- 1Zhejiang Chinese Medical University, Hangzhou, China
- 2Anji County Hospital of Traditional Chinese Medicine, Anji, China
- 3First Affiliated Hospital, Zhejiang Chinese Medical University, Hangzhou, China
Background: Observational studies suggest a potential association between atmospheric particulate matter 2.5 (PM2.5) and osteoporosis, but a causal association is unclear due to the presence of confounding factors.
Methods: We utilized bone mineral density indices at four specific sites to represent osteoporosis: femoral neck (FN-BMD), lumbar spine (LS-BMD), forearm (FA-BMD), and heel (HE-BMD). The PM2.5 data was obtained from the UK Biobank database, while the datasets for FN-BMD, LS-BMD, and FA-BMD were obtained from the GEFOS database, and the dataset for HE-BMD was obtained from the EBI database. A two-sample Mendelian randomization analysis was conducted using mainly the inverse variance weighted method, horizontal pleiotropy and heterogeneity were also assessed.
Results: The results indicated that PM2.5 was not correlated with a decrease in FN-BMD (β: −0.305, 95%CI: −0.762, 0.153), LS-BMD (β: 0.134, 95%CI: −0.396, 0.666), FA-BMD (β: -0.056, 95%CI: −1.172,1.060), and HE-BMD (β: −0.084, 95%CI: −0.261,0.093). Additionally, acceptable levels of horizontal pleiotropy and heterogeneity were observed.
Conclusion: In contrast to most observational studies, our research did not discover a potential causal relationship between PM2.5 and the development of osteoporosis.
Introduction
Osteoporosis stands as a prevalent orthopedic condition, characterized by a decrease in the mineral and bone tissue content of the bone and an increased risk of fracture, it predominantly affects the elderly population, particularly postmenopausal women (Camacho et al., 2020). Fragility fracture is a primary complication of osteoporosis, with a mortality rate as high as 30% within 1 year following the occurrence of the fracture (Sabri et al., 2023). In China alone, with the progression of societal aging, the number of individuals afflicted with osteoporosis has exceeded 90 million, it is estimated that by the year 2035, the healthcare expenditure for the treatment of fractures caused by osteoporosis in China will reach a staggering 199.2 million dollar (Si et al., 2015; Wang et al., 2021).
PM2.5 refers to particulate matter in the atmosphere with a diameter equal to or smaller than 2.5 μm, generated from both human activities and natural environmental processes (Fermo et al., 2019). Due to its extremely small particle size, PM2.5 can be absorbed by the alveoli of the human lungs and enter the bloodstream, posing a significant health risk to people (Huff et al., 2019). Research indicates that PM2.5 can stimulate the release of inflammatory factors and induce cellular carcinogenesis, long-term exposure to PM2.5 has been closely associated with increased incidence of cardiovascular, respiratory, and neurological diseases (Pang et al., 2021).
Common risk factors for osteoporosis include advanced age, BMI, alcohol consumption, high-dose hormones and so on (Kanis et al., 2019; Arceo-Mendoza and Camacho, 2021). In recent years, the impact of living environment on osteoporosis has received extensive attention. As one of the prominent air pollutants, the causal relationship between PM2.5 and osteoporosis has been a subject of controversy, different observational studies and prospective cohort studies have produced conflicting results regarding this association (Chen et al., 2015; Lin et al., 2020; Qiao et al., 2020). This discrepancy may be influenced by confounding factors such as regional variations, lifestyle habits, and ethnic differences, which are difficult to control in traditional research studies (Wilson, 2019; Jones et al., 2020).
Mendelian randomization (MR) is a research method that utilizes genetic variation as a tool to assess the relationship between exposure and outcome. It provides an effective means to mitigate the impact of confounding factors in observational and randomized controlled studies (Bowden and Holmes, 2019). Based on the MR method, multiple SNPs representing PM2.5 exposure (rs1537371; rs77205736, etc.) have been confirmed to be associated with diseases such as hypothyroidism, heart palpitations, and stroke (Zhang et al., 2022). Currently, there is a lack of relevant studies that have conducted an association analysis between PM2.5 and osteoporosis. Therefore, in this study, we aim to investigate whether PM2.5 exposure is a risk factor for osteoporosis by employing a two-sample Mendelian randomization research method, examining the relationship between PM2.5 exposure and bone mineral density (BMD) at multiple sites femoral neck (FN-BMD), lumbar spine (LS-BMD), forearm (FA-BMD), and heel (HE-BMD)).
Methods
Research design
This study employed two-sample Mendelian randomization analysis using the pooled genome-wide association studies (GWAS) data set to evaluate the potential causal relationship between PM2.5 and osteoporosis. Additionally, sensitivity analysis was conducted to assess the robustness and reliability of the findings. The specific process was shown in Figure 1.
Data sources
The GWAS data on PM2.5 exposure used in this study were obtained from the United Kingdom Biobank database (www.ukbiobank.ac.uk), a comprehensive dataset published in 2010, which included a total of 423,796 participants from the European region. The data used in this study is derived from the ESCAPE project (European Study of Cohorts for Air Pollution Effects) (Eeftens et al., 2012).
