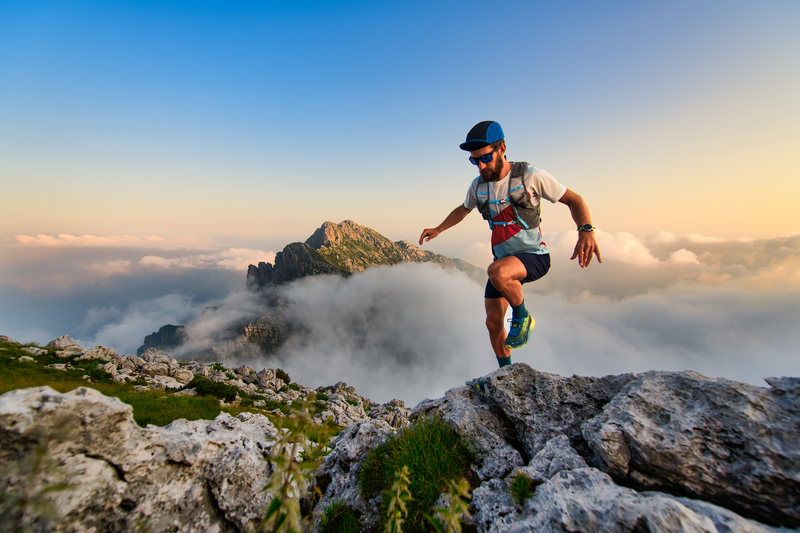
95% of researchers rate our articles as excellent or good
Learn more about the work of our research integrity team to safeguard the quality of each article we publish.
Find out more
ORIGINAL RESEARCH article
Front. Genet. , 19 January 2024
Sec. Genomics of Plants and the Phytoecosystem
Volume 14 - 2023 | https://doi.org/10.3389/fgene.2023.1292009
Introduction: Chickpea is a legume crop that thrives in regions with semi-arid or temperate climates. Its seeds are an excellent source of proteins, carbohydrates, and minerals, especially high-quality proteins. Chickpea cultivation faces several challenges including Fusarium wilt (FW), a major fungal disease that significantly reduces productivity.
Methods: In this study, a Genome-wide Association Analysis (GWAS) was conducted to identify multiple genomic loci associated with FW resistance in chickpea. We conducted a comprehensive evaluation of 180 chickpea genotypes for FW resistance across three distinct locations (Ethiopia, Tunisia, and Lebanon) during the 2-year span from 2015 to 2016. Disease infection measurements were recorded, and the wilt incidence of each genotype was calculated. We employed a set of 11,979 single nucleotide polymorphisms (SNPs) markers distributed across the entire chickpea genome for SNP genotyping. Population structure analysis was conducted to determine the genetic structure of the genotypes.
Results and Discussion: The population structure unveiled that the analyzed chickpea germplasm could be categorized into four sub-populations. Notably, these sub-populations displayed diverse geographic origins. The GWAS identified 11 SNPs associated with FW resistance, dispersed across the genome. Certain SNPs were consistent across trials, while others were specific to particular environments. Chromosome CA2 harbored five SNP markers, CA5 featured two, and CA4, CA6, CA7, and CA8 each had one representative marker. Four SNPs demonstrated an association with FW resistance, consistently observed across a minimum of three distinct environments. These SNPs included SNP5826041, SNP5825086, SNP11063413, SNP5825195, which located in CaFeSOD, CaS13like, CaNTAQ1, and CaAARS genes, respectively. Further investigations were conducted to gain insights into the functions of these genes and their role in FW resistance. This progress holds promise for reducing the negative impact of the disease on chickpea production.
Chickpea (Cicer arietinum L.) is a highly significant grain legume that holds global prominence. It is widely cultivated and consumed, particularly in developing countries, where it serves as a crucial food source (Jukanti et al., 2012; Ullah et al., 2020). According to the United Nations Food and Agriculture Organization (FAO), chickpea is cultivated in over 50 countries, with India being the leading producer, contributing to over 70% of the global production. Pakistan and Iran, the next two largest producers, account for 10% and 5% of the total production, respectively. Other notable producing nations such as Turkey and Australia contribute 4% and 3%, respectively (Muehlbauer and Sarker, 2017). Chickpea is grown in a variety of ecological conditions, exposing it to a variety of biotic and abiotic stresses. Among the biotic stresses, Fusarium wilt (FW) stands out as a significant constraint to chickpea production (Garg et al., 2018). FW is a disease caused by the fungus Fusarium oxysporum f. sp. ciceri, which targets chickpea plants (Schumann and Sands, 2006). FW is a significant soil-borne disease, particularly prevalent in the Mediterranean, South Asia, and East Africa regions, where it induces root and stem rot, ultimately impacting chickpea production (Alloosh et al., 2019). In warm and dry regions, FW stands as the most destructive root disease, resulting in yield losses ranging from 10% to 40% and, in some cases, complete crop failure (Garg et al., 2018). Symptoms of FW include vascular clogging, stunted growth, yellowing and wilting of leaves, and eventual plant death (Okungbowa and Shittu, 2012; Leitão et al., 2020). The fungus primarily infects plants through their roots, but it can also spread through contaminated soil or seeds (Singh and Sharma, 1997; Fan et al., 2022).
F. oxysporum is a root-inhabiting (soil invader) fungus that reproduces asexually (Jiménez-Díaz et al., 2015). It has the ability to persist in soil for extended periods and produces toxins that impair plant function, including water and nutrient transport (Singh and Singh, 2012). The fungus invades the vascular system of chickpea plants, leading to wilting and eventual death (Singh and Vyas, 2021). Chemicals are widely used to manage FW in chickpeas; however, their usage is neither environmentally friendly nor cost-effective. Additionally, improper chemical use can harm beneficial soil microorganisms (Maitlo et al., 2019). Hence, the most effective management approach for FW in chickpeas involves integrated disease management strategies, and the development of chickpea resistant genotypes (Singh et al., 2015; Soltanzadeh et al., 2016). Modern molecular techniques such as SNP genotyping can be used to achieve this goal (Uffelmann et al., 2021).
