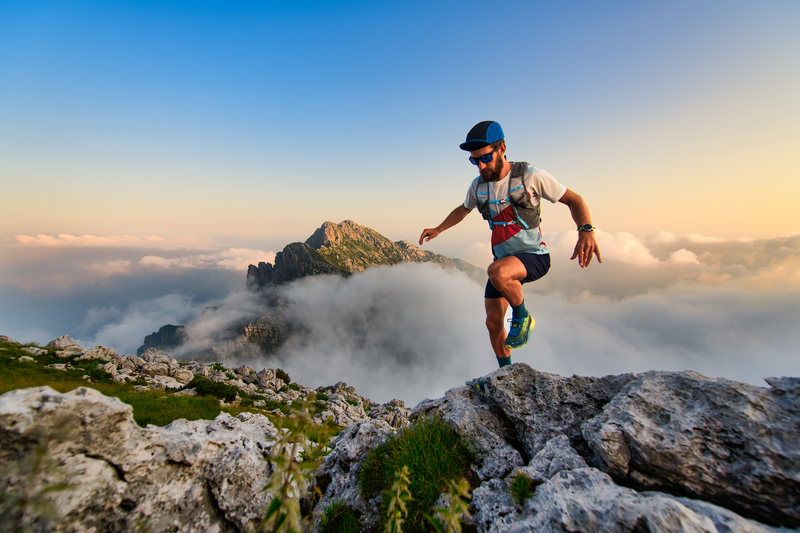
94% of researchers rate our articles as excellent or good
Learn more about the work of our research integrity team to safeguard the quality of each article we publish.
Find out more
ORIGINAL RESEARCH article
Front. Genet. , 31 July 2024
Sec. Applied Genetic Epidemiology
Volume 15 - 2024 | https://doi.org/10.3389/fgene.2024.1411931
Introduction: Vitamin C is an essential nutrient. Sex differences in serum vitamin C concentrations have been observed but are not fully known. Investigation of levels of metabolites may help shed light on how dietary and other environmental exposures interact with molecular processes. O-methylascorbate and ascorbic acid 2-sulfate are two metabolites in the vitamin C metabolic pathway. Past research has found genetic factors that influence the levels of these two metabolites. Therefore, we investigated possible effect modification by sex of genetic variant-metabolite associations and characterized the biological function of these interactions.
Methods: We included individuals of European descent from the Canadian Longitudinal Study on Aging with available genetic and metabolic data (n = 9004). We used linear mixed models to tests for genome-wide associations with O-methylascorbate and ascorbic acid 2-sulfate, with and without a sex interaction. We also investigated the biological function of the important genetic variant-sex interactions found for each metabolite.
Results: Two genome-wide statistically significant (p value < 5 × 10−8) interaction effects and several suggestive (p value < 10–5) interaction effects were found. These suggestive interaction effects were mapped to several genes including HSD11B2, associated with sex hormones, and AGRP, associated with hunger drive. The genes mapped to O-methylascorbate were differently expressed in the testis tissues, and the genes mapped to ascorbic acid 2-sulfate were differently expressed in stomach tissues.
Discussion: By understanding the genetic factors that impact metabolites associated with vitamin C, we can better understand its function in disease risk and the mechanisms behind sex differences in vitamin C concentrations.
Vitamin C consumption and its effects on aging have been extensively studied (Balboa-Castillo et al., 2018; Carr and Zawari, 2023). There has been evidence to suggest that older adults do not consume a high enough concentration, and this may lead to increased risk of frailty and other conditions due to the immune effects of the vitamin (Carr and Maggini, 2017; Carr and Zawari, 2023). Past research has also found differences between serum vitamin C concentrations in males and females, with males more likely to have lower concentrations (Schleicher et al., 2009; Carr and Lykkesfeldt, 2023). Several theories have been proposed to account for this difference, including external factors such as body size and lifestyle factors and internal or molecular factors such as sex hormones (Travica et al., 2020). Some studies which have noted the influence of sex hormones on vitamin C concentrations have relied on cellular and animal models (Nathani and Nath, 1972; Kume-Kick and Rice, 1998; Travica et al., 2020). One recent cross-sectional study in infertile men found there was an inverse association between serum ascorbic acid and luteinizing hormone levels, however, the relationship between vitamin C and sex hormones in humans needs to be further explored (Rastegar Panah et al., 2023).
The exposome is the totality of all environmental exposures in someone’s lifetime and how they influence disease (Wild, 2005). One way to quantify a portion of the exposome is through the levels of metabolites. A metabolite refers to a substance that is produced or consumed during metabolism. Metabolites are intermediates in a metabolic pathway and can be substrates or products. Quantifying metabolites can bring insight into mechanisms by which the environment affects biological/metabolic processes (Walker et al., 2019). For example, a study by Hysi et al. (2019) used this strategy to look at the metabolic associations of intraocular pressure (IOP) which is an endophenotype for glaucoma (Hysi et al., 2019).
Genetic factors can influence the levels of metabolites and there have been recently conducted large-scale genome-wide association studies (GWAS) to identify genetic associations (Lotta et al., 2021; Hysi et al., 2022; Yin et al., 2022; Chen et al., 2023). Chen et al. (2023) recently conducted a GWAS to identify genetic factors associated with all metabolites measured in participants of the Canadian Longitudinal Study on Aging (CLSA) (Raina et al., 2019; Michelotti et al., 2023). Among the metabolites investigated were O-methylascorbate and ascorbic acid 2-sulfate, both of which are involved in vitamin C metabolism (Blaschke and Hertting, 1971; Tolbert et al., 1975). A few single nucleotide polymorphisms (SNPs) were found to be significantly associated with these two metabolites (Chen et al., 2023). Yin et al. (2022), conducted a similar analysis which included both metabolites in a Finnish population and found significant associations (Yin et al., 2022). However, it is not known whether these genetic associations are affected by sex, which may explain some of the sex differences in vitamin C concentrations seen between males and females.