In clinical practice, osteoporosis is primarily diagnosed using dual-energy X-ray absorptiometry. (DXA) (Camacho et al., 2020). The BMD data for the femoral neck, lumbar spine, and forearm were obtained from the data published by the Genetic Factors for Osteoporosis Consortium website (GEFOS) in 2015, which included a total of 53,236 participants of European ancestry (Zheng et al., 2015). The data on heel bone density was obtained from a large-scale GWAS study that included 583,314 participants of European ancestry (Loh et al., 2018). The above data can be downloaded from IEU OpenGWAS project. For specific data, see Supplementary Material S1.
Instrumental variables
Mendelian randomization analysis relies on three core principles that must be met: 1) The instrumental variable must have a strong correlation with the exposure factor; 2) The instrumental variable should not be associated with confounding factors; 3) The instrumental variable’s value can only affect the outcome variable through the exposure factor (Bowden and Holmes, 2019).
To satisfy the principles, we conducted SNPs that showed significant correlation with PM2.5 at the genome-wide level (p < 5*10–8, r2 < 0.001, and kb = 10,000) (Wu et al., 2020). Then, to mitigate the risk of weak instrumental variables, we employed F-statistic (F = R2 (n − k − 1)/k (1 −R2)) to assess their presence (F < 10 indicates the presence of weak instrumental variables (IVs)) (Burgess et al., 2011). After excluding SNPs in linkage disequilibrium, we harmonized the data for the exposure and outcome SNPs. We then performed a manual search using the PhenoScanner database (www.phenoscanner.medschl.cam.ac.uk/) to determine whether the included SNPs were associated with confounding factors (p < 1*10–5).
Analysis strategy
After screening the relevant SNPs, we used the MR-PRESSO method to test the outliers in all the results. If p < 0.05, the SNP was considered as an outlier SNP and deleted to reduce the level of pleiotropy caused by it. Then, we employed the Inverse Variance Weighted method (IVW) to combine the effect sizes of SNPs (using a random-effects model when the exposure was assessed by at least 3 SNPs; otherwise, a fixed-effects model was used). Additionally, we utilized the Weighted Median and MR-Egger methods as supplementary approaches to validate the results (Hemani et al., 2018). Finally, we used Cochrane’s Q text and MR-Egger method (by intercept tests) to test heterogeneity and horizontal pleiotropy (Burgess and Thompson, 2017). In addition, the leave-one-out analysis was carried out by sequentially eliminating each instrumental variable to assess whether any single SNP disproportionately influenced the results.
Since the outcome was a continuous variable, this study reports the β values and their 95% confidence intervals. All statistical analyses were conducted using R software (version 4.0.3) with the assistance of the “TwoSampleMR” and “MR-PRESSO” packages. In addition, to exclude the interference of the false positive rate, we use the Bonferroni method to re-correct the threshold (p < 0.0125 is statistically significant).
Results
After removing SNPs in linkage disequilibrium, we identified 8 SNPs that were significantly associated with PM2.5 exposure in the GWAS studies. We manually searched for confounding SNPs and excluded two confounding SNPs (rs114708313 and rs77205736) that was related to rheumatoid arthritis and BMI. After matching with the bone density data of the four sites, we obtained a final set of 6 IVs (specific data in Table 1). After calculating, all IVs yielded F-values greater than 10, indicating the absence of weak IVs.
As shown in Table 2, the IVW suggests that the exposure to PM2.5 environment has no correlation with the changes in FN-BMD (β: −0.305, 95%CI: −0.762, 0.153; P: 0.191), LS-BMD (β: 0.134, 95%CI: −0.396, 0.666; P: 0.618), FA-BMD (β: −0.056, 95%CI: −1.172,1.060; P: 0.922), and HE-BMD (β: −0.084, 95%CI: −0.261,0.093; P: 0.353). The weighted median method and MR-Egger also showed the same results (shown in Figure 2). Both the Cochrane’s Q test and the MR-Egger method indicate the absence of horizontal pleiotropy and heterogeneity were observed (specific data in Table 3 and Figure 3). Furthermore, during the sensitivity analysis of the Mendelian randomization (MR) results using the leave-one-out method, we did not observe any causal shift (shown in Figure 4).
