- 1Department of Oncology Surgery, The Affiliated Hospital of Northwest University, Xi’an, China
- 2Department of Intensive Care Unit, The First Affiliated Hospital of Xi’an Jiaotong University, Xi’an, China
- 3Department of Surgical Oncology, The First Affiliated Hospital of Xi’an Jiaotong University, Xi’an, China
Objective: Numerous studies have highlighted an association between the gut microbiota (GM) and thyroid tumors. Employing Mendelian randomization methodology, we seek to elucidate the causal link between the gut microbiota and thyroid neoplasms.
Methods: We procured data from the Mibiogen database encompassing 211 distinct gut microbiota taxa, alongside extensive genome-wide association studies (GWAS) summary data for differentiated thyroid carcinoma (DTC). Our principal analytical approach involved the application of the Inverse-Variance Weighted method (IVW) within the framework of Mendelian randomization. Simultaneously, we conducted sensitivity analyses to assess result heterogeneity, horizontal pleiotropy, and outcome stability.
Results: IVW analysis revealed a dual role of the GM in thyroid carcinoma. The phylum Actinobacteria (OR, 0.249 [95% CI, 0.121–0.515]; p < 0.001) was associated with a decreased risk of DTC. Conversely, the genus Ruminiclostridium9 (OR, 11.276 [95% CI, 4.406–28.860]; p < 0.001), class Mollicutes (OR, 5.902 [95% CI, 1.768–19.699]; p = 0.004), genus RuminococcaceaeUCG004 (OR, 3.831 [95% CI, 1.516–9.683]; p = 0.005), genus Paraprevotella (OR, 3.536 [95% CI, 1.330–9.401]; p = 0.011), and phylum Tenericutes (OR, 5.902 [95% CI, 1.768–19.699]; p = 0.004) were associated with an increased risk of DTC.
Conclusion: Our findings underscore that the presence of genus Ruminiclostridium9, class Mollicutes, genus RuminococcaceaeUCG004, genus Paraprevotella, and phylum Tenericutes is associated with an elevated risk of DTC, whereas the presence of the phylum Actinobacteria is linked to a decreased risk. These discoveries enhance our comprehension of the relationship between the GM and DTC.
1 Introduction
Thyroid carcinoma, a common endocrine neoplasm of the head and neck, has experienced a steady increase in incidence, currently ranking as the fifth most prevalent cancer globally (Bray et al., 2018; WHO, 2020). Projections suggest that following its current trajectory, thyroid malignancies will become the fourth most common cancer in the United States by 2030 (Gonçalves et al., 2017). In 2020, global age-standardized incidence rates for thyroid cancer were 10.1 cases per 100,000 females and 3.1 cases per 100,000 males, with corresponding mortality rates of 0.5 and 0.3 cases per 100,000, respectively (Pizzato et al., 2022). An epidemiological survey covering 24% of the population in China (Yao et al., 2023) revealed that in 2019, the age-standardized incidence and mortality rates for thyroid cancer were 2.05 and 0.39 per 100,000, respectively. Over the last 30 years, the International Agency for Research on Cancer has noted an increasing incidence of thyroid cancer in diverse populations worldwide. In the United States, from 1970 to 2013, the annual growth rate of thyroid cancer incidence was reported to be 3% (Lim et al., 2017). Fortunately, the mortality rates for both males and females in most countries exhibit a stable or declining trend (Huang et al., 2023). DTC accounts for over 90% of all pathological diagnoses, with papillary carcinoma being its predominant histological subtype (Gu et al., 2014).The etiology of thyroid tumors is multifactorial, involving chromosomal mutations, genetic predisposition (Bonnefond and Davies, 2014), estrogen levels (Luo et al., 2016), ionizing radiation exposure (Bonnefond and Davies, 2014), autoimmune thyroid disorders (Khatami, 2009), and other factors. However, the risk factors for thyroid tumors are not fully understood, requiring additional research to uncover their pathogenic mechanisms.
Currently, the National Comprehensive Cancer Network (NCCN) guidelines recommend primary surgical intervention for DTC, reserving radioactive iodine-131 treatment for specific patient subsets (Haddad et al., 2022). Considering the global prevalence of thyroid tumors and their healthcare burden, as well as their profound impact on the wellbeing of affected individuals, our research is dedicated to uncovering the etiological underpinnings of this affliction.
Humans have coexisted with microorganisms throughout their existence, hosting a diverse array of microbes within various bodily niches, including the oral cavity, respiratory tract, gastrointestinal tract, genitourinary tract, and skin. Among these, the gut harbors the most intricate microbial ecosystem (Jiang et al., 2022). The human gut, in particular, teems with an assembly of microbial denizens numbering in the billions, with bacteria occupying the central stage (Gill et al., 2006). Such a vast consortium of GM also fulfills distinctive roles. Presently, microbiota are acknowledged for their substantial contributions to vitamin synthesis (B-complex vitamins, folate, vitamin K, among others) (Gu et al., 2016; Fang et al., 2017), facilitation of dietary fiber digestion, and regulation of immune responses (Bastiaanssen et al., 2019). Beyond these functions, microbiota also exhibit intricate associations with various diseases, encompassing gastrointestinal disorders, psychiatric illnesses, respiratory maladies, autoimmune conditions, and significantly, diverse malignancies, including lung, breast, colorectal, and esophageal cancers (Stebbings et al., 2002; Wang et al., 2016; Ishaq et al., 2021; Vitale et al., 2021).
A plethora of evidence has pointed toward the association between GM and thyroid malignancies, including thyroid carcinoma (Stebbings et al., 2002; Ishaq et al., 2021). (Ejtahed et al., 2020) Thyroid cancer patients exhibit dysbiosis in the gut microbiota, characterized by a reduction in the relative abundance of Faecalibacterium prausnitzii. Interestingly, an increase in the abundance of Faecalibacterium prausnitzii is observed after Radioactive Iodine Therapy (RAIT) (Fernandes et al., 2023). Lu et al. have identified significant alterations in the composition of the gut microbiota in thyroid cancer patients, with the Bacteroides enterotype emerging as the predominant bacterial type (Lu et al., 2022). Furthermore, gene sequencing results indicate a higher abundance of Firmicutes (Liu et al., 2021). In a study encompassing 74 patients, high-throughput sequencing was utilized to compare the microbial structural characteristics of 20 thyroid carcinoma patients, 18 thyroid nodule patients, and 36 healthy controls. The results underscored a close relationship between thyroid carcinoma, thyroid nodules, and altered microbiota (Zhang et al., 2019). Despite numerous indications suggesting an association between GM and thyroid malignancies, our understanding of this relationship remains incomplete, as these studies have not yet established causal links between thyroid tumors and GM.
Mendelian randomization represents an analytical framework harnessing genetic variation as instrumental variables (IV) to infer causal relationships between specific risk factors (i.e., exposures) and particular phenotypes (i.e., outcomes). Genetic variation, in this context, predominantly alludes to single nucleotide polymorphisms, signifying variations in specific nucleotides within the genetic material. In the realm of clinical investigation, myriad confounding factors often obscure the precision of our conclusions, rendering causal inferences tentative at best. The intrinsic merit of Mendelian randomization lies in its capacity to circumvent the impracticability of randomized controlled trials, such as the random allocation of microbiota to study individuals. Instead, this method leverages the natural grouping of single nucleotide polymorphisms (SNPs) and employs statistical techniques to ascertain the influence of SNPs on exposure and outcomes. nsequently, it helps to clarify causal associations between exposures and outcomes. Notably, owing to the even distribution of SNP loci, Mendelian randomization outcomes remain comparatively impervious to the interference of confounding factors, thereby conferring results akin to those derived from randomized controlled trials (Davies et al., 2018).
We utilize Mendelian randomization to disentangle the impact of confounding factors, enabling a precise evaluation of the causal relationship between GM and DTC., and Figure 1 provides an overview of the main research approach in this paper. This study’s overarching goal resides in employing Mendelian randomization as a methodological prism, utilizing genetic variation as instrumental variables to elucidate the causal nexus between microbiota and DTC. In doing so, we aspire to contribute novel evidence to the etiological and therapeutic paradigms within the domain of thyroid pathology.
