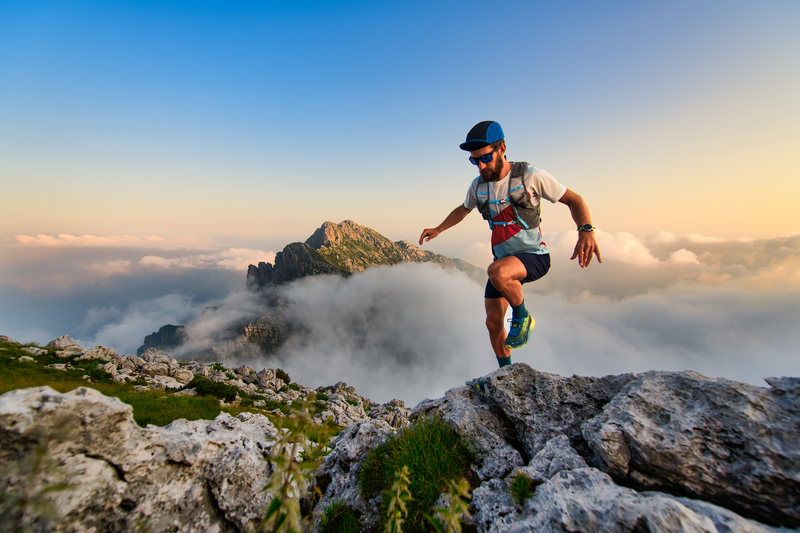
94% of researchers rate our articles as excellent or good
Learn more about the work of our research integrity team to safeguard the quality of each article we publish.
Find out more
ORIGINAL RESEARCH article
Front. Genet. , 11 January 2024
Sec. Statistical Genetics and Methodology
Volume 14 - 2023 | https://doi.org/10.3389/fgene.2023.1321484
This article is part of the Research Topic Mendelian Randomization: the Study of Causal Relationships between Tumors and Underlying Pathogenic Factors View all 15 articles
Background: Epidemiological research has established associations between various inflammatory cytokines and the occurrence of oral cancer and oropharyngeal cancer (OCPC). We performed a Mendelian randomization (MR) analysis to systematically investigate the causal relationship between inflammatory cytokines and OCPC.
Methods: We performed a bidirectional two-sample MR analysis using OCPC from 12 studies (6,034 cases and 6,585 controls) and genome-wide association study (GWAS) results for 41 serum cytokines from 8,293 Finns, respectively. Inverse variance weighting was used as the primary MR method and four additional MR methods (MR Egger, Weighted median, Simple mode, Weighted mode) were used to examine genetic associations between inflammatory traits and OCPC, and Cochran’s Q test, MR-Egger intercept, leave-one-out analysis, funnel plot, and multivariate MR (MVMR) analysis were used to assess the MR results.
Results: The results suggested a potential association between high gene expression of Macrophage inflammatory protein-1α (MIP1α/CCL3) and an increased risk of OCPC (Odds Ratio (OR): 1.71, 95% Confidence Interval (CI): 1.09–2.68, p = 0.019). Increasing the expression levels of the interleukin-7 (IL-7) gene by 1 standard deviation reduced the risk of OCPC (OR: 0.64, 95%CI: 0.48–0.86, p = 0.003). In addition, multivariate Mendelian randomization analysis also showed the same results (MIP1α/CCL3, OR: 1.002, 95% CI: 0.919–1.092, p = 0.044; IL-7, OR: 0.997, 95% CI: 0.994–0.999, p = 0.011). Conversely, there was a positive correlation between genetic susceptibility to OCPC and an increase in Interleukin-4 (IL-4) (OR: 1.04, 95%CI: 1.00–1.08, p = 0.027).
Conclusion: Our study systematically assessed the association between inflammatory cytokines and the risk of OCPC. We identified two upstream regulatory factors (IL-7 and CCL3) and one downstream effector factor (IL-4) that were associated with OCPC, offering potential avenues for the development of novel treatments.
Based on the latest estimates from Global Cancer Statistics 2020, Head and Neck Squamous Cell Carcinoma (HNSCC) ranks as the seventh most prevalent cancer worldwide, with an annual incidence of over 890,000 new cases and a mortality of over 450,000 (Sung et al., 2021). Among HNSCC cases, Oral Squamous Cell Carcinoma (OSCC) and Oropharyngeal Squamous Cell Carcinoma (OPSCC) are predominant, contributing to a global incidence of over 260,000 cases and over 128,000 deaths, respectively (Siegel et al., 2020). Despite some advances in the treatment of oral and oropharyngeal cancer (OCPC), the 10-year survival rate remains low at below 60%. Even if the treatment is successful, patients may still experience severe functional impairments, including compromised abilities in feeding, swallowing, and speech. Additionally, the recurrence rate remains high (Sung et al., 2021).
Earlier investigations in preclinical settings have indicated that inflammatory cytokines, such as TNF-α, IL-1β, and IL-6, promote the growth, invasion, and spread of cancer cells. Additionally, the transcription factors associated with these cytokines such as NF-kB and STAT3 show increased expression in most cancer types (Voronov et al., 2003; Pikarsky et al., 2004; Bierie and Moses, 2006; Luo et al., 2007). For example, inhibiting the activity or expression of IL-1β can prevent the occurrence of oral cancer by regulating specific key node genes in the tumor microenvironment (TME) (Wu et al., 2016). Moreover, mounting evidence indicates an increased risk of OCPC in the presence of inflammation, and inflammation commonly accompanies the development of OCPC (Leon et al., 2015). These observations suggest that the pharmacological targeting of additional inflammation biomarkers identified in the epidemiological literature through observational studies could offer a potentially effective approach for treating OCPC (Todoric et al., 2016). Nevertheless, the investigations conducted thus far have primarily concentrated on a limited range of inflammatory elements and have failed to acknowledge the impact of additional factors on the changes in inflammation levels. Hence, it is crucial to ascertain whether the changes in inflammatory factors cause the onset of tumors or if the tumors themselves modify the microenvironment, leading to differences in inflammatory factors. Due to the limited comprehension of the cause of OCPC, investigating the exact characteristics of the connections between inflammatory factors and OCPC has considerable clinical significance.