Genome-wide association study (GWAS) have gained increasing importance in plant science, enabling researchers to identify and comprehend the genetic basis of various traits (Alseekh et al., 2021; Tibbs Cortes et al., 2021). One of the main advantages of GWAS is its applicability to a wide range important traits, including disease resistance. For instance, GWAS has successfully identified genetic markers associated with resistance to diseases. In maize affected by Fusarium ear rot, many differentially expressed genes enriched in pathways related to plant immune responses, including plant hormone signal transduction, phenylpropanoid biosynthesis, and cytochrome P450 metabolism, were detected (Yao et al., 2020). And late blight in potatoes: 18 genes associated with blight resistance were identified, among them, PGSC0003DMG400028682 encodes chitinase 1, which is related to immune response, PGSC0003DMG400036902 encodes a glycine-rich protein involved in the defence ability of the cell wall and PGSC0003DMG400031878 encode NBS-LRR resistance proteins, which are directly related to potato late blight resistance (Wang et al., 2020). By identifying genetic markers associated with disease resistance, breeders can develop cultivars that are resistant to specific diseases, thus reducing their impact on crop yields and productivity (Bartoli and Roux, 2017). GWAS can also identify specific genetic markers associated with other important traits such as yield, quality, and stress tolerance (Tibbs Cortes et al., 2021). The application of GWAS in chickpea breeding holds great potential for significantly improving crop productivity and resistance to diseases such as F. oxysporum infection (Sharma et al., 2016; Li et al., 2018; Varshney et al., 2019). By identifying and selecting for infection-tolerant genes and genotypes (Alseekh et al., 2021; Tibbs Cortes et al., 2021), breeders can develop cultivars with enhanced resistance to F. oxysporum, thereby mitigating the impact of this disease on chickpea production. This is particularly crucial in developing countries where chickpeas play a vital role in food security and livelihoods (Karaca et al., 2019).
SNP markers which are highly significant, and remain underutilized in molecular breeding programs (Tyagi et al., 2019; Fayaz et al., 2021). SNP markers are the preferred choice due to their wide genome coverage, co-dominant inheritance, chromosome-specific location, cost-effectiveness, and rapid tracking capability (Farahani et al., 2019). The present study was designed to study the population structure of a set of international chickpea germplasms. These germplasms were genotyped using SNP technology to identify potential genomic loci and genes associated with FW resistance in chickpea using GWAS. The identified SNP markers could serve as a basis for marker assisted selection and provide insights into the genetic mechanisms underlying chickpea resistance.
One hundred and eighty chickpea genotypes were selected from the genetic resource section (GRS) of ICARDA based on the passport data using the focused identification of germplasm strategy (FIGS) protocol (Ahmed et al., 2021) (Supplementary Table S1). The experiment was conducted with an Alpha Lattice design in three locations: Terbol-Lebanon and Beja-Tunisia during the 2015 and 2016 seasons, and only in the 2016 season in Debre Zeit-Ethiopia. The susceptible chickpea genotype (ILC482) (Arfaoui et al., 2006) was planted every ten rows in the experimental setup. The genotypes under study were sown in rows measuring 250 cm in length, with 45 cm between each row. Two replications were maintained for all locations. Disease infection measurements were recorded for each genotype at 40 and 75 days after cultivation. The wilted plants of test entries were observed and recorded at various stages, specifically when the susceptible check had reached its peak susceptibility. The second stage data on wilted plants were recorded at the beginning of physiological maturity. Plots were scored (% of wilted or dead plants per plot). For data analysis, the maximum score was utilized. The wilt incidence of each test entry was calculated using the following formula:
The resistance and susceptibility levels of each test line were determined using a 1-9 rating scale (Iqbal et al., 1993). According to this scale, a rating of 1 indicates high resistance (0%–10% of plants wilted), 3- resistance (11%–20% plant mortality), 5- moderate resistance (21%–30% mortality), 7- susceptibility (31%–50% mortality), and 9- high susceptibility (more than 50% mortality). The analysis of variance was performed using Genstat software, a widely employed statistical package for data analysis and statistical modeling. The means generated across replicates were subsequently utilized in the GWAS analysis.
DNA was extracted from the young leaves of 180 chickpea genotypes aged 4–6 weeks using the cetyltrimethylammonium bromide (CTAB) method. In summary, fresh seedling leaf was dried and ground into a fine powder. Following that, we added the powder in a 2 mL Eppendorf tube with 1 mL pre-warmed 2X CTAB buffer 2% CTAB, 0.1 M Tris HCl (pH 8.0), 1.4 M NaCl, 20 mM Ethylenediaminetetraacetic acid (EDTA). The suspension was mixed and incubated for 30 min at 65°C. After cooling for 5 min at room temperature (RT), 1 mL chloroform-isoamyl alcohol (24:1) was added to the tube and gently mixed for 10 min by shaking. The suspension was centrifuged for 20 min at RT at 4,500 rpm (Beckmann YA-12), and the supernatant was transferred to a new tube. The DNA was precipitated in 1 mL of cold isopropanol. The DNA was placed in a micro-centrifuge tube and washed twice for 20 min with a washing buffer (70% ethanol and 200 mM sodium acetate). After about 10–20 min of air drying, the DNA was dissolved in 200 μ of 1X TE buffer 10 mM Tris HCl, pH 8.0, 1 mM EDTA.