In this paper we aimed to investigate how sex affects the genetic association of variants across the genome with O-methylascorbate and ascorbic acid 2-sulfate in the CLSA data. In addition, using the comprehensive functional annotation platform FUMA (Watanabe et al., 2017), we aimed to uncover the functional consequences of these genetic associations. This would help to investigate potential molecular mechanisms for sex-differences in vitamin C concentrations such as through an association with sex hormones. Understanding how genetic factors affect vitamin C-related metabolites can aid in understanding the influence of vitamin C on metabolic processes, and in turn how vitamin C influences disease phenotypes.
We used baseline data from the Comprehensive Cohort of the Canadian Longitudinal Study on Aging (CLSA) (Raina et al., 2019). The CLSA recruited participants between 2010 and 2015 who were between the ages of 45–85 years to investigate social, environmental, and other factors that affect aging and disease. The Comprehensive Cohort included 30,097 participants with baseline data collected between 2012 and 2015 via in-home interviews and in-person physical examinations and biospecimen sample collections at CLSA data collection sites located in Victoria, Vancouver, Surrey, Calgary, Winnipeg, Hamilton, Ottawa, Montreal, Sherbrooke, Halifax, and St. John’s, Canada. Inclusion criteria required participants to be community dwelling, be cognitively unimpaired, and to speak English or French. Not included were full-time members of the Canadian Armed Forces, those residing on a federal First Nations reserve or settlement, those living in a long-term care institution, and non-residents or citizens of Canada.
Of the Comprehensive Cohort, 9,992 participants had metabolite levels quantified and 26,662 individuals were genotyped (Forgetta et al., 2022; Michelotti et al., 2023). In this study we focused on ∼9,000 CLSA participants of European ancestry with genetic and metabolic information and without any missing covariate information. Written informed consent was obtained for all participants, and research ethics board approval was obtained for all CLSA affiliated sites. The analysis presented here was approved by the University of Ottawa research ethics board.
Blood samples were collected from consenting participants of the CLSA Comprehensive Cohort, and samples were moved to −80°C storage before shipment to the genomics facility where they were stored at −20°C. The Affymetrix Axiom array was used to perform genome-wide genotyping, resulting in 794,409 variants from 26,622 participants (Forgetta et al., 2022). We followed the genetic ancestry procedures performed by the CLSA to identify participants of European descent (Forgetta et al., 2022). The CLSA genomic data release included genotype data imputed using the TOPMed reference panel, resulting in ∼308 million variants imputed (Taliun et al., 2021). Prior to GWAS, we filtered out variants from the imputed data that had a minor allele frequency (MAF) <0.01, an imputation quality score <0.3 and missingness >0.1. After these filters, 8,836,359 variants remained for analysis. Both single nucleotide polymorphisms (SNPs) and insertions/deletions (INDELs) were included. All genomic positions are according to the human reference genome assembly GRCh38/hg38.
Metabolite levels were quantified using mass spectrometry and then identified using the Metabolon Discovery HD4TM LC-MS platform (Michelotti et al., 2023). Metabolite values underwent quality control measures and 1,314 metabolites were included in the final dataset. We were interested in two metabolites: O-methylascorbate and ascorbic acid 2-sulfate. In this GWAS we used the CLSA data with batch normalized values and where missing values were imputed with the lowest value recorded. Other researchers have reported using this imputation approach for missing values, and we assumed missing values were due to the limit of detection of the Metabolon platform (Playdon et al., 2019). For O-methyl ascorbate, there were no missing values, and for ascorbic acid 2-sulfate, there were 113 missing values. Prior to analysis, metabolites levels were log-transformed and extreme outliers (more than 3 SD away) removed followed by normalization to a mean of 0 and an SD of one which was done in prior studies (Chen et al., 2023).
We first performed a GWAS on the complete dataset using mixed linear models as implemented in the GCTA/fastGWA program (Jiang et al., 2019). Briefly, the model fit by GCTA/fastGWA is:
where y is the vector of metabolite levels,
After removing individuals with missing covariate values and outlier metabolites, there were 8,916 participants for the O-methylascorbate GWAS and 8,835 participants for the ascorbic acid 2-sulfate GWAS. Manhattan plots and qqplots were made using the qqman (Turner, 2018) package in R to visualize for any statistically significant variants. We only followed up genome-wide significant genetic variants (p-value <5 × 10−8). In our Supplementary Tables SA1, we also provide our results for variants meeting the suggestive level of significance (p-value <1 × 10−5) (Duggal et al., 2008) for ease of replication in future studies.
Independent (i.e., in linkage equilibrium) genetic variants were obtained using the GCTA/COJO program (Yang et al., 2012) which implements a stepwise selection procedure to identify variants within significantly associated genomic regions that remain independently associated with the trait after conditioning on most statistically significant variants. The program also incorporates linkage disequilibrium (LD) structure information from an input population, which was set as the same individuals as those used in the GWAS analysis. Significant variant threshold was based on the genome-wide significance level of 5 × 10−8.