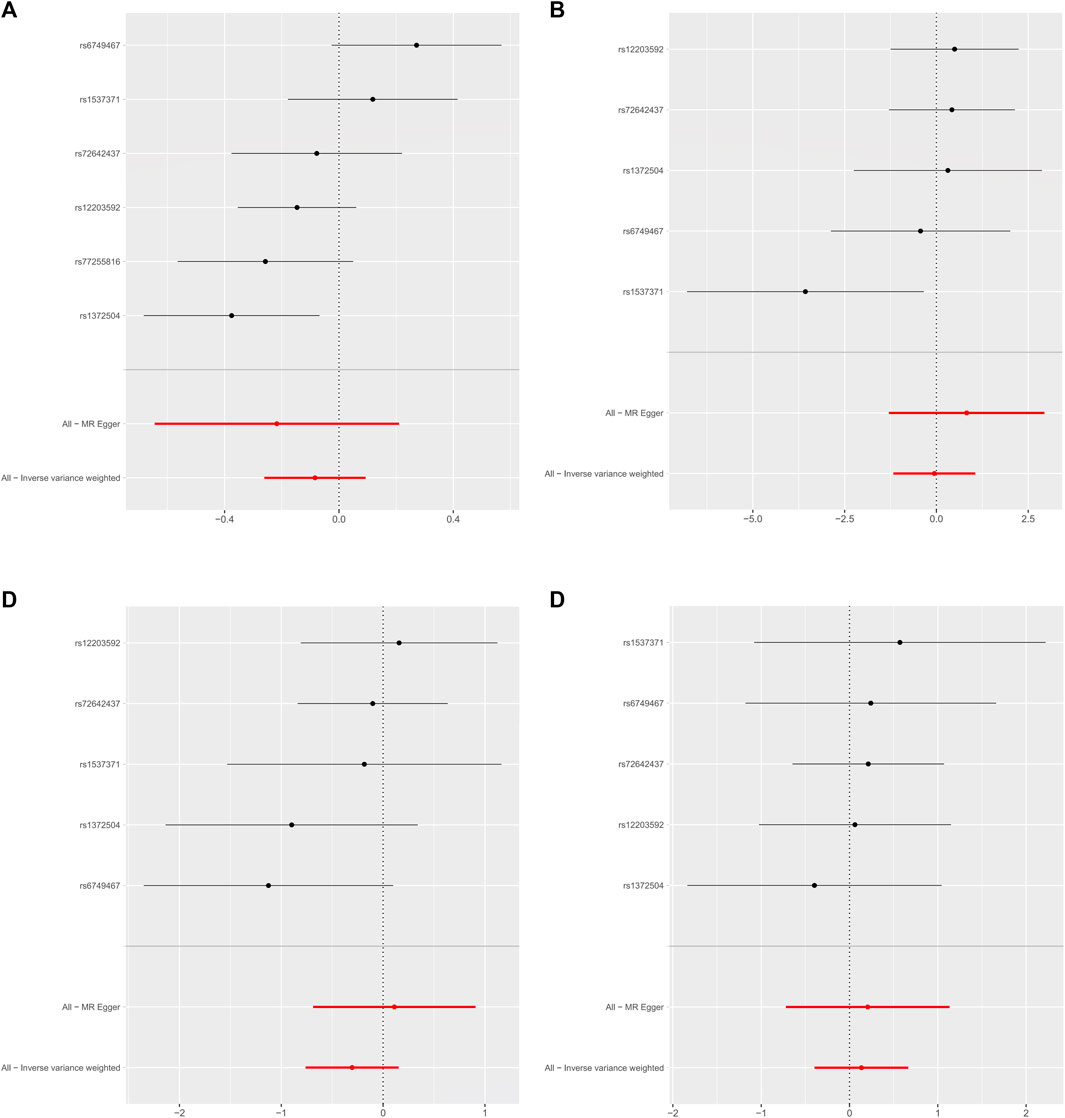
FIGURE 2. Forest plot of mendelian randomization Analysis. (A) HE-BMD, (B) FA-BMD, (C) FN-BMD, (D) LS-BMD.
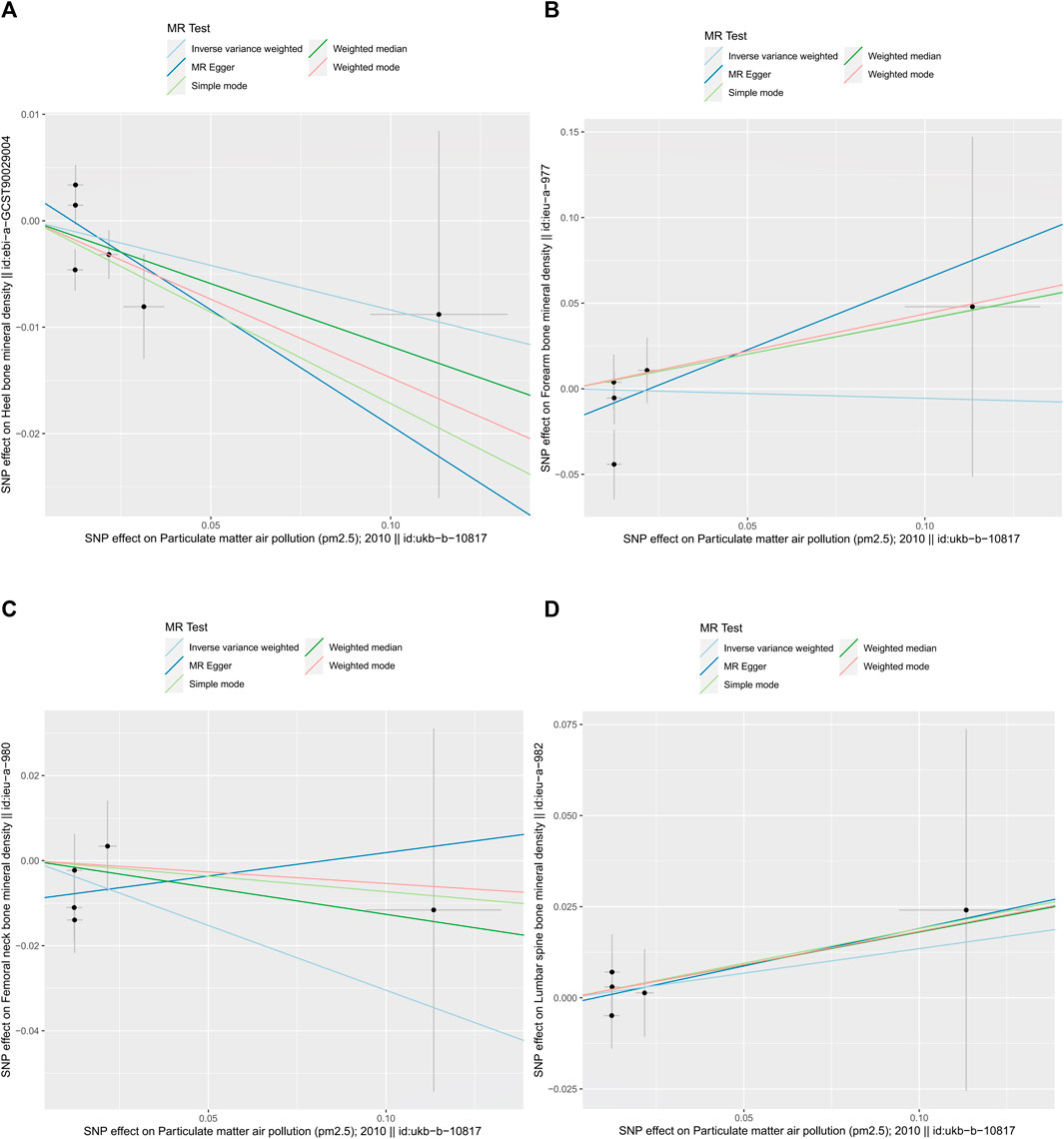
FIGURE 3. summary plot of MR-Egger method (by intercept tests) data. (A) HE-BMD, (B) FA-BMD, (C) FN-BMD, (D) LS-BMD.
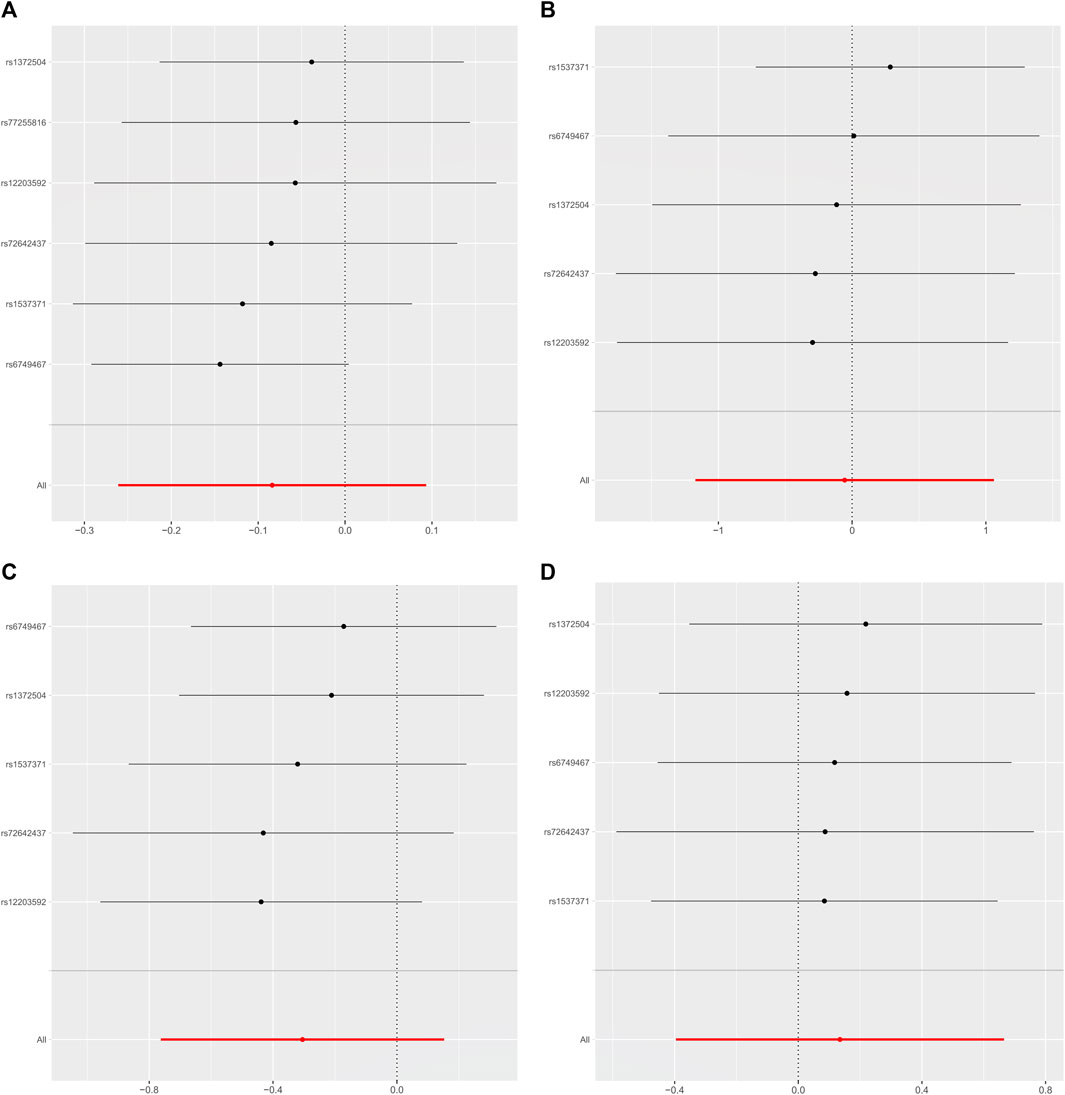
FIGURE 4. Leave-one-out data plot of the final included SNPs. (A) HE-BMD, (B) FA-BMD, (C) FN-BMD, (D) LS-BMD.
