- 1Division of Agricultural Bioinformatics, ICAR-Indian Agricultural Statistics Research Institute, New Delhi, India
- 2Graduate School, ICAR-Indian Agricultural Research Institute, New Delhi, India
Cyprinus carpio is regarded as a substitute vertebrate fish model for zebrafish. A varied category of non-coding RNAs is comprised of long non-coding RNAs (lncRNAs) and circular RNAs (circRNAs). These ncRNAs were once considered non-functional “junk DNA” but research now shows they play important roles in gene expression regulation, chromatin modification, and epigenetic regulation. The systemic tissue-specific research of the lncRNAs and circRNAs of C. carpio is yet unexplored. A total of 468 raw RNA-Seq dataset across 28 distinct tissues from different varieties of common carp retrieved from public domain were pre-processing, mapped and assembled for lncRNA identification/ classification using various bioinformatics tools. A total of 33,990 lncRNAs were identified along with revelation of 9 miRNAs having 19 unique lncRNAs acting as their precursors. Additionally, 2,837 miRNAs were found to target 4,782 distinct lncRNAs in the lncRNA-miRNA-mRNA interaction network analysis, which resulted in the involvement of 3,718 mRNAs in common carp. A total of 22,854 circRNAs were identified tissue-wise across all the 28 tissues. Moreover, the examination of the circRNA-miRNA-mRNA interaction network revealed that 15,731 circRNAs were targeted by 5,906 distinct miRNAs, which in turn targeted 4,524 mRNAs in common carp. Significant signaling pathways like necroptosis, NOD-like receptor signaling pathway, hypertrophic cardiomyopathy, small cell lung cancer, MAPK signaling pathway, etc. were identified using Gene Ontology and Kyoto Encyclopedia of Genes and Genomes. The web resource of common carp ncRNAs, named CCncRNAdb and available at http://backlin.cabgrid.res.in/ccncrnadb/ gives a comprehensive information about common carp lncRNAs, circRNAs, and ceRNAs interactions, which can aid in investigating their functional roles for its management.
1 Introduction
The deluge of next-generation sequencing data due to advanced high-throughput technology has facilitated the genome-wide identification of RNAs in various species, leading to the discovery of multiple non-coding RNA (ncRNAs) genes (Rinn and Chang, 2012; Kung et al., 2013). The ncRNAs are heterogeneous sets of RNA molecules that do not undergo protein translation (Alexander et al., 2010). Long non-coding RNAs (lncRNAs) are a class of ncRNA molecules with lengths >200 nucleotides (nts) that lack an identifiable open reading frame (ORF) and a conserved codon suggests that there is no possibility for protein-coding (Novikova et al., 2013). Initially lncRNAs were believed to be an insignificant by-product, produced during gene transcription by RNA polymerase II, with no biological purpose other than generating “noise genes”. However, a study has revealed their involvement in regulating mammalian X chromosome inactivation, leading to further exploration of non-coding RNAs (Brown et al., 1992; Lee et al., 1993).
Global gene expression data from various mammalian species, reveals that <2% of the genome comprises protein-coding sequences, while the remaining is transcribed into non-coding RNAs (Clark et al., 2011; Djebali et al., 2012; Berthelot et al., 2014). In recent years, there has been a surge in research interest in lncRNAs due to their potential involvement in regulating various biological processes through transcriptional or post-transcriptional regulatory mechanisms (Batista and Chang, 2013). LncRNA has categorized into three main types, namely, intergenic, intronic, and exonic. Intergenic lncRNAs originate from the intergenic regions, while intronic lncRNAs are solely derived from introns, and exonic lncRNAs are derived from exons of protein-coding genes (Mercer et al., 2009). The identification and annotation of lncRNA sequences are challenging due to their lower expression levels and less conserved nature, setting them apart from small non-coding RNAs and posing computational difficulties (Derrien et al., 2012). LncRNAs are recognized as significant gene regulators owing to their roles as decoys, scaffolds, or guides. By blocking regulatory proteins’ access to DNA, these lncRNAs influence the transcription of protein-coding genes (Kino et al., 2010; Hung et al., 2011). In addition to extensive studies conducted in humans, other mammals, and plants, various lncRNAs have also been identified in fish species including zebrafish (Pauli et al., 2012; Chen et al., 2018), coho salmon (Leiva et al., 2020), rainbow trout (Al-Tobasei et al., 2016), large yellow croaker (Jiang et al., 2016; Zhang et al., 2020), tilapia (Li et al., 2018), common carp (Song et al., 2019; Wang et al., 2021; Hu et al., 2023), koi carp (Luo et al., 2019; Yang et al., 2022), black carp (Zhang et al., 2022), bighead carp (Fu et al., 2019), grass carp (Gan et al., 2020), and amur carp (Zhao et al., 2021).
Besides the linear lncRNAs, a significant group of non-linear ncRNAs known as circRNAs (circular RNA) has arisen. CircRNAs are variants of transcripts that arise from unconventional splicing, whereby the RNA is circularized through the formation of covalent bonds between the 5′ donor end and 3′ acceptor junctions through back splicing (Salzman et al., 2012). In the 1970s, plant viroid and hepatitis delta virus were the first to be recognized as containing circRNA (Sanger et al., 1976; Kos et al., 1986). CircRNAs can control the expression of their linear counterparts by limiting the pre-mRNA available for traditional splicing, operating at a functional level (Salzman et al., 2012). The circRNA-related research has been carried out in zebrafish (Shen et al., 2017; Sharma et al., 2019; Ranjan et al., 2021), rainbow trout (Wu et al., 2022), tilapia (Fan et al., 2019), Japanese flounder (Ning and Sun, 2021b), golden pompano (Sun et al., 2023), and large yellow croaker (Xu et al., 2017).
As per SDG14, conservation and the sustainable use of ocean-based resources should be prioritized. SDG14 aims to conserve and sustainably use the oceans, seas and marine resources for sustainable development (https://sdgs.un.org/goals/goal14). The issue of overfishing can be controlled by improving productivity. In such approach, role of lncRNA-miRNA-mRNA axis has immense impact on aquaculture productivity, fish health, and quality (Zhou et al., 2023). Besides this, lncRNA has key role in immunity of fish which is directly linked with productivity (Haridevamuthu et al., 2023). Aquaculture is currently considered essential in ensuring food security and maintaining economic stability, and it is the most rapidly expanding farmed food industry on a global scale due to the depletion of natural fish populations (Jennings et al., 2016). Common carp (Cyprinus carpio) is a highly significant edible fish species that exist in over a hundred strains and forms worldwide (Balon, 1995; Teletchea, 2015). The freshwater portions of rivers in northern India, Bangladesh, Pakistan, and Burma are the natural habitat of Indian major carp, which are predominantly raised in those countries (Jhingran and Pullin, 1985). Even though a research provides information on the genome and genetic variety of C. carpio (Xu et al., 2014), but genome-wide or tissue-specific ncRNAs and interaction studies with miRNAs and mRNAs are still warranted. Here, tissue-specific means separate analysis were done for each of the 28 tissues to identify the lncRNAs and circRNAs. In this study, we aim at identification and characterization of lncRNAs and circRNAs in the common carp genome, collected from 28 tissues, establishing ceRNAs network involving lncRNA/circRNA-miRNA-mRNA, functional roles of genes and development of the first web-based database of common carp non-coding RNA database, CCncRNAdb.
2 Materials and methods
2.1 Data collection
For the study, a total of 468 raw RNA-Seq datasets were obtained from the National Center for Biotechnology Information (NCBI) database (https://www.ncbi.nlm.nih.gov/) for common carp, covering >9.7 billion transcript reads. The dataset encompassed 42 bioprojects from 23 institutions across 7 countries. These included data across 28 distinct tissues from different varieties of common carp (viz. koi, haematopterus, specularis, color, huanghe, wuyuanesis, singuonensis, jian), the details of which are provided in Supplementary Table S1. All the bioinformatics analysis were tissue-specific, i.e., performed separately for each of the 28 tissues.
2.2 Data quality analysis, mapping, and transcriptome assembly
The raw reads obtained from the NCBI were first visually assessed for the quality using FastQC tool ver. 0.11.8 (Schmieder and Edwards, 2011) followed by elimination of the adaptor sequences and low-quality reads using Trimmomatic software ver. 0.39 (Bolger et al., 2014). To ensure non-interference of polyA tail, reads were trimmed off with primer, followed by automatic transcriptome assembly. Moreover, lncRNAs are identified based on alignment with reference sequence which further ensures exclusion of such tails as they are not genetically coded. They are added post-transcriptionally for protection/shelf life regulating translational efficiency. Common carp reference genome and annotation files were downloaded from NCBI (https://www.ncbi.nlm.nih.gov/assembly/GCF_000951615.1/) (Xu et al., 2014). Index files of the reference genome were generated using the HISAT2-build function of HISAT2 version 2.2.0 (Kim et al., 2015; Pertea et al., 2016). Sam files were aligned and converted to binary bam files using Samtools software version 1.9 (Li et al., 2009). StringTie software version 2.1.4 was used for transcriptome assembly of the individual bam files and generate Gene Transfer Format (gtf) files for each transcriptome reads (Pertea et al., 2015; Pertea et al., 2016). Finally, the StringTie-merge feature was used to combine tissue-specific files and generate a single gtf file per tissue (Banerjee et al., 2021).