We investigated functional significance using the platform FUMA (Watanabe et al., 2017) for lead variants identified by COJO analysis. To identify associated genes, positional mapping, quantitative expression quantitative trait loci (eQTL) mapping, and chromatin interaction mapping were used. FUMA identifies all variants in LD (based on 1000 Genomes LD structure) with lead variants to use for mapping. Variants were filtered prior to mapping to only those with a Combined Annotation Dependent Depletion (CADD) (Rentzsch et al., 2019) score above a threshold established as associated with variants with deleterious effects (Amendola et al., 2015; Watanabe et al., 2017) that based on research classifying the pathogenicity of genetic variants was set at the suggested level of >12.37 (Amendola et al., 2015). For positional mapping, we additionally only used exonic or splicing variants. For eQTL mapping, a false discovery rate (FDR) threshold of <0.05 was adopted. For chromatin interaction mapping the threshold was an FDR of <1 × 10−06, in line with the default FUMA parameters (Watanabe et al., 2017). All tissues were used for mapping.
We performed a GWAS to test whether genetic effects were modified by chromosomal sex. To achieve this, we used the GCTA program fastGWA-GE (Zhong et al., 2023), which fits the following model:
where
We adjusted for the covariates age, batch number, province, hours since last meal and the first 10 principal components. We used the same sparse GRM as for the main GWAS above and filtered for MAF 0.01 using the GCTA program, leading to 8,580,042 variants.
We followed up all suggestive interaction signals based on a p-value threshold commonly used for suggestive significance in GWASs (p-value of interaction test <1 × 10−05) (Duggal et al., 2008). We used the FUMA application to select lead variants and define loci of interest. The same parameters were used as before, such as filtering by CADD score; however, lead variants were not provided to FUMA externally. In this case, the application selects lead variants and independent significant variants based on LD (r2) information (Watanabe et al., 2017). In brief, all variants with p-values below the suggestive threshold and independent from each other (r2 < 0.6) were identified. Variants that were in r2 ≥ 0.6 with these variants and had a p-value less than 0.05 were equally considered for gene mapping. Lead variants were chosen from the identified suggestive variants if they had r2 < 0.1 with other shortlisted variants.
In a small number of cases (8), variants that were suggestive were not recognized in the FUMA application, and required an alternative investigation. These variant regions were visualized using LD Link (Machiela and Chanock, 2015) and lead variants and any additional annotated variants were selected based on similar criteria to FUMA. The CADD score of resulting variants was determined to filter variants that could be used for mapping. None of the identified variants were above the CADD threshold for deleteriousness and therefore were not mapped to genes. To annotate the functions of the lead variants and variants that met FUMA criteria, but which were not recognized in the platform, the Variant Effect Predictor (VEP) platform from Ensembl was used (McLaren et al., 2016).
To follow-up on interaction results, we estimated the effect sizes for each lead variant in linear mixed models after stratifying the sample by sex. The models were the same as for the overall GWAS described above and were adjusted for the same covariates. For O-methylascorbate, the number of participants was 4,580 and 4,329 for males and females, respectively. For ascorbic acid 2-sulfate, the number of participants was 4,310 and 4,518 for males and females, respectively.
To illustrate the direction of effect for each lead identified variant stratified by sex, we plotted interaction graphs using the results from the GWAS stratified by sex. We plotted the effect size of each lead variant by sex with a 95% confidence interval using R/ggplot.
To report the results of this study, we were informed by Strengthening the Reporting of Genetic Associations (STREGA) guidelines (Little et al., 2009).
Using the GCTA-fastGWA program we found 592 statistically significantly associated variants (p-value <5 × 10−8) with O-methylascorbate. For ascorbic acid 2-sulfate, we found 56 statistically significantly associated variants (p-value < 5 × 10−8). The Manhattan plot of these results is displayed in Supplementary Figure S1 and Supplementary Figure S2. The full list of suggestive variants and p-values for O-methylascorbate and ascorbic acid 2-sulfate are found in Supplementary Table S1 and Supplementary Table S2 respectively.
Using the GCTA-COJO software to follow-up significantly associated variants (p-value < 5 × 10−8), we identified three independent lead variants for O-methylascorbate, all of which were on chromosome 22. For ascorbic acid 2-sulfate, we identified two lead variants on chromosome 16 and one lead variant at chromosome 10. The variant positions, effect allele frequencies, GWAS p-value and COJO-adjusted p-value are summarized in Table 1.
Table 1. Lead variants from overall GWAS analysis selected using GCTA-COJO. Details of chromosome, position, effect allele frequency, p-value from GWAS, and COJO-adjusted p-value. Variants were selected using the GCTA-COJO program.
Using the FUMA platform, we determined the functional consequences of the lead variants found in the COJO analysis and variants in LD with those variants which met FUMA criteria. Variants associated with O-methylascorbate were mapped to 13 genes, which included both known and novel gene associations (Supplementary Table S5). We subsequently used the FUMA platform to identify the functional consequences of the mapped genes, such as their expression levels in different tissue types and whether the set of genes was enriched in any functional pathways. The mapped genes were not significantly differentially expressed in any tissue types (Supplementary Table S6). The functional gene sets linked to some processes such as genes which are involved in a cancer cell-death evasion mechanism (Jinesh and Kamat, 2017). No gene sets could be directly related to vitamin C functions (Supplementary Table S7).
Variants associated with ascorbic acid 2-sulfate were mapped to 71 genes (Supplementary Table S8). Using the same process as before, we found that the mapped genes were not significantly differentially expressed in any tissue types (Supplementary Table S9). The mapped genes were linked to gene sets for chromosomal and proximal deletions syndromes; however, they did not link to any vitamin C functions (Supplementary Table S10). Several novel genes were mapped to ascorbic acid 2-sulfate as well.