2 Methods
2.1 Ethics statement
As the data used in this study comes from publicly available databases, after obtaining ethical approval from the Affiliated Hospital of Northwest University, Xi’an NO.3 hospital, the committee deemed formal ethical approval unnecessary. This decision was predicated upon the utilization of publicly accessible data devoid of identifiable patient information.
2.2 Study design
In this study, we define exposure as the GM, the outcome as malignant thyroid neoplasms, and instrumental variables as single nucleotide polymorphism (SNP) loci. In accordance with this premise, we discerned GM significantly associated with malignant thyroid neoplasms and subsequently proceeded with Mendelian randomization analysis. Throughout the course of MR analysis, we adhere to the following assumptions.
1. Associational Hypothesis: That is, SNP loci under investigation and GM demonstrate robust correlations. In our study, the significance threshold for the associational hypothesis is set at P < 1E-5.
2. Independence of SNPs and Confounding Factors: Among the SNPs ultimately incorporated into the MR study, those SNP loci exhibiting associations with either tumors or GM were excluded.
3. Exclusivity of Instrumental Variables’ Impact on Outcomes through Exposure: Instrumental variables should solely affect outcomes through the exposure and remain inert to other pathways, such as confounding. In essence, there should be no pleiotropy.
2.3 Data collection
The data pertaining to GM emanates from the consortium’s whole-genome association study summary data, Mibiogen. This dataset encapsulates 211 distinct taxonomic groups within the GM, spanning six taxonomic levels: kingdom, phylum, class, order, family, and genus. Access to this data can be procured from the website (https://mibiogen.gcc.rug.nl/). Notably, eight unidentified bacterial species were excluded from subsequent microbiota SNP locus analyses, leaving a total of 203 microbial SNPs for further investigation.
Thyroid tumor data, on the other hand, was sourced from Aleksandra Köhler et al.‘s prospective study (Köhler et al., 2013), which conducted a comprehensive genome-wide association study encompassing 701 patients afflicted with DTC. Diagnoses of thyroid tumors were ascertained through pathological results furnished by the Cisanello Hospital in Pisa, a prominent Italian referral center for thyroid disorders.
2.4 Variable selection
To assess the correlation between instrumental variables and microbiota, a filtration process for employed instrumental variables was enacted, involving the following steps:
1. The GM data, having been downloaded from the Mibiogen website, was validated and subsequently imported into R for analysis.
2. SNP loci exhibiting a stronger correlation with exposure were identified and filtered out based on a threshold of P < 1E-5 in the initial filtration process.
3. Instrumental variables demonstrating linkage disequilibrium were excluded. A standard of r2 > 0.001 and a physical distance (Kb) of 10,000 were employed for the removal of SNP loci exhibiting r2 values exceeding 0.001 with the most significant SNP within a 10,000 Kb range.
4. F-statistics were computed to assess the strength of instrumental variables. The calculation of F-statistics was predicated on beta values and standard errors (SE) for SNPs and exposure. In our study, all instrumental variables exhibited F-statistics exceeding 10.
5. SNP loci not conforming to the independence assumption were eliminated. We accessed the Phenoscanner database to identify secondary phenotypes associated with each SNP, verifying their correlation with confounding factors. SNP loci associated with both exposure outcomes were discarded based on criteria of p-value: < 1E-8 and r^2 > 0.8. Naturally, SNP loci directly correlated with thyroid tumors were also excluded. Following this comprehensive filtration, we gathered information regarding instrumental variables in outcomes and amalgamated effect sizes, commencing the MR analysis.
2.5 Statistical analyses
In the course of Mendelian randomization analysis, six distinct methodologies were employed, namely, IVW, IVW random-effects model, MR-Egger, MR-Egger bootstrap, Weighted Median, and Simple Median. Of these, the IVW analysis results, which calculated both the unadjusted p-values and the False Discovery Rate (FDR)-corrected p-values for each SNP locus, served as the primary analytical approach for this study. Multiple sensitivity analyses were additionally conducted, serving three primary objectives: firstly, to assess the robustness of the outcomes; secondly, to evaluate the potential presence of biases, including pleiotropy and data heterogeneity; and thirdly, to appraise the scenario where a specific instrumental variable unduly influenced the outcome.
To quantify heterogeneity in individual causal effects, Cochran’s Q was computed and subjected to examination, with a significance threshold of p ≤ 0.05 indicating the presence of pleiotropy. Within the context of heterogeneity testing, MR-Egger’s intercept and the Mendelian randomization residual sum and outlier (MR-PRESSO) method were employed. If the p-value exceeded 0.05, it indicated the absence of horizontal pleiotropy. All results underwent comprehensive visualization. To evaluate the scenario where a specific instrumental variable significantly impacted the outcome, a leave-one-out analysis was conducted by systematically excluding each SNP locus and observing the remaining SNPs’ Mendelian randomization. Finally, a reverse Mendelian randomization analysis was performed to ascertain the causal direction.
All statistical analyses were executed using the R programming language (https://www.r-project.org, R version 4.2.1). Statistical significance was deemed at p < 0.05. The initial date of analysis commenced in May 2023.
3 Results
3.1 Instrumental variable selection and initial MR results
Following our criteria, an initial set of 14,569 instrumental variable loci was established. Supplementary Table S1 provides a comprehensive breakdown of all microbiota details. By matching SNPs with thyroid tumor data, we obtained a subset of 3,302 SNPs.
Initial Mendelian randomization analysis yielded insights into the relationships between 203 GM and thyroid function, as presented in Supplementary Table S2. Based on the IVW-derived p-values, an initial selection identified 13 microbiota entities, namely,: Genus Ruminiclostridium 9 (ID:11357), Class Mollicutes (ID 3920), Phylum Tenericutes (ID:3919), Genus Ruminococcaceae UCG004 (ID:11362), Genus Paraprevotella (ID:962), Genus Ruminococcaceae UCG003 (ID:11361), Family Victivallaceae (ID:2,255), Genus Candidatus Soleaferrea (ID:11350), Phylum Actinobacteria (ID:400), However, through rigorous heterogeneity testing, horizontal pleiotropy assessments, and the exclusion of loci indirectly or directly related to thyroid tumor diseases, we ultimately distilled the selection down to six GM entities and 47 SNP loci as instrumental variables: Genus Ruminiclostridium 9 (ID:11357), Class Mollicutes (ID:3920), Genus Ruminococcaceae UCG004 (ID:11362), Genus Paraprevotella (ID:962), Phylum Actinobacteria (ID:400), Phylum Tenericutes (ID:3919).
The secondary features of these aforementioned SNPs were queried using PhenoScanner and are documented in Supplementary Table S3. It is noteworthy that these features have been confirmed as non-pleiotropic factors contributing to thyroid tumor etiology.
3.2 Detailed Mendelian randomization analysis results
We conducted Mendelian randomization analysis on the final set of six GM and 47 SNP loci. IVW results revealed significant associations as follows: genus Ruminiclostridium9(OR, 11.276, [95% CI, 4.406–28.860 ]; p < 0.001), class Mollicutes (OR, 5.902, [95% CI, 1.768–19.699]; p = 0.004), genus RuminococcaceaeUCG004(OR, 3.831, [95% CI, 1.516–9.683 ]; p = 0.005), genus Paraprevotella (OR, 3.536, [95% CI, 1.330–9.401 ]; p = 0.011), phylum Tenericutes (OR, 5.902 [95% CI, 1.768–19.699 ]; p = 0.004)exhibited an elevated risk of thyroid tumors, whereas phylum actinobacteria (OR, 0.249 [95% CI, 0.121–0.515]; p < 0.001)demonstrated a decreased risk of thyroid tumors. These findings are graphically depicted in the forest plot (Figure 2). Additional MR analysis outcomes are presented in Supplementary Table S4. In our findings, IVW and IVW-MRE methods demonstrated consistency, while other methodologies may yield differing results compared to IVW and IVW-MRE. We posit that the IVW approach derives an overall effect by weight-averaging estimates across distinct loci, whereas IVW-MRE additionally accounts for measurement error, employing a random-effects model to estimate the total effect. Discrepancies among other methods may be attributed to sample size, skewness, or heterogeneity. In case of inconsistencies, pay particular attention to the results from IVW and IVW-MRE methods, considering the unique attributes of alternative methodologies.