2.3 Identification of lncRNAs in common carp
To identify potential lncRNA transcripts, the FASTA sequences that corresponded to every transcript within the combined assembly file were obtained by the gffread program version 0.12.3 using the respective reference genome (Pertea and Pertea, 2020). Owing to the longer size of lncRNAs, transcripts <200 base pairs were removed using perl scripts. ORFs were predicted using ORFPredictor, and those exceeding 300 nucleotides were eliminated (Min et al., 2005; Wang et al., 2021). The coding potential of the transcripts were assessed using CPC2 ver 1.0.1 (Kang et al., 2017), and PLEK ver 1.2 (Li et al., 2014), eliminating the coding RNAs. Non-coding RNAs were discovered through a BlastN search against RNACentral (https://rnacentral.org/) and transcripts with at least 95% identity were excluded (Shumayla et al., 2017). The remaining transcripts might contain small classes of non-coding RNAs, such as mRNA, tRNA, rRNA, miRNA, and snRNA. These underwent Blastp search (Mount, 2007) against the Pfam (http://pfam.xfam.org/) database (Altschul et al., 1990) and non-redundant database (https://www.ncbi.nlm.nih.gov/protein/) to eliminate recognized protein-coding RNAs according to the C. carpio annotation. The remaining transcripts were considered to be the potential lncRNAs for further analysis. The FPKM values for these transcripts were also calculated. The pipeline for identification of lncRNA in C. carpio is delineated in Figure 1.
2.4 Classification of lncRNAs
The gffcompare software was used to categorize lncRNAs into different groups based on their position relative to protein-coding genes, namely, lincRNA (intergenic lncRNAs), labeled as “u”; exonic lncRNAs (intersecting with protein-coding exons), labeled as “x”; and intronic lncRNAs (existing in introns without sharing sequences with exons), labeled as “i” (Leiva et al., 2020). Further, the protein-coding genes were eliminated through an evaluation and elimination process.
2.5 Conservation analysis of lncRNAs
Compared to protein-coding mRNAs in different species, lncRNAs have lower conservation (Derrien et al., 2012). The conservation of lncRNAs in C. carpio with zebrafish, rainbow trout, and large yellow croaker was assessed using BLAST, with a cutoff E-value < 1e−6 (Zhang et al., 2022). Zebrafish data were obtained from the ZFLNC database (https://www.biochen.org/zflnc/) (Hu et al., 2018), while information on rainbow trout and large yellow croaker came from previous lncRNA profiling studies (Al-Tobasei et al., 2016; Zhang et al., 2020).
2.6 Characterization and functional annotation of lncRNAs and circRNAs
2.6.1 Interaction between lncRNAs and miRNAs
The relationship between lncRNAs and miRNAs is complex and intricate. Previous studies have suggested that lncRNAs might enhance pri-miRNA processing or serve as precursors for miRNAs (Augoff et al., 2012; Jiang et al., 2017). Through endonuclease activity, pre-miRNAs are cleaved to produce mature miRNAs, typically 18–24 nucleotides long. To determine if C. carpio lncRNAs act as miRNA precursors, the identified lncRNA sequences were aligned with miRNAs from the miRBase database using Blastn, aiming to identify any known miRNA precursors. The Vienna RNA package within the RNAfold program was utilized to predict the secondary structures of lncRNA transcripts (Leiva et al., 2020).
2.6.2 Construction of lncRNA-miRNA-mRNA interaction network
The microRNA target prediction tool psRNAtarget (V2, 2017 release) (http://plantgrn.noble.org/psRNATarget/) (Dai and Zhao, 2011) was used to carry out an analysis of the interactions between lncRNA-miRNA and miRNA-mRNA (Zhou et al., 2021; Khan et al., 2022). Identified common carp lncRNAs corresponding miRNA were taken from previous studies (Wang et al., 2017a) while the mRNAs data were retrieved from NCBI database. The parameters considered were the expectation ≤2, disallowance of bulges/gaps, and a maximum unpairing energy (Max UPE) up to 25. The ceRNAs (lncRNA-miRNA-mRNA) interaction network was then constructed and visualized by combining the lncRNA-miRNA network and the miRNA-mRNA network using Cytoscape (Shannon et al., 2003).
2.6.3 Identification of circular RNAs (circRNAs) in common carp genome
For identification of circular RNAs, CIRI2 circRNA identification pipeline was used. Raw reads from the NCBI database were evaluated using FastQC, followed by Trimmomatic to remove contaminants and low-quality reads. BWA software version 0.7.17 was used to align clean reads to the common carp reference genome. An index was created using the BWA -index module before alignment, which was done using BWA mem -T 19 (Li, 2013). SAM files of each tissue were merged using Samtools version 1.14 (Li et al., 2009). The merged SAM files were used for circRNA identification in common carp using CIRI2 tool (version 2.0) with default parameters (Gao et al., 2018) (Figure 2).
2.6.4 Construction of ceRNAs interaction (circRNA-miRNA-mRNA) network
A ceRNAs network was constructed based on the ceRNAs theory, specifically the circRNA-miRNA-mRNA network, in order to gain insight into the interactions among circRNAs, miRNAs, and mRNAs. The miRNA dataset for common carp was obtained from literature (Wang et al., 2017b), while the mRNA data was acquired from the NCBI database. TargetFinder was used to identify circRNA targets of miRNAs (Fahlgren and Carrington, 2010). MRNA targets of identified miRNAs were determined by submitting miRNA sequences and common carp mRNA sequences to the psRNAtarget webserver.
2.6.5 GO and KEGG analysis
GO and KEGG analyses were performed using annotation results for common carp. However, directly usable GO and KEGG data for carp is currently unavailable (Wang et al., 2021). To understand the roles of host genes for identified lncRNAs and circRNAs, parent genes underwent GO and KEGG enrichment analyses. Gene IDs and total gene count were extracted and functionally annotated using Blast2GO (Conesa et al., 2005), which served as the background for GO and KEGG enrichment analyses using http://geneontology.org/ and http://www.genome.jp/kegg/, respectively (Xu et al., 2017).
2.6.6 Web resource for ncRNAs of C. carpio
A web resource in the form of common carp non-coding RNA database (CCncRNAdb) was developed for significant impacts of research in this field by providing a valuable resource, promoting collaboration, and enhancing data accessibility. This is based on three-tier architecture viz., client, middle, and database tiers. The database tier utilizes MySQL for storage information related to tissue-specific lncRNAs, circRNAs, their interactions with miRNAs and mRNAs, and miRNA-mRNA interactions for both lncRNAs and circRNAs. The web interface was designed using PHP and HTML, and enhanced with CSS and JavaScript to make it dynamic. The database was hosted on an Apache server and XAMPP was used for webpage design and deployment. Data retrieval involves user requests, MySQL queries, database responses, and server-user communication.
3 Results
3.1 Overview of RNA-seq data, reads mapping and transcriptome assembly results
A total of 9,775,580,802 raw reads generated by Illumina HiSeq platform from 28 different tissues was collected from 468 RNA-seq datasets from the NCBI SRA database. After discarding adaptor sequences and low-quality reads, 9,385,180,448 clean reads (94.88%) were obtained. Approximately 80% of the clean reads from the 468 samples were aligned to the reference genome of the common carp using HISAT2 (Supplementary Table S2). A total of 268,353 transcripts for tissue-specific lncRNAs were generated using StringTie-merge module.
3.2 Genome-wide identification of lncRNAs of C. carpio
On comparing the four GTF files to the existing C. carpio annotation file using GffCompare, allowing transcript annotation based on genomic location relative to known genes, 300,218 transcripts were found to lie in the u (unknown intergenic), x (genic antisense) and i (intronic) classes (Figure 3A). A total of 33,990 putative lncRNAs were discovered in C. carpio after applying filtering criteria, namely, eliminating nucleotide sequences <200 nucleotides, ORF length >300 nucleotides, CPC2 score >0.5, housekeeping RNAs with >95% identity and transcripts similar to protein families or genes. Majority of the sequences showed GC% between 20 and 40 (Figure 3B), while the most abundant sequence length was 200–400 bp, followed by 400–600 bp (Figure 3C). Almost 56.13% of sequences had one exon, followed by 40.36% having two exons (Figure 3D). The distribution of these lncRNAs across different tissues shows kidney tissues to exhibit the highest number of identified lncRNAs, i.e., 8,003 (23.54%) (Figure 3E). We found three lncRNAs, TCONS_00121934, TCONS_00177318, and TCONS_00328247, abundantly expressed in all 28 tissue types we studied. Figure 3E shows the chromosome-wise distribution of lncRNAs in common carp. The calculate FPKM value across all 28 tissues and distinct levels of expression were discerned, as revealed by the comprehensive analysis of the bioprojects. The study unveiled a diverse range of average expression values for lncRNAs across multiple tissues as provided in Supplementary Table S3.
