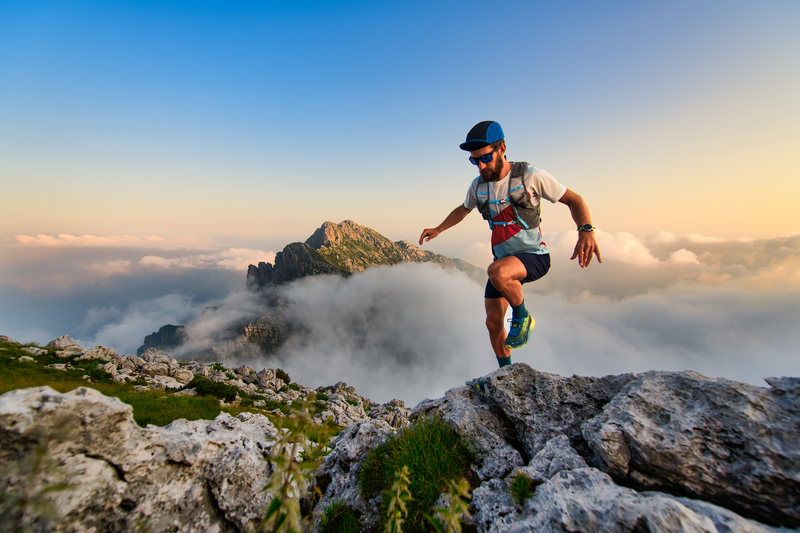
95% of researchers rate our articles as excellent or good
Learn more about the work of our research integrity team to safeguard the quality of each article we publish.
Find out more
DATA REPORT article
Front. Genet. , 21 August 2024
Sec. RNA
Volume 15 - 2024 | https://doi.org/10.3389/fgene.2024.1421955
Due to the increasing life expectancy and life style, the incidence of primary liver cancer is steadily rising. Worldwide, it is the fourth leading reason of cancer-associated death (Yang et al., 2019; Sung et al., 2021). The etiology of liver cancer is highly diverse including besides others viral, toxic, nutritional, etc. risk factors that render treatment options as complex as different pathogenic pathways are involved (Forner et al., 2006; Forner et al., 2018; Llovet et al., 2021). Besides novel pharmacological and cell therapy approaches, surgical interventions are the only potentially curative strategies (Angeli-Pahim et al., 2023). Among these, hepatic resection intends to remove the solid tumor taking into account tumor location and size as well as vascular supply of the parts of the liver to be removed and of the remaining liver. Since the lobar organisation of the liver is mirrored by separate venous blood drainage of the lobes, liver resection in general means removal of the tumor bearing lobe(s). The loss of even more than 60% of the liver mass may be tolerated given that the future liver remnant provides sufficient post-surgery regenerative and metabolic function. To enhance the function of the future liver remnant, the technique of portal vein embolisation is used clinically. This procedure aims at increasing the future liver volume anticipating that volume equals function, i.e., volume growth of the non-ligated lobe(s) compensates for the surgical liver mass loss. However, volume does not necessarily reflect function. Therefore, in order to assess post-surgery hepatic metabolic and regenerative capacities, it is necessary to characterise changes in gene expression in the atrophic and hypertrophic lobes, respectively, and to correlate these changes with the prospective functional efficiency of the liver remnant after resection of the tumor bearing ligated liver lobe(s).
Experimentally, portal vein ligation is comparable with portal vein embolisation applied in clinical settings. For the data collection presented here, we applied a model of 60% portal vein ligation in the rat, i.e., the left median and the lateral left lobes as well as the right superior and inferior lobes were deprived by ligation of the portal vein, while the right median and the left superior and inferior lobes remained unaffected (see Figure 1), accordingly modified as described (Sänger et al., 2015; Wei et al., 2020a).
Figure 1. Overview of the performed PVL surgery of the rat liver and the resulting analyzed samples. Left: During PVL surgery two portal vein branches were ligated (blue lines). Samples were taken from the right median lobe (RML, non-ligated during PVL) and the left median lobe (LML, ligated during PVL) for all animals. Right: PVL and sham surgery were performed for eight animals each. Half of the animals were sacrificed after 2 days (2d), the other half after 5 days (5d). Control animals were not operated at all. For PVL and sham animals, total RNA libraries were made, for PVL and control animals, small RNA libraries, resulting in 32 and 20 samples for total and small RNA, respectively.
