- 1Department of Anus and Intestinal Surgery, The Affiliated Hospital of Guizhou Medical University, Guiyang, China
- 2Department of Infectious Diseases, The Affiliated Hospital of Guizhou Medical University, Guiyang, China
- 3Department of Hepatobiliary Surgery, The Affiliated Hospital of Guizhou Medical University, Guiyang, China
Background: Colorectal cancer (CRC) poses a significant global health burden, with high incidence and mortality rates. Despite advances in diagnostic and therapeutic modalities, early diagnosis remains critical for improved outcomes. Recent research has realized the important role of gut microbiota in CRC development, highlighting the need to elucidate potential relationships.
Methods: In this study, we employed Mendelian randomization (MR) to establish a robust potential link between gut microbial genera and CRC. Data from the MiBioGen database provided curated genome-wide association study (GWAS) summary datasets for microbial genera, while the Finngen database provided CRC outcome data. Instrumental variables (IVs) were identified based on genetic variants associated with gut microbiota. Various MR methods, including Inverse Variance Weighted (IVW), Weighted Median, Weighted Mode, Simple Mode, and MR-Egger, were employed to estimate potential effects. Functional analysis of genes near single nucleotide polymorphisms (SNPs) was performed to unravel potential pathways.
Results: Analysis of microbial genera identified five potentially associated with CRC: Eubacterium fissicatena group, Anaerofilum, Defluviitaleaceae UCG011, Ruminococcus 2, and Sutterella. Notably, Defluviitaleaceae UCG011 emerged as the only risk factor. Gene analysis revealed hub genes PTPRD and DSCAM near Defluviitaleaceae UCG011 associated SNPs. Expression analysis showed that PTPRD decreased in colon cancer and DSCAM decreased in rectal cancer. The methylation status of the PTPRD gene promoter region indicated potential regulatory alterations.
Conclusion: This study establishes a potential relationship between five specific gut microbial genera, particularly Defluviitaleaceae UCG011, and CRC. Hub genes PTPRD and DSCAM provide insights into potential molecular mechanisms, suggesting the potential role of Defluviitaleaceae UCG011 in modulating the initiation and progression of CRC. Further research is essential to validate these associations and delve deeper into therapeutic implications.
Introduction
Colorectal cancer (CRC) poses a significant global health challenge, with 1.8 million new diagnoses and 1 million deaths annually (Bray et al., 2018). Advances in diagnostic technologies and treatments, including surgery and chemotherapy, have led to an improved prognosis for CRC. Despite these advancements, CRC continues to be a global health issue, exerting a substantial impact on morbidity and mortality rates worldwide (Sung et al., 2021; Zheng RS. et al., 2023). The 5-year survival rate for early-stage (stages I and II) CRC patients can reach 80%, but it declines to less than 20% for those with advanced-stage disease, particularly stage IV (Biller and Schrag, 2021). Thus, identifying and understanding the factors and mechanisms that promote CRC development are crucial for improving treatment outcomes. CRC development is complex, involving a combination of genetic, environmental, and dietary factors (Thanikachalam and Khan, 2019). Recently, gut microbiota has been identified as playing a significant role in CRC development (Si et al., 2021).
The gut microbiota, an integral part of the human microbiome, is increasingly recognized as a key ecological factor influencing human health (Long et al., 2023). A substantial body of evidence indicates an association between gut microbiota and various digestive cancers, including colorectal, gastric, liver, and esophageal cancers, among others (Chen et al., 2022; Eun et al., 2014; Gao et al., 2023; Ni et al., 2022). Previous research suggests that the gut microbiota can influence CRC development by releasing various metabolites, proteins, and macromolecules that interact with the host’s colonic epithelium and immune cells (Avuthu and Guda, 2022). Some clinical evidence also underscores the role of the gut microbiota in modifying the therapeutic responses of patients with CRC to chemotherapy and immunotherapy (Wong and Yu, 2023). Moreover, the gut microbiota plays a crucial role in CRC metastasis, although the underlying mechanisms remain elusive (Zheng Z. et al., 2023). Studies have documented characteristic changes in gut microbiota across different CRC stages, with distinct alterations evident even at the colorectal adenoma stage (Dai et al., 2018; Liu et al., 2021; Wong and Yu, 2019). However, it is important to recognize that some observational studies may yield inconsistent conclusions due to limitations in assessing gut microbiota dysbiosis.
To establish a more robust potential relationship between gut microbial genera and CRC, we adopted Mendelian randomization (MR), a method that uses random genetic variants to mimic a randomized controlled trial (RCT), thereby circumventing environmental and lifestyle confounders. MR essentially functions as a natural RCT (Burgess et al., 2015; Larsson et al., 2023). MR is based on three key assumptions: 1) Genetic variations are associated with the risk factor of interest (relevance assumption); 2) Genetic variations are independent of confounders (independence assumption); and 3) Genetic variations affect the outcome only through the risk factor (exclusion restriction assumption) (Birney, 2022) (Figure 1A). Two-sample MR estimates potential effects by leveraging exposure and outcome data from different samples, thereby addressing the limitations of conventional observational studies (Davies et al., 2018; Lawlor, 2016).
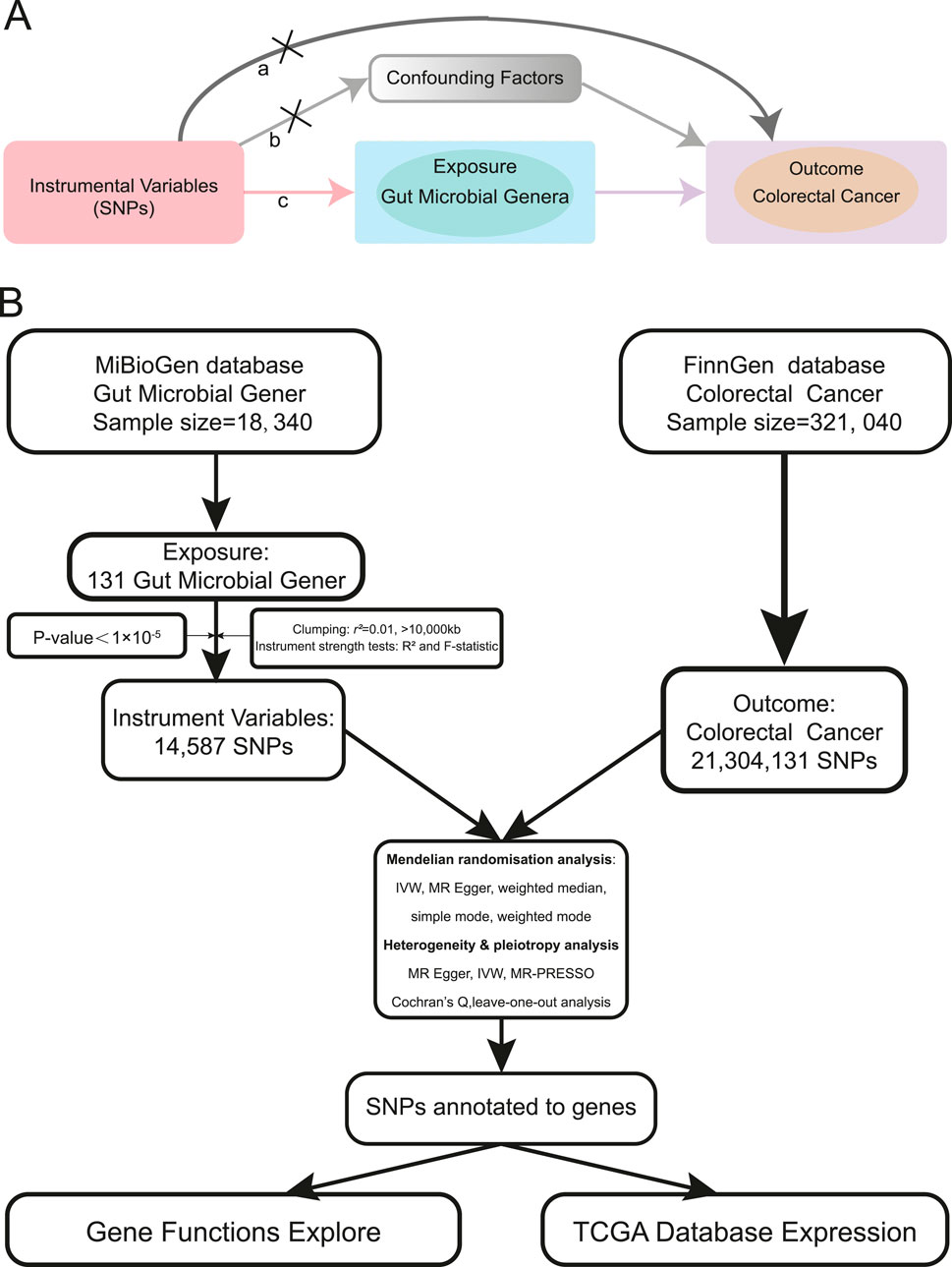
Figure 1. MR assumptions and analysis flow of this study. (A). The MR assumptions (a. The exclusion restriction assumption; b. The independence assumption; c. The relevance assumption). (B). The analysis flow of this study.