The extracted DNA samples were sent to Triticarte Pty. Ltd. Australia (http://www.triticarte.com.au) for SNP marker genotyping. A total of 11,979 SNP markers (Supplementary Tables S2, S3) were obtained. The SNP markers were filtered based on the following call rates greater than 80%, and minor allele frequency (MAF) ≥ 5%. After filtration 1,715 SNPs markers were accepted for data analysis.
We conducted a population structure analysis to identify clusters and gain insights into the genetic makeup of 180 chickpea genotypes. The LEA R package (Gain and François, 2021) was used for population structure. The analytical process commenced by utilizing the R function snmf to evaluate the genetic structure of the population. This involved a range of potential genetic groupings (K) from 1 to 10, each repeated ten times for robustness with 10,000 iterations. The cross-entropy criterion was employed to identify the optimal number of population groups that best elucidated the genotypic data (Frichot and François, 2015; François, 2016). The results of this analysis were visualized using a barplot generated with the R ggplot2 package, illustrating the relationship with the country of origin for the genotypes.
GWAS was carried out with the aim of identifying genetic markers linked to FW resistance. The phenotypic data encompassing the levels of resistance and susceptibility to FW, alongside the SNP genotyping data, were subjected to analysis using the vcf2gwas software (Vogt et al., 2022). The GWAS was conducted through the application of the mixed linear model (MLM), integrating both population structure (Q) and polygenic (K) factors, constituting the Q + K model (Wang et al., 2016). This modeling approach has gained widespread acceptance in GWAS due to its efficacy in mitigating the influence of numerous minor genetic effects and rectifying biases arising from population stratification (Wen et al., 2018). To enhance caution in our analysis, principal component analysis (PCA) was employed as a covariate. This step was taken to more thoroughly address potential confounding factors that could influence the outcomes of our study. Furthermore, an assessment using the Q-Q plot was performed, leveraging its capability to visually juxtapose the empirical data distribution against a theoretical probability distribution (Das and Resnick, 2008). To discern meaningful associations, we implemented two levels of thresholds. The initial criterion was set at a False Discovery Rate (FDR) of less than 0.1. Additionally, we adopted a p-value threshold of -log10-pvalue ≥2.3 (equivalent to p-value ≤0.005). Notably, we considered associations as significant only if they consistently manifested across a minimum of two distinct environments. This dual-threshold approach ensures a stringent and reliable identification of substantial associations in our study. We employed boxplot analysis to show the impact of each allele value on the expression of the studied phenotype. To uncover the genetic basis of FW resistance, we used the NCBI BLASTN tool to map marker sequences linked to FW-associated SNPs onto the chickpea genome, aiming to identify potential resistance genes (Varshney et al., 2013).
The analysis of population genetic structure is commonly used to demonstrate the genetic similarity between populations categorized based on their geographic location (Figure 1). The structural analysis determined the optimal number of clusters as K = 4. This analysis revealed that the analyzed chickpea germplasm can be classified into four populations (Figure 1). The first population (Q1) consists of Cyprus, Algeria, Spain, Iraq, and Lebanon. The second group (Q2) includes Azerbaijan, Greece, Pakistan, and Slovakia, while the third (Q3) is associated with Afghanistan. The fourth population (Q4) was identified in Afghanistan, Iran, and Uzbekistan. The first group was found to be the largest population spread across different geographical areas. Additionally, there was some genetic overlap between populations I, III, and IV in Afghanistan, indicating a mixture of genetic origins from various geographic regions.
FIGURE 1. Population structure plot of the 180 genotypes. The structural analysis revealed that the optimal number of clusters, denoted as K, is 4. In the plot, each vertical bar represents an individual genotype, and the colors indicate the country of origin for each genotype.
Initially, we employed 11,979 SNP markers for genotyping. However, only 1,715 markers met the quality control parameters for MAF and missing rate. The GWAS analysis utilized SNP genotyping data for the 180 chickpea genotypes, along with their phenotypic data replicated across three locations and 2 years. The GWAS revealed numerous SNPs associated with FW. Among these, certain SNPs were consistently observed across multiple regions, while others were specific to particular environments (Figure 2; Table 1). Three significant SNP markers associated with FW resistance, namely, SNP5825627, SNP8776047, and SNP5825086, distributed across the chickpea genome, with an FDR ≥0.1. SNP5825086, located on chromosome CA8, was detected in multiple environments Lebanon-2015 (p-value of 1.0 × 10−5) and Lebanon-2016 (p-value of 9.0 × 10−5), with an FDR ≥0.07. SNP5825627 on chromosome CA4 was exclusively identified in Ethiopia-2016 (p-value of 4.0 × 10−5) with an FDR of 0.05. Similarly, SNP8776047 on chromosome CA6 was observed in Lebanon-2016 (p-value of 1.0 × 10−4) with an FDR of 0.07 (Figure 2).
FIGURE 2. Manhattan plot and Q-Q plots depicting GWAS results for FW resistance across the chickpea genome. Red dots along the dashed red line signify significance with False Discovery Rate (FDR) ≤ 0.1. Blue dots along the dashed blue line represent SNPs associated with FW-associated resistance ≤ 0.005 (-log10-pvalue ≥ 2.3).
Moreover, we identified 11 SNPs markers significantly associated with FW resistance, each with a p-value of ≤ 0.005. These markers were dispersed throughout the genome and across diverse environments. Notably, chromosome CA2 harbored five SNP markers (SNP5825384, SNP10268227, SNP10264793, SNP10269791, and SNP10264807), while chromosome CA5 featured two (SNP5825195 and SNP5825194). Additionally, chromosomes CA4, CA6, CA7, and CA8 each exhibited a single representative SNP marker: SNP11063413, SNP8776047, SNP5826041, and SNP5825086, respectively. Among these SNPs, some exhibited shared occurrences across multiple environments. Specifically, SNP5826041 on chromosome CA7 was consistently detected in various environments (Ethiopia-2016, Lebanon-2015, and Lebanon-2016), with a p-value
FIGURE 3. Boxplot diagrams illustrate the allelic effects of specific SNPs. In each box plot, the box represents the lower quartile, median, and upper quartile values, while black dots indicate genotypes.