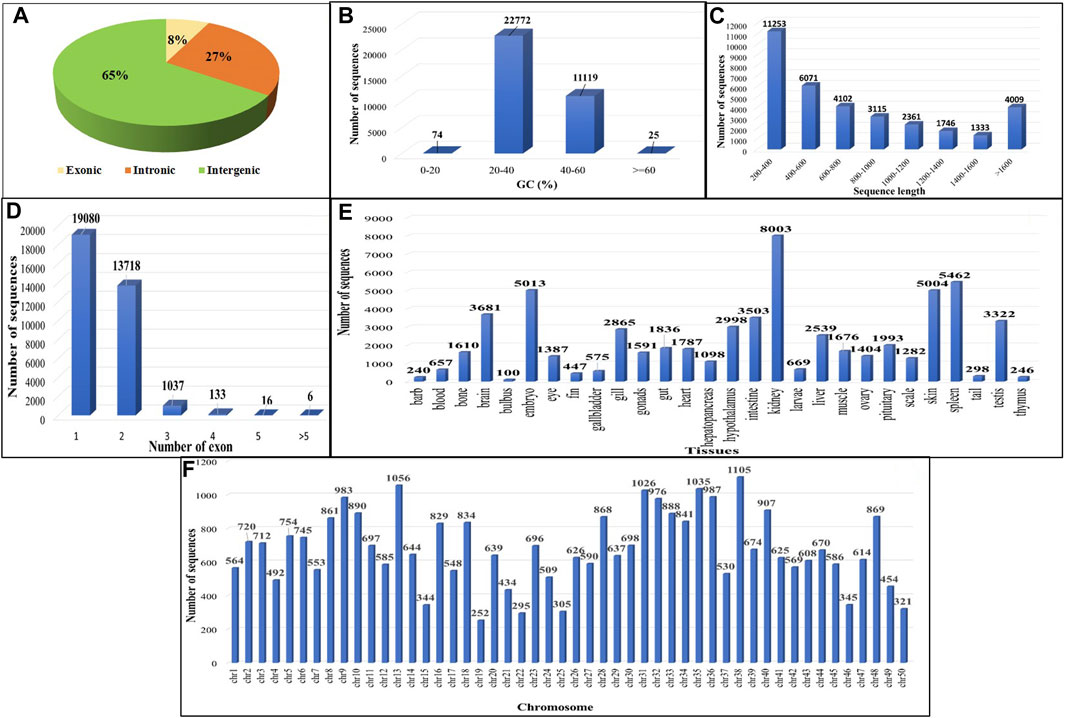
FIGURE 3. Features of common carp lncRNA (A) Distribution of lncRNA subtypes (B) Common carp lncRNAs GC content distribution (C) Distribution of common carp lncRNA length (D) Distribution of common carp lncRNA exon number (E) Tissue wise distribution of common carp lncRNAs (F) Common carp lncRNA distribution in chromosomes.
3.3 Classification and conservation of identified lncRNAs in C. carpio
Out of the total 33,990 lncRNAs, the majority (65.30%) belonged to intergenic class of lncRNAs (u), followed by intronic lncRNAs (i) and exonic lncRNAs (x) which were 27.28% and 7.39%, respectively (Figure 3A). These findings suggest that the majority of lncRNAs (i.e., intergenic or lincRNAs), do not overlap with protein-coding genes. To investigate the conservation of lncRNAs among various species, these putative lncRNAs discovered in C. carpio were compared to those of zebrafish, rainbow trout, and large yellow croaker through a blast analysis. The results showed that only a small number of lncRNAs, i.e., 3,484 (10.25%) in zebrafish (cyprinid), 138 (0.4%) in rainbow trout (salmonid), and 43 (0.12%) in large yellow croaker (sciaenidae) were conserved (Supplementary Table S4). This low level of similarity may be due to poor conservation of lncRNAs across species and tissues.
3.4 Characterization of common carp lncRNAs
It was observed from the analysis that the most common carp lncRNAs had a GC content ranging from 0% to 66.4%, but the majority (99.70%) were within 20%–60%, with an average GC content of 37.88% (Figure 3B). The length of lncRNAs in common carp varied from 200 to 15,799 nucleotides (Figure 3C), with 72.17% being between 0 and 999 nucleotides and 27.82% between 1,000 and 1,999 nucleotides. The average length of the lncRNAs was found to be 837 nucleotides, which is shorter than the length of protein-coding genes (2.914 kb). Common carp lncRNAs had 1–7 exons on average (1.48 exons). The majority (96.49%) were single or double exon types, with an average length of 849.82 nucleotides (Figure 3D). The chromosomal distribution of the identified lncRNA in common carp is shown in (Figure 3F), with chromosome 38 having the highest count of 1,105 lncRNAs and chromosome 19 having the lowest count of 252. This distribution was visualized using Circos software (Figure 4).
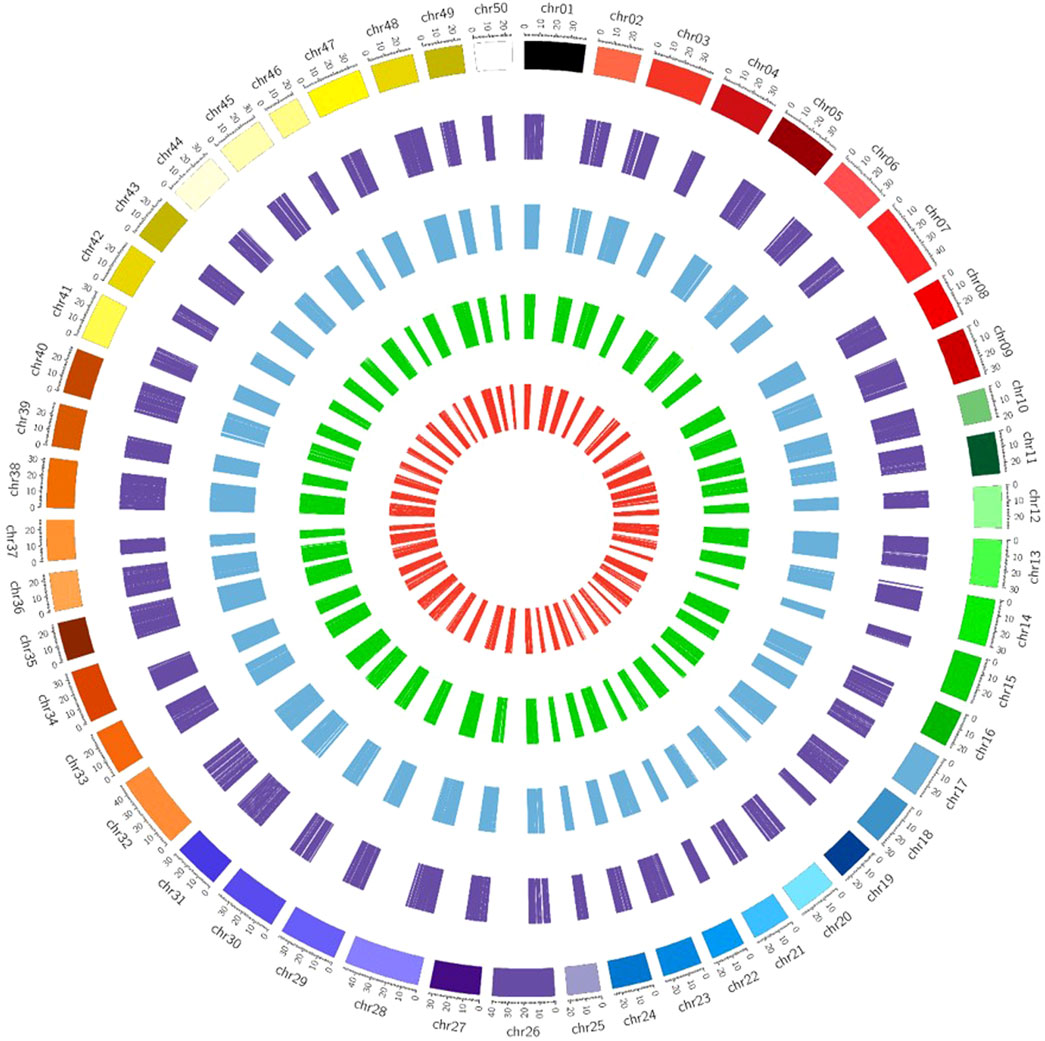
FIGURE 4. Chromosome-wise distribution of lncRNAs and circRNAs of common carp. The visual representation consists of different circles, with the outermost circle representing circRNAs (dark purple color), intergenic lncRNAs in blue color, intronic lncRNAs in dark green color, and exonic lncRNAs in the inner circle (red color).
3.5 lncRNAs mediating miRNA function
The identified lncRNAs were analyzed for their potential target genes based on their proximity to protein-coding genes. Additionally, the sequences of the lncRNAs were compared to miRNAs from miRBase using the BlastN program to determine if they function as miRNA precursors. Results showed that 9 pre-miRNAs matched with 19 distinct lncRNAs in common carp, indicating the potential for these lncRNAs to produce mature miRNAs at matching accuracy cut-off of ≥90% (Supplementary Table S5). Few lncRNAs were discovered to have a stable hairpin structure, indicating the presence of a miRNA precursor. In our study, TCONS_00417363 lncRNA (dark green), which contained the miRNA precursor ami-mir-133a-2 (red) was identified by Vienna RNA package and RNAfold program (Supplementary Figure S1A).
