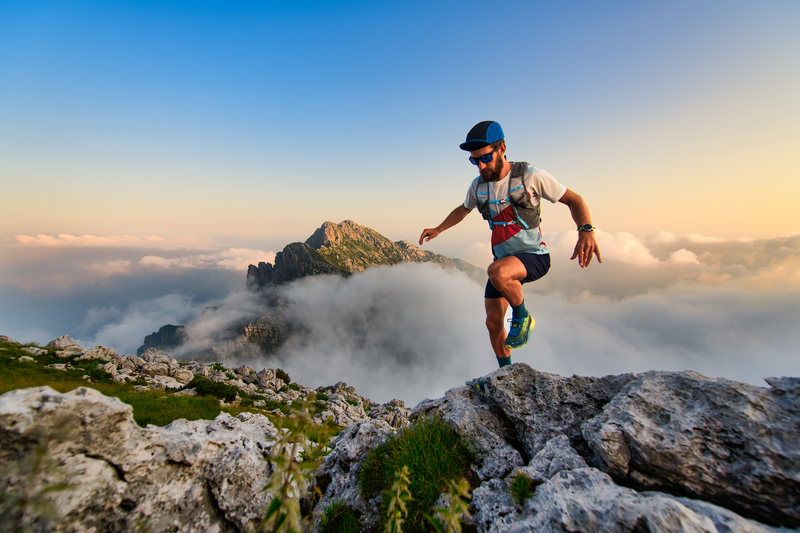
94% of researchers rate our articles as excellent or good
Learn more about the work of our research integrity team to safeguard the quality of each article we publish.
Find out more
ORIGINAL RESEARCH article
Front. Genet. , 20 December 2023
Sec. Applied Genetic Epidemiology
Volume 14 - 2023 | https://doi.org/10.3389/fgene.2023.1260367
This article is part of the Research Topic Mendelian Randomization: the Study of Causal Relationships between Tumors and Underlying Pathogenic Factors View all 15 articles
Background: The causation of Glycemic Traits and risks of Melanoma remains unknown. We used Mendelian Randomization (MR) to assess the links between Glycemic Traits and Melanoma.
Method: Pooled data from Genome-Wide Association Studies (GWAS) were utilized to examine the relationships that exist between Fasting Insulin (n = 26), 2-h Glucose (n = 10), Fasting Glucose (n = 47), HbA1c (n = 68), and Type-2 Diabetes (n = 105) and Melanoma. We evaluated the correlation of these variations with melanoma risk using Two-Samples MR.
Result: In the IVW model, Fasting Glucose (OR = 0.99, 95%CI = 0.993–0.998, p < 0.05, IVW), Type-2 Diabetes (OR = 0.998, 95%CI = 0.998–0.999, p < 0.01, IVW) and HbA1c (OR = 0.19, 95%CI = 0.0415–0.8788, p < 0.05, IVW) was causally associated with a lower risk of Melanoma. In all models analyzed, there was no apparent causal relationship between Fasting Insulin and Melanoma risk. There was no obvious causal difference in the IVW analysis of 2-h Glucose and Melanoma, but its p < 0.05 in MR Egger (OR = 0.99, 95%CI = 0.9883–0.9984, p < 0.05, MR Egger), and the direction was consistent in other MR analyses, suggesting that there may be a causal relationship.
Conclusion: The results of this study suggest that a higher risk of Fasting Glucose, Type-2 Diabetes, 2-h Glucose, and HbA1c may be associated with a lower risk of Melanoma. However, no causal relationship between fasting insulin and melanoma was found. These results suggest that pharmacological or lifestyle interventions that regulate plasma glucose levels in the body may be beneficial in the prevention of melanoma.
Melanoma is a malignant tumor produced by the malignant transformation of melanocytes, which has a high probability of local spread and metastatic spread. Studies have shown that its incidence increases linearly in young and middle-aged people aged 25 to 50, and is high in people aged 57 (Carr et al., 2020). It is less common than other types of skin cancer but accounts for 73 percent of skin cancer-related deaths (Gershenwald and Guy, 2016). Studies have shown that in the next 10 years, the incidence and mortality of melanoma will continue to rise (Whiteman et al., 2016). The intervention effect of early surgical treatment and late radiotherapy and chemotherapy on the prognosis of patients is not satisfactory, and the results of several clinical trials have shown that the objective remission rate of patient’s symptoms after treatment is less than 1% (O'Neill et al., 2006; Atzpodien et al., 2008; Bhatia et al., 2012). It is particularly important to look for risk factors to prevent the occurrence of Melanoma.