TABLE 2. SNP marker sequence, genomic information and genes associated with fusarium resistance in chickpea.
FIGURE 4. Spatial depiction of FW resistance-associated SNPs within the chickpea genome, illustrating their positions in relation to the gene structure. Introns are represented by green lines, while exons are depicted as green boxes. The depiction represents genes adjacent to SNPs (A) 10264793, (B) 8776047, (C) 5826041, and (D) 10264807, which are associated with chickpea resistance to FW in the current study.
Plants are permanently exposed to biotic stress, which induces changes in plant metabolism, resulting in physiological damage that ultimately reduces their productivity (Gimenez et al., 2018). Among these stresses, FW, which is a globally recognized menace, wielding substantial deleterious impacts on chickpea crop yields and quality. The genetic machinery governing FW resistance in chickpea is intricately woven, manifesting considerable genetic diversity. Notably, investigations into the genetic underpinnings, inheritance patterns, and the molecular markers underpinning FW resistance in chickpea have been conspicuously scarce (Li et al., 2021). The advent of GWAS has ushered in an era of potent tools for dissecting complex traits and genetic variations, particularly within the realm of SNP loci. These methodologies have been adeptly applied to diverse crop species in recent years, with the general linear model and mixed linear model firmly ensconced as common GWAS methodologies within the plant sciences (Wang et al., 2016; Chu et al., 2020; Thurow et al., 2020; Yuan et al., 2020; Ahmed et al., 2021; Li et al., 2021).
In this study, we assessed FW resistance across 180 chickpea genotypes in Ethiopia, Tunisia, and Lebanon during the 2015–2016 period. Utilizing SNP genotyping, we explored the genetic diversity and population structure of these accessions. Additionally, we conducted a thorough screening of the chickpea genome through GWAS to pinpoint genetic variations linked to FW resistance. The GWAS analysis revealed 11 robust SNPs with statistically significant associations to FW resistance, depicted in Figure 2. The identified 11 SNPs associated with FW resistance were distributed across the genome. Some SNPs were consistent across trials, while others were specific to certain environments. These markers were located on chromosomes CA2, CA5, CA4, CA6, CA7, and CA8. Remarkably, four SNPs consistently demonstrated associations with Fusarium wilt (FW) resistance in at least three environments—specifically, SNP5826041, SNP5825086, SNP11063413, and SNP5825195. In contrast, the remaining seven SNPs showed associations with FW in only two trials.
Guided by the reference genome sequence of chickpea, we successfully identified the genomic locations of significant SNPs in the chickpea genome. Several of these genes have been previously reported to play a role in the plant’s defense response and are associated with resistance to specific diseases or viruses. One such gene is Endo-1,3-β-glucanase (official name; glucan endo-1,3-β-d-glucosidase), which belongs to glycoside hydrolase family 17 and acts synergistically with chitinases to inhibit the growth of fungal pathogens by hydrolyzing the 1,3-β-glucosyl linkages (1,3-β-glucan and 1,3; 1,6-β-glucan) (Higa-Nishiyama et al., 2006; Shi et al., 2020). In plants, especially monocots, defense-associated endo-1,3-beta-glucanases (PR-2 proteins) are grouped into the highly divergent glucanase subfamily A, which also includes glucanases whose primary function is involved in plant defense, as well as in fundamental physiological processes such as germination, microspore generation, and embryogenesis (Higa-Nishiyama et al., 2006; Meirinho et al., 2010). Plants possess the remarkable ability to detect the presence of microbial organisms by utilizing components of their innate immune system. This system plays a crucial role in triggering an appropriate defense response upon pathogen invasion (Saucet et al., 2021). Recognition of pathogen effectors is a fundamental aspect of plant immunity, and it is mediated by intracellular NB-LRR immune receptors encoded by Resistance (R) genes (Saucet et al., 2015). Among these R genes, RPS4B stands out as a disease resistance R protein that specifically interacts with the Pseudomonas avirulence effector (AvrRps4) (Figure 4D; Table 2). The interaction between RPS4B and AvrRps4 triggers a hypersensitive response (Zhao et al., 2019). Moreover, RPS4B is capable of independently activating defense genes, thereby providing a level of resistance that is adequate in response to AvrRps4 (Saucet et al., 2015).
Abiotic and biotic stresses pose significant challenges to plant growth and productivity. When faced with stressors like pathogen attacks, plants activate a comprehensive array of genes dedicated to defensive molecular mechanisms. One crucial response is the oxidative burst, characterized by the rapid production of reactive oxygen species (ROS) (Faize et al., 2012). Plants possess robust defense mechanisms against ROS, including strategies to both limit their formation and facilitate their removal. At the forefront of this defense are superoxide dismutases (SODs), which constitute the initial line of defense against ROS (Alscher et al., 2002). These metal-containing enzymes catalyze the dismutation of superoxide radicals into oxygen and hydrogen peroxide, playing a pivotal role in defending against toxic-reduced oxygen species, generated as byproducts of numerous biological oxidations (Bowler et al., 1994). Furthermore, SODs play various roles in regulating H2O2 concentrations. This regulation is central to the expression of disease resistance in multiple pathosystems and plays a crucial role in signaling pathways that reinforce defense-related genes (Li et al., 2019). Additionally, H2O2 has been identified as a key regulator in a wide array of other physiological processes, including photosynthesis, senescence, and overall plant growth and development (Faize et al., 2012). Tryptophan synthase beta chain 2 (trpB2) is a component of the enzyme tryptophan synthase. It is an essential enzyme found in bacteria, yeasts, molds, and plants, and is involved in the biosynthesis of the amino acid tryptophan (Busch et al., 2014; Schattenkerk, 2017). Tryptophan is an essential amino acid required by animals for the production of proteins and other compounds, such as neurohormones, serotonin, and the vitamin nicotinic acid. In plants, it serves as a precursor to auxin and a variety of secondary metabolites, such as camalexin and glucosinolates, which protect plants from fungal, bacterial, and herbivore attacks (Miao et al., 2019; Liu et al., 2022).
