- 1Centre for Interdisciplinary Research in Basic Sciences, Jamia Millia Islamia, New Delhi, India
- 2Department of Biotechnology, Faculty of Natural Sciences, Jamia Millia Islamia, New Delhi, India
- 3Department of Bioinformatics, Maulana Abul Kalam Azad University of Technology, Haringhata, West Bengal, India
- 4Department of Clinical Laboratory Sciences, College of Applied Medical Sciences, Najran University, Najran, Saudi Arabia
- 5Clinical Pharmacy Department, Umm Al-Qura University, Makkah, Saudi Arabia
- 6Department of Medical Laboratory Sciences, College of Applied Medical Sciences, Prince Sattam Bin Abdulaziz University, Alkharj, Saudi Arabia
- 7Department of Clinical Laboratory Sciences, College of Applied Medical Sciences, Al- Quwayiyah, Shaqra University, Riyadh, Saudi Arabia
- 8Department of Medical Laboratory Technology, College of Applied Medical Sciences, Taibah University, Medina, Saudi Arabia
- 9Forensic Medical Services Center, Ministry of Health, Qassim, Saudi Arabia
Background: The COVID-19 pandemic caused by SARS-CoV-2 has led to millions of deaths worldwide, and vaccination efficacy has been decreasing with each lineage, necessitating the need for alternative antiviral therapies. Predicting host–virus protein–protein interactions (HV-PPIs) is essential for identifying potential host-targeting drug targets against SARS-CoV-2 infection.
Objective: This study aims to identify therapeutic target proteins in humans that could act as virus–host-targeting drug targets against SARS-CoV-2 and study their interaction against antiviral inhibitors.
Methods: A structure-based similarity approach was used to predict human proteins similar to SARS-CoV-2 (“hCoV-2”), followed by identifying PPIs between hCoV-2 and its target human proteins. Overlapping genes were identified between the protein-coding genes of the target and COVID-19-infected patient’s mRNA expression data. Pathway and Gene Ontology (GO) term analyses, the construction of PPI networks, and the detection of hub gene modules were performed. Structure-based virtual screening with antiviral compounds was performed to identify potential hits against target gene-encoded protein.
Results: This study predicted 19,051 unique target human proteins that interact with hCoV-2, and compared to the microarray dataset, 1,120 target and infected group differentially expressed genes (TIG-DEGs) were identified. The significant pathway and GO enrichment analyses revealed the involvement of these genes in several biological processes and molecular functions. PPI network analysis identified a significant hub gene with maximum neighboring partners. Virtual screening analysis identified three potential antiviral compounds against the target gene-encoded protein.
Conclusion: This study provides potential targets for host-targeting drug development against SARS-CoV-2 infection, and further experimental validation of the target protein is required for pharmaceutical intervention.
1 Introduction
At present, severe acute respiratory syndrome coronavirus 2 (SARS-CoV-2) has spread rapidly, infecting ∼769 million people globally, including ∼6 million reported deaths as of 16 August 2023 (https://covid19.who.int/). Treatment options for COVID-19 disease include oral medications and intravenous therapy for mild and severe patients, while vaccines are primarily administered as a preventive measure (Ali et al., 2020; Morse et al., 2020; Martín-Sánchez et al., 2023). The use of vaccines initially curbed the SARS-CoV-2 pandemic. However, with the emergence of the Omicron variant (Fan et al., 2022), the efficacy of vaccination against the infection has decreased with each viral lineage. Antiviral drug candidates have provided effective treatment against viral infections, yet their use may be limited by the rapid evolutionary rate of viruses and the possibility of resistance emerging (Dai et al., 2020; Dolgin, 2021; Burki, 2022). Therefore, it is essential to continue the development of alternative antiviral therapies to combat the ongoing threat of emerging viral infections. Host-directed therapy (HDT) has gained momentum in the past decade (Tripathi et al., 2021). Host-targeting drugs with a low evolutionary divergence compared to viruses may avoid treatment failure. Similarities in motif sequence or protein structures between the virus and host proteins can provide clues for predicting virus–human protein–protein interactions (PPIs) (Mariano and Wuchty, 2017; Liu X. et al., 2021). Thus, investigating these PPIs between a virus and its human host could contribute to discovering the viruses’ potential human host target proteins (Liu X. et al., 2021).
Although finding intraspecies PPIs is common, predicting PPIs among different species is rare; therefore, this study may allow researchers to understand not only how a pathogenic protein interacts with its host on a molecular level but also how such interactions function in a more extensive cellular network (Lee et al., 2008). In this study, to elucidate the host–virus protein–protein interactions (HV-PPIs), a structure-based interaction approach was implemented to identify PPIs among human proteins (HPs) with known interactions that are structurally similar to viral proteins (Franzosa and Xia, 2011; Keskin et al., 2016; Rajasekharan et al., 2016). The primary limitation of using a structure-based interaction approach is the inadequate availability of three-dimensional structures and protein interaction of either pathogens or their host (Mariano and Wuchty, 2017). However, several studies have used a structural similarity approach to identify human–pathogen PPIs. Davis et al. were the first to demonstrate the use of 3D structural homology for predicting interspecies PPIs. They scanned the genomes of the host and pathogen for proteins similar to known putative interactions, using structural information, and filtered the remaining interactions based on the biological context for various human pathogens (Davis et al., 2007).
Similarly, Doolittle and Gomez (2011) predicted 502 interactions between HIV and human proteins by using the structure similarity between nine HIV-1 proteins and human proteins with known interactions, functional data from RNAi studies, and cellular component annotation from the Gene Ontology database (Doolittle and Gomez, 2011). Sagar et al. (2021) used a structural similarity approach to identify host–pathogen interactions between 10 Zika virus (ZIKV) proteins and their two distinct hosts, human and D. melanogaster (Sagar et al., 2021). Based on previous studies, it has been proposed that if there are pairs of structure-similar virus–host proteins with known interacting host proteins, the target host proteins may also interact with the viral proteins (Sagar et al., 2021). Furthermore, these target host proteins could either enhance the function of the virus or have an inhibitory effect. Hence, exploring the common pathways, molecular functions, biological processes, cellular compartments, and other properties of the host proteins interacting with the virus can help inhibit infectious disease. These virus–host-targeting proteins may act as a potential drug target for the design of antiviral inhibitors.
The present study used a structure-based similarity approach to predict human host proteins similar to SARS-CoV-2 (“hCoV-2”), followed by the identification of PPIs between hCoV-2 and its target human proteins from different protein interaction databases (referred to as “T_hCoV-2”). Additionally, overlapping genes were identified between the protein-coding genes of T_hCoV-2 and COVID-19-infected patient’s messenger RNA (mRNA) expression data obtained from the NCBI-GEO. The overlapped genes between target and infected groups (TIG-DEGs) were further processed to identify differentially expressed genes (DEGs). Pathway and Gene Ontology (GO) enrichment analyses, construction of PPI networks, and detection of hub gene modules for TIG-DEGs were performed. Last, structure-based virtual screening with an antiviral compound database was performed to identify potential hits against the target gene-encoded protein. The vision of the present study was to identify target proteins in humans that may act as host-targeting drug targets against SARS-CoV-2 infection. Further experimental validation of the target protein is required for pharmaceutical intervention against SARS-CoV-2.
2 Materials and methods
2.1 Viral protein structure collection
The experimentally determined three-dimensional structures of SARS-CoV-2 [envelope protein (PDB ID: 7K3G), main protease (PDB ID: 5R7Y), spike glycoproteins (PDB ID: 6VSB, 6VXX), HR2 domain (PDB ID: 6LVN, 6M1V), NSP2 (PDB ID: 7MSW, 7MSX), NSP3 (PDB ID: 6W02, 6W6Y, 6W9C), NSP7 (PDB ID: 6WTC), NSP8 (6M5I), NSP9 (PDB ID: 6W9Q, 6W4B, 6WCI), NSP12 (PDB ID: 6XEZ, 6M71), NSP13 (PDB ID: 5RL6), NSP14 (PDB ID: 7DIY), NSP15 (PDB ID: 6VWW, 7K0R), NSP16 (PDB ID: 6W4H), ORF3a (PDB ID: 6XDC), ORF7a (PDB ID: 6W37), and ORF8 (PDB ID: 7JTL)] were obtained from the RCSB Database (https://www.rcsb.org/) (Rose et al., 2017). The remaining coronavirus structures [membrane protein (M), NSP4, and ORF6] that are not available in the Protein Data Bank (PDB) were modeled using Contact-guided Iterative Threading ASSEmbly Refinement (C-I-TASSER) (https://zhanggroup.org/C-I-TASSER/), an extended version of I-TASSER for high-accuracy protein structure and function predictions (Zheng et al., 2021). The modeled structures of M protein, NSP4, and ORF6 were verified using the Structural Analysis Verification Server (SAVES) program (https://saves.mbi.ucla.edu/) and protein structure analysis (ProSA)-web program (https://prosa.services.came.sbg.ac.at/prosa.php?pdbCode=1zyb) (Wiederstein and Sippl, 2007). The structural quality (PROCHECK) (Laskowski et al., 1993), non-bonded interactions (ERRAT) (Colovos and Yeates, 1993), and energy profile or Z-score (ProSA using molecular mechanics force field) were thoroughly checked and calibrated. The Z-scores obtained for the M protein, NSP4, and ORF6 were close to the related native conformations regarding their residue length, as determined by X-ray and NMR structures available in the RCSB database. Additionally, nearly 83%–88% of residues in these respective modeled structures were found to occupy favorable allowed regions in the Ramachandran plot (Hooft et al., 1997). It indicates that the modeled structures are high quality and likely biologically relevant.