The dynamic nature of the inflammatory response suggests that a specific time point’s measurement, whether high or low, might not precisely represent the overall trend of inflammatory factor variations. Epidemiological, genetic, and biological investigations have confirmed the link between inflammatory factors and OCPC. However, the outcomes derived from these investigations may be distorted by unforeseen confounding variables or reverse causal associations, thus complicating the establishment of clear causal relationships.
Observational studies may hinder an exhaustive comprehension of the connection between OCPC and inflammation due to their intrinsic research limitations. Thus, a detailed understanding of the role of circulating cytokines and their association with OCPC risk may aid in the development of prevention, prediction, and treatment strategies. Researchers may achieve a more comprehensive understanding of the connection between inflammation and OCPC by addressing these limitations.
Mendelian randomization (MR) is a commonly used tool in genetic epidemiology (10), which utilizes instrumental variable IV) variation derived from non-experimental data to assess the causal impact of exposure (e.g., circulating cytokines) on an outcome (e.g., OCPC) (Lawlor et al., 2008). Given the random allocation of alleles during meiosis, MR can mitigate confounding variables and reverse causality, thereby offering more robust evidence for causal inferences (Burgess et al., 2015). The use of two-sample MR analysis allows researchers to evaluate the associations between the instrument-exposure and instrument-outcome in two distinct population samples, thereby improving the applicability and efficiency of testing (Hartwig et al., 2016). In this study, we performed an analysis of the genome-wide association study (GWAS) summary data of 41 inflammatory cytokines to identify relevant genetic variations. These variations were then further investigated in relation to OCPC. Specifically, we examined the correlations between these genetic variations and OCPC by reversing the exposure and outcome. Our research results not only provided substantial Supplementary Data for previous epidemiological investigations but also offered fresh perspectives on the development and prevention of OCPC.
This study used a bidirectional MR approach to assess the causal relationship between the circulating cytokines and OCPCs. The study’s overall design is illustrated in Figure 1. To assess the causal relationship between circulating cytokines and OCPCs, MR analysis was performed to test the following three hypotheses: 1) Genetic instruments have a strong association with exposure; 2) Genetic instruments are not affected by any potential known confounding factors; 3) The association between the genetic instrument and the outcome is solely influenced by the exposure (Smith and Ebrahim, 2003). Simultaneously, the reverse MR method is used to examine potential reverse causal effects. The data used in this study were obtained from publicly available large-scale GWAS which can be accessed through the GWAS Catalog service (https://www.ebi.ac.uk/gwas/home). The studies included in the initial GWAS had obtained approval from their respective institutional review boards.
FIGURE 1. Illustration of the research design in the bidirectional Mendelian randomization (MR) analysis. Important instrumental variables, including 41 inflammatory cytokines and OCPC, were selected to explore the bidirectional causal relationships. This method is used to detect correlations (solid line) and violations of the Mendelian randomization assumption (dashed line). This figure was created using BioRender.com.
The research used an extensive meta-analysis of GWAS on the circulating levels of 41 cytokines. This analysis combined information from three separate cohort studies: FINRISK 1997, FINRISK 2002, and the Young Finns Study on Cardiovascular Risk (YFS). This study included a total of 8,293 participants. The genomic and cytokine data were contributed by 4,608 individuals from FINRISK 1997, while another 1,705 participants from FINRISK2002 also provided their cytokine data. Cytokine quantification was performed by analyzing the EDTA-treated plasma of FINRISK 1997, the heparinized plasma of FINRISK 2002, and the serum samples from YFS. To control for potential confounding factors, such as age, sex, body mass index, and genetic variations, genetic associations were properly adjusted. Specifically, the top 10 genetic principal components were included using genomic control correction. Bio-Rad’s pre-mixed Bio-Plex Pro Human Cytokine 27-plex Assay and 21-plex Assay were used to measure a total of 41 cytokines in YFS and FINRISK 2002, respectively. The measurements were performed by the Bio-Plex 200 reader equipped with Bio-Plex 6.0 software. However, it is important to note that, out of the 41 cytokines, 7 had to be excluded due to the presence of missing values exceeding 90% (Ahola-Olli et al., 2017; Kalaoja et al., 2021). A detailed overview of the GWAS data for the cytokines used in the MR analysis is presented in Table 1.
Using data from the most extensive GWAS to date, we investigated the impact of inflammatory elements on the vulnerability to OCPC. By extracting specific variations in single-nucleotide polymorphisms (SNPs) linked to exposure, we assessed a total of 6,034 cases and 6,585 controls enrolled in 12 different epidemiological studies (Lesseur et al., 2016). The Genetic Associations and Mechanisms of Oncology (GAME-ON) network is responsible for conducting these studies. Every participant has provided informed consent, and the relevant institutional review boards have approved this study. Furthermore, the study includes data from the European Prospective Investigation into Cancer and Nutrition (EPIC) study, and the Health and Nutrition 5,000 (HN5000) study, which specifically examines the health and lifestyle of 5,000 United Kingdom participants. Extensive information about the study, as well as the genotyping and imputation methods used, has been previously documented (Lesseur et al., 2016). The study sample comprised individuals from Europe (45.3%), North America (43.9%), and South America (10.8%). The study encompasses diverse types of cancer identified through the specific International Classification of Diseases, 10th Revision (ICD-10) codes, such as oral cancer (C02.0–C02.9, C03.0–C03.9, C04.0–C04.9, C05.0–C06.9) and oropharyngeal cancer (C01.9, C02.4, C09.0–C10.9). Exclusion criteria led to the removal of 954 individuals with hypopharyngeal cancer, unidentifiable codes, or overlapping cancers. To mitigate the effects of heterogeneity in these regions, GWAS studies were conducted exclusively on individuals with European ancestry, totaling 11,117 participants. Among these, there were 5,133 cases, comprising 2,700 cases of oral cancer and 2,433 cases of oropharyngeal cancer. In addition, there were 5,984 control cases (Supplementary Table S1).
The cytokines-OCPC pathway is a potential target for cancer prevention and treatment. To identify the possible mediators of this pathway, we applied MR methods to examine the causal links between cytokines and common risk factors for OCPC. We used an existing database to investigate the following risk factors that have been widely recognized as associated with OCPC: smoking, drinking, body mass index, type 2 diabetes, hypertension, and HPV16/18 infection (Argiris et al., 2008; Leemans et al., 2018). Supplementary Table S2 lists the GWAS summary data for the above risk factors.
