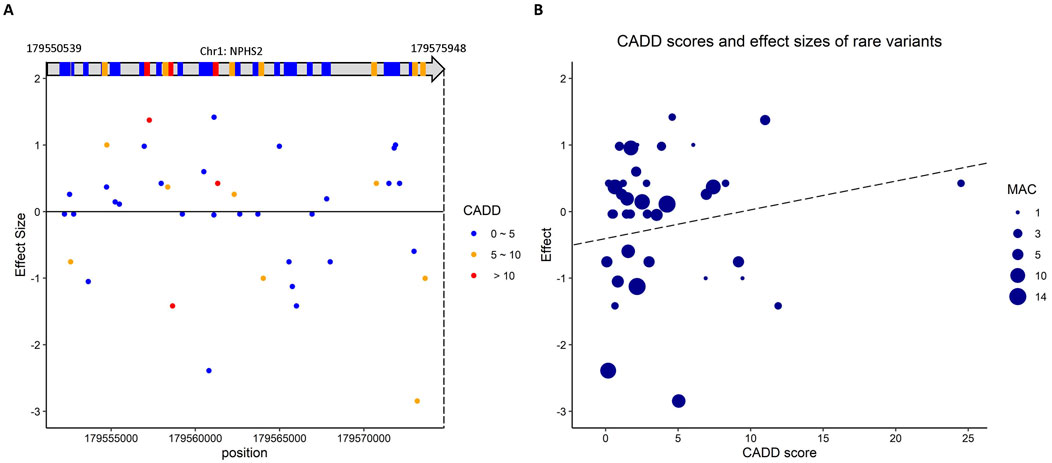
Figure 2. The putative rare variants in NPHS2 which is involved in serum urate and indirectly linked to gout. (A) The scheme shows the positions of these variants in NPHS2 and the corresponding effect sizes. (B) the rare variants of NPHS2 with higher CADD scores are associated with larger positive effects on gout susceptibility.
Another novel rare variant discovered
We also uncovered a novel rare variant 13-85340782-G-A (chr. 13; P = 3.5 × 10−8; ACAT-O), which has an odds ratio of 6.02 (P = 0.038) and is significant in the proportion test (P = 0.002). Two other RVs in the same window of 13-85340782-G-A also have |effect size| > 1.5, but their odds ratio for gout is insignificant (Supplementary Table S3-1). The RV 13-85340782-G-A is significantly associated with gout (P = 0.038; logistic regression), but the significance diminished when adjusted on serum urate (P = 0.124; logistic regression), which may indicate the association of this variant with gout is through serum urate. The 1 Mb-extended windows of this rare variant do not intersect with any known gene involved in gout or serum urate. The genes in this 1 Mb-extended window are in Supplementary Table S3-5.
Rs559954634 and 13-85340782-G-A jointly are significantly associated with gout susceptibility
Recently, several genetic studies have reported that multiple rare variants play essential roles in complex genetic diseases, e.g., Alzheimer’s disease (Cruchaga et al., 2014), which support the “Common Disease Multiple Rare Variants” (CDMRVs) or “Common Disease Rare Variants” (CDRV) hypothesis (Schork et al., 2009). CDMRV and CDRV hypotheses argue that multiple rare variants and a single rare variant, each with relatively high penetrance, are the major contributors to common diseases, respectively. In this section, we applied multivariate logistic regression analysis to evaluate the joint effect of the rare variant in NFIA rs186763678, rs559954634, and 13-85340782-G-A discovered by ACAT-O and CADD scores/or effect size. The results support the “CDMRVs” hypothesis at P < 0.06 (multiple logistic regression). Specifically, univariate logistic regression analysis reveals that rs559954634 and 13-85340782-G-A are significantly associated with gout susceptibility (Table 2). When analyzed jointly, 13-85340782-G-A (beta = 1.80, P = 0.037; OR = 6.06) and rs559954634 (beta = 1.60, P = 0.057; OR = 4.93) are significantly associated with gout susceptibility at P < 0.06; the result supports CDMRVs at a non-stringent criteria.