In comparison, Channale et al. investigated the genetic basis of P. thornei resistance in chickpeas through GWAS. They analyzed a dataset comprising 492,849 SNPs and 278 chickpea accessions. Their study identified 24 significant QTNs associated with P. thornei resistance, distributed across all chickpea chromosomes. Six candidate genes, including receptor-linked kinases (RLKs) and GDSL-like Lipase/Acylhydrolase, were identified in close proximity to these QTNs. These candidate genes have been previously linked to plant-pathogen interactions (Channale et al., 2023). Similarly, Agarwal et al. focused on the genetic basis of Pythium ultimum resistance in chickpeas using GWAS. They analyzed a diverse chickpea diversity panel of 184 accessions and performed an analysis of 302,902 SNPs. Their study identified a major quantitative trait locus (QTL) and 14 candidate genes associated with resistance to Pythium ultimum. The candidate genes, including Ca_19996, Ca_09957, and Ca_22742, represent significant advancements in understanding disease resistance in chickpeas (Agarwal et al., 2022). Furthermore, Raman et al. conducted a study to evaluate the resistance to Ascochyta blight (AB) in chickpea using GWAS. They analyzed a genome-wide association set of 251 genotypes and identified twenty-six genomic regions associated with AB resistance on chromosomes Ca1, Ca4, and Ca6. Their study employed various GWAS models, including single and multi-locus mixed models and haplotyping trend regression, to identify these genomic regions (Raman et al., 2022). Overall, these studies, along with our own, highlight the effectiveness of GWAS in identifying genetic markers and candidate genes associated with various disease resistances in chickpeas. The identified genes, such as CaFeSOD, CaS13like, CaNTAQ1, CaAARS, RLKs, GDSL-like Lipase/Acylhydrolase, and others, provide valuable insights into the molecular mechanisms underlying disease resistance and can contribute to the development of breeding strategies for improving chickpea cultivars.
Our study on the genetic basis of FW resistance in chickpeas has certain limitations that can be addressed to further enhance our understanding of this trait. Firstly, the inclusion of a larger and more diverse panel of chickpea genotypes would provide a broader representation of the genetic variations associated with FW resistance. Expanding the number of genotypes in future studies could uncover additional markers and genes linked to resistance. Additionally, conducting experiments across a wider range of locations and seasons would help account for environmental variations and increase the robustness of the findings. While our study focused on SNP markers, integrating other marker types and exploring structural variations could provide a more comprehensive understanding of the genetic architecture underlying FW resistance. Furthermore, functional validation of candidate genes identified in our study would strengthen their association with resistance. Gene expression analysis and functional genomics approaches can provide insights into the molecular mechanisms and pathways involved. Despite these limitations, our study contributes valuable insights into FW resistance and lays the foundation for further research and breeding efforts. By addressing these limitations, future studies can build upon our findings, leading to improved FW resistance in chickpeas and benefiting crop production and food security.
The datasets presented in this study can be found in online repositories. The names of the repository/repositories and accession number(s) can be found in the article/Supplementary Material.
AA: Writing–original draft, Formal Analysis, Methodology, Visualization, Software. KM: Formal Analysis, Methodology, Software, Visualization, Writing–original draft. TI: Conceptualization, Formal Analysis, Investigation, Methodology, Resources, Software, Writing–original draft, Writing–review and editing. MAE-M: Conceptualization, Methodology, Supervision, Validation, Writing–original draft, Writing–review and editing. ST: Conceptualization, Investigation, Methodology, Resources, Writing–original draft, Writing–review and editing. AH: Conceptualization, Funding acquisition, Methodology, Resources, Supervision, Writing–review and editing.
The author(s) declare financial support was received for the research, authorship, and/or publication of this article. This work was funded by the Grain Legume and Dryland Cereals (GLDC) and Grain Research and Development Cooperation (GRDC).
The authors declare that the research was conducted in the absence of any commercial or financial relationships that could be construed as a potential conflict of interest.
All claims expressed in this article are solely those of the authors and do not necessarily represent those of their affiliated organizations, or those of the publisher, the editors and the reviewers. Any product that may be evaluated in this article, or claim that may be made by its manufacturer, is not guaranteed or endorsed by the publisher.
The Supplementary Material for this article can be found online at: https://www.frontiersin.org/articles/10.3389/fgene.2023.1292009/full#supplementary-material
SUPPLEMENTARY TABLE S1 | The passport data for the studied 180 chickpea genotypes, including their country of origin and ICARDA Genbank ID.
SUPPLEMENTARY TABLE S2 | The SNP genotypic data of the studied 180 chickpea genotypes.
SUPPLEMENTARY TABLE S3 | The phenotypic data on FW infection from the field experiment conducted in three locations.