Although non-coding RNAs (ncRNAs) such as microRNA (miRNA) or long ncRNA (lncRNA) have been shown to be deeply involved in the pathophysiology of almost all acute and chronic liver diseases, many of the especially liver-specific ncRNAs, have been not even annotated yet (Roy et al., 2018). Our group developed new transcriptome assembly pipelines by combining existing tools to identify the different isoforms of mRNAs and lncRNAs (Hölzer and Marz, 2019; Faber and Hölzer, 2020).
From the pilot project of ENCODE we know that less than 3% of the human genome code for proteins (ENCODE Project Consortium et al., 2007; ENCODE Project Consortium, 2012). The remaining genome is divided into 45% repetitive elements (SINEs, LINEs, transposons), 26% introns and other unique non-coding DNA (Gregory, 2005). The question of their meaning has raised 4 replicates 5d is only being answered slowly. By now, we know that at least 80% of the human genome has a function (ENCODE Project Consortium, 2012). Some ncRNAs are known to be located within introns, in 5′ and 3′ untranslated regions (UTRs), antisense to protein-coding sequences or just close to them. However, the in silico identification of the ncRNAs is still a huge challenge. Due to their diverse and fast evolving sequence they are identified most efficiently by a combined in silico/ex vivo approach, that is sequencing the transcriptome and establishing tissue/organ/organism-specific bioinformatical tools. During the last decade, miRNAs, as regulators of various cell processes, received major attention. However, currently there are 3,016 ncRNA families described [Rfam v.14.1 (Kalvari et al., 2018)], of which 800 are associated to human, covering more than 16.000 genomic regions1. Additionally, the existence of long non-coding RNAs (
Although only a fraction of liver-specific ncRNAs are known, here we summarize important examples of ncRNA participating in the pathogenesis of different forms of liver disease and how they can be used as therapeutic tools or targets for novel treatment paradigms, following the suggestions of Roy et al. (2018), see Table 1. A large number of ncRNA genes being involved in metabolic processes, inflammation and immune response are differentially expressed during aging and other biological processess. We therefore expect to see ncRNAs and especially miRNAs to play a role in senescence and inflammation in rat liver when comparing PVL with healthy liver (Barth et al., 2019).
Table 1. NcRNAs altered in liver diseases (Roy et al., 2018), and citations in there. HSC–hepatic stellate cells.
MicroRNAs (miRNAs) are a class of small, non-protein coding RNAs that play a crucial role in mediating post-transcriptional gene silencing (Carthew and Sontheimer, 2009). These molecules, typically 18 to 25 nucleotides in length, act as regulators of gene expression by binding to the 3′ untranslated region of target genes (Bartel, 2009). Interestingly, the intricate machinery governing miRNAs holds significant importance not only in the context of liver diseases (Szabo and Bala, 2013), but also in processes related to portal vein ligation (Song et al., 2010). In the realm of liver biology, miRNAs exert profound effects on various aspects of liver function and pathology. They participate in regulating processes such as hepatocyte proliferation (Song et al., 2010), differentiation, apoptosis, and lipid metabolism (Agbu and Carthew, 2021). Dysregulation of miRNAs has been implicated in the pathogenesis of liver diseases ranging from viral hepatitis (Shrivastava et al., 2015) to hepatocellular carcinoma (Nagy et al., 2018). Moreover, the role of miRNAs in portal vein ligation, a surgical procedure often used in experimental models to study liver regeneration, underscores their significance beyond disease states (Starlinger et al., 2019). MiRNAs are intricately involved in the molecular pathways underlying the response to portal vein ligation, influencing the regeneration capacity of the liver and impacting overall hepatic function (Chen et al., 2017). Overall, the multifaceted involvement of miRNAs in liver biology and portal vein ligation highlights their versatility and potential as therapeutic targets in the context of liver diseases and surgical interventions.
