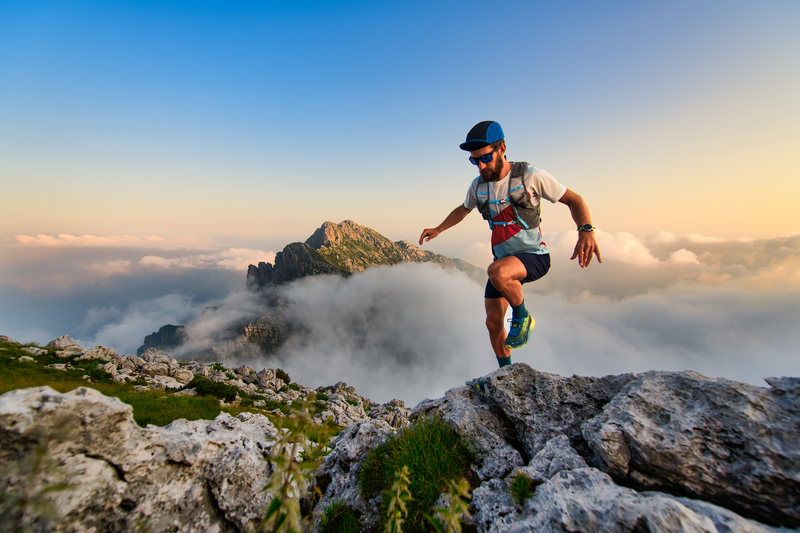
94% of researchers rate our articles as excellent or good
Learn more about the work of our research integrity team to safeguard the quality of each article we publish.
Find out more
ORIGINAL RESEARCH article
Front. Genet. , 13 October 2023
Sec. Computational Genomics
Volume 14 - 2023 | https://doi.org/10.3389/fgene.2023.1230998
This article is part of the Research Topic Multi-Omics Approaches for Decoding Heterogeneity in Cancer Immunotherapy View all 17 articles
Objective: Estrogen receptor breast cancer (BC) is characterized by the expression of estrogen receptors. It is the most common cancer among women, with an incidence rate of 2.26 million cases worldwide. The aim of this study was to identify differentially expressed genes and isoform switching between estrogen receptor positive and triple negative BC samples.
Methods: The data were collected from ArrayExpress, followed by preprocessing and subsequent mapping from HISAT2. Read quantification was performed by StringTie, and then R package ballgown was used to perform differential expression analysis. Functional enrichment analysis was conducted using Enrichr, and then immune genes were shortlisted based on the ScType marker database. Isoform switch analysis was also performed using the IsoformSwitchAnalyzeR package.
Results: A total of 9,771 differentially expressed genes were identified, of which 86 were upregulated and 117 were downregulated. Six genes were identified as mainly associated with estrogen receptor positive BC, while a novel set of ten genes were found which have not previously been reported in estrogen receptor positive BC. Furthermore, alternative splicing and subsequent isoform usage in the immune system related genes were determined.
Conclusion: This study identified the differential usage of isoforms in the immune system related genes in cancer cells that suggest immunosuppression due to the dysregulation of CXCR chemokine receptor binding, iron ion binding, and cytokine activity.
Breast cancer (BC) is one of the most commonly diagnosed global malignancies and is a leading cause of mortality among women. BC is a heterogeneous disease involving multiple environmental and genetic factors such as age, hormones, unhygienic diet, or toxic environmental exposure. The BRCA1 and BRCA2 tumor suppressor genes play a significant role in BC development (Li et al., 2017). Despite advances in treatments like chemotherapy, endocrine therapy, and human epidermal growth factor receptor-2 (HER2)-targeted therapy, the chance of relapse and BC metastasis remains a great challenge (Zhu and Yu, 2022). BC is a global health challenge as the most commonly diagnosed cancer, with an estimated incidence of 2.26 million cases worldwide according to GLOBOCAN 2020 global cancer statistics. The reported BC incidence rate is higher in Asia at 45.4% (Sung et al., 2021). There are different types of BC, depending on which cells in the breast become cancerous. Estrogen receptor positive (ERP) and triple-negative BC (TNBC) are the most aggressive types of BC. ERP BC is characterized by the presence of estrogen receptors (ERs) on tumor cells that help them grow and proliferate rapidly based on estrogen fueling. It is the largest subtype of BC as it involves the expression and activity of the estrogen receptor. It is estimated that approximately 80% of BCs are ERP (Lamb et al., 2019). TNBC is defined as a type of BC with a negative expression of ER, progesterone receptor (PR), and HER2. The mortality rate of TNBC is higher because of its high invasiveness and because approximately 46% of TNBC patients are more likely to have distant metastasis (Yin et al., 2020).
The ERP BC microenvironment (BCM) consists of immune cells, fibroblasts, adipocytes, mesenchymal stem cells, extracellular matrix, and tumor-associated macrophages (TAMs) (Munir et al., 2021). During breast tumorigenesis, tumor cells escape the immune surveillance by modifying surface antigens and altering their surrounding environment (Segovia-Mendoza and Morales-Montor, 2019). Chemokine, a family of signaling proteins, functions to induce leukocyte migration. Chemokine CC receptor type 5 (CCR5) is a cell surface receptor that has a high affinity for chemotropic cytokines called chemokines. A 32-bp deletion in this receptor (CCR5Δ32) results in a non-functional and deformed receptor which, in turn, results in the activation and invasion of immune cells at the site of tumorigenesis and ultimately leads to its progression (Fatima et al., 2019). In mammalian cells, alternative splicing (AS) is a key mechanism of gene expression regulation. AS occurs when intron and exon elements become rearranged by splicing at different splice-sites, resulting in multiple RNA transcripts. AS regulation is influenced by multiple factors such as cancer or other diseases (Vitting-Seerup and Sandelin, 2017). It occurs when there is differential usage of gene transcripts between different conditions (Baralle and Giudice, 2017). Thus, gene expression should be analyzed at the isoform level because isoform switching (IS) with predicted functional consequences is more common and important in dysfunctional cells (Kahraman et al., 2020).
RNA sequencing (RNA-seq) is a proven quantitative tool for the expression estimation of cells and facilitates the detection and identification of novel transcripts generated by AS. This study identified differential isoform usage (DIU) across conditions (ERP vs. TNBC) in immune system-related genes that may assist targeted therapies for ERP BC. Identifying novel biomarkers and isoform switching may pave the way for the early detection and successful treatment of ERP BC (Chen et al., 2022).
The data were collected from ArrayExpress: E-MTAB-4993. Further processing and analysis were performed by RNA-seq analysis consisting of preprocessing, mapping, quantifying, and differential expression analysis (DEA) methods (Costa-Silva et al., 2017). Isoform switching and DIU were ultimately detected in immune system-related dysregulated genes in ERP vs. TNBC. This study considered two biological conditions of BC, ERP, and TNBC. The raw data comprised 63 samples (ERP = 51, TNBC = 12).