Discussion
To our knowledge, this study represents the inaugural exploration of the causal relationship between PM2.5 exposure and osteoporosis. We explored the potential impact of PM2.5 on HE-BMD, FA-BMD, FN-BMD, and LS-BMD. The results showed that after correction for multiple comparisons, no causal relationship between PM2.5 and osteoporosis was found.
So far, there is no conclusion on the relationship between PM2.5 and osteoporosis. An Italian cohort study of 59,950 female participants found that exposure to particulate matter caused a decrease in bone density, particularly in the femoral neck (Adami et al., 2022). Simultaneously, a cross-sectional study focused on males similarly observed a declining trend in bone density after prolonged exposure to PM2.5-10 environments (Alvaer et al., 2007). Intriguingly, two extensive database studies conducted in the United Kingdom and China similarly identified PM2.5 exposure as a risk factor for osteoporotic fractures (Chiu et al., 2021; Shi et al., 2022). Contrary to these, Heo et al. and Alver et al. found no changes in bone density or osteoporotic fracture risk associated with short-term or long-term exposure to PM2.5 (Alver et al., 2010; Heo et al., 2022). Similarly, our study did not identify a potential causal relationship between them. In observational studies, whether it is multi-factor regression or other large-sample prediction models, it is impossible to eliminate the influence of confounding factors, such as alcohol abuse, smoking, high-fat diet, etc (Aibar-Almazan et al., 2022). Concurrently, drug utilization is another crucial influencing factor, the usage of hormones as common respiratory medications significantly rises in regions with severe air pollution (Liu et al., 2022). However, our investigation did not identify SNPs associated with confounders in the included IVs, suggesting that our study was not affected by the confounders mentioned above. It is noteworthy that due to the diversity of sources, there are considerable variations in PM2.5 components across different regions, making it challenging to avoid regional disparities in all studies (Albinet et al., 2007; Shah et al., 2013).
Due to its small particle size, PM2.5 can penetrate the alveoli and enter the bloodstream, leading to potential harm to various organs in the body (Thiankhaw et al., 2022). Research has indicated that PM2.5 can trigger oxidative stress and inflammation in the compromised airways through pathways involving protein kinases and Toll-like receptors (Guo et al., 2017). This activation can result in the upregulation of inflammatory markers such as TNF-α, IL-17, and RANKL, which in turn may lead to enhanced bone resorption (Shen et al., 2005; Saha et al., 2016). Postmenopausal women, due to the decline in estrogen levels, experience a diminished capacity to inhibit osteoclasts, making them a high-risk population for osteoporosis (Singh et al., 2022). Furthermore, several studies have observed that exposure to PM2.5 can induce apoptosis in follicle cells, consequently leading to a decline in ovarian reserve function, this decline in ovarian function may contribute to an increased incidence of osteoporosis in women (Luderer et al., 2022). These findings further underscore the potential impact of PM2.5 on women’s skeletal health. Vitamin D (VD), an essential nutrient for calcium circulation in the human body, is synthesized predominantly (95%) through the skin (Wintermeyer et al., 2016). The results of our study indicate that rs12203592 may be a crucial locus linking PM2.5 exposure to osteoporosis. Previous research suggests that rs12203592, located on chromosome 6 near the IRF4 gene, plays a regulatory role in skin pigmentation and modulation of the VD receptor, affecting the synthesis of VD precursors (Ramagopalan et al., 2010). Additionally, some researchers propose an association between PM2.5 exposure and VD deficiency, potentially linked to PM2.5-induced renal damage and the presence of polycyclic aromatic hydrocarbons in PM2.5 accelerating VD degradation (Calderon-Garciduenas et al., 2015; Xu et al., 2023). While numerous experimental studies suggest a potential association between PM2.5 and osteoporosis, our MR analysis within the studied population did not reveal such a link, warranting further validation.
Our study has several strengths. First, the MR method effectively circumvents confounding factors and reverse causation prevalent in traditional observational studies. Furthermore, we applied more stringent confounding factor exclusion criteria (p < 1*10–5). Second, the instrumental variables (IVs) for PM2.5 exposure and osteoporosis were concurrently derived from existing large-scale GWAS, allowing for a more precise assessment of effect sizes compared to individual-level data or studies with limited sample sizes. However, it is important to acknowledge some potential limitations. The dataset used in our study has limitations in population stratification: there was no stratification based on age and gender, restricting our ability to analyze and identify populations at risk of osteoporosis under PM2.5 exposure. Future research should consider datasets with finer population segmentation for more accurate conclusions through stratified analysis. Besides, due to limitations in GWAS data, our study only supports the causal relationship between PM2.5 exposure and osteoporosis in the European population. Subsequent investigations should utilize larger sample data from different populations for in-depth analysis.