2.2 Identification of structurally similar proteins among SARS-CoV-2 and its host
The structurally similar human proteins of SARS-CoV-2 were obtained by submitting the PDB IDs of viral protein structures (either known or C-I-TASSER-generated) to the Dali webserver (http://ekhidna2.biocenter.helsinki.fi/dali/) (Holm, 2022). In this study, only the Homo sapiens proteins with structural similarity score (Z-score)
2.3 Refinement of predictions
The Dali database may contain multiple structures of the same proteins in the PDB, which could result in repetitive interaction predictions. This issue can arise with certain SARS-CoV-2 proteins with multiple PDB structures, leading to repeating similar predictions. Hence, duplicate PDB IDs were removed before converting them to UniProt accession ID and gene names for target prediction. In addition, multiple hCoV-2 proteins (human proteins structurally similar to SARS-CoV-2) can have common cellular partners. Among these, unique pairs of interactions between human UniProt accession and SARS-CoV-2 proteins were considered.
2.4 Prediction of SARS-CoV-2–host protein interactions
Human endogenous protein interactors of SARS-CoV-2 were identified by obtaining the target protein interactors of hCoV-2 during various cellular processes. These cellular partners of hCoV-2 were obtained from HPRD (https://www.hprd.org/), BioGRID (https://thebiogrid.org/), HIPPIE (http://cbdm-01.zdv.uni-mainz.de/∼mschaefer/hippie/), and MINT (https://mint.bio.uniroma2.it/) databases and are referred to as T_hCoV-2 (target protein interactors of hCOV-2) (Baolin et al., 2007; Licata et al., 2012; Alanis-Lobato et al., 2017; Oughtred et al., 2019). These datasets are from literature-curated interactions established through in vitro and/or in vivo methods among human proteins. These cellular proteins, known to interact with hCoV-2 proteins, are presumed to interact with SARS-CoV-2 proteins due to their structural similarities with hCoV-2 proteins.
2.5 Comparison with the pre-processed dataset and differentially expressed gene analysis
The target protein interactors of hCoV-2, referred to as T_hCoV-2, of each of the SARS-CoV-2 proteins, were merged into one dataset corresponding to their HUGO Gene Nomenclature Committee (HGNC) symbols (https://www.genenames.org/) by eliminating the duplicate gene IDs to avoid redundancy. Pre-processed data corresponding to COVID-19 mRNA expression profiling were obtained from the NCBI-GEO (https://www.ncbi.nlm.nih.gov/geo/) (Jha et al., 2022). The unique target genes of T_hCoV-2 are then compared with the COVID-19 patient gene sample dataset from which only the overlapping genes between targets and infected datasets were considered for further DEG analyses.
To identify DEGs between T-hCoV-2 and COVID-19 samples, a two-sample statistical t-test was used, followed by obtaining their log2 (fold change) and Benjamin–Hochberg (BH)
2.6 Pathway, Gene Ontology terms, and PPI network construction of TIG-DEGs
Pathway and GO term enrichment data for TIG-DEGs were compiled using various libraries, i.e., Kyoto Encyclopedia of genes and genomes (KEGG) and GO-biological process (BP), GO-molecular function (MF), and GO-cellular compartment (CC) terms available within the Enrichr database (https://maayanlab.cloud/Enrichr/) (Kuleshov et al., 2016). All the pathways and GO terms corresponding to p < 0.01 were determined as statistically significant.
The genes corresponding to the enrichment analysis were then merged into one dataset (eliminating duplicate gene names) and subjected to PPI network construction using the STRING v11.5 web-based tool (https://string-db.org/) (Szklarczyk et al., 2021). The construction of this PPI network was based on the highest confidence score >0.9, and it was visualized using Cytoscape v3.9.1 (Shannon et al., 2003). The topological properties of the PPI network were analyzed using the NetworkAnalyzer plugin in Cytoscape. Furthermore, the Molecular Complex Detection (MCODE) plugin was used to identify highly correlated gene clusters/modules within the PPI network. The parameters set in MCODE for cluster detection were as follows: “degree cutoff = 2,” “node score cutoff = 0.2,” “k-score = 2,” “max. depth = 100,” and “cut style = haircut.” The top-scoring genes in the PPI cluster were considered the hub genes.
2.7 Target identification and preparation for molecular docking
The RPS3 gene has the most significant interaction partners of the top-scoring PPI cluster genes. Ribosomal protein subunit 3 (RPS3) is a protein-coding gene involved in the viral mRNA translation pathway. Since the viruses exploited the ribosomal proteins and the ribosomal biogenic processes to facilitate their replication, the RPs have been considered effective targets for developing antiviral agents (Dong et al., 2021). The three-dimensional electron microscopy structure of the human ribosomal subunit, PDB ID: 6ZLW (resolution: 2.60 Å), bounded to the SARS-CoV-2 NSP1 protein, was downloaded from the Protein Data Bank (https://www.rcsb.org/) (Rose et al., 2017). The two α-helices of NSP1 (residues: 154–179) of SARS-CoV-2 directly interact with RPS3, RPS2, and the phosphate backbone of rRNA helix 18 (h18) of 40S ribosomal protein. This interaction with RPS3, RPS2, and h18 rigidly anchors NSP1, obstructing the mRNA entry channel. Therefore, for this study, we took a subcomplex comprising RPS3, RPS2, and h18 as the targets for the molecular docking studies.
2.8 Virtual screening and ligand structure preparation
An in silico high-throughput virtual screening of known therapeutic COVID-19 drugs from the PubChem library was obtained (https://pubchem.ncbi.nlm.nih.gov/#query=covid-19) (Kim et al., 2019). The total search results were filtered thrice for final docking studies. Following are the filters for the selection of final screened compounds: filter 1, database filters (molecular weights should be from 100 to 500 g/mol, rotatable bond count from 0 to 7, h-bond acceptor count from 0 to 10, the polar area from 0 to 150 Å2, and XlogP from −6.3 to 5); filter 2, calculation of physicochemical properties (no Lipinski’s rule violations, no lead likeness violations, and high GI absorption) using the SwissADME web server (http://www.swissadme.ch/) (Daina et al., 2017); and filter 3, calculation of pharmacokinetics and toxicological properties (cLogP, solubility, TPSA, drug-likeness, drug score, mutagenic, tumorigenic, irritant, and reproductive effective) using the Osiris Property Explorer (Sander, 2001).
The 3D structures of the final screened compounds were generated using the OpenBabel program (O Boyle et al., 2011). The 2D SDF files were used as input files for OpenBabel for converting them to 3D structures, followed by energy minimization in MMFF94 force field (Halgren, 1996). Finally, all the structures of ligands were converted to the PDBQT format with appropriate rotatable bonds.
2.9 Molecular docking
The PDBQT file of the subcomplex receptor was prepared using Kollman united atom charges for molecular docking study using AutoDockTools v 1.5.6 (Singh and Kollman, 1984; Morris et al., 2009). As the subcomplex receptor contained two proteins and one nucleic acid, the tentative binding site for the inhibitor is unknown. Hence, a blind docking study using AutoDock Vina (v1.1.2) was performed (Trott and Olson, 2009). Grid point spacing was set at 1 Å, grid point 56 was taken in each direction of the grid box, and the concerned box was centered at the subcomplex receptor. The ligands bind to two probable sites of the subcomplex receptor—one at the rRNA h18 (ligand-binding site-I) and another at the RPS3 protein (II). The best three conformations of each ligand at two binding locations were identified after cluster analysis according to their lowest binding energy. The receptor–ligand hydrogen bond interactions were analyzed using the Swiss PDB viewer (Guex and Peitsch, 1997) and visualized using PyMOL v 2.5 (the PyMOL Molecular Graphics System, Version 1.2r3pre, Schrödinger, LLC).