The data obtained from ArrayExpress were in the form of raw reads and required preprocessing and quality control to reduce noise by trimming poor quality reads, adaptors, and primers. The first step in data preprocessing was quality assessment, which was performed by the FastQC tool to generate individual quality reports for each sample (v0.11.9) (Rostovskaya et al., 2022). The fastp tool (v0.20.0) was then used to trim poor quality reads to remove primer and adaptor content, resulting in filtered reads (Chen et al., 2018). These filtered reads then underwent quality check analysis by FastQC. Next, the filtered reads were used as input in the HISAT2 tool (v2.1.0) for mapping against the reference genome of Homo sapiens (GRCh38) (Kim et al., 2015). This generated files in SAM (sequence alignment map) format which contained aligned reads. Mapping rates indicative of the quality of RNA sequencing are presented in Supplementary File 6.
Before read quantification, the SAM files were first converted to BAM (binary alignment map) format, which is the compressed and binary format of aligned reads, using Samtools (v1.16) (Danecek et al., 2021). Next, BamUtil (v1.0.15) was used to remove duplicates (deduplication) from mapped reads (Jun et al., 2017). The quantification of deduplicated sorted reads was then performed using StringTie (v2.2.0) (Shumate et al., 2022) in three steps. In the first step, the StringTie assembler was employed to assemble the aligned reads of each sample into a transcriptome. In the second step, the full set of transcriptome assemblies was passed to the StringTie merge module to merge the genomic features among all samples to create a consistent set of transcripts across all samples. In the final step, this merged assembly was used to estimate the transcript abundances (Pertea et al., 2016). The identification of differentially expressed genes (DEGs) and their enrichment analysis offers biological insights into the processes that are affected by certain conditions (Frazee et al., 2015). R package ballgown (v3.15) was used to perform differential gene expression (DGE) analysis of all the transcripts and abundances in ERP vs. TNBC (Frazee et al., 2014). Criteria of a p-value less than 0.05 and log2 FoldChange value of <1.5 and >1.5 were used to identify biologically and statistically significant DEGs in ERP vs. TNBC. DEGs were graphically represented by the volcano plot (Nisar et al., 2021).
For Gene Ontology (GO) and pathway enrichment analysis, the Enrichr package was used (Xie et al., 2021). The analysis of both up- and downregulated DEGs was performed separately. The plotEnrich () function was used to plot bar charts of biological processes (BP), molecular functions (MF), cellular components (CC), and KEGG pathways. The results were ordered according to p-value.
The ScType cell marker database was used to filter the genes involved in immune functions (regulation of immune cells through signaling pathway and immune response against tumors) from the DEGs identified in the previous step (Gonzalez et al., 2018; Ianevski et al., 2022). Genes common to the DEGs set and the ScType database (immune system) were selected for further analysis.
Isoform switch analysis was performed to identify transcript-level expression profiles between ERP and TNBC to detect potential functional consequences resulting from isoform switch. The IsoformSwitchAnalyzeR package (v1.16.0) was used for this analysis (Vitting-Seerup and Sandelin, 2017). The package's input was the quantification files from StringTie, transcript files, a file containing merged annotations of all samples, and a design file containing sample IDs and relevant condition status. The IsoformSwitchTestDEXSeq () function was used to identify DIU based on differential isoform (dIF) cutoff. A dIF criteria of 0.1 was used to find the relative abundances of all isoforms of a gene between two sample groups, and gene ExpressionCutoff of 0.5 was applied. The open reading frames (ORF) were analyzed using the analyzeORF() function, where the longest orfMethod was selected in order to shortlist only long ORFs due to their functional importance. The longest ORFs were then extracted using the extractSequence () function that outputs two files—one containing nucleotide sequences and the other including protein sequences. The functional consequences of ORFs were identified in order to add functional knowledge to the transcripts. Four types of functional consequences were identified for ORFs: coding potential, protein domains, signal peptides, and intrinsically disordered regions (IDRs). The coding potential of the genes was identified through the CPC2 tool that takes nucleotide sequences as an input. The Pfam tool was used to predict protein domains. The signal peptides of ORFs were identified through SignalP, whereas intrinsically disordered regions (IDRs) were predicted by the IUPred3 tool. To assign the predicted functional consequences to the transcripts, R package was employed using functions such as analyzeCPC2 (), analyzeSignalP (), analyzePFAM (), and analyzeIUPred2A (). Moreover, the switchPlot () function was used to plot the shortlisted immune system-related genes that were dysregulated in ERP BC.
The gene expression profiling of 63 samples (ERP = 51, TNBC = 12) by ballgown R/Bioconductor identified 15,947 DEGs between ERP and TNBC tissue samples. Genes with no annotation were filtered out, with 9,771 DEGs remaining. Using DEA, 86 genes were identified as upregulated (logFC >1.5 and p-value <0.05) and 117 were downregulated (logFC < −1.5 and p-value <0.05) (Figure 1). The top 10 upregulated DEGs were FOXA1, RHOB, AR, CMBL, AGR2, ESR1, TFF3, SYBU, CBLC, and DNALI1 (Table 1; Supplementary Information S1); the top 10 downregulated DEGs were CENPW, EN1, A2ML1, TMSB15A, FOXC1, KRT16, SLC7A5, CDK6, MELTF, and CA9 (Table 2; Supplementary Information S2).
FIGURE 1. Volcano plot for DEGs. The red dots represent up- (right) and downregulated (left) DEGs. Upregulated genes having logFC >1.5 and p-value <0.05 can be seen on the right of the plot; downregulated genes having logFC < −1.5 and p-value <0.05 can be seen on the left of the plot.
