- 1Shantou University Medical College, Shantou, Guangdong, China
- 2Research Center of Translational Medicine, Second Affiliated Hospital of Shantou University Medical College, Shantou, Guangdong, China
- 3Plastic Surgery Institute of Shantou University Medical College, Shantou, Guangdong, China
- 4Shantou Plastic Surgery Clinical Research Center, Shantou, Guangdong, China
- 5Department of Plastic Surgery and Burns Center, Second Affiliated Hospital, Shantou University Medical College, Shantou, Guangdong, China
Background: Varicose veins (VV) are a common chronic venous disease that is influenced by multiple factors. It affects the quality of life of patients and imposes a huge economic burden on the healthcare system. This study aimed to use integrated analysis methods, including Mendelian randomization analysis, to identify potential pathogenic genes and drug targets for VV treatment.
Methods: This study conducted Summary-data-based Mendelian Randomization (SMR) analysis and colocalization analysis on data collected from genome-wide association studies and cis-expression quantitative trait loci databases. Only genes with PP.H4 > 0.7 in colocalization were chosen from the significant SMR results. After the above analysis, we screened 12 genes and performed Mendelian Randomization (MR) analysis on them. After sensitivity analysis, we identified four genes with potential causal relationships with VV. Finally, we used transcriptome-wide association studies and The Drug-Gene Interaction Database data to identify and screen the remaining genes and identified four drug targets for the treatment of VV.
Results: We identified four genes significantly associated with VV, namely, KRTAP5-AS1 [Odds ratio (OR) = 1.08, 95% Confidence interval (CI): 1.05–1.11, p = 1.42e-10] and PLEKHA5 (OR = 1.13, 95% CI: 1.06–1.20, p = 6.90e-5), CBWD1 (OR = 1.05, 95% CI: 1.01–1.11, p = 1.42e-2) and CRIM1 (OR = 0.87, 95% CI: 0.81–0.95, p = 3.67e-3). Increased expression of three genes, namely, KRTAP5-AS1, PLEKHA5, and CBWD1, was associated with increased risk of the disease, and increased expression of CRIM1 was associated with decreased risk of the disease. These four genes could be targeted for VV therapy.
Conclusion: We identified four potential causal proteins for varicose veins with MR. A comprehensive analysis indicated that KRTAP5-AS1, PLEKHA5, CBWD1, and CRIM1 might be potential drug targets for varicose veins.
1 Introduction
Varicose veins (VV) is a common chronic venous disease affecting approximately one-third of the global population (Oliveira et al., 2021). VV can manifest with a wide range of clinical presentations, ranging from asymptomatic to severe symptoms, including edema, pigmentation changes, eczema, lipodermatosclerosis, atrophie blanche, and healing or active venous ulcers (Youn and Lee, 2019). The economic burden of VV on healthcare systems is substantial (Malgor and Labropoulos, 2012). Current treatment approaches primarily involve pharmacotherapy, which is not always universally effective, necessitating more targeted therapeutic methods (Belramman et al., 2019).
The incidence of VV is influenced by various factors, such as genetic susceptibility, environmental factors, hormones, endothelial dysfunction, activation of inflammatory cells and molecules, and disruption of cytokines and matrix metalloproteinase balance (Raffetto, 2018). Observational epidemiological studies are prone to confounding, reverse causation, and various biases, which limit a deep understanding of the disease’s pathogenesis and treatment targets. However, Mendelian randomization (MR) methods, which are based on the random distribution of genetic polymorphisms, simulate the effects of randomized controlled trials and can eliminate the influence of confounding factors. In the context of VV research, this implies a more accurate assessment of the causal relationship between the drug targets and disease while excluding factors that could interfere with the results (Smith and Hemani, 2014). There are also many studies that use machine learning and other methods to explore drug targets (Yang et al., 2021; Yang et al., 2022).
MR employs genetic variants as instrumental variables to estimate the causal effect of an exposure on the outcomes. It has been widely applied in other disease studies and helped successfully identify therapeutic targets for various diseases (Georgakis and Gill, 2021; Cao et al., 2023). However, there is limited research on employing MR methods to explore potential drug targets for VV. Therefore, to gain a deeper understanding of the pathogenesis of VV and identify more effective treatment approaches, further MR studies are needed to evaluate the drug targets for VV. This will help eliminate factors that could interfere with the results and offer new perspectives and methods for the treatment of VV.
Conceptually, single nucleotide polymorphisms (SNPs) are randomly distributed and not influenced by environmental factors, making them an ideal tool for causal inference. MR is a form of instrumental variable analysis that primarily utilizes SNPs as genetic instruments to estimate the causal effect of an exposure (in this case, circulatory proteins) on the outcomes (Byrska-Bishop et al., 2022). MR has been successfully used in previous studies to identify biomarkers and treatment targets for various diseases, including aortic aneurysms (Chen et al., 2022), multiple sclerosis (Lin et al., 2023b), and breast cancer (Wang et al., 2023).
In the MR analysis of drug targets, cis-expression quantitative trait loci (cis-eQTLs) located in the drug target gene regions are often considered proxies that act as regulators of gene expression. Previous research has identified potential pathogenic proteins for VV, such as IRF3, LUM, POSTN, RSPO3, and SARS2, through a drug target MR analysis that focused on 2,004 plasma proteins (Lin et al., 2023a). However, this study had significant limitations, and the analysis of drug targets for VV remains insufficient, with numerous potential drug targets awaiting discovery. To address this research gap, our study focuses on conducting drug-target research with genes as the exposure factor for VV. Unlike previous studies that used proteins as the exposure factor, we explored the role of genes in the development of VV and as potential drug targets. Research using genes as the exposure factor has several advantages. First, genes have stable genetic characteristics, and they are unaffected by environmental factors, allowing for a more accurate assessment of the causal relationship between genes and VV (Shah et al., 2020). Second, using genes as the exposure factor can provide more direct information and help reveal potential pathogenic mechanisms and drug targets for VV (Crous-Bou et al., 2016). By exploring VV’s potential drug targets through genes as the exposure factor, we aim to offer new perspectives and methods for the treatment and prevention of VV.
In this study, our goal was to identify potential pathogenic genes for VV. We conducted summary-data-based Mendelian randomization (SMR, 2022), MR, and transcriptome-wide association study (TWAS, 2022) analyses by combining eQTLs identified in blood with independent genome-wide association study (GWAS) datasets for varicose veins.
2 Materials and methods
2.1 Datasets
2.1.1 GWAS
We obtained summary-level GWAS data on VV from the FinnGen consortium, which comprise 17,027 cases with VV and 190,028 controls. To the best of our knowledge, these data constitute the most recent GWAS findings, encompassing the largest number of VV cases to date. The overarching objective of FinnGen is to accumulate and meticulously analyze the genome and national health register data from 500,000 Finnish individuals (Kurki et al., 2023).
For external replication with an independent sample cohort, we used the summary statistics from the UK Biobank. The UK Biobank is a large-scale biomedical database that encompasses genetic and health information of more than 500,000 participants in the United Kingdom. This resource provides a valuable platform for researchers and offers extensive data on various health conditions, lifestyle factors, and genetic profiles. In our study, the UK Biobank dataset included 10,044 patients with VV and 452,966 controls and contributed to the robustness and generalizability of our findings (Fry et al., 2017; Hemani et al., 2018).
2.1.2 eQTL
In our study’s context of drug development, we focused on cis-eQTLs that were in proximity to the target gene. These cis-eQTLs for the SMR analysis were sourced from the eQTLGen Consortium and the eQTL meta-analysis conducted on peripheral blood samples from a cohort of 31,684 individuals (Võsa et al., 2021). These selected cis-eQTLs served as valuable inputs for our SMR, colocalization (Giambartolomei et al., 2014), and MR analyses.