To ensure that the conclusion regarding the causal relationship between cytokines and the risk of OCPC is authentic and accurate, we implement various quality control measures in the selection of optimal genetic instrumental variables. Our approach involves the selection of SNPs that are closely associated with inflammatory factors and demonstrate genome-wide significance with a p-value lower than 5 × 10−8. By focusing on these specific SNPs, we establish robust instrumental variables for our study (Burgess et al., 2011). We proceeded to eliminate linkage disequilibrium (LD). Our criteria for removal included an r2 value below 0.001 and a distance of 5,000 kb. SNPs exceeding the r2 threshold of 0.001, which includes the most significant SNP within a 5,000 kb range, were excluded. After aligning the chosen SNPs with the outcome data, we found that only ten out of the 41 systemic inflammation factors available exhibited two or more independent SNPs at a significance level of p-value less than 5 × 10−8. Additionally, it was found that nine of them displayed three or more independent SNPs. Since inflammatory cytokines are a class of cytokines with multiple functions and interactions, and they may involve multiple genes and SNPs, a less stringent criterion is needed to capture their genetic variation. When selecting instrumental variables, we established A less stringent p-value threshold (5 × 10−6) to capture more SNPs of inflammatory cytokines (Li et al., 2021; Pan et al., 2023). If there is an insufficient number of exposure-associated SNPs detected in the GWAS findings, proxy SNPs exhibiting high LD with a correlation coefficient (r2 > 0.90) will be employed as alternative substitutes. These proxy SNPs can be accessed through LDlink (https://ldlink.nci.nih.gov/) as a resource (Machiela and Chanock, 2015). To exclude all SNPs associated with exposure and avoid potential pleiotropic effects, we performed a comprehensive investigation using the PhenoScanner V2 tool (http://www.phenoscanner.medschl.cam.ac.uk/) (Staley et al., 2016). Through the aforementioned steps, we acquired 41 cytokines associated with inflammation. As an instrumental variable with a significance threshold of F < 10 is considered weak, we will exclude it from our study. Detailed information on the identified SNPs is listed in Supplementary Tables S3 and S4. Furthermore, to uphold the fundamentals of MR, we shall examine the desired SNPs to exclude any that exhibit associations with the resulting outcomes.
To explore the causal relationship between inflammatory regulators and OCPC, we utilized GWAS data and employed two-sample MR and multivariate MR methods. Statistical analysis was conducted using R software (v4.1.3) and the “MendelianRandomization”, “MVMR” and “MRPRESSO” software packages. Multivariable MR (MVMR) is mainly used to evaluate the impact of multiple potential exposures on overall outcomes and identify potential risk factors (Tian and Burgess, 2023). The relationship between inflammatory factors and OCPC was examined using the inverse variance weighted (IVW) method. The instrumental variable method was used to estimate the mean effects of SNPs by regressing SNP-inflammatory factors on SNP-OCPC. Additionally, we utilized the weighted median estimator (WME) and the MR-Egger regression. WME is a statistical method that applies weights to the empirical distribution function of ratio estimates for SNPs within the study range, minimizing biases in estimating causal effects. MR-Egger regression employs weighted linear regression to estimate the effect of SNP-OCPC, considering SNP-inflammatory factors, and provides an evaluation of causal effects, even in the presence of invalid instruments (Bowden et al., 2015). If the directions of the β-values of other methods are the same, it may be interpreted as a positive result (Chen et al., 2020). Moreover, the “Leave-one-out” strategy was employed to visually illustrate whether a single SNP substantially influences the causal association. To ensure the dependability of the MR findings, we performed diverse evaluations of diversity and sensitivity. We employed Cochran’s Q test to assess heterogeneity among SNPs. If no indications of heterogeneity were observed, we utilized a fixed-effect model; otherwise, a random-effects model was implemented. The Egger-intercept test was carried out for horizontal pleiotropy examination (Verbanck et al., 2018; Song et al., 2022). The results of the study were presented using odds ratios (ORs) and their corresponding 95% confidence intervals (CIs). Any results with a p-value less than 0.05 were considered statistically significant. We applied the FDR method to correct for multiple testing, which is a method implemented by the q-value program. It is a commonly used method for multiple testing correction, which can control the proportion of false rejections of the null hypothesis among multiple hypothesis tests. We chose a q-value of less than 0.1 as the significance level, which is a reasonable choice because it can reduce the false positive rate while maintaining the statistical power. When the p-value is less than 0.05 but the q-value is greater than or equal to 0.1, we consider it a suggestive association result (Storey and Tibshirani, 2003; Li et al., 2022). The analysis of the MR study adhered to the guidelines set forth by the Strengthening the Reporting of Observational Studies in Epidemiology using Mendelian Randomization (STROBE-MR) statement, which emphasizes the scientific rigor and reporting standards for observational studies in epidemiology (Skrivankova et al., 2021).
The association between systemic inflammation factors and OCPC was revealed through gene prediction, supported by the following findings (Figure 2). The IVW method uncovered a significant surge in OCPC risk, linked to higher levels of Macrophage inflammatory protein-1α (MIP1α/CCL3) (OR: 1.71, 95% CI: 1.09–2.68, p = 0.019). Both the MR-Egger heterogeneity test and Cochran’s Q test failed to identify any signs of heterogeneity, indicating an absence of variation (p > 0.05). Additionally, by utilizing the IVW method, increased Interleukin-7 (IL-7) levels were associated with a decreased likelihood of OCPC(OR: 0.64, 95%CI: 0.48–0.86, p = 0.003). No evidence of heterogeneity or horizontal pleiotropy was discovered (p > 0.05) (Table 2). Figures 3, 4 show forest plots and scatter plots illustrating the genetic association of MIP1α/CCL3 and IL-7 SNPs with OCPC. The funnel plot demonstrates overall symmetry, indicating little evidence of heterogeneity (Supplementary Figure S1). A sensitivity analysis, using a leave-one-out approach, provided evidence of the lack of a single SNP with substantial impact on the overall effect. This verifies the dependability and consistency of the estimation of the causal effect (Supplementary Figure S3).