Several recent studies have shown that obesity is positively associated with the risk of melanoma (Dusingize et al., 2020; Larsson and Burgess, 2021). Obese adults have a higher prevalence of metabolic problems, such as insulin irregularities, hyperglycemia, and Type-2 Diabetes. Some researches has found that Type-2 Diabetes may be associated with an increased risk of Melanoma (Harding et al., 2015; Yuan et al., 2020). However, other cohort studies and case-control studies have found the opposite results (Qi et al., 2014; Malavolti et al., 2017). Previous prospective studies have shown that higher Fasting Glucose is closely related to the occurrence and development of Melanoma (Stattin et al., 2007). Recent studies have demonstrated a positive association between Glycemic Traits and the risk of colorectal cancer (Murphy et al., 2022a). But the MR studies of the associations between various Glycemic Traits and melanoma have not yet been reported.
This study used MR to explore the causal relationship between Glycemic Traits and Melanoma risk. MR is a comparable method to randomization in randomized controlled trials. When parents with two or more pairs of qualities cross when alleles are separated, genes on non-homologous chromosomes operate as free combinations, according to the law of independent assortment. Since germline genetic variation, and the random nature of allelic segregation is fixed at conception, MR analysis is less susceptible to traditional confounding and reverse causation. In this study, we used GWAS related to Fasting Glucose, Fasting Insulin, 2-h Glucose, HbA1c, and Type-2 Diabetes, GWAS data on Melanoma from risk on United Kingdom Biobank cohort study and FinnGen cohort study (Mahajan et al., 2018; Chen et al., 2021). Two-sample MR was used to explore the potential causal influence of the Glycemic Traits on the risk of Melanoma.
MR investigates the link between exposure and illness by employing genetic variation Single Nucleotide Polymorphisms (SNPs) as Instrumental Variables IV). IV was extracted from a disease-specific Genome-Wide Association Studies (GWAS) dataset for this investigation. The IV in this work should fulfill three criteria: there should be a high connection between IV and exposure, IV should only affect the outcomes through exposure, and IV should not have horizontal pleiotropy. Appropriate SNPs for usage as IVs must be strongly linked to malignancy (p < 5 × 10−8). To ensure independence, SNPs were restricted by low linkage disequilibrium (LD, r2 < 0.001, window size = 10,000 kb) using clumping. By MR GWAS data. from different sources were analyzed to assess the causal relationship between glycemic signature and melanoma risk. Assess the strength of IV using the F statistic (F = beta2/se2), where β is the effect size of the allele and SE is the standard error (Feng et al., 2022). If F > 10, the correlation between IV and exposure was considered strong enough to protect the results of MR analysis from weak instrument bias. Meanwhile, it will ensure no confounders like UV radiation, light skin type, the presence of multiple atypical nevi, and a positive family history using the Phenoscanner (http://www.phenoscanner.medschl.cam.ac.uk/phenoscanner) website to have a search over each SNP (Rastrelli et al., 2014; Ugurel and Gutzmer, 2023).
Glycemic Traits data come from the largest GWAS to date (Glucose And Insulin-related Traits Consortium). A GWAS study of 2 h Glucose, Fasting Glucose, and Fasting Insulin included 63,396 (SNPs= 27,330,879), 200,622 (SNPs= 31,008,728), and 151,013 (SNPs= 29,664,438) participants of European ancestry, respectively (Chen et al., 2021). The GWAS for Type-2 Diabetes included 74,124 individuals with Type-2 Diabetes and 824,006 controls of European ancestry (SNPs= 21,000,000) (Mahajan et al., 2018). The HbA1c GWAS from the United Kingdom biobank (http://www.nealelab.is/uk-biobank) included 361,194 participants of European ancestry (SNPs= 1,048,575). The GWAS of Melanoma were obtained from United Kingdom biobank (https://www.ukbiobank.ac.uk/) and FinnGen (https://www.finngen.fi/en/access_results), the GWAS of United Kingdom biobank included 3,598 patients and 459,335 for controls (SNPs= 9,851,867) of European ancestry, the GWAS of FinnGen included 393 patients and 180,622 controls (SNPs= 16,380,337) of European ancestry. All study participants gave written informed consent, and the ethics committee approved all studies. SNPs data can be found in Supplementary Table S1.