We conducted a genetic variant-by-sex GWAS and found that, for O-methylascorbate, there were no statistically significant interactions while there were 69 suggestive interaction variants. For ascorbic acid 2-sulfate, we found two statistically significant interaction variants and 83 suggestive interaction variants. The significant variants were rs1296721356 (chr1:13354706) and rs1301173408 (chr1:13341668). Manhattan plots visualizing the associations are shown in Figure 1. The full list of suggestive interaction variants and p-values for O-methylascorbate and ascorbic acid 2-sulfate are found in Supplementary Table S3 and Supplementary Table S4 respectively.
Figure 1. O-methylascorbate and ascorbic acid 2-sulfate interaction with sex results. Results from the GWAS analysis using the FastGWA-GE program from GCTA in the form of a Manhattan plot. GWAS conducted using a mixed linear model adjusted for age, batch number, province, 10 principal components, and hours since last meal or drink which incorporated a genetic relatedness matrix to account for population stratification. The models also included an interaction term for sex. Each point represents the p-value of the interaction between sex and the variant on the associated chromosome. The red line is the significant (5 × 10−8) threshold and the blue line is the suggestive (1 × 10−5) threshold.
We investigated all the signals at statistically significant and suggestive levels. Using the default parameters of FUMA, we identified 25 lead variants for O-methylascorbate and 23 lead variants for ascorbic acid 2-sulfate (Supplementary Tables S11, S12). After conducting separate sex stratified GWAS analyses for comparison, we saw that all interactions were qualitative in nature, meaning that the variants had opposite effects in males and females in the sex-stratified analyses (Figure 2).
Figure 2. O-methylascorbate (A) and ascorbic acid 2-sulfate (B) effect sizes of lead interacting variant by sex. Effect sizes and 95% confidence intervals from the GWAS stratified by sex. GWAS conducted using a mixed linear model adjusted for age, batch number, province, 10 principal components, and hours since last meal or drink which incorporated a genetic relatedness matrix to account for population stratification. The list of variants are the lead variants for each suggestive locus found in the interaction GWAS analysis. Red represents female values and blue represents male values.
In Tables 2, 3, we summarized the lead variants for each suggestive interaction loci, nearest genes, annotated functions, and number of genes mapped at each suggestive genomic loci for O-methylascorbate and ascorbic acid 2-sulfate respectively. Overall, most of the genomic loci included variants in intronic or intergenic regions, which were not mapped to any genes. However, some regions had variants of likely functional importance which were mapped to more than one gene.
Table 2. O-methylascorbate suggestive interaction loci functions. Lead variants for each suggestive interaction locus were selected based on FUMA criteria. For each locus, variants in LD of the lead variant were analyzed to note down the nearest gene and function. The number of genes mapped to each locus is based on FUMA criteria. Loci that did not register in FUMA, and whose functions and nearest gene information were retrieved using VEP are marked.
Table 3. Ascorbic acid 2-sulfate suggestive interaction loci functions. Lead variants for each suggestive interaction locus were selected based on FUMA criteria. For each locus, variants in LD of the lead variant here analyzed to note down the nearest gene and function. The number of genes mapped to each locus is based on FUMA criteria. Loci that did not register in FUMA, and whose functions and nearest gene information were retrieved using VEP are marked.
Variants rs1470819913 and rs1296721356 were not available in FUMA and were annotated separately. No genes were mapped to these regions, and the summary of their functions is included in Tables 2, 3.
The variants that interacting with sex were significantly associated with O-methylascorbate levels, were mapped to 23 different genes (Supplementary Table S13). Looking at tissue expression data, the set of genes is statistically significantly differentially expressed (corrected p-value <0.05) in testis (Supplementary Table S14).
The variants that interacted with sex for ascorbic acid 2-sulfate were mapped to 59 genes (Supplementary Table S15). Looking at tissue expression data, the set of genes is statistically significantly differentially expressed (correct p-value <0.05) in the stomach (Supplementary Table S16). One of the mapped genes was AGRP which is a neuropeptide which controls feeding behavior as a stimulating hormone antagonist (Deem et al., 2022). Another gene mapped was HSD11B2, which is an enzyme involved in cortisol metabolism, and which has been shown to be controlled by sex hormones (Garbrecht et al., 2007). The significant genomic loci identified on chromosome one was not mapped to any genes and we did not find evidence of a potential functional consequence of this region based on our criteria. For both O-methylascorbate and ascorbic acid 2-sulfate, no gene-sets related to vitamin C metabolism or sex hormones were identified (Supplementary TableS S17, S18).
In the present study, we investigated associations and the functional consequences of genetic variant-metabolite associations for two specific metabolites related to vitamin C: O-methylascorbate and ascorbic acid 2-sulfate. In addition, our study is the first to investigate potential genetic variant-sex interactions influencing these two metabolites by conducting a gene by sex GWAS. We also examined the functionality of these variants of interest and found some associations with hormone-related genes.
Past research has studied the genomic associations of these metabolite levels, and Chen et al., 2023, conducted their study using the CLSA metabolite and genomic data. We aimed to have a more focused analysis with a less stringent threshold to gain a deeper understanding of the two vitamin C-related metabolites specifically. We report the same associations, with rs144009214 for ascorbic acid 2-sulfate and rs61484427 and rs4680 for O-methylascorbate (Chen et al., 2023). We also examined additional lead variants than was previously reported due to our less stringent significance threshold of 5 × 10−8 (not corrected for examining all measured metabolites). Chen et al. also identified COMT as the closest protein coding genes to the O-methylascorbate variants, which we also identified using FUMA. Using this platform, we were able to find several novel genes that were mapped to our GWAS results and should be investigated more thoroughly. Yin et al., 2022 also identified variant rs4680 as significant with O-methylascorbate in their analysis (Yin et al., 2022).