Experiments were conducted from March 1 until April 13, 2022. Male Sprague-Dawley rats (Charles river, 320–440 g) were housed under a 12 h dark/light cycle at ambient temperature with free access to food and water. The experiments were run with four different groups including four animals each for the PVL and the sham operation at two different time points (2 and 5 days) after surgery (16 animals). An additional weight-matched two animals without any treatment were run along as control in order to identify potential surgery-induced differences as compared with the sham-operated animals. The two time points were chosen following the rational that post-surgery regeneration after PVL might follow similar kinetics as regeneration after partial hepatecomy featuring a maximum regenerative response after 2 days and returning to starting conditions again after 5 days (Gerlach et al., 1997; Andersen et al., 2013). The PVL operation was a modified procedure as described previously (Wei et al., 2020b) by ligating in addition to the left median and left lateral portal vein before the bifurcation, the right superior and inferior portal veins before the bifurcation. In sham-treated animals, the abdominal cavity was opened by a midline incision and closed again thereafter. After the sham or the PVL operation, the animals were left under housing conditions. At 2 and 5 days after surgery, respectively, the animals were euthanized under 2% isoflurane anesthesia and livers explanted. The weight-matched control animals without any treatment were run along. Tissue pieces (ca. 50 mg) from the left (LML, ligated) and the right (RML, non-ligated) median lobes (Figure 1) were immediately collected in 750 µL QIAzol reagent (Quiagen GmbH, Hilden, Germany) in 2 mL reaction tubes (Biozym Scientific GmbH, Hessisch Oldendorf, Germany) containing 5 PreCelly beads (PEQLAB Biotechnologie GmbH, Erlangen, Germany), snap frozen and stored at −80°C until further analysis. In total, 52 samples from 18 animals were passed to RNA extraction and subsequent sequencing.
Frozen samples were thawed and centrifuged twice for 10 s at 5,500 rpm in the PRECELLYS® 24 homogenizer (VWR International GmbH, Darmstadt; Germany), and mixed with 150 µL chloroform for 30 s using a vortexer. After incubation for 10 min on crashed ice, phases were separated by centrifugation at 4°C for 15 min and 11,000 rpm in the Fresco 21 centrifuge (Haereus, Hanau, Germany). The aqueous phase was collected, the RNA precipitated with 500 µL isopropanol overnight at −20°C and subsequently centrifuged for 10 min at 4°C in the benchtop microcentrifuge. The pellet was washed with 500 µL 3 M sodium acetate and the suspension pelleted for 10 min at 4°C and 11,000 rpm. This step was repeated, the final pellet dissolved in 300 µL RNA grade water at 4°C for 2 h. Thereafter, the RNA was precipitated with 600 µL ethanol abs. at −80°C for 1 h and pelleted for 10 min at 4°C and 11,000 rpm. The pellet was washed with 500 µL 80% ethanol and the pellet collected for 10 min at 4°C and 11,000 rpm. After repetition of this step, the final pellet was collected and shortly air dried. The RNA was dissolved in 75–100 µL of RNA grade water overnight in the fridge. The RNA content and quality were determined using the Amersham NanoVue equipment (GE Healthcare, Freiburg, Germany).
Total cellular RNA from the tissues were isolated. cDNA libraries were prepared for all 16 operated animals, utilizing the NEBNext Ultra II Directional RNA library preparation kit including rRNA depletion (rRNA-) according manufactures instructions. The complete experiment was performed in four independent, biological replicates; thus, 32 rRNA-libraries were sequenced on a NovaSeq6000 run yielding 251 bp reads on paired-end mode (further referred to as “total RNA dataset”). For all animals which underwent a PVL surgery, additional cDNA libraries were prepared utilizing the TruSeq Small RNA library preparation kit according manufactures instructions, to sequence especially small RNAs. Additionally, for the two control animals, cDNA libraries were prepared utilizing the TruSeq Small RNA library preparation kit according manufactures instructions. The resulting 20 libraries were sequenced on a NovaSeq6000 run yielding 51 bp reads on paired-end mode (further referred to as “small RNA dataset”).
The sequencing data was quality controlled using fastQC (v0.11.9)3. Adapter sequences and low quality bases were removed by trimmimg with fastp (v0.20.1) (Chen et al., 2018). Parameters for total RNA dataset: --detect_adapter_for_pe --length_required 20 --cut_right --cut_mean_quality 28; parameters for small RNA dataset: --adapter_fasta --cut_right --length_required 15 --cut_mean_quality 28; Parameters for adapter file see Supplementary Data Sheet 1.FASTA, then quality was controlled again using fastQC (v0.11.9).