3 Result
3.1 Identification of structurally similar human proteins of SARS-CoV-2 and hCoV-2 host PPIs
We used the Dali Lite webserver to identify human host proteins that exhibit structural similarity to the proteins of SARS-CoV-2. Twenty SARS-CoV-2 proteins were selected for this study (17 proteins from known sources, i.e., available via the RCSB PDB, and 3 proteins predicted or modeled using the C-I-TASSER program) (Table 1). The viral protein structures were then submitted to the Dali webserver to compare 3D structural coordinates of virus and human host proteins. To make the study more comprehensive and avoid redundancy, all the structurally similar host proteins for each viral protein were merged into one dataset, and duplicates were removed. For example, for NSP3 of SARS-CoV-2, the human proteins structurally similar to these three PDB IDs: 6W02, 6W6Y, and 6W9C were merged into one column, and duplicate human PDB IDs were then removed. A similar protocol was followed for the other viral proteins, with more than one PDB ID obtained for this study. It resulted in the identification of 6,116 human proteins (hCoV-2) similar to 20 SARS-CoV-2 proteins (Table 2 and Supplementary Table S1).
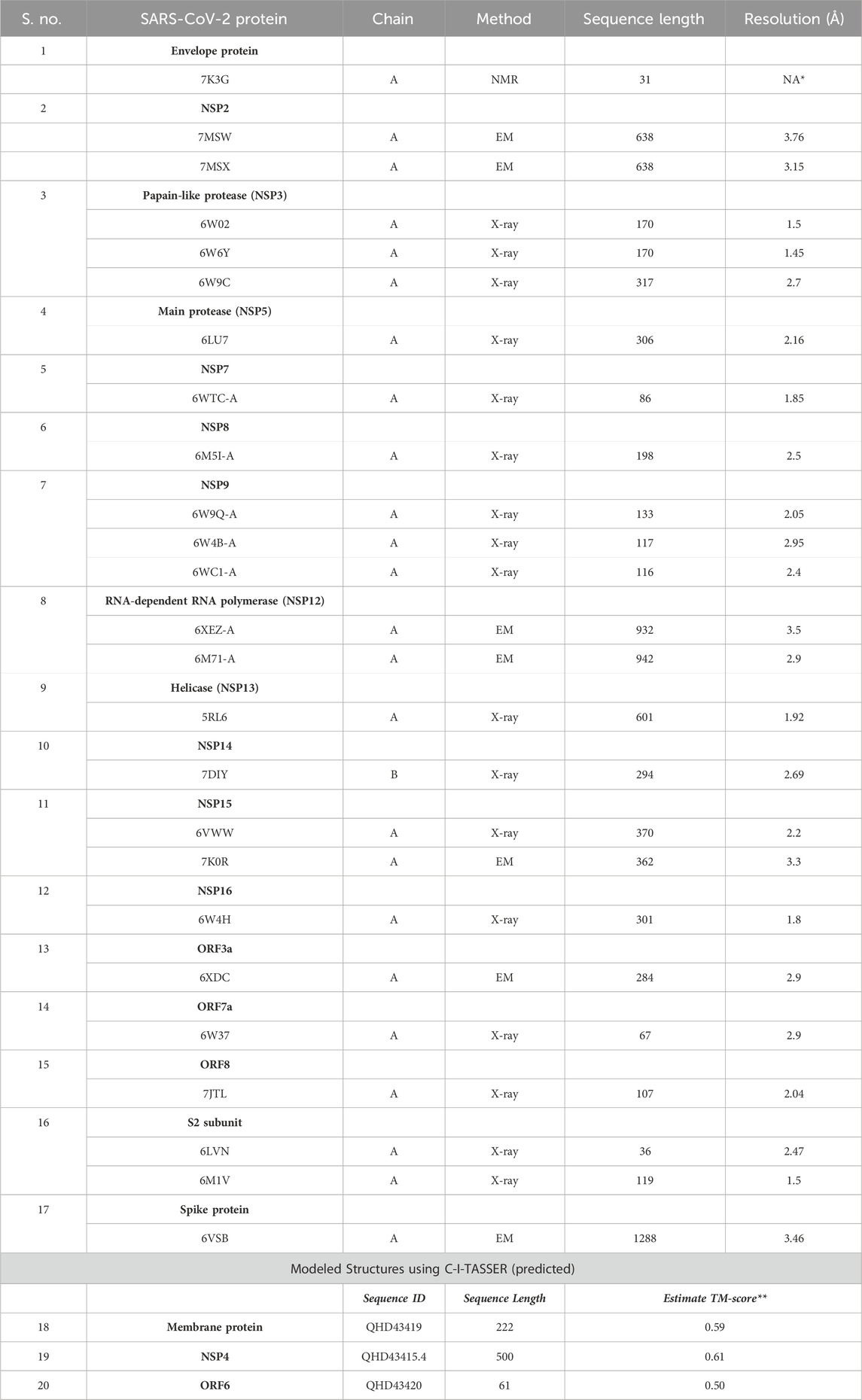
TABLE 1. List of 20 SARS-CoV-2 proteins selected for identifying structurally similar human host proteins.
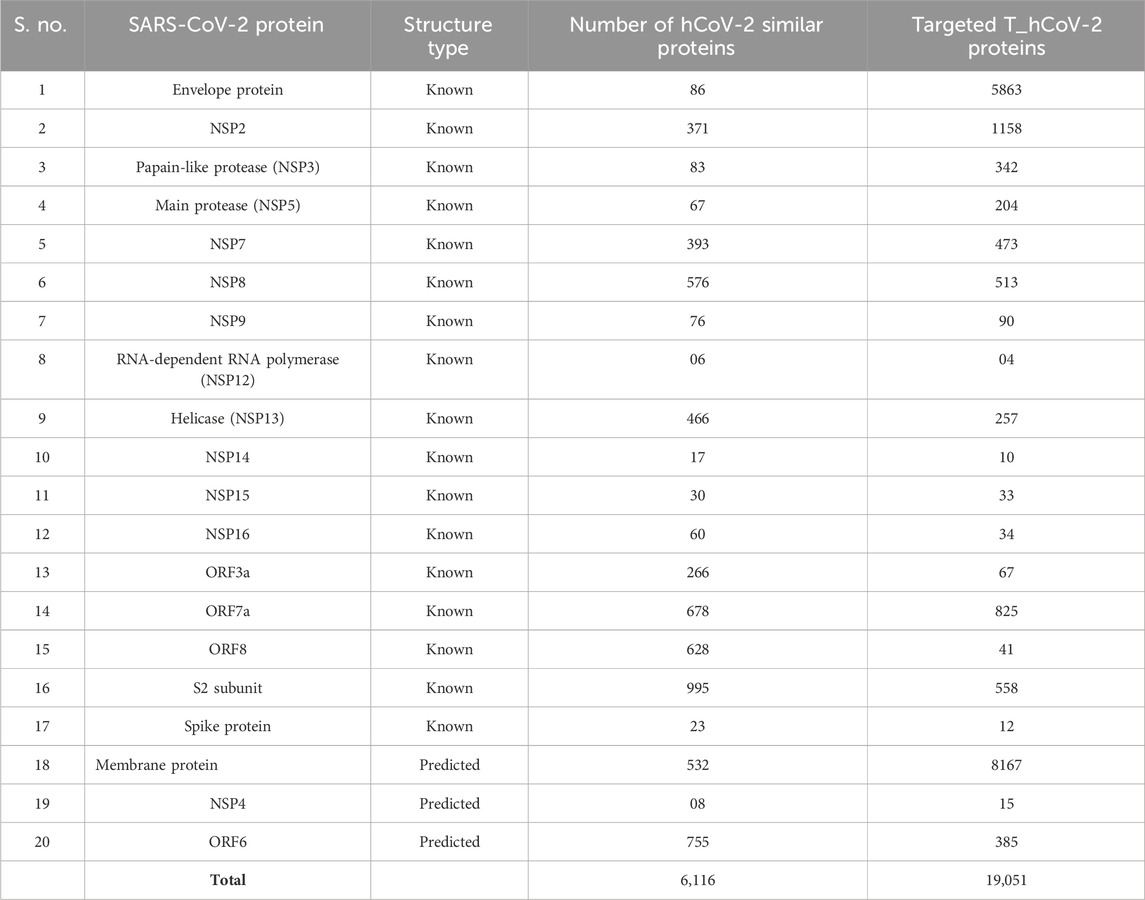
TABLE 2. Number of hCoV-2 structurally similar proteins (known and predicted) to the SARS-CoV-2 proteins and its unique host interactors known as T_hCoV-2 proteins.
Afterward, we identified all possible interacting partners of hCoV-2 proteins of the 20 proteins of SARS-CoV-2 from four different databases (HPRD, MINT, HIPPIE, and BioGRID). The target protein interactors of hCoV-2 from each database corresponding to 165,051 genes were merged into one unique dataset without duplicates. A total of 19,051 unique interactors were identified for these 20 SARS-CoV-2 proteins. These unique interactors are termed T_hCoV-2 proteins (Table 2 and Supplementary Table S2).
