- 1Institute of Basic Medical Sciences (IBMS), Khyber Medical University, Peshawar, Pakistan
- 2Institute of Pathology and Diagnostic Medicine (IPDM), Khyber Medical University, Peshawar, Pakistan
- 3College of Medicine, Gulf Medical University, Ajman, United Arab Emirates
- 4School of Medicine, University of Glasgow, Glasgow, United Kingdom
- 5Department of Pathology, Lady Reading Hospital Medical Teaching Institution, Peshawar, Pakistan
- 6Department of Surgery, Khyber Teaching Hospital, Medical Teaching Institution, Peshawar, Pakistan
- 7Alpha Genomics (Private) Limited, Islamabad, Pakistan
- 8Microbiological Analysis Team, Group for Biometrology, The Korea Research Institute of Standards and Science (KRISS), Daejeon, Republic of Korea
- 9Department of Pathology, College of Medicine, Qassim University, Unaizah, Saudi Arabia
- 10Martin-Luther-Universität Halle-Wittenberg, Halle, Germany
In this study, we report the mutational profiles, pathogenicity, and their association with different clinicopathologic and sociogenetic factors in patients with Pashtun ethnicity for the first time. A total of 19 FFPE blocks of invasive ductal carcinoma (IDC) from the Breast Cancer (BC) tissue and 6 normal FFPE blocks were analyzed by whole-exome sequencing (WES). Various somatic and germline mutations were identified in cancer-related genes, i.e., ATM, CHEK2, PALB2, and XRCC2. Among a total of 18 mutations, 14 mutations were somatic and 4 were germline. The ATM gene exhibited the maximum number of mutations (11/18), followed by CHEK2 (3/18), PALB2 (3/18), and XRCC2 (1/18). Except one frameshift deletion, all other 17 mutations were nonsynonymous single-nucleotide variants (SNVs). SIFT prediction revealed 7/18 (38.8%) mutations as deleterious. PolyPhen-2 and MutationTaster identified 5/18 (27.7%) mutations as probably damaging and 10/18 (55.5%) mutations as disease-causing, respectively. Mutations like PALB2 p.Q559R (6/19; 31.5%), XRCC2 p.R188H (5/19; 26.31%), and ATM p.D1853N (4/19; 21.05%) were recurrent mutations and proposed to have a biomarker potential. The protein network prediction was performed using GeneMANIA and STRING. ISPRED-SEQ indicated three interaction site mutations which were further used for molecular dynamic simulation. An average increase in the radius of gyration was observed in all three mutated proteins revealing their perturbed folding behavior. Obtained SNVs were further correlated with various parameters related to the clinicopathological status of the tumors. Three mutation positions (ATM p. D1853N, CHEK2 p.M314I, and PALB2 p.T1029S) were found to be highly conserved. Finally, the wild- and mutant-type proteins were screened for two drugs: elagolix (DrugBank ID: DB11979) and LTS0102038 (a triterpenoid, isolated from the anticancer medicinal plant Fagonia indica). Comparatively, a higher number of interactions were noted for normal ATM with both compounds, as compared to mutants.
Introduction
Breast cancer (BC) has emerged as the second foremost cause of mortalities related to cancers in women (Liu and Hu, 2023). Approximately 2.3 million cases of BC were reported in 2020 with 685,000 deaths. It is expected that by 2040, the BC incidence rates can increase up to 33.8% (Vidra et al., 2022). Statistically, 1 in 8 women generally have a lifetime risk of BC development (Dutta et al., 2023). Initially, BC was proposed as a single disease originating in the mammary gland. However, it is now established that BC is a complex disease with inter-tumor heterogeneity, and the heterogeneous nature has a significant impact on the progression of the disease and its treatment (Liu et al., 2022). Although the incidence of BC is on the rise all across the world, the mortalities and survival rates vary in different regions, which are attributed to changes in risk factors, hormonal profiles, environmental conditions, access to and standards of healthcare, and genetic features (Momenimovahed and Salehiniya, 2019). The survival rates in patients with BC are relatively higher in developed countries, as compared to LMIC (Ma and Jemal, 2013), and the general trend indicates that the BC malignancy is rapidly rising in low- and middle-income countries (Mubarik et al., 2023). Pakistan is among the countries with a higher incidence of BC among Asian countries, and one-ninth of women have the potential risk of developing breast cancer during their lifetime (Khan et al., 2021). According to the international report of the International Agency for Research on Cancer (IARC), in the year 2020, 25,928 (28.7%) new cases of BC in women were diagnosed in Pakistan with a cumulative death count of 13,725 (IARC International Agency for Resaerch on Cancer, 2020). Some reports have suggested an annual incidence of 90,000 cases/year annually with ∼16,000 deaths/year (Khaliq et al., 2019). The situation regarding BC is expected to further worsen as it is estimated that by 2030, the annual incidence of BC may rise up to 62% in Pakistan, with middle-aged patients being most vulnerable (Rubi et al., 2022). Previous reports have revealed that ∼ 89% of BC patients are diagnosed at a later stage due to the lack of awareness (Gulzar et al., 2019). With scarce resources, the lack of adequate screening points, financial constraints, lack of awareness, structural barriers, and a combination of various socio-economic factors like stigmatization, feminine sensitivity, and reluctance to visit male doctors are major challenges in the context of BC prevention and management (Sarwar et al., 2018; Khaliq et al., 2019).
Genetic anomalies and mutations are the hallmarks of cancer progression (Bao et al., 2019). The exploration of the oncogenes that are abnormally expressed in the progression of BC is, therefore, critical to develop effective BC therapeutic regimens by understanding the mechanistic aspects and propose evidence-based management strategies. The progression of BC is related to the sequence of mutations (somatic and germline) that eventually causes abnormal cell cycle, angiogenesis and apoptotic suppression, and abnormal cell proliferation that eventually ends up in a full-fledged malignancy (Economopoulou et al., 2015). Recently, the oncogenomic research has focused on the characterization of the driver somatic and germline mutations and reflects the association with various clinical phenotypes with an aim of strengthening the therapeutic regime selection for better treatment response (Mathioudaki et al., 2020).
Pathogenic variants of ATM (ataxia-telangiectasia mutated), CHEK2 (checkpoint kinase 2), PALB2 (partner and localizer of BRCA2), and XRCC2 (X-ray repair cross-complementing 2) tumor suppressor genes (TSGs) have been associated with the development of cancers (Moslemi et al., 2021). Mutations in these genes are known to compromise their tumor suppression functions, which eventually cause cancerous conditions.
Previously, we reported the mutational signatures in PTEN, PIK3CA, and TP53 in Pashtun ethnicity patients from KP (Ahmad et al., 2023). To date, no studies have been conducted on breast cancer-driven genes such as ATM, PALB2, CHEK2, and XRCC2 reported from the Khyber Pakhtunkhwa region or Pashtun ethnicity and their potential associations with the various clinicopathologic and hormonal characteristics. A total of 19 confirmed BC patients with IDC and 6 paired adjacent normal tissues were used for NGS-WES. These mutations were analyzed using various in silico tools (MutationTaster, PolyPhen-2, SIFT, SAAFEQ-SEQ, ISPRED-SEQ, ConSurf, cBioPortal, PyMOL, etc.). Molecular dynamic simulations were carried out for selected wild and mutant proteins. Furthermore, we further applied molecular docking methods to study protein (wild and mutant)–drug interactions, which were visualized in BioDiscovery.
Materials and methods
Participants' enrollment and data collection
Patients with BC diagnosed with invasive ductal carcinoma (IDC) were enrolled in the study after obtaining informed consent from the patients/guardian. Formalin-fixed paraffin-embedded (FFPE) blocks of tumor with at least 70% tumor purity were included. Patients with secondary tumors were excluded. The enrolled patients were from major tertiary care hospitals (KTH-MTI and HMC-MTI). All participants were briefed on the aims and objectives of the research. Written informed consent was obtained, and their medical history was noted.
Ethical approval
This study was approved by the Research Ethics Committee of Khyber Medical University, Peshawar vide: DIR/KMU-EB/VA/000651. All steps were performed according to the principles of the Helsinki Declaration.