Mapping and counting of reads were performed differently for the total and the small RNA dataset. For the total RNA dataset, the reads were mapped with HISAT2 (v2.2.1) (Kim et al., 2015) using default parameters to the R. Norvegicus reference genome mRatBN7.2 (release 108, retrieved on 11/09/2022 from Ensembl4). Data was converted into sorted bam files using samtools (v1.12) (Li et al., 2009) and reads per gene were counted using featureCounts (v2.0.1) (Liao et al., 2014) using the reference annotation mRatBN7.2 (release 108, retrieved on 11/09/2022 from Ensembl5) including multimapping reads (featureCounts -M -p -T 20 -t ’exon’). For the small RNA dataset, the reads were mapped with HISAT2 (v2.2.1) (Kim et al., 2015) using default parameters to the R. norvegicus reference genome mRatBN7.2 (release 108, assembly accession GCF_015227675.2, retrieved on 06/01/2023 from NCBI6). Data was converted into sorted bam files using samtools (v1.12) (Li et al., 2009). For counting, a miRNA specific annotation file was generated, based on the NCBI assembly GCF_015227675.2 for mRatBN7.2 (retrieved on 06/01/2023 from NCBI7). The created miRNA annotation file contains a 5′ and 3′ miRNA entry for each miRNA, either taken from the original annotation or generated by splitting the pre-miRNA entry for the miRNA into two-halves (see Supplementary Data Sheet 3.csv). The reads per miRNA were counted using featureCounts (v2.0.1) (Liao et al., 2014) including multimapping reads (featureCounts -M -p -T 20 -t ’exon’).
Sequencing statistics (see Tables 2, 3) were extracted from fastp report files (amount of reads, read length) and from featureCounts summary file. We used the percentage of reads assigned to annotated features and percentage of reads, which could not be (unambiguously) assigned to features, or not mapped to the reference at all. The percentage of risobosomal RNA (rRNA) was calculated using sortMeRNA (v4.3.6) (Kopylova et al., 2012) with the default sortMeRNA reference database v4.3.4 (smr_v4.3_default_db.fasta).
The sequencing of the 32 total RNA samples (see Table 2) resulted in 251 nt paired end reads (R1 and R2), with an average of 24.5 million reads per sample with number of reads per sample ranging from about 18.2 million reads for sample PVL-2d-RML-02 up to about 29.4 million reads for sample sham-2d-RML-03. The read duplication rate ranges from 12.88% in sample 2d-RLM-02 to 14.31% in sample 5d-RML-03, with an average read duplication rate of 13.64%. After trimming low quality bases and removing adapter sequences, the number of reads and the average read length decreased to about 23 million reads per sample and average read length of 184 and 174 nt for R1 and R2 reads, respectively. For all samples, about 60% of the reads could be assigned to annotated features by using featureCounts and including multimapping reads (min. 52%, max. 62%). On average, 36% of the reads could be mapped unambiguously onto the genome into regions with no annotated features, while about 4% of the reads could not be mapped unambiguously onto the rat genome (either reads not mapping to the reference genome at all or reads mapping to multiple features). Up to 58% of the unprocessed reads could be aligned to ribosomal RNA (rRNA), based on the sortMeRNA default database with an average of 45% aligning to rRNA over all total RNA samples.
The sequencing of the 20 small RNA samples (see Table 3) resulted in 51 nt paired end reads (R1 and R2), with an average of 90.81 million reads per sample with number of reads per sample having a wide range from 65.91 million reads for sample PVL-2d-RML-02 up to 119.16 million reads for sample PVL-2d-LML-02. The read duplication rate ranges from 37.79% in sample 2d-LML-02 to 52.94% in sample 0w-LML-02, with an average read duplication rate of 47.25%. After trimming low quality bases and removing adapter sequences, the number of reads and the average read length decreased to about 82 million reads per sample and an expected average read length of 23 nt for both R1 and R2 reads. For all samples about 62% of the reads could be assigned to annotated features by using featureCounts and including multimapping reads (between 58%–68%). On average, 15% of the reads could be mapped unambiguously onto the genome into regions with no annotated features, while about 23% of the reads could not be mapped unambiguously onto the rat genome (either reads not mapping to the reference genome at all or reads mapping to multiple features). Up to 2.69% of the unprocessed reads could be aligned to rRNA based on the sortMeRNA default database with an average of 1.33% aligning to rRNA over all small RNA samples.