We estimated the relationship between Glycemic Traits and Melanoma risk using MR Egger, Inverse Variance Weighting (IVW), weighted Median, Simple Mode, and Weighted Mode MR methods. The IVW method assumes that all SNPs do not have horizontal pleiotropy (The impact of genetic variation on results is solely influenced by exposure of interest) and that all SNPs are effective tools. The fixed-effect inverse variance weighting (IVW) method was mainly used as the main analysis method (Kamiza et al., 2022). The intercept of the MR-Egger test was used to examine potential pleiotropic effects. Scatterplots are used to display the findings of several MR procedures. Odds ratios (ORs) and 95% confidence intervals (CIs) were used to represent the causal effects of overall and Melanoma. We utilized scatterplots to show the genetic relationship between glycemic characteristics and melanoma risk, and funnel plots to visually analyze the consistency of MR estimations and potential related biases. R software was used for these analyses, where the “Two-Sample MR” and “MR-PRESSO” R packages were used.
Pleiotropy was investigated using the MR-Egger approach, which was used to determine if a single locus impacts numerous phenotypes. Second, the Leave-one-out sensitivity test was used to gradually remove the SNPs to ensure that the results were credible. The Cochran Q statistic was used to standardize heterogeneity analyses. In addition, MR PRESSO was used to detect and eliminate anomalous instrumental factors.
After a quality control process, we obtained 10 SNPs strongly associated with 2-h Glucose, 47 SNPs strongly associated with Fasting Glucose, 26 SNPs associated with Fasting Insulin, and 105 strongly associated with Type-2 Diabetes from GWAS and 68 SNPs closely related to Fasting Glucose. The F-statistics of these SNPs were all greater than 10, indicating that our instrumental variables were closely related to Glucose Traits. Furthermore, our instrumental variables were not directly associated with the risk of Melanoma (Table 1).
TABLE 1. Power calculation for Mendelian randomization analyses for glycemic traits in relation to Melanoma risk.
IVW provides accurate estimates since the lack of heterogeneity and directional pleiotropy between exposure and outcome variables. Focusing primarily on the results of the IVW analysis, we assessed the causal relationship between these SPNs and melanoma risk with the Glycemic Traits (Table 2). The results showed that Fasting Glucose (OR = 0.99, 95%CI = 0.993–0.998, p < 0.05, IVW), Type-2 Diabetes (OR = 0.998, 95%CI = 0.998–0.999, p < 0.01, IVW) and HbA1c (OR= 0.19, 95%CI = 0.0415–0.8788, p < 0.05, IVW) was causally associated with a lower risk of Melanoma. Its orientation is consistent with several other MR analysis methods (Figures 1A–C). The 2-h Glucose and Fasting Insulin results showed no apparent causal relationship with Melanoma risk. Among them, no obvious causal difference was found in the IVW analysis of 2-h Glucose, but its p < 0.05 in MR Egger, and the direction was consistent in other MR analyses, suggesting that there may be a causal relationship (Figure 1D).
FIGURE 1. Scatter plot of genetic causality between Glycemic Traits and Melanoma using different MR methods. (A) Fasting Glucose (B) Type 2 Diabetes (C) HbA1c (D) 2-h Glucose. The dark blue line represents MR Egger, the light green line represents simple mode, the dark green line represents Weighted median, the light blue line represents IVW, and the red line represents Weighted mode.
In MR Egger, the p-values of MR Egger intercepts in each instrumental variable of Glycemic Traits were greater than 0.05, suggesting that the intercept does not exist, indicating fasting There was no horizontal pleiotropy for Fasting Glucose, 2-h Glucose, Fasting Insulin, HbA1c, and Type-2 Diabetes (Table 3). However, we found evidence of heterogeneity between HbA1c and melanoma risk with a p-value of 0.03442561 for the Q statistic. As we used the random-effects IVW as main result in MR of HbA1c, heterogeneity is acceptable (Burgess et al., 2019). No heterogeneity was found in the other analyses. Then we performed the Leave-one-out (Figures 2A–D) method and MR-PRESSO (Figures 3A–D) to identify and delete abnormal instrumental variables. The results showed that no abnormal instrumental variables were found, and the above results suggested that the MR analysis results were relatively stable.
TABLE 3. Estimates of Egger intercept to evaluate evidence for directional pleiotropy in MR association.
FIGURE 2. Forest map of Melanoma based on Glycemic Traits. (A) 2-h glucose (B) Fasting Glucose (C) Type 2 Diabetes (D) HbA1c. Black dots represent estimates of causal effects of Glycemic Traits on Melanoma (beta coefficients). The black line represents the estimated 95% confidence interval.