Chen et al., identified CD2BP2 as the closest protein coding genes to the ascorbic acid-2 sulfate associated variant, which we also identified in FUMA, among several other genes (Chen et al., 2023; Schlosser et al., 2023 conducted a GWAS on plasma and urine metabolite levels with individuals with chronic kidney disease. In their analysis, ascorbic acid-2 sulfate was associated with rs111894927 which is in the genetic region of the MAPK3 gene, which we also identified in FUMA (Schlosser et al., 2023). Yin et al. found some genetic associations with ascorbic acid-2 sulfate, however none of their identified putative causal genes were found in our analysis (Yin et al., 2022).
The FUMA analysis from the initial GWAS identified several new genes that were associated with metabolite levels. However, this comprehensive evaluation did not provide any new evidence of mechanisms or pathways through which these gene associations influence metabolite levels. In addition, none of the novel genes associated could be connected to vitamin C metabolism.
Of the mapped genes, COMT is a known factor in vitamin C metabolism. O-methylascorbate is a product of O-methylation by the COMT gene (Krumsiek et al., 2012). Therefore, this work strengthens an existing connection and further emphasizes the potential importance of this gene in vitamin C metabolism.
Our analysis of a potential sex effect on the gene associations seen for each metabolite found two significant interactions and several suggestive interactions. The effect of sex is an important factor to consider in disease etiology, and in this case, the sex differences of vitamin C concentrations between males and females and not completely understood. One of the proposed theories for the sex differences seen between vitamin C concentrations in males and females is due to influence by sex hormones (Travica et al., 2020). We explored all suggestive interactions to determine their functional significance as well as if there are any connections to genes related to hormone signaling or sex hormones.
For both ascorbic acid 2-sulfate and O-methylascorbate, there were no gene-sets which are enriched and may be related to vitamin C metabolism or sex hormones. Interestingly, the set of genes mapped for ascorbic acid 2-sulfate was significantly differentially expressed in stomach tissues while the set of genes mapped for O-methylascorbate was differentially expressed in testis tissues.
The variants that interacted with sex for ascorbic acid 2-sulfate were mapped to several genes, one of which was AGRP, which produces a peptide agonist molecule important for initiating hunger cues. In general, the AGRP neurons signal for increased food intake (Deem et al., 2022). In one animal study using a bird model, researchers found that this protein was differentially expressed between male and female chickens (Caughey et al., 2018). Since vitamin C intake is heavily influenced by dietary exposures, this association with a hunger-driving signal protein may be something of further consideration.
Other variants that interacted with sex for ascorbic acid 2-sulfate were mapped to HSD11B2. The role of 11-β hydroxysteroid dehydrogenase type 2, the enzyme encoded by the HSD11B2 gene, is to oxidize cortisol, a glucocorticoid, into its inactive version cortisone (Chapman et al., 2013). Several animal and in vivo studies have shown that the activity of this enzyme is regulated by various sex hormones (Darnel et al., 1999; Garbrecht et al., 2007; Wang et al., 2009). In addition, cortisol may potentially affect vitamin C concentrations in the body; however, it is unclear whether this acts in a sex dependent manner (Travica et al., 2020). Overall, this may point to an important mechanism for future research.
This study has several strengths, including the use of high quality genetic and metabolic datasets. In addition, the use of such a comprehensive tool to annotate variant functions allowed us to use several bioinformatics tools to identify novel associations and areas for future research.
This study also had some limitations to consider. One limitation is the sample size, which may have hindered the ability to find more statistically significant associations, especially in the interaction analysis. However, these findings showed several associations at the suggestive level, which could be followed up by other researchers. Future studies should replicate these findings ideally with a larger sample size. Another limitation is that this study was only conducted using participants of European descent to avoid problems with population stratification. The CLSA had a very high percentage of participants of European descent so using populations from other ancestries would not provide enough power for an accurate analysis in those groups. Finally, because we decided to use the suggestive threshold for the interaction signals to follow up with for functional annotation and mapping, there is a possibility that some of these associations represent type I error. As this represents the first analysis of a potential sex interaction, future studies should evaluate these regions with larger sample sizes to determine if they are true associations.
In conclusion, our study found potential evidence for an effect modification by sex of genetic associations with two vitamin C related metabolites. In addition, a comprehensive analysis of the functions of genomic regions showing suggestive evidence of genetic variant-sex interactions led to some insight into potential mechanisms for these differences. Future studies are needed to expand on this analysis and further understand the different mechanisms which influence vitamin C concentrations in the body. Mechanisms influencing vitamin C concentrations have several implications for different disease phenotypes (Age-Related Eye Disease Study Research Group, 2001; Villagran et al., 2021) and are an especially important consideration for older adults.
The data analyzed in this study is subject to the following licenses/restrictions: Data are available from the Canadian Longitudinal Study on Aging for researchers who meet the criteria for access to de-identified CLSA data. Requests to access these datasets should be directed to www.clsa-elcv.ca. GWAS data from the genetic variant-sex GWAS are deposited in the GWAS catalogue (https://www.ebi.ac.uk/gwas/home), accession numbers GCST90399837 and GCST90399838. Code is made available on GitHub (https://github.com/Roy-Gagnon-lab).
The studies involving humans were approved by the University of Ottawa Research Ethics Board. The studies were conducted in accordance with the local legislation and institutional requirements. Written informed consent for participation was not required from the participants or the participants’ legal guardians/next of kin in accordance with the national legislation and institutional requirements.