3.2 Comparison with the pre-processed dataset and differentially expressed gene analysis
The pre-processed SARS-CoV-2 mRNA expression profile (GSE164805) datasets comprised five controls, and 10 COVID-19 (five mild and five severe) samples were compared with the T_hCoV-2 genes. The overlapping genes between targets and mRNA datasets amounted to 8,336 genes (Figure 1A). These genes were then differentially expressed corresponding to
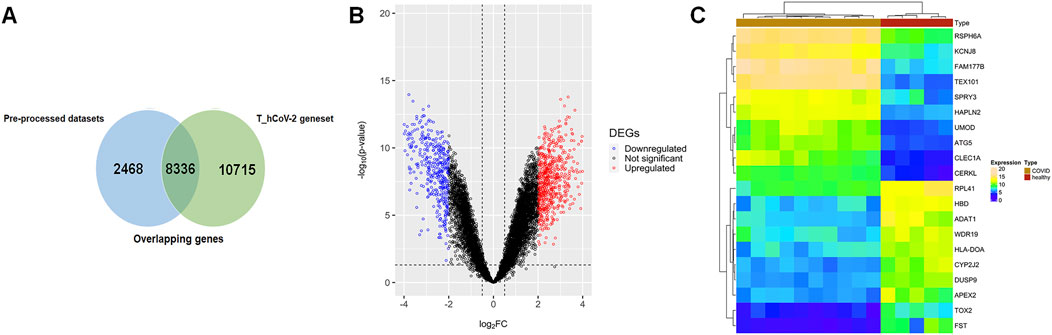
FIGURE 1. (A) Venn plot showing overlapping of pre-processed COVID-19 datasets across the T_hCoV-2 geneset. The blue-colored areas represent genesets pertaining to mild and severe infected COVID-19 microarray data, and green-colored areas represent genesets of T_hCOV-2. (B) Volcano plots show the distribution of significant (colored plots) and non-significant (black-colored points) genes in the microarray group. (C) Annotation heatmap showing the expression distribution of top 10 down- and upregulated DEGs across overlapping genes of COVID-19 and T_hCoV-2 datasets.
3.3 Pathway and Gene Ontology enrichment analyses
All the 1,120 TIG-DEGs participated in the Gene Ontology and pathway enrichment analyses. All the significant pathways and GO-BP, GO-MF, and GO-CC terms (
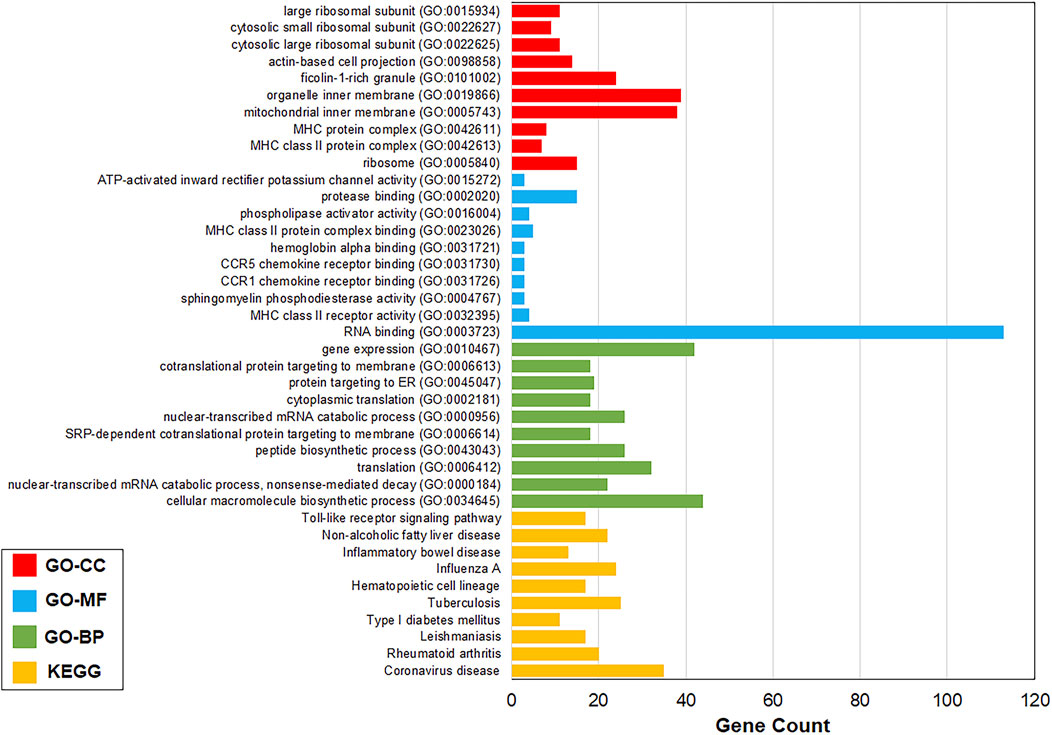
FIGURE 2. Top 10 most significant pathways and GO terms. Most significant pathways and GO-BP, GO-MF, and GO-CC terms were coronavirus disease (
3.4 PPI network analysis of TIG-DEGs
A total of 606 genes comprising all the pathways and GO terms
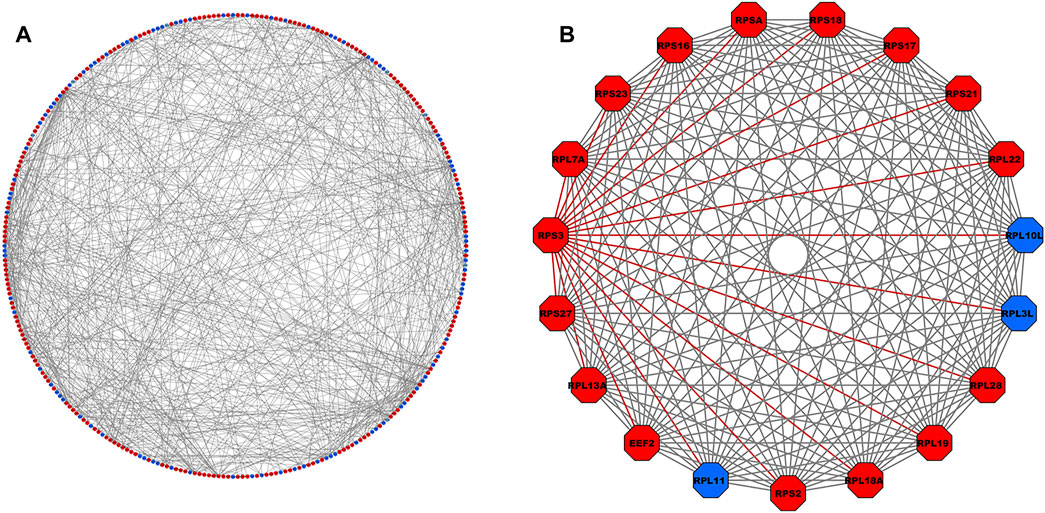
FIGURE 3. (A) PPI network comprising 302 nodes and 688 interacting edges constructed using the STRING database corresponding to interaction score >0.9. The red- and blue-colored nodes signify up- and downregulated proteins, respectively. (B) Top-scoring PPI module consisting 19 nodes and 171 edges, where the adjacent edges of the RPS3 gene with the highest degree (=25) is highlighted in red. PPI, protein–protein interaction.
3.5 Virtual screening of ligands
The PubChem library was searched with the keyword “COVID-19,” resulting in 1,709 compounds. The compounds were filtered out through three filtering processes (Martín-Sánchez et al., 2023): database filters (Ali et al., 2020), pharmacokinetic properties, and (Morse et al., 2020) toxicological properties. Through database filtering, only 886 candidates were selected that have a molecular weight between 100 and 500 g/mol, rotatable bond count between 0 and 7, h-bond acceptor count between 0 and 10, polar area between 0 and 150 Å2, and XlogP between −6.3 and 5. Government organizations, research and development, journal publishers, and NIH initiatives were selected as the data source category. The SwissADME webserver was accessed to calculate pharmacokinetic parameters. After the pharmacokinetic filtration process, 42 compounds that show zero violations of Lipinski’s rule, zero lead likeness violations, and high gastrointestinal (GI) were selected. To calculate toxicological parameters, the OSIRIS Property Explorer was used to predict the toxicity risks and drug scores of the 42 compounds. The program evaluated the risks of different side effects, such as mutagenic, irritant, tumorigenic, reproductive effects, and drug-related properties. Furthermore, the overall drug score was calculated by summing up various parameters such as cLogP, solubility (logS), molecular weight, drug-likeness, and toxicity risk. The final filtration process resulted in 28 compounds showing no toxicity risks and drug score values between 0.40 and 1.00, making it a good library for further docking studies (Table 3).