In this study, we explored the potential relationship between gut microbial genera and CRC through a two-sample Mendelian randomization analysis. Additionally, we conducted a functional analysis of genes near single nucleotide polymorphisms (SNPs) identified in the study, to better understand the potential pathways through which these genera may influence CRC occurrence and development. Our aim was to elucidate the potential impact of gut bacterial genera on CRC, thereby providing new insights into the mechanisms underlying the development of colorectal cancer.
Methods
Data sources and screening of instrumental variables
We queried the MiBioGen database (https://mibiogen.gcc.rug.nl/) (Swertz et al., 2010; Swertz and Jansen, 2007; van der Velde et al., 2019) for curated GWAS summary datasets related to gut microbiota. Our focus was on GWAS data related to microbial genera. Using publicly accessible data, we conducted a two-sample MR study. We used genetic variants associated with gut microbial genera as instrumental variables (IVs), employing a p-value threshold of 1 × 10-5 based on previous reports (Bonder et al., 2016; Sanna et al., 2019). Additionally, we pruned the IVs based on linkage disequilibrium (LD) criteria (r2 ≥ 0.01, kb > 10,000) (Pritchard and Przeworski, 2001). Furthermore, we excluded SNPs that were palindromic with minor allele frequencies (Hemani et al., 2018a). After evaluating the IVs for exposure, we computed the F statistic for each individual variant. Each variant demonstrated an F statistic above 10, indicating a strong IV. It is generally accepted that an F statistic below 10 indicates a weak IV (Bowden et al., 2019; Burgess et al., 2011). GWAS data for colorectal cancer as the outcome was obtained from the most recent Finngen database (https://www.finngen.fi/) (Kurki et al., 2023). The FinnGen study is a large-scale genomics initiative that has analyzed over 500,000 Finnish biobank samples, correlating genetic variation with health data to understand disease mechanisms and predispositions (Kurki et al., 2022). This project is a collaboration between research organizations and biobanks in Finland and international industry partners. The latest release of the Finngen database is R10, updated in December 2023. The analysis flow of our study is illustrated in Figure 1B.
Mendelian randomization analysis
This study employed the Inverse Variance Weighted (IVW) Random Effects as the primary MR method, complemented by four additional approaches: Weighted Median, Weighted Mode, Simple Mode, and MR-Egger. These diverse techniques provide a comprehensive perspective on the potential relationship, accounting for various assumptions and biases. After conducting a two-sample MR analysis on the overall microbial genera, those potentially associated with colorectal cancer were identified using the IVW method, with a significance threshold of p < 0.05. All p-values were adjusted using the Benjamini–Hochberg method to evaluate false discovery rate (FDR) (Benjamini and Hochberg, 2024). The results were visualized using forest plots and circos plots. Subsequently, a dedicated MR analysis was performed on the genera identified as potentially associated with colorectal cancer. All MR analyses were conducted using RStudio software (Version: 2023.06.0 Build 421) and R software (Version: 4.3.2) (Hemani et al., 2018b).
Heterogeneity and pleiotropy test
We assessed heterogeneity among SNPs using Cochran’s Q-statistics (Egger et al., 1997) and the I2 statistic (Bowden et al., 2016; Higgins and Thompson, 2002). We used MR-Egger and Mendelian Randomization Pleiotropy Residual Sum and Outlier (MR-PRESSO) to assess horizontal pleiotropy of SNPs(Verbanck et al., 2018). Additionally, we conducted a ‘Leave-one-out’ analysis to explore the influence of individual SNPs on the overall association (Mikshowsky et al., 2017).
Gene functions explore
Based on the MR analysis results, we compiled a list of all SNPs included in the study. Using R software, we searched for genes within 50 base pairs of each SNP. We then explored the interactions among the identified genes using the STRING database (https://string-db.org/)(Szklarczyk et al., 2019) and Cytoscape software (Version 3.10.1) (Maere et al., 2005) to identify key functional genes. We performed functional enrichment analysis of these genes using Gene Ontology (GO) (Ashburner et al., 2000; Gene Ontology et al., 2023) and the Kyoto Encyclopedia of Genes and Genomes (KEGG) (Kanehisa and Goto, 2000), and visualized the results for better interpretation using RStudio software (Version: 2023.06.0 Build 421). Next, we analyzed the expression profiles of the relevant genes in colorectal cancer using The Cancer Genome Atlas (TCGA) database. We further analyzed the methylation status of the PTPRD gene promoter region using the TCGA database. We then analyzed the relationship between PTPRD gene expression and colon cancer survival by mapping the Kaplan-Meier (KM) survival curve.
Results
Research datasets and instrumental variables
We obtained GWAS data summaries for 131 microbial genera from the MiBioGen database (Kurilshikov et al., 2021). After filtering with a significance threshold of p < 1 × 10-5, we identified 14,587 SNPs. Subsequently, we pruned for linkage disequilibrium and selected strong instrumental variables, resulting in a final set of 1,531 eligible SNPs for inclusion in the MR analysis. Details and F statistics for the SNPs included in this study are provided in Supplementary Table S1. The outcome data were retrieved from the FinnGen database, focusing on the colorectal cancer phenotype. The GWAS data included in the MR analysis consisted of 6,847 cases and 314,193 controls, encompassing a total of 21,304,131 SNPs. Information on the GWAS data used in this study is presented in Table 1.
Mendelian randomization results
The results of the MR analysis for all microbial genera are provided in Supplementary Table S2. Among them, 75 genera in the IVW method were visually represented in a circos plot, with those potentially associated with colorectal cancer highlighted in red (Figure 2). As shown in Figure 2, five genera demonstrated a potential relationship with colorectal cancer, namely, Eubacterium fissicatena group [Beta = −0.136, OR (95%CI) = 0.873 (0.779–0.978), p = 0.019], Anaerofilum [Beta = −0.118, OR (95%CI) = 0.889 (0.795–0.994), p = 0.039], Defluviitaleaceae UCG011 [Beta = 0.192, OR (95%CI) = 1.212 (1.037–1.416), p = 0.016], Ruminococcus 2 [Beta = −0.162, OR (95%CI) = 0.850 (0.735–0.984), p = 0.029], and Sutterella [Beta = −0.226, OR (95%CI) = 0.798 (0.669–0.951), p = 0.012]. The MR results for these five genera were further illustrated using forest plots (Figure 3) and scatter plots (Figure 4). Additionally, Eubacterium xylanophilum group [Beta = −0.781, OR (95%CI) = 0.458 (0.247–0.850), p = 0.035]and Barnesiella [Beta = −0.902, OR (95%CI) = 0.406 (0.179–0.920), p = 0.049] showed a potential relationship with colorectal cancer in the MR Egger method, while Defluviitaleaceae UCG011 [Beta = 0.218, OR (95%CI) = 1.243 (1.007–1.535), p = 0.043] and Sutterella [Beta = −0.269, OR (95%CI) = 0.764 (0.601–0.972), p = 0.028]exhibited a potential relationship in the Weighted Median method. We adjusted the p-values of IVW method and found that all FDR were close to 1 (Supplementary Table S3).