RL: Writing–original draft, Writing–review and editing, Formal Analysis, Investigation, Conceptualization, Data curation, Methodology, Visualization. MR: Validation, Writing–review and editing, Formal Analysis. PH: Conceptualization, Funding acquisition, Writing–review and editing. JL: Funding acquisition, Writing–review and editing, Conceptualization. EF: Conceptualization, Funding acquisition, Supervision, Writing–review and editing, Resources. M-HR-G: Conceptualization, Funding acquisition, Supervision, Writing–review and editing, Investigation, Methodology, Project administration, Resources.
The author(s) declare that financial support was received for the research, authorship, and/or publication of this article. This research was funded by CIHR operating grant PJT-180615 to M-HR-G and EF. Research was funded by a CIHR Canadian Graduate Scholarships Master’s Award to RL.
Funding for the Canadian Longitudinal Study on Aging (CLSA) is provided by the Government of Canada through the Canadian Institutes of Health Research (CIHR) under grant reference: LSA 94473 and the Canada Foundation for Innovation, as well as the following provinces, Newfoundland, Nova Scotia, Quebec, Ontario, Manitoba, Alberta, and British Columbia. The funders had no role in the design, analysis, or the interpretation of results. This research was made possible using the data/biospecimens collected by the Canadian Longitudinal Study on Aging (CLSA). This research has been conducted using the CLSA Comprehensive Baseline Dataset version 7.0, the CLSA Metabolomics dataset version 1.0 and the CLSA Genomic dataset version 3.0 under Application Number 180911. The CLSA is led by Parminder Raina, Christina Wolfson, and Susan Kirkland. The authors thank Dr. Julie St-Pierre for helpful comments regarding the metabolomics data.
The authors declare that the research was conducted in the absence of any commercial or financial relationships that could be construed as a potential conflict of interest.
All claims expressed in this article are solely those of the authors and do not necessarily represent those of their affiliated organizations, or those of the publisher, the editors and the reviewers. Any product that may be evaluated in this article, or claim that may be made by its manufacturer, is not guaranteed or endorsed by the publisher.
The opinions expressed in this article are the authors’ own and do not reflect the views of the Canadian Longitudinal Study on Aging.
The Supplementary Material for this article can be found online at: https://www.frontiersin.org/articles/10.3389/fgene.2024.1411931/full#supplementary-material
Age-Related Eye Disease Study Research Group (2001). A randomized, placebo-controlled, clinical trial of high-dose supplementation with vitamins C and E, beta carotene, and zinc for age-related macular degeneration and vision loss: AREDS report no. 8. Arch. Ophthalmol. 119 (10), 1417–1436. doi:10.1001/archopht.119.10.1417
Amendola, L. M., Dorschner, M. O., Robertson, P. D., Salama, J. S., Hart, R., Shirts, B. H., et al. (2015). Actionable exomic incidental findings in 6503 participants: challenges of variant classification. Genome Res. 25 (3), 305–315. doi:10.1101/gr.183483.114
Balboa-Castillo, T., Struijk, E. A., Lopez-Garcia, E., Banegas, J. R., Rodríguez-Artalejo, F., and Guallar-Castillon, P. (2018). Low vitamin intake is associated with risk of frailty in older adults. Age Ageing 47 (6), 872–879. doi:10.1093/ageing/afy105
Blaschke, E., and Hertting, G. (1971). Enzymic methylation of L-ascorbic acid by catechol O-methyltransferase. Biochem. Pharmacol. 20 (7), 1363–1370. doi:10.1016/0006-2952(71)90263-2
Carr, A., and Maggini, S. (2017). Vitamin C and immune function. Nutrients 9 (11), 1211. doi:10.3390/nu9111211
Carr, A. C., and Lykkesfeldt, J. (2023). Factors affecting the vitamin C dose-concentration relationship: implications for global vitamin C dietary recommendations. Nutrients 15 (7), 1657. doi:10.3390/nu15071657
Carr, A. C., and Zawari, M. (2023). Does aging have an impact on vitamin C status and requirements? A scoping review of comparative studies of aging and institutionalisation. Nutrients 15 (4), 915. doi:10.3390/nu15040915
Caughey, S. D., Wilson, P. W., Mukhtar, N., Brocklehurst, S., Reid, A., D’Eath, R. B., et al. (2018). Sex differences in basal hypothalamic anorectic and orexigenic gene expression and the effect of quantitative and qualitative food restriction. Biol. Sex Differ. 9 (1), 20. doi:10.1186/s13293-018-0178-6