Rare variants in known gout-related genes ABCG2, SLC2A9 and SLC22A12
This study found 16 (16, 3) rare variants in the known gout-related gene ABCG2 (SLC2A9, SLC22A12). However, none of these are significant in ACAT-O; the smallest P values of these variants in ABCG2, SLC2A9, and SLC22A12 are 0.004, 0.012, and 0.122, respectively (Supplementary Table S4-1). This may be due to our samples and those in previous studies being of different ancestry or our sample size not being large (under budget constraints). The position, allele frequency, and CADD score of these variants are summarized in Supplementary Table S4-2.
In the following, we report the rare variants in these genes, which have CADD scores ≥10 and are significant in both the odds ratio and the proportion test. The rare variant rs199897813 in ABCG2 has a CADD score of 28.3, an effect size of 1.96, and an odds ratio equal to 9.71 (P = 0.038), respectively, and its proportion test of patients versus controls has P = 0.051. Moreover, three rare variants identified in ABCG2, rs548254708, rs34678167, and rs149106245, were previously reported in a Japanese cohort (Higashino et al., 2017), but neither is significant in odds ratio and the proportion test in this study; this is reasonable as individuals of these two cohorts are of different ancestry. Of the rare variant revealed in SLC2A9, rs150391338 has a CADD score of 10.4, effect size of −2.37, odds ratio 0.25 (P = 0.068), and it is significant in the proportion test (p = 0.018); detailed information is summarized in Supplementary Table S4-3.
The rare variants in the remaining merged windows
We also computed the odds ratio and conducted the proportion test in the remaining merged windows of the significant rare variants (P < 3.8 × 10−8; ACAT-O). However, none of the rare variants therein satisfies both CADD ≥10 and is significant in odds ratio or the proportion test (P < 0.10).
Discussion
Rare variants often contribute to complex diseases with large effect sizes per allele; however, the power to detect these variants remains limited (Chen et al., 2022). This study integrated data from male gout patients from CMUH and TWB and normal controls of TWB to identify 682 significant rare variants by ACAT-O. We further sifted these variants by the measure of variant deleteriousness CADD >10 (|effect size| > 1.5) to find rs559954634 and rs186763678 (13-85340782-G-A). These variants have been identified for the first time as associated with gout susceptibility in Taiwanese males. The carrier frequency of potentially pathogenic rs559954634 and 13-85340782-G-A is higher in gout patients when compared to normal controls (1.4% versus 0.30%; P = 0.046, and 3.1% versus 0.15%; P = 0.011; the proportion test). Conversely, the carrier frequency of potentially protective variant rs186763678 is higher in controls than in patients (0.29% versus 1.2%; P = 0.037; the proportion test). The identified potentially pathogenic (protective) RVs with a prevalence >1% in cases (controls) indicate that these RVs may be associated with gout in this population. Nevertheless, further studies are warranted, as the inflation factor λ of the ∼8.7 million QC-passed RVs is moderate (1.42).
In the neighborhood of rs559954634, we found NPHS2 that encodes podocin, which is directly involved in hyperuricemia (Romi et al., 2017). Moreover, NPHS2 is expressed in kidney tissues (queried from the GTEx portal), and the kidneys play a major role in regulating uric acid levels. This suggests that rs559954634 may be involved in gout, though further research is warranted. The rare variant rs186763678 is in the intron of NFIA which interacts with SLC2A9, while SLC2A9 is known for lowering serum urate and protecting gout (Tin et al., 2018). The RV 13-85340782-G-A has an odds ratio of 6.06 (P = 0.037) and a large effect size (3.15).
We caution that these findings were statistically significant and prioritized by CADD scores, but the revealed RVs have not been verified biologically.