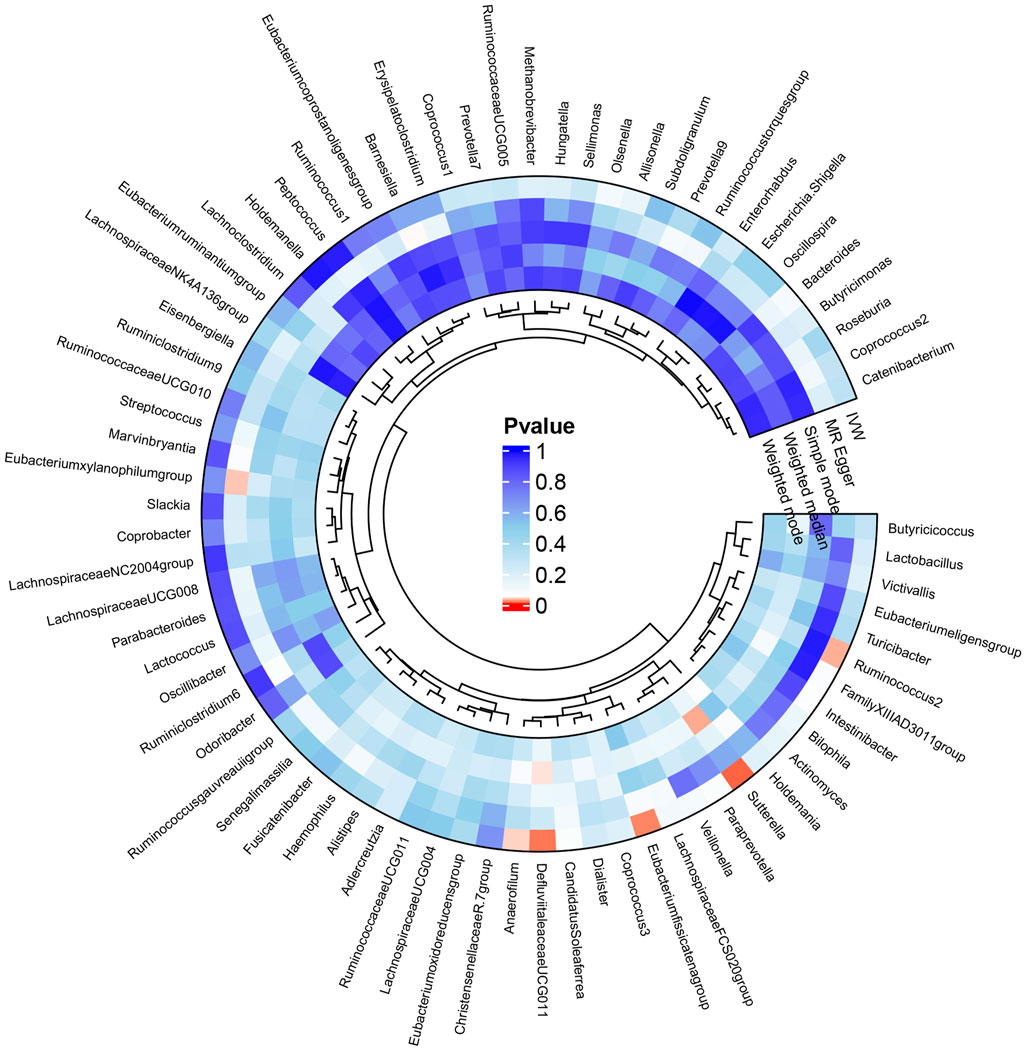
Figure 2. Circos plot of MR results for the associations between all gut microbial genera and the risk of colorectal cancer. From the inner to outer circles, they represent the estimates of weighted mode, weighted median, simple mode, MR-Egger, and inverse-variance weighted methods, respectively. The shades of color reflect the magnitude of the value of p-value, and red shades reflect p-value <0.05.
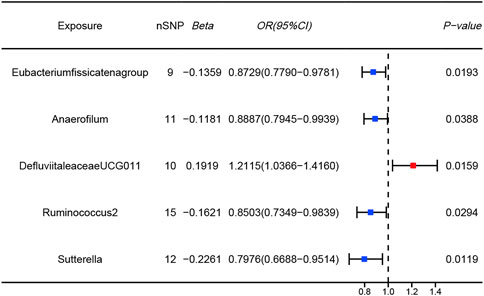
Figure 3. Forest plots of MR results for five gut microbial genera potentially associated with colorectal cancer using IVW method (Blue dots represent protective factors; Red dot represents risk factors).
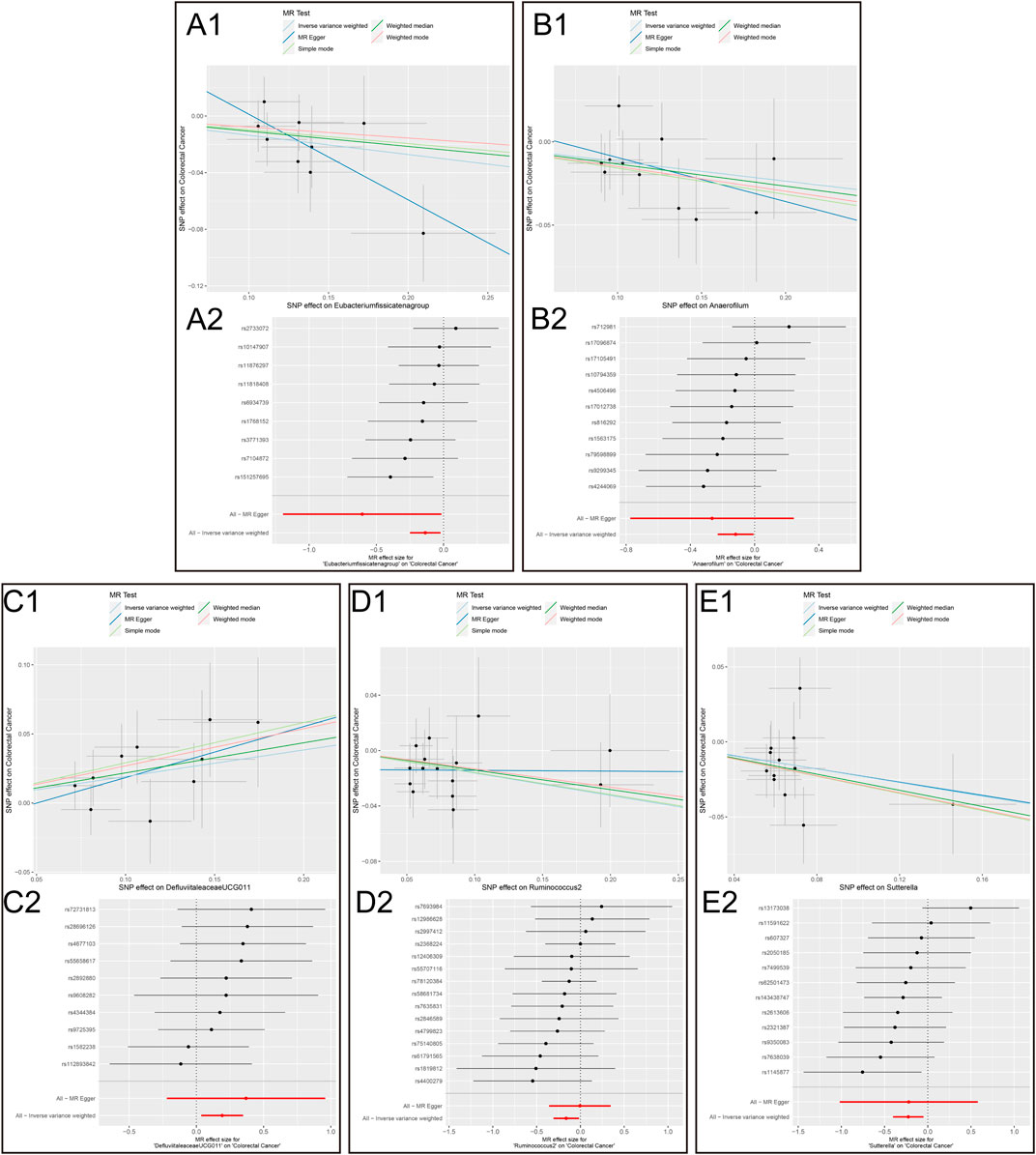
Figure 4. Scatter plots and MR effect size plots for five gut microbial genera potentially associated with colorectal cancer. (A) Scatter plots (B) MR effect size plots (1. Eubacterium fissicatena group on Colorectal Cancer. 2. Anaerofilum on Colorectal Cancer. 3. Defluviitaleaceae UCG011 on Colorectal Cancer. 4. Ruminococcus 2 on Colorectal Cancer.5. Sutterella on Colorectal Cancer).