Collection of samples
Breast tumor biopsy samples were collected in 10% formalin and subsequently transferred to Histopathology Laboratory at KMU, Peshawar. Cancer Reporting Protocols and Guidelines, as described by the College of American Pathologists, were used for gross examination and reporting. A structured proforma was used to collect data regarding the color, general appearance, and consistency. Formalin-fixed paraffin-embedded (FFPE) tissue blocks were prepared in embedding cassettes, which were labeled accordingly. Rotary microtome was used for making 5-µm sections, which were placed on the glass slides and deparaffinized in xylene. H&E stain was applied for the assessment of IDC and immunohistochemical markers, i.e., ER (ERα ISO8430), PR (PR ISO6830), Her2/neu (Her2; A048529), and Ki-67 (IS 62630), for further assessment and characterization. Only the FFPE blocks having enriched tumor cell populations (at least 70%) confirmed using the florescence microscope were included in the study. Normal FFPE tissue blocks were taken from the adjacent normal tissues located at least 2 cm away from the site of the tumor (Li et al., 2018).
Whole-exome sequencing (NGS-WES)
A total of 19 tumor rich FFPE blocks and 6 normal tissue FFPE blocks were sent for commercial next-generation whole-exome sequencing to Macrogen (Korea). The whole-exome sequence data were obtained using the Illumina Hi-Seq NGS platform with 151-bp paired-end reads. The SureSelect V6-(FFPE) reagent kit was used for the construction of library.
Preparation of the sample and library
After the extraction of the genomic DNA, 1% agarose gel was used for QC on gel electrophoresis (30 min at 160V) after adding genomic DNA (10 µL). Samples with sufficient quantity, complemented by good bands on gel, were considered for the preparation of the library. First, random fragmentation was used for library preparation, which was followed by 5′- and 3′-end adapter ligation. The obtained fragments were amplified by PCR and gel-purified. Fluorescent quantification was used to quantify the genomic DNA.
Whole-exome sequencing
The whole-exome sequencing data were obtained through Illumina Hi-Seq with reversible terminator-based technology for detecting single bases which are incorporated into DNA template strands. The raw files were supplied in the form of FASTQ format.
Sequence analysis
The FASTQ files were assessed for QC by submitting these files to Babraham Bioinformatics (https://www.bioinformatics.babraham.ac.uk/projects/fastqc/). The sequence filers were aligned with human reference genome (hg38-UCSC) by the BWA (Burrows–Wheeler aligner) tool (Li and Durbin, 2010). The alignment files were then converted to BAM files using SAMtools and were further examined using the SAM mpileup tool. BCF tools were used for obtaining the Variant Call Format (VCF) file, which was submitted to the ANNOVAR program obtaining .csv files. RStudio was used for identifying various kinds of mutations such as single-nucleotide variants (SNVs), indels (insertion deletion), stop gain, and frameshift. The obtained mutations were then assessed through databases like MutationTaster, PolyPhen-2, and SIFT for characterizing the nature of the mutations. Later, these mutations were studied within the context of potential clinicopathologic factors and other social determinants.
Bioinformatics-based predictions
The pathogenicity of the mutations was determined using the three most commonly used databases, i.e., MutationTaster, SIFT, and PolyPhen-2. The SAAFEQ-SEQ tool was used to determine the destabilizing SNVs (http://compbio.clemson.edu/lab/). Interaction site (IS) mutations were predicted using ISPRED-SEQ (https://ispredws.biocomp.unibo.it/sequence/). The evolutionary conservation scores were determined using the ConSurf web server (https://consurf.tau.ac.il/). The ConSurf web server includes an empirical Bayesian algorithm for conservation scores ranging from 1 to 9. The exposed and buried nature, as well as the functional and structural nature, was predicted. PROCHECK was used to generate Ramachandran plots.
Mutation mapping and modeling
The lollipop plots were produced in the cBioPortal database by uploading the amino acid sequence, as discussed previously (Gao et al., 2013). The 3D structure of the mutant proteins ATM, CHEK2, PALB2, and XRCC2 was visualized using PyMOL.
Molecular dynamic simulations
GROMACS package 4.5 was used for MDS simulations for only selected interaction site mutations, as predicted through ISPRED-SEQ, and the results of the various parameters, particularly the radius of gyration (Rg) and root mean square deviation (RMSD), were compared for the wild-type and mutant proteins. The water molecules were removed, and the OPLS-AA/L all-atom force field was selected. Solvation was performed using an equilibrated three-point solvent model, i.e., spc216.gro. grompp was used to assemble the binary input file. The MDS was initiated with parameters like radius of gyration, RMSD, pressure, temperature, density, and potential.
Molecular docking
Molecular docking of the proteins and their mutated forms were done with compounds from DrugBank (Wishart et al., 2018) and metabolites/compounds of F. indica. Estradiol benzoate (DrugBank ID: DB13953), estradiol valerate (DrugBank ID) elagolix (DrugBank ID: DB11979), and ketorolac (DrugBank ID: DB00465) implicated in either cancer or menstrual issues were obtained from DrugBank, while 16 compounds were obtained from the LOTUS database (https://lotus.naturalproducts.net/search/simple/Fagonia%20indica; retrieved 18 September 2023) for F. indica. The best scoring DB11979 and LTS0102038 against ATM, PALB2, CHEK2, and XRCC2 proteins were used for docking and comparative analysis. Pocket identification was done using fpocket (https://github.com/Discngine/fpocket; retrieved 18 September 2023) (Le Guilloux et al., 2009), and docking was conducted using AutoDock Vina (Trott and Olson, 2010) deployed in BioExcel (Bayarri et al., 2022). Only one pocket was selected with the radius of 3–6 Å, volume range of 100–2000 ų, and box offset of 12 Å. Affinities were recorded, and interacting residues were noted. Results of the docking were visualized in the BIOVIA Discovery Studio Visualizer (Biovia et al., 2000).
Results
Mutational landscape
Results from the WES data revealed a total of 18 mutations spanning across ATM, CHEK2, PALB2, and XRCC2 genes (Table 1). It was observed that 8/18 (44%) of these mutations were reported for the first time (Figure 1A). The frequency of mutations was highest in the ATM gene, i.e., 11/18 (61.1%). Mutations in CHEK2, PALB2, and XRCC2 were found to be 3/18 (16.6%), 3/18 (16.6%), and 1/18 (5.5%), respectively, as depicted in Figure 1B. The inset in Figures 2A–C indicates further characterization of the obtained mutations. The total number of novel mutations in ATM was found to be 5/11 (45.5%). The novel reported mutations of the ATM gene were identified as ATM p.V1070Efs*38, p.M1760I, p.W266R, p.S1403R, and p.H1568N. For CHEK2, out of the three mutations, CHEK2 p.M314I was identified as novel, whereas two mutations PALB2 p.I1013K and p.T1029S were reported to be novel. Figure 2B reveals the number of germline and somatic mutations across ATM, CHEK2, PALB2 and XRCC2. A total of 4/18 (22.2%) mutations were germline, and the rest, i.e., 14/18 (77.7%), were found to be somatic mutations. In the ATM gene, 2/11 (18.18%) mutations were found to be germline mutations and 9/11 (81.81%) were somatic mutations. For CHEK2, three somatic mutations (CHEK2 p.R180C, p.M314I, and p.W114L) were identified, while for PALB2, 2/3 mutations were somatic mutations (PALB2 p.T1029S and PALB2 p.I1013K) and 1/3 (PALB2 p.Q559R) was germline mutation. For XRCC2, the only one identified mutation was germline, i.e., XRCC2 p.R188H. Figure 2C reveals the nature of the mutations. The major chunk of the mutations were nonsynonymous SNVs (17/18; 94.4%), whereas only single frameshift deletion was identified in the ATM gene, i.e., ATM p.V1070Efs*38.
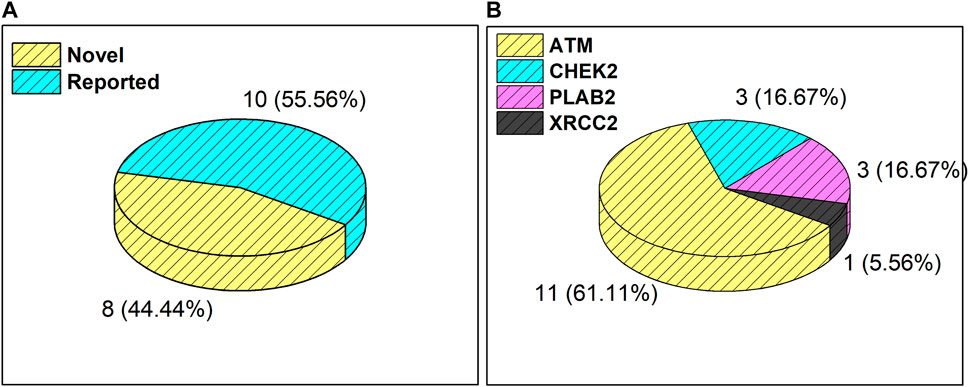
Figure 1. (A) Total number and percentage of reported and novel mutations. (B) Breakdown of total mutations in ATM, CHEK2, PALB2, and XRCC2 genes.