Both up- and downregulated DEGs were subjected to GO enrichment analysis. This analysis revealed BP, CC, and MF that were affected due to change in gene expression. Upregulated DEGs of biological processes were enriched in steroid hormone-mediated signaling pathway, intracellular steroid hormone receptor signaling pathway, regulation of smooth muscle cell proliferation, and response to estrogen, indicating that upregulated genes are involved in the regulation of breast stem cells, increased cell proliferation, increased estrogen hormone and cancerous T-cells, angiogenesis, and excessive mitochondrial and sodium ion transport (Figure 2A; Table 3). The downregulated DEGs enriched in mitotic spindle organization, chemokine-mediated signaling pathway, cellular response to chemokine, microtubule cytoskeleton organization involved in mitosis, antimicrobial humoral immune response mediated by antimicrobial peptides, neutrophil chemotaxis, granulocyte chemotaxis, and the attachment of mitotic spindle microtubules to kinetochore and kinetochore organization indicated that they may have cancer development-related functions because of disrupted cell signaling and a dysregulated cell cycle due to incorrectly organized proteins and a suppressed immune system (Figure 2B; Table 4). Alternatively, the upregulated DEGs of molecular functioning show transcription coactivator binding, RNA polymerase II general transcription initiation factor binding, epidermal growth factor receptor binding, BMP receptor binding, SH3 domain binding, ATPase binding, general transcription initiation factor binding, metallocarboxypeptidase activity, and IgG binding (Figure 3A; Table 5). This denotes disrupted cell signaling, increased cell proliferation, growth, differentiation, and epithelial–mesenchymal transition (EMT) due to upregulated transcription. On the other hand, the downregulated DEGs were mainly enriched in CXCR3 and CXCR chemokine receptor binding, chemokine and cytokine activity, peptidase inhibitor activity, L-leucine transmembrane transporter activity, and chitinase activity, which indicate suppressed immune system response and increased abnormal proteins which may result in cancer progression and development (Figure 3B; Table 6). The cellular component enrichment of upregulated DEGs, collagen-containing extracellular matrix, elastic fiber, Golgi lumen, intracellular organelle lumen, basement membrane, and sodium:potassium-exchanging ATPase complex indicate that these may have been involved in the initiation and progression of cancer due to changes in the cellular cytoskeleton, membrane remodeling, and alterations in protein secretions (Figure 4A; Table 7). Moreover, the downregulated DEGs of cellular components show intermediate filament, intermediate filament cytoskeleton, polymeric cytoskeletal fiber, spindle, cornified envelope, desmosome, and endoplasmic reticulum lumen, which indicate cancer development and progression due to disrupted cytoskeletal proteins, dysregulated cell division, misfolded proteins, and DNA damage (Figure 4B; Table 8).
FIGURE 2. GO biological processes up- and downregulated by DEGs. Bar chart plots of top 15 BPs in ERP vs. TNBC. The x-axis is the gene ratio, while the color represents p-value. (A) Steroid hormone-mediated signaling pathway and response to estrogen and endothelial tube morphogenesis as significant upregulated BP. (B) Chemokine-mediated signaling pathway, cellular response to chemokine, granulocyte chemotaxis, and attachment of mitotic spindle microtubules to kinetochore as significant downregulated BP.
FIGURE 3. GO molecular functions up- and downregulated by DEGs. Bar chart plots of top 15 MF in ERP vs. TNBC. X-axis is the gene ratio, while the color represents p-value. (A) Transcription coactivator binding, RNA polymerase II general transcription initiation factor binding, epidermal growth factor receptor binding, and BMP receptor binding as significant upregulated MF. (B) CXCR3 chemokine receptor binding, CXCR chemokine receptor binding, chemokine activity, and L-leucine transmembrane transporter activity as significant downregulated MF.
FIGURE 4. GO cellular components up- and downregulated by DEGs. Bar chart plots of top-15 CC in ERP vs. TNBC. X-axis is the gene ratio, while the color represents p-value. (A) Collagen-containing extracellular matrix, elastic fiber, Golgi lumen, and intracellular organelle lumen as significant upregulated CC. (B) Intermediate filament, intermediate filament cytoskeleton, polymeric cytoskeletal fiber, spindle, and desmosome as significant downregulated CC.
KEGG pathways were predicted using Enrichr for the DEGs to identify biological pathways that are disrupted due to the up- and downregulation of genes involved in those pathways. As indicated in Figure 5A, upregulated genes such as BMP4, GSTM3, FOS, HMGCS2, ADH1B, COL4A5, ESR1, NAT1, IL6ST, and PGR were enriched in pathways in cancer, chemical carcinogenesis, ECM–receptor interaction, tyrosine metabolism, valine, leucine and isoleucine degradation, the PI3K-Akt signaling pathway, estrogen signaling pathway, caffeine metabolism, signaling pathways that regulate the pluripotency of stem cells, and BC (Table 9). This indicates that the upregulation of genes promotes pathways that are mainly involved in DNA repair, cell motility and proliferation, cell cycle regulation, the inhibition of apoptosis, and increased EMT, resulting in tumor development and prognosis. The downregulated genes such as CXCL8, CXCL10, CXCL11, CDC20, CDK6, CDKN2A, CXCL13, and SHC4 (Table 10) were enriched in chemokine signaling pathway, toll-like receptor signaling pathway, cell cycle, bladder cancer, IL-17 signaling pathway, cellular senescence, p53 signaling pathway, and microRNAs in cancer (Figure 5B). The downregulation of genes involved in these pathways plays a crucial role in the tumor microenvironment by disrupting immune response, cell cycle arrest in the G2/M phase, increased cell growth, metastasis, proliferation and invasiveness, and the angiogenic potential of cancer cells. The analysis revealed that cancer-related pathways that were dysregulated due to DEGs have also been reported in various other cancers.
FIGURE 5. KEGG pathways analysis of upregulated DEGs. Bar chart plots of top 15 KEGG pathways in ERP vs. TNBC. X-axis is the gene ratio, while the color represents p-value. (A) Pathways in cancer, chemical carcinogenesis, ECM–receptor interaction, and tyrosine metabolism as significant upregulated pathways. (B) Chemokine signaling pathway, toll-like receptor signaling pathway, cell cycle, bladder cancer, and IL-17 signaling pathway as significant downregulated pathways.
A total of 86 upregulated and 117 downregulated genes were used as a query against the marker genes database of the ScType R package, revealing that three upregulated and 10 downregulated genes were directly involved in immune system-related functions (Table 11, 12).
Isoform switching analysis was performed on shortlisted immune system related genes, facilitating the identification of known and novel isoform switches from RNA-seq derived quantification data. Of 10 downregulated immune genes, three (STMN1, MELTF, and CXCL8) were found to have isoforms that were significantly used in ERP and have been validated through the Expression Atlas (Supplementary Information Table S3). Moreover, no significant isoform switch was observed in upregulated immune genes.
STMN1, MELTF, and CXCL8 represent significant switches in isoform usage across ERP vs. TNBC, as shown in sashimi plots in Supplementary Information Figures S1–S3 respectively. By comparing the isoform usage across conditions, it was revealed that STMN1 has a single isoform (ENST00000485226) which was overexpressed in ERP. CXCL8 also has one isoform (ENST00000483500) which was significantly used in ERP. Furthermore, it was found that a novel isoform (MSTRG.29921.1) of MELTF was overexpressed in ERP (Figure 6).
FIGURE 6. Isoform switch of differentially expressed immune genes. “*” represents significant isoform usage.
Gene Ontology analysis of downregulated immune genes indicates that these genes may be involved in key immune system molecular functions such as CXCR chemokine receptor binding, chemokine activity, iron ion binding, and cytokine activity (Figure 7).
