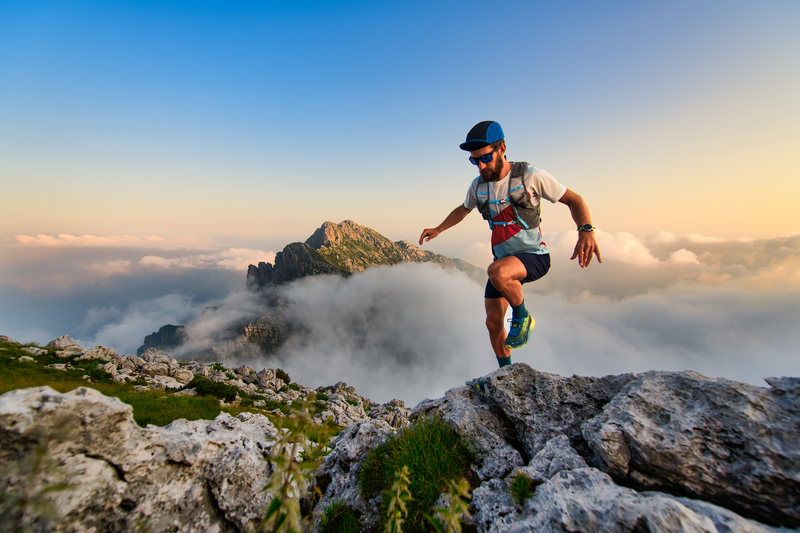
95% of researchers rate our articles as excellent or good
Learn more about the work of our research integrity team to safeguard the quality of each article we publish.
Find out more
EDITORIAL article
Front. Genet. , 25 September 2023
Sec. Human and Medical Genomics
Volume 14 - 2023 | https://doi.org/10.3389/fgene.2023.1287894
This article is part of the Research Topic Insights in Human and Medical Genomics: 2022 View all 7 articles
Editorial on the Research Topic
Insights in human and medical genomics: 2022
Genomics has matured considerably over the last decades. Increasingly, genome-scale genetic data are accompanied by other omic data: metabolomic, proteomic, epigenetic or methylomic, single-cell and spatial, lipidomic, diverse organ or tissue type, exosomal, imaging, in vivo and model organism, electronic health record (EHR), wearable device, cognitive or intrinsic capacity, and other health and healthspan data. At the frontiers of genomics, we increasingly welcome research that integrates multiple data types. More importantly: the maturation of genomics is not merely expansion into more dimensions of multidimensional omics dataspace, but also application of increasing wisdom in analysis and interpretation (Weiskittel et al., 2021).
Most modern translational science is built on two epistemological pillars: statistical significance and strength. However, the most impactful translational knowledge learned in the 20th century—that smoking causes cancer—necessitated the use of more diversified and robust epistemological techniques, collectively known as Hill’s criteria (Figure 1). Systems biology incorporates all of these lines of evidence, providing a holistic view of biomedical problems (Brigandt, 2013). These analyses particularly leverage the epistemological concepts of coherence and plausibility by fitting the ensemble of the data together consistently with prior knowledge. In the context of translational research, this emphasis is referred to as biological plausibility (Fedak et al., 2015). This synergy results from the integration of knowledge from various evidence streams, particularly multi-omics (Hood, 2013). Biological plausibility places current research coherently and consistently in the context of previous research. New observations are interpreted not only in the light of past and current observations, but also in the light of previous knowledge. Today, fuller implementation of epistemology for translational genomics is more feasible than it was a few years ago—because then we had much less knowledge of human molecular physiology.
FIGURE 1. Multifaceted evidence will be necessary to best apply systems genomics to complex diseases. These lines of evidence were used in the 20th century to prove that smoking causes cancer and were termed “Hill’s Criteria”. The most important proofs of translational knowledge in this century will also require robust and diverse evidence. Both human and artificial intelligence (AI) analysis of basic research and translational medical and health data will leverage all of these pillars of knowledge.
For complex diseases, including many with substantial public health impacts such as dementia, allergies, infectious diseases, cancer, autoimmune disorders, and metabolic conditions, it is necessary to adapt the criterion of epistemological coherence. Hypotheses for complex diseases must assume multiple causes and potential interventions. Therefore, when seeking evidence of coherence, we should not anticipate a single unified coherent system but rather several potentially distinct coherent systems. Occam’s razor is rarely suitable for study of these complex diseases, as ample evidence already points to complex interactions shaped by non-parsimonious processes of evolution.