Agarwal, C., Chen, W., Varshney, R. K., and Vandemark, G. (2022). Linkage qtl mapping and genome-wide association study on resistance in chickpea to pythium ultimum. Front. Genet. 13, 945787. doi:10.3389/fgene.2022.945787
Ahmed, S. M., Alsamman, A. M., Jighly, A., Mubarak, M. H., Al-Shamaa, K., Istanbuli, T., et al. (2021). Genome-wide association analysis of chickpea germplasms differing for salinity tolerance based on dartseq markers. Plos one 16, e0260709. doi:10.1371/journal.pone.0260709
Alloosh, M., Hamwieh, A., Ahmed, S., and Alkai, B. (2019). Genetic diversity of fusarium oxysporum f. sp. ciceris isolates affecting chickpea in Syria. Crop Prot. 124, 104863. doi:10.1016/j.cropro.2019.104863
Alscher, R. G., Erturk, N., and Heath, L. S. (2002). Role of superoxide dismutases (sods) in controlling oxidative stress in plants. J. Exp. Bot. 53, 1331–1341. doi:10.1093/jexbot/53.372.1331
Alseekh, S., Kostova, D., Bulut, M., and Fernie, A. R. (2021). Genome-wide association studies: assessing trait characteristics in model and crop plants. Cell. Mol. Life Sci. 78, 5743–5754. doi:10.1007/s00018-021-03868-w
Arfaoui, A., Sifi, B., Boudabous, A., Hadrami, I. E., and Cherif, M. (2006). Identification of rhizobium isolates possessing antagonistic activity against fusarium oxysporum f. sp. ciceris, the causal agent of fusarium wilt of chickpea. J. Plant Pathology, 67–75.
Bartoli, C., and Roux, F. (2017). Genome-wide association studies in plant pathosystems: toward an ecological genomics approach. Front. plant Sci. 8, 763. doi:10.3389/fpls.2017.00763
Bowler, C., Van Camp, W., Van Montagu, M., Inzé, D., and Asada, K. (1994). Superoxide dismutase in plants. Crit. Rev. Plant Sci. 13, 199–218. doi:10.1080/07352689409701914
Busch, F., Rajendran, C., Mayans, O., Loffler, P., Merkl, R., and Sterner, R. (2014). Trpb2 enzymes are o-phospho-l-serine dependent tryptophan synthases. Biochemistry 53, 6078–6083. doi:10.1021/bi500977y
Channale, S., Thompson, J. P., Varshney, R. K., Thudi, M., and Zwart, R. S. (2023). Multi-locus genome-wide association study of chickpea reference set identifies genetic determinants of pratylenchus thornei resistance. Front. plant Sci. 14, 1139574. doi:10.3389/fpls.2023.1139574
Chu, B. B., Keys, K. L., German, C. A., Zhou, H., Zhou, J. J., Sobel, E. M., et al. (2020). Iterative hard thresholding in genome-wide association studies: generalized linear models, prior weights, and double sparsity. GigaScience 9, giaa044. doi:10.1093/gigascience/giaa044
Das, B., and Resnick, S. I. (2008). Qq plots, random sets and data from a heavy tailed distribution. Stoch. Models 24, 103–132. doi:10.1080/15326340701828308
Faize, M., Burgos, L., Faize, L., Petri, C., Barba-Espin, G., Díaz-Vivancos, P., et al. (2012). Modulation of tobacco bacterial disease resistance using cytosolic ascorbate peroxidase and cu, zn-superoxide dismutase. Plant Pathol. 61, 858–866. doi:10.1111/j.1365-3059.2011.02570.x
Fan, P., Lai, C., Yang, J., Hong, S., Yang, Y., Wang, Q., et al. (2022). Crop rotation suppresses soil-borne fusarium wilt of banana and alters microbial communities. Archives Agron. Soil Sci. 68, 447–459. doi:10.1080/03650340.2020.1839058
Farahani, S., Maleki, M., Mehrabi, R., Kanouni, H., Scheben, A., Batley, J., et al. (2019). Whole genome diversity, population structure, and linkage disequilibrium analysis of chickpea (cicer arietinum l.) genotypes using genome-wide dartseq-based snp markers. Genes 10, 676. doi:10.3390/genes10090676
Fayaz, H., Mir, A. H., Tyagi, S., Wani, A. A., Jan, N., Yasin, M., et al. (2021). Assessment of molecular genetic diversity of 384 chickpea genotypes and development of core set of 192 genotypes for chickpea improvement programs. Genet. Resour. Crop Evol. 69, 1193–1205. doi:10.1007/s10722-021-01296-0
François, O. (2016). Running structure-like population genetic analyses with r. R. Tutor Popul. Genet. U Grenoble-Alpes 1–9.
Frichot, E., and François, O. (2015). Lea: an r package for landscape and ecological association studies. Methods Ecol. Evol. 6, 925–929. doi:10.1111/2041-210x.12382
Gain, C., and François, O. (2021). Lea 3: factor models in population genetics and ecological genomics with r. Mol. Ecol. Resour. 21, 2738–2748. doi:10.1111/1755-0998.13366
Garg, T., Mallikarjuna, B., Thudi, M., Samineni, S., Singh, S., Sandhu, J., et al. (2018). Identification of qtls for resistance to fusarium wilt and ascochyta blight in a recombinant inbred population of chickpea (cicer arietinum l.). Euphytica 214, 45–11. doi:10.1007/s10681-018-2125-3
Gimenez, E., Salinas, M., and Manzano-Agugliaro, F. (2018). Worldwide research on plant defense against biotic stresses as improvement for sustainable agriculture. Sustainability 10, 391. doi:10.3390/su10020391
Higa-Nishiyama, A., Ohsato, S., Banno, S., Woo, S., Fujimura, M., Yamaguchi, I., et al. (2006). Cloning and characterization of six highly similar endo-1, 3-β-glucanase genes in hexaploid wheat. Plant Physiology Biochem. 44, 666–673. doi:10.1016/j.plaphy.2006.10.022
Iqbal, M., Iftikhar, K., and Ilyas, M. (1993). Evaluation of chickpea germplasm for resistance against wilt disease (fusarium oxysporum). J. Agric. Res. Pak. 31.