FIGURE 3. Funnel plot of Melanoma based on Glycemic Traits genetic variants. (A) 2-h glucose (B) Fasting Glucose (C) Type 2 Diabetes (D) HbA1c. Overall causal estimates (beta coefficients) of Glycemic Traits and Melanoma estimated by the IVW (light blue line) and MR-Egger (dark blue line) methods are shown.
Melanoma is a malignant tumor caused by melanocytes that is also a very deadly disease due to its high metastatic potential, accounting for 75% of skin cancer deaths (Davis et al., 2019). People who have a family history of skin cancer, have a high amount of common or underdeveloped nevi, or are excessively exposed to UV light are at high risk for melanoma (Dummer et al., 2009; Guo et al., 2016). It is still unknown whether endocrine factors can also affect the occurrence of melanoma (Guo et al., 2016). This study used the MR method for the first time to explore the causal relationship between the characteristics of Fasting Glucose (including 2-h Glucose, Fasting Glucose, Fasting Insulin, Type-2 Diabetes, and HbA1c) and the risk of Melanoma from the perspective of genetics. Overall, we found that Fasting Glucose, Type-2 Diabetes, and higher HbA1c levels were negatively associated with the risk of Melanoma. The results of MR Egger suggest that 2-h Glucose may be negatively related to the risk of Melanoma. But there is no evidence that gene-predicted Fasting Insulin levels increase the risk of Melanoma. Due to the use of suitable genetic instrument tools (F-statistics>10 and r2<0.001) in this study, no significant SNP was detected in the retention method or MR-PRESSO, and the results were highly consistent among the 5 MR algorithms. Therefore, we believe that the results of this study are to some extent reliable. The results of this study suggest that people with higher Glycemic Traits levels may be at low risk for melanoma. Patients and high-risk populations may reduce the risk of melanoma by adjusted dietary structure regulating Glycemic Traits.
In previous studies, we have noticed that Glycemic Traits exhibit different causal relationships among different tumors. There is a positive correlation between plasma glucose index and disease risk in lung cancer, but there is no significant correlation with colon cancer (Murphy et al., 2022b; Du et al., 2022). A meta-analysis shows that glycemic index will increase the overall risk of cancer, increase the risk of breast cancer (Long et al., 2022), and is positively correlated with the risk of bladder cancer and gastric cancer (Zhu et al., 2020; Kim et al., 2022). Relevant studies have shown that higher HbA1c levels are associated with an increased risk of colorectal cancer, pancreatic cancer, respiratory cancer, and female reproductive tract cancer, and are not associated with an increased risk of breast cancer, gastrointestinal or urinary system malignancies (Hong et al., 2009; Lu et al., 2015; Hope et al., 2016; Murphy et al., 2022b), but are linearly associated with overall cancer-related deaths (Yoo et al., 2022). A study shows that Type-2 Diabetes will increase the risk of colorectal cancer (Murphy et al., 2022b). The cohort study and case-control study in Melanoma suggest that Type-2 Diabetes may be negatively related to the risk of Melanoma (Harding et al., 2015; Yuan et al., 2020), but the opposite results have appeared in other studies (Qi et al., 2014; Malavolti et al., 2017). The above studies suggest that the specific relationship between Glycemic Traits and Melanoma is still contradictory.
The results of this study suggest that 2-h Glucose (OR = 0.99, 95% CI = 0.984–0.998, p < 0.05, MR Egger), Fasting Glucose (OR = 0.99, 95% CI = 0.993–0.998, p < 0.05, IVW), Type-2 Diabetes (OR = 0.998, 95% CI = 0.998–0.999, p < 0.01, IVW) and higher HbA1c level (OR = 0.19, 95% CI = 0.0415–0.8788, p < 0.05, IVW) are all possible negatively related to the risk of Melanoma. This is different from the manifestation of Glycemic Traits in causal relationships with other tumors. This may be due to the reduction of melanocytes in Diabetes patients, whose melanin content is related to plasma glucose control in diabetes and obesity (Mackiewicz-Wysocka et al., 2014). Research shows that people with light skin are about 30 times more likely to suffer from Melanoma than people with dark skin (Doepner et al., 2022). This is because the pigmentation of human skin is determined by the transfer of mature melanin synthesized by epidermal Melanoma cells to the surrounding keratinocytes. Human Chromatophores synthesize two types of melanin, namely, eumelanin (EM) and phaeomelanin (PM). The content of eumelanin is directly related to skin pigmentation and has a photoprotective effect, which can protect the skin from ultraviolet rays, thereby reducing the incidence rate of Melanoma (Upadhyay et al., 2022). Moreover, a high level of plasma glucose is often associated with high BMI and obesity. Studies have shown that obesity is associated with elevated circulating estradiol levels due to the aromatase activity of adipose tissue converting androgens into estrogen compounds (Schneider et al., 1979). In primary Melanoma, there may be high expression of estrogen receptors β with anti-melanoma-proliferative, and sending non-classical estrogen signals through G protein-coupled receptors (de Giorgi et al., 2013; Marzagalli et al., 2015). This may explain the different causal relationships between Glycemic Traits in Melanoma and other tumors.