ACAT-O is a sliding-window-based method that uses the conventional 4 kb window size with 2 kb overlaps. Varying the window size to 5 kb (3 kb), we reran ACAT-O to yield the p value of rs559954634, rs186763678, and 13-85340782-G-A equal to 6.2 × 10−9, 3.15 × 10−8, and 3.4 × 10−8 (6.36 × 10−9, 3.15 × 10−8, and 3.40 × 10−8), respectively; all the three RVs remain significant for the ACAT-O (with 5 kb-windows) cutoff 4.7 × 10−8 (resulted from ∼1.06 million windows), but only rs559954634 is significant for the ACAT-O (with 3 kb-windows) cutoff 2.86 × 10−8 (resulted from ∼1.78 million windows). As the number of windows increases, e.g., prespecifying 3 kb for window size, the Bonferroni correction for multiple testing becomes too conservative, leading to power loss. Furthermore, it is noted in Li et al. (2019) that the sliding window methods are likely to lose power if the pre-specified window size is too big because it might include too many neutral variants, or if the pre-specified window size is too small that it might exclude adjacent regions containing association signals. To circumvent the difficulty of specifying a window size a priori, Li and colleagues introduced a dynamic scan procedure (SCANG) to flexibly detect the sizes and locations of RV association regions of WGS studies. Thus, applying SCANG to our data to yield robust RVs of gout in Taiwanese males is an interesting future research. As the number of subjects in this study is moderate, it will be valuable to further sequence whole genomes of male gout patients in Taiwan or analyze an independent cohort, such as the UK Biobank, to confirm/compare these unraveled RVs. Moreover, the combination of individual variants into a polygenic risk score (PRS) has the potential to be a predictor for a disease (Elliott et al., 2020); it may be helpful for the diagnosis of gout, in addition to increasing statistical power to detect genetic associations. Therefore, deriving a PRS using the identified 682 rare variants and integrating our data with WGS data of individuals of other ancestry, e.g., the UK Biobank, are also promising future research directions for gout study.
Data availability statement
The datasets presented in this article are not readily available because the data of 64 male gout patients and normal controls from TWB belongs to the Taiwan Biobank and can be accessed upon application at: https://www.biobank.org.tw/. The data of 321 male gout patients at CMUH are under IRB restriction and thus are not publicly available. Requests to access the datasets should be directed to the corresponding authors and with permission of the CMUH. However, WGS summary statistics for the CMUH-TWB data are available at https://staff.stat.sinica.edu.tw/gshieh/WGS-data.htm and at GWAS catalog ID: GCST90432173.
Ethics statement
The IRB-BM committee of Academia Sinica (AS-IRB02-113170) approved this study. The studies involving humans were approved by The ethics committee of the China Medical University Hospital Institutional Review Board in Taiwan (CMUH108-REC1-091) approved this study. The studies were conducted in accordance with the local legislation and institutional requirements. The participants provided their written informed consent to participate in this study.
Author contributions
Y-PT: Writing–original draft, Formal Analysis, Software, Visualization. Y-SC: Data curation, Resources, Writing–review and editing. VM: Formal Analysis, Writing–review and editing. T-YL: Writing–review and editing, Data curation, Resources. J-GC: Writing–review and editing, Conceptualization, Funding acquisition, Methodology, Project administration, Supervision. GS: Conceptualization, Methodology, Supervision, Writing–review and editing, Funding acquisition, Project administration, Formal Analysis, Investigation, Writing–original draft.
Funding
The author(s) declare that financial support was received for the research, authorship, and/or publication of this article. This research was supported by Academia Sinica, Taiwan (Tukey and Group research Grant to GS.S); and National Science & Technology Council, Taiwan, Republic of China (111-2320-B-039-064 to J.G.C, and 111-2118-M-001-009-MY2 and 113-2118-M-001-002 to GS.S.).