For the TWAS analysis of the eQTL data, we used the Genotype-Tissue Expression (GTEx) project v8 European whole blood dataset. GTEx is a notable biomedical research endeavor dedicated to comprehending the diversity in human gene expression across diverse tissues and organs to elucidate its intricate connections with genotype. Given our specific emphasis on VV, we meticulously extracted comprehensive eQTL results exclusively from the whole blood samples within the GTEx dataset (GTEx Consortium, 2020).
2.1.3 DGIdb
The Drug Gene Interaction Database (DGIdb) is as a comprehensive drug-gene interaction network resource. It amalgamates various data sources that elucidate the interactions between drugs and genes (Wagner et al., 2016) as well as the pharmaceutical relevance of genes. In this study, we used the DGIdb for the identification of candidate gene targets for gene therapy from the previously identified genes.
2.2 SMR analysis
A flow diagram summarizing the methodology is shown in Figure 1. We conducted summary data-based Mendelian randomization (SMR) and heterogeneity in dependent instruments (HEIDI) tests analyses on cis regions using the SMR software (version 1.03) (SMR, 2022). The methodologies for SMR analysis are detailed in the original work by (Zhu et al., 2016). In brief, SMR analysis uses a well-established MR approach. This technique employs a SNP at a prominent cis-eQTL as an instrumental variable (IV). The summary-level eQTL data act as the exposure, and the GWAS data for a specific trait serve as the outcome. The primary objective is to explore a potential causal or pleiotropic association, wherein the same causal variant is shared between gene expression and the trait.
It is crucial to acknowledge that the SMR method lacks the ability to distinguish between a causal association, in which gene expression causally influences the trait, and a pleiotropic association, in which the same SNP affects both gene expression and the trait. This limitation arises due to the single IV in the MR method, which cannot differentiate causality from pleiotropy. Nevertheless, the HEIDI test can make this distinction by discerning causality and pleiotropy from linkage. Linkage denotes cases in which two different SNPs in linkage disequilibrium (LD) independently impact gene expression and the trait. Although less biologically intriguing than causality and pleiotropy, the HEIDI test brings clarity in such scenarios.
For the SMR tests, statistical significance was set at a p-value less than 3.198362 × 10^-6 (Bonferroni correction of 0.05 divided by 15,633, the number of results in the eQTL data). For the HEIDI test, a p-value below 0.05 was considered significant and suggesting that the observed association was attributable to linkage (Zhu et al., 2016).
2.3 Colocalization analysis
We conducted colocalization analysis using the coloc package within the R software environment (version 4.0.3) (Giambartolomei et al., 2014). The purpose of colocalization analysis is to evaluate whether SNPs associated with both gene expression and phenotype at a given locus are indeed shared causal variants, indicating the “colocalization” of gene expression and the phenotype. The analysis, implemented through colocalization analysis, computes posterior probabilities (PPs) for five hypotheses: 1) H0, indicating no association with either gene expression or the phenotype; 2) H1, denoting an association solely with gene expression; 3) H2, signifying an association exclusively with the phenotype; 4) H3, suggesting an association with both gene expression and the phenotype through independent SNPs; and 5) H4, implying an association with both the gene expression and phenotype through shared causal SNPs. A substantial PP for H4 (PP.H4 above 0.70) strongly indicates the existence of shared causal variants influencing both gene expression and the phenotype (Giambartolomei et al., 2014).
2.4 MR analysis
The two-sample MR analysis was chosen due to its robustness in assessing causality in observational data, by using SNPs as IVs, this method avoids the behavioral and environmental factors that influence epidemiological studies, reducing potential bias in observational studies. To perform the two-sample MR analysis (Hemani et al., 2018; Two Sample, 2022), we used the TwoSampleMR R package (version 0.5.6). The application of the two-sample MR framework requires the use of two distinct datasets. In this study, genetic instruments, specifically cis-eQTL, were employed as the exposures, and GWAS data served as the outcome trait data. The MR methodology evaluates the association between gene expression and diseases or traits by leveraging genetic variants linked to gene expression as the instrumental variables (exposure) and GWAS as the outcomes. MR allows for the exploration of whether changes in gene expression causally influence diseases or traits. For instruments represented by a SNP, the Wald ratio was applied. In the cases in which the instruments comprised multiple SNPs, the inverse variance-weighted MR approach was implemented.
The MR analysis was exclusively conducted for the results of the SMR and colocalization analyses. We applied the false discovery rate (FDR) method to correct the p values. The analysis of pleiotropy employed the MR_pleiotropy_test function, where a p-value less than 0.05 was considered to indicate the presence of pleiotropy, and a p-value greater than 0.05 indicated its absence. Significance parameters were set to p < 5 × 10^-8 for genome-wide significance; the linkage disequilibrium parameter (r^2) was set to 0.1, and the genetic distance was set to 10 Mb.
2.5 TWAS analysis
TWAS is a research approach employed to establish connections between the gene expression levels and intricate networks governing gene expression. By scrutinizing the extensive transcriptomic datasets, researchers can discern distinct gene expression patterns across varied biological conditions. This method enables the identification of pivotal genes linked to specific physiological processes or diseases while elucidating intricate gene interactions. Through these investigations, essential genes crucial to distinct biological processes are pinpointed, revealing their regulatory networks and potential signaling pathways (Gusev et al., 2016).
In this study, a TWAS analysis was conducted on autosomal chromosomes using Fusion software (TWAS), following the default parameters specified in the fusion guidelines (Gusev et al., 2016). To assess colocalization, we employed the coloc R package for genes that achieved transcriptome-wide significance and were located within a 1.5 Mb range. This Bayesian methodology calculates posterior probabilities (PP), representing the likelihood of shared causal variants within a locus for two outcomes. The analytical approach employed in this study distinguishes associations influenced by horizontal pleiotropy (a solitary causal SNP impacting both transcription and VV; posterior probability PP4) from those arising due to linkage (two causal SNPs in LD affecting transcription and VV independently; posterior probability PP3) (Giambartolomei et al., 2014).
2.6 GWAS analysis
The Multimarker Analysis of Genomic Annotation (MAGMA) serves as a versatile instrument for gene examination and comprehensive gene-set analysis of GWAS data (MAGMA, 2022). It facilitates the analysis of raw genotype data and summary SNP p-values derived from prior GWAS endeavors or meta-analyses. MAGMA utilizes a multiple regression model for assessing the combined effect of several SNPs assigned to a specific gene (±10 kb) (Leeuw et al., 2015). The Phase 3 dataset from the European population of the 1000 Genomes Project is employed as the reference panel for computing linkage disequilibrium (LD) (The 1000 Genomes Project Consortium et al., 2015).
3 Result
3.1 SMR analysis and colocalization for preliminary identifying potential genes
In the initial phase of our analysis, we conducted SMR analysis using the eQTLGen dataset to identify genes significantly associated with VV features. To establish the significance threshold for the p values, we applied the Bonferroni method to establish the threshold for the p values. Specifically, for the eQTLGen dataset, we obtained a wealth of information, resulting in 15,633 candidate genes. To maintain a robust level of significance, we set the significance threshold at (0.05/15,633 = 3.198362e-06). In the SMR analysis, we identified 21 genes (SLC2A1-AS1, CRIM1, PDK1, AC007401.2, AC093818.1, HMCES, HSPA4, TRIM10, HCG22, NOS3, CBWD1, ZNF438, ARHGEF17, ADM, KRTAP5-AS1, PLEKHA5, NFATC3, PPL, DPEP3, MAP2K4, and LSM4) that met the significance threshold of p < 3.198362 E-06. To enhance interpretability, Manhattan plots were generated for a clear visualization of the SMR results (Table 1; Figure 2).