Conclusion
In summary, this study verified that there is no necessary relationship between PM2.5 exposure and the risk of osteoporosis through Mendelian randomization analysis. In addition, due to differences in the environment and population genes in different regions, more up-to-date data are needed for verification.
Data availability statement
The original contributions presented in the study are included in the article/Supplementary Material, further inquiries can be directed to the corresponding author.
Author contributions
YZ: Conceptualization, Data curation, Writing–original draft. JY: Validation, Visualization, Writing–review and editing. HP: Writing–original draft. XZ: Investigation, Writing–original draft. CW: Project administration, Writing–original draft. GW: Investigation, Writing–original draft. ZS: Writing–original draft. JH: Funding acquisition, Resources, Writing–review and editing. BH: Funding acquisition, Project administration, Resources, Supervision, Writing–review and editing.
Funding
The author(s) declare financial support was received for the research, authorship, and/or publication of this article. This work was supported by 2023 Medical Rookie—BH (1S22317). Natural Science Foundation of Zhejiang Province (LY21H270008). National Natural Science Foundation of China (82074469). Zhejiang Administration of Traditional Chinese Medicine (2023ZF019). JH Famous Expert Inheritance Studio of Traditional Chinese Medicine (GZS2021020).
Conflict of interest
The authors declare that the research was conducted in the absence of any commercial or financial relationships that could be construed as a potential conflict of interest.
Publisher’s note
All claims expressed in this article are solely those of the authors and do not necessarily represent those of their affiliated organizations, or those of the publisher, the editors and the reviewers. Any product that may be evaluated in this article, or claim that may be made by its manufacturer, is not guaranteed or endorsed by the publisher.
Supplementary material
The Supplementary Material for this article can be found online at: https://www.frontiersin.org/articles/10.3389/fgene.2024.1263916/full#supplementary-material
References
Adami, G., Cattani, G., Rossini, M., Viapiana, O., Olivi, P., Orsolini, G., et al. (2022). Association between exposure to fine particulate matter and osteoporosis: a population-based cohort study. Osteoporos. Int. 33, 169–176. doi:10.1007/s00198-021-06060-9
Aibar-Almazan, A., Voltes-Martinez, A., Castellote-Caballero, Y., Afanador-Restrepo, D. F., Carcelen-Fraile, M. D. C., and Lopez-Ruiz, E. (2022). Current status of the diagnosis and management of osteoporosis. Int. J. Mol. Sci. 23, 9465. doi:10.3390/ijms23169465
Albinet, A., Leoz-Garziandia, E., Budzinski, H., and Viilenave, E. (2007). Polycyclic aromatic hydrocarbons (PAHs), nitrated PAHs and oxygenated PAHs in ambient air of the Marseilles area (South of France): concentrations and sources. Sci. Total Environ. 384, 280–292. doi:10.1016/j.scitotenv.2007.04.028
Alvaer, K., Meyer, H. E., Falch, J. A., Nafstad, P., and Sogaard, A. J. (2007). Outdoor air pollution and bone mineral density in elderly men - the Oslo Health Study. Osteoporos. Int. 18, 1669–1674. doi:10.1007/s00198-007-0424-y
Alver, K., Meyer, H. E., Falch, J. A., and Sogaard, A. J. (2010). Outdoor air pollution, bone density and self-reported forearm fracture: the Oslo Health Study. Osteoporos. Int. 21, 1751–1760. doi:10.1007/s00198-009-1130-8
Arceo-Mendoza, R. M., and Camacho, P. M. (2021). Postmenopausal osteoporosis: latest guidelines. Endocrinol. Metab. Clin. North Am. 50, 167–178. doi:10.1016/j.ecl.2021.03.009
Bowden, J., and Holmes, M. V. (2019). Meta-analysis and Mendelian randomization: a review. Res. Synth. Methods 10, 486–496. doi:10.1002/jrsm.1346
Burgess, S., and Thompson, S. G. (2017). Interpreting findings from Mendelian randomization using the MR-Egger method. Eur. J. Epidemiol. 32, 377–389. doi:10.1007/s10654-017-0255-x
Burgess, S., Thompson, S. G., and Collaboration, C. C. G. (2011). Avoiding bias from weak instruments in Mendelian randomization studies. Int. J. Epidemiol. 40, 755–764. doi:10.1093/ije/dyr036
Calderon-Garciduenas, L., Franco-Lira, M., D'Angiulli, A., Rodríguez-Díaz, J., Blaurock-Busch, E., Busch, Y., et al. (2015). Mexico City normal weight children exposed to high concentrations of ambient PM2.5 show high blood leptin and endothelin-1, vitamin D deficiency, and food reward hormone dysregulation versus low pollution controls. Relevance for obesity and Alzheimer disease. Environ. Res. 140, 579–592. doi:10.1016/j.envres.2015.05.012