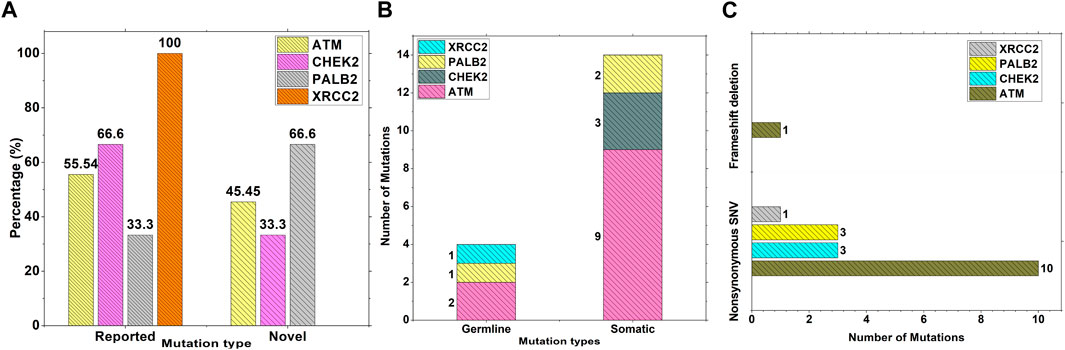
Figure 2. Characterization and nature of mutations on ATM, CHEK2, PALB2, and XRCC2 cancer-driver genes: (A) Percentage of novel and reported mutations; (B) number of somatic and germline mutations; and (C) mutation types.
The inset in Figures 3A–C reveals the overall prediction of the obtained mutations which were assessed using different databases like SIFT, PolyPhen-2, and MutationTaster. Overall, 7/18 (38.8%) predications were made by SIFT as deleterious, while 1/18 mutations, i.e., ATM p.V1070Efs*38, have no prediction SIFT, PolyPhen-2 and Mutation Taster. The prediction from PolyPhen-2 databases revealed 5/18 (27.7%) and 3/18 (16.6%) mutations as probably damaging and possibly damaging, respectively. The MutationTaster database revealed that 10/18 (55%) mutations is disease-causing. Further predictions related to the mutation pathogenicity such as FATHMM and PROVEAN are supplied, as depicted in Supplementary Table S2.
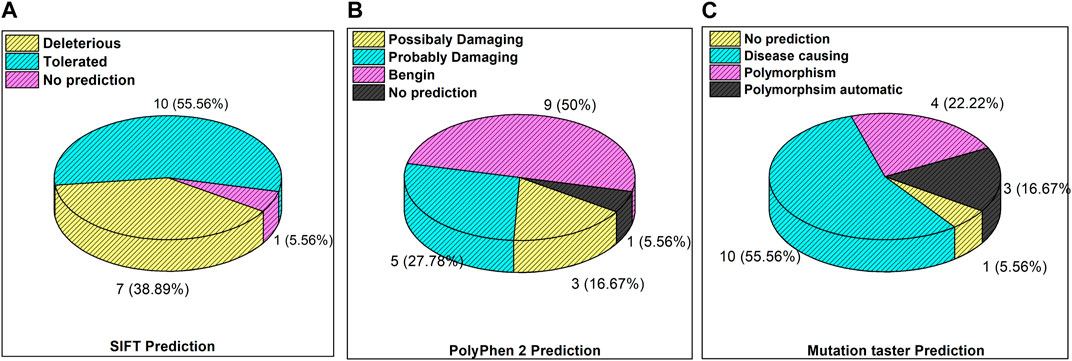
Figure 3. Mutation predictions from different databases in the enrolled cohort: (A) SIFT prediction; (B) PolyPhen-2 prediction; and (C) MutationTaster prediction.
Supplementary Figure S4 (A–C) depicts the gene wise prediction of the pathogenic mutations. The SIFT prediction for ATM, CHEK2, PALB2 revealed a total of 4/11, 2/3 and 1/3 mutations as deleterious respectively. The Poly–Phen 2 prediction for ATM revealed 4/11 as possibly damaging and 6/11 benign mutations. For PALB2, 2/3 mutations were identified as possibly damaging. Mutation taster revealed 6/11, 3/3 and 1/3 disease causing mutations in ATM, CHEK2 and PALB2 respectively.
Supplementary Figures S5A–D indicate the distribution of mutations on exons. For ATM, two mutations each were found to be located on exon 22, exon 28, and exon 37. In CHEK2, one mutation each was identified on exon 3, exon 4, and exon 10, whereas, for PALB2, two mutations were found on exon 10 and 1 mutation on exon 4. For XRCC2, only one mutation was identified located on exon 3. The lollipop plot acquired from the cBioPortal is depicted in Figures 4A–D, which shows the frequency of mutations in the enrolled cohort. Mutations like PALB2 p.Q559R (6/19; 31.5%), XRCC2 p.R188H (5/19; 26.31%), and ATM p.D1853N (4/19; 21.05%) were found to be recurring in the enrolled cohort (Supplementary Figure S7) and hence have a biomarker potential.
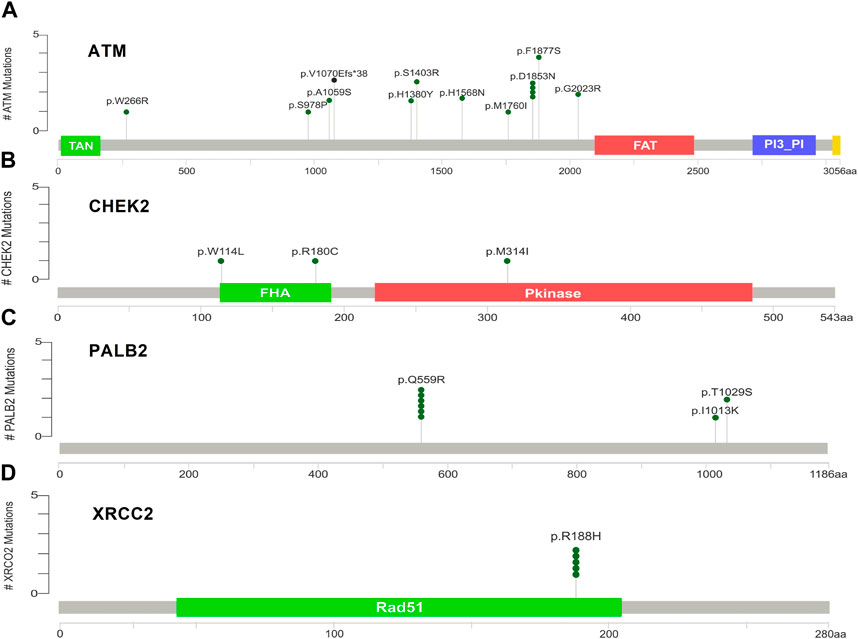
Figure 4. Lollipop plot of mutations on (A) ATM; (B) CHEK2; (C) PALB2; and (D) XRCC2. cBioPortal was used to obtain these plots.
Table 2 reveals the SAAFEQ-SEQ predictions that relate to the effect of the SNVs on protein stability. The algorithm is based on various parameters that compute the change in stability-free energy resulting from SNVs. The destabilizing effect was predicted for all obtained SNVs. To further scrutinize these mutations, we applied the ISPRED-SEQ tool for identifying only the interaction site (IS) mutations, and the results are summarized in Table 3. Only three IS mutations were identified one each on ATM p.G2023R, PALB2 p.Q559R, and XRCC2 p.R188H, whereas no IS mutations were identified on CHECK2. The IS mutations were superimposed and visualized in PyMOL, as depicted in Figures 5A–C, and later further evaluated for MDS simulation to identify the differences in the wild-type and mutant proteins in terms of various parameters, especially radius of gyration (Rg) and root mean square deviation (RMSD), as revealed in Supplementary Figures S9A–H, Supplementary Figures S10A–H, and Supplementary Figures S11A–H.