Chapman, K., Holmes, M., and Seckl, J. (2013). 11β-hydroxysteroid dehydrogenases: intracellular gate-keepers of tissue glucocorticoid action. Physiol. Rev. 93 (3), 1139–1206. doi:10.1152/physrev.00020.2012
Chen, Y., Lu, T., Pettersson-Kymmer, U., Stewart, I. D., Butler-Laporte, G., Nakanishi, T., et al. (2023). Genomic atlas of the plasma metabolome prioritizes metabolites implicated in human diseases. Nat. Genet. 55 (1), 44–53. doi:10.1038/s41588-022-01270-1
Darnel, A. D., Archer, T. K., and Yang, K. (1999). Regulation of 11beta-hydroxysteroid dehydrogenase type 2 by steroid hormones and epidermal growth factor in the Ishikawa human endometrial cell line. J. Steroid Biochem. Mol. Biol. 70 (4-6), 203–210. doi:10.1016/S0960-0760(99)00116-8
Deem, J. D., Faber, C. L., and Morton, G. J. (2022). AgRP neurons: regulators of feeding, energy expenditure, and behavior. FEBS J. 289 (8), 2362–2381. doi:10.1111/febs.16176
Duggal, P., Gillanders, E. M., Holmes, T. N., and Bailey-Wilson, J. E. (2008). Establishing an adjusted p-value threshold to control the family-wide type 1 error in genome wide association studies. BMC Genomics 9, 516. doi:10.1186/1471-2164-9-516
Forgetta, V., Li, R., Darmond-Zwaig, C., Belisle, A., Balion, C., Roshandel, D., et al. (2022). Cohort profile: genomic data for 26 622 individuals from the Canadian Longitudinal Study on Aging (CLSA). BMJ Open 12 (3), e059021. doi:10.1136/bmjopen-2021-059021
Garbrecht, M. R., Klein, J. M., McCarthy, T. A., Schmidt, T. J., Krozowski, Z. S., and Snyder, J. M. (2007). 11-Beta hydroxysteroid dehydrogenase type 2 in human adult and fetal lung and its regulation by sex steroids. Pediatr. Res. 62 (1), 26–31. doi:10.1203/PDR.0b013e3180676cf3
Hysi, P. G., Khawaja, A. P., Menni, C., Tamraz, B., Wareham, N., Khaw, K.-T., et al. (2019). Ascorbic acid metabolites are involved in intraocular pressure control in the general population. Redox Biol. 20, 349–353. doi:10.1016/j.redox.2018.10.004
Hysi, P. G., Mangino, M., Christofidou, P., Falchi, M., Karoly, E. D., Nihr Bioresource, I., et al. (2022). Metabolome genome-wide association study identifies 74 novel genomic regions influencing plasma metabolites levels. Metabolites 12 (1), 61. doi:10.3390/metabo12010061
Jiang, L., Zheng, Z., Qi, T., Kemper, K. E., Wray, N. R., Visscher, P. M., et al. (2019). A resource-efficient tool for mixed model association analysis of large-scale data. Nat. Genet. 51 (12), 1749–1755. doi:10.1038/s41588-019-0530-8
Jinesh, G. G., and Kamat, A. M. (2017). The blebbishield emergency program overrides chromosomal instability and phagocytosis checkpoints in cancer stem cells. Cancer Res. 77 (22), 6144–6156. doi:10.1158/0008-5472.CAN-17-0522
Krumsiek, J., Suhre, K., Evans, A. M., Mitchell, M. W., Mohney, R. P., Milburn, M. V., et al. (2012). Mining the unknown: a systems approach to metabolite identification combining genetic and metabolic information. PLoS Genet. 8 (10), e1003005. doi:10.1371/journal.pgen.1003005
Kume-Kick, J., and Rice, M. E. (1998). Estrogen-dependent modulation of rat brain ascorbate levels and ischemia-induced ascorbate loss. Brain Res. 803 (1-2), 105–113. doi:10.1016/s0006-8993(98)00628-3
Little, J., Higgins, J. P., Ioannidis, J. P., Moher, D., Gagnon, F., von Elm, E., et al. (2009). STrengthening the REporting of genetic association studies (STREGA): an extension of the STROBE statement. PLoS Med. 6 (2), e22. doi:10.1371/journal.pmed.1000022
Lotta, L. A., Pietzner, M., Stewart, I. D., Wittemans, L. B. L., Li, C., Bonelli, R., et al. (2021). A cross-platform approach identifies genetic regulators of human metabolism and health. Nat. Genet. 53 (1), 54–64. doi:10.1038/s41588-020-00751-5
Machiela, M. J., and Chanock, S. J. (2015). LDlink: a web-based application for exploring population-specific haplotype structure and linking correlated alleles of possible functional variants. Bioinformatics 31 (21), 3555–3557. doi:10.1093/bioinformatics/btv402
McLaren, W., Gil, L., Hunt, S. E., Riat, H. S., Ritchie, G. R., Thormann, A., et al. (2016). The Ensembl variant effect predictor. Genome Biol. 17 (1), 122. doi:10.1186/s13059-016-0974-4
Michelotti, G., Wong, K., Forgetta, V., Chen, Y., Richards, B., Ivica, J., et al. (2023). Metabolomic profiling on 9,992 participants using ultra-performance liquid chromatography and mass spectrometer data support document. Available at: https://www.clsa-elcv.ca/doc/5126.