Heterogeneity and pleiotropy test results
Cochran’s Q test and the I2 statistic were conducted to evaluate heterogeneity among instrumental variable estimates derived from individual genetic variants. The results revealed no significant evidence of heterogeneity, as presented in Table 2. This lack of heterogeneity suggests that the MR estimates are more reliable. The ‘leave-one-out’ analysis, where each SNP was systematically removed to assess its impact on the IVW point estimate (Figures 5A–E), indicated that no single SNP significantly influenced the overall result. The funnel plot exhibited no significant asymmetry, indicating minimal publication bias (Figure 5F). Furthermore, MR-Egger regression and the MR-PRESSO global test both indicated no horizontal pleiotropy, as detailed in Table 2. In summary, the combination of minimal heterogeneity, consistent ‘leave-one-out’ results, and the absence of asymmetry enhances confidence in the reliability of the MR estimates and mitigates concerns regarding bias.
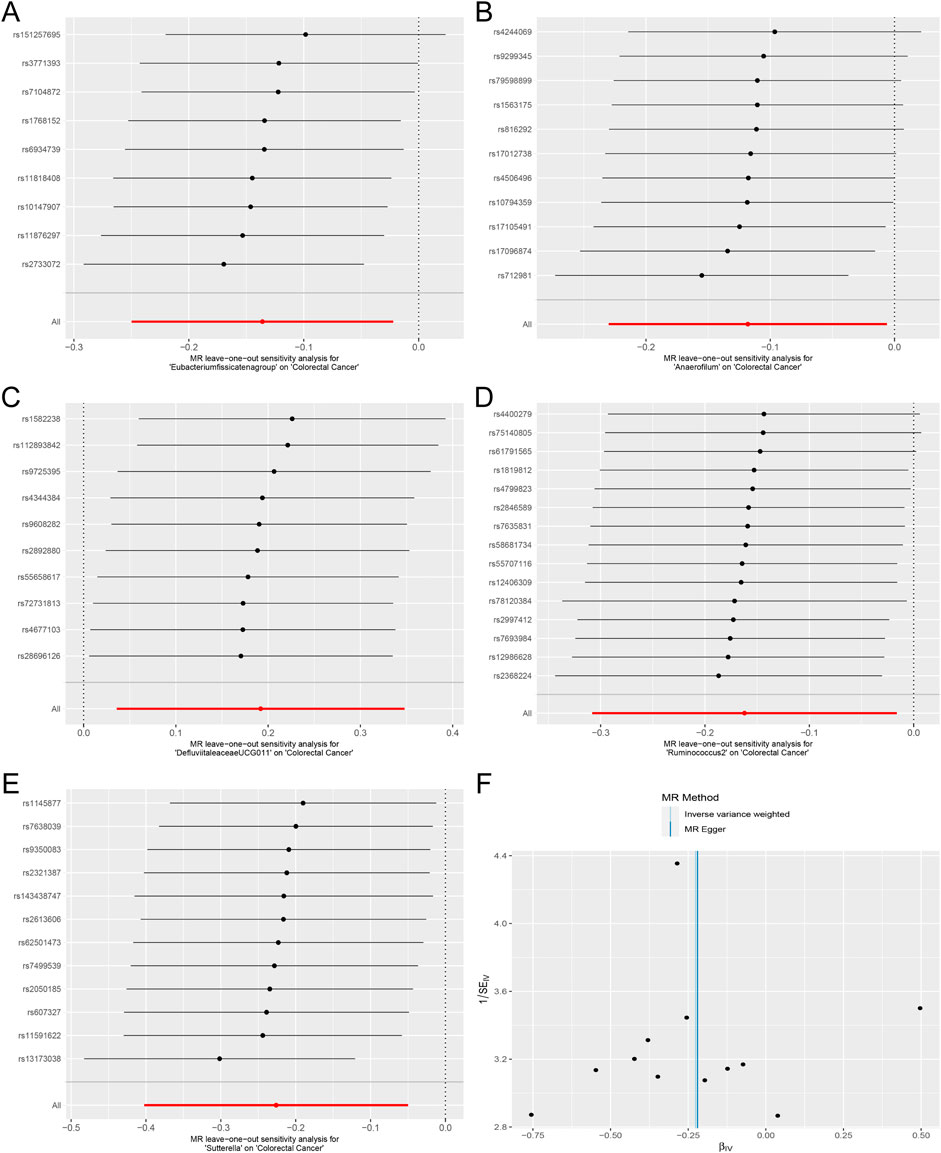
Figure 5. Forest plots of MR Leave-one-out sensitivity analysis and the funnel plot. (A). MR Leave-one-out sensitivity analysis for Eubacterium fissicatena group on Colorectal Cancer. (B). MR Leave-one-out sensitivity analysis for Anaerofilum on Colorectal Cancer. (C). MR Leave-one-out sensitivity analysis for Defluviitaleaceae UCG011 on Colorectal Cancer. (D). MR Leave-one-out sensitivity analysis for Ruminococcus 2 on Colorectal Cancer. (E). MR Leave-one-out sensitivity analysis for Sutterella on Colorectal Cancer. (F). The funnel plot of Defluviitaleaceae UCG011 on Colorectal Cancer.).
Hub gene and gene functions
A total of 57 SNPs associated with the potential relationship between colorectal cancer and the five microbial genera were included in the MR analysis. Using the R package, we identified all genes within 50 base pairs of these SNPs, resulting in a total of 61 unique genes after removing duplicates. The list of genes is provided in Supplementary Table S4. Pathway analysis through KEGG and GO revealed enrichment in pathways such as the cAMP signaling pathway, synaptic membrane adhesion, cell adhesion, and neuron-to-neuron synapse (Figure 6). Using the STRING online tool and Cytoscape software, we identified five hub genes: NLGN1, GRIP1, PTPRD, CADM1, and DSCAM (Figure 7A). Among these genes, PTPRD and DSCAM are located near the SNP sites (rs112893842; rs55658617) analyzed in the Defluviitaleaceae UCG011 genus MR analysis. Analysis of the TCGA database revealed a significant decrease in PTPRD expression in colon cancer, while DSCAM showed a significant decrease in rectal cancer (PTPRD: p = 1.500E-02; DSCAM: p = 4.556E-02) (Figures 7B,C). Further analysis of the PTPRD gene promoter region’s methylation status using TCGA data revealed a significant increase in methylation levels in colon cancer tissues. Moreover, a significant correlation was observed between the methylation levels of the PTPRD gene promoter and the staging of colon cancer (methylation levels of the PTPRD: p = 1.624E-12) (Figures 7D,E). Survival analysis revealed a significant correlation between PTPRD gene expression and colon cancer survival time (Figure 7F).