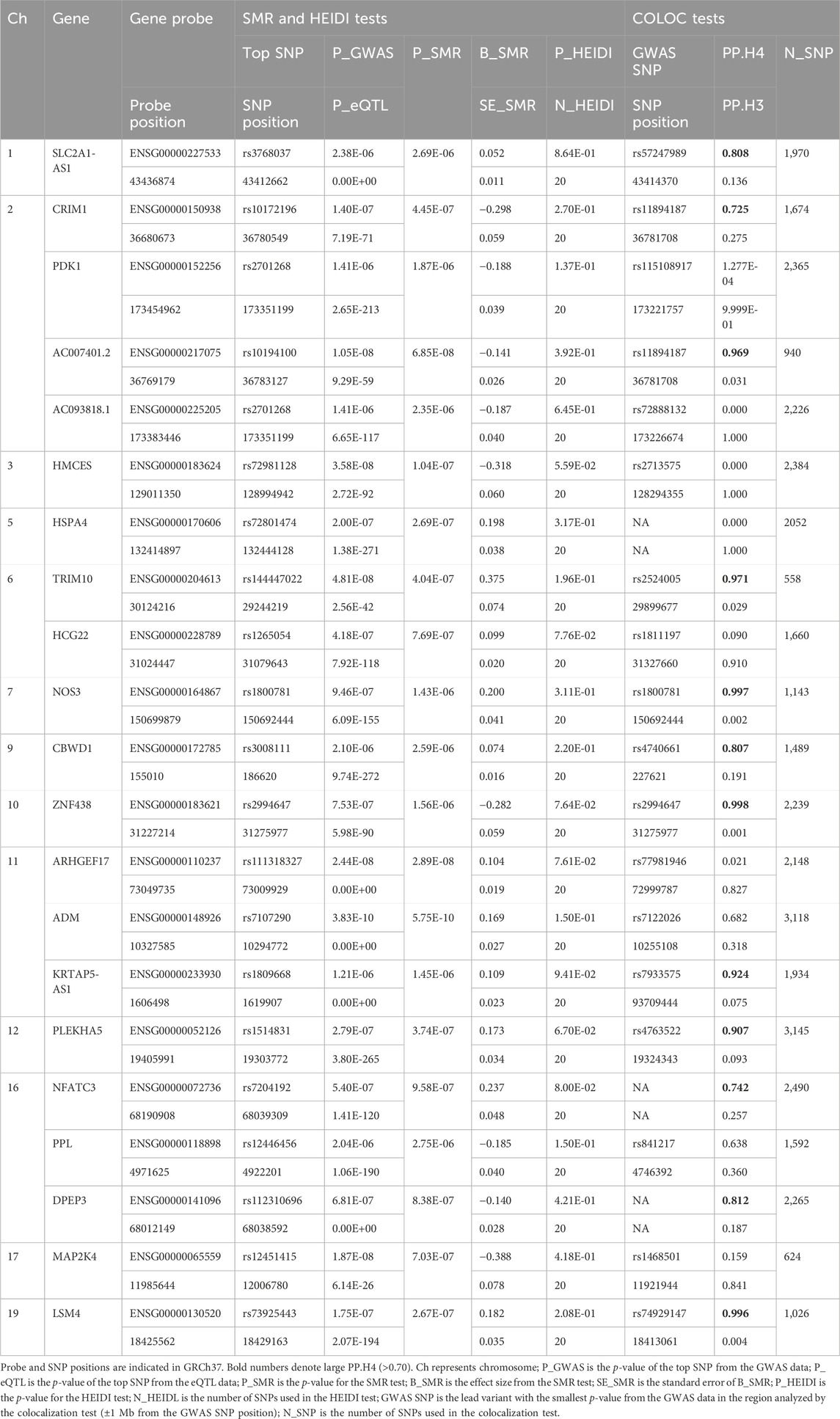
Table 1. SMR/HEIDI results of the GWAS data on VV, blood eQTL data, and colocalization results between GWAS and the blood eQTL data for genes passing the SMR Test.
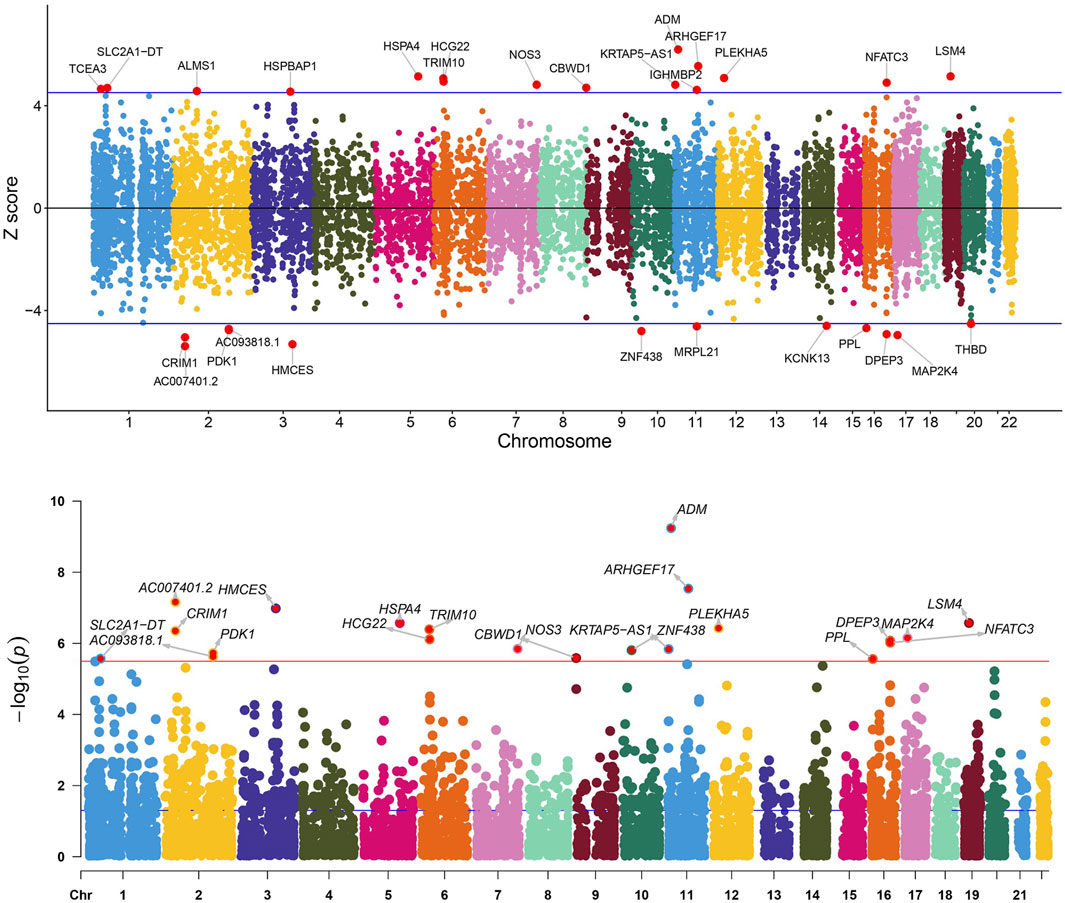
Figure 2. Manhattan plot of the SMR analysis results using QTLs and VV GWAS summary statistics. The red dashed line represents the Bonferroni-corrected significance threshold (Sig_P_Thresh). Z-score filtration was applied, with the blue dashed line indicating the Z score significance level, where Sig_Z_Thresh was calculated as qnorm [1—(0.05/Sig_P_Thresh)].