3.6 Analysis of lncRNA-miRNA-mRNA interaction network
LncRNAs play a crucial role in regulating gene expression by acting as competitive endogenous RNAs (ceRNAs), which capture miRNAs and prevent them from binding to their target mRNAs. This interaction between lncRNAs and miRNAs significantly affects gene expression and various biological processes. The miRNAs also regulate gene expression by binding to the 3′ untranslated region (UTR) of target mRNAs, leading to mRNA degradation or translational repression. These miRNA-mediated gene expression regulations are essential for the normal development and function of fish organs and tissues. Using the psRNAtarget server, 18,977 interactions (lncRNA-miRNA) involving 2,837 distinct miRNAs and 4,782 distinct lncRNAs in common carp were identified. Using the RNAfold program with the Vienna RNA package, a visualization of the secondary structure of lncRNA TCONS_00239471 (displayed in dark green), along with the locations of its binding sites for miRNAs ccr-miR-338 (depicted in red) and mir-338-y (depicted in blue) were generated (Supplementary Figure S1B). In the case of miRNA-mRNA, a total of 8,079 interactions, which involved 1,900 unique miRNAs and 3,718 unique mRNAs were revealed. The Cytoscape software was utilized to construct individual lncRNA-miRNA and miRNA-mRNA interaction networks after that merged both the network in a single network and visualizing the whole lncRNA-miRNA-mRNA interaction network. From this interaction analysis, we observe that mir-8499-y target 2 lncRNAs of TCONS_00413548, TCONS_00007855 and six mRNAs these are lcl|LHQP01046800.1_mrna_46126, lcl|LHQP01016561.1_mrna_24063, lcl|LHQP01011828.1_mrna_17740, lcl|LHQP01021498.1_mrna_29844, lcl|LHQP01064132.1_mrna_49370 and lcl|LHQP01015883.1_mrna_23319 (Supplementary Figure S2).
3.7 Identification of circular RNAs in common carp
The identification of circRNAs in common carp involved four main step: i) quality check of 9,775,580,802 raw RNA-seq reads from NCBI ii) final clean reads aligned to the common carp reference genome using BWA-mem -T 19 ii) generation of 468 SAM files for each sample, followed by merging for each of the 28 tissues. iv) Submission of these merged files to the widely used command-line tool, CIRI2. This resulted into 22,854 distinct potential circRNAs (Supplementary Table S6). In this study, we have not only identified tissue-specific common carp circRNAs but have also uncovered striking differences in their abundance across tissues. A total of 11,621 circRNAs (50.84%) were derived from intergenic regions, while only 2,575 (11.26%) were generated by introns (Figure 5A). Out of the identified circRNAs, 8,655 (37.87%) originated from exons of protein-coding genes, indicating they were exonic circRNAs. These exonic circRNAs had both back-splice sites aligned with known exonic boundaries. A visual representation of the length distribution of circRNAs reflected that majority of circRNAs were over 1,600 nucleotides long (Figure 5B). Specifically, embryonic tissue showed the abundance of circRNAs (8635) (Figure 5C). Chromosome 38 was seen to have highest number of circRNA (745) (Figure 5D). The chromosome-wise circos-map of circular RNAs in common carp is summarized in Figure 4 (outermost dark purple circle).
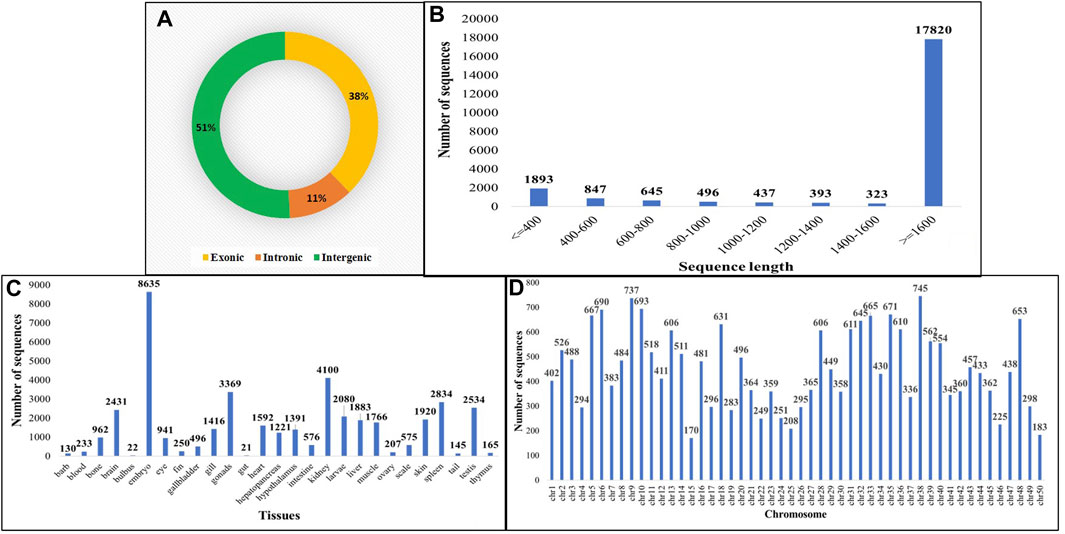
FIGURE 5. Features of common carp circRNA (A) circRNA subtypes distribution (B) Length-wise distribution of common carp circRNA (C) Tissue-wise distribution of common carp circRNAs (D) Chromosome-wise distribution of circRNA.
3.8 Analysis of circRNA-miRNA-mRNA interaction network
CircRNA-miRNA-mRNA interactions have been identified as an important regulatory mechanism in gene expression which is also called the ceRNAs network, where circRNAs act as a sponge for miRNAs, thereby inhibiting the degradation of target mRNAs by miRNAs. By using TargetFinder software we get a total of 970,159 interactions (circRNA-miRNA) where 5,906 unique miRNAs were found targeting 15,731 circRNAs in the tissues under study, except the pituitary tissue. As a result, 10,484 interaction (miRNA-mRNA) was found from the psRNATarget server analysis where 4,524 distinct mRNAs were targeted by 2,871 unique miRNAs of common carp. An entire circRNA–miRNA-mRNA interaction network was delineated by Cytoscape (Supplementary Figure S3). The figures show that miRNA, mir-6627-y targeted a total of seven mRNAs, miRNA mir-6651-x targeted nine circRNAs and two mRNAs, while another miRNA, mir-7371-x targeted a total of 13 circRNAs.
3.9 Functional annotation of common carp lncRNAs and circRNAs
In this study, we utilized GO and KEGG annotations to gain understanding of the functions of lncRNAs/circRNAs, based on the hypothesis that their functions may be linked to those of their parent genes. An analysis of GO categories and KEGG pathways was done on 3,718 host genes of 33,990 lncRNAs and 4,524 host genes of 22,854 circRNAs to investigate their possible roles in common carp. For the lncRNAs in cellular component category, the top three largest groups were the nucleus (7.31%), plasma membrane (5.85%), and membrane (2.62%); for the biological process: anatomical structure development (10.02%), signaling (8.02%), and protein modification process (5.69%) and for molecular function: transferase activity (7.29%), catalytic activity (5.38%), and hydrolase activity (3.78%). The significantly enriched KEGG pathways were mainly necroptosis, NOD-like receptor signaling pathway, hypertrophic cardiomyopathy, small cell lung cancer, MAPK signaling pathway, pathways of neurodegeneration, and axon guidance (Supplementary Tables S7, S8). The analyses of GO and KEGG indicate a strong potential for lncRNAs and circRNAs to play various roles in biological processes within the common carp fish.
3.10 Web resource for common carp lncRNAs and circRNAs
A web-based database, named CCncRNAdb for common carp ncRNAs is accessible at http://backlin.cabgrid.res.in/ccncrnadb/index.php, which contains information on 33,990 lncRNAs and 22,854 circRNAs, including their characterization, and interactions with miRNAs and mRNAs. CCncRNAdb has six tabs, namely, Home, lncRNA, Interactions, circRNA, Download, and Teams (Figure 6). The main features of this database are: CCncRNAdb offers extensive information on tissue-specific lncRNAs and circRNAs, such as their chromosome locations, sequence length, and coding potential. To obtain details regarding the lncRNA/circRNA-miRNA-mRNA interaction network, users can visit the “Interaction” tab. The results can be downloaded directly for all the tissues.
4 Discussion
The research is oriented towards the tissue-wise studies of long non-coding RNAs and circular RNAs in common carp from the retrieved RNA-seq data from NCBI, followed by tissue-specific/tissue-wise analysis. The tissue-specific identification and ceRNAs analysis in common carp ncRNAs is very limited as compared to humans or other fish species (Nielsen et al., 2014; Chen et al., 2018; Zhang et al., 2020). Previous research on common carp is primarily focused on genes, and miRNAs, with very limited developmental stage-specific lncRNAs. However, the rising importance of non-coding RNAs (ncRNAs), including miRNAs, lncRNAs, and circRNAs, relates to their critical roles in gene expression networks, particularly immune regulation and other biological processes. Possible mRNAs and ncRNAs related to immune regulation were used to create lncRNA-miRNA-mRNA and circRNA-miRNA-mRNA interaction networks. Exploring tissue-specific non-coding RNA identification in common carp is crucial, and this study provides the most comprehensive analysis of lncRNAs and circRNAs using RNA-seq data. To the best of our knowledge, this is the first report that methodically distinguishes lncRNAs and circRNAs based on tissue-wise RNA-seq data.