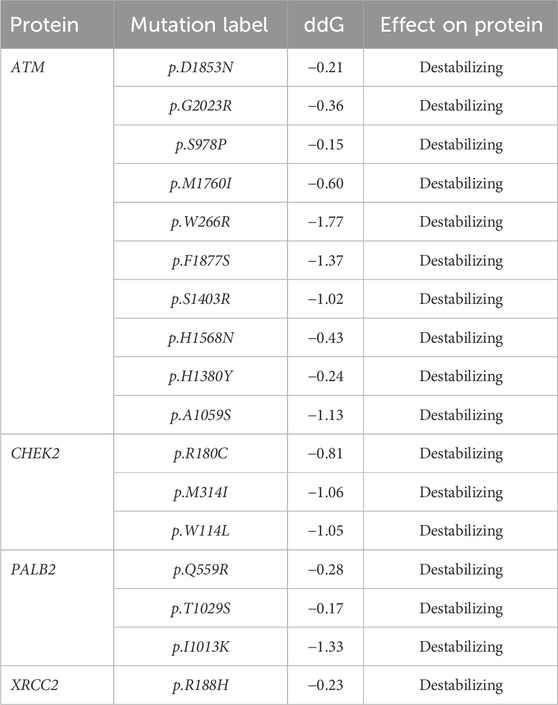
Table 2. SAAFEC-SEQ predictions for protein stability (https://ispredws.biocomp.unibo.it/sequence/).
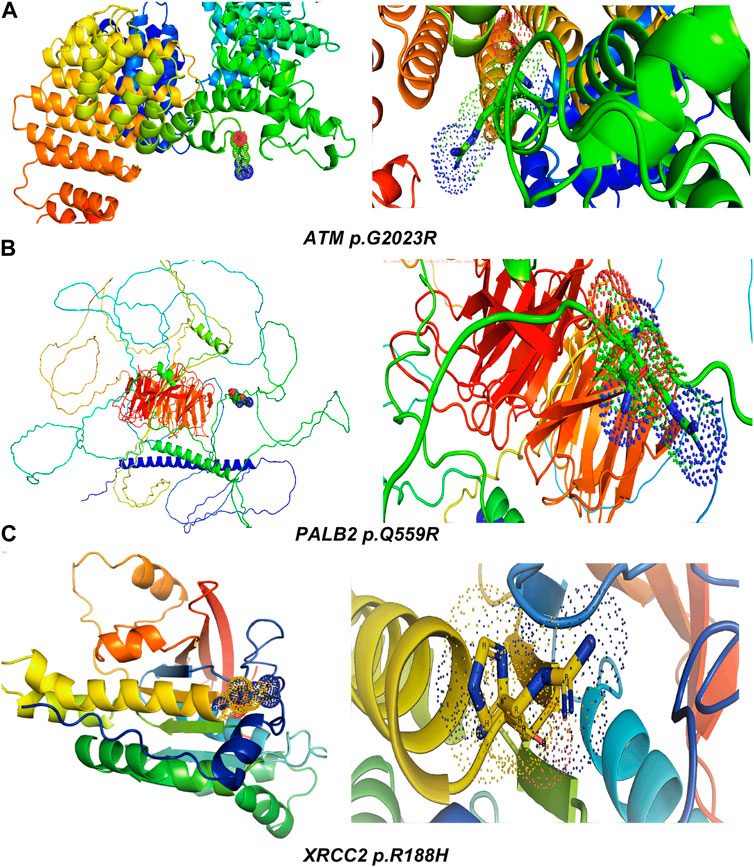
Figure 5. Visualization and superimposition of interaction site mutations in PyMOL: (A) ATM p.G2023R; (B) PALB2 p.Q559R; (C) XRCC2 p.R188H.
The MDS simulation results of ATM p.G2023R revealed an average Rg of 3.126 nm and 3.36 nm for the wild and mutant type, respectively. Similarly, the average Rg for PALB2 p.Q559R was reported to be 3.79 nm (wild type) and 4.16 nm (mutant type), whereas for XRCC2 p.R188H, the average Rg was recorded as 1.65 nm (wild) and 1.713 nm (mutant), as depicted in Figure 6. RMSD values were calculated for the normal and mutant proteins for determining the overall changes to the stability of protein. For ATM p.G2023R, the RMSD simulation revealed structural differences starting from ∼0.4 ns. Across the simulation, the wild-type and mutant ATM showed minor and major deviations. Similarly, for PALB2 p.Q559R, the structural deviations were observed, which started approximately ∼ 1.3 ns and remained deviated along the course of trajectory. For XRCC2 p.R188H, major structural deviations were noted during the simulation, which started around 1.4 ns. Other MDS simulation results are depicted in the inset in Supplementary Figures S9A–H, Supplementary Figures S10A–H, and Supplementary Figures S11A–H, whereas the corresponding Ramachandran plots for the wild-type and mutant proteins are also indicated. The major difference was observed only in ATM p.G2023R, in which the residues in the favorable region for the wild type (93.4%) were decreased (93.2%) in the mutant type. For PALB2 p.Q559R and XRCC2 p.R188H, no differences were observed.
Table 4 summarizes the results obtained from the ConSurf tool, which relates to the evolutionary conservation of the residue position based on its structural and functional importance. Among the obtained SNVs, three mutations, i.e., ATM p.D1853N, CHEK2 p.M314I, and PALB2 p.T1029S were found to be highly conserved (ConSurf score: 08), and among them, ATM p.D1853N was of functional importance. ATM p.S978P, ATM p.G2023R, and PALB2 p.I1013K were also identified as a conserved position (ConSurf score: 07).
Clinicopathologic association
The inset in Supplementary Figures S12A,B further characterizes the specific ATM mutations. The mutation ATM p.D1853N was found to be present in grade 2 and grade 3 tumors. ATM p.S978P was the only ATM mutation present in Her2+, while all the rest of the mutations were only present in Her2−. Three ATM mutations (ATM p.H1380Y, p.S1403R, and p.H1568N) were found to be associated only with triple negative molecular subtypes. The inset in Supplementary Figures S13A–C summarizes the immunohistochemical association of the mutations in CHEK2, PALB2, and XRCC2. It is evident from the results that CHEK2 p.R180C, p.M314I, and p.W114L were not identified in ER− and Her2+. CHEK2 p.R180C was found only in PR+, and the rest CHEK2 p.M314I and p.W114L were only found in PR−. PALB2 p.Q559R was found to be distributed in all major categories, i.e., ER+, ER−, PR+, PR−, Her2+, and Her2, as depicted in Supplementary Figures S13A–C. Similarly, PALB2 p.Q559R was found to be associated with Her+, triple negative, and Luminal A and Luminal B molecular subtypes. The same mutation, i.e., PALB2 p.Q559R, was found both in grade 2 and grade 3 tumors, as depicted in Supplementary Figure S14B. The PALB2 p.I1013K mutation was only found in the triple-negative breast cancer patients, as shown in Supplementary Figure S14B. XRCC2 p.R188H was found in grade 2 and grade 3 tumors. Similarly, XRCC2 p.R188H was found in ER+, ER−, PR+, PR−, Her2+, and Her2− patients and was also identified in Luminal A, Luminal B, and triple-negative molecular subtypes.
Docking of ATM, CHEK2, PALB2, and XRCC2 mutants
Molecular docking was done for both the normal and mutated proteins with an FDA-approved drug elagolix (DrugBank databse ID: DB11979) and a triterpenoid saponin (IUPAC name: (2S,3R,4S,5S,6R)-3,4,5-trihydroxy-6-(hydroxymethyl)oxan-2-yl (1S,2R,4aS,6aS,6bR,8aR,10S,12aR,12bR,14bS)-10-{[(2S,3R,4S, 5S)-5-hydroxy-3 {[(2S,3R,4S,5S,6R)-3,4,5-trihydroxy-6-(hydroxymethyl)oxan-2-yl]oxy}-4-{[(2S,3R,4S,5S) 3,4,5-trihydroxy oxan-2-yl]oxy}oxan-2-yl]oxy}-1,2,6a,6b,9,9,12a-heptamethyl 1,2,3,4,4a,5,6,6a,6b,7,8,8a,9,10,11,12,12a,12b,13,14b-icosahydropicene-4a-carboxylate) (LOTUS database ID: LTS0102038) from F. indica for comparison (Table 5). A higher number of interactions were observed for normal ATM with both compounds, compared to mutants. Around 14 residues were conserved in an interaction with normal proteins, while the residues were themselves changed for a mutant interaction compared to the normal protein. Overall, the binding affinity was better for the F1877S mutant of ATM, compared to the normal protein. The binding affinity of these compounds was good for normal ATM p.G2023R but low for the mutant ATM p.G2023R. The ATM p.W266R did not show good affinity in the normal or mutated state (ATM p.W266R), as well as XRCC p.R188H. PALB2 showed good binding affinity in both normal and mutated states. ATM mutants and PALB2 normal and mutants, showing good binding affinities for the studied compounds may be explored further through other in silico, in vitro, and in vivo assays.