Following the identification of these significant genes, we employed the HEIDI heterogeneity test (p_HEIDI >0.05) to filter out genes lacking horizontal pleiotropy. In summary, through the integrated analysis of GWAS and blood eQTL data, we identified a total of 21 genes significantly associated with VV features in blood.
Moving forward, we conducted colocalization analysis to integrate the GWAS and blood eQTL data for the genes that successfully passed the SMR test. This analysis aimed to assess whether these genes were colocalized with the trait of interest, VV. The results from the colocalization test provided robust evidence supporting colocalization between the trait and all 12 genes (KRTAP5-AS1, SLC2A1-DT, PLEKHA5, NOS3, LSM4, DPEP3, CRIM1, ZNG1A, ZNF438, NFATC3, TRIM10, and AC007401.2) that met the criteria of both the SMR and HEIDI tests. Therefore, we consider these genes as high-priority candidates for subsequent functional studies (Figures 3, 4).
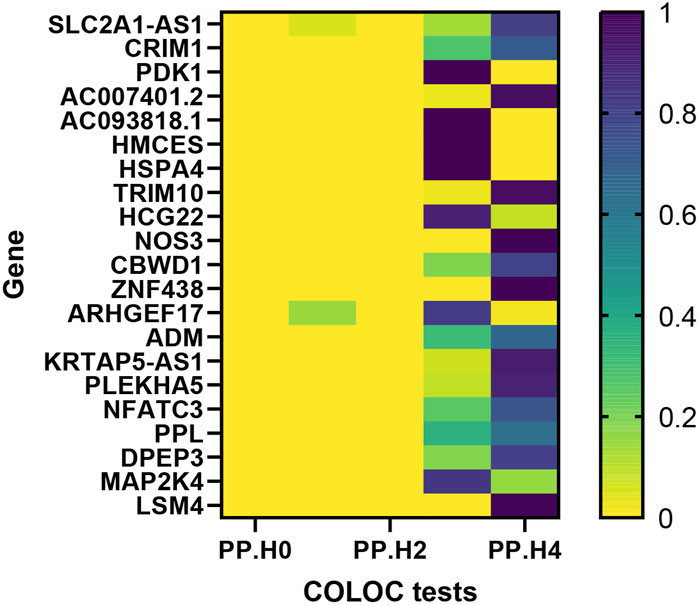
Figure 3. Heat map of the colocalisation analysis results using QTLs and VV GWAS summary statistics. The x-axis represents five hypotheses of colocalization, while the y-axis indicates the genes involved in the analysis. Each colored block in the heat map represents the probability associated with a specific colocalization hypothesis.
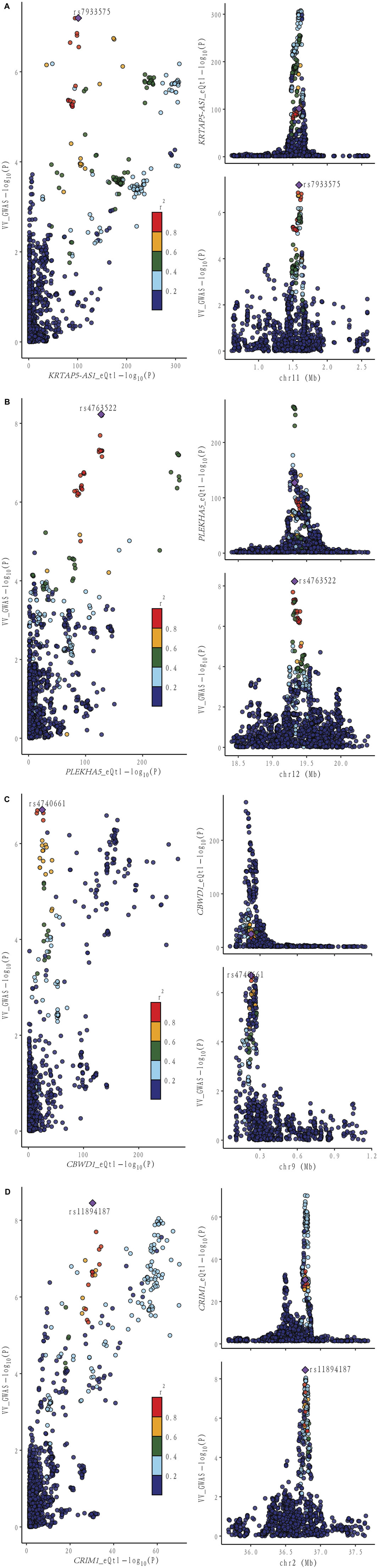
Figure 4. Locus comparison plot illustrating the colocalization analysis results for the single nucleotide variants associated with gene expression in both blood and VV data. Each dot on the plot represents a SNP, and its color corresponds to the LD value (r2) with the GWAS lead variant, depicted as a purple diamond. In the right panel, the genomic positions (in megabases, GRCh37) on the chromosome are presented on the x-axis. The -log10 p values for SNPs from the VV GWAS (top) and the eQTL study for the genes (bottom) are displayed on the y-axis. In the left panel, a comparison of p values is shown for both the VV GWAS and the gene expression eQTL study. Different genes are displayed separately: (A) KRTAP-AS1. (B) PLEKHA5. (C) CBWD1. (D) CRIM1.
3.2 MR analysis validates potential gene causal relationships
3.2.1 Discovery cohort
Utilizing cis-eQTL data provided by the eQTLGen Consortium and by employing SMR and colocalization analyses, we identified 12 potential genes that could influence VV. Subsequently, a two-sample MR analysis was conducted on the European summary statistics involving patients with VV. In the discovery cohort, which comprised 17,027 patients and 190,028 controls from the FinnGen cohort, the inverse variance weighted (IVW) MR analysis amalgamated the effect estimates from each genetic instrument. The genetically predicted expression of nine genes was found to be associated with VV risk, after multiple testing adjustments (FDR correction). To ensure the robustness of our findings, we performed tests for horizontal pleiotropy, which indicated no evidence of horizontal pleiotropy in the dataset.
In our study, we performed a two-sample MR analysis using the 12 genes. The results are presented in Table 2. In the discovery cohort, significant associations were observed for nine genes (KRTAP5-AS1, SLC2A1-DT, NOS3, PLEKHA5, LSM4, DPEP3, CRIM1, AC007401.2, and ZNG1A). Heterogeneity tests and horizontal pleiotropy tests were conducted. The heterogeneity test revealed heterogeneity for PLEKHA5 and CBWD1. Consequently, we applied the random effects inverse variance weighted method to address this heterogeneity in the subsequent analysis. The results of the horizontal pleiotropy analysis indicated the absence of horizontal pleiotropy. These additional details provide a nuanced understanding of the robustness and validity of our findings in the context of VV genetics.
3.2.2 Replication cohort
In our endeavor to validate the genetic associations identified in the discovery phase, we conducted a rigorous replication analysis using data from the UK Biobank cohort, comprising 10,044 cases and 452,966 controls. This replication effort aimed to reproduce the effect estimates of the top nine genes identified in the discovery stage, providing crucial insights into the robustness and generalizability of our findings.
We observed significant associations with VV for KRTAP5-AS1 [Odds ratio (OR) = 1.08, 95% CI: 1.05–1.11, p = 1.42e-10], PLEKHA5 (OR = 1.13, 95% CI: 1.06–1.20, p = 6.90e-5), CBWD1 (OR = 1.05, 95% CI: 1.01–1.11, p = 1.42e-2), and CRIM1 (OR = 0.87, 95% CI: 0.81–0.95, p = 3.67e-3). Notably, these findings underscore the robustness and consistency of the genetic associations across different cohorts, reinforcing the potential relevance of these genes to VV.