Understanding complex systems cannot be achieved by examining a single dimension at a time. Research portfolios centered on single-dimensional hypotheses would take centuries, incur exorbitant costs, and, in the case of clinical trials, necessitate more participants than could be recruited. In practice, embracing such a portfolio would mean no sufficiently complex disease could ever be adequately researched. Some variables, such as diet, offer an infinite range of possibilities, considering factors like doses, frequencies, and modalities. Synergy between variables may be important. Imagine being marooned on a desert island, and attempting to construct an escape raft using only individual materials at hand, such as driftwood, salvaged boards, coconuts, vines, and nails. Each of these materials, except for nails, may have some efficacy when used in isolation. However, even using the best material alone might not result in a successful raft, and the entire endeavor could be prematurely abandoned. The most effective raft is constructed by combining these materials in a multimodal manner, including nails—despite the fact that nails, in isolation, do not even float. Systems epistemology is required to build the raft, leveraging both past knowledge of how rafts can be constructed (consistency) and an understanding of how the available parts fit together in the current situation (coherency).
Correlation is not always causation, but sometimes is. Correlation of two variables (e.g., intervention and outcome) resulting from prospective randomization in an RCT is a robust argument for causation and has been considered a gold standard for clinical evidence (Ashcroft, 2004). Such a correlation must be mechanistic: the intervention causes the change in outcome. However, correlation from almost any other source of evidence introduces two additional possibilities. The outcome might cause the intervention or a third bioentity might cause both. Consequently, it is easy to dismiss correlations as epistemological evidence, often summarized with the phrase, “Correlation is not causation.” This statement can sometimes be taken too literally. Correlation is evidence for causation when it is combined with other evidence including dose-response, time-dependency, and biological-plausibility arguments (Hwang et al., 2005). Research that provides evidence for causation and mechanism leveraging multiple lines of epistemological inference will be increasingly valuable (Weith and Beyer, 2023). Although human analyses remain far from obsolete, we anticipate that “cyborg” analyses, which combine human insight with artificial intelligence (AI), will become increasingly compelling.
In this Research Topic, authors survey several aspects of these new frontiers in genomics. Varzari et al. leveraged classical techniques of family-based genetics to investigate the genetic influences on environmental exposure to mycobacterial infection and the resulting susceptibility to disease (Bennett et al., 2002). Savytska et al. contribute to tool development for studying the unexplored contribution of transposable elements to gene regulatory networks impacting complex traits and diseases (Kaplow et al., 2023). Fan et al. review the growing importance of omics in translational medicine by highlighting examples of non-coding RNAs as promising biomarkers (Skroblin and Mayr, 2014). Xulu et al. argue that multidimensional omics evidence is needed to best tailor personalized medicine for cancer, using evolution to anchor the coherence of these lines of evidence (Rivenbark et al., 2013). Diaz-Gonzalez et al. expand the spectrum of variants influencing Saethre-Chotzen syndrome (Paumard-Hernández et al., 2015). Shum et al. leverage high-throughput pairwise end-sequencing (Roach et al., 1995) and advances in bioinformatics to achieve newborn screening for spinal muscular atrophy (Nishio et al., 2023).
At Frontiers in Genetics: Human and Medical Genomics we continue to encourage submission of high-quality research. Priorities include encouraging authors from developing countries and disadvantaged authors from all countries. We solicit not just novel “first to the finish line” research, but also research that confirms previous findings, particularly in new and diverse populations. We look forward to crossing future genomics frontiers together.
JR: Writing–original draft, Writing–review and editing. MF: Writing–original draft, Writing–review and editing.
We are grateful for all the reviewers and editors who have contributed their time and expertise to advancing Frontiers in Genetics: Human and Medical Genomics over the last year.
The authors declare that the research was conducted in the absence of any commercial or financial relationships that could be construed as a potential conflict of interest.
The authors declared that they were an editorial board member of Frontiers, at the time of submission. This had no impact on the peer review process and the final decision.
All claims expressed in this article are solely those of the authors and do not necessarily represent those of their affiliated organizations, or those of the publisher, the editors and the reviewers. Any product that may be evaluated in this article, or claim that may be made by its manufacturer, is not guaranteed or endorsed by the publisher.