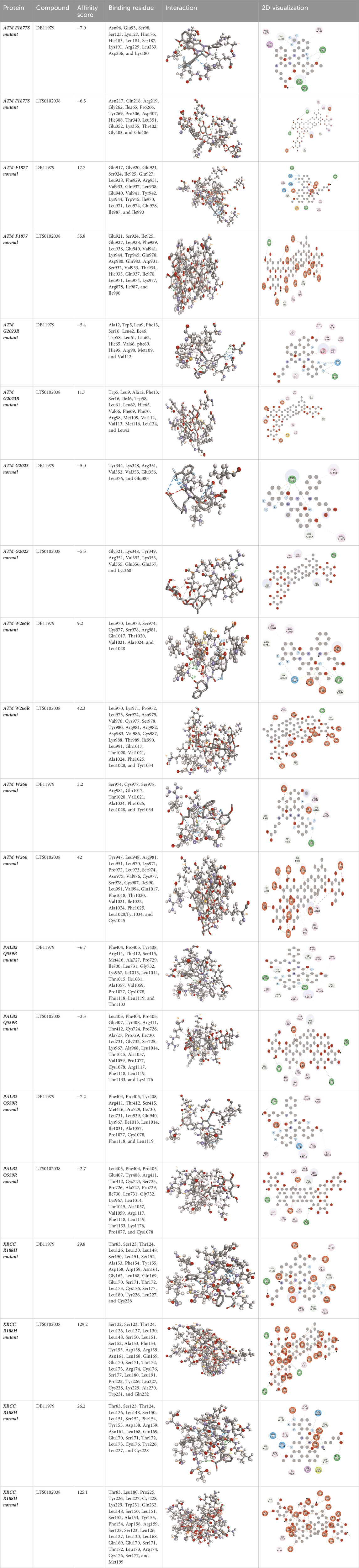
Table 5. Details of the interactions of proteins and their mutants with DB11979 and LTS0102038. Histidine with hydrogen on epsilon nitrogen is mentioned as Hie.
Discussion
Whole-exome sequencing is now considered an important tool for screening the somatic and germline mutations, which are of particular interest in cancer. Pathogenic mutations in cancer-driven genes like ATM, CHEK2, PALB2, and XRCC2 have been reported to increase the risk of different malignancies, especially breast and prostate cancers. These candidate genes have been involved in the plethora of cellular functions related to response to DNA damage, cell cycle, cell growth, etc. The PPI network was retrieved through GeneMANIA and STRING server for ATM, CHEK2, PALB2, and XRCC2, as depicted in Figures 7A–H. These networks show that the candidate genes are involved intricate networks related to cellular repair mechanisms.
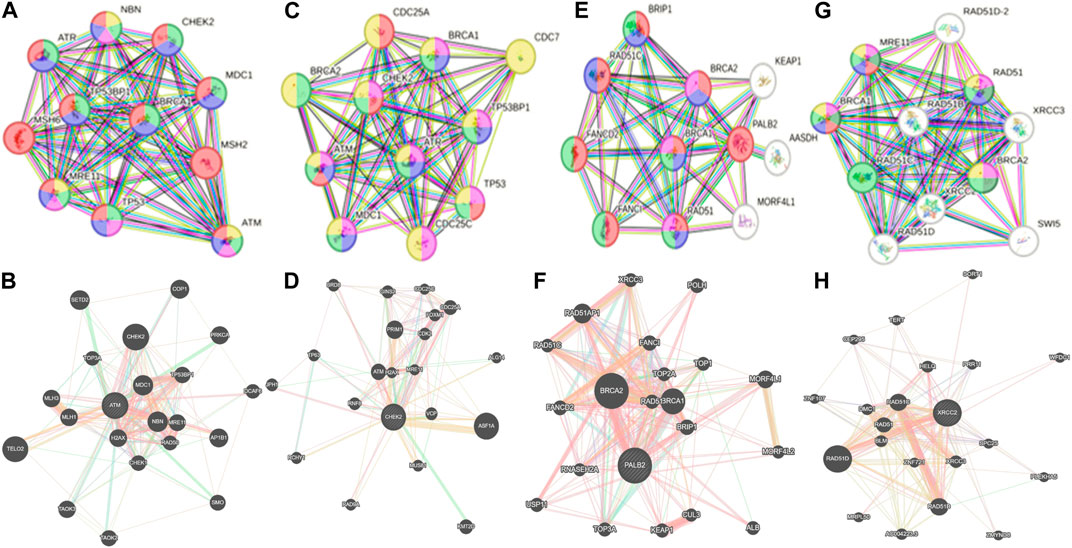
Figure 7. Various interaction networks of candidate genes retrieved from STRING and GeneMANIA; (A,B) ATM; (C,D) CHEK2; (E,F) PALB2, and (G,H) XRCC2.
In the current study, we have used the NG-WES for identifying the frequency of mutations and mutational landscape. It is the first report on characterizing the mutational landscape of BC patients from Khyber Pakhtunkhwa in a specifically enrolled cohort with Pashtun ethnicity. Furthermore, we have assessed the mutational spectrum in the context of their association with various hormonal, non-hormonal, and clinicopathologic features.
Ataxia-telangiectasia mutated (ATM) is an oncosuppressor gene which codes for a 350-KDa protein with 3,056 amino acids that perform the function of activating DNA repair pathways. The ATM gene plays different roles in cellular processes like energy production, oxidative homeostasis, telomere maintenance, chromatin remodeling, and genomic integrity, which are considered crucial processes in the context of cancer progression (Phan and Rezaeian, 2021). ATM mutations have been detected in up to 40% of BC patients (Stucci et al., 2021). ATM mutations are frequently reported in the development of BC. Approximately 5% solid tumors are reported with ATM aberrations (Stucci et al., 2021). Structurally, the ATM gene comprises four major domains including the spiral domain (1–1160), pincer domain (1161–1890); FAT domain (1903–2612), and kinase domain (2618–3056) (Ueno et al., 2022). We reported three mutations in the ATM spiral domain (residues 1–1161), which plays a potential role in the binding adaptors, regulators, and substrates (Baretić et al., 2017). Six mutations are reported in the pincer domain of ATM. We have reported one mutation ATM p.G2023R in the FAT domain, and such mutations have the potential to increase the risk of breast cancer (Cavaciuti et al., 2005).
In our results, various ATM mutations have been reported previously. One of them, i.e., ATM p.D1853N, has been previously reported; however, it is considered of least predictive value and has a weak association with developing breast cancer (Moslemi et al., 2021; Schrauder et al., 2008). ATM p.G2023R has been previously reported in breast cancer patients from Brazil; however, we obtained the same mutation on exon 41, whereas, in the Brazilian cohort, the same mutation was reported on exon 42 (Mangone et al., 2015). ATM p.S978P has been reported to have implications in pancreatic cancer (Ding et al., 2021).