Of particular significance, our replication analysis demonstrated successful replication beyond the FDR method for four genes (KRTAP5-AS1, PLEKHA5, CBWD1, and CRIM1), exhibiting 100% consistency in the direction of the effect. This remarkable consistency further validates the genetic associations and supports the reliability of our findings. Importantly, horizontal pleiotropy tests indicated no evidence suggesting the presence of this confounding factor in the data, further bolstering the robustness and validity of our replicated genetic associations with VV.
3.3 Validation
3.3.1 TWAS analysis validates transcriptome-level causal relationships
In our pursuit of strengthening the causal inference and gaining deeper insights into the genetic associations with VV, we conducted a TWAS analysis on the four genes identified in the previous analyses. This comprehensive approach aimed to elucidate the transcriptional level associations of these genes with VV and to bolster the evidence supporting their potential causal role. Additionally, we performed colocalization analysis to further validate the potential causal relationship between these genes and VV.
The results revealed significant associations at the transcriptional level for the four genes with VV, with all colocalization probabilities (PP.H4) exceeding 0.7. Specifically, elevated transcription levels of KRTAP5-AS1 (Z score = 4.896, p = 9.76e-7), PLEKHA5 (Z score = 5.205, p = 1.94e-7), and CBWD1 (Z score = 4.839, p = 1.30e-6) were significantly correlated with an increased risk of VV, whereas increased transcription level of CRIM1 (Z score = −3.629, p = 2.85e-4) was significantly associated with a decreased risk of VV. Notably, these TWAS findings were consistent with those from the SMR analysis, providing robust support for the transcriptional associations of these genes with VV.
We also conducted a TWAS colocalization analysis and found that the probability of these four genes sharing the same causal variant (PP.H4: KRTAP5-AS1 = 0 .943, PLEKHA5 = 0.993, CBWD1 = 0.802, CRIM1 = 0.932) exceeded the predefined threshold (p > 0.7).This robust result unequivocally strengthens the indication of the causal relationship between these four genes and VV, further solidifying the genetic basis of VV and providing crucial insights into the potential mechanisms underlying this condition (Table 3).

Table 3. TWAS results between GWAS and blood eQTL data for genes through the TWAS/Colocalization Test.
3.3.2 GWAS analysis validates genome-level causal relationships
We also conducted a GWAS analysis using MAGMA software on the quartet of genes highlighted in preceding studies. This approach aimed to illuminate the associations of these genes with VV in genome-level, thereby reinforcing the evidence underpinning their potential causal involvement.
The outcomes unveiled noteworthy genome-level associations for the aforementioned genes with VV. Specifically, heightened transcription levels of KRTAP5-AS1 (p = 0.022), PLEKHA5 (p = 3.34E-06), and CBWD1 (p = 0.001) exhibited significant correlations with an elevated VV risk, whereas increased transcription levels of CRIM1 (p = 0.008) were significantly linked with a reduced VV risk (Table 4). Importantly, these GWAS findings corroborated those from the SMR analysis, thereby fortifying the robustness of the transcriptional associations of these genes with VV.
3.4 DGIdb screening for pharmacologically relevant genes
Incorporating the comprehensive resources provided by the DGIdb database, our analysis identified four pharmacologically relevant genes (KRTAP5-AS1, PLEKHA5, CBWD1, and CRIM1). This crucial step has unveiled potential drug targets with direct relevance to the treatment of VV, shedding light on promising avenues for targeted therapeutic interventions. By leveraging this pharmacogenomic information, we have advanced our understanding of the molecular underpinnings of VV and have laid the groundwork for the exploration of novel therapeutic strategies tailored to the genetic landscape of this condition.
4 Discussion
To study the potential pathogenic genes of VV and to search for new drug targets, we used an integrated analysis method combining SMR analysis and colocalization analysis. We used an eQTL meta-analysis dataset based on peripheral blood samples as the exposure data with a relatively large sample size for the outcome data from two large databases, FinnGen and UK Biobank. After the analysis of the preliminary results, we screened out some candidate genes and identified them using the DGIdb database. Finally, four VV-related genes were identified: KRTAP5-AS1, PLEKHA5, CBWD1, and CRIM1. The results suggested that there was a potential causal relationship between VV and these four genes. The increased expression of KRTAP5-AS1, PLEKHA5, and CBWD1 was associated with increased risk of VV, and the increased expression of CRIM1 was associated with a significantly decreased risk of VV. The results of this study may provide important evidence for drug development and individualized treatment of VV and contribute to improving the quality of life of VV patients.
There are few studies on KRTAP5-AS1 and CBWD1. The research on KRTAP5-AS1 is mainly focused on its long-chain non-coding RNA (lncRNA), which is a kind of conserved RNA with a length of more than 200 nucleotides and no significant protein-coding ability. There is evidence that it can be used as a new biomarker to predict the prognosis of various cancers (You et al., 2018). For example, Song et al. found that KRTAP5-AS1 can exert the role of a cRNA in regulating claudin-4, and that KRTAP5-AS1 can regulate CLDN4 expression as a competitive endogenous RNA of miR-596 and miR-3620-3p. CLDN4 has been shown to alter expression patterns in various types of cancer, including gastric, pancreatic, and ovarian cancer (Song et al., 2017). In addition, studies have used KRTAP5-AS1 as a lncRNA signal to predict the prognosis of papillary thyroid cancer (You et al., 2018). Other studies have found the potential of eight lncRNA markers, including KRTAP5-AS1, as independent prognostic biomarkers in HBV-positive hepatocellular carcinoma patients (Zhao et al., 2020). Based on the above evidence, we hypothesized that KRTAP5-AS1 could play a regulatory role as ceRNA, affecting the expression of its neighboring genes or negatively regulating the expression of miRNA target genes as miRNA to improve the risk of varicose veins.
CBWD1 is located at 9p24.3:121038-179075 (GRCH37/HG19) and located near the 9p telomere. The absence of 9p syndrome can lead to facial deformity, hypotonia, and intellectual disability. Researchers have found that CBWD1 is associated with some cases of congenital kidney and urinary tract abnormalities. The results have suggested that CBWD1 is associated with the development of ureteric buds into the urinary tract, and homozygous deletion involving CBWD1 can manifest as renal hypoplasia (Kanda et al., 2020). Similar to KRTAP5-AS1, there is an association between CBWD1 and cancer, as reported by Wang et al. (2022), who constructed a brisk tmmodel of CBWD1 as a pivotal gene to predict survival in patients with ovarian cancer. There is evidence that variants on the CBWD1/DOCK8 locus (9P22.3) have enhancer histone markers of CBWD1, promoter histone markers, TF-binding motifs, and cis-EQTL in lung, pancreas, liver, fat, musculoskeletal, artery, heart, fibroblasts, blood, and other tissues (Feitosa et al., 2022). This suggests that variations in CBWD1 may affect the normal function of musculoskeletal, fibroblasts, heart, and blood, thereby causing abnormalities at the venous end and increasing the risk of varicose veins. However, as no such reported cases have been found, further experiments are needed to confirm this conjecture.