The 33,990 putative lncRNAs and 22,854 circRNAs across 468 RNA-seq datasets in 28 tissues of common carp fish used in this study were categorized into three groups, based on their proximity to protein-coding genes. Similar to the comparative research on other organisms, the identified putative lncRNAs had lower expression levels, shorter transcript lengths, and fewer exons compared to protein-coding genes (Al-Tobasei et al., 2016; Ning and Sun, 2021a; Chen et al., 2021). Kidney tissue was observed to have the most abundant lncRNAs (23.54%), while the least was seen in bulbus tissue (0.29%). Almost 65% of identified lncRNAs were intergenic (lincRNAs) which was in concordance with the other vertebrate species. Our results revealed distinctive features of identified lncRNAs compared to mRNAs, including reduced exon count, shorter transcript lengths, lower GC content, and lower conversion rates which are in consistent with prior findings (Niazi and Valadkhan, 2012; Al-Tobasei et al., 2016; Bakhtiarizadeh and Salami, 2019). The lncRNA expression value (FPKM) specifically tissue-wise, the details of which are provided in Supplementary Table S3.
Our study on miRNA-lncRNA/circRNA interactions in common carp supports the notion of lncRNAs acting as miRNA targets, limiting their mRNA regulation, which is called lncRNA sponge or competing endogenous RNA (Salmena et al., 2011). The results of a study conducted on mice revealed that miR-338-3p can directly target the SRY-box transcription factor 4 (SOX4) in ESCC cells. The study also showed that SNHG17 can act as an endogenous “sponge” by competing with miR-338-3p to regulate SOX4, consequently promoting tumor progression. These findings suggest that targeting these molecular interactions could serve as a potential therapeutic intervention for ESCC. In the same way, our study found that lncRNA TCONS_00239471 is targeted by miRNAs mir-338-y and ccr-miR-338 so its function will be like therapeutic targets for ESCC (Chen et al., 2021)
Multiple studies have provided evidence that lncRNAs can serve as targets for miRNAs, thereby inhibiting the interaction between miRNAs and coding genes (Paneru et al., 2016; Pereiro et al., 2020). In recent times, there has been evidence to support crucial role of lncRNAs in regulating innate antiviral responses in teleost fish, for example, MARL operates as a ceRNAs for miR-122, thereby controlling the quantity of mitochondrial antiviral signaling proteins (MAVS) and impeding the replication of SCRV while stimulating antiviral responses (Anderson et al., 2015). We also found mir-8499-y to target two lncRNAs, i.e., TCONS_00413548, TCONS_00007855, and six mRNAs to mediate the role in regulating a wide range of cellular processes.
The investigation into tissue-specific circRNAs in common carp gave 22,854 circRNAs and GO analysis suggests that lncRNAs/circRNAs play a crucial role in various cellular processes, including transcriptional regulation, signaling pathways, and enzymatic reactions, which could have implications for various biological processes, including development, growth, directly involved in immunity, and disease (Xiu et al., 2021). For identification of circRNAs on common carp, CIRI2 was used owing to its robustness and reliability as compared to other methods, based on numerous literature (Gao et al., 2018; Chen et al., 2022; Kumar et al., 2023; Rbbani et al., 2023). In tilapia fish, ten and eleven circular RNAs were predicted to target miR-221 and miR-222, correspondingly. One of these, Oni_circRNA_002834, has the capability to bind with miR-221, miR-222, and miR-734, which consequently target certain mRNAs. It has been proposed that alterations in these miRNAs, due to bacterial invasion, may modify the expression of immunomodulatory proteins in tilapia’s brain, potentially enhancing the immune response through an alternative mechanism. In the case of rainbow trout’s circRNA-miRNA-mRNA network found that circRNA5279 and circRNA5277 co-expressed with tap2 via competitive binding with oni-mir-124a-2-p5_1ss13GA. Mir124a regulates T cell activation and differentiation and is critical, having a crucial role in rainbow trout’s skin immunity (Htet and Tennyson, 2016; Zhao et al., 2017). Similarly, our study shows that miRNA mir-6627-y targeted a total of 7 mRNAs, miRNA mir-6651-x targeted 9 circRNAs and 2 mRNAs, and another miRNA mir-7371-x targeted a total of 13 circRNAs so the function also is related to immunity. In this study, the enriched KEGG pathways included necroptosis, NOD-like receptor signaling, hypertrophic cardiomyopathy, small cell lung cancer, MAPK signaling, Fc gamma R-mediated phagocytosis, neurodegeneration pathways, axon guidance, cellular senescence, and more. In zebrafish, KEGG analysis showed processes related to viral infections like endocytosis, MAPK signaling, herpes simplex infection, and NOD-like receptor signaling (Valenzuela-Muñoz et al., 2019). This implies that lncRNAs and circRNAs in common carp may be involved in the immune response and protecting the host from pathogens and tissue damage. This fish species holds significant ecological importance; however, there is currently no existing genomic resource for it. Furthermore, specific tissue-wise reports on lncRNAs are lacking. Consequently, our pioneering investigation into tissue-wise lncRNAs in common carp is the inaugural effort in this field. The outcomes of this study are poised to benefit forthcoming research endeavors greatly.
This study provides the information of circular RNA for the very first time in common carp fish. The extensive web-resource on common carp lncRNAs in the form of CCncRNAdb, is freely accessible at http://backlin.cabgrid.res.in/ccncrnadb/index.php which catalogues common carp specific lncRNAs and circRNAs and their interaction studies. This resource will aid in comprehending the fundamental roles that these lncRNAs and circRNAs perform in the growth, development, and response to diseases in common carp. The annotation of the common carp reference genome has been significantly enhanced by the detection of lncRNAs and circRNAs. These putative lncRNAs and circRNAs can aid in improving our comprehension of the biological mechanisms governing regulatory interactions involving mRNA, miRNA, and lncRNA/circRNA.
5 Conclusion
This study involves 468 RNA-seq datasets across 28 tissues of common carp for the identification of tissue-specific lncRNAs and circRNAs, and their interactions with miRNAs and mRNAs. A total of 33,990 lncRNAs and 22,854 circRNAs were recognized and characterized. The analysis of the conservation of the identified lncRNAs confirms that lncRNAs are poorly conserved in nature. This study discovered that 19 distinct lncRNAs serve as precursors for 9 miRNAs, which may help in understanding the complex mechanisms of gene regulation. Through GO and KEGG analyses, tissue-specific lncRNAs/circRNAs revealed multiple signaling pathways including necroptosis, NOD-like receptor signaling, hypertrophic cardiomyopathy, small cell lung cancer, MAPK signaling, etc., these findings enhance our comprehension of common carp fish’s evolution, augmentation, and immune system, shedding light on the role of lncRNAs and circRNAs in immune response and their impact on common carp growth and development. The freely accessible CCncRNAdb will provide information about lncRNAs and circRNAs in common carp establishing a robust platform for further exploration of lncRNA/circRNAs tissue-specific mechanisms and functions in this species for better management.
Data availability statement
The datasets presented in this study can be found in online repositories. The names of the repository/repositories and accession number(s) can be found in the article/Supplementary Material.
Ethics statement
The animal study data was obtained from public domain, details of which are provided in the manuscript, so there is no involvement of ethics committee at our level. The study was conducted in accordance with the local legislation and institutional requirements.
Author contributions
The study was planned and designed by SJ, DK, and MI. JD and BK conducted the data curation and analysis. JD, BS, MI, and UA collaborated on the development of the database. The initial draft of the manuscript was written by JD, SJ, and MI. This was reviewed and edited by DK, UA, MI, and SJ. All authors contributed to the article and approved the submitted version.
Acknowledgments
The authors are thankful to CABin grant, Indian Council of Agricultural Research, Ministry of Agriculture and Farmers’ Welfare, Govt. of India (F. No. Agril. Edn. 4–1/2013-A&P) for providing financial and infrastructural support to carry out this research and for creation of Advanced Super Computing Hub for Omics Knowledge in Agriculture (ASHOKA) facility where the work was carried out. The grant of IARI Merit scholarship to JD is duly acknowledged.
Conflict of interest
The authors declare that the research was conducted in the absence of any commercial or financial relationships that could be construed as a potential conflict of interest.
Publisher’s note
All claims expressed in this article are solely those of the authors and do not necessarily represent those of their affiliated organizations, or those of the publisher, the editors and the reviewers. Any product that may be evaluated in this article, or claim that may be made by its manufacturer, is not guaranteed or endorsed by the publisher.
Supplementary material
The Supplementary Material for this article can be found online at: https://www.frontiersin.org/articles/10.3389/fgene.2023.1239434/full#supplementary-material
Supplementary Figure 1 | Secondary structures of (A) lncRNA TCONS_00417363 (dark green), and precursor form of the miRNA, ami-mir-133a-2 (color in red); (B) lncRNA TCONS_00239471 (dark green) with target locations for two distinct miRNAs, ccr-miR-338 (red) and mir-338-y (blue).
Supplementary Figure 2 | Relationship between ceRNA networks where blue rectangle represent lncRNAs, red diamond represents mRNAs and light green ellipse represent miRNAs.