The dataset from our study offers a valuable resource for understanding the quantitative relationship between hepatic perfusion and function in the transcriptomes of healthy and venous-ligated livers. It enables comprehensive analyses not only on mRNAs but also on lncRNAs and miRNAs. Given the limited knowledge about lncRNAs and the lack of general computer programs for their identification, including essential secondary structure information and protein interactions, this dataset is particularly significant. It serves as a potential source for marker genes related to regeneration capacity and potential therapeutic targets. More specifically, the reader may systematically investigate the following: 1) Description of the liver-specific ncRNA landscape; 2) Comparison of ncRNAs and isoforms in different conditions of the liver: healthy and after portal vein ligation; 3) Comparison of ncRNAs and isoforms at different time points after ligation of the liver lobe.
The datasets presented in this study can be found in online repositories. The names of the repository and accession number can be found below: https://www.ebi.ac.uk/ena, PRJEB74462. The assignment of samples to accession IDs is listed in Supplementary Data Sheet 2.csv.
The animal study was approved by and authorized by the federal state of Saxony (file no. TVV19-21) and was in accordance with the guidelines of the animal welfare act. The study was conducted in accordance with the local legislation and institutional requirements.
DM: Data curation, Visualization, Writing–original draft, Writing–review and editing. JK: Visualization, Writing–original draft, Writing–review and editing. MvB: Visualization, Writing–original draft, Writing–review and editing. BC: Visualization, Writing–original draft, Writing–review and editing. MM: Visualization, Writing–original draft, Writing–review and editing.
The author(s) declare that financial support was received for the research, authorship, and/or publication of this article. Funded by the DFG within the Research Unit Programme FOR 5151 QuaLiPerF (Quantifying Liver Perfusion–Function Relationship in Complex Resection—A Systems Medicine Approach) by grant 436883643 with project identifiers MA5082/15-1 and CH109/26-1. We acknowledge support by the German Research Foundation Projekt-Nr. 512648189 and the Open Access Publication Fund of the Thueringer Universitaets—und Landesbibliothek Jena.
We thank the Core Facility next-generation sequencing of the Leibniz Institute on Aging–Fritz Lipmann Institute in Jena for their help with Illumina sequencing.
Author DM was employed by Oncgnostics GmbH.
The remaining authors declare that the research was conducted in the absence of any commercial or financial relationships that could be construed as a potential conflict of interest.
All claims expressed in this article are solely those of the authors and do not necessarily represent those of their affiliated organizations, or those of the publisher, the editors and the reviewers. Any product that may be evaluated in this article, or claim that may be made by its manufacturer, is not guaranteed or endorsed by the publisher.
The Supplementary Material for this article can be found online at: https://www.frontiersin.org/articles/10.3389/fgene.2024.1421955/full#supplementary-material
2https://www.gencodegenes.org/human/
3https://www.bioinformatics.babraham.ac.uk/projects/fastqc
4https://ftp.ensembl.org/pub/release-108/fasta/rattus_norvegicus/dna/Rattus_norvegicus.mRatBN7.2.dna.toplevel.fa.gz
5https://ftp.ensembl.org/pub/release-108/gtf/rattus_norvegicus/Rattus_norvegicus.mRatBN7.2.108.chr.gtf.gz
6https://ftp.ncbi.nlm.nih.gov/refseq/R_norvegicus/annotation_releases/current/108/GCF_015227675.2_mRatBN7.2/GCF_015227675.2_mRatBN7.2_genomic.fna.gz
7https://ftp.ncbi.nlm.nih.gov/refseq/R_norvegicus/annotation_releases/current/108/GCF_015227675.2_mRatBN7.2/GCF_015227675.2_mRatBN7.2_genomic.gtf.gz