FIGURE 7. GO molecular functions of downregulated immune system genes (STMN1, MELTF, and CXCL8) having significant isoform switches (p-value <0.05).
BC is the most prevalent type of cancer worldwide. It is thus essential to understand and explore ways to prevent its occurrence while identifying the genetic changes that are more susceptible to its incidence. The present study identified 9,771 DEGs, of which 86 genes were significantly upregulated and 117 were downregulated. The identified upregulated genes were FOXA1, RHOB, AR, CMBL, AGR2, ESR1, TFF3, SYBU, CBLC, and DNALI1; the downregulated genes were CENPW, EN1, A2ML1, TMSB15A, FOXC1, KRT16, SLC7A5, CDK6, MELTF, and CA9. Functional enrichment analysis of these DEGs revealed that the intracellular steroid hormone receptor signaling pathway, chemokine-mediated signaling pathway, kinetochore organization, pathways in cancer, BC, toll-like receptor signaling pathway, and cell cycle were the most dysregulated biological pathways and processes.
In the present study, FOXA1 was found to be upregulated and has been reported to inhibit STAT2, a transcription factor and its target IFN signaling pathway in BC; this may result in cancer progression due to suppressed immune response (He et al., 2021). Furthermore, upregulated RHOB results in ER-α (estrogen receptor alpha) overexpression that leads to increased estrogen uptake by BC cells which helps them grow and proliferate (Médale-Giamarchi et al., 2013). It has been reported that AR overexpression increases the transcription of genes involved in the cell cycle, resulting in increased proliferation of prostate cancer cells (Formaggio et al., 2021). This study found that CMBL (p-value: 0.00003) is suppressed in TNBC compared to non-TNBC types of BC, such as ERP. It encodes a cysteine hydrolase that cleaves cyclic esters which activate an angiotensin receptor blocker that helps lower blood pressure (Guo et al., 2017). Upregulated AGR2 is found in BC due to ER signaling and endoplasmic reticulum stress, and it results in increased cell proliferation, survival, and metastasis in BC (Ann et al., 2018). Moreover, ESR1 upregulation makes BC cells more prone to estrogen uptake which may lead to the increased growth and proliferation of cancer cells (Lei et al., 2019). According to the literature, TFF3 acts as an oncogene because it regulates other genes (FOXA1, HER2, and AR) involved in EMT, thus promoting invasiveness, survival, and increased proliferation in multiple carcinomas such as gastric cancer, mammary carcinoma, and prostate cancer (Yuan et al., 2017). It has been reported that SYBU, a microtubule-associated protein, is overexpressed in hepatocellular carcinoma (HCC), which results in disrupted cell cycle and increased proliferation (Zheng and Yu, 2021). Breast tumor formation is increased by CBLC overexpression, which suppresses TGF-β (transforming growth factor beta). This results in the deactivation of its target Smad3 pathway which is responsible for proliferation, differentiation, and apoptosis (Kang et al., 2012). This study found that DNALI1 (p-value: 0.0000148), a flagellar protein, is overexpressed in BC, which has not been reported previously for any other carcinoma.
According to the literature, CENPW was downregulated in BC and HCC. It is involved in kinetochore organization and centromere complex assembly. This downregulation results in subsequent function disruption, resulting in chromosomal instability due to mis-segregation of chromosomes (Liu and Liu, 2022). It has been reported that EN1 is downregulated in lung cancer due to altered DNA methylation which promotes cell proliferation and differentiation (Jiang et al., 2017). The downregulation of A2ML1, a protease inhibitor, results in MAPK pathway mutation, which leads to apoptotic resistance and uncontrolled cell division in BC (Li et al., 2016). FOXC1 suppression induces ER-α expression in BC cells, which helps in increased estrogen uptake, resulting in the growth and proliferation of tumor cells (Wang et al., 2017). It has been reported that KRT16 is overexpressed in basal-like TNBC, along with increased expression of EMT-associated proteins. In contrast, in luminal A and B subtypes of BC which include ER+ and PR+ tumors, KRT16 expression was suppressed, but E-cadherin (CDH1), an EMT protein was overexpressed, leading to metastasis due to increased cellular motility (Elazezy et al., 2021). SLC7A5 has been reported to be overexpressed in TNBC due to its glutamine transporting activity to tumor cells for energy production—TNBC is thus glutamine dependent and requires glutaminase for its catabolism. In addition, cells with increased proliferation use transaminases to catabolize glutamate, in contrast to glutamate dehydrogenase (GLUD), to reduce ammonia production. However, ER+ tumors are glutamine-independent and show increased GLUD expression (Wang et al., 2020). It has been reported that the downregulation of CDK6 also suppresses its interacting gene, RB1—a tumor suppressor gene. This results in dysregulated cell growth, apoptosis, and increased proliferation in tumor cells (Knudsen et al., 2020). MELTF downregulation also dysregulates its interacting genes such as ACO2, a gene-encoding Krebs’ cycle enzyme. The disruption of Krebs’ cycle enzymes leads to the production of oncometabolites, which stabilize hypoxia-inducible factor 1 and activate cell growth signaling by regulating DNA methylation—crucial factors in cancer progression (Sajnani et al., 2017). CA9 suppression leads to the disruption of interacting genes such as HIF3A and EPAS1 which are involved in regulating hypoxic conditions. Such conditions are favorable for the increased proliferation of tumor cells (Jun et al., 2017). TMSB15A has been reported to be upregulated in TNBC; it plays a crucial role in the organization of the cytoskeleton, which is responsible for cancer cell motility and is involved in cancer metastasis (Darb-Esfahani et al., 2012).