Supplementary Figure 3 | Relationship between ceRNA networks, where lncRNAs are denoted by blue rectangles, mRNAs by red diamonds, and miRNAs by light green ellipses.
Supplementary Table 1 | Tissue-wise details of the datasets used in the study from different varieties of common carp.
Supplementary Table 2 | Tissue-wise summary of sequence reads and alignment statistics from different varieties of common carp.
Supplementary Table 3 | Tissue-wise identified lncRNAs from common carp and their characteristic information.
Supplementary Table 4 | Conserved lncRNAs among the identified common carp lncRNAs.
Supplementary Table 5 | LncRNAs acting as precursors of miRNAs.
Supplementary Table 6 | Tissue-wise identified circRNAs from common carp.
Supplementary Table 7 | KEGG Pathways associated with common carp lncRNAs.
Supplementary Table 8 | KEGG Pathways associated with common carp circRNAs.
References
Alexander, R. D., Innocente, S. A., Barrass, J. D., and Beggs, J. D. (2010). Splicing-dependent RNA polymerase pausing in yeast. Mol. Cell. 40 (4), 582–593. doi:10.1016/j.molcel.2010.11.005
Al-Tobasei, R., Paneru, B., and Salem, M. (2016). Genome-wide discovery of long non-coding RNAs in rainbow trout. PLoS One 11 (2), e0148940. doi:10.1371/journal.pone.0148940
Altschul, S. F., Gish, W., Miller, W., Myers, E. W., and Lipman, D. J. (1990). Basic local alignment search tool. J. Mol. Biol. 215 (3), 403–410. doi:10.1016/S0022-2836(05)80360-2
Anderson, D. M., Anderson, K. M., Chang, C. L., Makarewich, C. A., Nelson, B. R., McAnally, J. R., et al. (2015). A micropeptide encoded by a putative long noncoding RNA regulates muscle performance. Cell. 160 (4), 595–606. doi:10.1016/j.cell.2015.01.009
Augoff, K., McCue, B., Plow, E. F., and Sossey-Alaoui, K. (2012). miR-31 and its host gene lncRNA LOC554202 are regulated by promoter hypermethylation in triple-negative breast cancer. Mol. cancer 11 (1), 5–13. doi:10.1186/1476-4598-11-5
Bakhtiarizadeh, M. R., and Salami, S. A. (2019). Identification and expression analysis of long noncoding RNAs in fat-tail of sheep breeds. G3 Genes., Genomes, Genet. 9 (4), 1263–1276. doi:10.1534/g3.118.201014
Balon, E. K. (1995). Origin and domestication of the wild carp, Cyprinus carpio: from Roman gourmets to the swimming flowers. Aquaculture 129 (1-4), 3–48. doi:10.1016/0044-8486(94)00227-F
Banerjee, B., Koner, D., Karasik, D., and Saha, N. (2021). Genome-wide identification of novel long non-coding RNAs and their possible roles in hypoxic zebrafish brain. Genomics 113 (1), 29–43. doi:10.1016/j.ygeno.2020.11.023
Batista, P. J., and Chang, H. Y. (2013). Cytotopic localization by long noncoding RNAs. Curr. Opin. Cell. Biol. 25 (2), 195–199. doi:10.1016/j.ceb.2012.12.001
Berthelot, C., Brunet, F., Chalopin, D., Juanchich, A., Bernard, M., Noël, B., et al. (2014). The rainbow trout genome provides novel insights into evolution after whole-genome duplication in vertebrates. Nat. Commun. 5 (1), 3657. doi:10.1038/ncomms4657
Bolger, A. M., Lohse, M., and Usadel, B. (2014). Trimmomatic: a flexible trimmer for Illumina sequence data. Bioinformatics 30 (15), 2114–2120. doi:10.1093/bioinformatics/btu170
Brown, C. J., Hendrich, B. D., Rupert, J. L., Lafreniere, R. G., Xing, Y., Lawrence, J., et al. (1992). The human XIST gene: analysis of a 17 kb inactive X-specific RNA that contains conserved repeats and is highly localized within the nucleus. Cell. 71 (3), 527–542. doi:10.1016/0092-8674(92)90520-M
Chen, S., Cao, X., Zhang, J., Wu, W., Zhang, B., and Zhao, F. (2022). circVAMP3 drives CAPRIN1 phase separation and inhibits hepatocellular carcinoma by suppressing c-myc translation. Adv. Sci. 9 (8), e2103817. doi:10.1002/ADVS.202103817
Chen, W., Zhang, X., Li, J., Huang, S., Xiang, S., Hu, X., et al. (2018). Comprehensive analysis of coding-lncRNA gene co-expression network uncovers conserved functional lncRNAs in zebrafish. Bmc Genomics 19 (2), 112–185. doi:10.1186/s12864-018-4458-7
Chen, Y., Wan, S., Li, Q., Dong, X., Diao, J., Liao, Q., et al. (2021). Genome-wide integrated analysis revealed functions of lncRNA–miRNA–mRNA interaction in growth of intermuscular bones in Megalobrama amblycephala. Front. Cell. Dev. Biol. 8, 603815. doi:10.3389/fcell.2020.603815
Clark, M. B., Amaral, P. P., Schlesinger, F. J., Dinger, M. E., Taft, R. J., Rinn, J. L., et al. (2011). The reality of pervasive transcription. PLoS Biol. 9 (7), e1000625. doi:10.1371/journal.pbio.1000625
Conesa, A., Götz, S., García-Gómez, J. M., Terol, J., Talón, M., and Robles, M. (2005). Blast2GO: a universal tool for annotation, visualization and analysis in functional genomics research. Bioinformatics 21 (18), 3674–3676. doi:10.1093/bioinformatics/bti610
Dai, X., and Zhao, P. X. (2011). psRNATarget: a plant small RNA target analysis server. Nucleic acids Res. 39 (Suppl. l_2), W155–W159. doi:10.1093/nar/gkr319
Derrien, T., Johnson, R., Bussotti, G., Tanzer, A., Djebali, S., Tilgner, H., et al. (2012). The GENCODE v7 catalog of human long noncoding RNAs: analysis of their gene structure, evolution, and expression. Genome Res. 22 (9), 1775–1789. doi:10.1101/gr.132159.111
Djebali, S., Davis, C. A., Merkel, A., Dobin, A., Lassmann, T., Mortazavi, A., et al. (2012). Landscape of transcription in human cells. Nature 489 (7414), 101–108. doi:10.1038/nature11233
Fahlgren, N., and Carrington, J. C. (2010). “miRNA target prediction in plants,” in Plant MicroRNAs. Methods in molecular biology. Editors B. Meyers, and P. Green (New York: Springer), 592. doi:10.1007/978-1-60327-005-2_4
Fan, B., Chen, F., Li, Y., Wang, Z., Wang, Z., Lu, Y., et al. (2019). A comprehensive profile of the tilapia (Oreochromis niloticus) circular RNA and circRNA–miRNA network in the pathogenesis of meningoencephalitis of teleosts. Mol. omics 15 (3), 233–246. doi:10.1039/C9MO00025A
Fu, B., Yu, X., Tong, J., Pang, M., Zhou, Y., Liu, Q., et al. (2019). Comparative transcriptomic analysis of hypothalamus-pituitary-liver axis in bighead carp (Hypophthalmichthys nobilis) with differential growth rate. BMC Genomics 20 (1), 328–8. doi:10.1186/s12864-019-5691-4
Gan, L., Wang, Y., Chen, S., Tang, H., Peng, J., Guo, H., et al. (2020). Identification and characterization of long non-coding RNAs in muscle sclerosis of grass carp, Ctenopharyngodon idellus fed with faba bean meal. Aquaculture 516, 734521. doi:10.1016/J.AQUACULTURE.2019.734521
Gao, Y., Zhang, J., and Zhao, F. (2018). Circular RNA identification based on multiple seed matching. Briefings Bioinforma. 19 (5), 803–810. doi:10.1093/bib/bbx014
Haridevamuthu, B., Guru, A., Velayutham, M., Priya, P. S., Arshad, A., and Arockiaraj, J. (2023). Long non-coding RNA, a supreme post-transcriptional immune regulator of bacterial or virus-driven immune evolution in teleost. Rev. Aquac. 15 (1), 163–178. doi:10.1111/raq.12709
Htet, Y., and Tennyson, A. G. (2016). NAD+ as a hydride donor and reductant. J. Am. Chem. Soc. 138 (49), 15833–15836. doi:10.1021/jacs.6b10451
Hu, S., Chen, L., Bai, Y., He, Q., Liu, Y., and Xu, P. (2023). Epigenetic mechanisms of lncRNA in response to thermal stress during embryogenesis of allotetraploid Cyprinus carpio. Genomics 115 (5), 110698. doi:10.1016/J.YGENO.2023.110698
Hu, X., Chen, W., Li, J., Huang, S., Xu, X., Zhang, X., et al. (2018). ZFLNC: a comprehensive and well-annotated database for zebrafish lncRNA. Database 2018, bay114. doi:10.1093/database/bay114
Hung, T., Wang, Y., Lin, M. F., Koegel, A. K., Kotake, Y., Grant, G. D., et al. (2011). Extensive and coordinated transcription of noncoding RNAs within cell-cycle promoters. Nat. Genet. 43 (7), 621–629. doi:10.1038/ng.848
Jennings, S., Stentiford, G. D., Leocadio, A. M., Jeffery, K. R., Metcalfe, J. D., Katsiadaki, I., et al. (2016). Aquatic food security: insights into challenges and solutions from an analysis of interactions between fisheries, aquaculture, food safety, human health, fish and human welfare, economy and environment. Fish Fish. 17 (4), 893–938. doi:10.1111/faf.12152
Jhingran, V. G., and Pullin, R. S. (1985). A hatchery manual for the common, Chinese, and Indian major carps (No. 252). Manila: Asian Development Bank. Avaliable at: https://pdf.usaid.gov/pdf_docs/pnaav716.pdf.