PLEKHA5 and CRIM1 are two relatively popular genes that have been studied extensively. PLEKHA5 belongs to the PLEKHA family and contains the pleckstring homology domain. The domain is thought to mediate phosphatidylinositol binding properties and is associated with multiple intracellular functions, such as signaling, cytoskeleton rearrangement, membrane protein targeting, and vesicle trafficking (Dowler et al., 2000). PLEKHA5 has been shown to be expressed on the plasma membrane and associated with microtubules, and it has also been demonstrated to play an important role in cell migration by wound healing assay experiments (Zou and Zhong, 2012). To date, the most thoroughly studied aspect of PLEKHA5 has been its potential regulatory role in melanoma brain metastasis. PLEKHA5 is associated with brain cell activity, and decreased expression of PLEKHA5 inhibited the proliferation of brain nutrient cells as well as the migration of pro-brain cells in an in vitro blood-brain barrier model. PLEKHA5 expression in melanoma is associated with the early development of brain metastases, and up to 75% of patients with stage IV melanoma develop central nervous system metastases during the course of the disease, according to a study by Jilaveanu et al. (2015). PLEKHA5 has also been reported to be involved in the central nervous system homing mechanism in metastatic disease, and inhibition of PLEKHA5 may reduce the passage across the blood-brain barrier and reduce the proliferation and survival of melanoma cells in the brain and extrabrain regions (Jilaveanu et al., 2015). Since there is no experimental evidence to support this, we can only speculate that PLEKHA5’s positive causal relationship to VV may also be mediated by brain metastasis. In addition to melanoma, PLEKHA5 has also been associated with gastric cancer, with studies finding that tyrosine phosphorylation of PLEKHA5 is MET-dependent and associated with MET expression and phosphorylation. PLEKHA5 is able to regulate the survival and peritoneal dissemination of diffuse gastric cancer cells through MET gene amplification (Nagamura et al., 2021). In summary, PLEKHA5 is closely related to multiple internal functions of cells and plays an important role in cell migration, so it may cause varicose veins by promoting the migration of vascular smooth muscle cells.
CRIM1 is a gene encoding a cysteine-rich repeat protein that is developmentally regulated and involved in vertebrate central nervous system development and organogenesis (Kolle et al., 2000). Evidence that CRIM1 haploinsufficiency leads to defects in eye development in humans and mice suggests CRIM1 as a potential causative gene of Macrophthalmia, colobomatous, with microcornea (MACOM) and underscores the importance of CRIM1 in eye development (Beleggia et al., 2015). In addition, CRIM1 is also associated with the formation and maintenance of blood vessels in vivo. During angiogenesis, CRIM1 is strongly upregulated in endothelial cells and expressed by a variety of cell lines with adhesion growth, and the formation of capillary structures is impaired in endothelial cells that are transfected in vitro (Glienke et al., 2002). We hypothesize that the increased expression of CRIM1 might reduce the risk of VV because the endothelial structure of blood vessels is not susceptible to damage. We hypothesized that upregulated expression of CRIM1 reduces the risk of varicose veins because VV is associated with disruption of normal angiogenesis (Chang et al., 2009), however, the endothelial cell structure of blood vessels was less damaged after the gene expression was upregulated, thus reducing the risk of varicose veins.
In the existing research on PLEKHA5 and CRIM1, we did not find any studies linking PLEKHA5 and CRIM1 with VV. Our experimental results demonstrate the existence of a potential causal relationship between PLEKHA5 and CRIM1 and VV, but the possible pathways or mechanisms are unknown. In the past, dissection of the great saphenous vein and the small saphenous vein, which often occur in VV, were the main treatment. However, this method was risky, and the recurrence rate was relatively high, and up to 24% of patients required additional treatment. As a result, minimally invasive procedures such as radiofrequency ablation, intravenous laser therapy, ultrasound-guided foam sclerosis, and others started to be favored (Nijsten et al., 2009). Minimally invasive surgery has also been shown to be relatively safe with few serious complications (Al Samaraee, McCallum, and Mudawi, 2009). In recent years, many articles have discussed and compared these minimally invasive procedures, for example, researchers have found that in many studies, EVLT appears to be more effective than RFA (Ahadiat et al., 2018). In addition, studies have proposed an alternative method of varicose vein implantation, the reduced sine microchannel (DSMC), which can maintain good blood flow in the human leg and avoid tissue damage and other problems (Afzal et al., 2018).
At present, most studies on VV are investigation, descriptive clinical studies and randomized clinical trials, and few studies analyze the pathogenesis and treatment of VV at the genetic level. However, we used the latest GWAS data, combined with the information of eQTL database and DGIdb database. The original data were screened and verified strictly to ensure the accuracy and robustness of the results. Our study also used a comprehensive analysis method including MR Analysis to avoid various biases that may exist in observational epidemiological studies. In addition, different from previous studies that used proteins as exposure factors, we use genes with stable genetic characteristics that are not affected by environmental factors as exposure factors, which can more intuitively and accurately reflect the causal relationship between genes and VV. The four genes we identified have the potential to be targeted pharmacologically and could serve as therapeutic targets in future VV therapies. The current VV treatment is mainly surgical. Although the safety of minimally invasive surgery has been improved, the existence of surgical complications still cannot be completely avoided. Our research findings have contributed to the pharmacological treatment of VV. At the same time, our results provide a new idea and direction for the study of the pathogenesis of VV. In the future, we will continue to conduct more in-depth research and experiments on these four genes, verify and analyze the above conjecture, and strive to give a reasonable explanation of the pathogenesis of varicose veins at the gene level.
There are some limitations in this study. First, our study was only related to theoretical drug target discovery. Second, the two-sample MR analysis used in the study was based on publicly available and free GWAS aggregates with limitations in updating, selection, and screening of the data. Due to the limitations of sample type and public databases, we could not further conduct subgroup analysis on sex differences in varicose veins. The samples included in this study were from a European population. MR can effectively reduce the statistical bias caused by population differences, but the results still may not be generalizable to non-European populations. In addition, although we used a variety of methods to validate the results of our experiments, we cannot rule out the possibility that other confounding factors that are not considered may have influenced the effects of the genes on the disease. Further exploration and validation are required.
In conclusion, this study identified the effects of KRTAP5-AS1, PLEKHA5, CBWD1, and CRIM1 genes on VV. No previous study has found a potential causal relationship between these four genes and VV. Our results provide new causal evidence for VV-related genes as well as potential drug targets and therapeutic ideas for VV therapy.
Data availability statement
The original contributions presented in the study are included in the article/Supplementary material, further inquiries can be directed to the corresponding authors.
Ethics statement
Ethical approval was not required for the study involving humans in accordance with the local legislation and institutional requirements. Written informed consent to participate in this study was not required from the participants or the participants’ legal guardians/next of kin in accordance with the national legislation and the institutional requirements.
Author contributions
YC: Writing–original draft, Writing–review and editing. MH: Writing–original draft, Writing–review and editing. HZ: Writing–original draft, Writing–review and editing. JG: Validation, Writing–review and editing. QW: Validation, Writing–review and editing. ZX: Validation, Writing–review and editing. LC: Formal Analysis, Resources, Writing–review and editing. WZ: Funding acquisition, Resources, Validation, Writing–review and editing. ST: Funding acquisition, Resources, Validation, Writing–review and editing.
Funding
The author(s) declare that financial support was received for the research, authorship, and/or publication of this article. This study was funded by the Guangdong Basic and Applied Basic Research Foundation (2019A1515011857, 2021A1515011142, 2023A1515012343, 2022A1515220099), Guangdong University Innovation Team Project (2021KCXTD047), Provincial science and technology innovation strategy special project funding program (200114165897946, 210714106901245, STKJ202209067, STKJ2023004), 2022 Shantou University Graduated Student Innovation Program [Shandafa (2022)207].
Acknowledgments
The authors appreciate the publicly available data of the FinnGen consortium, the eQTLGen consortium, the GTEx project, and the MRC IEU OpenGWAS database.
Conflict of interest
The authors declare that the research was conducted in the absence of any commercial or financial relationships that could be construed as a potential conflict of interest.
Publisher’s note
All claims expressed in this article are solely those of the authors and do not necessarily represent those of their affiliated organizations, or those of the publisher, the editors and the reviewers. Any product that may be evaluated in this article, or claim that may be made by its manufacturer, is not guaranteed or endorsed by the publisher.