Insulin is a protein hormone secreted by cells stimulated by endogenous or exogenous substances such as glucose, lactose, ribose, arginine, glucagon, etc. Related research suggests that it may be related to the development of tumors. Lilalutide, an analog of glucagon-like peptide 1, is a molecule that regulates glucose by increasing insulin production and inhibiting glucagon secretion. It can significantly reduce the formation of NET in tumor mice by improving the plasma glucose of patients, inhibiting tumor progression, and enhancing the anti-tumor effect of PD-1 inhibitors (Chen et al., 2022). Insulin is also an important cell growth factor that can promote cell growth, proliferation, and migration (Leitner et al., 1997). Higher fasting insulin is positively correlated with the risk of colorectal cancer (Murphy et al., 2022b). Extracellular vesicles secreted by breast cancer cells inhibit insulin secretion through miR-122, thereby damaging systemic glucose homeostasis and promoting tumor growth (Cao et al., 2022). However, in this study, the results suggest that there is no correlation between insulin level and the risk of Melanoma (OR = 0.99, 95% CI = 0.994–1.005, p = 0.91, IVW). Therefore, the causal relationship between Insulin and the risk of Melanoma requires more research.
There are still limitations to this study. Firstly, we restricted the relevance of the findings to other groups by concentrating on research subjects with European populations. Secondly, this study did not consider the impact of gender on MR analysis and did not conduct further subgroup analysis. Thirdly, to verify the findings, this study does not analyze additional data sources. Finally, This study used Mendelian Randomization analysis, which has the potential for weak instrument bias and pleiotropy. Future research is needed to address these limitations and to confirm the findings of this study.
Although previous observational studies can identify the relationship between Glucose, Type-2 Diabetes, Insulin and the risk of Melanoma, however, because of research confounding variables, a causal association cannot be established. In conclusion, this study used MR technology for the first time to analyze the causal relationship between Glycemic Traits and Melanoma and found that there is a negative correlation, and the underlying mechanism may provide valuable insights for carcinogenesis.
The raw data supporting the conclusion of this article will be made available by the authors, without undue reservation.
Y-CZ: Conceptualization, Data curation, Project administration, Writing–original draft, Writing–review and editing. C-DL: Conceptualization, Formal Analysis, Investigation, Resources, Validation, Writing–review and editing. Q-YL: Data curation, Formal Analysis, Methodology, Project administration, Validation, Writing–original draft. J-NS: Conceptualization, Data curation, Investigation, Methodology, Writing–original draft. JS: Data curation, Funding acquisition, Investigation, Resources, Software, Supervision, Visualization, Writing–original draft, Writing–review and editing. MY: Conceptualization, Formal Analysis, Methodology, Project administration, Supervision, Writing–original draft, Writing–review and editing, Funding acquisition.
The author(s) declare financial support was received for the research, authorship, and/or publication of this article. This work was funded by a Research Assistance Project of the Second Affiliated Hospital of Zhejiang Chinese Medicine University.
Thanks for the sharing of datasets provided by public databases.
The authors declare that the research was conducted in the absence of any commercial or financial relationships that could be construed as a potential conflict of interest.
All claims expressed in this article are solely those of the authors and do not necessarily represent those of their affiliated organizations, or those of the publisher, the editors and the reviewers. Any product that may be evaluated in this article, or claim that may be made by its manufacturer, is not guaranteed or endorsed by the publisher.