Jiang, L., Liu, W., Zhu, A., Zhang, J., Zhou, J., and Wu, C. (2016). Transcriptome analysis demonstrate widespread differential expression of long noncoding RNAs involve in Larimichthys crocea immune response. Fish Shellfish Immunol. 51, 1–8. doi:10.1016/j.fsi.2016.02.001
Jiang, L., Shao, C., Wu, Q. J., Chen, G., Zhou, J., Yang, B., et al. (2017). NEAT1 scaffolds RNA-binding proteins and the Microprocessor to globally enhance pri-miRNA processing. Nat. Struct. Mol. Biol. 24 (10), 816–824. doi:10.1038/nsmb.3455
Kang, Y. J., Yang, D. C., Kong, L., Hou, M., Meng, Y. Q., Wei, L., et al. (2017). CPC2: a fast and accurate coding potential calculator based on sequence intrinsic features. Nucleic acids Res. 45 (W1), W12-W16–W16. doi:10.1093/nar/gkx428
Khan, A., Singh, K., Jaiswal, S., Raza, M., Jasrotia, R. S., Kumar, A., et al. (2022). Whole-genome-based web genomic resource for water Buffalo (Bubalus bubalis). Front. Genet. 13, 809741. doi:10.3389/fgene.2022.809741
Kim, D., Langmead, B., and Salzberg, S. L. (2015). HISAT: a fast spliced aligner with low memory requirements. Nat. methods 12 (4), 357–360. doi:10.1038/nmeth.3317
Kino, T., Hurt, D. E., Ichijo, T., Nader, N., and Chrousos, G. P. (2010). Noncoding RNA gas5 is a growth arrest–and starvation-associated repressor of the glucocorticoid receptor. Sci. Signal. 3 (107), ra8. doi:10.1126/scisignal.2000568
Kos, A., Dijkema, R., Arnberg, A. C., Van der Meide, P. H., and Schellekens, H. (1986). The hepatitis delta (delta) virus possesses a circular RNA. Nature 323 (6088), 558–560. doi:10.1038/323558a0
Kumar, B., Saha, B., Jaiswal, S., Angadi, U. B., Rai, A., and Iquebal, M. A. (2023). Genome-wide identification and characterization of tissue-specific non-coding RNAs in black pepper (Piper nigrum L.). Front. Plant Sci. 14, 1079221. doi:10.3389/fpls.2023.1079221
Kung, J. T., Colognori, D., and Lee, J. T. (2013). Long noncoding RNAs: past, present, and future. Genetics 193 (3), 651–669. doi:10.1534/genetics.112.146704
Lee, R. C., Feinbaum, R. L., and Ambros, V. (1993). The C. elegans heterochronic gene lin-4 encodes small RNAs with antisense complementarity to lin-14. Cell. 75 (5), 843–854. doi:10.1016/0092-8674(93)90529-Y
Leiva, F., Rojas-Herrera, M., Reyes, D., Bravo, S., Garcia, K. K., Moya, J., et al. (2020). Identification and characterization of miRNAs and lncRNAs of coho salmon (Oncorhynchus kisutch) in normal immune organs. Genomics 112 (1), 45–54. doi:10.1016/j.ygeno.2019.07.015
Li, A., Zhang, J., and Zhou, Z. (2014). PLEK: a tool for predicting long non-coding RNAs and messenger RNAs based on an improved k-mer scheme. BMC Bioinforma. 15, 311–410. doi:10.1186/1471-2105-15-311
Li, B. J., Jiang, D. L., Meng, Z. N., Zhang, Y., Zhu, Z. X., Lin, H. R., et al. (2018). Genome-wide identification and differentially expression analysis of lncRNAs in tilapia. BMC genomics 19 (1), 729–812. doi:10.1186/s12864-018-5115-x
Li, H. (2013). Aligning sequence reads, clone sequences and assembly contigs with BWA-MEM. arXiv. doi:10.48550/arXiv.1303.3997
Li, H., Handsaker, B., Wysoker, A., Fennell, T., Ruan, J., Homer, N., et al. (2009). The sequence alignment/map format and SAMtools. Bioinformatics 25 (16), 2078–2079. doi:10.1093/bioinformatics/btp352
Luo, M., Wang, L., Yin, H., Zhu, W., Fu, J., and Dong, Z. (2019). Integrated analysis of long non-coding RNA and mRNA expression in different colored skin of koi carp. BMC Genomics 20 (1), 515. doi:10.1186/s12864-019-5894-8
Mercer, T. R., Dinger, M. E., and Mattick, J. S. (2009). Long non-coding RNAs: insights into functions. Nat. Rev. Genet. 10 (3), 155–159. doi:10.1038/nrg2521
Min, X. J., Butler, G., Storms, R., and Tsang, A. (2005). OrfPredictor: predicting protein-coding regions in EST-derived sequences. Nucleic acids Res. 33 (2), W677–W680. doi:10.1093/nar/gki394
Mount, D. W. (2007). Using the basic local alignment search tool (BLAST). Cold Spring Harb. Protoc. 2007 (7), pdb.top17–top17. doi:10.1101/pdb.top17
Niazi, F., and Valadkhan, S. (2012). Computational analysis of functional long noncoding RNAs reveals lack of peptide-coding capacity and parallels with 3′ UTRs. RNA 18 (4), 825–843. doi:10.1261/rna.029520.111
Nielsen, M. M., Tehler, D., Vang, S., Sudzina, F., Hedegaard, J., Nordentoft, I., et al. (2014). Identification of expressed and conserved human noncoding RNAs. RNA 20 (2), 236–251. doi:10.1261/rna.038927.113
Ning, X., and Sun, L. (2021a). Identification and characterization of immune-related lncRNAs and lncRNA-miRNA-mRNA networks of Paralichthys olivaceus involved in Vibrio anguillarum infection. BMC genomics 22 (1), 447. doi:10.1186/s12864-021-07780-2
Ning, X., and Sun, L. (2021b). Systematic identification and analysis of circular RNAs of Japanese flounder (Paralichthys olivaceus) in response to Vibrio anguillarum infection. Genes. 12 (1), 100. doi:10.3390/genes12010100
Novikova, I. V., Hennelly, S. P., Tung, C. S., and Sanbonmatsu, K. Y. (2013). Rise of the RNA machines: exploring the structure of long non-coding RNAs. J. Mol. Biol. 425 (19), 3731–3746. doi:10.1016/j.jmb.2013.02.030
Paneru, B., Al-Tobasei, R., Palti, Y., Wiens, G. D., and Salem, M. (2016). Differential expression of long non-coding RNAs in three genetic lines of rainbow trout in response to infection with Flavobacterium psychrophilum. Sci. Rep. 6 (1), 36032–36114. doi:10.1038/srep36032
Pauli, A., Valen, E., Lin, M. F., Garber, M., Vastenhouw, N. L., Levin, J. Z., et al. (2012). Systematic identification of long noncoding RNAs expressed during zebrafish embryogenesis. Genome Res. 22 (3), 577–591. doi:10.1101/gr.133009.111
Pereiro, P., Lama, R., Moreira, R., Valenzuela-Muñoz, V., Gallardo-Escárate, C., Novoa, B., et al. (2020). Potential involvement of lncRNAs in the modulation of the transcriptome response to nodavirus challenge in European sea bass (Dicentrarchus labrax L.). Biology 9 (7), 165. doi:10.3390/biology9070165
Pertea, G., and Pertea, M. (2020). GFF utilities: GffRead and GffCompare. F1000Research 9, 304. doi:10.12688/f1000research.23297.2
Pertea, M., Kim, D., Pertea, G. M., Leek, J. T., and Salzberg, S. L. (2016). Transcript-level expression analysis of RNA-seq experiments with HISAT, StringTie and Ballgown. Nat. Protoc. 11 (9), 1650–1667. doi:10.1038/nprot.2016.095
Pertea, M., Pertea, G. M., Antonescu, C. M., Chang, T. C., Mendell, J. T., and Salzberg, S. L. (2015). StringTie enables improved reconstruction of a transcriptome from RNA-seq reads. Nat. Biotechnol. 33 (3), 290–295. doi:10.1038/nbt.3122
Ranjan, G., Sehgal, P., Sharma, D., Scaria, V., and Sivasubbu, S. (2021). Functional long non-coding and circular RNAs in zebrafish. Briefings Funct. Genomics 20 (6), elab014–360. doi:10.1093/bfgp/elab014
Rbbani, G., Nedoluzhko, A., Siriyappagouder, P., Sharko, F., Galindo-Villegas, J., Raeymaekers, J. A. M., et al. (2023). The novel circular RNA CircMef2c is positively associated with muscle growth in Nile tilapia. Genomics 115 (3), 110598. doi:10.1016/j.ygeno.2023.110598
Rinn, J. L., and Chang, H. Y. (2012). Genome regulation by long noncoding RNAs. Annu. Rev. Biochem. 81, 145–166. doi:10.1146/annurev-biochem-051410-092902