FIGURE 2. Using SNPs with a significance level of p < 5 × 10−6, we predicted the potential impact of inflammation regulatory factors across the genome on OCPC. To establish a causal link between circulating cytokine levels and OCPC, we conducted a two-sample Mendelian randomization (MR) analysis employing the IVW method. By estimating the odds ratio of OCPC for every 1-SD rise in predicted circulating cytokine levels, as determined by genetic prediction, we derived a 95% confidence interval (CI) value. This approach primarily determines the causal relationship between OCPC and the levels of circulating cytokines. A detailed overview of cytokines is provided in Table 1.
TABLE 2. Our research examined whether there is a causal relationship between the levels of MIP1α/CCL3 and IL-7 in the circulatory system and OCPC. To achieve this, we employed genetic prediction techniques. To assess the variation in estimates of individual SNP effects, we used Cochran’s Q test, and we employed the MR-Egger intercept test to evaluate horizontal pleiotropy.
FIGURE 3. MIP1α/CCL3 and IL-7 related SNPs are depicted in the forest plot for OCPC risk. (A) Forest plot for MIP1α/CCL3 exposure. (B) Forest plot for IL-7 exposure. The X-axis represents the effect estimates of MIP1α/CCL3 and IL-7 on OCPC analyzed using MR. The Y-axis represents the MR effect values for each SNP site.
FIGURE 4. Using various MR methods, the scatter plots demonstrate the genetic correlation between SNP and OCPC for MIP1α/CCL3 and IL-7. (A) The exposure of MIP1α/CCL3 is illustrated in the scatter plot. (B) The exposure of IL-7 is illustrated in the scatter plot. Each scatter plot illustrates the associations between the alleles and the risk of the outcome, plotted against the association with one standard deviation of exposure. The effects are represented by the gray error bars, which indicate the 95% confidence intervals. The IVW, MR Egger, Weighted median, Simple mode, and Weighted mode were employed for the analysis. The estimated MR effect of each method can be determined by the slope of each line.
Supplementary Table S5 presents the MR outcomes concerning the prediction of genetic susceptibility to systemic inflammation factors and OCPC risk assessment. Furthermore, it contains summaries of the analyses conducted to assess heterogeneity, pleiotropy, and sensitivity.
To evaluate the reverse causal effects, we conducted a study in which we identified 12 SNPs that have a significant and independent association with OCPC. The association reached a significance level of p < 5 × 10–6. We used a varying number of SNPs for each cytokine due to the lack of certain SNPs to be used universally. Detailed information about the number of SNPs used for each cytokine can be found in Supplementary Table S6. A suggestive association was observed between genetic susceptibility to OCPC and increased levels of Interleukin-4 (IL-4) based on the IVW method (OR: 1.04, 95%CI: 1.00–1.08, p = 0.03). No other significant associations were found, except for IL-4 (Figure 5). Furthermore, significant results of the MR and sensitivity analysis of OCPC and cytokines are shown in Table 3. Figures 6, 7 present forest plots and scatter plots illustrating the causal impact of OCPC-associated SNPs on IL-4.
FIGURE 5. The influence of OCPC gene estimation on systemic inflammatory regulators (SNPs reaching p < 5 × 10−6) can lead to causal consequences. The connection between OCPC and levels of circulating cytokines can be predominantly ascertained through a two-sample Mendelian randomization (MR) analysis using the IVW technique. The estimated range of the 95% confidence interval (CI) reflects the odds ratio of circulating cytokines for each 1-SD rise in genetically anticipated OCPC levels. A detailed overview of cytokines can be found in Table 1.
TABLE 3. We evaluated the effects of genetic prediction (OCPC) on the levels of circulating IL-4. To assess heterogeneity between individual SNP estimates, we utilized Cochran’s Q test. The MR-Egger intercept test was employed to investigate horizontal pleiotropy.
FIGURE 6. Forest plot of OCPC-related SNPs and IL-4 risk (The effect estimates of OCPC on IL-4 analyzed through MR are represented on the X-axis. Each SNP site’s MR effect values are represented on the Y-axis).
FIGURE 7. The scatter plot in this passage shows the genetic associations between SNPs related to OCPC and IL-4 using different MR methods. The gray error bars represent the 95% confidence intervals of the effects. These scatter plots represent the associations between each allele and the risk of the outcome, plotted against the association with one standard deviation of exposure. The analysis was conducted using IVW, MR Egger, Weighted median, Simple mode, and Weighted mode. The slope of each line represents the estimated MR effect of each method.
In univariate MR analysis, while examining the causal relationship between cytokines and OCPC, we also found that after removing SNPs associated with confounding factors, there were still some SNPs that were not only associated with OCPC but also strongly associated with other risk factors. Therefore, assessing the causal relationship between cytokines and common risk factors for OCPC is beneficial to identifying interfering factors that may mediate the association between cytokines and OCPC. We combined all SNPs related to MIP1α/CCL3 and IL-7 as cytokine instrumental variables to find the greatest genetic confounding. Preliminary results show a potential causal relationship between cytokines and common OCPC risk factors (including smoking, drinking, body mass index, and hypertension) (Figure 8, Supplementary Table S7). To control for pleiotropic pathways, when we further applied the MVMR model, the results showed that cytokines still have a potential causal effect on OCPC (MIP1α/CCL3, OR: 1.002, 95% CI: 0.919–1.092, p = 0.044; IL-7, OR: 0.997, 95% CI: 0.994–0.999, p = 0.011) (Table 4).
Numerous observational studies have found that the levels of circulating cytokines are related to the occurrence of OCPC. However, observational studies may be subject to bias due to inadequate sample size and confounding factors, resulting in skewed results. Moreover, there is insufficient genetic evidence to support this relationship in this field. Therefore, we utilized the latest GWAS data and adopted a systematic analysis approach to explore the causal effects of 41 different cytokines on OCPC. Unlike traditional MR studies, we not only focused on single cytokines but also performed bidirectional MR analysis, which can simultaneously test whether two factors have a causal relationship and what the causal direction is. In this way, we can identify the cytokines before or after the disease pathway. In addition, we also used various MR methods to enhance the robustness of the study and minimize the interference of pleiotropic effects. The objective of this study was to systematically assess the potential causal relationship between 41 circulating cytokines and OCPC risk using bidirectional MR analysis.