CHEK2 is well-characterized for playing an important role in cell cycle regulation and apoptosis after the cells are exposed to DNA damage. CHEK2 is activated by ATM, and its activation triggers a downstream cascade of cellular events that ensure genomic integrity and DNA repair (Boonen et al., 2022). Mutations in CHEK2 have been reported to have a moderate risk of BC. We have obtained three mutations in CHEK2, i.e., CHEK2 p.W114L, p.R180C, and p.M314I, in which the first two mutations are located in the region referred to as forkhead-associated domain (FHA) located approximately from 113 to 180 residues, whereas CHEK2 p.M314I is located in the kinase domain that further activates the downstream effector proteins (Stubbins et al., 2022). The FHA domain is responsible for CHEK2 dimerization in a phosphorylation-dependent manner (Durocher and Jackson, 2002), which is considered important for full activation of CHEK2 by trans-phosphorylation within the kinase domain (Kleibl et al., 2008). Mutations in these regions can compromise the functional properties of CHEK2, eventually, leading to the BC. The CHEK2 mutations have previously been reported in breast cancer. CHEK2 p.R180C has been reported in breast cancer of familial nature in German and Jewish ethnicity (Dufault et al., 2004). CHEK2 p.R180C mutations have also been reported in Chinese and Malay (Mohamad et al., 2015).
PALB2 (partner and localizer of BRCA2) is considered a high-risk gene in breast cancer, and it encodes for a protein with tumor suppressor activity. Its pathogenic variants have the risk of 30%–60% of developing BC in women (Nepomuceno et al., 2021). The PALB2-encoded protein binds and colocalizes with BRCA2 and forms a BRCA1–PALB2–BRCA2 complex. The major function of PALB2 is to associate with BRCA2 for maintaining the genomic integrity and preventing the accumulation of DNA mutations (Evans and Longo, 2014). Our results revealed three PALB2 mutations, i.e., PALB2 p.Q559R, p.I1013K, and p.T1029S in the enrolled cohort. PALB2 p.Q559R was reported previously in the Italian cohort of breast cancer patients with comparatively higher penetrance (Silvestri et al., 2010), which correlates with our findings for the Pashtun ethnicity.
XRCC2 (X-ray repair cross-complementing) is another gene with cancer predisposition. The XRCC2 protein product plays a role in repairing the DSBs through homologous recombination repair (HRR) and apoptosis. A defective homologous recombination eventually leads to cancer progression. XRCC2 is a RAD51 paralogue and considered essential in the HRR process. Mutations in XRCC2 compromise the DNA repair mechanisms and increase susceptibility to cancers (He et al., 2014). We have reported one mutation XRCC2 p.R188H, which is already well-established to have an association with the cancer progression (García-Closas et al., 2006). XRCC2 p.R188H was previously reported from India (Datkhile et al., 2023).
The molecular dynamic simulation results revealed differences in the radius of gyration and root mean square deviation. It has been reported that the SNVs can perturb the nature of the proteins or its segments and may cause shift in the equilibrium between different conformations, destabilizes the protein, or modifies the conformational dynamics. From the results of the MDS, it was clearly evident that for all the IS mutations, the radius of gyration for the mutant proteins has increased, as compared to their wild type. This signifies that the mutant proteins have a perturbed unfolded nature, as compared to the wild types.
The RMSD trajectories were used to compare the differences in the backbone from initial conformation to its final conformation. The protein stability in the context of its conformation can be deduced from the deviations produced during the simulation time. Larger deviations are synonymous with the perturbed structure of the protein (Aier et al., 2016). We observed major deviations in the RMSD comparison of XRCC p.R188H, as compared to the others, where relatively smaller deviations were observed. These results of the MDS simulations complemented the in silico predictions using SAAFEQ-SEQ, which concluded the destabilizing effect for all of the obtained SNVs including the interacting site mutations.
We docked the proteins and their mutants with two compounds of interest (DB11979 and LTS0102038). The effect of mutations on binding affinity varied depending on the protein and the compound. Binding residues also got altered, while some were conserved in binding in normal or mutated states. Mutations can have varying effects on affinity due to the alteration of involved binding residues. Understanding these interactions is essential for drug design and understanding the impact of genetic mutations on protein function. We propose that FDA-approved drug repurposing, nutraceutical, and natural product screening should be attempted at a wider scale against the proteins and their mutants of interest. We also propose that the in silico impact should be further explored by complementary in silico, in vitro, and in vivo assays.
Conclusion
The present study pioneered the acquisition of the mutational landscape of the breast cancer susceptibility genes (ATM, CHEK2, PALB2, and XRCC2) using next-generation whole-exome sequencing from paraffin-fixed FFPE tissue blocks obtained from the breast cancer patients of Pashtun ethnicity. The acquired sequence data were studied in the context of sociogenetic and clinicopathologic features. We found that the ATM was frequently mutated, as compared to others. Out of the total 18 mutations (14 somatic and 4 germline), 8 mutations were identified as novel. Seventeen mutations were nonsynonymous SNVs. SIFT, PolyPhen-2, and MutationTaster databases were used to examine pathogenicity and tolerability. PALB2 p.Q559R was the most prevalent mutation among the patients that can be further studied in larger cohorts for biomarker implications. The molecular dynamics simulation study revealed that the SNVs contributed to a perturbed protein-folding behavior. CHEK2 p.R180C was found only in PR+, and the remaining CHEK2 p.M314I and p.W114L were only found in PR-. Molecular docking results showed that mutations altered the drug interactions.
Less sample size and enrollments forming a single region are the limitations to the study.
Data availability statement
The datasets presented in this study can be found in online repositories. The names of the repository/repositories and accession number(s) can be found at: https://www.ncbi.nlm.nih.gov/, PRJNA941166.
Ethics statement
The studies involving humans were approved by the Research Ethics Committee, Khyber Medical University. The studies were conducted in accordance with the local legislation and institutional requirements. The participants provided their written informed consent to participate in this study.
Author contributions
HA: conceptualization, investigation, and writing–original draft. AA: conceptualization, funding acquisition, project administration, resources, supervision, and writing–review and editing. AK: conceptualization, investigation, writing–original draft, and writing–review and editing. RA: methodology, investigation, and writing–review and editing. IK: investigation, methodology, and writing–original draft. MK: resources and writing–original draft. IA: methodology and writing–original draft. ZB: investigation, visualization, and writing–review and editing. MA: formal analysis, methodology, software, validation, writing–original draft, and writing–review and editing. AM: conceptualization, data curation, formal analysis, and writing–review and editing.
Funding
The authors declare that financial support was received for the research, authorship, and/or publication of this article. This research is funded by the Higher Education Commission Pakistan under the National Research Program for Universities vide Project No. 6820/KPK/NRPU/R&D/HEC/2016. IA is a recipient of the Brain Pool Fellowship by the National Research Foundation of Korea (grant number RS-2023-00223245).
Acknowledgments
The authors would like to acknowledge the financial support obtained through HEC-NRPU project vide 6820/KPK/NRPU/R&D/HEC/2016. We would also like to thank Dr. Abid Jamil from Hayat Abad Medical Complex-Peshawar for allowing the collection of the samples. We would also like to thank ORIC-KMU for faclitation.
Conflict of interest
Authors IA and ZB were employed by the Alpha Genomics (Private) Limited.
The remaining authors declare that the research was conducted in the absence of any commercial or financial relationships that could be construed as a potential conflict of interest.
Publisher’s note
All claims expressed in this article are solely those of the authors and do not necessarily represent those of their affiliated organizations, or those of the publisher, the editors, and the reviewers. Any product that may be evaluated in this article, or claim that may be made by its manufacturer, is not guaranteed or endorsed by the publisher.