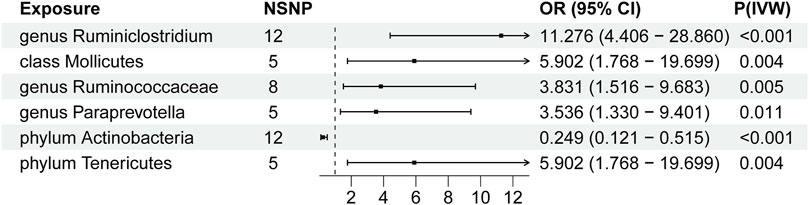
FIGURE 2. Forest Plot of Primary MR Analysis Results. NSNP = number of SNPs; OR = odds ratio; P = significance p-value; IVW = Inverse-Variance Weighted method.
To visualize our findings comprehensively, we presented all results in a scatter plot (Figure 3), where each data point represents an SNP, the upper and lower lines delineate confidence intervals, and the horizontal and vertical axes respectively denote the SNP’s effects on GM and thyroid tumor outcomes. The colored lines signify the fitting effects of the MR. Intriguingly, IVW and IVW-MRE methods exhibited remarkable consistency across all results.
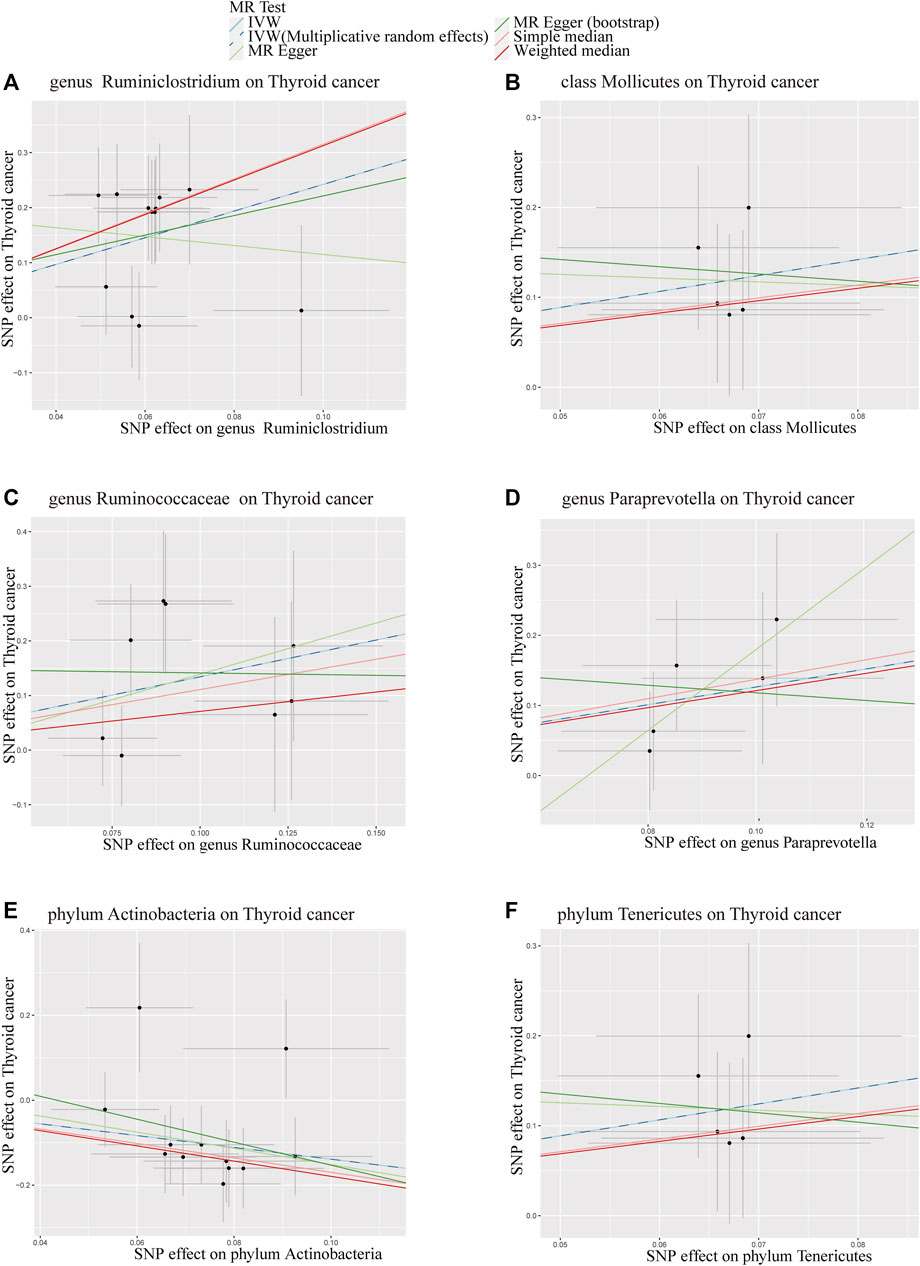
FIGURE 3. Scatter Plot of MR Analysis for the Influence of 6 Gut Microbiota on Thyroid Tumors. (A) = genus Ruminiclostridium; (B) = class Mollicutes; (C) = genus Ruminococcaceae; (D) = genus Paraprevotella; (E) = phylum Actinobacteria; (F) = phylum Tenericutes; MR Test = Statistical analysis methods.
3.3 Sensitivity analysis
Initially, we conducted heterogeneity checks on the results obtained from the selected six bacteria and 47 SNP loci. We observed that all I2 values for the microbiota were <50%, and the p-values obtained using two different methods were both greater than 0.05 (Table 1). This suggests that our results exhibited minimal heterogeneity. Additionally, the MR-PRESSO outlier test did not identify any anomalies (Table 1). To assess the horizontal pleiotropy of SNP loci, we utilized the global test from MRPRESSO and the MR-Egger intercept test. The p-values for all tests of horizontal pleiotropy exceeded 0.3, signifying that the impact of instrumental variables on thyroid cancer is unlikely to be influenced by factors other than the microbiota (Table 1).
In addition to MR-Egger and MR-PRESSO, we conducted individual SNP MR estimates (Supplementary Figure S1) and systematically removed individual SNPs to compute the remaining SNPs’ Mendelian randomization effects (Figure 4). Leave-one-out analysis, where each SNP is excluded in turn, showed that all error bars were consistently on the right or left of zero in Figure 4. This indicates minimal variation in the overall error bars, signifying high robustness in the results. The causal estimates were not driven by any single SNP. In the forest plot of Supplementary Figure S1, each solid horizontal line represents the results estimated using the Wald ratio method for individual SNPs, while the red line represents the composite outcome, reflecting the risk of thyroid cancer for each microbiota under the IVW method.