References
Afzal, M. J., Ashraf, M. W., Tayyaba, S., Khalid Hossain, M., and Afzulpurkar, N. (2018). Sinusoidal microchannel with descending curves for varicose veins implantation. Micromachines 9 (2), 59. doi:10.3390/mi9020059
Ahadiat, O., Higgins, S., Ly, A., Nazemi, A., and Wysong, A. (2018). Review of endovenous thermal ablation of the great saphenous vein: endovenous laser therapy versus radiofrequency ablation. Dermatologic Surg. Official Publ. Am. Soc. Dermatologic Surg. 44 (5), 679–688. doi:10.1097/DSS.0000000000001478
Al Samaraee, A., McCallum, I. J. D., and Mudawi, A. (2009). Endovenous therapy of varicose veins: a better outcome than standard surgery? Surg. J. R. Coll. Surg. Edinb. Irel. 7 (3), 181–186. doi:10.1016/s1479-666x(09)80043-7
Beleggia, F., Li, Y., Fan, J., Elcioğlu, N. H., Toker, E., Wieland, T., et al. (2015). CRIM1 haploinsufficiency causes defects in eye development in human and mouse. Hum. Mol. Genet. 24 (8), 2267–2273. doi:10.1093/hmg/ddu744
Belramman, A., Bootun, R., Lane, T. R. A., and Davies, A. H. (2019). Foam sclerotherapy versus ambulatory phlebectomy for the treatment of varicose vein tributaries: study protocol for a randomised controlled trial. Trials 20 (1), 392. doi:10.1186/s13063-019-3398-0
Byrska-Bishop, M., Evani, Us, Zhao, X., Basile, Ao, Abel, Hj, Regier, Aa, et al. (2022). High-coverage whole-genome sequencing of the expanded 1000 genomes project cohort including 602 trios. Cell. 185 (18), 3426–3440.e19. doi:10.1016/j.cell.2022.08.004
Cao, H., Yang, Y., Hu, Q., and Wei, G. (2023). Identification of potential drug targets for rheumatoid arthritis from genetic insights: a mendelian randomization study. J. Transl. Med. 21 (1), 616. doi:10.1186/s12967-023-04474-z
Chang, M.-Y., Chiang, P.-T., Chung, Y.-C., Ho, S.-Y., Lin, S.-D., Lin, S.-Ru, et al. (2009). Apoptosis and angiogenesis in varicose veins using gene expression profiling. Fooyin J. Health Sci. 1 (2), 85–91. doi:10.1016/S1877-8607(10)60005-7
Chen, Y., Xu, X., Wang, L., Li, Ke, Sun, Y., Xiao, L., et al. (2022). Genetic insights into therapeutic targets for aortic aneurysms: a mendelian randomization study. eBioMedicine 83 (September), 104199. doi:10.1016/j.ebiom.2022.104199
Crous-Bou, M., De Vivo, I., Camargo, C. A., Varraso, R., Grodstein, F., Jensen, M. K., et al. (2016). Interactions of established risk factors and a GWAS-based genetic risk score on the risk of venous thromboembolism. Thrombosis Haemostasis 116 (4), 705–713. doi:10.1160/TH16-02-0172
Dowler, S., Currie, R. A., Campbell, D. G., Deak, M., Kular, G., Downes, C. P., et al. (2000). Identification of pleckstrin-homology-domain-containing proteins with novel phosphoinositide-binding specificities. Biochem. J. 351 (Pt 1), 19–31. doi:10.1042/0264-6021:3510019
Feitosa, M. F., Wojczynski, M. K., Anema, J. A., Warwick Daw, E., Wang, L., Santanasto, A. J., et al. (2022). Genetic pleiotropy between pulmonary function and age-related traits: the long life family study. Journals Gerontology. Ser. A, Biol. Sci. Med. Sci. 79 (3), glac046. doi:10.1093/gerona/glac046
Fry, A., Littlejohns, T. J., Sudlow, C., Doherty, N., Adamska, L., Sprosen, T., et al. (2017). Comparison of sociodemographic and health-related characteristics of UK Biobank participants with those of the general population. Am. J. Epidemiol. 186 (9), 1026–1034. doi:10.1093/aje/kwx246
Georgakis, M. K., and Gill, D. (2021). Mendelian randomization studies in stroke: exploration of risk factors and drug targets with human genetic data. Stroke 52 (9), 2992–3003. doi:10.1161/STROKEAHA.120.032617
Giambartolomei, C., Vukcevic, D., Schadt, E. E., Franke, L., Hingorani, A. D., Wallace, C., et al. (2014). Bayesian test for colocalisation between pairs of genetic association studies using summary statistics. PLoS Genet. 10 (5), e1004383. doi:10.1371/journal.pgen.1004383
Glienke, J., Sturz, A., Menrad, A., and Thierauch, K. H. (2002). CRIM1 is involved in endothelial cell capillary formation in vitro and is expressed in blood vessels in vivo. Mech. Dev. 119 (2), 165–175. doi:10.1016/s0925-4773(02)00355-6
GTEx Consortium (2020). The GTEx consortium atlas of genetic regulatory effects across human tissues. Sci. (New York, N.Y.) 369 (6509), 1318–1330. doi:10.1126/science.aaz1776
Gusev, A., Ko, A., Shi, H., Bhatia, G., Chung, W., Penninx, B. W. J. H., et al. (2016). Integrative approaches for large-scale transcriptome-wide association studies. Nat. Genet. 48 (3), 245–252. doi:10.1038/ng.3506
Hemani, G., Zheng, J., Benjamin, E., Wade, K. H., Haberland, V., Baird, D., et al. (2018). The MR-base platform supports systematic causal inference across the human phenome. eLife 7, e34408. doi:10.7554/eLife.34408
Jilaveanu, L. B., Parisi, F., Barr, M. L., Zito, C. R., Cruz-Munoz, W., Kerbel, R. S., et al. (2015). PLEKHA5 as a biomarker and potential mediator of melanoma brain metastasis. Clin. Cancer Res. Official J. Am. Assoc. Cancer Res. 21 (9), 2138–2147. doi:10.1158/1078-0432.CCR-14-0861
Kanda, S., Ohmuraya, M., Akagawa, H., Horita, S., Yoshida, Y., Kaneko, N., et al. (2020). Deletion in the cobalamin synthetase W domain-containing protein 1 gene is associated with congenital anomalies of the kidney and urinary tract. J. Am. Soc. Nephrol. JASN 31 (1), 139–147. doi:10.1681/ASN.2019040398
Kolle, G., Georgas, K., Holmes, G. P., Little, M. H., and Yamada, T. (2000). CRIM1, a novel gene encoding a cysteine-rich repeat protein, is developmentally regulated and implicated in vertebrate CNS development and organogenesis. Mech. Dev. 90 (2), 181–193. doi:10.1016/s0925-4773(99)00248-8
Kurki, M. I., Karjalainen, J., Palta, P., Sipilä, T. P., Kristiansson, K., Donner, K. M., et al. (2023). FinnGen provides genetic insights from a well-phenotyped isolated population. Nature 613 (7944), 508–518. doi:10.1038/s41586-022-05473-8
Leeuw, C. A. de, Mooij, J. M., Heskes, T., and Posthuma, D. (2015). MAGMA: generalized gene-set analysis of GWAS data. PLoS Comput. Biol. 11 (4), e1004219. doi:10.1371/journal.pcbi.1004219
Lin, J., Zhou, J., Liu, Z., Zeng, R., Wang, L., Li, F., et al. (2023a). Identification of potential drug targets for varicose veins: a mendelian randomization analysis. Front. Cardiovasc. Med. 10, 1126208. doi:10.3389/fcvm.2023.1126208
Lin, J., Zhou, J., and Xu, Y. (2023b). Potential drug targets for multiple sclerosis identified through mendelian randomization analysis. Brain 146 (8), 3364–3372. doi:10.1093/brain/awad070
MAGMA (2022). MAGMA - CNCR. Available at: https://cncr.nl/research/magma/ (Accessed April 11, 2024).