The Supplementary Material for this article can be found online at: https://www.frontiersin.org/articles/10.3389/fgene.2023.1260367/full#supplementary-material
Atzpodien, J., Terfloth, K., Fluck, M., and Reitz, M. (2008). Cisplatin, gemcitabine and treosulfan is effective in chemotherapy-pretreated relapsed stage IV uveal melanoma patients. Cancer Chemother. Pharmacol. 62 (4), 685–688. doi:10.1007/s00280-007-0655-9
Bhatia, S., Moon, J., Margolin, K. A., Weber, J. S., Lao, C. D., Othus, M., et al. (2012). Phase II trial of sorafenib in combination with carboplatin and paclitaxel in patients with metastatic uveal melanoma: SWOG S0512. PLoS One 7 (11), e48787. doi:10.1371/journal.pone.0048787
Burgess, S., Davey Smith, G., Davies, N. M., Dudbridge, F., Gill, D., Glymour, M. M., et al. (2019). Guidelines for performing Mendelian randomization investigations: update for summer 2023. Wellcome Open Res. 4, 186. doi:10.12688/wellcomeopenres.15555.2
Cao, M., Isaac, R., Yan, W., Ruan, X., Jiang, L., Wan, Y., et al. (2022). Cancer-cell-secreted extracellular vesicles suppress insulin secretion through miR-122 to impair systemic glucose homeostasis and contribute to tumour growth. Nat. Cell Biol. 24 (6), 954–967. doi:10.1038/s41556-022-00919-7
Carr, S., Smith, C., and Wernberg, J. (2020). Epidemiology and risk factors of melanoma. Surg. Clin. North Am. 100 (1), 1–12. doi:10.1016/j.suc.2019.09.005
Chen, D., Liang, H., Huang, L., Zhou, H., and Wang, Z. (2022). Liraglutide enhances the effect of checkpoint blockade through the inhibition of neutrophil extracellular traps in murine lung and liver cancers. FEBS Open Bio. doi:10.1002/2211-5463.13499
Chen, J., Spracklen, C. N., Marenne, G., Varshney, A., Corbin, L. J., Luan, J., et al. (2021). The trans-ancestral genomic architecture of glycemic traits. Nat. Genet. 53 (6), 840–860. doi:10.1038/s41588-021-00852-9
Davis, L. E., Shalin, S. C., and Tackett, A. J. (2019). Current state of melanoma diagnosis and treatment. Cancer Biol. Ther. 20 (11), 1366–1379. doi:10.1080/15384047.2019.1640032
de Giorgi, V., Gori, A., Gandini, S., Papi, F., Grazzini, M., Rossari, S., et al. (2013). Oestrogen receptor beta and melanoma: a comparative study. Br. J. Dermatol 168 (3), 513–519. doi:10.1111/bjd.12056
Doepner, M., Lee, I., Natale, C. A., Brathwaite, R., Venkat, S., Kim, S. H., et al. (2022). Endogenous DOPA inhibits melanoma through suppression of CHRM1 signaling. Sci. Adv. 8 (35), eabn4007. doi:10.1126/sciadv.abn4007
Du, H., Zhang, T., Lu, X., Chen, M., Li, X., and Li, Z. (2022). Glycemic index, glycemic load, and lung cancer risk: a meta-analysis of cohort and case-control studies. PloS One 17 (9), e0273943. doi:10.1371/journal.pone.0273943
Dummer, R., Hauschild, A., and Pentheroudakis, G. (2009). Cutaneous malignant melanoma: ESMO clinical recommendations for diagnosis, treatment and follow-up. Ann. Oncol. 20 (Suppl. 4), 129–131. doi:10.1093/annonc/mdp152
Dusingize, J. C., Olsen, C. M., An, J., Pandeya, N., Law, M. H., Thompson, B. S., et al. (2020). Body mass index and height and risk of cutaneous melanoma: mendelian randomization analyses. Int. J. Epidemiol. 49 (4), 1236–1245. doi:10.1093/ije/dyaa009
Feng, R., Lu, M., Xu, J., Zhang, F., Yang, M., Luo, P., et al. (2022). Pulmonary embolism and 529 human blood metabolites: genetic correlation and two-sample Mendelian randomization study. BMC Genom Data 23 (1), 69. doi:10.1186/s12863-022-01082-6
Gershenwald, J. E., and Guy, G. P. (2016). Stemming the rising incidence of melanoma: calling prevention to action. J. Natl. Cancer Inst. 108 (1), djv381. doi:10.1093/jnci/djv381
Guo, J., Qin, S., Liang, J., Lin, T., Si, L., Chen, X., et al. (2016). Chinese guidelines on the diagnosis and treatment of melanoma (2015 edition). Chin. Clin. Oncol. 5 (4), 57. doi:10.21037/cco.2015.12.02
Harding, J. L., Shaw, J. E., Peeters, A., Cartensen, B., and Magliano, D. J. (2015). Cancer risk among people with type 1 and type 2 diabetes: disentangling true associations, detection bias, and reverse causation. Diabetes Care 38 (2), 264–270. doi:10.2337/dc14-1996