Agbu, P., and Carthew, R. W. (2021). Microrna-mediated regulation of glucose and lipid metabolism. Nat. Rev. Mol. Cell Biol. 22, 425–438. doi:10.1038/s41580-021-00354-w
Andersen, K. J., Knudsen, A. R., Kannerup, A.-S., Sasanuma, H., Nyengaard, J. R., Hamilton-Dutoit, S., et al. (2013). The natural history of liver regeneration in rats: description of an animal model for liver regeneration studies. Int. J. Surg. 11, 903–908. doi:10.1016/j.ijsu.2013.07.009
Angeli-Pahim, I., Chambers, A., Duarte, S., and Zarrinpar, A. (2023). Current trends in surgical management of hepatocellular carcinoma. Cancers (Basel) 15, 5378. doi:10.3390/cancers15225378
Bartel, D. P. (2009). Micrornas: target recognition and regulatory functions. Cell 136, 215–233. doi:10.1016/j.cell.2009.01.002
Barth, E., Srivastava, A., Stojiljkovic, M., Frahm, C., Axer, H., Witte, O. W., et al. (2019). Conserved aging-related signatures of senescence and inflammation in different tissues and species. Aging (Albany NY) 11, 8556–8572. doi:10.18632/aging.102345
Carthew, R. W., and Sontheimer, E. J. (2009). Origins and mechanisms of mirnas and sirnas. Cell 136, 642–655. doi:10.1016/j.cell.2009.01.035
Chen, S., Zhou, Y., Chen, Y., and Gu, J. (2018). fastp: an ultra-fast all-in-one fastq preprocessor. Bioinformatics 34, i884–i890. doi:10.1093/bioinformatics/bty560
Chen, X.-B., Zheng, X.-B., Cai, Z.-X., Lin, X.-J., and Xu, M.-Q. (2017). Microrna-203 promotes liver regeneration after partial hepatectomy in cirrhotic rats. J. Surg. Res. 211, 53–63. doi:10.1016/j.jss.2016.11.043
ENCODE Project Consortium, Birney, E., Stamatoyannopoulos, J. A., Dutta, A., Guigó, R., Gingeras, T. R., et al. (2007). Identification and analysis of functional elements in 1 % of the human genome by the ENCODE pilot project. Nature 447, 799–816. doi:10.1038/nature05874
ENCODE Project Consortium (2012). An integrated encyclopedia of DNA elements in the human genome. Nature 489, 57–74. doi:10.1038/nature11247
Faber, L., and Hölzer, M. (2020). Combining k-mer and quasi-read-mapping methods to improve the clustering of multiple de novo transcriptome assemblies. in preparation.
Forner, A., Hessheimer, A. J., Isabel Real, M., and Bruix, J. (2006). Treatment of hepatocellular carcinoma. Crit. Rev. Oncol. Hematol. 60, 89–98. doi:10.1016/j.critrevonc.2006.06.001
Forner, A., Reig, M., and Bruix, J. (2018). Hepatocellular carcinoma. Lancet 391, 1301–1314. doi:10.1016/S0140-6736(18)30010-2
Gerlach, C., Sakkab, D. Y., Scholzen, T., Daßler, R., Alison, M. R., and Gerdes, J. (1997). Ki-67 expression during rat liver regeneration after partial hepatectomy. Hepatology 26, 573–578. doi:10.1002/hep.510260307
Gregory, T. R. (2005). Synergy between sequence and size in large-scale genomics. Nat. Rev. Genet. 6, 699–708. doi:10.1038/nrg1674
Hölzer, M., and Marz, M. (2019). De novo transcriptome assembly: a comprehensive cross-species comparison of short-read RNA-Seq assemblers. GigaScience 8, giz039. doi:10.1093/gigascience/giz039
Kalvari, I., Argasinska, J., Quinones-Olvera, N., Nawrocki, E. P., Rivas, E., Eddy, S. R., et al. (2018). Rfam 13.0: shifting to a genome-centric resource for non-coding RNA families. Nucleic Acids Res. 46, D335–D342. doi:10.1093/nar/gkx1038
Kim, D., Langmead, B., and Salzberg, S. L. (2015). HISAT: a fast spliced aligner with low memory requirements. Nat. methods 12, 357–360. doi:10.1038/nmeth.3317
Kopylova, E., Noé, L., and Touzet, H. (2012). Sortmerna: fast and accurate filtering of ribosomal rnas in metatranscriptomic data. Bioinformatics 28, 3211–3217. doi:10.1093/bioinformatics/bts611
Li, H., Handsaker, B., Wysoker, A., Fennell, T., Ruan, J., Homer, N., et al. (2009). The sequence alignment/map format and samtools. bioinformatics 25, 2078–2079. doi:10.1093/bioinformatics/btp352
Liao, Y., Smyth, G. K., and Shi, W. (2014). featurecounts: an efficient general purpose program for assigning sequence reads to genomic features. Bioinformatics 30, 923–930. doi:10.1093/bioinformatics/btt656