KEGG pathway enrichment analysis revealed protein digestion and absorption, pathways in cancer, chemical carcinogenesis, and ECM–receptor interaction as upregulated pathways, while downregulated pathways include chemokine signaling, toll-like receptor signaling, cell cycle, bladder cancer, IL-17 signaling, cellular senescence, cytokine–cytokine receptor interaction, and p53 signaling. We found that the protein digestion and absorption pathway was upregulated, which demonstrates the use of proteins as an alternative fuel by tumor cells to fulfill their metabolic needs. This occurs due to the limited supply and metabolism of glucose by cancer cells (Lieu et al., 2020). Moreover, it was found that pathways in cancer were upregulated that involve the disruption of the ErbB, p-53-mediated apoptotic, and GSK3 signaling pathways, which are involved in DNA repair, cell growth, migration, differentiation, and metabolism (Yip and Papa, 2021). The upregulation of chemical carcinogenesis activates certain hormonal pathways that make mammary glands more susceptible to carcinogenesis due to altered DNA repair genes (Rodgers et al., 2018). Furthermore, upregulated ECM–receptor interaction results in interaction with HMMR and SDC1 genes, the dysregulation of which promotes BC cell motility and differentiation (Yeh et al., 2018). A downregulated chemokine signaling pathway and cytokine–cytokine receptor interaction cannot recruit immune cells (leukocytes) to the tumor microenvironment, thus resulting in tumor progression (Gil Del Alcazar et al., 2020). The downregulation of toll-like receptor signaling pathways results in non-recognition and the escape of cancer cells from the immune system, leading to the invasiveness, migration, and angiogenic potential of cancer cells (Javaid and Choi, 2020). The disruption of the cell cycle at the G2/M phase results in cells that contain damaged DNA and genomic instability, a hallmark of cancer (Thu et al., 2018). It has been reported that increased ER-α in BC cells suppresses bladder cancer cell growth by downregulating INPP4B which, in turn, suppresses the AKT signaling pathway (Hsu et al., 2014). Research has found that the IL-17 signaling pathway becomes downregulated due to increased estrogen receptor expression, resulting in dysregulated PD-1/PD-L1 and CD8+ T cell expression—a suppressed immune response (Shuai et al., 2020). Furthermore, downregulated cellular senescence results in increased cell proliferation and tumor development (Milczarek, 2020). Moreover, a downregulated p53 signaling pathway cannot perform DNA damage repair and cell death, thereby facilitating the increased growth and metastasis of tumor cells (Marei et al., 2021).
According to the GTEx portal, cells express an average of 3.42 transcripts per gene (Tung et al., 2020). The expression dominance of major isoform transcripts compared to others from the same gene is crucial for normal cellular homeostasis (Hu et al., 2017). However, splicing regulation is often disrupted in cancer with a dominant expression of alternative transcripts in a tumor microenvironment (TME) which promotes switches that contribute to tumor progression and metastasis (Kahraman et al., 2020). The interaction pattern of the cancer-specific most dominant transcript (cMDT) differs from the generally expressed isoform of normal cells because of changes caused by alternative splicing; this could affect protein domains due to mutations caused by tumor and subsequent disruption in cancer-related pathways (Yang et al., 2016; Climente-González et al., 2017). According to the literature, apoptosis, ubiquitin, signaling, and spliceosomes were the most disrupted protein interactions (Kahraman et al., 2020). It has been reported that isoform switching leads to the loss of the DNA sequence that encodes for protein domains, promoting functional loss. The subsequent switches have functional consequences for cancer development and progression (Vitting-Seerup and Sandelin, 2017).
The present study has identified the differential usage of transcript isoforms among ERP and TNBC. It revealed isoforms that are significantly expressed and used by shortlisted downregulated genes (STMN1, MELTF, and CXCL8). CXCL8 encodes a protein that is involved in chemotaxis (Łukaszewicz-Zając et al., 2020). It transcribes five transcripts; among them, only one isoform transcript was significantly used. Differential gene expression plotting shows that CXCL8 is downregulated in ERP (Figure 6). On the other hand, there is in isoform usage an increased use of isoform ENST00000483500 in ERP. However, this isoform is non-coding due to retained introns and the unavailability of any domain, leading to functional loss of CXCL8 and immune suppression as a consequence of the non-recruitment of macrophages and neutrophils to the TME (Xiong et al., 2022). STMN1, a cytosolic phosphoprotein, is involved in microtubule destabilization by regulating the microtubule filament system and signal transduction (Bao et al., 2017). It transcribes four known and two novel isoforms (Figure 6). The isoform usage plot indicates that a single isoform (ENST00000485226) is significantly used in ERP, as compared to TNBC. However, it lacks a domain, which results in its non-coding behavior. Furthermore, STMN1 is repressed in ERP, as shown in differential gene expression plotting which promotes ERP progression due to the disruption of microtubules and their subsequent role in the growth of immune cells such as T-cells and natural killer cells (Zhang et al., 2022). MELTF, a cell surface glycoprotein, is involved in cellular iron uptake (Sawaki et al., 2019). MELTF could transcribe six novel and two known isoforms (Figure 6); however, increased use of the single isoform MSTRG.29921.1 has been identified in ERP. Moreover, this isoform contains nonsense codons that prematurely terminate translation—nonsense-mediated decay (NMD). Differential gene expression plotting also shows that MELTF is downregulated in ERP, leading to the decreased proliferation and maturation of immune cells such as lymphocytes due to decreased iron uptake (Roemhild et al., 2021). Gene Ontology analysis of shortlisted immune genes revealed that CXCR chemokine receptor binding, iron ion binding, and cytokine activity are the most dysregulated molecular functions (Figure 7). These functions mediate immune response by recruiting immune cells such as monocytes, T cells, lymphocytes, and natural killer cells, and assist their growth and proliferation. The downregulation of these functions promotes ERP BC due to suppressed immune response in TME (Bates et al., 2018).
This research therefore provides key insights into the genes that are differentially expressed in ERP. Moreover, DNALI1 is a novel gene that has not been previously reported and is involved in ERP BC development. Furthermore, the identification of three immune system-related genes (STMN1, MELTF, and CXCL8) reveals that the dysregulation of the immune system due to isoform switching is the major factor in ERP BC development and progression. Downregulation and isoform switching of key immune system genes suggest BC progression and possible metastasis due to the non-recruitment of cytokines in the TME.
ERP BC is characterized by the growth of tumor cells in response to estrogen hormone. The dysregulation of gene expression results in the development of significant biological changes that are key features of multiple human carcinomas such as prostate cancer, gastric cancer, hepatocellular carcinoma, and lung cancer. In this study, 9,771 DEGs were identified; among these, 86 genes were upregulated and 117 were downregulated. Six genes (FOXA1, RHOB, AGR2, ESR1, CBLC, and FOXC1) were found to be significantly associated with the development and progression of ERP BC. This study also identified a novel set of genes (DNALI1, TMSB15A, AR, TFF3, SYBU, CENPW, EN1, CDK6, MELTF, and CA9) not previously reported positive for estrogen receptors but that has been reported in other carcinomas. Moreover, alternative splicing and subsequent isoform expression in three downregulated immune system genes (STMN1, MELTF, and CXCL8) had been identified that were mainly responsible for ERP progression due to suppression of the immune system and the non-recruitment of cytokines against cancer cells. It was found that CXCR chemokine receptor binding, iron ion binding, and cytokine activity were the most dysregulated functions due to immune system suppression. This study reveals that dysregulation of the immune system due to isoform switching is the major factor in ERP BC development and progression. Therefore, these crucial immune system genes should be targeted as therapeutic biomarkers.
The original contributions presented in the study are included in the article/Supplementary Material, further inquiries can be directed to the corresponding author.