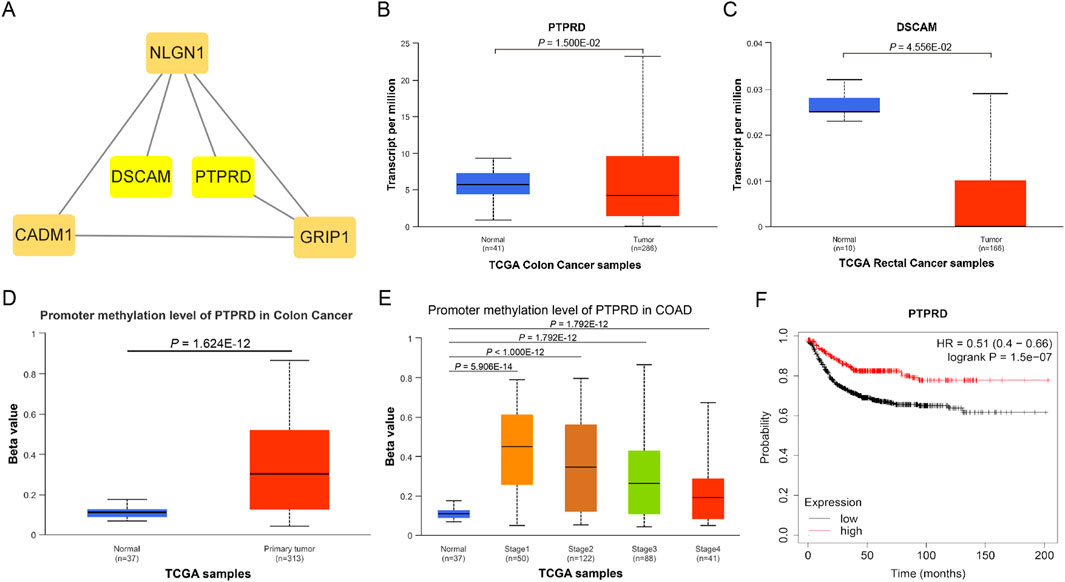
Figure 7. Hub genes and expression in TCGA samples. (A). Hub genes; (B). Expression of PTPRD in TCGA Colon cancer samples; (C). Expression of DSCAM in TCGA rectal cancer samples; (D). Promoter methylation level of PTPRD in TCGA Colon cancer samples (Normal to Tumor); (E). Promoter methylation level of PTPRD in TCGA Colon cancer samples (Normal to Different stages); (F). KM survival curve of PTPRD in TCGA Colon cancer samples.
Discussion
Colorectal cancer is one of the most common malignant tumors of the digestive tract, with incidence and mortality rates rising annually (Bray et al., 2024; Siegel et al., 2022). Recent studies have shown that the gut microbiome plays a complex role in the development and progression of colorectal cancer (Wong and Yu, 2023). Here, we conducted a two-sample Mendelian Randomization analysis and found a potential association between five gut microbial genera and CRC: E. fissicatena group, Anaerofilum, Defluviitaleaceae UCG011, Ruminococcus 2, and Sutterella. This aligns with findings from previous research (Ni, Li, Zhang, Xu, Wei, Feng, Zhao, Zhang, Zhang, Shen and Li, 2022; Xiang et al., 2023). However, our study identified Defluviitaleaceae UCG011 as a unique risk factor for CRC, underscoring its potential role in promoting the occurrence and development of colorectal cancer.
Defluviitaleaceae UCG011 is a genus within the Defluviitaleaceae family, belonging to the Firmicutes phylum, Clostridia class, and Lachnospirales order. Kawamoto et al. observed an increased abundance of Defluviitaleaceae in the intestines of patients with periodontitis, suggesting its potential involvement in the body’s inflammatory response (Kawamoto et al., 2021). Another study by Chen et al. found that bacteria from the Defluviitaleaceae family, particularly Defluviitaleaceae UCG011, influenced the immune-mediated disease Granulomatosis with Polyangiitis through CD11c in granulocytes, highlighting its role in human immune responses (Chen and Tang, 2023). Additionally, Wang et al. revealed a significant positive correlation between Defluviitaleaceae UCG011 and susceptibility to the immune-related disease ankylosing spondylitis (Wang et al., 2023). To the best of our knowledge, there is currently no literature reporting the role of Defluviitaleaceae UCG011 in CRC. Based on our research results, we speculate that the Defluviitaleaceae UCG011 genus may promote the occurrence and development of colorectal cancer by altering intestinal inflammation and immune responses. However, the specific mechanisms require more in-depth investigation.
To gain a deeper understanding of the link between the five specific microbial genera and colorectal cancer, we conducted a detailed analysis of genes located in close proximity to each SNP identified in our study. Our analysis focused on the results obtained from the MR study. Enrichment analysis revealed that these genes were significantly enriched in the cell signaling pathway and cAMP signaling pathway. Subsequent analysis identified five hub genes: NLGN1, GRIP1, PTPRD, CADM1, and DSCAM. Among them, the PTPRD and DSCAM genes are located near the SNP sites (rs112893842, rs55658617) analyzed in the Defluviitaleaceae UCG011 genus MR analysis. These findings suggest that this microbial genus may play a significant role in biological processes related to colorectal cancer.
PTPRD (Protein Tyrosine Phosphatase Receptor Type D) is a protein-coding gene that encodes a protein belonging to the protein tyrosine phosphatase (PTP) family. PTPs are known signaling molecules that regulate various cellular processes, including cell growth, differentiation, the cell cycle, and oncogenic transformation. Relevant pathways include protein-protein interactions at synapses and signal transmission across chemical synapses (Pulido et al., 1995; Veeriah et al., 2009). Through analysis of the TCGA database, we found that PTPRD is significantly downregulated in colon cancer tissues, suggesting that PTPRD may play a role in inhibiting colon cancer. Further analysis revealed a markedly increased methylation level of PTPRD in colon cancer samples, leading us to hypothesize that PTPRD inactivation due to methylation may promote the progression of colorectal cancer. DSCAM (Down Syndrome Cell Adhesion Molecule) is another protein-coding gene belonging to the immunoglobulin superfamily of cell adhesion molecules (Ig-CAM). It is involved in the development of the human central and peripheral nervous systems (Agarwala et al., 2000) and mediates intracellular signal transduction by activating MAPK8 and P38 MAP kinases (Liu et al., 2009; Ly et al., 2008). We examined the expression of these two genes in colorectal cancer using the TCGA database and found that PTPRD expression was significantly decreased in colon cancer, while DSCAM expression was significantly decreased in rectal cancer. This may be related to differences in intestinal bacterial diversity, and further investigation is needed to understand the precise mechanisms. Moreover, our analysis of PTPRD gene methylation levels using TCGA data revealed a substantial increase in methylation within the PTPRD promoter region of tumor tissues. Notably, this elevated methylation status was strongly associated with the stage of CRC. This suggests that Defluviitaleaceae UCG011 may influence the expression of PTPRD by altering methylation levels in its promoter region, thereby contributing to the development and progression of CRC. Survival analysis revealed a significant correlation between PTPRD gene expression and colon cancer prognosis, highlighting the potential role of Defluviitaleaceae UCG011 in modulating the initiation and progression of CRC.