Acknowledgments
We are grateful to the AE and two anomynous reviewers for constructive comments and suggestions that improved earlier versions of our manuscript. We thank Chia-Ni Hsiung at the Data Science Statistical Cooperation Center of Academia Sinica (AS-CFII-111-215) for statistical support, and Shi-Kai Chu and Yu-Jen Liang for discussions.
Conflict of interest
The authors declare that the research was conducted in the absence of any commercial or financial relationships that could be construed as a potential conflict of interest.
The author(s) declared that they were an editorial board member of Frontiers, at the time of submission. This had no impact on the peer review process and the final decision.
Publisher’s note
All claims expressed in this article are solely those of the authors and do not necessarily represent those of their affiliated organizations, or those of the publisher, the editors and the reviewers. Any product that may be evaluated in this article, or claim that may be made by its manufacturer, is not guaranteed or endorsed by the publisher.
Supplementary material
The Supplementary Material for this article can be found online at: https://www.frontiersin.org/articles/10.3389/fgene.2024.1423714/full#supplementary-material
References
Anderson, C. A., Pettersson, F. H., Clarke, G. M., Cardon, L. R., Morris, A. P., and Zondervan, K. T. (2010). Data quality control in genetic case-control association studies. Nat. Protoc. 5 (9), 1564–1573. doi:10.1038/nprot.2010.116
Chang, Y.-S., Lin, C.-Y., Liu, T.-Y., Huang, C.-M., Chung, C.-C., Chen, Y.-C., et al. (2022). Polygenic risk score trend and new variants on chromosome 1 are associated with male gout in genome-wide association study. Arthritis Res. and Ther. 24 (1), 229. doi:10.1186/s13075-022-02917-4
Chen, C.-J., Tseng, C.-C., Yen, J.-H., Chang, J.-G., Chou, W.-C., Chu, H.-W., et al. (2018). ABCG2 contributes to the development of gout and hyperuricemia in a genome-wide association study. Sci. Rep. 8 (1), 3137. doi:10.1038/s41598-018-21425-7
Chen, W., Coombes, B. J., and Larson, N. B. (2022). Recent advances and challenges of rare variant association analysis in the biobank sequencing era. Front. Genet. 13, 1014947. doi:10.3389/fgene.2022.1014947
Cruchaga, C., Karch, C. M., Jin, S. C., Benitez, B. A., Cai, Y., Guerreiro, R., et al. (2014). Rare coding variants in the phospholipase D3 gene confer risk for Alzheimer’s disease. Nature 505 (7484), 550–554. doi:10.1038/nature12825
Dinour, D., Gray, N. K., Campbell, S., Shu, X., Sawyer, L., Richardson, W., et al. (2010). Homozygous SLC2A9 mutations cause severe renal hypouricemia. J. Am. Soc. Nephrol. JASN 21 (1), 64–72. doi:10.1681/ASN.2009040406
Elliott, J., Bodinier, B., Bond, T. A., Chadeau-Hyam, M., Evangelou, E., Moons, K. G., et al. (2020). Predictive accuracy of a polygenic risk score–enhanced prediction model vs a clinical risk score for coronary artery disease. Jama 323 (7), 636–645. doi:10.1001/jama.2019.22241
Enomoto, A., Kimura, H., Chairoungdua, A., Shigeta, Y., Jutabha, P., Ho Cha, S., et al. (2002). Molecular identification of a renal urate–anion exchanger that regulates blood urate levels. Nature 417 (6887), 447–452. doi:10.1038/nature742