Conceptualization, methodology, formal analysis, investigation, and validation: MK, WH, NA, BJ, IS, AhA, MA, SA, AS, DF, SA, YM, AmA, A-UR, and BH; data curation, writing—original draft preparation, and writing—review and editing: MK, WH, NA, BJ, and IS. All authors contributed to the article and approved the submitted version.
The authors extend their appreciation to the Researchers supporting project number (RSP2023R470), King Saud University, Riyadh, Saudi Arabia.
Author A-UR was employed by the company Keystone Pharmacogenomics, LLC.
The remaining authors declare that the research was conducted in the absence of any commercial or financial relationships that could be construed as a potential conflict of interest.
All claims expressed in this article are solely those of the authors and do not necessarily represent those of their affiliated organizations, or those of the publisher, the editors, and the reviewers. Any product that may be evaluated in this article, or claim that may be made by its manufacturer, is not guaranteed or endorsed by the publisher.
The Supplementary Material for this article can be found online at: https://www.frontiersin.org/articles/10.3389/fgene.2023.1230998/full#supplementary-material
Ann, P., Seagle, B.-L. L., Shilpi, A., Kandpal, M., and Shahabi, S. (2018). Association of increased primary breast tumor AGR2 with decreased disease-specific survival. Oncotarget 9, 23114–23125. doi:10.18632/oncotarget.25225
Bao, P., Yokobori, T., Altan, B., Iijima, M., Azuma, Y., Onozato, R., et al. (2017). High STMN1 expression is associated with cancer progression and chemo-resistance in lung squamous cell carcinoma. Ann. Surg. Oncol. 24, 4017–4024. doi:10.1245/s10434-017-6083-0
Baralle, F. E., and Giudice, J. (2017). Alternative splicing as a regulator of development and tissue identity. Nat. Rev. Mol. Cell Biol. 18, 437–451. doi:10.1038/nrm.2017.27
Bates, J. P., Derakhshandeh, R., Jones, L., and Webb, T. J. (2018). Mechanisms of immune evasion in breast cancer. BMC cancer 18, 556–614. doi:10.1186/s12885-018-4441-3
Chen, S., Zhou, Y., Chen, Y., and Gu, J. (2018). fastp: an ultra-fast all-in-one FASTQ preprocessor. Bioinformatics 34, i884–i890. doi:10.1093/bioinformatics/bty560
Chen, Y.-L., Wang, K., Xie, F., Zhuo, Z.-L., Liu, C., Yang, Y., et al. (2022). Novel biomarkers identified in triple-negative breast cancer through RNA-sequencing. Clin. Chim. Acta 531, 302–308. doi:10.1016/j.cca.2022.04.990
Climente-González, H., Porta-Pardo, E., Godzik, A., and Eyras, E. (2017). The functional impact of alternative splicing in cancer. Cell Rep. 20, 2215–2226. doi:10.1016/j.celrep.2017.08.012
Costa-Silva, J., Domingues, D., and Lopes, F. M. (2017). RNA-seq differential expression analysis: an extended review and a software tool. PloS one 12, e0190152. doi:10.1371/journal.pone.0190152
Danecek, P., Bonfield, J. K., Liddle, J., Marshall, J., Ohan, V., Pollard, M. O., et al. (2021). Twelve years of SAMtools and BCFtools. Gigascience 10, giab008. doi:10.1093/gigascience/giab008
Darb-Esfahani, S., Kronenwett, R., Von Minckwitz, G., Denkert, C., Gehrmann, M., Rody, A., et al. (2012). Thymosin beta 15A (TMSB15A) is a predictor of chemotherapy response in triple-negative breast cancer. Br. J. cancer 107, 1892–1900. doi:10.1038/bjc.2012.475
Elazezy, M., Schwentesius, S., Stegat, L., Wikman, H., Werner, S., Mansour, W. Y., et al. (2021). Emerging insights into keratin 16 expression during metastatic progression of breast cancer. Cancers 13, 3869. doi:10.3390/cancers13153869
Fatima, F., Saleem, S., Hameed, A., Haider, G., Ali Zaidi, S. A., Kanwal, M., et al. (2019). Association analysis and allelic distribution of deletion in CC chemokine receptor 5 gene (CCR5Δ32) among breast cancer patients of Pakistan. Mol. Biol. Rep. 46, 2387–2394. doi:10.1007/s11033-019-04699-6
Formaggio, N., Rubin, M. A., and Theurillat, J.-P. (2021). Loss and revival of androgen receptor signaling in advanced prostate cancer. Oncogene 40, 1205–1216. doi:10.1038/s41388-020-01598-0
Frazee, A. C., Pertea, G., Jaffe, A. E., Langmead, B., Salzberg, S. L., and Leek, J. T. (2015). Ballgown bridges the gap between transcriptome assembly and expression analysis. Nat. Biotechnol. 33, 243–246. doi:10.1038/nbt.3172
Frazee, A. C., Pertea, G., Jaffe, A. E., Langmead, B., Salzberg, S. L., and Leek, J. T. (2014). Flexible isoform-level differential expression analysis with Ballgown. Biorxiv, 003665. doi:10.1101/003665
Gil Del Alcazar, C. R., Alečković, M., and Polyak, K. (2020). Immune escape during breast tumor progression. Cancer Immunol. Res. 8, 422–427. doi:10.1158/2326-6066.CIR-19-0786
Gonzalez, H., Hagerling, C., and Werb, Z. (2018). Roles of the immune system in cancer: from tumor initiation to metastatic progression. Genes and Dev. 32, 1267–1284. doi:10.1101/gad.314617.118
Guo, J., Gong, G., and Zhang, B. (2017). Screening and identification of potential biomarkers in triple-negative breast cancer by integrated analysis. Oncol. Rep. 38, 2219–2228. doi:10.3892/or.2017.5911
He, Y., Wang, L., Wei, T., Xiao, Y.-T., Sheng, H., Su, H., et al. (2021). FOXA1 overexpression suppresses interferon signaling and immune response in cancer. J. Clin. Investigation 131, e147025. doi:10.1172/JCI147025
Hsu, I., Yeh, C.-R., Slavin, S., Miyamoto, H., Netto, G. J., Muyan, M., et al. (2014). Estrogen receptor alpha prevents bladder cancer via INPP4B inhibited akt pathway in vitro and in vivo. Oncotarget 5, 7917–7935. doi:10.18632/oncotarget.1421
Hu, J., Boritz, E., Wylie, W., and Douek, D. C. (2017). Stochastic principles governing alternative splicing of RNA. PLoS Comput. Biol. 13, e1005761. doi:10.1371/journal.pcbi.1005761