While our research provides valuable insights into the underlying mechanisms involved in the development and progression of CRC, it does have several limitations. 1) The GWAS data used in this research are derived exclusively from European populations, and there is a known disparity in gut microbiota diversity across different populations. Therefore, the generalizability of our findings to other regional population may require further validation. 2) With the rapid evolution of technology, the functionality of genetic variations may change, and the SNPs included in our study may not be suitable for future research. 3) The information provided by the gut microbiota GWAS at the species or strain level is limited, reducing the precision of two-sample MR analyses in accurately inferring potential associations. 4) Despite preliminary MR results suggesting potential connections between gut microbial genera, genes, and CRC, this relationship loses significance after p-value correction, possibly due to limited sample size or a small effect size. The associated microbial genera and genes identified in this study require further in-depth research and clinical validation due to existing limitations in this study.
In conclusion, our study identified five gut microbial genera potentially linked to CRC, with only one genus, Defluviitaleaceae UCG011, identified as a risk factor for CRC. Through SNP annotation, we pinpointed two hub genes associated with Defluviitaleaceae UCG011: PTPRD and DSCAM. Our analysis suggests that these genes may play a role in developing CRC, and Defluviitaleaceae UCG011 may potentially influence the development and progression of CRC. Our study offers new insights on researching the mechanisms involved in the onset and progression of CRC. Therefore, we recommend that in-depth investigations be conducted into the role of gut microbial genera in CRC.
Data availability statement
Publicly available datasets were analyzed in this study. This data can be found here: MiBioGen database (https://mibiogen.gcc.rug.nl/) and Finngen database (https://www.finngen.fi/).
Ethics statement
Ethical approval was not required for the study involving humans in accordance with the local legislation and institutional requirements. Written informed consent to participate in this study was not required from the participants or the participants’ legal guardians/next of kin in accordance with the national legislation and the institutional requirements.
Author contributions
LW: Conceptualization, Data curation, Formal Analysis, Funding acquisition, Investigation, Methodology, Project administration, Resources, Software, Writing–original draft, Writing–review and editing. HW: Conceptualization, Data curation, Funding acquisition, Investigation, Methodology, Project administration, Resources, Writing–original draft, Writing–review and editing. FH: Data curation, Formal Analysis, Investigation, Writing–original draft, Writing–review and editing. SM: Conceptualization, Formal Analysis, Software, Writing–original draft, Writing–review and editing. X-YL: Data curation, Investigation, Resources, Writing–original draft, Writing–review and editing. B-FZ: Conceptualization, Funding acquisition, Investigation, Methodology, Project administration, Resources, Supervision, Validation, Visualization, Writing–original draft, Writing–review and editing. Y-HZ: Conceptualization, Funding acquisition, Methodology, Resources, Supervision, Visualization, Writing–original draft, Writing–review and editing. H-YL: Conceptualization, Methodology, Project administration, Resources, Software, Supervision, Validation, Visualization, Writing–original draft, Writing–review and editing.
Funding
The author(s) declare that financial support was received for the research, authorship, and/or publication of this article. Science and Technology Fund Project of Guizhou Health Commission, No. gzwkj 2023-042; gzwkj 2024-010. The Guizhou Provincial Science and Technology Projects, No. QKH JC-ZK [2024]-210. National Natural Science Foundation of China, No. 82060440.
Acknowledgments
We are enormously grateful to participants and investigators of the GWAS data. We want to acknowledge the participants and investigators of the FinnGen study and MiBioGen study. Our deep appreciation is extended to the National Natural Science Foundation of China (No. 82060440), Science and Technology Fund Project of Guizhou Health Commission (No. gzwkj 2023-042; gzwkj 2024-010), and the Guizhou Provincial Science and Technology Projects [No. QKH JC-ZK (2024)-210] for its invaluable financial support in enabling this study to be undertaken.
Conflict of interest
The authors declare that the research was conducted in the absence of any commercial or financial relationships that could be construed as a potential conflict of interest.
Publisher’s note
All claims expressed in this article are solely those of the authors and do not necessarily represent those of their affiliated organizations, or those of the publisher, the editors and the reviewers. Any product that may be evaluated in this article, or claim that may be made by its manufacturer, is not guaranteed or endorsed by the publisher.
Supplementary material
The Supplementary Material for this article can be found online at: https://www.frontiersin.org/articles/10.3389/fgene.2024.1379003/full#supplementary-material
SUPPLEMENTARY TABLE S1 | Details and F-statistics for Each SNP in the Exposure.
SUPPLEMENTARY TABLE S2 | MR Analysis Results for all gut Microbial Genera.
SUPPLEMENTARY TABLE S3 | FDR of IVW method.
SUPPLEMENTARY TABLE S4 | Nearest Gene to SNPs Associated with the Five Microbial Genera Potentially Related to Colorectal Cancer.
References
Agarwala, K. L., Nakamura, S., Tsutsumi, Y., and Yamakawa, K. (2000). Down syndrome cell adhesion molecule DSCAM mediates homophilic intercellular adhesion. Brain Res. Mol. Brain Res. 79, 118–126. Epub 2000/08/05. doi:10.1016/s0169-328x(00)00108-x
Ashburner, M., Ball, C. A., Blake, J. A., Botstein, D., Butler, H., Cherry, J. M., et al. (2000). Gene ontology: tool for the unification of biology. The Gene Ontology Consortium. Nat. Genet. May 25, 25–29. Epub 2000/05/10. doi:10.1038/75556