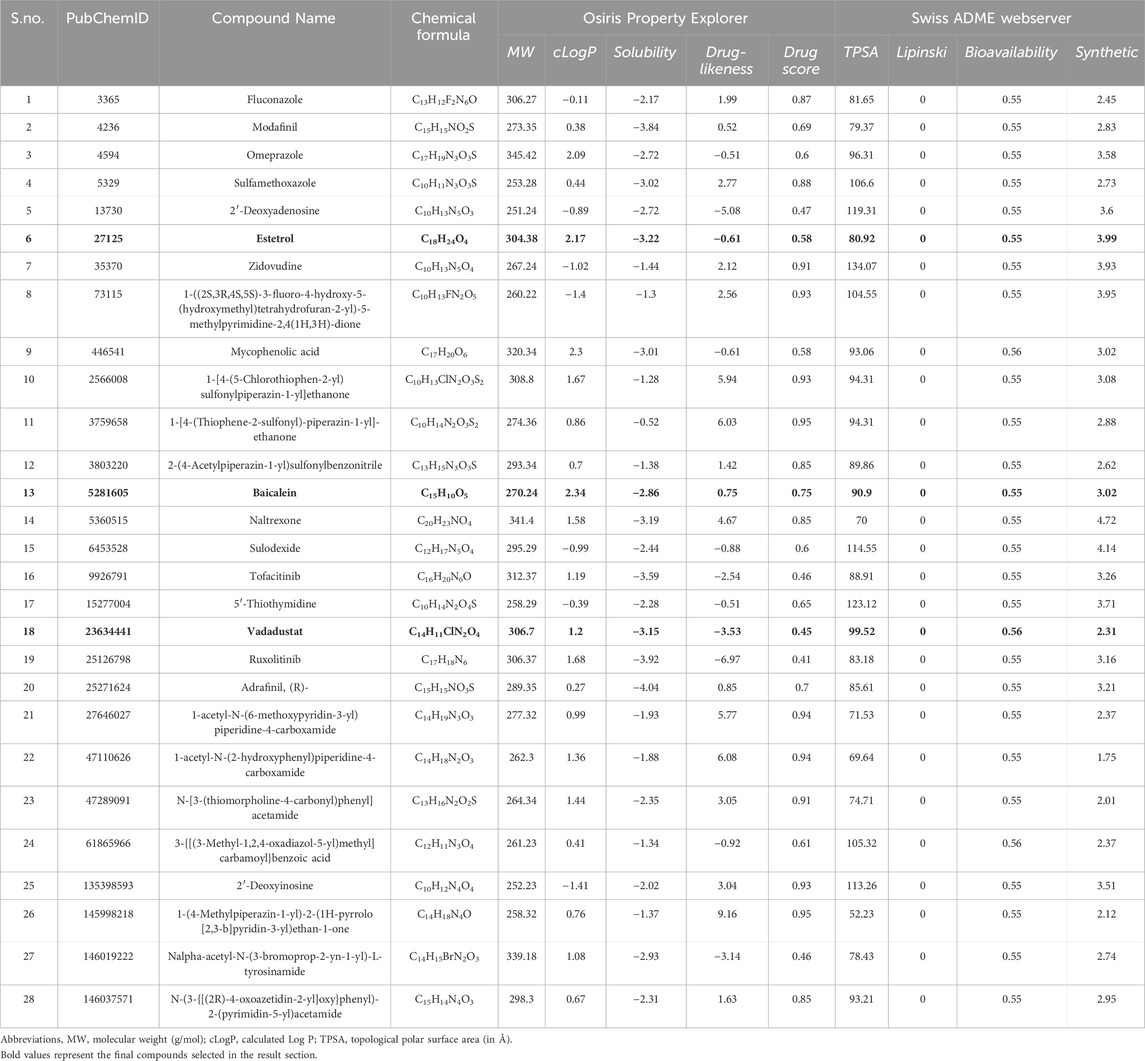
TABLE 3. Physiochemical properties of 28 selected drugs calculated after filtering thrice database filter, pharmacokinetic filter, and toxicological filter. The following drugs show no toxicity risks (mutagenicity, tumorigenic, irritant, and reproductive effective).
3.6 Molecular docking
In this study, blind docking was performed since the subcomplex receptor of ribosomal protein has no ligand-binding site (Figures 4A, B). The rotatable bonds of the 28 ligands were kept flexible, while the subcomplex macromolecule was adopted as a rigid structure. The analysis of the molecular docking results shows that most of the ligands screened bind into two active sites: (I) the center of RPS3 protein and (II) the head of rRNA helix 18 (Figure 4C). The top three binding poses with the best binding energies at each ligand-binding site are given in Table 4. The best binding energies of compound baicalein, vadadustat, and estetrol are observed at the head of the rRNA helix 18 site with binding energies −8.24, −8.01, and −7.98 kcal/mol, respectively. Similarly, baicalein, estetrol, and 3-{[(3-methyl-1,2,4-oxadiazol-5-yl)methyl]carbamoyl}benzoic acid show the best binding energies at the center of RPS3 protein with binding energies −7.54, −7.33, and −7.24 kcal/mol, respectively. Baicalein has been shown to have the highest binding pose for both ligand-binding sites. All the compounds form hydrogen bonds with the rRNA helix18 site that contains the following nucleotides: U607, C608, U609, G610, G611, G613, U630, A629, and U631, whereas the RPS3-binding site contains the following residues: Ala30, Gly33, Arg54, Arg94, Ala100, Gln101, Glu103, and Ser104 (Table 4). The interactions of baicalein, estetrol, and vadadustat (rRNA h18) are shown in Figure 5A, whereas the interactions of baicalein, estetrol, and 3-{[(3-methyl-1,2,4-oxadiazol-5-yl)methyl]carbamoyl}benzoic acid (RPS3 protein) are shown in Figure 5B. In Figure 5A, (i) baicalein binds in the region of rRNA h18 through h-bond interactions with U607, G609, G610, U630, and U631; (ii) estetrol shows h-bond interactions with U607, U609, G611, G613, and A629; and (iii) vadadustat forms h-bond interactions with U608, G610, G611, and U630. Meanwhile, in Figure 5B, (iv) baicalein binds in the region of RPS3 protein through h-bond interactions with residues Ala30, Gly33, Arg54, Arg94, and Ala100; (v) estetrol forms h-bond interactions with residues Arg94, Ala100, and Gln101; and (vi) 3-{[(3-methyl-1,2,4-oxadiazol-5-yl)methyl]carbamoyl}benzoic acid forms h-bond with residues Arg54, Arg94, Gln101, Glu103, and Ser104.
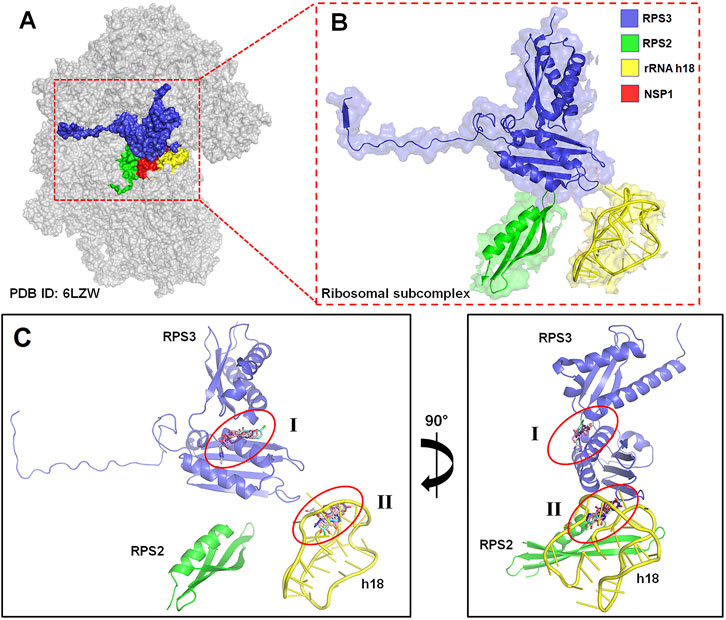
FIGURE 4. (A) Three-dimensional EM structure of the human 40S ribosomal subunit (PDB ID: 6ZLW), bounded to the ribosomal subcomplex and SARS-CoV-2 NSP1 protein (in red). (B) Magnified figure in cartoon representation of the ribosomal subcomplex comprised RPS3 (in blue), RPS2 (in green), and the phosphate backbone of rRNA helix 18 (h18) (in yellow) of 40S ribosomal protein. (C) Schematic representation of the two possible ligand-binding site pockets in the ribosomal subcomplex (I) in the center of RPS3 and (II) the head of rRNA h18 identified through blind docking.