Ianevski, A., Giri, A. K., and Aittokallio, T. (2022). Fully-automated and ultra-fast cell-type identification using specific marker combinations from single-cell transcriptomic data. Nat. Commun. 13, 1246. doi:10.1038/s41467-022-28803-w
Javaid, N., and Choi, S. (2020). Toll-like receptors from the perspective of cancer treatment. Cancers 12, 297. doi:10.3390/cancers12020297
Jiang, C.-L., He, S.-W., Zhang, Y.-D., Duan, H.-X., Huang, T., Huang, Y.-C., et al. (2017). Air pollution and DNA methylation alterations in lung cancer: A systematic and comparative study. Oncotarget 8, 1369–1391. doi:10.18632/oncotarget.13622
Jun, J. C., Rathore, A., Younas, H., Gilkes, D., and Polotsky, V. Y. (2017). Hypoxia-inducible factors and cancer. Curr. Sleep. Med. Rep. 3, 1–10. doi:10.1007/s40675-017-0062-7
Kahraman, A., Karakulak, T., Szklarczyk, D., and Von Mering, C. (2020). Pathogenic impact of transcript isoform switching in 1,209 cancer samples covering 27 cancer types using an isoform-specific interaction network. Sci. Rep. 10, 14453. doi:10.1038/s41598-020-71221-5
Kang, J. M., Park, S., Kim, S. J., Hong, H., Jeong, J., Kim, H., et al. (2012). CBL enhances breast tumor formation by inhibiting tumor suppressive activity of TGF-β signaling. Oncogene 31, 5123–5131. doi:10.1038/onc.2012.18
Kim, D., Langmead, B., and Salzberg, S. L. (2015). Hisat: A fast spliced aligner with low memory requirements. Nat. methods 12, 357–360. doi:10.1038/nmeth.3317
Knudsen, E. S., Nambiar, R., Rosario, S. R., Smiraglia, D. J., Goodrich, D. W., and Witkiewicz, A. K. (2020). Pan-cancer molecular analysis of the RB tumor suppressor pathway. Commun. Biol. 3, 158. doi:10.1038/s42003-020-0873-9
Lamb, C. A., Vanzulli, S., and Lanari, C. L. M. (2019). Hormone receptors in breast cancer: more than estrogen receptors. Med. (B Aires) 79, 540–545.
Lei, J. T., Gou, X., Seker, S., and Ellis, M. J. (2019). ESR1 alterations and metastasis in estrogen receptor positive breast cancer. J. cancer metastasis Treat. 5, 38. doi:10.20517/2394-4722.2019.12
Li, G., Hu, J., and Hu, G. (2017). Biomarker studies in early detection and prognosis of breast cancer. Transl. Res. Breast Cancer Biomark. Diagnosis, Target. Ther. Approaches Precis. Med. 1026, 27–39. doi:10.1007/978-981-10-6020-5_2
Li, Y., Tang, X.-Q., Bai, Z., and Dai, X. (2016). Exploring the intrinsic differences among breast tumor subtypes defined using immunohistochemistry markers based on the decision tree. Sci. Rep. 6, 35773. doi:10.1038/srep35773
Lieu, E. L., Nguyen, T., Rhyne, S., and Kim, J. (2020). Amino acids in cancer. Exp. Mol. Med. 52, 15–30. doi:10.1038/s12276-020-0375-3
Liu, X., and Liu, Y. (2022). Comprehensive analysis of the expression and prognostic significance of the CENP family in breast cancer. Int. J. General Med. 15, 3471–3482. doi:10.2147/IJGM.S354200
Łukaszewicz-Zając, M., Pączek, S., and Mroczko, B. (2020). The significance of chemokine CXCL-8 in esophageal carcinoma. Archives Med. Sci. 13. doi:10.5114/aoms.2017.71933
Marei, H. E., Althani, A., Afifi, N., Hasan, A., Caceci, T., Pozzoli, G., et al. (2021). p53 signaling in cancer progression and therapy. Cancer Cell Int. 21, 703–715. doi:10.1186/s12935-021-02396-8
Médale-Giamarchi, C., Lajoie-Mazenc, I., Malissein, E., Meunier, E., Couderc, B., Bergé, Y., et al. (2013). RhoB modifies estrogen responses in breast cancer cells by influencing expression of the estrogen receptor. Breast Cancer Res. 15, R6–R13. doi:10.1186/bcr3377
Milczarek, M. (2020). The premature senescence in breast cancer treatment strategy. Cancers 12, 1815. doi:10.3390/cancers12071815
Munir, M. T., Kay, M. K., Kang, M. H., Rahman, M. M., Al-Harrasi, A., Choudhury, M., et al. (2021). Tumor-associated macrophages as multifaceted regulators of breast tumor growth. Int. J. Mol. Sci. 22, 6526. doi:10.3390/ijms22126526
Nisar, M., Paracha, R. Z., Arshad, I., Adil, S., Zeb, S., Hanif, R., et al. (2021). Integrated analysis of microarray and RNA-Seq data for the identification of hub genes and networks involved in the pancreatic cancer. Front. Genet. 12, 663787. doi:10.3389/fgene.2021.663787
Pertea, M., Kim, D., Pertea, G. M., Leek, J. T., and Salzberg, S. L. (2016). Transcript-level expression analysis of RNA-seq experiments with HISAT, StringTie and Ballgown. Nat. Protoc. 11, 1650–1667. doi:10.1038/nprot.2016.095
Rodgers, K. M., Udesky, J. O., Rudel, R. A., and Brody, J. G. (2018). Environmental chemicals and breast cancer: an updated review of epidemiological literature informed by biological mechanisms. Environ. Res. 160, 152–182. doi:10.1016/j.envres.2017.08.045
Roemhild, K., Von Maltzahn, F., Weiskirchen, R., Knüchel, R., Von Stillfried, S., and Lammers, T. (2021). Iron metabolism: pathophysiology and pharmacology. Trends Pharmacol. Sci. 42, 640–656. doi:10.1016/j.tips.2021.05.001
Rostovskaya, M., Andrews, S., Reik, W., and Rugg-Gunn, P. J. (2022). Amniogenesis occurs in two independent waves in primates. Cell Stem Cell 29, 744–759.e6. doi:10.1016/j.stem.2022.03.014
Sajnani, K., Islam, F., Smith, R. A., Gopalan, V., and Lam, A. K.-Y. (2017). Genetic alterations in Krebs cycle and its impact on cancer pathogenesis. Biochimie 135, 164–172. doi:10.1016/j.biochi.2017.02.008
Sawaki, K., Kanda, M., Umeda, S., Miwa, T., Tanaka, C., Kobayashi, D., et al. (2019). Level of melanotransferrin in tissue and sera serves as a prognostic marker of gastric cancer. Anticancer Res. 39, 6125–6133. doi:10.21873/anticanres.13820
Segovia-Mendoza, M., and Morales-Montor, J. (2019). Immune tumor microenvironment in breast cancer and the participation of estrogen and its receptors in cancer physiopathology. Front. Immunol. 10, 348. doi:10.3389/fimmu.2019.00348