Avuthu, N., and Guda, C. (2022). Meta-analysis of altered gut microbiota reveals microbial and metabolic biomarkers for colorectal cancer. Microbiol. Spectr. 31, 10. Epub 2022/06/30. doi:10.1128/spectrum.00013-22
Benjamini, Y., and Hochberg, Y. (2024). Controlling the false discovery rate: a practical and powerful approac h to multiple testing. J. R. Stat. Soc. Ser. B 57, 289–300. Statistical Methodo logy. 1995/1/1/. doi:10.1111/j.2517-6161.1995.tb02031.x
Biller, L. H., and Schrag, D. (2021). Diagnosis and treatment of metastatic colorectal cancer: a review. JAMA 325, 669–685. Epub 2021/02/17. doi:10.1001/jama.2021.0106
Birney, E. (2022). Mendelian randomization. Cold Spring Harb. Perspect. Med. May 17, 12. Epub 2021/12/08. doi:10.1101/cshperspect.a041302
Bonder, M. J., Kurilshikov, A., Tigchelaar, E. F., Mujagic, Z., Imhann, F., Vila, A. V., et al. (2016). The effect of host genetics on the gut microbiome. Nat. Genet. Nov. 48, 1407–1412. Epub 2016/10/28. doi:10.1038/ng.3663
Bowden, J., Del Greco, M. F., Minelli, C., Davey Smith, G., Sheehan, N. A., and Thompson, J. R. (2016). Assessing the suitability of summary data for two-sample Mendelian randomization analyses using MR-Egger regression: the role of the I2 statistic. Int. J. Epidemiol. 45, 1961–1974. Epub 2016/09/13. doi:10.1093/ije/dyw220
Bowden, J., Del Greco, M. F., Minelli, C., Zhao, Q., Lawlor, D. A., Sheehan, N. A., et al. (2019). Improving the accuracy of two-sample summary-data Mendelian randomization: moving beyond the NOME assumption. Int. J. Epidemiol. 48, 728–742. Epub 2018/12/19. doi:10.1093/ije/dyy258
Bray, F., Ferlay, J., Soerjomataram, I., Siegel, R. L., Torre, L. A., and Jemal, A. (2018). Global cancer statistics 2018: GLOBOCAN estimates of incidence and mortality worldwide for 36 cancers in 185 countries. CA Cancer J. Clin. 68, 394–424. Epub 2018/09/13. doi:10.3322/caac.21492
Bray, F., Laversanne, M., Sung, H., Ferlay, J., Siegel, R. L., Soerjomataram, I., et al. (2024). Global cancer statistics 2022: GLOBOCAN estimates of incidence and mortality worldwide for 36 cancers in 185 countries. CA Cancer J. Clin. 74, 229–263. May-Jun. doi:10.3322/caac.21834
Burgess, S., Daniel, R. M., Butterworth, A. S., Thompson, S. G., and Consortium, E. P.-I. (2015). Network Mendelian randomization: using genetic variants as instrumental variables to investigate mediation in causal pathways. Int. J. Epidemiol. 44, 484–495. Epub 2014/08/26. doi:10.1093/ije/dyu176
Burgess, S., Thompson, S. G., and Collaboration, C. C. G. (2011). Avoiding bias from weak instruments in Mendelian randomization studies. Int. J. Epidemiol. 40, 755–764. Epub 2011/03/19. doi:10.1093/ije/dyr036
Chen, W., Wen, L., Bao, Y., Tang, Z., Zhao, J., Zhang, X., et al. (2022). Gut flora disequilibrium promotes the initiation of liver cancer by modulating tryptophan metabolism and up-regulating SREBP2. Proc. Natl. Acad. Sci. U. S. A. Dec 27, e2203894119. Epub 2022/12/20. doi:10.1073/pnas.2203894119
Chen, Y., and Tang, S. (2023). Gut microbiota and immune mediation: a Mendelian randomization study on granulomatosis with polyangiitis. Front. Immunol. 14, 1296016. Epub 2023/12/13. doi:10.3389/fimmu.2023.1296016
Dai, Z., Coker, O. O., Nakatsu, G., Wu, W. K. K., Zhao, L., Chen, Z., et al. (2018). Multi-cohort analysis of colorectal cancer metagenome identified altered bacteria across populations and universal bacterial markers. Microbiome 6, 70. Epub 2018/04/13. doi:10.1186/s40168-018-0451-2
Davies, N. M., Holmes, M. V., and Davey Smith, G. (2018). Reading Mendelian randomisation studies: a guide, glossary, and checklist for clinicians. BMJ, 362. Epub 2018/07/14. doi:10.1136/bmj.k601
Egger, M., Smith, G. D., and Phillips, A. N. (1997). Meta-analysis: principles and procedures. BMJ 315 (315), 1533–1537. Epub 1998/02/12. doi:10.1136/bmj.315.7121.1533
Eun, C. S., Kim, B. K., Han, D. S., Kim, S. Y., Kim, K. M., Choi, B. Y., et al. (2014). Differences in gastric mucosal microbiota profiling in patients with chronic gastritis, intestinal metaplasia, and gastric cancer using pyrosequencing methods. Helicobacter 19, 407–416. Epub 2014/07/24. doi:10.1111/hel.12145
Gao, X., Wang, Z., Liu, B., and Cheng, Y. (2023). Causal association of gut microbiota and esophageal cancer: a Mendelian randomization study. Front. Microbiol. 14, 1286598. Epub 2023/12/18. doi:10.3389/fmicb.2023.1286598
Gene Ontology, C., Aleksander, S. A., Balhoff, J., Carbon, S., Cherry, J. M., Drabkin, H. J., et al. (2023). The gene ontology knowledgebase in 2023. Genetics 4, 224. Epub 2023/03/04. doi:10.1093/genetics/iyad031
Hemani, G., Zheng, J., Elsworth, B., Wade, K. H., Haberland, V., Baird, D., et al. (2018a). The MR-Base platform supports systematic causal inference across the h uman phenome. Elife 7, e34408. doi:10.7554/eLife.34408
Hemani, G., Zheng, J., Elsworth, B., Wade, K. H., Haberland, V., Baird, D., et al. (2018b). The MR-Base platform supports systematic causal inference across the human phenome. Elife 30, e34408. Epub 2018/05/31. doi:10.7554/eLife.34408
Higgins, J. P., and Thompson, S. G. (2002). Quantifying heterogeneity in a meta-analysis. Stat. Med. 21, 1539–1558. Epub 2002/07/12. doi:10.1002/sim.1186
Kanehisa, M., and Goto, S. (2000). KEGG: kyoto encyclopedia of genes and genomes. Nucleic Acids Res. 28, 27–30. Epub 1999/12/11. doi:10.1093/nar/28.1.27
Kawamoto, D., Borges, R., Ribeiro, R. A., de Souza, R. F., Amado, P. P. P., Saraiva, L., et al. (2021). Oral dysbiosis in severe forms of periodontitis is associated with gut dysbiosis and correlated with salivary inflammatory mediators: a preliminary study. Front. Oral Health 2, 722495. Epub 2022/01/21. doi:10.3389/froh.2021.722495
Kurilshikov, A., Medina-Gomez, C., Bacigalupe, R., Radjabzadeh, D., Wang, J., Demirkan, A., et al. (2021). Large-scale association analyses identify host factors influencing human gut microbiome composition. Nat. Genet. Feb 53, 156–165. Epub 2021/01/20. doi:10.1038/s41588-020-00763-1
Kurki, M. I., Karjalainen, J., Palta, P., Sipilä, T. P., Kristiansson, K., Donner, K., et al. (2022). FinnGen: unique genetic insights from combining isolated population and national health register data. medrxiv. 2022:2022–2003.