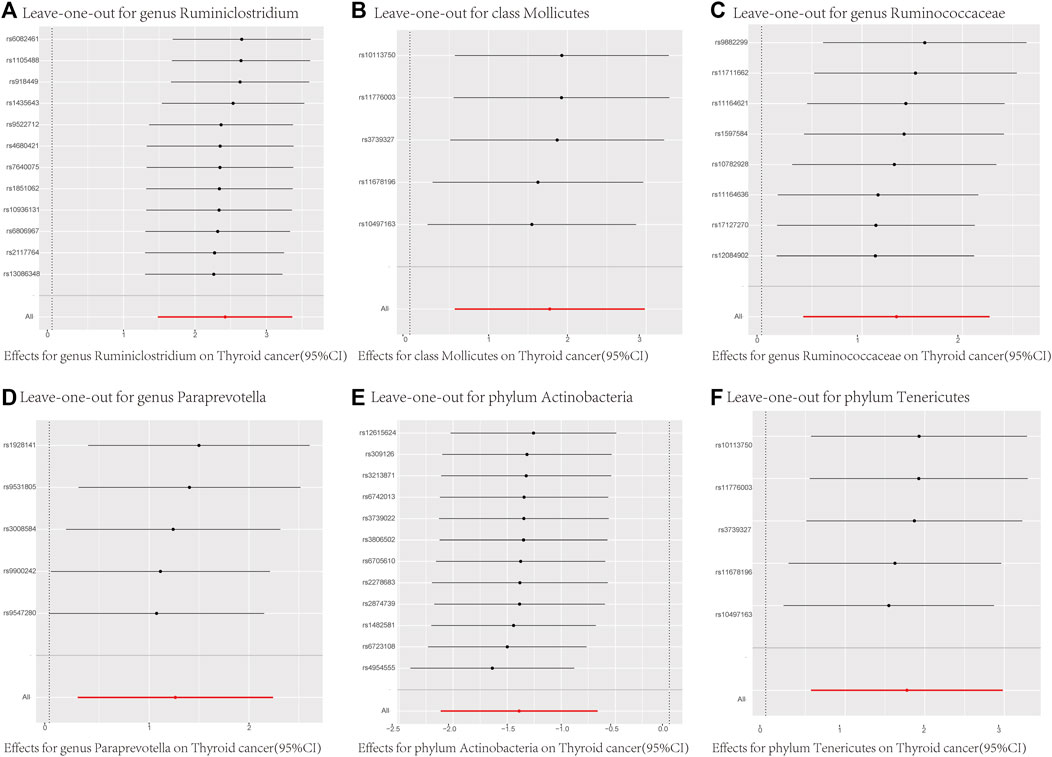
FIGURE 4. MR Analysis Results Using Leave-One-Out for Different SNP Loci of the 6 Gut Microbiota. (A) = genus Ruminiclostridium; (B) = class Mollicutes; (C) = genus Ruminococcaceae; (D) = genus Paraprevotella; (E) = phylum Actinobacteria; (F) = phylum Tenericutes; MR Test = Statistical analysis methods.
3.4 Reverse MR analysis
In the reverse MR analysis, exposure and outcome were interchanged. However, we did not observe any significant causal relationship between the outcome and exposure, except in the case of the Phylum Tenericutes using the MR Egger method, where the p-value exceeded 0.05 (Table 2).
We conducted a global test for horizontal pleiotropy using the MR-PRESSO method, and no evidence of horizontal pleiotropy was detected among the SNPs. Moreover, no outliers were detected in the outlier test. Hence, we have reasonable grounds to posit that these various bacteria are causative factors for thyroid tumors, rather than being outcomes of the condition.
4 Discussion
In spite of the prior research on the relationship between the GM and thyroid cancer, the concept of the association between DTC and the GM remains relatively uncharted (Samimi and Haghpanah, 2020). To the best of our knowledge, this study represents the first causal investigation into the link between the GM and DTC. Through a Mendelian randomization analysis involving two-sample datasets, we report that five microbiota entities, namely, genus Ruminiclostridium 9 (p < 0.001), class Mollicutes (p = 0.004), genus Ruminococcaceae UCG004 (p = 0.005), genus Paraprevotella (p = 0.011), and phylum Tenericutes (p = 0.004), are associated with an increased risk of developing non-differentiated thyroid carcinoma. Additionally, phylum Actinobacteria (p < 0.001) appears to be associated with a lower the risk of non-differentiated thyroid carcinoma. We utilized various sensitivity analysis techniques to affirm the reliability and robustness of our findings.
The GM is known to be influenced by a multitude of factors. Notably, infants born via cesarean section exhibit lower diversity in their GM(31). Throughout one’s life, the GM remains under the continual influence of various factors including diet, medication, genetics, environment, disease, and the use of antibiotics (Maslowski and Mackay, 2011). This substantiates the significance of the GM as a potential therapeutic target for diseases.
Most of the research concerning the GM has been concentrated on gastrointestinal diseases, such as colorectal cancer and inflammatory bowel disease. In the context of colorectal cancer, gut bacteria may promote tumorigenesis by influencing bile acid secretion or undergoing changes in their taxonomic composition. Decreased bile acid secretion results in gut dysbiosis, accelerating inflammation and DNA damage, thereby directly contributing to tumorigenesis (Louis et al., 2014; Ridlon et al., 2014). Furthermore, an increase in Fusobacterium nucleatum, a type of gut bacteria, can promote colon tumorigenesis through its metabolites and cytotoxicity, (Wu et al., 2004; Wu et al., 2009), thereby affecting signaling pathways such as E-cadherin, NF-κB, and STAT3 (Maslowski and Mackay, 2011; Rautava et al., 2012). These studies suggest that the role of microbiota in tumorigenesis seems to be associated with DNA damage and the regulation of local inflammation via metabolic products.
Microbes can impact thyroid disease through various pathways. The GM may influence the secretion of thyroid-stimulating hormones via the hypothalamus-pituitary axis, thereby playing a role in thyroid diseases (Fröhlich and Wahl, 2019). For instance, in studies of patients with Hashimoto’s thyroiditis (HT), a form of hypothyroidism, researchers observed dysbiosis in the patients’ GM, along with overgrowth of certain microbes (Ishaq et al., 2017; Zhao et al., 2018). This influence is thought to occur because thyroid-related nutrients need to be acquired through the gut (Knezevic et al., 2020). Furthermore, in Graves’ disease patients, there is a higher abundance of Bacteroidaceae and Prevotellaceae and a lower abundance of Veillonellaceae, Enterobacteriaceae, and Lachnospiraceae compared to healthy individuals (Ishaq et al., 2018). In the realm of researching the relationship between the GM and thyroid cancer, the first study proposing a potential connection between thyroid cancer and the GM was published in 2017. Shen et al. employed gas chromatography-time-of-flight mass spectrometry to analyze the serum of thyroid cancer patients with and without distant metastases. They found elevated levels of serum ammonia, pyruvic acid, and γ-aminobutyric acid in patients with distant metastases, suggesting a potential connection to differences in GM or diet (Shen et al., 2017). The first study to specifically investigate the relationship between thyroid cancer and the GM was conducted by Jing Feng in 2019 (Feng et al., 2019). Feng reported higher gut microbial richness and α-diversity in patients with TC compared to healthy controls, but the sample size was limited (30 patients from Harbin, China, versus 35 healthy controls), and the study design was observational. In other observational studies, GM taxa that were found to be increased in abundance in thyroid cancer patients included Clostridiaceae, Nesterenkonia, and Streptococcus (Zhang et al., 2019), while decreased taxa primarily included Lactobacillus (Zhang et al., 2019), Bacteroides, Clostridium, and Prevotella. These studies shed light on the potential link between the GM and thyroid cancer.
Our study highlights the significance of five specific microbiota entities—genus Ruminiclostridium 9, class Mollicutes, genus Ruminococcaceae UCG004, genus Paraprevotella, phylum Tenericutes, and phylum Actinobacteria—in relation to DTC.
Genus Ruminiclostridium 9, formerly known as Ruminoclostridium, is a characteristic bacterium found in the colon. Research indicates that interactions among colonic microorganisms are more intricate compared to the duodenum (Wu et al., 2020). This bacterium is associated with various diseases; it can reduce the risk of Alzheimer’s disease (OR 0.969, 95% CI 0.943–0.996, p = 0.009) (Ning et al., 2022). Furthermore, it has a positive correlation with cognitive function (Guo et al., 2021). In tumor immunology studies, Ruminiclostridium has shown a negative correlation with CD8+ T cells (Singh et al., 2023). In an autoimmune model of the central nervous system, medium-chain fatty acids (MCFAs) produced by this genus counteract the anti-inflammatory effects of short-chain fatty acids (SCFAs) by enhancing TH1 and TH17 cell differentiation (Haghikia et al., 2015).Therefore, certain genera, such as Ruminiclostridium 9, may contribute to the initiation of DTC through analogous mechanisms, potentially by reducing short-chain fatty acidsand modulating the immune response.