Hong, S. K., Lee, S. T., Kim, S. S., Min, K. E., Byun, S. S., Cho, S. Y., et al. (2009). Significance of preoperative HbA1c level in patients with diabetes mellitus and clinically localized prostate cancer. Prostate 69 (8), 820–826. doi:10.1002/pros.20932
Hope, C., Robertshaw, A., Cheung, K. L., Idris, I., and English, E. (2016). Relationship between HbA1c and cancer in people with or without diabetes: a systematic review. Diabet. Med. 33 (8), 1013–1025. doi:10.1111/dme.13031
Kamiza, A. B., Fatumo, S., Singini, M. G., Yeh, C. C., and Chikowore, T. (2022). Hepatitis B infection is causally associated with extrahepatic cancers: a Mendelian randomization study. EBioMedicine 79, 104003. doi:10.1016/j.ebiom.2022.104003
Kim, S. Y., Eun, C. S., Han, D. S., Kim, Y. S., Song, K. S., Choi, B. Y., et al. (2022). A high glycemic index and glycemic load increased the risk of gastric cancer: a case-control study in Korea. Nutr. Res. 105, 11–19. doi:10.1016/j.nutres.2022.06.001
Larsson, S. C., and Burgess, S. (2021). Causal role of high body mass index in multiple chronic diseases: a systematic review and meta-analysis of Mendelian randomization studies. BMC Med. 19 (1), 320. doi:10.1186/s12916-021-02188-x
Leitner, J. W., Kline, T., Carel, K., Goalstone, M., and Draznin, B. (1997). Hyperinsulinemia potentiates activation of p21Ras by growth factors. Endocrinology 138 (5), 2211–2214. doi:10.1210/endo.138.5.5240
Long, T., Liu, K., Long, J., Li, J., and Cheng, L. (2022). Dietary glycemic index, glycemic load and cancer risk: a meta-analysis of prospective cohort studies. Eur. J. Nutr. 61 (4), 2115–2127. doi:10.1007/s00394-022-02797-z
Lu, Y., García Rodríguez, L. A., Malgerud, L., González-Pérez, A., Martín-Pérez, M., Lagergren, J., et al. (2015). New-onset type 2 diabetes, elevated HbA1c, anti-diabetic medications, and risk of pancreatic cancer. Br. J. Cancer 113 (11), 1607–1614. doi:10.1038/bjc.2015.353
Mackiewicz-Wysocka, M., Araszkiewicz, A., Schlaffke, J., Kuczynski, S., Micek, I., and Zozulinska-Ziolkiewicz, D. (2014). Lower melanin content in the skin of type 1 diabetic patients and the risk of microangiopathy. Exp. Clin. Endocrinol. Diabetes 122 (4), 231–235. doi:10.1055/s-0034-1367003
Mahajan, A., Taliun, D., Thurner, M., Robertson, N. R., Torres, J. M., Rayner, N. W., et al. (2018). Fine-mapping type 2 diabetes loci to single-variant resolution using high-density imputation and islet-specific epigenome maps. Nat. Genet. 50 (11), 1505–1513. doi:10.1038/s41588-018-0241-6
Malavolti, M., Malagoli, C., Crespi, C. M., Brighenti, F., Agnoli, C., Sieri, S., et al. (2017). Glycaemic index, glycaemic load and risk of cutaneous melanoma in a population-based, case-control study. Br. J. Nutr. 117 (3), 432–438. doi:10.1017/S000711451700006X
Marzagalli, M., Casati, L., Moretti, R. M., Montagnani Marelli, M., and Limonta, P. (2015). Estrogen receptor β agonists differentially affect the growth of human melanoma cell lines. PLoS One 10 (7), e0134396. doi:10.1371/journal.pone.0134396
Murphy, N., Song, M., Papadimitriou, N., Carreras-Torres, R., Langenberg, C., Martin, R. M., et al. (2022a). Associations between glycemic traits and colorectal cancer: a mendelian randomization analysis. J. Natl. Cancer Inst. 114 (5), 740–752. doi:10.1093/jnci/djac011
Murphy, N., Song, M., Papadimitriou, N., Carreras-Torres, R., Langenberg, C., Martin, R. M., et al. (2022b). Associations between glycemic traits and colorectal cancer: a mendelian randomization analysis. J. Natl. Cancer Inst. 114 (5), 740–752. doi:10.1093/jnci/djac011
O'Neill, P. A., Butt, M., Eswar, C. V., Gillis, P., and Marshall, E. (2006). A prospective single arm phase II study of dacarbazine and treosulfan as first-line therapy in metastatic uveal melanoma. Melanoma Res. 16 (3), 245–248. doi:10.1097/01.cmr.0000205017.38859.07
Qi, L., Qi, X., Xiong, H., Liu, Q., Li, J., Zhang, Y., et al. (2014). Type 2 diabetes mellitus and risk of malignant melanoma: a systematic review and meta-analysis of cohort studies. Iran. J. Public Health 43 (7), 857–866.