Jiménez-Díaz, R. M., Castillo, P., del Mar Jiménez-Gasco, M., Landa, B. B., and Navas-Cortés, J. A. (2015). Fusarium wilt of chickpeas: biology, ecology and management. Crop Prot. 73, 16–27. doi:10.1016/j.cropro.2015.02.023
Jukanti, A. K., Gaur, P. M., Gowda, C., and Chibbar, R. N. (2012). Nutritional quality and health benefits of chickpea (cicer arietinum l.): a review. Br. J. Nutr. 108, S11–S26. doi:10.1017/S0007114512000797
Karaca, N., Ates, D., Nemli, S., Ozkuru, E., Yilmaz, H., Yagmur, B., et al. (2019). Genome-wide association studies of protein, lutein, vitamin c, and fructose concentration in wild and cultivated chickpea seeds. Crop Sci. 59, 2652–2666. doi:10.2135/cropsci2018.12.0738
Leitão, S. T., Malosetti, M., Song, Q., van Eeuwijk, F., Rubiales, D., and Vaz Patto, M. C. (2020). Natural variation in Portuguese common bean germplasm reveals new sources of resistance against fusarium oxysporum f. sp. phaseoli and resistance-associated candidate genes. Phytopathology 110, 633–647. doi:10.1094/PHYTO-06-19-0207-R
Li, Y., Cao, X.-L., Zhu, Y., Yang, X.-M., Zhang, K.-N., Xiao, Z.-Y., et al. (2019). Osa-mir398b boosts h2o2 production and rice blast disease-resistance via multiple superoxide dismutases. New Phytol. 222, 1507–1522. doi:10.1111/nph.15678
Li, Y., Ruperao, P., Batley, J., Edwards, D., Khan, T., Colmer, T. D., et al. (2018). Investigating drought tolerance in chickpea using genome-wide association mapping and genomic selection based on whole-genome resequencing data. Front. Plant Sci. 9, 190. doi:10.3389/fpls.2018.00190
Li, Y., Wang, Y., Wu, X., Wang, J., Wu, X., Wang, B., et al. (2021). Novel genomic regions of fusarium wilt resistance in bottle gourd [lagenaria siceraria (mol.) standl.] discovered in genome-wide association study. Front. Plant Sci. 12, 650157. doi:10.3389/fpls.2021.650157
Liu, W.-C., Song, R.-F., Zheng, S.-Q., Li, T.-T., Zhang, B.-L., Gao, X., et al. (2022). Coordination of plant growth and abiotic stress responses by tryptophan synthase β subunit 1 through modulation of tryptophan and aba homeostasis in arabidopsis. Mol. plant 15, 973–990. doi:10.1016/j.molp.2022.04.009
Maitlo, S. A., Rajput, N. A., Syed, R., Khanzada, M. A., Rajput, A. Q., Lodhi, A. M., et al. (2019). Microbial control of fusarium wilt of chickpea caused by fusarium oxysporum f. sp. ciceris. Pak. J. Bot. 51, 2261–2268. doi:10.30848/pjb2019-6(23)
Meirinho, S., Carvalho, M., Dominguez, Á., and Choupina, A. (2010). Isolation and characterization by asymmetric pcr of the endo1 gene for glucan endo-1, 3-β-d-glucosidase in phytophthora cinnamomi associated with the ink disease of castanea sativa mill. Braz. Archives Biol. Technol. 53, 513–518. doi:10.1590/s1516-89132010000300003
Miao, Y., Xu, L., He, X., Zhang, L., Shaban, M., Zhang, X., et al. (2019). Suppression of tryptophan synthase activates cotton immunity by triggering cell death via promoting sa synthesis. Plant J. 98, 329–345. doi:10.1111/tpj.14222
Muehlbauer, F. J., and Sarker, A. (2017). Economic importance of chickpea: production, value, and world trade. chickpea genome 5–12.
Raman, R., Warren, A., Krysinska-Kaczmarek, M., Rohan, M., Sharma, N., Dron, N., et al. (2022). Genome-wide association analyses track genomic regions for resistance to ascochyta rabiei in australian chickpea breeding germplasm. Front. Plant Sci. 13, 877266. doi:10.3389/fpls.2022.877266
Saucet, S. B., Esmenjaud, D., and Van Ghelder, C. (2021). Integrity of the post-lrr domain is required for tir-nb-lrr function. Mol. Plant-Microbe Interact. 34, 286–296. doi:10.1094/MPMI-06-20-0156-R
Saucet, S. B., Ma, Y., Sarris, P. F., Furzer, O. J., Sohn, K. H., and Jones, J. D. (2015). Two linked pairs of arabidopsis tnl resistance genes independently confer recognition of bacterial effector avrrps4. Nat. Commun. 6, 6338. doi:10.1038/ncomms7338
Schattenkerk, J. (2017). Molecular modeling of novel tryptamine analogs with antibiotic potential through their inhibition of tryptophan synthase. CMC Sr. Theses, 1656.
Sharma, M., Sengupta, A., Ghosh, R., Agarwal, G., Tarafdar, A., Nagavardhini, A., et al. (2016). Genome wide transcriptome profiling of fusarium oxysporum f sp. ciceris conidial germination reveals new insights into infection-related genes. Sci. Rep. 6, 37353–37411. doi:10.1038/srep37353
Shi, F., Wang, Y., Zhang, F., Yuan, X., Chen, H., Chen, X., et al. (2020). Soybean endo-1, 3-beta-glucanase (gmglu) interaction with soybean mosaic virus-encoded p3 protein may contribute to the intercelluar movement. Front. Genet. 11, 536771. doi:10.3389/fgene.2020.536771
Singh, C., and Vyas, D. (2021). “The trends in the evaluation of fusarium wilt of chickpea,” in Diagnostics of plant diseases (London, UK: IntechOpen).
Singh, D., and Sharma, A. (1997). Fusarium wilt of chickpea. Wallingford, UK: CAB International, 201–216.
Singh, D., and Singh, K. (2012). Chickpea diseases and their management: a review. Int. J. Plant Prot. 5, 7–14.