Class Mollicutes, representing the smallest self-replicating bacteria without cell walls, belongs to the phylum Tenericutes (Chernova et al., 2021). Mollicutes exhibit versatility, as they have been associated with both decreased relative abundance in severe depression patients (Zhu et al., 2019) and as a risk factor for Graves’ disease (Cao et al., 2023). Phylum Tenericutes has also been linked to various diseases, such as breast cancer (Niccolai et al., 2023), Crohn’s disease (Russo et al., 2022), lower risk of intrahepatic cholestasis of pregnancy (Li et al., 2023), and polycystic ovary syndrome (Li et al., 2023). However, research on the mechanisms underlying these associations remains limited. Genus Ruminococcaceae, like Ruminiclostridium, is present in the colonic mucosa. A decrease in Ruminococcaceae has been associated with various inflammatory bowel diseases, including ulcerative colitis and Crohn’s disease (Sokol et al., 2008; Joossens et al., 2011; Morgan et al., 2012). This bacterium produces short-chain fatty acids (SCFAs) and other small molecules, which serve as an energy source for colonic epithelial cells. A deficiency in these SCFAs may lead to disturbances and dysfunction in colonic mucosa (Young and Schmidt, 2004; Wong et al., 2006). SCFAs, particularly butyrate, are known to influence immune regulation and possess anti-inflammatory properties (Köhling et al., 2017), and butyrate can inhibit the activity and life cycle of cancer cells (Davie, 2003). This knowledge, seemingly at odds with our study results, suggests the involvement of unknown mechanisms. Interestingly, it has also been identified as a potential etiological factor contributing to Graves’ Disease (GD) (Cao et al., 2023). This association may be attributed to the ability of short-chain fatty acids (SCFA) to inhibit histone deacetylases (HDAC) and activate the re-expression of transport proteins in thyroid cancer cells, thereby inducing the differentiation of tumor cells and enhancing iodine uptake (Zhou et al., 2018; Rathod et al., 2020). Genus Paraprevotella has been the subject of several studies. It is a polymorphic, anaerobic, non-spore-forming Gram-negative rod isolated from human feces (Morotomi et al., 2009). This bacterium exhibits complex effects. On one hand, it is more abundant in individuals with genetic longevity (Liu et al., 2023) and correlates with small intestinal mucosal healing in Crohn’s disease patients (Hattori et al., 2020). On the other hand, it is more abundant in patients with heart failure and depression (Gutiérrez-Calabrés et al., 2020) and positively correlates with the severity of depression (Liśkiewicz et al., 2021). Previous studies have indicated its utility in distinguishing untreated primary hypothyroidism patients from healthy individuals.Currently, Paraprevotella is considered to have a positive correlation with plasma butyrate and valerate concentrations and contributes to the regulation of colonic motility, possibly through regulating fecal butyrate levels and serum IL-8 concentrations. The Paraprevotella strain proves to be an efficient pancreatic protease-degrading symbiont. The autolysis of pancreatic proteases facilitates bacterial invasion and destruction, potentially culminating in inflammation and injury, thereby creating a conducive environment for the onset of thyroid cancer.In summary, Paraprevotella is associated with various diseases, but its potential impact on human health remains unclear (Morotomi et al., 2009), and its specific mechanisms are yet to be explored. Phylum Actinobacteria is one of the most diverse bacterial phyla in nature (Lewin et al., 2016). Due to its diversity, it has both positive and negative effects on human health. Actinobacteria includes many bacteria that produce antibiotics, such as Streptomyces, which is known for synthesizing eptomycin, kanamycin, chloramphenicol, and erythromycin (Bérdy, 2005). It also encompasses several human health-threatening pathogens, such as Mycobacterium tuberculosis, which causes pulmonary tuberculosis. In the order Bifidobacteriales, members like Bifidobacterium are known for their beneficial effects on host health. A lower abundance of these bacteria has been associated with various diseases (Binda et al., 2018). Some researchers propose that species of Bifidobacterium in the human gut may contribute to host health by exerting antibacterial activity against pathogens and possibly by aiding in the development and function of the immune system as defensive symbionts (Servin, 2004). This aligns with our research findings. We also postulate that Actinobacteria may indirectly inhibit the occurrence of thyroid cancer by suppressing the processes of inflammation and oxidative stress.
What mechanisms might bacteria employ to induce DTC? Carcinogenesis primarily relies on two mechanisms: DNA damage and cell apoptosis, as well as the immune surveillance against tumor growth (Docimo et al., 2020; Liu et al., 2022). Intestinal bacteria can impact tumor proliferation through both of these mechanisms. New mechanisms involving bacteria and their metabolites or toxins causing direct DNA damage and carcinogenic mutations have been identified. For instance, infection with Enterococcus can lead to an increase in hydroxyl free radical production, resulting in DNA damage (Wang and Huycke, 2007). Additionally, oxidative stress can disrupt the homeostasis of the host gut microbiota (Riaz Rajoka et al., 2021). Therefore, drugs targeting both oxidative stress and gut microbiota may hold prognostic and therapeutic significance for thyroid cancer.Concerning immune surveillance, the gut microbiota significantly regulates the balance within the body and the development of immune cells. It modulates both innate and adaptive immune systems, particularly outside the intestinal tract (Maslowski and Mackay, 2011), making it a potential immune modulator (Pitt et al., 2016). As it is widely known, more than 70% of the entire immune system is associated with the gastrointestinal lymphoid tissues, and the gut microbiota can regulate immune balance and cell development (Maslowski and Mackay, 2011; Docimo et al., 2020). Certain metabolic products can also induce autoimmune reactions, leading to an imbalance in endocrine homeostasis and the occurrence of autoimmune diseases (Liu et al., 2022). Microbial dysbiosis can stimulate CD8 (+) T cells to promote chronic inflammation and early T-cell exhaustion, thereby diminishing the immune capacity against tumors (Yu et al., 2020). Therefore, the gut microbiota holds significant potential as a biomarker for predicting immune-related adverse events (Von Itzstein et al., 2020) and may influence the progression of thyroid cancer through immune modulation.In the future, we can develop novel strategies for diagnosing, predicting prognosis, actively monitoring, and intervening in DTC by studying the correlation between different bacterial enterotypes and thyroid cancer.This study also presents certain limitations. Firstly, we solely analyzed common microbiota and SNP loci, leaving unidentified microbiota and SNPs unexamined, thereby limiting our analysis. Secondly, our GWAS data was derived from individuals of European descent, and we did not identify other patient characteristics, which may restrict the generalizability of our conclusions to other populations, given the varying compositions of microbiota across different countries and ethnicities. Additionally, while we employed different SNP loci as “natural groupings,” it is essential to acknowledge the numerous unknown or potential interactions among distinct SNP loci. Finally, it is essential to note that this study is an observational investigation, lacking foundational research to mechanistically substantiate the observed outcomes. In the future, in addition to requiring more Genome-Wide Association Studies (GWAS) and microbiota data, further in-depth research is warranted to elucidate the mechanisms underlying the association between gut microbiota and thyroid tumor development. These studies will contribute to enhancing our understanding of the causal link between thyroid cancer and the gut microbiota.
In conclusion, our findings indicate a bidirectional role of GM in thyroid cancer. Genus Ruminiclostridium9, class Mollicutes, genus RuminococcaceaeUCG004, genus Paraprevotella, and phylum Tenericutes were associated with an increased risk of undifferentiated thyroid cancer, while phylum Actinobacteria (p < 0.001) was associated with a reduced risk of undifferentiated thyroid cancer. However, the underlying processes involved are intricate, necessitating further mechanistic research.
Data availability statement
The original contributions presented in the study are included in the article/Supplementary Material, further inquiries can be directed to the corresponding author.
Ethics statement
The requirement of ethical approval was waived by The Medical Ethics Committee of Xi’an Third Hospital for the studies involving humans because Utilization of publicly accessible data devoid of identifiable patient information. The studies were conducted in accordance with the local legislation and institutional requirements. The ethics committee/institutional review board also waived the requirement of written informed consent for participation from the participants or the participants’ legal guardians/next of kin because Utilization of publicly accessible data devoid of identifiable patient information. Written informed consent was not obtained from the individual(s) for the publication of any potentially identifiable images or data included in this article because utilization of publicly accessible data.
Author contributions
ZQ: Writing–original draft, Writing–review and editing. XZ: Writing–original draft, Writing–review and editing. SW: Conceptualization, Software, Supervision, Writing–review and editing. YM: Conceptualization, Investigation, Writing–review and editing.
Funding
The authors declare financial support was received for the research, authorship, and/or publication of this article. This work was funded by the Xi’an NO. 3 Hospital Institutional Medical Research Fund (Grant NO. Y2023yxyj09), Clinical Research Award of the First Affiliated Hospital of Xi’an Jiaotong University (Grant NO. XJTU1AF2021CRF-009).