Camacho, P. M., Petak, S. M., Binkley, N., Diab, D. L., Eldeiry, L. S., Farooki, A., et al. (2020). American association of clinical endocrinologists/American college of endocrinology clinical practice guidelines for the diagnosis and treatment of postmenopausal osteoporosis-2020 update. Endocr. Pract. 26, 1–46. doi:10.4158/GL-2020-0524SUPPL
Chen, Z., Salam, M. T., Karim, R., Toledo-Corral, C. M., Watanabe, R. M., Xiang, A. H., et al. (2015). Living near a freeway is associated with lower bone mineral density among Mexican Americans. Osteoporos. Int. 26, 1713–1721. doi:10.1007/s00198-015-3051-z
Chiu, Y. C., Lin, Y. T., Hsia, Y. F., Jung, C. R., Lo, Y. C., Chen, T. M., et al. (2021). Long-term exposure to fine particulate matter and osteoporotic fracture: a case-control study in Taiwan. Environ. Res. 196, 110888. doi:10.1016/j.envres.2021.110888
Eeftens, M., Beelen, R., de Hoogh, K., Bellander, T., Cesaroni, G., Cirach, M., et al. (2012). Development of Land Use Regression models for PM(2.5), PM(2.5) absorbance, PM(10) and PM(coarse) in 20 European study areas; results of the ESCAPE project. Environ. Sci. Technol. 46, 11195–11205. doi:10.1021/es301948k
Fermo, P., Comite, V., Falciola, L., Guglielmi, V., and Miani, A. (2019). Efficiency of an air cleaner device in reducing aerosol particulate matter (PM) in indoor environments. Int. J. Environ. Res. Public Health 17, 18. doi:10.3390/ijerph17010018
Guo, Z., Hong, Z., Dong, W., Deng, C., Zhao, R., Xu, J., et al. (2017). PM(2.5)-Induced oxidative stress and mitochondrial damage in the nasal mucosa of rats. Int. J. Environ. Res. Public Health 14, 134. doi:10.3390/ijerph14020134
Hemani, G., Zheng, J., Elsworth, B., Wade, K. H., Haberland, V., Baird, D., et al. (2018). The MR-Base platform supports systematic causal inference across the human phenome. Elife 7, e34408. doi:10.7554/eLife.34408
Heo, S., Kim, H., Kim, S., Choe, S. A., Byun, G., Lee, J. T., et al. (2022). Effects of virtual reality-based graded exposure therapy on ptsd symptoms: a systematic review and meta-analysis. Int. J. Environ. Res. Public Health 19, 15911. doi:10.3390/ijerph192315911
Huff, R. D., Carlsten, C., and Hirota, J. A. (2019). An update on immunologic mechanisms in the respiratory mucosa in response to air pollutants. J. Allergy Clin. Immunol. 143, 1989–2001. doi:10.1016/j.jaci.2019.04.012
Jones, A. R., Goh, M., Langham, R., Boyle, J., Milat, F., Ebeling, P. R., et al. (2020). Osteoporosis and premature ovarian insufficiency: geographic variation in clinicians' and consumers' knowledge gaps and barriers to care. Arch. Osteoporos. 15, 38. doi:10.1007/s11657-020-00713-8
Kanis, J. A., Cooper, C., Rizzoli, R., and Reginster, J. Y.Scientific Advisory Board of the European Society for Clinical, Economic Aspects of Osteoporosis, the Committees of Scientific Advisors, National Societies of the International Osteoporosis Foundation (2019). European guidance for the diagnosis and management of osteoporosis in postmenopausal women. Osteoporos. Int. 30, 3–44. doi:10.1007/s00198-018-4704-5
Lin, Y. H., Wang, C. F., Chiu, H., Lai, B. C., Tu, H. P., Wu, P. Y., et al. (2020). Air pollutants interaction and gender difference on bone mineral density T-score in Taiwanese adults. Int. J. Environ. Res. Public Health 17, 9165. doi:10.3390/ijerph17249165
Liu, K., Hua, S., and Song, L. (2022). PM2.5 exposure and asthma development: the key role of oxidative stress. Oxid. Med. Cell Longev. 2022, 3618806. doi:10.1155/2022/3618806
Loh, P. R., Kichaev, G., Gazal, S., Schoech, A. P., and Price, A. L. (2018). Mixed-model association for biobank-scale datasets. Nat. Genet. 50, 906–908. doi:10.1038/s41588-018-0144-6
Luderer, U., Lim, J., Ortiz, L., Nguyen, J. D., Shin, J. H., Allen, B. D., et al. (2022). Exposure to environmentally relevant concentrations of ambient fine particulate matter (PM(2.5)) depletes the ovarian follicle reserve and causes sex-dependent cardiovascular changes in apolipoprotein E null mice. Part Fibre Toxicol. 19, 5. doi:10.1186/s12989-021-00445-8
Pang, K. L., Ekeuku, S. O., and Chin, K. Y. (2021). Particulate air pollution and osteoporosis: a systematic review. Risk Manag. Healthc. Policy 14, 2715–2732. doi:10.2147/RMHP.S316429
Qiao, D., Pan, J., Chen, G., Xiang, H., Tu, R., Zhang, X., et al. (2020). Long-term exposure to air pollution might increase prevalence of osteoporosis in Chinese rural population. Environ. Res. 183, 109264. doi:10.1016/j.envres.2020.109264