Higashino, T., Takada, T., Nakaoka, H., Toyoda, Y., Stiburkova, B., Miyata, H., et al. (2017). Multiple common and rare variants of ABCG2 cause gout. RMD open 3 (2), e000464. doi:10.1136/rmdopen-2017-000464
Kircher, M., Witten, D. M., Jain, P., O'roak, B. J., Cooper, G. M., and Shendure, J. (2014). A general framework for estimating the relative pathogenicity of human genetic variants. Nat. Genet. 46 (3), 310–315. doi:10.1038/ng.2892
Landrum, M. J., Lee, J. M., Benson, M., Brown, G. R., Chao, C., Chitipiralla, S., et al. (2018). ClinVar: improving access to variant interpretations and supporting evidence. Nucleic acids Res. 46 (D1), D1062-D1067–D1067. doi:10.1093/nar/gkx1153
Li, B., and Leal, S. M. (2008). Methods for detecting associations with rare variants for common diseases: application to analysis of sequence data. Am. J. Hum. Genet. 83 (3), 311–321. doi:10.1016/j.ajhg.2008.06.024
Li, Z., Li, X., Liu, Y., Shen, J., Chen, H., Zhou, H., et al. (2019). Dynamic scan procedure for detecting rare-variant association regions in whole-genome sequencing studies. Am. J. Hum. Genet. 104 (5), 802–814. doi:10.1016/j.ajhg.2019.03.002
Liu, Y., Chen, S., Li, Z., Morrison, A. C., Boerwinkle, E., and Lin, X. (2019). ACAT: a fast and powerful p value combination method for rare-variant analysis in sequencing studies. Am. J. Hum. Genet. 104 (3), 410–421. doi:10.1016/j.ajhg.2019.01.002
Madsen, B. E., and Browning, S. R. (2009). A groupwise association test for rare mutations using a weighted sum statistic. PLoS Genet. 5 (2), e1000384. doi:10.1371/journal.pgen.1000384
Matsuo, H., Chiba, T., Nagamori, S., Nakayama, A., Domoto, H., Phetdee, K., et al. (2008). Mutations in glucose transporter 9 gene SLC2A9 cause renal hypouricemia. Am. J. Hum. Genet. 83 (6), 744–751. doi:10.1016/j.ajhg.2008.11.001
Matsuo, H., Takada, T., Ichida, K., Nakamura, T., Nakayama, A., Ikebuchi, Y., et al. (2009). Common defects of ABCG2, a high-capacity urate exporter, cause gout: a function-based genetic analysis in a Japanese population. Sci. Transl. Med. 1 (5), 5ra11–15ra11. doi:10.1126/scitranslmed.3000237
McAdams, M. A., Maynard, J. W., Baer, A. N., Köttgen, A., Clipp, S., Coresh, J., et al. (2011). Reliability and sensitivity of the self-report of physician-diagnosed gout in the campaign against cancer and heart disease and the atherosclerosis risk in the community cohorts. J. rheumatology 38 (1), 135–141. doi:10.3899/jrheum.100418
McLaren, W., Gil, L., Hunt, S. E., Riat, H. S., Ritchie, G. R., Thormann, A., et al. (2016). The ensembl variant effect predictor. Genome Biol. 17, 122. doi:10.1186/s13059-016-0974-4
Price, A. L., Kryukov, G. V., de Bakker, P. I., Purcell, S. M., Staples, J., Wei, L.-J., et al. (2010). Pooled association tests for rare variants in exon-resequencing studies. Am. J. Hum. Genet. 86 (6), 832–838. doi:10.1016/j.ajhg.2010.04.005
Reginato, A. M., Mount, D. B., Yang, I., and Choi, H. K. (2012). The genetics of hyperuricaemia and gout. Nat. Rev. Rheumatol. 8 (10), 610–621. doi:10.1038/nrrheum.2012.144
Rentzsch, P., Witten, D., Cooper, G. M., Shendure, J., and Kircher, M. (2019). CADD: predicting the deleteriousness of variants throughout the human genome. Nucleic acids Res. 47 (D1), D886-D894–D894. doi:10.1093/nar/gky1016