Acknowledgments
We extend our gratitude to Mibiogene and Kohler A for providing the thyroid data, which have advanced the etiological research of malignant thyroid tumors in the world.
Conflict of interest
The authors declare that the research was conducted in the absence of any commercial or financial relationships that could be construed as a potential conflict of interest.
Publisher’s note
All claims expressed in this article are solely those of the authors and do not necessarily represent those of their affiliated organizations, or those of the publisher, the editors and the reviewers. Any product that may be evaluated in this article, or claim that may be made by its manufacturer, is not guaranteed or endorsed by the publisher.
Supplementary material
The Supplementary Material for this article can be found online at: https://www.frontiersin.org/articles/10.3389/fgene.2023.1299930/full#supplementary-material
References
Bastiaanssen, T. F. S., Cowan, C. S. M., Claesson, M. J., Dinan, T. G., and Cryan, J. F. (2019). Making sense of the microbiome in psychiatry. Int. J. Neuropsychopharmacol. 22 (1), 37–52. doi:10.1093/ijnp/pyy067
Bérdy, J. (2005). Bioactive microbial metabolites: a personal view. J. Antibiot. 58 (1), 1–26. doi:10.1038/ja.2005.1
Binda, C., Lopetuso, L. R., Rizzatti, G., Gibiino, G., Cennamo, V., and Gasbarrini, A. (2018). Actinobacteria: a relevant minority for the maintenance of gut homeostasis. Dig. Liver Dis. 50 (5), 421–428. doi:10.1016/j.dld.2018.02.012
Bonnefond, S., and Davies, T. F. (2014). Thyroid cancer—risks and causes. Oncol. Hematol. Rev. (US) 10 (2), 144. doi:10.17925/ohr.2014.10.2.144
Bray, F., Ferlay, J., Soerjomataram, I., Siegel, R. L., Torre, L. A., and Jemal, A. (2018). Global cancer statistics 2018: GLOBOCAN estimates of incidence and mortality worldwide for 36 cancers in 185 countries. CA Cancer J. Clin. 68 (6), 394–424. doi:10.3322/caac.21492
Cao, J., Wang, N., Luo, Y., Ma, C., Chen, Z., Chenzhao, C., et al. (2023). A cause–effect relationship between Graves’ disease and the gut microbiome contributes to the thyroid–gut axis: a bidirectional two-sample Mendelian randomization study. Front. Immunol. 14, 977587. doi:10.3389/fimmu.2023.977587
Chernova, O. A., Chernov, V. M., Mouzykantov, A. A., Baranova, N. B., Edelstein, I. A., and Aminov, R. I. (2021). Antimicrobial drug resistance mechanisms among Mollicutes. Int. J. Antimicrob. Agents 57 (2), 106253. doi:10.1016/j.ijantimicag.2020.106253
Davie, J. R. (2003). Inhibition of histone deacetylase activity by butyrate. J. Nutr. 133 (7), 2485S–2493S. doi:10.1093/jn/133.7.2485S
Davies, N. M., Holmes, M. V., and Davey Smith, G. (2018). Reading Mendelian randomisation studies: a guide, glossary, and checklist for clinicians. BMJ 362, k601. doi:10.1136/bmj.k601
Docimo, G., Cangiano, A., Romano, R. M., Pignatelli, M. F., Offi, C., Paglionico, V. A., et al. (2020). The human microbiota in endocrinology: implications for pathophysiology, treatment, and prognosis in thyroid diseases. Front. Endocrinol. 11, 586529. doi:10.3389/fendo.2020.586529
Ejtahed, H. S., Angoorani, P., Soroush, A. R., Siadat, S. D., Shirzad, N., Hasani-Ranjbar, S., et al. (2020). Our little friends with big roles: alterations of the gut microbiota in thyroid disorders. EMIDDT 20 (3), 344–350. doi:10.2174/1871530319666190930110605
Fang, H., Kang, J., and Zhang, D. (2017). Microbial production of vitamin B12: a review and future perspectives. Microb. Cell Fact. 16 (1), 15. doi:10.1186/s12934-017-0631-y
Feng, J., Zhao, F., Sun, J., Lin, B., Zhao, L., Liu, Y., et al. (2019). Alterations in the gut microbiota and metabolite profiles of thyroid carcinoma patients. Int. J. Cancer 144 (11), 2728–2745. doi:10.1002/ijc.32007
Fernandes, A., Oliveira, A., Carvalho, A. L., Soares, R., and Barata, P. (2023). Faecalibacterium prausnitzii in differentiated thyroid cancer patients treated with radioiodine. Nutrients 15 (12), 2680. doi:10.3390/nu15122680
Fröhlich, E., and Wahl, R. (2019). Microbiota and thyroid interaction in health and disease. Trends Endocrinol. Metabolism 30 (8), 479–490. doi:10.1016/j.tem.2019.05.008
Gill, S. R., Pop, M., DeBoy, R. T., Eckburg, P. B., Turnbaugh, P. J., Samuel, B. S., et al. (2006). Metagenomic analysis of the human distal gut microbiome. Science 312 (5778), 1355–1359. doi:10.1126/science.1124234
Gonçalves, C. F. L., de Freitas, M. L., and Ferreira, A. C. F. (2017). Flavonoids, thyroid iodide uptake and thyroid cancer-A review. Int. J. Mol. Sci. 18 (6), 1247. doi:10.3390/ijms18061247
Gu, Q., and Li, P. (2016). “Biosynthesis of vitamins by probiotic bacteria,” in Probiotics and prebiotics in human nutrition and health. Editors V. Rao, and L. G. Rao (IntechOpen) Available from: https://www.intechopen.com/about-intechopen (Accessed September 4, 2023).
Gu, Y., Yu, Y., Ai, L., Shi, J., Liu, X., Sun, H., et al. (2014). Association of the ATM gene polymorphisms with papillary thyroid cancer. Endocrine 45 (3), 454–461. doi:10.1007/s12020-013-0020-1
Guo, M., Peng, J., Huang, X., Xiao, L., Huang, F., and Zuo, Z. (2021). Gut microbiome features of Chinese patients newly diagnosed with Alzheimer’s disease or mild cognitive impairment. JAD 80 (1), 299–310. doi:10.3233/JAD-201040
Gutiérrez-Calabrés, E., Ortega-Hernández, A., Modrego, J., Gómez-Gordo, R., Caro-Vadillo, A., Rodríguez-Bobada, C., et al. (2020). Gut microbiota profile identifies transition from compensated cardiac hypertrophy to heart failure in hypertensive rats. Hypertension 76 (5), 1545–1554. doi:10.1161/HYPERTENSIONAHA.120.15123
Haddad, R. I., Bischoff, L., Ball, D., Bernet, V., Blomain, E., Busaidy, N. L., et al. (2022). Thyroid carcinoma, version 2.2022, NCCN clinical practice guidelines in oncology. J. Natl. Compr. Canc Netw. 20 (8), 925–951. doi:10.6004/jnccn.2022.0040
Haghikia, A., Jörg, S., Duscha, A., Berg, J., Manzel, A., Waschbisch, A., et al. (2015). Dietary fatty acids directly impact central nervous system autoimmunity via the small intestine. Immunity 43 (4), 817–829. doi:10.1016/j.immuni.2015.09.007
Hattori, S., Nakamura, M., Yamamura, T., Maeda, K., Sawada, T., Mizutani, Y., et al. (2020). The microbiome can predict mucosal healing in small intestine in patients with Crohn’s disease. J. Gastroenterol. 55 (12), 1138–1149. doi:10.1007/s00535-020-01728-1
Huang, J., Ngai, C. H., Deng, Y., Pun, C. N., Lok, V., Zhang, L., et al. (2023). Incidence and mortality of thyroid cancer in 50 countries: a joinpoint regression analysis of global trends. Endocrine 80 (2), 355–365. doi:10.1007/s12020-022-03274-7
Ishaq, H. M., Mohammad, I. S., Guo, H., Shahzad, M., Hou, Y. J., Ma, C., et al. (2017). Molecular estimation of alteration in intestinal microbial composition in Hashimoto’s thyroiditis patients. Biomed. Pharmacother. 95, 865–874. doi:10.1016/j.biopha.2017.08.101