Rastrelli, M., Tropea, S., Rossi, C. R., and Alaibac, M. (2014). Melanoma: epidemiology, risk factors, pathogenesis, diagnosis and classification. Vivo 28 (6), 1005–1011.
Schneider, G., Kirschner, M. A., Berkowitz, R., and Ertel, N. H. (1979). Increased estrogen production in obese men. J. Clin. Endocrinol. Metab. 48 (4), 633–638. doi:10.1210/jcem-48-4-633
Stattin, P., Björ, O., Ferrari, P., Lukanova, A., Lenner, P., Lindahl, B., et al. (2007). Prospective study of hyperglycemia and cancer risk. Diabetes Care 30 (3), 561–567. doi:10.2337/dc06-0922
Ugurel, S., and Gutzmer, R. (2023). Melanom. J. Dtsch. Dermatol Ges. 21 (4), 343–347. doi:10.1111/ddg.15053
Upadhyay, P. R., Starner, R. J., Swope, V. B., Wakamatsu, K., Ito, S., and Abdel-Malek, Z. A. (2022). Differential induction of reactive oxygen species and expression of antioxidant enzymes in human melanocytes correlate with melanin content: implications on the response to solar UV and melanoma susceptibility. Antioxidants (Basel) 11 (6), 1204. doi:10.3390/antiox11061204
Whiteman, D. C., Green, A. C., and Olsen, C. M. (2016). The growing burden of invasive melanoma: projections of incidence rates and numbers of new cases in six susceptible populations through 2031. J. Invest. Dermatol 136 (6), 1161–1171. doi:10.1016/j.jid.2016.01.035
Yoo, T. K., Lee, M. Y., Lee, S. A., Cheong, E. S., Seo, M. H., and Sung, K. C. (2022). Association of glycosylated hemoglobin level and cancer-related mortality in patients without diabetes. J. Clin. Med. 11 (19), 5933. doi:10.3390/jcm11195933
Yuan, S., Kar, S., Carter, P., Vithayathil, M., Mason, A. M., Burgess, S., et al. (2020). Is type 2 diabetes causally associated with cancer risk? Evidence from a two-sample mendelian randomization study. Diabetes 69 (7), 1588–1596. doi:10.2337/db20-0084
Keywords: two-sample mendelian randomization, glycemic traits, melanoma, genome-wide association study, causality
Citation: Zhang Y-C, Lu C-D, Li Q-Y, Shi J-N, Shi J and Yang M (2023) Association between glycemic traits and melanoma: a mendelian randomization analysis. Front. Genet. 14:1260367. doi: 10.3389/fgene.2023.1260367
Received: 17 July 2023; Accepted: 05 December 2023;
Published: 20 December 2023.
Edited by:
Xuanye Cao, University of Texas MD Anderson Cancer Center, United StatesReviewed by:
Ziheng Wang, University of Macau, ChinaCopyright © 2023 Zhang, Lu, Li, Shi, Shi and Yang. This is an open-access article distributed under the terms of the Creative Commons Attribution License (CC BY). The use, distribution or reproduction in other forums is permitted, provided the original author(s) and the copyright owner(s) are credited and that the original publication in this journal is cited, in accordance with accepted academic practice. No use, distribution or reproduction is permitted which does not comply with these terms.
*Correspondence: Min Yang, MjAyMDQwNDFAemNtdS5lZHUuY24=; Jun Shi, c2hpanVuZG9jdG9yQDE2My5jb20=
Disclaimer: All claims expressed in this article are solely those of the authors and do not necessarily represent those of their affiliated organizations, or those of the publisher, the editors and the reviewers. Any product that may be evaluated in this article or claim that may be made by its manufacturer is not guaranteed or endorsed by the publisher.
Research integrity at Frontiers
Learn more about the work of our research integrity team to safeguard the quality of each article we publish.