Supplementary material
The Supplementary Material for this article can be found online at: https://www.frontiersin.org/articles/10.3389/fgene.2024.1383284/full#supplementary-material
References
Ahmad, H., Ali, A., Ali, R., Khalil, A. T., Khan, I., Khan, M. M., et al. (2023). Mutational landscape and in-silico analysis of TP53, PIK3CA, and PTEN in patients with breast cancer from khyber Pakhtunkhwa. ACS Omega 8, 43318–43331. doi:10.1021/acsomega.3c07472
Aier, I., Varadwaj, P. K., and Raj, U. (2016). Structural insights into conformational stability of both wild-type and mutant EZH2 receptor. Sci. Rep. 6 (1), 34984. doi:10.1038/srep34984
Ansari, N., Shahrabi, S., Khosravi, A., Shirzad, R., and Rezaeean, H. (2019). Prognostic significance of CHEK2 mutation in progression of breast cancer. Lab. Med. 50 (3), e36–e41. doi:10.1093/labmed/lmz009
Bao, Y., Wang, L., Shi, L., Yun, F., Liu, X., Chen, Y., et al. (2019). Transcriptome profiling revealed multiple genes and ECM-receptor interaction pathways that may be associated with breast cancer. Cell. Mol. Biol. Lett. 24 (1), 38–20. doi:10.1186/s11658-019-0162-0
Baretić, D., Pollard, H. K., Fisher, D. I., Johnson, C. M., Santhanam, B., Truman, C. M., et al. (2017). Structures of closed and open conformations of dimeric human ATM. Sci. Adv. 3 (5), e1700933. doi:10.1126/sciadv.1700933
Bayarri, G., Andrio, P., Hospital, A., Orozco, M., and Gelpí, J. L. (2022). BioExcel Building Blocks Workflows (BioBB-Wfs), an integrated web-based platform for biomolecular simulations. Nucleic Acids Res. 50 (W1), W99–W107. doi:10.1093/nar/gkac380
Biovia, D. S., Berman, H. M., Westbrook, J., Feng, Z., Gilliland, G., Bhat, T. N., et al. (2000). Dassault systèmes BIOVIA, [Discovery studio visualizer, V. 17.2], San Diego: dassault systèmes. J. Chem. Phys. 10 (21). doi:10.1016/0021-9991(74)90010-2
Boonen, R. A., Vreeswijk, M. P., and van Attikum, H. (2022). CHEK2 variants: linking functional impact to cancer risk. Trends Cancer 8, 759–770. doi:10.1016/j.trecan.2022.04.009
Cavaciuti, E., Lauge, A., Janin, N., Ossian, K., Hall, J., Stoppa-Lyonnet, D., et al. (2005). Cancer risk according to type and location of ATM mutation in ataxia-telangiectasia families. Genes, Chromosomes Cancer 42 (1), 1–9. doi:10.1002/gcc.20101
Cerami, E., Gao, J., Dogrusoz, U., Gross, B. E., Sumer, S. O., Aksoy, B. A., et al. (2012). The cBio cancer genomics portal: an open platform for exploring multidimensional cancer genomics data. Cancer Discov. 2 (5), 401–404. doi:10.1158/2159-8290.CD-12-0095
Datkhile, K. D., Gudur, R. A., Bhosale, S. J., Durgawale, P. P., Jagdale, N. J., More, A. L., et al. (2023). Impact of interaction between single nucleotide polymorphism of XRCC1, XRCC2, XRCC3 with tumor suppressor Tp53 gene increases risk of breast cancer: a hospital based case-control study. Asian Pac. J. Cancer Prev. 24 (9), 3065–3075. doi:10.31557/APJCP.2023.24.9.3065
Ding, D., Javed, A. A., Cunningham, D., Teinor, J., Wright, M., Javed, Z. N., et al. (2021). Challenges of the current precision medicine approach for pancreatic cancer: a single institution experience between 2013 and 2017. Cancer Lett. 497, 221–228. doi:10.1016/j.canlet.2020.10.039
Dufault, M. R., Betz, B., Wappenschmidt, B., Hofmann, W., Bandick, K., Golla, A., et al. (2004). Limited relevance of the CHEK2 gene in hereditary breast cancer. Int. J. cancer 110 (3), 320–325. doi:10.1002/ijc.20073
Durocher, D., and Jackson, S. P. (2002). The FHA domain. FEBS Lett. 513 (1), 58–66. doi:10.1016/s0014-5793(01)03294-x
Dutta, P., Keung, M. Y., Wu, Y., and Vadgama, J. V. (2023). Genetic variants in African-American and Hispanic patients with breast cancer. Oncol. Lett. 25 (2), 51–12. doi:10.3892/ol.2022.13637
Economopoulou, P., Dimitriadis, G., and Psyrri, A. (2015). Beyond BRCA: new hereditary breast cancer susceptibility genes. Cancer Treat. Rev. 41 (1), 1–8. doi:10.1016/j.ctrv.2014.10.008
Evans, M. K., and Longo, D. L. (2014). PALB2 mutations and breast-cancer risk. N. Engl. J. Med. 371 (6), 566–568. doi:10.1056/NEJMe1405784
Gao, J., Aksoy, B., Dogrusoz, U., Dresdner, G., Gross, B., Sumer, S., et al. (2013). Integrative analysis of complex cancer genomics and clinical profiles using the cBioPortal. Sci. Signal. 269, pl1. doi:10.1126/scisignal.2004088
García-Closas, M., Egan, K. M., Newcomb, P. A., Brinton, L. A., Titus-Ernstoff, L., Chanock, S., et al. (2006). Polymorphisms in DNA double-strand break repair genes and risk of breast cancer: two population-based studies in USA and Poland, and meta-analyses. Hum. Genet. 119, 376–388. doi:10.1007/s00439-006-0135-z
Griffin, C. S., Simpson, P. J., Wilson, C. R., and Thacker, J. (2000). Mammalian recombination-repair genes XRCC2 and XRCC3 promote correct chromosome segregation. Nat. Cell Biol. 2 (10), 757–761. doi:10.1038/35036399
Gulzar, F., Akhtar, M. S., Sadiq, R., Bashir, S., Jamil, S., and Baig, S. M. (2019). Identifying the reasons for delayed presentation of Pakistani breast cancer patients at a tertiary care hospital. Cancer Manag. Res. 11, 1087–1096. doi:10.2147/CMAR.S180388
Han, J., Hankinson, S. E., Zhang, S. M., De Vivo, I., and Hunter, D. J. (2004). Interaction between genetic variations in DNA repair genes and plasma folate on breast cancer risk. Cancer Epidemiol. Biomarkers Prev. 13 (4), 520–524. doi:10.1158/1055-9965.520.13.4
He, Y., Zhang, Y., Jin, C., Deng, X., Wei, M., Wu, Q., et al. (2014). Impact of XRCC2 Arg188His polymorphism on cancer susceptibility: a meta-analysis. PLoS One 9 (3), e91202. doi:10.1371/journal.pone.0091202
IARC International Agency for Resaerch on Cancer (2020). Globocan 2020, factsheet on Pakistan, Lyon, France: IARC International Agency for Resaerch on Cancer.
Johnson, R. D., Liu, N., and Jasin, M. (1999). Mammalian XRCC2 promotes the repair of DNA double-strand breaks by homologous recombination. Nature 401 (6751), 397–399. doi:10.1038/43932
Khaliq, I. H., Mahmood, H. Z., Sarfraz, M. D., Gondal, K. M., and Zaman, S. (2019). Pathways to care for patients in Pakistan experiencing signs or symptoms of breast cancer. Breast 46, 40–47. doi:10.1016/j.breast.2019.04.005
Khan, N. H., Duan, S.-F., Wu, D.-D., and Ji, X.-Y. (2021). Better reporting and awareness campaigns needed for breast cancer in Pakistani women. Cancer Manag. Res. 13, 2125–2129. doi:10.2147/CMAR.S270671
Kleibl, Z., Havranek, O., Novotny, J., Kleiblova, P., Soucek, P., and Pohlreich, P. (2008). Analysis of CHEK2 FHA domain in Czech patients with sporadic breast cancer revealed distinct rare genetic alterations. Breast cancer Res. Treat. 112, 159–164. doi:10.1007/s10549-007-9838-7
Kuschel, B., Auranen, A., McBride, S., Novik, K. L., Antoniou, A., Lipscombe, J. M., et al. (2002). Variants in DNA double-strand break repair genes and breast cancer susceptibility. Hum. Mol. Genet. 11 (12), 1399–1407. doi:10.1093/hmg/11.12.1399
Laitman, Y., Kaufmann, B., Lahad, E. L., Papa, M. Z., and Friedman, E. (2007). Germline CHEK2 mutations in Jewish Ashkenazi women at high risk for breast cancer. IMAJ-RAMAT GAN- 9 (11), 791–796.