Ishaq, H. M., Mohammad, I. S., Shahzad, M., Ma, C., Raza, M. A., Wu, X., et al. (2018). Molecular alteration analysis of human gut microbial composition in Graves’ disease patients. Int. J. Biol. Sci. 14 (11), 1558–1570. doi:10.7150/ijbs.24151
Ishaq, H. M., Mohammad, I. S., Sher Muhammad, K., Li, H., Abbas, R. Z., Din Sindhu, Z. U., et al. (2021). Gut microbial dysbiosis and its association with esophageal cancer. J. Appl. Biomed. 19 (1), 1–13. doi:10.32725/jab.2021.005
Jiang, W., Lu, G., Gao, D., Lv, Z., and Li, D. (2022). The relationships between the gut microbiota and its metabolites with thyroid diseases. Front. Endocrinol. 13, 943408. doi:10.3389/fendo.2022.943408
Joossens, M., Huys, G., Cnockaert, M., De Preter, V., Verbeke, K., Rutgeerts, P., et al. (2011). Dysbiosis of the faecal microbiota in patients with Crohn’s disease and their unaffected relatives. Gut 60 (5), 631–637. doi:10.1136/gut.2010.223263
Khatami, M. (2009). Inflammation, aging, and cancer: tumoricidal versus tumorigenesis of immunity: a common denominator mapping chronic diseases. Cell Biochem. Biophys. 55 (2), 55–79. doi:10.1007/s12013-009-9059-2
Knezevic, J., Starchl, C., Tmava Berisha, A., and Amrein, K. (2020). Thyroid-Gut-Axis: how does the microbiota influence thyroid function? Nutrients 12 (6), 1769. doi:10.3390/nu12061769
Köhler, A., Chen, B., Gemignani, F., Elisei, R., Romei, C., Figlioli, G., et al. (2013). Genome-wide association study on differentiated thyroid cancer. J. Clin. Endocrinol. Metabolism 98 (10), E1674–E1681. doi:10.1210/jc.2013-1941
Köhling, H. L., Plummer, S. F., Marchesi, J. R., Davidge, K. S., and Ludgate, M. (2017). The microbiota and autoimmunity: their role in thyroid autoimmune diseases. Clin. Immunol. 183, 63–74. doi:10.1016/j.clim.2017.07.001
Lewin, G. R., Carlos, C., Chevrette, M. G., Horn, H. A., McDonald, B. R., Stankey, R. J., et al. (2016). Evolution and ecology of actinobacteria and their bioenergy applications. Annu. Rev. Microbiol. 70 (1), 235–254. doi:10.1146/annurev-micro-102215-095748
Li, C., Li, N., Liu, C., and Yin, S. (2023). Causal association between gut microbiota and intrahepatic cholestasis of pregnancy: mendelian randomization study. BMC Pregnancy Childbirth 23 (1), 568. doi:10.1186/s12884-023-05889-8
Lim, H., Devesa, S. S., Sosa, J. A., Check, D., and Kitahara, C. M. (2017). Trends in thyroid cancer incidence and mortality in the United States, 1974-2013. JAMA 317 (13), 1338–1348. doi:10.1001/jama.2017.2719
Liśkiewicz, P., Kaczmarczyk, M., Misiak, B., Wroński, M., Bąba-Kubiś, A., Skonieczna-Żydecka, K., et al. (2021). Analysis of gut microbiota and intestinal integrity markers of inpatients with major depressive disorder. Prog. Neuro-Psychopharmacology Biol. Psychiatry 106, 110076. doi:10.1016/j.pnpbp.2020.110076
Liu, C. J., Chen, S. Q., Zhang, S. Y., Wang, J. L., Tang, X. D., Yang, K. X., et al. (2021). The comparison of microbial communities in thyroid tissues from thyroid carcinoma patients. J. Microbiol. 59 (11), 988–1001. doi:10.1007/s12275-021-1271-9
Liu, Q., Sun, W., and Zhang, H. (2022). Interaction of gut microbiota with endocrine homeostasis and thyroid cancer. Cancers 14 (11), 2656. doi:10.3390/cancers14112656
Liu, X., Zou, L., Nie, C., Qin, Y., Tong, X., Wang, J., et al. (2023). Mendelian randomization analyses reveal causal relationships between the human microbiome and longevity. Sci. Rep. 13 (1), 5127. doi:10.1038/s41598-023-31115-8
Louis, P., Hold, G. L., and Flint, H. J. (2014). The gut microbiota, bacterial metabolites and colorectal cancer. Nat. Rev. Microbiol. 12 (10), 661–672. doi:10.1038/nrmicro3344
Lu, G., Yu, X., Jiang, W., Luo, Q., Tong, J., Fan, S., et al. (2022). Alterations of gut microbiome and metabolite profiles associated with anabatic lipid dysmetabolism in thyroid cancer. Front. Endocrinol. 13, 893164. doi:10.3389/fendo.2022.893164
Luo, J., Hendryx, M., Manson, J. E., Liang, X., and Margolis, K. L. (2016). Hysterectomy, oophorectomy, and risk of thyroid cancer. J. Clin. Endocrinol. Metab. 101 (10), 3812–3819. doi:10.1210/jc.2016-2011
Maslowski, K. M., and Mackay, C. R. (2011). Diet, gut microbiota and immune responses. Nat. Immunol. 12 (1), 5–9. doi:10.1038/ni0111-5
Morgan, X. C., Tickle, T. L., Sokol, H., Gevers, D., Devaney, K. L., Ward, D. V., et al. (2012). Dysfunction of the intestinal microbiome in inflammatory bowel disease and treatment. Genome Biol. 13 (9), R79. doi:10.1186/gb-2012-13-9-r79
Morotomi, M., Nagai, F., Sakon, H., and Tanaka, R. (2009). Paraprevotella clara gen. nov., sp. nov. and Paraprevotella xylaniphila sp. nov., members of the family “Prevotellaceae” isolated from human faeces. Int. J. Syst. Evol. Microbiol. 59 (8), 1895–1900. doi:10.1099/ijs.0.008169-0
Niccolai, E., Baldi, S., Nannini, G., Gensini, F., Papi, L., Vezzosi, V., et al. (2023). Breast cancer: the first comparative evaluation of oncobiome composition between males and females. Biol. Sex. Differ. 14 (1), 37. doi:10.1186/s13293-023-00523-w
Ning, J., Huang, S. Y., Chen, S. D., Zhang, Y. R., Huang, Y. Y., and Yu, J. T. (2022). Investigating casual associations among gut microbiota, metabolites, and neurodegenerative diseases: a mendelian randomization study. J. Alzheimers Dis. 87 (1), 211–222. doi:10.3233/JAD-215411
Pitt, J. M., Vétizou, M., Daillère, R., Roberti, M. P., Yamazaki, T., Routy, B., et al. (2016). Resistance mechanisms to immune-checkpoint blockade in cancer: tumor-intrinsic and -extrinsic factors. Immunity 44 (6), 1255–1269. doi:10.1016/j.immuni.2016.06.001
Pizzato, M., Li, M., Vignat, J., Laversanne, M., Singh, D., La Vecchia, C., et al. (2022). The epidemiological landscape of thyroid cancer worldwide: GLOBOCAN estimates for incidence and mortality rates in 2020. Lancet Diabetes Endocrinol. 10 (4), 264–272. doi:10.1016/S2213-8587(22)00035-3
Rathod, M., Kelkar, M., Valvi, S., Salve, G., and De, A. (2020). FOXA1 regulation turns benzamide HDACi treatment effect-specific in BC, promoting NIS gene-mediated targeted radioiodine Therapy. Mol. Ther. - Oncolytics 19, 93–104. doi:10.1016/j.omto.2020.08.015
Rautava, S., Luoto, R., Salminen, S., and Isolauri, E. (2012). Microbial contact during pregnancy, intestinal colonization and human disease. Nat. Rev. Gastroenterol. Hepatol. 9 (10), 565–576. doi:10.1038/nrgastro.2012.144
Riaz Rajoka, M. S., Thirumdas, R., Mehwish, H. M., Umair, M., Khurshid, M., Hayat, H. F., et al. (2021). Role of food antioxidants in modulating gut microbial communities: novel understandings in intestinal oxidative stress damage and their impact on host health. Antioxidants 10 (10), 1563. doi:10.3390/antiox10101563
Ridlon, J. M., Kang, D. J., Hylemon, P. B., and Bajaj, J. S. (2014). Bile acids and the gut microbiome. Curr. Opin. Gastroenterology 30 (3), 332–338. doi:10.1097/MOG.0000000000000057