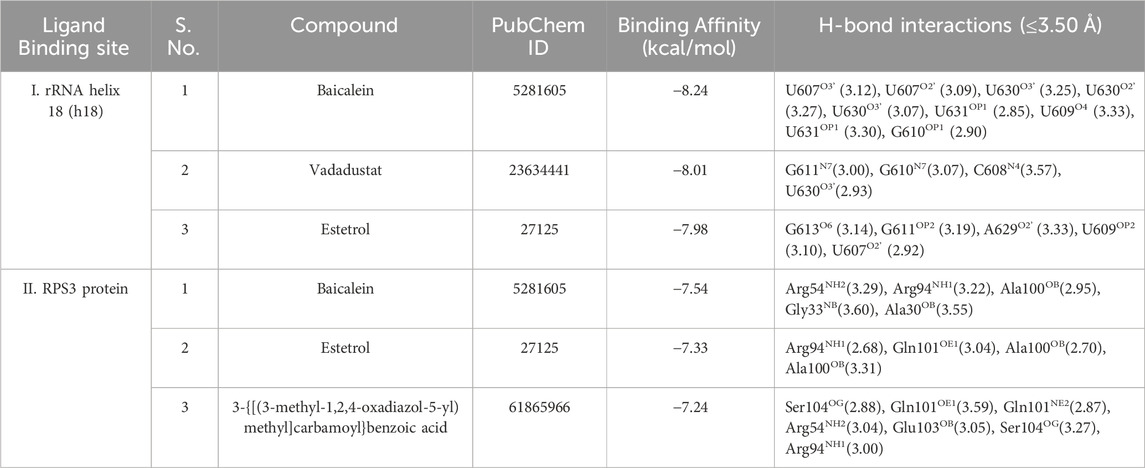
TABLE 4. Top three docking pose prediction results for each ligand-binding sites: (I) the head of rRNA helix 18 and (II) the center of RPS3 protein.
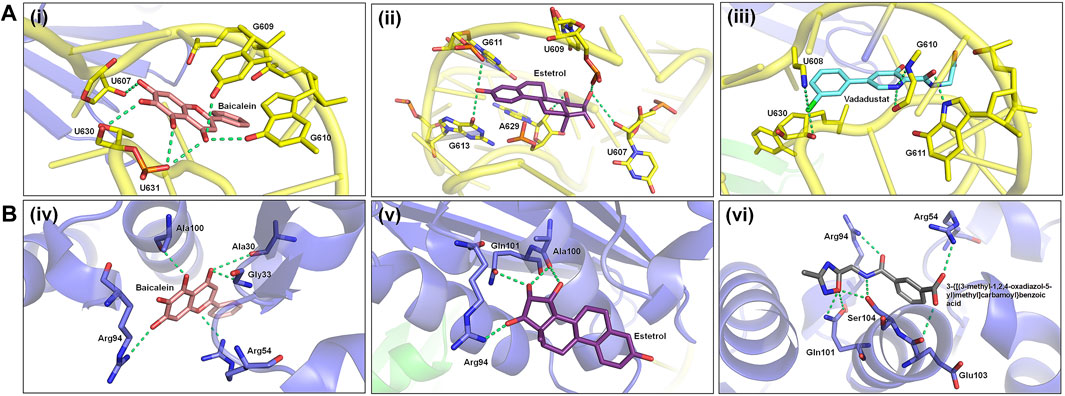
FIGURE 5. (A) Hydrogen bond interactions of baicalein, estetrol, and vadadustat (docked conformations) with the nucleotides of the phosphate backbone of h18. (B) Hydrogen bond interactions of baicalein, estetrol, and 3-{[(3-methyl-1,2,4-oxadiazol-5-yl)methyl]carbamoyl}benzoic acid (docked conformations) with active site residues of the RPS3 protein.
4 Discussion
COVID-19 has highlighted several therapeutic gaps in healthcare. One of the most significant gaps is the lack of effective antiviral drugs to treat the disease. Although some drugs have been repurposed to treat COVID-19, such as remdesivir, their efficacy is limited, and they are not a cure for the disease. Another gap is the lack of effective treatments for severe cases of COVID-19. While some patients recover from the disease with supportive care, there are still many who die from the disease. There is an urgent need for more effective treatments to reduce the mortality rate of COVID-19.
The present study used bioinformatics tools to explore the structural similarities between human host proteins and SARS-CoV-2 proteins and their interacting partners. This analysis led to the identification of an impressive number of 6,116 human proteins and 19,051 unique interacting partners of SARS-CoV-2 proteins. Further analyses of the target interactors of hCoV-2 proteins, combined with the differentially expressed genes (DEGs) of COVID-19 patients, revealed a total of 1,120 TIG-DEGs that participated in Gene Ontology and pathway enrichment analyses. Remarkably, the most significant pathways and GO terms identified were related to coronavirus disease, the cellular macromolecule biosynthetic process, RNA binding, and ribosome. Subsequently, the PPI network analysis identified RPS3 as the hub gene in a cluster of 19 nodes and 171 edges.
It should be noted that the approach of identifying host proteins structurally similar to viral proteins has been used before, as evidenced by Gordon et al. (2020). Nonetheless, the current study identified a much higher number of host proteins and interacting partners, due to the utilization of a different method of merging structurally similar host proteins and removing duplicates. Similarly, the approach of comparing the target interactors of hCoV-2 proteins with the DEGs of COVID-19 patients has been used before, as demonstrated by Blanco-Melo et al. (2020). Despite this, the present study identified a larger number of TIG-DEGs, indicating greater level of sensitivity in the methodology used.
The PPI network analysis of TIG-DEGs using Cytoscape software is a well-established method in systems biology that allows the identification of hub genes and protein complexes. The identification of RPS3 as a hub gene in the present study is particularly noteworthy, as it has also been identified by Prasad et al. (2021) as a hub gene in their PPI network analysis of SARS-CoV-2 interactors. The current study and findings from these two earlier studies suggest that RPS3 could be a potential therapeutic target for COVID-19.
Last, filtering potential drug candidates from an extensive library of compounds based on various pharmacokinetic and toxicological properties is a conventional strategy in drug discovery (Reyaz et al., 2021; Sultan et al., 2021; Sultan et al., 2022). This study identified three compounds—baicalein, vadadustat, and estetrol—with promising properties, such as binding affinity and residual interaction with RPS3, for COVID-19 treatment. These compounds could be further tested in vitro and in vivo for their efficacy. Although different research groups have tried to investigate that baicalein may act as an anti-SARS-CoV-2 drug through in vitro analysis, its safety and efficacy in SARS-CoV-2-infected transgenic animals have not been studied yet (Huang et al., 2020; Su et al., 2020; Liu H. et al., 2021; Dinda et al., 2023). However, Song et al. (2021) investigated the therapeutic effect of baicalein against SARS-CoV-2 both in vivo and in vitro (Song et al., 2021). Further experimental studies are recommended to prove that baicalein may act as an effective anti-COVID-19 molecule.
In summary, this study’s bioinformatics analysis provides novel insights into the biology of SARS-CoV-2 infection and potential therapeutic targets. Although some of the methodologies used have been used before, this study’s findings highlight the importance of leveraging multiple bioinformatics tools to identify host proteins, interacting partners, and potential drug candidates. Nonetheless, further experimental validation is necessary to confirm this study’s findings and facilitate the translation of these findings into clinically applicable interventions. This study has some strengths and some limitations. One of the major strengths of this study is the comprehensive and systematic approach toward identifying the host proteins that interact with SARS-CoV-2 proteins and their potential roles in COVID-19 pathogenesis. This study used multiple databases and tools to identify the interactors and DEGs in COVID-19 patients. This approach provides a more comprehensive understanding of the host–virus interactions and their potential roles in the disease pathogenesis. This study also identified significant pathways and GO terms that could provide potential targets for drug development. However, this study also has some limitations that need to be addressed. This study relied solely on in silico analysis and did not validate the identified DEGs or hub genes through experimental studies. Additionally, this study used only one mRNA expression profile dataset, which may not be sufficient to capture the diversity of gene expression patterns in COVID-19 patients. This study also did not investigate the functional roles of the identified hub gene containing RPS3, which limits the understanding of its potential importance in COVID-19 pathogenesis.
5 Conclusion
In conclusion, the current study provides a comprehensive and systematic approach to identifying potential therapeutic targets for COVID-19 by exploring the structural similarity of human host proteins to SARS-CoV-2 proteins and investigating their interactions and pathways. This study identified 19,051 unique interactors for SARS-CoV-2 proteins, 1,120 differentially expressed genes, and a significant hub gene containing RPS3. The screening of the PubChem library identified three compounds, baicalein, vadadustat, and estetrol, with the best binding energies and residual interaction with the target molecule. Overall, current findings suggest that exploring the human host proteins’ interactions and pathways can provide valuable insights for developing effective treatments for COVID-19.
Data availability statement
The dataset used in our study is publicly available and can be downloaded from the National Center for Biotechnology Information Gene Expression Omnibus under accession number GSE164805 (https://www.ncbi.nlm.nih.gov/geo/query/acc.cgi?acc=GSE164805).
Author contributions
AT: conceptualization, data curation, formal analysis, methodology, software, validation, visualization, writing–original draft, and writing–review and editing. AS: writing–original draft. PS: software, validation, and writing–review and editing. HB: validation and writing–review and editing. HA: writing–review and editing. AYA: writing–review and editing. AB: writing–review and editing. MH: writing–review and editing. BA: writing–review and editing. AAE: writing–review and editing. ASA: writing–review and editing. MH: writing–review and editing. KD: writing–review and editing. RD: conceptualization, project administration, supervision, validation, writing–original draft, and writing–review and editing.
Funding
The authors declare that no financial support was received for the research, authorship, and/or publication of this article.