The tumor microenvironment and the malignant properties of tumor cells are crucial factors in shaping the biological behavior of tumors, directly driving their growth, invasion, and metastasis (Hanahan and Coussens, 2012). Systemic cytokines are a group of molecules that play a broad role in controlling inflammation throughout the body. These cytokines maintain the balance between pro- and anti-inflammatory processes in the TME, thereby ensuring that the immune system functions effectively during infection, injury, or disease while avoiding excessive damage to body tissues (Zhang et al., 2020; Ozga et al., 2021; Bhat et al., 2022). Accumulating evidence indicates that chemokines may exert pro-tumor effects in different types of cancer (Soria and Ben-Baruch, 2008; Levina et al., 2009; Hwang et al., 2012). Our findings show a positive association between high levels of CCL3 and an elevated risk of OCPC. CCL3, a chemokine that belongs to the CC chemokine subfamily, is synthesized by monocytes/macrophages, lymphocytes, neutrophils, as well as various immune cells including eosinophils, mast cells, fibroblasts, and dendritic cells. It is also called as macrophage inflammatory protein-1α (MIP-1α) (Hanahan and Coussens, 2012). CCL3 plays a pivotal role in recruiting inflammatory cells under both homeostatic and pathological conditions. CCL3 may contribute to cancer progression by stimulating leukocyte accumulation, angiogenesis, and tumorigenesis. OCPC cells and tissues exhibit overexpression of CCL3, which correlates with poorer survival rates among OCPC patients (Silva et al., 2007; da Silva et al., 2017). CCL3 can stimulate cancer cell growth and migration (da Silva et al., 2017; Hsu et al., 2013), whereas blocking CCL3 can suppress tumor growth, and angiogenesis, and increase cell sensitivity to therapeutic drugs (Liao et al., 2016; Kim et al., 2017). These mechanisms could elucidate the role of CCL3 in driving the pathophysiology of OCPC.
Research has shown that inflammation in specific organs can affect the risk of cancer, and inflammatory factors also exist in the tumor microenvironment to alter the proliferation, survival, and metastasis of malignant cells. An association between OCPC and inflammatory cytokines, wound-healing genes, growth factors, and cell cycle genes has been reported (Perez-Sayans et al., 2009; Mantovani, 2010). Interestingly, our study suggests that an increase of 1 standard deviation (SD) in the predicted levels of the IL-7 gene is related to a decreased risk of OCPC. IL-7, an indispensable cytokine for the adaptive immune system, is primarily secreted by the bone marrow, thymus, and lymph nodes. IL-7 plays a crucial role in lymphocyte development and survival, contributing to the maintenance of immune self-stability in the body (Fry and Mackall, 2002; Jiang et al., 2005; Hong et al., 2012; Barata et al., 2019). This effect may be due to the potential role of active inflammatory responses in decreasing the incidence of OCPC. Due to the powerful biological effects of IL-7, specifically, its role in promoting T cell longevity, growth, replication, and preservation of memory, various scientific groups have used IL-7 as a molecular enhancer to improve the immune response generated by cancer vaccines and maintain long-lasting memory reactions against cancer (Zhao et al., 2022). In conclusion, our study suggests a possible relationship between IL-7 and inflammatory tumors in OCPC, which may have some clinical significance for exploring the pathogenesis of OCPC and reducing its incidence.
We also explored potential mediators in the cytokines-OCPC pathway. Previous MR studies have shown that MIP1α/CCL3 and IL-7 are associated with OCPC risk. In observational studies, smoking, drinking, body mass index, type 2 diabetes, hypertension, and HPV16/18 infection have been suggested as risk factors for the development of OCPC. To account for the above potential mediating factors, the MVMR model was further applied to examine the possibility of the observed confounders introducing horizontal pleiotropy. However, MVMR analysis showed that OCPC was no longer significantly associated with these factors at the conventional 5% level. Therefore, it seems unlikely that these factors play a substantial role in the pathway of inflammatory exposure. Notably, MIP1α/CCL3 and IL-7 still have estimated causal effects on OCPC even if mediating factors are excluded.
Bidirectional MR analysis during the OCPC stage suggested that OCPC is potentially associated with alterations in IL-4 levels in blood, despite the limited available evidence on their correlation. Hypotheses propose that elevated levels of IL-4 might signify the presence of analogous immune-suppressing mechanisms within the microenvironment of OCPC. These mechanisms potentially facilitate the expansion of tumors and evasion of immune vigilance. Previous studies have reported significant IL-4 expression in the tumor microenvironment (Setrerrahmane and Xu, 2017; Gao et al., 2021). Inhibiting it can alter inflammation and improve the tumor’s response to immunotherapy (Ito et al., 2017). To sum up, the complex interactions between the immune system and the tumor are reflected by the varying IL-4 levels in the blood of patients with OCPC. These results highlight the importance of immune regulation and the imbalance of cytokines in the development of OCPC. Therefore, additional research is necessary to understand the exact mechanisms behind these changes in cytokines and how they impact the occurrence, advancement, and treatment strategies for OCPC.
This study is remarkable for its novel use of large-scale GWAS data to investigate multiple exposure factors related to OCPC risk, which enhances the stability and accuracy of estimating the effects. Second, the bidirectional MR design is employed to mitigate confounding factors and eliminate reverse causality. Third, the MVMR model was further applied to examine the possibility of introducing horizontal pleiotropy by common confounders of OCPC. Finally, this study comprehensively investigates the association between OCPC and 41 circulating cytokines, making it the most extensive MR study to date on this topic. They may provide oncologists and therapists with new perspectives and implications for designing more personalized and effective treatments for patients who may develop or suffer from OCPC. For example, host genotype could be exploited to enable more precise diagnosis and treatment by investigating inflammatory exposure factors in clinical patients. The use of monoclonal antibodies to reduce the concentration of certain inflammatory factors or exogenous supplementation of specific inflammatory factors can also be studied to prevent or treat OCPC. While the MR design offers advantages, this study also has limitations. First, although GWAS statistical data of European ancestry were used to mitigate population bias, the generalizability of our findings across different populations remains uncertain. Additional GWAS with larger sample sizes are necessary to validate and update the findings of this study. Moreover, the results of these studies may be confounded by various factors, including the production and interplay of cytokines. Despite conducting a MVMR analysis, it seems that the estimates of time-varying exposure may not accurately reflect the causal effects within a specific time period. When the exposure only affects the outcome at a few distinct time points and the risk factors in the MVMR analysis are the exposure values at these specific time points, it is possible to obtain a reliable estimation of the causal effect during these time points. However, if these time points are not correctly identified, estimates obtained from ambiguous models will be incorrect. This can mislead any inferences made about the magnitude, existence, or direction of the causal effects. Finally, it is important to note that all research findings require validation in clinical and basic research. As a result, caution should be exercised in interpreting potential causal relationships, and further investigation of potential physiopathological mechanisms is needed.