Ashcroft, R. E. (2004). Current epistemological problems in evidence-based medicine. J. Med. Ethics 30, 131–135. doi:10.1136/jme.2003.007039
Bennett, S., Lienhardt, C., Bah-Sow, O., Gustafson, P., Manneh, K., Del Prete, G., et al. (2002). Investigation of environmental and host-related risk factors for tuberculosis in Africa. II. Investigation of host genetic factors. Am. J. Epidemiol. 155, 1074–1079. doi:10.1093/aje/155.11.1074
Brigandt, I. (2013). Systems biology and the integration of mechanistic explanation and mathematical explanation. Stud. Hist. Philosophy Biol. Biomed. Sci. 44, 477–492. doi:10.1016/j.shpsc.2013.06.002
Fedak, K. M., Bernal, A., Capshaw, Z. A., and Gross, S. (2015). Applying the bradford Hill criteria in the 21st century: how data integration has changed causal inference in molecular epidemiology. Emerg. Themes Epidemiol. 12, 14. doi:10.1186/s12982-015-0037-4
Hood, L. (2013). Systems biology and p4 medicine: past, present, and future. Rambam Maimonides Med. J. 4, e0012. doi:10.5041/RMMJ.10112
Hwang, D., Rust, A. G., Ramsey, S., Smith, J. J., Leslie, D. M., Weston, A. D., et al. (2005). A data integration methodology for systems biology. Proc. Natl. Acad. Sci. U. S. A. 102, 17296–17301. doi:10.1073/pnas.0508647102
Kaplow, I. M., Lawler, A. J., Schäffer, D. E., Srinivasan, C., Sestili, H. H., Wirthlin, M. E., et al. (2023). Relating enhancer genetic variation across mammals to complex phenotypes using machine learning. Science 380, eabm7993–172. doi:10.1126/science.abm7993
Nishio, H., Niba, E. T. E., Saito, T., Okamoto, K., Takeshima, Y., and Awano, H. (2023). Spinal muscular atrophy: the past, present, and future of diagnosis and treatment. Int. J. Mol. Sci. 24, 11939. doi:10.3390/ijms241511939
Paumard-Hernández, B., Berges-Soria, J., Barroso, E., Rivera-Pedroza, C. I., Pérez-Carrizosa, V., Benito-Sanz, S., et al. (2015). Expanding the mutation spectrum in 182 Spanish probands with craniosynostosis: identification and characterization of novel TCF12 variants. Eur. J. Hum. Genet. 23, 907–914. doi:10.1038/ejhg.2014.205
Rivenbark, A. G., O'Connor, S. M., and Coleman, W. B. (2013). Molecular and cellular heterogeneity in breast cancer: challenges for personalized medicine. Am. J. Pathology 183, 1113–1124. doi:10.1016/j.ajpath.2013.08.002
Roach, J. C., Boysen, C., Wang, K., and Hood, L. (1995). Pairwise end sequencing: A unified approach to genomic mapping and sequencing. Genomics 26, 345–353. doi:10.1016/0888-7543(95)80219-c
Skroblin, P., and Mayr, M. (2014). Going long": long non-coding RNAs as biomarkers. Circulation Res. 115, 607–609. doi:10.1161/CIRCRESAHA.114.304839
Weiskittel, T. M., Correia, C., Yu, G. T., Ung, C. Y., Kaufmann, S. H., Billadeau, D. D., et al. (2021). The trifecta of single-cell, systems-biology, and machine-learning approaches. Genes 12, 1098. doi:10.3390/genes12071098
Keywords: genomics, Hill’s Criteria, multiomics, systems biology, epistemology, biological plausibility, correlation and causation, translational science
Citation: Roach JC and Freidin MB (2023) Editorial: Insights in human and medical genomics: 2022. Front. Genet. 14:1287894. doi: 10.3389/fgene.2023.1287894
Received: 03 September 2023; Accepted: 15 September 2023;
Published: 25 September 2023.
Edited and reviewed by:
Veronique Vitart, University of Edinburgh, United KingdomCopyright © 2023 Roach and Freidin. This is an open-access article distributed under the terms of the Creative Commons Attribution License (CC BY). The use, distribution or reproduction in other forums is permitted, provided the original author(s) and the copyright owner(s) are credited and that the original publication in this journal is cited, in accordance with accepted academic practice. No use, distribution or reproduction is permitted which does not comply with these terms.
*Correspondence: Jared C. Roach, amFyZWQucm9hY2hAaXNic2NpZW5jZS5vcmc=
Disclaimer: All claims expressed in this article are solely those of the authors and do not necessarily represent those of their affiliated organizations, or those of the publisher, the editors and the reviewers. Any product that may be evaluated in this article or claim that may be made by its manufacturer is not guaranteed or endorsed by the publisher.
Research integrity at Frontiers
Learn more about the work of our research integrity team to safeguard the quality of each article we publish.