Nathani, M. G., and Nath, M. C. (1972). Effect of corticosteroids on ascorbic acid metabolism in rats. Metabolism 21 (8), 779–786. doi:10.1016/0026-0495(72)90125-4
Playdon, M. C., Joshi, A. D., Tabung, F. K., Cheng, S., Henglin, M., Kim, A., et al. (2019). Metabolomics analytics workflow for epidemiological research: perspectives from the consortium of metabolomics studies (COMETS). Metabolites 9 (7), 145. doi:10.3390/metabo9070145
Raina, P., Wolfson, C., Kirkland, S., Griffith, L. E., Balion, C., Cossette, B., et al. (2019). Cohort profile: the Canadian longitudinal study on aging (CLSA). Int. J. Epidemiol. 48 (6), 1752–1753j. doi:10.1093/ije/dyz173
Rastegar Panah, M., Tahir, I., Garcia-Bailo, B., Lo, K., Jarvi, K., and El-Sohemy, A. (2023). Ascorbic acid is associated with favourable hormonal profiles among infertile males. Front. Reprod. Health 5, 1143579. doi:10.3389/frph.2023.1143579
Rentzsch, P., Witten, D., Cooper, G. M., Shendure, J., and Kircher, M. (2019). CADD: predicting the deleteriousness of variants throughout the human genome. Nucleic Acids Res. 47 (D1), D886-D894–D894. doi:10.1093/nar/gky1016
Schleicher, R. L., Carroll, M. D., Ford, E. S., and Lacher, D. A. (2009). Serum vitamin C and the prevalence of vitamin C deficiency in the United States: 2003-2004 national health and nutrition examination survey (NHANES). Am. J. Clin. Nutr. 90 (5), 1252–1263. doi:10.3945/ajcn.2008.27016
Schlosser, P., Scherer, N., Grundner-Culemann, F., Monteiro-Martins, S., Haug, S., Steinbrenner, I., et al. (2023). Genetic studies of paired metabolomes reveal enzymatic and transport processes at the interface of plasma and urine. Nat. Genet. 55 (6), 995–1008. doi:10.1038/s41588-023-01409-8
Taliun, D., Harris, D. N., Kessler, M. D., Carlson, J., Szpiech, Z. A., Torres, R., et al. (2021). Sequencing of 53,831 diverse genomes from the NHLBI TOPMed Program. Nature 590 (7845), 290–299. doi:10.1038/s41586-021-03205-y
Tolbert, B. M., Downing, M., Carlson, R. W., Knight, M. K., and Baker, E. M. (1975). Chemistry and metabolism of ascorbic acid and ascorbate sulfate. Ann. N. Y. Acad. Sci. 258, 48–69. doi:10.1111/j.1749-6632.1975.tb29267.x
Travica, N., Ried, K., Hudson, I., Sali, A., Scholey, A., and Pipingas, A. (2020). The contribution of plasma and brain vitamin C on age and gender-related cognitive differences: a mini-review of the literature. Front. Integr. Neurosci. 14, 47. doi:10.3389/fnint.2020.00047
Turner, S. D. (2018). qqman: an R package for visualizing GWAS results using Q-Q and manhattan plots. J. Open Source Softw. 3 (25), 731. doi:10.21105/joss.00731
Villagran, M., Ferreira, J., Martorell, M., and Mardones, L. (2021). The role of vitamin C in cancer prevention and therapy: a literature review. Antioxidants (Basel) 10 (12), 1894. doi:10.3390/antiox10121894
Walker, D. I., Valvi, D., Rothman, N., Lan, Q., Miller, G. W., and Jones, D. P. (2019). The metabolome: a key measure for exposome research in epidemiology. Curr. Epidemiol. Rep. 6, 93–103. doi:10.1007/s40471-019-00187-4
Wang, Q., Zhang, P., and Gao, H.-B. (2009). Luteinizing hormone induces expression of 11beta-hydroxysteroid dehydrogenase type 2 in rat Leydig cells. Reproductive Biol. Endocrinol. 7 (1), 39. doi:10.1186/1477-7827-7-39
Watanabe, K., Taskesen, E., van Bochoven, A., and Posthuma, D. (2017). Functional mapping and annotation of genetic associations with FUMA. Nat. Commun. 8 (1), 1826. doi:10.1038/s41467-017-01261-5
Wild, C. P. (2005). Complementing the genome with an “exposome”: the outstanding challenge of environmental exposure measurement in molecular epidemiology. Cancer Epidemiol. Biomarkers Prev. 14 (8), 1847–1850. doi:10.1158/1055-9965.EPI-05-0456
Yang, J., Ferreira, T., Morris, A. P., Medland, S. E., Madden, P. A. F., Heath, A. C., et al. (2012). Conditional and joint multiple-SNP analysis of GWAS summary statistics identifies additional variants influencing complex traits. Nat. Genet. 44 (4), 369–S3. doi:10.1038/ng.2213
Yin, X., Chan, L. S., Bose, D., Jackson, A. U., VandeHaar, P., Locke, A. E., et al. (2022). Genome-wide association studies of metabolites in Finnish men identify disease-relevant loci. Nat. Commun. 13 (1), 1644. doi:10.1038/s41467-022-29143-5
Keywords: vitamin C, metabolites, GWAS, gene-environment interaction, CLSA
Citation: Lelievre R, Rakesh M, Hysi PG, Little J, Freeman EE and Roy-Gagnon M-H (2024) Effect modification by sex of genetic associations of vitamin C related metabolites in the Canadian Longitudinal study on aging. Front. Genet. 15:1411931. doi: 10.3389/fgene.2024.1411931
Received: 03 April 2024; Accepted: 04 July 2024;
Published: 31 July 2024.
Edited by:
Yan Wen, Xi’an Jiaotong University, ChinaReviewed by:
Haiping Duan, Qingdao Municipal Center for Disease Control and Prevention, ChinaCopyright © 2024 Lelievre, Rakesh, Hysi, Little, Freeman and Roy-Gagnon. This is an open-access article distributed under the terms of the Creative Commons Attribution License (CC BY). The use, distribution or reproduction in other forums is permitted, provided the original author(s) and the copyright owner(s) are credited and that the original publication in this journal is cited, in accordance with accepted academic practice. No use, distribution or reproduction is permitted which does not comply with these terms.
*Correspondence: Marie-Hélène Roy-Gagnon, bXJveWdhZ25AdW90dGF3YS5jYQ==
Disclaimer: All claims expressed in this article are solely those of the authors and do not necessarily represent those of their affiliated organizations, or those of the publisher, the editors and the reviewers. Any product that may be evaluated in this article or claim that may be made by its manufacturer is not guaranteed or endorsed by the publisher.
Research integrity at Frontiers
Learn more about the work of our research integrity team to safeguard the quality of each article we publish.