Our comprehensive MR analysis revealed potential causal relationships between 41 circulating cytokines and OCPC, providing new insights into their interactions. The following conclusions were drawn: MIP1α/CCL3 and IL-7 may be potential factors driving OCPC. And susceptibility to OCPC may also increase IL-4 levels in prognosis. However, due to the limitations of our study, including the relatively small number of OCPC cases and the use of only a single ancestry population, our findings should be validated in a larger cohort and the exact underlying biological mechanisms require further investigation.
The datasets presented in this study can be found in online repositories. The names of the repository/repositories and accession number(s) can be found in the article/Supplementary Material.
Ethical approval was not required for the study involving humans in accordance with the local legislation and institutional requirements. Written informed consent to participate in this study was not required from the participants or the participants’ legal guardians/next of kin in accordance with the national legislation and the institutional requirements.
KW: Conceptualization, Data curation, Methodology, Software, Validation, Visualization, Writing–original draft, Writing–review and editing. QS: Conceptualization, Methodology, Supervision, Validation, Visualization, Writing–original draft. DL: Conceptualization, Supervision, Validation, Writing–review and editing. JL: Formal Analysis, Software, Validation, Writing–review and editing. DW: Formal Analysis, Investigation, Validation, Writing–review and editing. XZ: Formal Analysis, Investigation, Supervision, Writing–review and editing. LG: Conceptualization, Project administration, Supervision, Writing–review and editing.
The author(s) declare financial support was received for the research, authorship, and/or publication of this article. Medical Wisdom Research Fund supported this research by the Heilongjiang Provincial Natural Science Foundation (LH2021H033).
The authors thank all investigators and participants contributing to the GWAS, YFS, and FINRISK studies.
The authors declare that the research was conducted in the absence of any commercial or financial relationships that could be construed as a potential conflict of interest.
All claims expressed in this article are solely those of the authors and do not necessarily represent those of their affiliated organizations, or those of the publisher, the editors and the reviewers. Any product that may be evaluated in this article, or claim that may be made by its manufacturer, is not guaranteed or endorsed by the publisher.
The Supplementary Material for this article can be found online at: https://www.frontiersin.org/articles/10.3389/fgene.2023.1321484/full#supplementary-material
Ahola-Olli, A. V., Wurtz, P., Havulinna, A. S., Aalto, K., Pitkanen, N., Lehtimaki, T., et al. (2017). Genome-wide association study identifies 27 loci influencing concentrations of circulating cytokines and growth factors. Am. J. Hum. Genet. 100, 40–50. doi:10.1016/j.ajhg.2016.11.007
Argiris, A., Karamouzis, M. V., Raben, D., and Ferris, R. L. (2008). Head and neck cancer. Lancet 371, 1695–1709. doi:10.1016/S0140-6736(08)60728-X
Barata, J. T., Durum, S. K., and Seddon, B. (2019). Flip the coin: IL-7 and IL-7R in health and disease. Nat. Immunol. 20, 1584–1593. doi:10.1038/s41590-019-0479-x
Bhat, A. A., Nisar, S., Singh, M., Ashraf, B., Masoodi, T., Prasad, C. P., et al. (2022). Cytokine- and chemokine-induced inflammatory colorectal tumor microenvironment: emerging avenue for targeted therapy. Cancer Commun. (Lond) 42, 689–715. doi:10.1002/cac2.12295
Bierie, B., and Moses, H. L. (2006). TGF-beta and cancer. Cytokine Growth Factor Rev. 17, 29–40. doi:10.1016/j.cytogfr.2005.09.006
Bowden, J., Davey Smith, G., and Burgess, S. (2015). Mendelian randomization with invalid instruments: effect estimation and bias detection through Egger regression. Int. J. Epidemiol. 44, 512–525. doi:10.1093/ije/dyv080
Burgess, S., Scott, R. A., Timpson, N. J., Davey Smith, G., Thompson, S. G., and Consortium, E.-I. (2015). Using published data in Mendelian randomization: a blueprint for efficient identification of causal risk factors. Eur. J. Epidemiol. 30, 543–552. doi:10.1007/s10654-015-0011-z
Burgess, S., Thompson, S. G., and Collaboration, C. C. G. (2011). Avoiding bias from weak instruments in Mendelian randomization studies. Int. J. Epidemiol. 40, 755–764. doi:10.1093/ije/dyr036
Chen, X., Kong, J., Diao, X., Cai, J., Zheng, J., Xie, W., et al. (2020). Depression and prostate cancer risk: a Mendelian randomization study. Cancer Med. 9, 9160–9167. doi:10.1002/cam4.3493
Da Silva, J. M., Moreira Dos Santos, T. P., Sobral, L. M., Queiroz-Junior, C. M., Rachid, M. A., Proudfoot, A. E. I., et al. (2017). Relevance of CCL3/CCR5 axis in oral carcinogenesis. Oncotarget 8, 51024–51036. doi:10.18632/oncotarget.16882
Fry, T. J., and Mackall, C. L. (2002). Interleukin-7: from bench to clinic. Blood 99, 3892–3904. doi:10.1182/blood.v99.11.3892
Gao, S., Hsu, T. W., and Li, M. O. (2021). Immunity beyond cancer cells: perspective from tumor tissue. Trends Cancer 7, 1010–1019. doi:10.1016/j.trecan.2021.06.007