Singh, R., Singh, S., and Singh, K. (2015). Molecular markers for the identification of fusarium wilt resistant genes in chickpea. Plant Genet. Resour. 13, 259–266.
Soltanzadeh, M., Soltani Nejad, M., and Shahidi Bonjar, G. H. (2016). Application of soil-borne actinomycetes for biological control against fusarium wilt of chickpea (cicer arietinum) caused by fusarium solani fsp pisi. J. Phytopathology 164, 967–978. doi:10.1111/jph.12517
Thurow, L. B., Gasic, K., Bassols Raseira, M. d. C., Bonow, S., and Marques Castro, C. (2020). Genome-wide snp discovery through genotyping by sequencing, population structure, and linkage disequilibrium in brazilian peach breeding germplasm. Tree Genet. Genomes 16, 10–14. doi:10.1007/s11295-019-1406-x
Tibbs Cortes, L., Zhang, Z., and Yu, J. (2021). Status and prospects of genome-wide association studies in plants. plant genome 14, e20077. doi:10.1002/tpg2.20077
Tyagi, S., Sharma, S., Ganie, S. A., Tahir, M., Mir, R. R., and Pandey, R. (2019). Plant micrornas: biogenesis, gene silencing, web-based analysis tools and their use as molecular markers. 3 Biotech. 9, 413–512. doi:10.1007/s13205-019-1942-y
Uffelmann, E., Huang, Q. Q., Munung, N. S., De Vries, J., Okada, Y., Martin, A. R., et al. (2021). Genome-wide association studies. Nat. Rev. Methods Prim. 1, 59–21. doi:10.1038/s43586-021-00056-9
Ullah, A., Farooq, M., Rehman, A., Hussain, M., and Siddique, K. H. (2020). Zinc nutrition in chickpea (cicer arietinum): a review. Crop Pasture Sci. 71, 199–218. doi:10.1071/cp19357
Varshney, R. K., Pandey, M. K., Bohra, A., Singh, V. K., Thudi, M., and Saxena, R. K. (2019). Toward the sequence-based breeding in legumes in the post-genome sequencing era. Theor. Appl. Genet. 132, 797–816. doi:10.1007/s00122-018-3252-x
Varshney, R. K., Song, C., Saxena, R. K., Azam, S., Yu, S., Sharpe, A. G., et al. (2013). Draft genome sequence of chickpea (cicer arietinum) provides a resource for trait improvement. Nat. Biotechnol. 31, 240–246. doi:10.1038/nbt.2491
Vogt, F., Shirsekar, G., and Weigel, D. (2022). vcf2gwas: Python api for comprehensive gwas analysis using gemma. Bioinformatics 38, 839–840. doi:10.1093/bioinformatics/btab710
Wang, F., Zou, M., Zhao, L., Li, H., Xia, Z., and Wang, J. (2020). Genome-wide association mapping of late blight tolerance trait in potato (Solanum tuberosum L.). Front. Genet. 12, 714575. doi:10.3389/fgene.2021.714575
Wang, S.-B., Feng, J.-Y., Ren, W.-L., Huang, B., Zhou, L., Wen, Y.-J., et al. (2016). Improving power and accuracy of genome-wide association studies via a multi-locus mixed linear model methodology. Sci. Rep. 6, 19444–19510. doi:10.1038/srep19444
Wen, Y.-J., Zhang, H., Ni, Y.-L., Huang, B., Zhang, J., Feng, J.-Y., et al. (2018). Methodological implementation of mixed linear models in multi-locus genome-wide association studies. Briefings Bioinforma. 19, 700–712. doi:10.1093/bib/bbw145
Yao, L., Li, Y., Ma, C., Tong, L., Du, F., and Xu, M. (2020). Combined genome-wide association study and transcriptome analysis reveal candidate genes for resistance to fusarium ear rot in maize. J. Integr. plant Biol. 62, 1535–1551. doi:10.1111/jipb.12911
Yuan, J., Wang, X., Zhao, Y., Khan, N. U., Zhao, Z., Zhang, Y., et al. (2020). Genetic basis and identification of candidate genes for salt tolerance in rice by gwas. Sci. Rep. 10, 9958–9959. doi:10.1038/s41598-020-66604-7
Keywords: chickpea, Fusarium wilt, SNP genotyping, genome-wide association study (GWAS), population structure
Citation: Alsamman AM, H. Mousa K, Istanbuli T, Abd El-Maksoud MM, Tawkaz S and Hamwieh A (2024) Unveiling the genetic basis of Fusarium wilt resistance in chickpea using GWAS analysis and characterization of candidate genes. Front. Genet. 14:1292009. doi: 10.3389/fgene.2023.1292009
Received: 10 September 2023; Accepted: 26 December 2023;
Published: 19 January 2024.
Edited by:
Ertugrul Filiz, Duzce University, TürkiyeCopyright © 2024 Alsamman, H. Mousa, Istanbuli, Abd El-Maksoud, Tawkaz and Hamwieh. This is an open-access article distributed under the terms of the Creative Commons Attribution License (CC BY). The use, distribution or reproduction in other forums is permitted, provided the original author(s) and the copyright owner(s) are credited and that the original publication in this journal is cited, in accordance with accepted academic practice. No use, distribution or reproduction is permitted which does not comply with these terms.
*Correspondence: Alsamman M. Alsamman, c21haG1vdWRAYWdlcmkuc2NpLmVn; Aladdin Hamwieh, YS5oYW13aWVoQGNnaWFyLm9yZw==
Disclaimer: All claims expressed in this article are solely those of the authors and do not necessarily represent those of their affiliated organizations, or those of the publisher, the editors and the reviewers. Any product that may be evaluated in this article or claim that may be made by its manufacturer is not guaranteed or endorsed by the publisher.
Research integrity at Frontiers
Learn more about the work of our research integrity team to safeguard the quality of each article we publish.