Malgor, R. D., and Labropoulos, N. (2012). Diagnosis and follow-up of varicose veins with duplex ultrasound: how and why? Phlebology 27 (March), 10–15. doi:10.1258/phleb.2011.012s05
Nagamura, Y., Miyazaki, M., Nagano, Y., Yuki, M., Fukami, K., Yanagihara, K., et al. (2021). PLEKHA5 regulates the survival and peritoneal dissemination of diffuse-type gastric carcinoma cells with met gene amplification. Oncogenesis 10 (3), 25. doi:10.1038/s41389-021-00314-1
Nijsten, T., van den Bos, R. R., Goldman, M. P., Kockaert, M. A., Proebstle, T. M., Rabe, E., et al. (2009). Minimally invasive techniques in the treatment of saphenous varicose veins. J. Am. Acad. Dermatology 60 (1), 110–119. doi:10.1016/j.jaad.2008.07.046
Oliveira, Á., de, R., Riera, R., Vasconcelos, V., and Baptista-Silva, J.Cc (2021). Injection sclerotherapy for varicose veins. Cochrane Database Syst. Rev. 12 (12), CD001732. doi:10.1002/14651858.CD001732.pub3
Raffetto, J. D. (2018). Pathophysiology of chronic venous disease and venous ulcers. Surg. Clin. N. Am. 98 (2), 337–347. doi:10.1016/j.suc.2017.11.002
Shah, S., Henry, A., Roselli, C., Lin, H., Sveinbjörnsson, G., Fatemifar, G., et al. (2020). Genome-wide association and mendelian randomisation analysis provide insights into the pathogenesis of heart failure. Nat. Commun. 11 (1), 163. doi:10.1038/s41467-019-13690-5
Smith, D., and Hemani, G. (2014). Mendelian randomization: genetic anchors for causal inference in epidemiological studies. Hum. Mol. Genet. 23 (R1), R89–R98. doi:10.1093/hmg/ddu328
SMR (2022). SMR | Yang Lab. Available at: https://yanglab.westlake.edu.cn/software/smr/#Overview (Accessed April 11, 2024).
Song, Y.-Xi, Sun, J.-Xu, Zhao, J.-H., Yang, Y.-C., Shi, J.-X., Wu, Z.-H., et al. (2017). Non-coding RNAs participate in the regulatory network of CLDN4 via ceRNA mediated miRNA evasion. Nat. Commun. 8 (1), 289. doi:10.1038/s41467-017-00304-1
The 1000 Genomes Project Consortium authors, C., Auton, A., Abecasis, G. R., committee, S., Altshuler, D. M., Durbin, R. M., et al. (2015). A global reference for human genetic variation. Nature 526 (7571), 68–74. doi:10.1038/nature15393
TWAS (2022). TWAS/FUSION. Available at: http://gusevlab.org/projects/fusion/ (Accessed April 11, 2024).
Two Sample (2022). Two sample MR functions and interface to MR base database • TwoSampleMR. Available at: https://mrcieu.github.io/TwoSampleMR/ (Accessed April 11, 2024).
Võsa, U., Claringbould, A., Westra, H.-J., Bonder, M. J., Deelen, P., Zeng, B., et al. (2021). Large-scale cis- and trans-eQTL analyses identify thousands of genetic loci and polygenic scores that regulate blood gene expression. Nat. Genet. 53 (9), 1300–1310. doi:10.1038/s41588-021-00913-z
Wagner, A. H., Coffman, A. C., Ainscough, B. J., Spies, N. C., Skidmore, Z. L., Campbell, K. M., et al. (2016). DGIdb 2.0: mining clinically relevant drug-gene interactions. Nucleic Acids Res. 44 (D1), D1036–D1044. doi:10.1093/nar/gkv1165
Wang, H., Liu, J., Yang, J., Wang, Z., Zhang, Z., Peng, J., et al. (2022). A novel tumor mutational burden-based risk model predicts prognosis and correlates with immune infiltration in ovarian cancer. Front. Immunol. 13, 943389. doi:10.3389/fimmu.2022.943389
Wang, Yu, Liu, F., Sun, L., Jia, Y., Yang, P., Guo, D., et al. (2023). Association between human blood metabolome and the risk of breast cancer. Breast Cancer Res. 25 (1), 9. doi:10.1186/s13058-023-01609-4
Yang, Q., Li, Bo, Chen, S., Tang, J., Li, Y., Yi, Li, et al. (2021). MMEASE: online meta-analysis of metabolomic data by enhanced metabolite annotation, marker selection and enrichment analysis. J. Proteomics 232 (February), 104023. doi:10.1016/j.jprot.2020.104023
Yang, Q., Li, Bo, Wang, P., Xie, J., Feng, Y., Liu, Z., et al. (2022). LargeMetabo: an out-of-the-Box tool for processing and analyzing large-scale metabolomic data. Briefings Bioinforma. 23 (6), bbac455. doi:10.1093/bib/bbac455
You, X., Yang, S., Sui, J., Wu, W., Liu, T., Xu, S., et al. (2018). Molecular characterization of papillary thyroid carcinoma: a potential three-lncRNA prognostic signature. Cancer Manag. Res. 10, 4297–4310. doi:10.2147/CMAR.S174874
Youn, Y. J., and Lee, J. (2019). Chronic venous insufficiency and varicose veins of the lower extremities. Korean J. Intern. Med. 34 (2), 269–283. doi:10.3904/kjim.2018.230
Zhao, X., Bai, Z., Li, C., Sheng, C., and Li, H. (2020). Identification of a novel eight-lncRNA prognostic signature for HBV-HCC and analysis of their functions based on coexpression and ceRNA networks. BioMed Res. Int. 2020, 8765461. doi:10.1155/2020/8765461
Zhu, Z., Zhang, F., Hu, H., Bakshi, A., Robinson, Mr, Powell, Je, et al. (2016). Integration of summary data from GWAS and eQTL studies predicts complex trait gene targets. Nat. Genet. 48 (5), 481–487. doi:10.1038/ng.3538
Keywords: varicose veins, drug targets, Mendelian randomization analysis, colocalization analysis, genome-wide association studies, expression quantitative trait locus
Citation: Cui Y, Hu M, Zhou H, Guo J, Wang Q, Xu Z, Chen L, Zhang W and Tang S (2024) Identifying potential drug targets for varicose veins through integration of GWAS and eQTL summary data. Front. Genet. 15:1385293. doi: 10.3389/fgene.2024.1385293
Received: 12 February 2024; Accepted: 17 April 2024;
Published: 15 May 2024.
Edited by:
Yufei Zheng, Sir Run Run Shaw Hospital, ChinaReviewed by:
Qingxia Yang, Nanjing University of Posts and Telecommunications, ChinaShi Xue Dai, Guangdong Provincial People’s Hospital, China
Copyright © 2024 Cui, Hu, Zhou, Guo, Wang, Xu, Chen, Zhang and Tang. This is an open-access article distributed under the terms of the Creative Commons Attribution License (CC BY). The use, distribution or reproduction in other forums is permitted, provided the original author(s) and the copyright owner(s) are credited and that the original publication in this journal is cited, in accordance with accepted academic practice. No use, distribution or reproduction is permitted which does not comply with these terms.
*Correspondence: Wancong Zhang, martine2007@sina.com; Shijie Tang, sjtang3@stu.edu.cn
†These authors have contributed equally to this work and share first authorship