Hanahan, D., and Coussens, L. M. (2012). Accessories to the crime: functions of cells recruited to the tumor microenvironment. Cancer Cell 21, 309–322. doi:10.1016/j.ccr.2012.02.022
Hartwig, F. P., Davies, N. M., Hemani, G., and Davey Smith, G. (2016). Two-sample Mendelian randomization: avoiding the downsides of a powerful, widely applicable but potentially fallible technique. Int. J. Epidemiol. 45, 1717–1726. doi:10.1093/ije/dyx028
Hong, C., Luckey, M. A., and Park, J. H. (2012). Intrathymic IL-7: the where, when, and why of IL-7 signaling during T cell development. Semin. Immunol. 24, 151–158. doi:10.1016/j.smim.2012.02.002
Hsu, C. J., Wu, M. H., Chen, C. Y., Tsai, C. H., Hsu, H. C., and Tang, C. H. (2013). AMP-activated protein kinase activation mediates CCL3-induced cell migration and matrix metalloproteinase-2 expression in human chondrosarcoma. Cell Commun. Signal 11, 68. doi:10.1186/1478-811X-11-68
Hwang, T. L., Lee, L. Y., Wang, C. C., Liang, Y., Huang, S. F., and Wu, C. M. (2012). CCL7 and CCL21 overexpression in gastric cancer is associated with lymph node metastasis and poor prognosis. World J. Gastroenterol. 18, 1249–1256. doi:10.3748/wjg.v18.i11.1249
Ito, S. E., Shirota, H., Kasahara, Y., Saijo, K., and Ishioka, C. (2017). IL-4 blockade alters the tumor microenvironment and augments the response to cancer immunotherapy in a mouse model. Cancer Immunol. Immunother. 66, 1485–1496. doi:10.1007/s00262-017-2043-6
Jiang, Q., Li, W. Q., Aiello, F. B., Mazzucchelli, R., Asefa, B., Khaled, A. R., et al. (2005). Cell biology of IL-7, a key lymphotrophin. Cytokine Growth Factor Rev. 16, 513–533. doi:10.1016/j.cytogfr.2005.05.004
Kalaoja, M., Corbin, L. J., Tan, V. Y., Ahola-Olli, A. V., Havulinna, A. S., Santalahti, K., et al. (2021). The role of inflammatory cytokines as intermediates in the pathway from increased adiposity to disease. Obes. (Silver Spring) 29, 428–437. doi:10.1002/oby.23060
Kim, J. H., Kim, W. S., Hong, J. Y., Ryu, K. J., Kim, S. J., and Park, C. (2017). Epstein-Barr virus EBNA2 directs doxorubicin resistance of B cell lymphoma through CCL3 and CCL4-mediated activation of NF-κB and Btk. Oncotarget 8, 5361–5370. doi:10.18632/oncotarget.14243
Lawlor, D. A., Harbord, R. M., Sterne, J. A., Timpson, N., and Davey Smith, G. (2008). Mendelian randomization: using genes as instruments for making causal inferences in epidemiology. Stat. Med. 27, 1133–1163. doi:10.1002/sim.3034
Leemans, C. R., Snijders, P. J. F., and Brakenhoff, R. H. (2018). The molecular landscape of head and neck cancer. Nat. Rev. Cancer 18, 269–282. doi:10.1038/nrc.2018.11
Leon, X., Bothe, C., Garcia, J., Parreno, M., Alcolea, S., Quer, M., et al. (2015). Expression of IL-1α correlates with distant metastasis in patients with head and neck squamous cell carcinoma. Oncotarget 6, 37398–37409. doi:10.18632/oncotarget.6054
Lesseur, C., Diergaarde, B., Olshan, A. F., Wunsch-Filho, V., Ness, A. R., Liu, G., et al. (2016). Genome-wide association analyses identify new susceptibility loci for oral cavity and pharyngeal cancer. Nat. Genet. 48, 1544–1550. doi:10.1038/ng.3685
Levina, V., Nolen, B. M., Marrangoni, A. M., Cheng, P., Marks, J. R., Szczepanski, M. J., et al. (2009). Role of eotaxin-1 signaling in ovarian cancer. Clin. Cancer Res. 15, 2647–2656. doi:10.1158/1078-0432.CCR-08-2024
Liao, Y. Y., Tsai, H. C., Chou, P. Y., Wang, S. W., Chen, H. T., Lin, Y. M., et al. (2016). CCL3 promotes angiogenesis by dysregulation of miR-374b/VEGF-A axis in human osteosarcoma cells. Oncotarget 7, 4310–4325. doi:10.18632/oncotarget.6708
Li, P., Wang, H., Guo, L., Gou, X., Chen, G., Lin, D., et al. (2022). Association between gut microbiota and preeclampsia-eclampsia: a two-sample Mendelian randomization study. BMC Med. 20, 443. doi:10.1186/s12916-022-02657-x
Li, Y., Lu, J., Wang, J., Deng, P., Meng, C., and Tang, H. (2021). Inflammatory cytokines and risk of ischemic stroke: a mendelian randomization study. Front. Pharmacol. 12, 779899. doi:10.3389/fphar.2021.779899
Luo, J. L., Tan, W., Ricono, J. M., Korchynskyi, O., Zhang, M., Gonias, S. L., et al. (2007). Nuclear cytokine-activated IKKalpha controls prostate cancer metastasis by repressing Maspin. Nature 446, 690–694. doi:10.1038/nature05656
Machiela, M. J., and Chanock, S. J. (2015). LDlink: a web-based application for exploring population-specific haplotype structure and linking correlated alleles of possible functional variants. Bioinformatics 31, 3555–3557. doi:10.1093/bioinformatics/btv402
Mantovani, A. (2010). Molecular pathways linking inflammation and cancer. Curr. Mol. Med. 10, 369–373. doi:10.2174/156652410791316968
Ozga, A. J., Chow, M. T., and Luster, A. D. (2021). Chemokines and the immune response to cancer. Immunity 54, 859–874. doi:10.1016/j.immuni.2021.01.012
Pan, S., Wu, S., Wei, Y., Liu, J., Zhou, C., Chen, T., et al. (2023). Exploring the causal relationship between inflammatory cytokines and inflammatory arthritis: a Mendelian randomization study. Cytokine 173, 156446. doi:10.1016/j.cyto.2023.156446
Perez-Sayans, M., Somoza-Martin, J. M., Barros-Angueira, F., Reboiras-Lopez, M. D., Gandara Rey, J. M., and Garcia-Garcia, A. (2009). Genetic and molecular alterations associated with oral squamous cell cancer (Review). Oncol. Rep. 22, 1277–1282. doi:10.3892/or_00000565