Acknowledgments
Authors would like to acknowledge the support of the Deputy for Research and Innovation Ministry of Education, Kingdom of Saudi Arabia for this research through a grant (NU/IFC/02//MRC/-004) under the Institutional Funding Committee at Najran University, Kingdom of Saudi Arabia.
Conflict of interest
The authors declare that the research was conducted in the absence of any commercial or financial relationships that could be construed as a potential conflict of interest.
Publisher’s note
All claims expressed in this article are solely those of the authors and do not necessarily represent those of their affiliated organizations, or those of the publisher, the editors, and the reviewers. Any product that may be evaluated in this article, or claim that may be made by its manufacturer, is not guaranteed or endorsed by the publisher.
Supplementary material
The Supplementary Material for this article can be found online at: https://www.frontiersin.org/articles/10.3389/fgene.2024.1292280/full#supplementary-material
Abbreviations
COVID-19, coronavirus disease 2019; h18, rRNA helix 18; hCoV-2, human proteins structurally similar to SARS-CoV-2; HIV, human immunodeficiency virus; HPs, human proteins; HV-PPIs, host–virus protein–protein interactions; NSP, non-structural protein; ORF, open reading frame; RPS2, ribosomal protein subunit 2; RPS3, ribosomal protein subunit 3; SARS-CoV-2, severe acute respiratory syndrome coronavirus 2; T_hCoV-2, targets of hCoV-2; TIG-DEGs, targets and infected group DEGs; and ZIKV, Zika virus.
References
Alanis-Lobato, G., Andrade-Navarro, M. A., and Schaefer, M. H. (2017). HIPPIE v2.0: enhancing meaningfulness and reliability of protein–protein interaction networks. Nucleic Acids Res. 45 (D1), D408–D414. doi:10.1093/nar/gkw985
Ali, M. J., Hanif, M., Haider, M. A., Ahmed, M. U., Sundas, F., Hirani, A., et al. (2020). Treatment options for COVID-19: a review. Front. Med. 7, 480. doi:10.3389/fmed.2020.00480
Baolin, L., and Bo, H. (2007). “HPRD: a high performance rdf database,” in Network and parallel computing. Editors K. Li, C. Jesshope, H. Jin, and J. L. Gaudiot (Berlin, Heidelberg: Springer Berlin Heidelberg), 4672, 364–374. Lecture Notes in Computer Science. doi:10.1007/978-3-540-74784-0_37
Blanco-Melo, D., Nilsson-Payant, B. E., Liu, W. C., Uhl, S., Hoagland, D., Møller, R., et al. (2020). Imbalanced host response to SARS-CoV-2 drives development of COVID-19. Cell 181 (5), 1036–1045. doi:10.1016/j.cell.2020.04.026
Burki, T. K. (2022). The role of antiviral treatment in the COVID-19 pandemic. Lancet Respir. Med. 10 (2), e18. doi:10.1016/S2213-2600(22)00011-X
Colovos, C., and Yeates, T. O. (1993). Verification of protein structures: patterns of nonbonded atomic interactions. Protein Sci. 2 (9), 1511–1519. doi:10.1002/pro.5560020916
Dai, W., Zhang, B., Jiang, X. M., Su, H., Li, J., Zhao, Y., et al. (2020). Structure-based design of antiviral drug candidates targeting the SARS-CoV-2 main protease. Science 368 (6497), 1331–1335. doi:10.1126/science.abb4489
Daina, A., Michielin, O., and Zoete, V. (2017). SwissADME: a free web tool to evaluate pharmacokinetics, drug-likeness and medicinal chemistry friendliness of small molecules. Sci. Rep. 7 (1), 42717. doi:10.1038/srep42717
Davis, F. P., Barkan, D. T., Eswar, N., McKerrow, J. H., and Sali, A. (2007). Host-pathogen protein interactions predicted by comparative modeling. Protein Sci. 16 (12), 2585–2596. doi:10.1110/ps.073228407
Dinda, B., Dinda, M., Dinda, S., and De, U. C. (2023). An overview of anti-SARS-CoV-2 and anti-inflammatory potential of baicalein and its metabolite baicalin: insights into molecular mechanisms. Eur. J. Med. Chem. 258, 115629. doi:10.1016/j.ejmech.2023.115629
Dolgin, E. (2021). The race for antiviral drugs to beat COVID — and the next pandemic. Nature 592 (7854), 340–343. doi:10.1038/d41586-021-00958-4
Dong, H. J., Zhang, R., Kuang, Y., and Wang, X. J. (2021). Selective regulation in ribosome biogenesis and protein production for efficient viral translation. Arch. Microbiol. 203 (3), 1021–1032. doi:10.1007/s00203-020-02094-5
Doolittle, J. M., and Gomez, S. M. (2011). Mapping protein interactions between Dengue virus and its human and insect hosts. PLoS Negl. Trop. Dis. 5 (2), e954. doi:10.1371/journal.pntd.0000954
Fan, Y., Li, X., Zhang, L., Wan, S., Zhang, L., and Zhou, F. (2022). SARS-CoV-2 Omicron variant: recent progress and future perspectives. Signal Transduct. Target Ther. 7 (1), 141. doi:10.1038/s41392-022-00997-x
Franzosa, E. A., and Xia, Y. (2011). Structural principles within the human-virus protein-protein interaction network. Proc. Natl. Acad. Sci. 108 (26), 10538–10543. doi:10.1073/pnas.1101440108
Gordon, D. E., Jang, G. M., Bouhaddou, M., Xu, J., Obernier, K., White, K. M., et al. (2020). A SARS-CoV-2 protein interaction map reveals targets for drug repurposing. Nature 583 (7816), 459–468. doi:10.1038/s41586-020-2286-9
Guex, N., and Peitsch, M. C. (1997). SWISS-MODEL and the Swiss-Pdb Viewer: an environment for comparative protein modeling. Electrophoresis 18 (15), 2714–2723. doi:10.1002/elps.1150181505
Halgren, T. A. (1996). Merck molecular force field. I. Basis, form, scope, parameterization, and performance of MMFF94. J. Comput. Chem. 17 (5–6), 490–519. doi:10.1002/(sici)1096-987x(199604)17:5/6<490::aid-jcc1>3.0.co;2-p
Holm, L. (2022). Dali server: structural unification of protein families. Nucleic Acids Res. 50 (W1), W210–W215. doi:10.1093/nar/gkac387
Hooft, R. W. W., Sander, C., and Vriend, G. (1997). Objectively judging the quality of a protein structure from a Ramachandran plot. Bioinformatics 13 (4), 425–430. doi:10.1093/bioinformatics/13.4.425
Huang, S., Liu, Y., Zhang, Y., Zhang, R., Zhu, C., Fan, L., et al. (2020). Baicalein inhibits SARS-CoV-2/VSV replication with interfering mitochondrial oxidative phosphorylation in a mPTP dependent manner. Signal Transduct. Target Ther. 5 (1), 266. doi:10.1038/s41392-020-00353-x
Jha, P., Singh, P., Arora, S., Sultan, A., Nayek, A., Ponnusamy, K., et al. (2022). Integrative multiomics and in silico analysis revealed the role of ARHGEF1 and its screened antagonist in mild and severe COVID-19 patients. J. Cell Biochem. 123 (3), 673–690. doi:10.1002/jcb.30213
Keskin, O., Tuncbag, N., and Gursoy, A. (2016). Predicting protein–protein interactions from the molecular to the proteome level. Chem. Rev. 116 (8), 4884–4909. doi:10.1021/acs.chemrev.5b00683
Kim, S., Chen, J., Cheng, T., Gindulyte, A., He, J., He, S., et al. (2019). PubChem 2019 update: improved access to chemical data. Nucleic Acids Res. 47 (D1), D1102–D1109. doi:10.1093/nar/gky1033
Kuleshov, M. V., Jones, M. R., Rouillard, A. D., Fernandez, N. F., Duan, Q., Wang, Z., et al. (2016). Enrichr: a comprehensive gene set enrichment analysis web server 2016 update. Nucleic Acids Res. 44 (W1), W90–W97. doi:10.1093/nar/gkw377
Laskowski, R. A., MacArthur, M. W., Moss, D. S., and Thornton, J. M. (1993). PROCHECK: a program to check the stereochemical quality of protein structures. J. Appl. Crystallogr. 26 (2), 283–291. doi:10.1107/s0021889892009944
Lee, S. A., hsiung, C. C., Tsai, C. H., Lai, J. M., Wang, F. S., Kao, C. Y., et al. (2008). Ortholog-based protein-protein interaction prediction and its application to inter-species interactions. BMC Bioinforma. 9 (S12), S11. doi:10.1186/1471-2105-9-S12-S11
Licata, L., Briganti, L., Peluso, D., Perfetto, L., Iannuccelli, M., Galeota, E., et al. (2012). MINT, the molecular interaction database: 2012 update. Nucleic Acids Res. 40 (D1), D857–D861. doi:10.1093/nar/gkr930