Le Guilloux, V., Schmidtke, P., and Tuffery, P. (2009). Fpocket: an open source platform for ligand pocket detection. BMC Bioinforma. 10 (1), 168–211. doi:10.1186/1471-2105-10-168
Li, G., Guo, X., Chen, M., Tang, L., Jiang, H., Day, J. X., et al. (2018). Prevalence and spectrum of AKT1, PIK3CA, PTEN and TP53 somatic mutations in Chinese breast cancer patients. PLoS One 13 (9), e0203495. doi:10.1371/journal.pone.0203495
Li, H., and Durbin, R. (2010). Fast and accurate long-read alignment with Burrows–Wheeler transform. Bioinformatics 26 (5), 589–595. doi:10.1093/bioinformatics/btp698
Liu, Q., Cheng, B., Jin, Y., and Hu, P. (2022). Bayesian tensor factorization-drive breast cancer subtyping by integrating multi-omics data. J. Biomed. Inf. 125, 103958. doi:10.1016/j.jbi.2021.103958
Liu, Q., and Hu, P. (2023). Radiogenomic association of deep MR imaging features with genomic profiles and clinical characteristics in breast cancer. Biomark. Res. 11 (1), 9. doi:10.1186/s40364-023-00455-y
Ma, J., and Jemal, A. (2013). Breast cancer statistics. Breast Cancer Metastasis Drug Resist. Prog. Prospects, 1–18. doi:10.1007/978-1-4614-5647-6_1
Mangone, F. R., Miracca, E. C., Feilotter, H. E., Mulligan, L. M., and Nagai, M. A. (2015). ATM gene mutations in sporadic breast cancer patients from Brazil. Springerplus 4, 23–10. doi:10.1186/s40064-015-0787-z
Mathioudaki, A., Ljungström, V., Melin, M., Arendt, M. L., Nordin, J., Karlsson, Å., et al. (2020). Targeted sequencing reveals the somatic mutation landscape in a Swedish breast cancer cohort. Sci. Rep. 10 (1), 19304. doi:10.1038/s41598-020-74580-1
Mohamad, S., Isa, N. M., Muhammad, R., Emran, N. A., Kitan, N. M., Kang, P., et al. (2015). Low prevalence of CHEK2 gene mutations in multiethnic cohorts of breast cancer patients in Malaysia. PLoS One 10 (1), e0117104. doi:10.1371/journal.pone.0117104
Momenimovahed, Z., and Salehiniya, H. (2019). Epidemiological characteristics of and risk factors for breast cancer in the world. Breast Cancer Targets Ther. 11, 151–164. doi:10.2147/BCTT.S176070
Moslemi, M., Moradi, Y., Dehghanbanadaki, H., Afkhami, H., Khaledi, M., Sedighimehr, N., et al. (2021). The association between ATM variants and risk of breast cancer: a systematic review and meta-analysis. BMC cancer 21 (1), 27–12. doi:10.1186/s12885-020-07749-6
Mubarik, S., Wang, F., Luo, L., Hezam, K., and Yu, C. (2023). Evaluation of Lee–Carter model to breast cancer mortality prediction in China and Pakistan. Front. Oncol. 13, 1101249. doi:10.3389/fonc.2023.1101249
Nepomuceno, T. C., Carvalho, M. A., Rodrigue, A., Simard, J., Masson, J.-Y., and Monteiro, A. N. (2021). PALB2 variants: protein domains and cancer susceptibility. Trends Cancer 7 (3), 188–197. doi:10.1016/j.trecan.2020.10.002
Park, D., Lesueur, F., Nguyen-Dumont, T., Pertesi, M., Odefrey, F., Hammet, F., et al. (2012). Rare mutations in XRCC2 increase the risk of breast cancer. Am. J. Hum. Genet. 90 (4), 734–739. doi:10.1016/j.ajhg.2012.02.027
Park, J.-Y., Zhang, F., and Andreassen, P. R. (2014). PALB2: the hub of a network of tumor suppressors involved in DNA damage responses. Biochimica Biophysica Acta (BBA)-Reviews Cancer 1846 (1), 263–275. doi:10.1016/j.bbcan.2014.06.003
Phan, L. M., and Rezaeian, A.-H. (2021). ATM: main features, signaling pathways, and its diverse roles in DNA damage response, tumor suppression, and cancer development. Genes 12 (6), 845. doi:10.3390/genes12060845
Rubi, G., Amir, M., Ashraf, N., Bashir, A., and Andaleeb, H. (2022). Breast cancer in Pakistan-an updated overview. Pak. Postgrad. Med. J. 33 (04), 120–123. doi:10.51642/ppmj.v33i04.531
Saeed, S., Mahjabeen, I., Sarwar, R., Bashir, K., and Kayani, M. A. (2017). Haplotype analysis of XRCC2 gene polymorphisms and association with increased risk of head and neck cancer. Sci. Rep. 7 (1), 13210. doi:10.1038/s41598-017-13461-6
Sarwar, M. R., Iftikhar, S., and Saqib, A. (2018). Availability of anticancer medicines in public and private sectors, and their affordability by low, middle and high-income class patients in Pakistan. BMC cancer 18 (1), 14–11. doi:10.1186/s12885-017-3980-3
Schrauder, M., Frank, S., Strissel, P., Lux, M., Bani, M., Rauh, C., et al. (2008). Single nucleotide polymorphism D1853N of the ATM gene may alter the risk for breast cancer. J. cancer Res. Clin. Oncol. 134, 873–882. doi:10.1007/s00432-008-0355-9
Silvestri, V., Rizzolo, P., Zanna, I., Falchetti, M., Masala, G., Bianchi, S., et al. (2010). PALB2 mutations in male breast cancer: a population-based study in Central Italy. Breast cancer Res. Treat. 122, 299–301. doi:10.1007/s10549-010-0797-z
Stubbins, R. J., Korotev, S., and Godley, L. A. (2022). Germline CHEK2 and ATM variants in myeloid and other hematopoietic malignancies. Curr. Hematol. Malignancy Rep. 17 (4), 94–104. doi:10.1007/s11899-022-00663-7
Stucci, L. S., Internò, V., Tucci, M., Perrone, M., Mannavola, F., Palmirotta, R., et al. (2021). The ATM Gene in breast cancer: its relevance in clinical practice. Genes 12 (5), 727. doi:10.3390/genes12050727
Trott, O., and Olson, A. J. (2010). AutoDock Vina: improving the speed and accuracy of docking with a new scoring function, efficient optimization, and multithreading. J. Comput. Chem. 31 (2), 455–461. doi:10.1002/jcc.21334
Ueno, S., Sudo, T., and Hirasawa, A. (2022). ATM: functions of ATM kinase and its relevance to hereditary tumors. Int. J. Mol. Sci. 23 (1), 523. doi:10.3390/ijms23010523
Vidra, R., Ciuleanu, T. E., Nemeș, A., Pascu, O., Heroiu, A. M., Antone, N., et al. (2022). Spectrum of BRCA1/2 mutations in Romanian breast and ovarian cancer patients. Int. J. Environ. Res. Public Health 19 (7), 4314. doi:10.3390/ijerph19074314
Wishart, D. S., Feunang, Y. D., Guo, A. C., Lo, E. J., Marcu, A., Grant, J. R., et al. (2018). DrugBank 5.0: a major update to the DrugBank database for 2018. Nucleic Acids Res. 46 (D1), D1074–D1082. doi:10.1093/nar/gkx1037
Keywords: breast cancer, oncogenes, mutations, Pashtun, docking, clinicopathologic association, evolutionary significance
Citation: Ahmad H, Ali A, Khalil AT, Ali R, Khan I, Khan MM, Ahmed I, Basharat Z, Alorini M and Mehmood A (2024) Clinico-genomic findings, molecular docking, and mutational spectrum in an understudied population with breast cancer patients from KP, Pakistan. Front. Genet. 15:1383284. doi: 10.3389/fgene.2024.1383284
Received: 07 February 2024; Accepted: 03 April 2024;
Published: 09 May 2024.
Edited by:
Nicoletta Coccaro, University of Bari Aldo Moro, ItalyReviewed by:
Rasa Ugenskiene, Lithuanian University of Health Sciences, LithuaniaIsil Ezgi Eryilmaz, Bursa Uludağ University, Türkiye
Copyright © 2024 Ahmad, Ali, Khalil, Ali, Khan, Khan, Ahmed, Basharat, Alorini and Mehmood. This is an open-access article distributed under the terms of the Creative Commons Attribution License (CC BY). The use, distribution or reproduction in other forums is permitted, provided the original author(s) and the copyright owner(s) are credited and that the original publication in this journal is cited, in accordance with accepted academic practice. No use, distribution or reproduction is permitted which does not comply with these terms.
*Correspondence: Asif Ali, ZHJhbGlhc2lmN0BnbWFpbC5jb20=; Ali Talha Khalil, YWxpdGFsaGEua2hhbGlsQGxyaC5lZHUucGs=; Amna Mehmood, YW1uYS5tZWhtb29kQHBoeXNpay51bmktaGFsbGUuZGU=