Russo, E., Cinci, L., Di Gloria, L., Baldi, S., D’Ambrosio, M., Nannini, G., et al. (2022). Crohn’s disease recurrence updates: first surgery vs. surgical relapse patients display different profiles of ileal microbiota and systemic microbial-associated inflammatory factors. Front. Immunol. 13, 886468. doi:10.3389/fimmu.2022.886468
Samimi, H., and Haghpanah, V. (2020). Gut microbiome and radioiodine-refractory papillary thyroid carcinoma pathophysiology. Trends Endocrinol. Metabolism 31 (9), 627–630. doi:10.1016/j.tem.2020.03.005
Servin, A. L. (2004). Antagonistic activities of lactobacilli and bifidobacteria against microbial pathogens. FEMS Microbiol. Rev. 28 (4), 405–440. doi:10.1016/j.femsre.2004.01.003
Shen, C. T., Zhang, Y., Liu, Y. M., Yin, S., Zhang, X. Y., Wei, W. J., et al. (2017). A distinct serum metabolic signature of distant metastatic papillary thyroid carcinoma. Clin. Endocrinol. 87 (6), 844–852. doi:10.1111/cen.13437
Singh, A., Ashar, H., Butcher, J. T., and Ranjan, A. (2023). Age-associated changes in the gut microbiome impact efficacy of tumor immunomodulatory treatments. Exp. Gerontol. 181, 112268. doi:10.1016/j.exger.2023.112268
Sokol, H., Pigneur, B., Watterlot, L., Lakhdari, O., Bermúdez-Humarán, L. G., Gratadoux, J. J., et al. (2008). Faecalibacterium prausnitzii is an anti-inflammatory commensal bacterium identified by gut microbiota analysis of Crohn disease patients. Proc. Natl. Acad. Sci. U. S. A. 105 (43), 16731–16736. doi:10.1073/pnas.0804812105
Stebbings, S., Munro, K., Simon, M. A., Tannock, G., Highton, J., Harmsen, H., et al. (2002). Comparison of the faecal microflora of patients with ankylosing spondylitis and controls using molecular methods of analysis. Rheumatology 41 (12), 1395–1401. doi:10.1093/rheumatology/41.12.1395
Vitale, G., Dicitore, A., Barrea, L., Sbardella, E., Razzore, P., Campione, S., et al. (2021). From microbiota toward gastro-enteropancreatic neuroendocrine neoplasms: are we on the highway to hell? Rev. Endocr. Metab. Disord. 22 (3), 511–525. doi:10.1007/s11154-020-09589-y
Von Itzstein, M. S., Khan, S., and Gerber, D. E. (2020). Investigational biomarkers for checkpoint inhibitor immune-related adverse event prediction and diagnosis. Clin. Chem. 66 (6), 779–793. doi:10.1093/clinchem/hvaa081
Wang, H., Naghavi, M., Allen, C., Barber, R. M., Bhutta, Z. A., Carter, A., et al. (2016). Global, regional, and national life expectancy, all-cause mortality, and cause-specific mortality for 249 causes of death, 1980–2015: a systematic analysis for the Global Burden of Disease Study 2015. Lancet 388 (10053), 1459–1544. doi:10.1016/S0140-6736(16)31012-1
Wang, X., and Huycke, M. M. (2007). Extracellular superoxide production by Enterococcus faecalis promotes chromosomal instability in mammalian cells. Gastroenterology 132 (2), 551–561. doi:10.1053/j.gastro.2006.11.040
WHO (2020). IARC. Available from: https://gco.iarc.fr/today/data/factsheets/populations/620-portugal-fact-sheets.pdf (Accessed September 4, 2023).
Wong, J. M. W., De Souza, R., Kendall, C. W. C., Emam, A., and Jenkins, D. J. A. (2006). Colonic health: fermentation and short chain fatty acids. J. Clin. Gastroenterology 40 (3), 235–243. doi:10.1097/00004836-200603000-00015
Wu, M., Li, P., Li, J., An, Y., Wang, M., and Zhong, G. (2020). The differences between luminal microbiota and mucosal microbiota in mice. J. Microbiol. Biotechnol. 30 (2), 287–295. doi:10.4014/jmb.1908.08037
Wu, S., Powell, J., Mathioudakis, N., Kane, S., Fernandez, E., and Sears, C. L. (2004). Bacteroides fragilis enterotoxin induces intestinal epithelial cell secretion of interleukin-8 through mitogen-activated protein kinases and a tyrosine kinase-regulated nuclear factor-kappaB pathway. Infect. Immun. 72 (10), 5832–5839. doi:10.1128/IAI.72.10.5832-5839.2004
Wu, S., Rhee, K. J., Albesiano, E., Rabizadeh, S., Wu, X., Yen, H. R., et al. (2009). A human colonic commensal promotes colon tumorigenesis via activation of T helper type 17 T cell responses. Nat. Med. 15 (9), 1016–1022. doi:10.1038/nm.2015
Yao, C. Z., Zhang, M., Zeng, Y. K., Zhang, Y. Y., Wu, X., Xiong, W. J., et al. (2023). Analysis and prediction of thyroid cancer morbidity and mortality trends in China. Zhonghua Liu Xing Bing Xue Za Zhi 44 (6), 917–923. doi:10.3760/cma.j.cn112338-20221010-00869
Young, V. B., and Schmidt, T. M. (2004). Antibiotic-associated diarrhea accompanied by large-scale alterations in the composition of the fecal microbiota. J. Clin. Microbiol. 42 (3), 1203–1206. doi:10.1128/jcm.42.3.1203-1206.2004
Yu, A. I., Zhao, L., Eaton, K. A., Ho, S., Chen, J., Poe, S., et al. (2020). Gut microbiota modulate CD8 T cell responses to influence colitis-associated tumorigenesis. Cell Rep. 31 (1), 107471. doi:10.1016/j.celrep.2020.03.035
Zhang, J., Zhang, F., Zhao, C., Xu, Q., Liang, C., Yang, Y., et al. (2019). Dysbiosis of the gut microbiome is associated with thyroid cancer and thyroid nodules and correlated with clinical index of thyroid function. Endocrine 64 (3), 564–574. doi:10.1007/s12020-018-1831-x
Zhao, F., Feng, J., Li, J., Zhao, L., Liu, Y., Chen, H., et al. (2018). Alterations of the gut microbiota in Hashimoto’s thyroiditis patients. Thyroid 28 (2), 175–186. doi:10.1089/thy.2017.0395
Zhou, L., Zhang, M., Wang, Y., Dorfman, R. G., Liu, H., Yu, T., et al. (2018). Faecalibacterium prausnitzii produces butyrate to maintain Th17/treg balance and to ameliorate colorectal colitis by inhibiting histone deacetylase 1. Inflamm. Bowel Dis. 24 (9), 1926–1940. doi:10.1093/ibd/izy182
Keywords: gut microbiota, differentiated thyroid carcinoma, heterogeneity assessment, Mendelian randomization study, causal relationship
Citation: Quan Z, Zhang X, Wang S and Meng Y (2023) Causal analysis of the gut microbiota in differentiated thyroid carcinoma: a two-sample Mendelian randomization study. Front. Genet. 14:1299930. doi: 10.3389/fgene.2023.1299930
Received: 23 September 2023; Accepted: 04 December 2023;
Published: 13 December 2023.
Edited by:
Eleonore Fröhlich, Medical University of Graz, AustriaCopyright © 2023 Quan, Zhang, Wang and Meng. This is an open-access article distributed under the terms of the Creative Commons Attribution License (CC BY). The use, distribution or reproduction in other forums is permitted, provided the original author(s) and the copyright owner(s) are credited and that the original publication in this journal is cited, in accordance with accepted academic practice. No use, distribution or reproduction is permitted which does not comply with these terms.
*Correspondence: Yong Meng, bWVuZ3lvbmdAbnd1LmVkdS5jbg==
†These authors have contributed equally to this work and share first authorship