Ramagopalan, S. V., Heger, A., Berlanga, A. J., Maugeri, N. J., Lincoln, M. R., Burrell, A., et al. (2010). A ChIP-seq defined genome-wide map of vitamin D receptor binding: associations with disease and evolution. Genome Res. 20, 1352–1360. doi:10.1101/gr.107920.110
Sabri, S. A., Chavarria, J. C., Ackert-Bicknell, C., Swanson, C., and Burger, E. (2023). Osteoporosis: an update on screening, diagnosis, evaluation, and treatment. Orthopedics 46, e20–e26. doi:10.3928/01477447-20220719-03
Saha, H., Mukherjee, B., Bindhani, B., and Ray, M. R. (2016). Changes in RANKL and osteoprotegerin expression after chronic exposure to indoor air pollution as a result of cooking with biomass fuel. J. Appl. Toxicol. 36, 969–976. doi:10.1002/jat.3275
Shah, A. S., Langrish, J. P., Nair, H., McAllister, D. A., Hunter, A. L., Donaldson, K., et al. (2013). Global association of air pollution and heart failure: a systematic review and meta-analysis. Lancet 382, 1039–1048. doi:10.1016/S0140-6736(13)60898-3
Shen, F., Ruddy, M. J., Plamondon, P., and Gaffen, S. L. (2005). Cytokines link osteoblasts and inflammation: microarray analysis of interleukin-17- and TNF-alpha-induced genes in bone cells. J. Leukoc. Biol. 77, 388–399. doi:10.1189/jlb.0904490
Shi, W., Huang, C., Chen, S., Yang, C., Liu, N., Zhu, X., et al. (2022). Long-term exposure to air pollution increases hip fracture incidence rate and related mortality: analysis of National Hip Fracture Database. Osteoporos. Int. 33, 1949–1955. doi:10.1007/s00198-022-06445-4
Si, L., Winzenberg, T. M., Chen, M., Jiang, Q., and Palmer, A. J. (2015). Residual lifetime and 10 year absolute risks of osteoporotic fractures in Chinese men and women. Curr. Med. Res. Opin. 31, 1149–1156. doi:10.1185/03007995.2015.1037729
Singh, S., Dutta, S., Khasbage, S., Kumar, T., Sachin, J., Sharma, J., et al. (2022). A systematic review and meta-analysis of efficacy and safety of Romosozumab in postmenopausal osteoporosis. Osteoporos. Int. 33, 1–12. doi:10.1007/s00198-021-06095-y
Thiankhaw, K., Chattipakorn, N., and Chattipakorn, S. C. (2022). PM2.5 exposure in association with AD-related neuropathology and cognitive outcomes. Environ. Pollut. 292, 118320. doi:10.1016/j.envpol.2021.118320
Wang, L., Yu, W., Yin, X., Cui, L., Tang, S., Jiang, N., et al. (2021). Prevalence of osteoporosis and fracture in China: the China osteoporosis prevalence study. JAMA Netw. Open 4, e2121106. doi:10.1001/jamanetworkopen.2021.21106
Wilson, D. J. (2019). Osteoporosis and sport. Eur. J. Radiol. 110, 169–174. doi:10.1016/j.ejrad.2018.11.010
Wintermeyer, E., Ihle, C., Ehnert, S., Stockle, U., Ochs, G., de Zwart, P., et al. (2016). Crucial role of vitamin D in the musculoskeletal system. Nutrients 8, 319. doi:10.3390/nu8060319
Wu, F., Huang, Y., Hu, J., and Shao, Z. (2020). Mendelian randomization study of inflammatory bowel disease and bone mineral density. BMC Med. 18, 312. doi:10.1186/s12916-020-01778-5
Xu, C., Zhang, Q., Huang, G., Huang, J., and Zhang, H. (2023). The impact of PM2.5 on kidney. J. Appl. Toxicol. 43, 107–121. doi:10.1002/jat.4356
Zhang, Y., Liu, S., Wang, Y., and Wang, Y. (2022). Causal relationship between particulate matter 2.5 and hypothyroidism: a two-sample Mendelian randomization study. Front. Public Health 10, 1000103. doi:10.3389/fpubh.2022.1000103
Keywords: PM2.5, air pollution, osteoporosis, mendelian randomization, bone mineral density
Citation: Zhang Y, Yu J, Pei H, Zhao X, Wang C, Wang G, Shen Z, Hua J and He B (2024) Potential causal associations of PM2.5 and osteoporosis: a two-sample mendelian randomization study. Front. Genet. 15:1263916. doi: 10.3389/fgene.2024.1263916
Received: 09 September 2023; Accepted: 12 February 2024;
Published: 22 February 2024.
Edited by:
Simon Charles Heath, Center for Genomic Regulation (CRG), SpainReviewed by:
Kiarash Saleki, Shahid Beheshti University of Medical Sciences, IranMelissa M. Formosa, University of Malta, Malta
Copyright © 2024 Zhang, Yu, Pei, Zhao, Wang, Wang, Shen, Hua and He. This is an open-access article distributed under the terms of the Creative Commons Attribution License (CC BY). The use, distribution or reproduction in other forums is permitted, provided the original author(s) and the copyright owner(s) are credited and that the original publication in this journal is cited, in accordance with accepted academic practice. No use, distribution or reproduction is permitted which does not comply with these terms.
*Correspondence: Jiang Hua, MzA0MzEyOTAwNkBxcS5jb20=; Bangjian He, aGViYW5namlhbkAxNjMuY29t
†These authors have contributed equally to this work and share first authorship