Liu, H., Ye, F., Sun, Q., Liang, H., Li, C., Li, S., et al. (2021b). Scutellaria baicalensis extract and baicalein inhibit replication of SARS-CoV-2 and its 3C-like protease in vitro. J. Enzyme Inhib. Med. Chem. 36 (1), 497–503. doi:10.1080/14756366.2021.1873977
Liu, X., Huuskonen, S., Laitinen, T., Redchuk, T., Bogacheva, M., Salokas, K., et al. (2021a). SARS-CoV-2–host proteome interactions for antiviral drug discovery. Mol. Syst. Biol. 17 (11), e10396. doi:10.15252/msb.202110396
Mariano, R., and Wuchty, S. (2017). Structure-based prediction of host–pathogen protein interactions. Curr. Opin. Struct. Biol. 44, 119–124. doi:10.1016/j.sbi.2017.02.007
Martín-Sánchez, F. J., Martínez-Sellés, M., Molero García, J. M., Moreno Guillén, S., Rodríguez-Artalejo, F., Ruiz-Galiana, J., et al. (2023). Insights for COVID-19 in 2023. Rev. Esp. Quimioter. 36 (2), 114–124. doi:10.37201/req/122.2022
Morris, G. M., Huey, R., Lindstrom, W., Sanner, M. F., Belew, R. K., Goodsell, D. S., et al. (2009). AutoDock4 and AutoDockTools4: automated docking with selective receptor flexibility. J. Comput. Chem. 30 (16), 2785–2791. doi:10.1002/jcc.21256
Morse, J. S., Lalonde, T., Xu, S., and Liu, W. R. (2020). Learning from the past: possible urgent prevention and treatment options for severe acute respiratory infections caused by 2019-nCoV. ChemBioChem 21 (5), 730–738. doi:10.1002/cbic.202000047
O Boyle, N., Banck, M., James, C., Morley, C., Vandermeersch, T., and Hutchison, G. (2011). Open Babel: an open chemical toolbox. J. Cheminformatics 3, 33. doi:10.1186/1758-2946-3-33
Oughtred, R., Stark, C., Breitkreutz, B. J., Rust, J., Boucher, L., Chang, C., et al. (2019). The BioGRID interaction database: 2019 update. Nucleic Acids Res. 47 (D1), D529–D541. doi:10.1093/nar/gky1079
Prasad, K., AlOmar, S. Y., Alqahtani, S. A. M., Malik, M. Z., and Kumar, V. (2021). Brain disease network analysis to elucidate the neurological manifestations of COVID-19. Mol. Neurobiol. 58 (5), 1875–1893. doi:10.1007/s12035-020-02266-w
Pundir, S., Martin, M. J., and O Donovan, C.UniProt Consortium (2016). UniProt tools. Curr. Protoc. Bioinforma. 53 (1), 1.29.1–1.29.15. doi:10.1002/0471250953.bi0129s53
Rajasekharan, S., and Gupta, S. (2016). “Bioinformatics based approaches to study virus–host interactions during chikungunya virus infection,” in Chikungunya virus. Editors J. J. H. Chu, and S. K. Ang (New York, NY: Springer New York), 1426, 195–200. Methods in Molecular Biology. doi:10.1007/978-1-4939-3618-2_17
Reyaz, S., Tasneem, A., Rai, G. P., and Bairagya, H. R. (2021). Investigation of structural analogs of hydroxychloroquine for SARS-CoV-2 main protease (Mpro): a computational drug discovery study. J. Mol. Graph Model 109, 108021. doi:10.1016/j.jmgm.2021.108021
Ritchie, M. E., Phipson, B., Wu, D., Hu, Y., Law, C. W., Shi, W., et al. (2015). Limma powers differential expression analyses for RNA-sequencing and microarray studies. Nucleic Acids Res. 43 (7), e47. doi:10.1093/nar/gkv007
Rose, P. W., Prlić, A., Altunkaya, A., Bi, C., Bradley, A. R., Christie, C. H., et al. (2017). The RCSB protein data bank: integrative view of protein, gene and 3D structural information. Nucleic Acids Res. 45 (D1), D271–D281. doi:10.1093/nar/gkw1000
Sagar, S. K., Kumar, M., Singh, P., Sankhwar, S., and Dohare, R. (2021). Prediction of putative protein interactions between zika virus and its hosts using computational techniques. J. Commun. Dis. 53 (04), 84–96. doi:10.24321/0019.5138.202178
Sander, T. (2001). OSIRIS property explorer. Org Chem Portal. Available at: https://www.organic-chemistry.org/prog/peo/.
Shannon, P., Markiel, A., Ozier, O., Baliga, N. S., Wang, J. T., Ramage, D., et al. (2003). Cytoscape: a software environment for integrated models of biomolecular interaction networks. Genome Res. 13 (11), 2498–2504. doi:10.1101/gr.1239303
Singh, U. C., and Kollman, P. A. (1984). An approach to computing electrostatic charges for molecules. J. Comput. Chem. 5 (2), 129–145. doi:10.1002/jcc.540050204
Song, J., Zhang, L., Xu, Y., Yang, D., Zhang, L., Yang, S., et al. (2021). The comprehensive study on the therapeutic effects of baicalein for the treatment of COVID-19 in vivo and in vitro. Biochem. Pharmacol. 183, 114302. doi:10.1016/j.bcp.2020.114302
Su, H., Yao, S., Zhao, W., Li, M., Liu, J., Shang, W. J., et al. (2020). Anti-SARS-CoV-2 activities in vitro of Shuanghuanglian preparations and bioactive ingredients. Acta Pharmacol. Sin. 41 (9), 1167–1177. doi:10.1038/s41401-020-0483-6
Sultan, A., Ali, R., Ishrat, R., and Ali, S. (2022). Anti-HIV and anti-HCV small molecule protease inhibitors in-silico repurposing against SARS-CoV-2 M pro for the treatment of COVID-19. J. Biomol. Struct. Dyn. 40 (23), 12848–12862. doi:10.1080/07391102.2021.1979097
Sultan, A., Ali, R., Sultan, T., Ali, S., Khan, N. J., and Parganiha, A. (2021). Circadian clock modulating small molecules repurposing as inhibitors of SARS-CoV-2 M pro for pharmacological interventions in COVID-19 pandemic. Chronobiol Int. 38 (7), 971–985. doi:10.1080/07420528.2021.1903027
Szklarczyk, D., Gable, A. L., Nastou, K. C., Lyon, D., Kirsch, R., Pyysalo, S., et al. (2021). The STRING database in 2021: customizable protein–protein networks, and functional characterization of user-uploaded gene/measurement sets. Nucleic Acids Res. 49 (D1), D605–D612. doi:10.1093/nar/gkaa1074
Tripathi, D., Sodani, M., Gupta, P. K., and Kulkarni, S. (2021). Host directed therapies: COVID-19 and beyond. Curr. Res. Pharmacol. Drug Discov. 2, 100058. doi:10.1016/j.crphar.2021.100058
Trott, O., and Olson, A. J. (2009). AutoDock Vina: improving the speed and accuracy of docking with a new scoring function, efficient optimization, and multithreading. J. Comput. Chem. 31, 455–461. doi:10.1002/jcc.21334
Wiederstein, M., and Sippl, M. J. (2007). ProSA-web: interactive web service for the recognition of errors in three-dimensional structures of proteins. Nucleic Acids Res. 35, 407–410. doi:10.1093/nar/gkm290
Keywords: COVID-19, virus–host target proteins, structural-based similarity, PPI network, SARS-CoV-2
Citation: Tasneem A, Sultan A, Singh P, Bairagya HR, Almasoudi HH, Alhazmi AYM, Binshaya AS, Hakami MA, Alotaibi BS, Abdulaziz Eisa A, Alolaiqy ASI, Hasan MR, Dev K and Dohare R (2024) Identification of potential therapeutic targets for COVID-19 through a structural-based similarity approach between SARS-CoV-2 and its human host proteins. Front. Genet. 15:1292280. doi: 10.3389/fgene.2024.1292280
Received: 11 September 2023; Accepted: 08 January 2024;
Published: 02 February 2024.
Edited by:
Nimisha Ghosh, Siksha O Anusandhan University, IndiaReviewed by:
Mohsen Norouzinia, Shahid Beheshti University of Medical Sciences, IranXuedong An, China Academy of Chinese Medical Sciences, China
Copyright © 2024 Tasneem, Sultan, Singh, Bairagya, Almasoudi, Alhazmi, Binshaya, Hakami, Alotaibi, Abdulaziz Eisa, Alolaiqy, Hasan, Dev and Dohare. This is an open-access article distributed under the terms of the Creative Commons Attribution License (CC BY). The use, distribution or reproduction in other forums is permitted, provided the original author(s) and the copyright owner(s) are credited and that the original publication in this journal is cited, in accordance with accepted academic practice. No use, distribution or reproduction is permitted which does not comply with these terms.
*Correspondence: Ravins Dohare, ravinsdohare@gmail.com; Kapil Dev, kdev@jmi.ac.in