Kurki, M. I., Karjalainen, J., Palta, P., Sipila, T. P., Kristiansson, K., Donner, K. M., et al. (2023). FinnGen provides genetic insights from a well-phenotyped isolated population. Nat. Jan. 613, 508–518. Epub 2023/01/19. doi:10.1038/s41586-022-05473-8
Larsson, S. C., Butterworth, A. S., and Burgess, S. (2023). Mendelian randomization for cardiovascular diseases: principles and applications. Eur. Heart J. 44, 4913–4924. Epub 2023/11/08. doi:10.1093/eurheartj/ehad736
Lawlor, D. A. (2016). Commentary: two-sample Mendelian randomization: opportunities and challenges. Int. J. Epidemiol. 45, 908–915. Epub 2016/07/19. doi:10.1093/ije/dyw127
Liu, G., Li, W., Wang, L., Kar, A., Guan, K. L., Rao, Y., et al. (2009). DSCAM functions as a netrin receptor in commissural axon pathfinding. Proc. Natl. Acad. Sci. U. S. A. 106, 2951–2956. Epub 2009/02/07. doi:10.1073/pnas.0811083106
Liu, W., Zhang, X., Xu, H., Li, S., Lau, H. C., Chen, Q., et al. (2021). Microbial community heterogeneity within colorectal neoplasia and its correlation with colorectal carcinogenesis. Gastroenterology 160, 2395–2408. Epub 2021/02/14. doi:10.1053/j.gastro.2021.02.020
Long, Y., Tang, L., Zhou, Y., Zhao, S., and Zhu, H. (2023). Causal relationship between gut microbiota and cancers: a two-sample Mendelian randomisation study. BMC Med. 21 (21), 66. Epub 2023/02/23. doi:10.1186/s12916-023-02761-6
Ly, A., Nikolaev, A., Suresh, G., Zheng, Y., Tessier-Lavigne, M., and Stein, E. (2008). DSCAM is a netrin receptor that collaborates with DCC in mediating turning responses to netrin-1. Cell. 133, 1241–1254. Epub 2008/07/01. doi:10.1016/j.cell.2008.05.030
Maere, S., Heymans, K., and Kuiper, M. (2005). BiNGO: a Cytoscape plugin to assess overrepresentation of gene ontology categories in biological networks. Bioinformatics 21 (21), 3448–3449. Epub 2005/06/24. doi:10.1093/bioinformatics/bti551
Mikshowsky, A. A., Gianola, D., and Weigel, K. A. (2017). Assessing genomic prediction accuracy for Holstein sires using bootstrap aggregation sampling and leave-one-out cross validation. J. Dairy Sci. Jan. 100, 453–464. Epub 2016/11/28. doi:10.3168/jds.2016-11496
Ni, J. J., Li, X. S., Zhang, H., Xu, Q., Wei, X. T., Feng, G. J., et al. (2022). Mendelian randomization study of causal link from gut microbiota to colorectal cancer. BMC Cancer 30 (22)–1371. Epub 2022/12/31. doi:10.1186/s12885-022-10483-w
Pritchard, J. K., and Przeworski, M. (2001). Linkage disequilibrium in humans: models and data. Am. J. Hum. Genet. 69, 1–14. doi:10.1086/321275
Pulido, R., Krueger, N. X., Serra-Pages, C., Saito, H., and Streuli, M. (1995). Molecular characterization of the human transmembrane protein-tyrosine phosphatase delta. Evidence for tissue-specific expression of alternative human transmembrane protein-tyrosine phosphatase delta isoforms. J. Biol. Chem. Mar. 24, 6722–6728. Epub 1995/03/24. doi:10.1074/jbc.270.12.6722
Sanna, S., van Zuydam, N. R., Mahajan, A., Kurilshikov, A., Vich Vila, A., Vosa, U., et al. (2019). Causal relationships among the gut microbiome, short-chain fatty acids and metabolic diseases. Nat. Genet. Apr 51, 600–605. Epub 2019/02/20. doi:10.1038/s41588-019-0350-x
Si, H., Yang, Q., Hu, H., Ding, C., Wang, H., and Lin, X. (2021). Colorectal cancer occurrence and treatment based on changes in intestinal flora. Semin. Cancer Biol. 70, 3–10. Epub 2020/05/15. doi:10.1016/j.semcancer.2020.05.004
Siegel, R. L., Miller, K. D., Fuchs, H. E., and Jemal, A. (2022). Cancer statistics, 2022. Ca. Cancer J. Clin. 72, 7–33. Epub 2022/01/13. doi:10.3322/caac.21708
Sung, H., Ferlay, J., Siegel, R. L., Laversanne, M., Soerjomataram, I., Jemal, A., et al. (2021). Global cancer statistics 2020: GLOBOCAN estimates of incidence and mortality worldwide for 36 cancers in 185 countries. CA Cancer J. Clin. 71, 209–249. Epub 2021/02/05. doi:10.3322/caac.21660
Swertz, M. A., Dijkstra, M., Adamusiak, T., van der Velde, J. K., Kanterakis, A., Roos, E. T., et al. (2010). The MOLGENIS toolkit: rapid prototyping of biosoftware at the push of a button. BMC Bioinforma. 11 S12. Epub 2011/01/19. doi:10.1186/1471-2105-11-S12-S12
Swertz, M. A., and Jansen, R. C. (2007). Beyond standardization: dynamic software infrastructures for systems biology. Nat. Rev. Genet. 8, 235–243. Epub 2007/02/14. doi:10.1038/nrg2048
Szklarczyk, D., Gable, A. L., Lyon, D., Junge, A., Wyder, S., Huerta-Cepas, J., et al. (2019). STRING v11: protein-protein association networks with increased coverage, supporting functional discovery in genome-wide experimental datasets. Nucleic Acids Res. Jan. 8 (47), D607–D613. Epub 2018/11/27. doi:10.1093/nar/gky1131
Thanikachalam, K., and Khan, G. (2019). Colorectal cancer and nutrition. Nutr. Jan. 14, 11. Epub 2019/01/17. doi:10.3390/nu11010164
van der Velde, K. J., Imhann, F., Charbon, B., Pang, C., van Enckevort, D., Slofstra, M., et al. (2019). MOLGENIS research: advanced bioinformatics data software for non-bioinformaticians. Bioinformatics 35 (35), 1076–1078. Epub 2018/08/31. doi:10.1093/bioinformatics/bty742
Veeriah, S., Brennan, C., Meng, S., Singh, B., Fagin, J. A., Solit, D. B., et al. (2009). The tyrosine phosphatase PTPRD is a tumor suppressor that is frequently inactivated and mutated in glioblastoma and other human cancers. Proc. Natl. Acad. Sci. U. S. A. 106, 9435–9440. Epub 2009/05/30. doi:10.1073/pnas.0900571106
Verbanck, M., Chen, C. Y., Neale, B., and Do, R. (2018). Detection of widespread horizontal pleiotropy in causal relationships inferred from Mendelian randomization between complex traits and diseases. Nat. Genet. May 50, 693–698. Epub 2018/04/25. doi:10.1038/s41588-018-0099-7
Wang, D., Li, R., Jin, Y., Shen, X., and Zhuang, A. (2023). The causality between gut microbiota and ankylosing spondylitis: insights from a bidirectional two-sample Mendelian randomization analysis. Int. J. Rheum. Dis. 26, 2470–2477. Epub 2023/10/25. doi:10.1111/1756-185X.14938
Wong, C. C., and Yu, J. (2023). Gut microbiota in colorectal cancer development and therapy. Nat. Rev. Clin. Oncol. 20, 429–452. Epub 2023/05/12. doi:10.1038/s41571-023-00766-x
Wong, S. H., and Yu, J. (2019). Gut microbiota in colorectal cancer: mechanisms of action and clinical applications. Nat. Rev. Gastroenterol. Hepatol. 16, 690–704. Epub 2019/09/27. doi:10.1038/s41575-019-0209-8
Xiang, Y., Zhang, C., Wang, J., Cheng, Y., Wang, L., Tong, Y., et al. (2023). Identification of host gene-microbiome associations in colorectal cancer patients using mendelian randomization. J. Transl. Med. 21, 535. Epub 2023/08/11. doi:10.1186/s12967-023-04335-9
Zheng, R. S., Zhang, S. W., Sun, K. X., Chen, R., Wang, S. M., Li, L., et al. (2023a). Cancer statistics in China, 2016. Zhonghua Zhong Liu Za Zhi Chin. J. Oncol. 45, 212–220. doi:10.3760/cma.j.cn112152-20220922-00647
Keywords: colorectal cancer, gut microbial genera, Mendelian randomization, potentiality, genes, single nucleotide polymorphisms
Citation: Wu L, Wu H, Huang F, Mu S, Li X-Y, Zhang B-F, Zhen Y-H and Li H-Y (2024) Mendelian randomization and bioinformatics unveil potential links between gut microbial genera and colorectal cancer. Front. Genet. 15:1379003. doi: 10.3389/fgene.2024.1379003
Received: 31 January 2024; Accepted: 11 November 2024;
Published: 21 November 2024.
Edited by:
Xuehua Wan, Nankai University, ChinaReviewed by:
Amir Ariff, University of New South Wales, AustraliaShaohua Chen, Guangxi Medical University Cancer Hospital, China
Copyright © 2024 Wu, Wu, Huang, Mu, Li, Zhang, Zhen and Li. This is an open-access article distributed under the terms of the Creative Commons Attribution License (CC BY). The use, distribution or reproduction in other forums is permitted, provided the original author(s) and the copyright owner(s) are credited and that the original publication in this journal is cited, in accordance with accepted academic practice. No use, distribution or reproduction is permitted which does not comply with these terms.
*Correspondence: Hai-Yang Li, bGloYWl5YW5nQGdtYy5lZHUuY24=; Yun-Huan Zhen, eXVuaHVhbnpoZW43MkAxNjMuY29t
†These authors have contributed equally to this work and share first authorship