Shuai, C., Yang, X., Pan, H., and Han, W. (2020). Estrogen receptor downregulates expression of PD-1/PD-L1 and infiltration of CD8+ T cells by inhibiting IL-17 signaling transduction in breast cancer. Front. Oncol. 10, 582863. doi:10.3389/fonc.2020.582863
Shumate, A., Wong, B., Pertea, G., and Pertea, M. (2022). Improved transcriptome assembly using a hybrid of long and short reads with StringTie. PLoS Comput. Biol. 18, e1009730. doi:10.1371/journal.pcbi.1009730
Sung, H., Ferlay, J., Siegel, R. L., Laversanne, M., Soerjomataram, I., Jemal, A., et al. (2021). Global cancer statistics 2020: GLOBOCAN estimates of incidence and mortality worldwide for 36 cancers in 185 countries. CA a cancer J. Clin. 71, 209–249. doi:10.3322/caac.21660
Thu, K., Soria-Bretones, I., Mak, T., and Cescon, D. (2018). Targeting the cell cycle in breast cancer: towards the next phase. Cell Cycle 17, 1871–1885. doi:10.1080/15384101.2018.1502567
Tung, K.-F., Pan, C.-Y., Chen, C.-H., and Lin, W.-C. (2020). Top-ranked expressed gene transcripts of human protein-coding genes investigated with GTEx dataset. Sci. Rep. 10, 16245. doi:10.1038/s41598-020-73081-5
Vitting-Seerup, K., and Sandelin, A. (2017). The landscape of isoform switches in human cancers. Mol. Cancer Res. 15, 1206–1220. doi:10.1158/1541-7786.MCR-16-0459
Wang, J., Xu, Y., Li, L., Wang, L., Yao, R., Sun, Q., et al. (2017). FOXC 1 is associated with estrogen receptor alpha and affects sensitivity of tamoxifen treatment in breast cancer. Cancer Med. 6, 275–287. doi:10.1002/cam4.990
Wang, Z., Jiang, Q., and Dong, C. (2020). Metabolic reprogramming in triple-negative breast cancer. Cancer Biol. Med. 17, 44–59. doi:10.20892/j.issn.2095-3941.2019.0210
Xie, Z., Bailey, A., Kuleshov, M. V., Clarke, D. J., Evangelista, J. E., Jenkins, S. L., et al. (2021). Gene set knowledge discovery with Enrichr. Curr. Protoc. 1, e90. doi:10.1002/cpz1.90
Xiong, X., Liao, X., Qiu, S., Xu, H., Zhang, S., Wang, S., et al. (2022). CXCL8 in tumor biology and its implications for clinical translation. Front. Mol. Biosci. 9, 723846. doi:10.3389/fmolb.2022.723846
Yang, X., Coulombe-Huntington, J., Kang, S., Sheynkman, G. M., Hao, T., Richardson, A., et al. (2016). Widespread expansion of protein interaction capabilities by alternative splicing. Cell 164, 805–817. doi:10.1016/j.cell.2016.01.029
Yeh, M.-H., Tzeng, Y.-J., Fu, T.-Y., You, J.-J., Chang, H.-T., Ger, L.-P., et al. (2018). Extracellular matrix–receptor interaction signaling genes associated with inferior breast cancer survival. Anticancer Res. 38, 4593–4605. doi:10.21873/anticanres.12764
Yin, L., Duan, J.-J., Bian, X.-W., and Yu, S.-C. (2020). Triple-negative breast cancer molecular subtyping and treatment progress. Breast Cancer Res. 22, 61–13. doi:10.1186/s13058-020-01296-5
Yip, H. Y. K., and Papa, A. (2021). Signaling pathways in cancer: therapeutic targets, combinatorial treatments, and new developments. Cells 10, 659. doi:10.3390/cells10030659
Yuan, Z., Chen, D., Chen, X., Yang, H., and Wei, Y. (2017). Overexpression of trefoil factor 3 (TFF3) contributes to the malignant progression in cervical cancer cells. Cancer Cell Int. 17, 7–13. doi:10.1186/s12935-016-0379-1
Zhang, E.-D., Li, C., Fang, Y., Li, N., Xiao, Z., Chen, C., et al. (2022). STMN1 as a novel prognostic biomarker in HCC correlating with immune infiltrates and methylation. World J. Surg. Oncol. 20, 301. doi:10.1186/s12957-022-02768-y
Zheng, C., and Yu, S. (2021). Expression and gene regulatory network of SNHG1 in hepatocellular carcinoma. BMC Med. Genomics 14, 28–10. doi:10.1186/s12920-021-00878-2
Keywords: estrogen receptor, breast cancer, isoform switching, differential expression, functional enrichment, cytokine, immunosuppression, CXCR chemokine receptor
Citation: Khan MS, Hanif W, Alsakhen N, Jabbar B, Shamkh IM, Alsaiari AA, Almehmadi M, Alghamdi S, Shakoori A, Al Farraj DA, Almutairi SM, Hussein Issa Mohammed Y, Abouzied AS, Rehman A-U and Huwaimel B (2023) Isoform switching leads to downregulation of cytokine producing genes in estrogen receptor positive breast cancer. Front. Genet. 14:1230998. doi: 10.3389/fgene.2023.1230998
Received: 29 May 2023; Accepted: 18 September 2023;
Published: 13 October 2023.
Edited by:
Lin Qi, Central South University, ChinaReviewed by:
Tian Tian, Children’s Hospital of Philadelphia, United StatesCopyright © 2023 Khan, Hanif, Alsakhen, Jabbar, Shamkh, Alsaiari, Almehmadi, Alghamdi, Shakoori, Al Farraj, Almutairi, Hussein Issa Mohammed, Abouzied, Rehman and Huwaimel. This is an open-access article distributed under the terms of the Creative Commons Attribution License (CC BY). The use, distribution or reproduction in other forums is permitted, provided the original author(s) and the copyright owner(s) are credited and that the original publication in this journal is cited, in accordance with accepted academic practice. No use, distribution or reproduction is permitted which does not comply with these terms.
*Correspondence: Mohammad Shahbaz Khan, bXNraGFuMkBjaGlsZHJlbnNuYXRpb25hbC5vcmc=
Disclaimer: All claims expressed in this article are solely those of the authors and do not necessarily represent those of their affiliated organizations, or those of the publisher, the editors and the reviewers. Any product that may be evaluated in this article or claim that may be made by its manufacturer is not guaranteed or endorsed by the publisher.
Research integrity at Frontiers
Learn more about the work of our research integrity team to safeguard the quality of each article we publish.