Romi, M. M., Arfian, N., Tranggono, U., Setyaningsih, W. A. W., and Sari, D. C. R. (2017). Uric acid causes kidney injury through inducing fibroblast expansion, Endothelin-1 expression, and inflammation. BMC Nephrol. 18, 326–328. doi:10.1186/s12882-017-0736-x
Schork, N. J., Murray, S. S., Frazer, K. A., and Topol, E. J. (2009). Common vs. rare allele hypotheses for complex diseases. Curr. Opin. Genet. and Dev. 19 (3), 212–219. doi:10.1016/j.gde.2009.04.010
Sherry, S. T., Ward, M.-H., Kholodov, M., Baker, J., Phan, L., Smigielski, E. M., et al. (2001). dbSNP: the NCBI database of genetic variation. Nucleic acids Res. 29 (1), 308–311. doi:10.1093/nar/29.1.308
Taliun, D., Harris, D. N., Kessler, M. D., Carlson, J., Szpiech, Z. A., Torres, R., et al. (2021). Sequencing of 53,831 diverse genomes from the NHLBI TOPMed Program. Nature 590 (7845), 290–299. doi:10.1038/s41586-021-03205-y
Tin, A., Li, Y., Brody, J. A., Nutile, T., Chu, A. Y., Huffman, J. E., et al. (2018). Large-scale whole-exome sequencing association studies identify rare functional variants influencing serum urate levels. Nat. Commun. 9 (1), 4228. doi:10.1038/s41467-018-06620-4
Uffelmann, E., Huang, Q. Q., Munung, N. S., De Vries, J., Okada, Y., Martin, A. R., et al. (2021). Genome-wide association studies. Nat. Rev. Methods Prim. 1 (1), 59. doi:10.1038/s43586-021-00056-9
van der Velde, K. J., Kuiper, J., Thompson, B. A., Plazzer, J. P., van Valkenhoef, G., de Haan, M., et al. (2015). Evaluation of CADD scores in curated mismatch repair gene variants yields a model for clinical validation and prioritization. Hum. Mutat. 36 (7), 712–719. doi:10.1002/humu.22798
Wei, W., Hemani, G., Hicks, A. A., Vitart, V., Cabrera-Cardenas, C., Navarro, P., et al. (2011). Characterisation of genome-wide association epistasis signals for serum uric acid in human population isolates. PLoS One 6 (8), e23836. doi:10.1371/journal.pone.0023836
Keywords: association study, CADD, gout, rare variant, serum urate, whole-genome sequencing
Citation: Tseng Y-P, Chang Y-S, Mekala VR, Liu T-Y, Chang J-G and Shieh GS (2024) Whole-genome sequencing reveals rare variants associated with gout in Taiwanese males. Front. Genet. 15:1423714. doi: 10.3389/fgene.2024.1423714
Received: 26 April 2024; Accepted: 28 August 2024;
Published: 25 September 2024.
Edited by:
Jing Chen, Cincinnati Children’s Hospital Medical Center, United StatesReviewed by:
Qing Li, Vanderbilt University Medical Center, United StatesXinping Cui, University of California, Riverside, United States
Copyright © 2024 Tseng, Chang, Mekala, Liu, Chang and Shieh. This is an open-access article distributed under the terms of the Creative Commons Attribution License (CC BY). The use, distribution or reproduction in other forums is permitted, provided the original author(s) and the copyright owner(s) are credited and that the original publication in this journal is cited, in accordance with accepted academic practice. No use, distribution or reproduction is permitted which does not comply with these terms.
*Correspondence: Jan-Gowth Chang, amdjaGFuZzk5QGdtYWlsLmNvbQ==; Grace S. Shieh, Z3NoaWVoQHN0YXQuc2luaWNhLmVkdS50dw==
†These authors have contributed equally to this work
‡Present address: Ya-Sian Chang, Precision and Cell Center, Show Chwan Memorial Hospital, Changhua, TaiwanJan-Gowth Chang, Office of Superintendent, Hualien Tzu Chi Hospital, Hualien, Taiwan