Salmena, L., Poliseno, L., Tay, Y., Kats, L., and Pandolfi, P. P. (2011). A ceRNA hypothesis: the Rosetta Stone of a hidden RNA language? Cell. 146 (3), 353–358. doi:10.1016/j.cell.2011.07.014
Salzman, J., Gawad, C., Wang, P. L., Lacayo, N., and Brown, P. O. (2012). Circular RNAs are the predominant transcript isoform from hundreds of human genes in diverse cell types. PloS one 7 (2), e30733. doi:10.1371/journal.pone.0030733
Sanger, H. L., Klotz, G., Riesner, D., Gross, H. J., and Kleinschmidt, A. K. (1976). Viroids are single-stranded covalently closed circular RNA molecules existing as highly base-paired rod-like structures. Proc. Natl. Acad. Sci. 73 (11), 3852–3856. doi:10.1073/pnas.73.11.3852
Schmieder, R., and Edwards, R. (2011). Quality control and preprocessing of metagenomic datasets. Bioinformatics 27 (6), 863–864. doi:10.1093/bioinformatics/btr026
Shannon, P., Markiel, A., Ozier, O., Baliga, N. S., Wang, J. T., Ramage, D., et al. (2003). Cytoscape: a software environment for integrated models of biomolecular interaction networks. Genome Res. 13 (11), 2498–2504. doi:10.1101/gr.1239303
Sharma, D., Sehgal, P., Mathew, S., Vellarikkal, S. K., Singh, A. R., Kapoor, S., et al. (2019). A genome-wide map of circular RNAs in adult zebrafish. Sci. Rep. 9 (1), 3432. doi:10.1038/s41598-019-39977-7
Shen, Y., Guo, X., and Wang, W. (2017). Identification and characterization of circular RNAs in zebrafish. FEBS Lett. 591 (1), 213–220. doi:10.1002/1873-3468.12500
Shumayla, M., Sharma, S., Taneja, M., Tyagi, S., Singh, K., and Upadhyay, S. K. (2017). Survey of high throughput RNA-Seq data reveals potential roles for lncRNAs during development and stress response in bread wheat. Front. Plant Sci. 8, 1019. doi:10.3389/fpls.2017.01019
Song, F., Wang, L., Zhu, W., and Dong, Z. (2019). Long noncoding RNA and mRNA expression profiles following igf3 knockdown in common carp, Cyprinus carpio. Sci. Data 6 (1), 190024–190028. doi:10.1038/sdata.2019.24
Sun, S., Song, F., Shi, L., Zhang, K., Gu, Y., Sun, J., et al. (2023). Transcriptome analysis of differentially expressed circular RNAs in the testis and ovary of golden pompano (Trachinotus blochii). Comp. Biochem. Physiology Part D Genomics Proteomics 45, 101052. doi:10.1016/j.cbd.2022.101052
Teletchea, F. (2015). Domestication of marine fish species: update and perspectives. J. Mar. Sci. Eng. 3 (4), 1227–1243. doi:10.3390/jmse3041227
Valenzuela-Muñoz, V., Pereiro, P., Álvarez-Rodríguez, M., Gallardo-Escárate, C., Figueras, A., and Novoa, B. (2019). Comparative modulation of lncRNAs in wild-type and rag1-heterozygous mutant zebrafish exposed to immune challenge with spring viraemia of carp virus (SVCV). Sci. Rep. 9 (1), 14174–14213. doi:10.1038/s41598-019-50766-0
Wang, F., Jia, Y., Wang, P., Yang, Q., Du, Q., and Chang, Z. (2017a). Identification and profiling of Cyprinus carpio microRNAs during ovary differentiation by deep sequencing. BMC genomics 18 (1), 333–417. doi:10.1186/s12864-017-3701-y
Wang, L., Song, F., Zhu, W., Fu, J., Dong, Z., and Xu, P. (2021). The stage-specific long non-coding RNAs and mRNAs identification and analysis during early development of common carp, Cyprinus carpio. Cyprinus Carpio. Genomics. 113 (1), 20–28. doi:10.1016/J.YGENO.2020.11.025
Wang, N., Wang, R., Wang, R., Tian, Y., Shao, C., Jia, X., et al. (2017b). The integrated analysis of RNA-seq and microRNA-seq depicts miRNA-mRNA networks involved in Japanese flounder (Paralichthys olivaceus) albinism. PLOS ONE 12 (8), e0181761. doi:10.1371/JOURNAL.PONE.0181761
Wu, S., Huang, J., Li, Y., Liu, Z., and Zhao, L. (2022). Integrated analysis of lncRNA and circRNA mediated ceRNA regulatory networks in skin reveals innate immunity differences between wild-type and yellow mutant rainbow trout (Oncorhynchus mykiss). Front. Immunol. 13, 802731. doi:10.3389/fimmu.2022.802731
Xiu, Y., Li, Y., Liu, X., Su, L., Zhou, S., and Li, C. (2021). Identification and characterization of long non-coding RNAs in the intestine of olive flounder (Paralichthys olivaceus) during Edwardsiella tarda infection. Front. Immunol. 12, 623764. doi:10.3389/fimmu.2021.623764
Xu, P., Zhang, X., Wang, X., Li, J., Liu, G., Kuang, Y., et al. (2014). Genome sequence and genetic diversity of the common carp, Cyprinus carpio. Nat. Genet. 46 (11), 1212–1219. doi:10.1038/ng.3098
Xu, S., Xiao, S., Qiu, C., and Wang, Z. (2017). Transcriptome-wide identification and functional investigation of circular RNA in the teleost large yellow croaker (Larimichthys crocea). Mar. Genomics 32, 71–78. doi:10.1016/j.margen.2016.12.004
Yang, Z., Luo, W., Huang, Z., Guo, M., He, X., Fan, Z., et al. (2022). Genome-wide analysis of differentially expressed mRNAs and lncRNAs in koi carp infected with koi herpesvirus. Viruses 14 (11), 2555. doi:10.3390/v14112555
Zhang, X., Sun, B., Bai, Y., Canário, A. V., Xu, X., and Li, J. (2022). Long non-coding RNAs are involved in immune resistance to Aeromonas hydrophila in black carp (Mylopharyngodon piceus). Fish Shellfish Immunol. 127, 366–374. doi:10.1016/j.fsi.2022.06.031
Zhang, X., Zhou, T., Chen, B., Bai, H., Bai, Y., Zhao, J., et al. (2020). Identification and expression analysis of long non-coding RNA in large yellow croaker (Larimichthys crocea) in response to Cryptocaryon irritans infection. Front. Genet. 11, 590475. doi:10.3389/fgene.2020.590475
Zhao, B. W., Zhou, L. F., Liu, Y. L., Wan, S. M., and Gao, Z. X. (2017). Evolution of fish let-7 microRNAs and their expression correlated to growth development in blunt snout bream. Int. J. Mol. Sci. 18 (3), 646. doi:10.3390/ijms18030646
Zhao, X. F., Liang, L. Q., Liew, H. J., Chang, Y. M., Sun, B., Wang, S. Y., et al. (2021). Identification and analysis of long non-coding RNAs in Leuciscus waleckii adapted to highly alkaline conditions. Front. physiology 12, 665268. doi:10.3389/fphys.2021.665268
Zhou, R., Sanz-Jimenez, P., Zhu, X. T., Feng, J. W., Shao, L., Song, J. M., et al. (2021). Analysis of rice transcriptome reveals the lncRNA/circRNA regulation in tissue development. Rice 14 (1), 14–16. doi:10.1186/s12284-021-00455-2
Keywords: Cyprinus carpio, circRNAs, circRNA-miRNA-mRNA interaction, GO, lncRNAs, lncRNA-miRNA-mRNA interaction, KEGG, RNA-seq
Citation: Das J, Kumar B, Saha B, Jaiswal S, Iquebal MA, Angadi UB and Kumar D (2023) Genome-wide identification and characterization of tissue specific long non-coding RNAs and circular RNAs in common carp (Cyprinus carpio L.). Front. Genet. 14:1239434. doi: 10.3389/fgene.2023.1239434
Received: 13 June 2023; Accepted: 31 October 2023;
Published: 28 November 2023.
Edited by:
QZ Zhou, National University of Singapore, SingaporeCopyright © 2023 Das, Kumar, Saha, Jaiswal, Iquebal, Angadi and Kumar. This is an open-access article distributed under the terms of the Creative Commons Attribution License (CC BY). The use, distribution or reproduction in other forums is permitted, provided the original author(s) and the copyright owner(s) are credited and that the original publication in this journal is cited, in accordance with accepted academic practice. No use, distribution or reproduction is permitted which does not comply with these terms.
*Correspondence: Sarika Jaiswal, c2FyaWthQGljYXIuZ292Lmlu