Pikarsky, E., Porat, R. M., Stein, I., Abramovitch, R., Amit, S., Kasem, S., et al. (2004). NF-kappaB functions as a tumour promoter in inflammation-associated cancer. Nature 431, 461–466. doi:10.1038/nature02924
Setrerrahmane, S., and Xu, H. (2017). Tumor-related interleukins: old validated targets for new anti-cancer drug development. Mol. Cancer 16, 153. doi:10.1186/s12943-017-0721-9
Siegel, R. L., Miller, K. D., and Jemal, A. (2020). Cancer statistics, 2020. CA Cancer J. Clin. 70, 7–30. doi:10.3322/caac.21590
Silva, T. A., Ribeiro, F. L., Oliveira-Neto, H. H., Watanabe, S., Alencar Rde, C., Fukada, S. Y., et al. (2007). Dual role of CCL3/CCR1 in oral squamous cell carcinoma: implications in tumor metastasis and local host defense. Oncol. Rep. 18, 1107–1113. doi:10.3892/or.18.5.1107
Skrivankova, V. W., Richmond, R. C., Woolf, B. A. R., Davies, N. M., Swanson, S. A., Vanderweele, T. J., et al. (2021). Strengthening the reporting of observational studies in epidemiology using mendelian randomisation (STROBE-MR): explanation and elaboration. BMJ 375, n2233. doi:10.1136/bmj.n2233
Smith, G. D., and Ebrahim, S. (2003). Mendelian randomization': can genetic epidemiology contribute to understanding environmental determinants of disease? Int. J. Epidemiol. 32, 1–22. doi:10.1093/ije/dyg070
Song, J., Li, A., Qian, Y., Liu, B., Lv, L., Ye, D., et al. (2022). Genetically predicted circulating levels of cytokines and the risk of cancer. Front. Immunol. 13, 886144. doi:10.3389/fimmu.2022.886144
Soria, G., and Ben-Baruch, A. (2008). The inflammatory chemokines CCL2 and CCL5 in breast cancer. Cancer Lett. 267, 271–285. doi:10.1016/j.canlet.2008.03.018
Staley, J. R., Blackshaw, J., Kamat, M. A., Ellis, S., Surendran, P., Sun, B. B., et al. (2016). PhenoScanner: a database of human genotype-phenotype associations. Bioinformatics 32, 3207–3209. doi:10.1093/bioinformatics/btw373
Storey, J. D., and Tibshirani, R. (2003). Statistical significance for genomewide studies. Proc. Natl. Acad. Sci. U. S. A. 100, 9440–9445. doi:10.1073/pnas.1530509100
Sung, H., Ferlay, J., Siegel, R. L., Laversanne, M., Soerjomataram, I., Jemal, A., et al. (2021). Global cancer statistics 2020: GLOBOCAN estimates of incidence and mortality worldwide for 36 cancers in 185 countries. CA Cancer J. Clin. 71, 209–249. doi:10.3322/caac.21660
Tian, H., and Burgess, S. (2023). Estimation of time-varying causal effects with multivariable Mendelian randomization: some cautionary notes. Int. J. Epidemiol. 52, 846–857. doi:10.1093/ije/dyac240
Todoric, J., Antonucci, L., and Karin, M. (2016). Targeting inflammation in cancer prevention and therapy. Cancer Prev. Res. (Phila) 9, 895–905. doi:10.1158/1940-6207.CAPR-16-0209
Verbanck, M., Chen, C. Y., Neale, B., and Do, R. (2018). Detection of widespread horizontal pleiotropy in causal relationships inferred from Mendelian randomization between complex traits and diseases. Nat. Genet. 50, 693–698. doi:10.1038/s41588-018-0099-7
Voronov, E., Shouval, D. S., Krelin, Y., Cagnano, E., Benharroch, D., Iwakura, Y., et al. (2003). IL-1 is required for tumor invasiveness and angiogenesis. Proc. Natl. Acad. Sci. U. S. A. 100, 2645–2650. doi:10.1073/pnas.0437939100
Wu, T., Hong, Y., Jia, L., Wu, J., Xia, J., Wang, J., et al. (2016). Modulation of IL-1β reprogrammes the tumor microenvironment to interrupt oral carcinogenesis. Sci. Rep. 6, 20208. doi:10.1038/srep20208
Zhang, W., Wang, H., Sun, M., Deng, X., Wu, X., Ma, Y., et al. (2020). CXCL5/CXCR2 axis in tumor microenvironment as potential diagnostic biomarker and therapeutic target. Cancer Commun. (Lond) 40, 69–80. doi:10.1002/cac2.12010
Keywords: oral cavity and pharyngeal cancer, inflammation factors, cytokines, mendelian randomization, HNSCC, genome-wide association study
Citation: Wu K, Sun Q, Liu D, Lu J, Wen D, Zang X and Gao L (2024) Genetically predicted circulating levels of cytokines and the risk of oral cavity and pharyngeal cancer: a bidirectional mendelian-randomization study. Front. Genet. 14:1321484. doi: 10.3389/fgene.2023.1321484
Received: 14 October 2023; Accepted: 26 December 2023;
Published: 11 January 2024.
Edited by:
Ziheng Wang, University of Macau, ChinaReviewed by:
Jiahao Cai, Guangzhou Medical University, ChinaCopyright © 2024 Wu, Sun, Liu, Lu, Wen, Zang and Gao. This is an open-access article distributed under the terms of the Creative Commons Attribution License (CC BY). The use, distribution or reproduction in other forums is permitted, provided the original author(s) and the copyright owner(s) are credited and that the original publication in this journal is cited, in accordance with accepted academic practice. No use, distribution or reproduction is permitted which does not comply with these terms.
*Correspondence: Li Gao, Z2FvbGkwNTE5QDEyNi5jb20=
Disclaimer: All claims expressed in this article are solely those of the authors and do not necessarily represent those of their affiliated organizations, or those of the publisher, the editors and the reviewers. Any product that may be evaluated in this article or claim that may be made by its manufacturer is not guaranteed or endorsed by the publisher.
Research integrity at Frontiers
Learn more about the work of our research integrity team to safeguard the quality of each article we publish.