Llovet, J. M., Kelley, R. K., Villanueva, A., Singal, A. G., Pikarsky, E., Roayaie, S., et al. (2021). Hepatocellular carcinoma. Nat. Rev. Dis. Prim. 7 (6), 6. doi:10.1038/s41572-020-00240-3
Nagy, Á., Lánczky, A., Menyhárt, O., and Győrffy, B. (2018). Validation of mirna prognostic power in hepatocellular carcinoma using expression data of independent datasets. Sci. Rep. 8, 9227. doi:10.1038/s41598-018-27521-y
Roy, S., Trautwein, C., Luedde, T., and Roderburg, C. (2018). A general overview on non-coding RNA-based diagnostic and therapeutic approaches for liver diseases. Front. Pharmacol. 9, 805. doi:10.3389/fphar.2018.00805
Sänger, C., Schenk, A., Schwen, L. O., Wang, L., Gremse, F., Zafarnia, S., et al. (2015). Intrahepatic vascular anatomy in rats and mice–variations and surgical implications. PLoS One 10, e0141798. doi:10.1371/journal.pone.0141798
Shrivastava, S., Steele, R., Ray, R., and Ray, R. B. (2015). Micrornas: role in hepatitis c virus pathogenesis. Genes and Dis. 2, 35–45. doi:10.1016/j.gendis.2015.01.001
Song, G., Sharma, A. D., Roll, G. R., Ng, R., Lee, A. Y., Blelloch, R. H., et al. (2010). Micrornas control hepatocyte proliferation during liver regeneration. Hepatology 51, 1735–1743. doi:10.1002/hep.23547
Starlinger, P., Hackl, H., Pereyra, D., Skalicky, S., Geiger, E., Finsterbusch, M., et al. (2019). Predicting postoperative liver dysfunction based on blood-derived microrna signatures. Hepatology 69, 2636–2651. doi:10.1002/hep.30572
Sung, H., Ferlay, J., Siegel, R. L., Laversanne, M., Soerjomataram, I., Jemal, A., et al. (2021). Global cancer statistics 2020: globocan estimates of incidence and mortality worldwide for 36 cancers in 185 countries. CA a cancer J. Clin. 71, 209–249. doi:10.3322/caac.21660
Szabo, G., and Bala, S. (2013). Micrornas in liver disease. Nat. Rev. Gastroenterology hepatology 10, 542–552. doi:10.1038/nrgastro.2013.87
Wei, W., Hua, C., Zhang, T., Dirsch, O., Gremse, F., Homeyer, A., et al. (2020a). Size of portally deprived liver lobe after portal vein ligation and additional partial hepatectomy: result of balancing proliferation and apoptosis. Sci. Rep. 10, 4893. doi:10.1038/s41598-020-60310-0
Wei, W., Hua, C., Zhang, T., Dirsch, O., Gremse, F., Homeyer, A., et al. (2020b). Size of portally deprived liver lobe after portal vein ligation and additional partial hepatectomy: result of balancing proliferation and apoptosis. Sci. Rep. 10, 4893. doi:10.1038/s41598-020-60310-0
Keywords: portal vein ligation, PVL, rat liver, liver, RNA-seq, gene expression, transcriptomics
Citation: Meyer D, Kosacka J, von Bergen M, Christ B and Marz M (2024) Data report on gene expression after hepatic portal vein ligation (PVL) in rats. Front. Genet. 15:1421955. doi: 10.3389/fgene.2024.1421955
Received: 14 May 2024; Accepted: 09 July 2024;
Published: 21 August 2024.
Edited by:
Sanjeev Kumar Srivastava, Mitchell Cancer Institute, United StatesReviewed by:
Saima Subhani, Simulations Plus, United StatesCopyright © 2024 Meyer, Kosacka, von Bergen, Christ and Marz. This is an open-access article distributed under the terms of the Creative Commons Attribution License (CC BY). The use, distribution or reproduction in other forums is permitted, provided the original author(s) and the copyright owner(s) are credited and that the original publication in this journal is cited, in accordance with accepted academic practice. No use, distribution or reproduction is permitted which does not comply with these terms.
*Correspondence: Daria Meyer, ZGFyaWEubWV5ZXJAdW5pLWplbmEuZGU=; Manja Marz, bWFuamFAdW5pLWplbmEuZGU=
Disclaimer: All claims expressed in this article are solely those of the authors and do not necessarily represent those of their affiliated organizations, or those of the publisher, the editors and the reviewers. Any product that may be evaluated in this article or claim that may be made by its manufacturer is not guaranteed or endorsed by the publisher.
Research integrity at Frontiers
Learn more about the work of our research integrity team to safeguard the quality of each article we publish.